- 1Facultad de Industrias Alimentarias, Universidad Nacional Agraria La Molina, Lima, Peru
- 2Departamento de Química, Universidad Nacional de Colombia, Bogotá, Colombia
- 3Facultad de Economía y Planificación, Universidad Nacional Agraria La Molina, Lima, Peru
The present study aimed to extract polyphenolic compounds from lucuma (Pouteria lucuma) seeds using natural deep eutectic solvents (NADES) as a green, efficient, and environmentally friendly extraction. This was optimized by using the Response Surface Method (RSM) and comparing its predictive capacity with Artificial Neural Networks (ANN) and Convolutional Neural Networks (CNN). Four NADES were prepared by mixing lactic acid (LA) with each of the following reagents: sodium acetate (SA), urea (U), glucose (G), and ammonium acetate (AA), separately. The yield of total phenolic compounds (TPC) obtained from lucuma seeds with each NADES was measured as an optimization criterion with the Box-Benhken design. The following factors were evaluated: time, temperature, and the lucuma seed flour (LSF): NADES ratio. The response variables were TPC and antioxidant activity. The LA-AA extract was selected because it exhibited the highest TPC value and was analyzed by UHPLC–MS (Ultra-performance Liquid Chromatography-Mass Spectrometry). From the RSM, the optimal extraction parameters were 80 min, 52°C, and LSF: NADES ratio of 8:100 (w/v), obtaining a TPC value of 3601.51 ± 0.51 mg GAE/100 g LFS. UHPLC–MS analysis evidenced the formation of epigallocatechin isomers from epigallocatechin gallate. The predictive ability of ANNs compared to RSM was demonstrated.
1 Introduction
The lucuma tree (Pouteria lucuma) is a member of the Sapotaceae and Pouteria genus. It is native to the Andean region of Peru, Ecuador, and Chile (Gómez-Maqueo et al., 2020). The fruit of this plant is commonly referred to as the “gold of the Incas” (Fuentealba et al., 2016) or “golden fruit” due to its bright yellow color and unique flavor (Dini, 2011). The Inca civilization used it as a staple food in their diet (Inga et al., 2019). The lucuma fruit has a significant total polyphenol content (TPC), especially the Rosalia and Leiva varieties (45.3–61.6 mg GAE/g ms) (Fuentealba et al., 2016). Dini (2011), reported TPC values for lucuma flour of 51.1 mg GAE/kg, being higher than various fruits such as apples, blueberries, or strawberries (5.1, 14.3, and 34.7 mg GAE/kg, respectively) (Threlfall et al., 2007). The discovery and dissemination of its functional properties attributed to its bioactive compounds such as ascorbic acid, polyphenols, tocopherols, phytosterols and terpenoids that give it antioxidant capacity (Fuentealba et al., 2016; Campos et al., 2018; García-Ríos et al., 2020; Aguilar-Galvez et al., 2021; Inga et al., 2021). Due to its functional properties, the demand for lucuma has increased, leading to the generation of thousands of tons of seeds as agro-industrial waste (Guerrero-Castillo et al., 2019). According to the statistical reports of MIDAGRI of Peru (MIDAGRI, 2023), during the year 2022, the agricultural production of lucuma (Pouteria lucuma) was 12,933 tons, of which 1,682 tons of seed were generated as waste.
Recently, Inga et al. (2024) evaluated lucuma seeds as a potential raw material for use within the framework of the circular economy, finding high carbohydrates, protein, and crude fibre contents. They also studied its content of phenolic compounds, antioxidant properties and antimicrobial activity. The main phenolic compounds identified in lucuma seeds were gallocatechin, epigallocatechin, catechin, epicatechin, and their derivatives epigallocatechin gallate (EGCG) and epicatechin gallate. Also, myricetin 3-O-deoxyhexoside, taxifolin, and naringenin were identified. According to reports, EGCG and its derivatives have biofunctional properties that can exert an anticancer effect on various types of cancer cells in vivo, such as lung (Chen et al., 2020), colon (Sugihara et al., 2017), liver (Wen et al., 2019), and blood (Della Via et al., 2021), among others. In addition, they have good antioxidant and protective properties in the case of neurodegenerative diseases such as Alzheimer’s and Parkinson’s (Bakun et al., 2023).
For many years, extraction techniques for bioactive compounds from fruits and medicinal plants were carried out with organic solvents such as hexane, chloroform, acetone, methanol, or ethyl acetate (Poole and Poole, 2010); many of them are volatile and of petrochemical origin that is incompatible with the environment and with the development of an integrated green extraction process (Makris, 2018). Lately, environmental pollution has impacted the awareness of people and the scientific community, thus the so-called natural deep eutectic solvents (NADES) have emerged as environmentally friendly solvents, greener, cost-effective solvents with high capacity for the bioactive compound extraction in food wastes (Cui et al., 2018; Bertolo et al., 2023). NADES are innovative ecological solvents composed of a minimum of two components, one is a hydrogen bond donor (HBD), and the other is a hydrogen bond acceptor (HBA). The most common HBDs are organic acids or carbohydrates, while the most common HBAs are quaternary ammonium salts and amino acids (De Los Ángeles Fernández et al., 2018; Mišan et al., 2020). These compounds interact, creating a low-temperature transition mixture with a melting point of 100°C or less (Smith et al., 2014). In addition, they have interesting properties, such as low vapor pressure and the ability to dissolve various compounds. These characteristics, in addition to their easy and rapid preparation of deep eutectic solvents (DES) and NADES, make them suitable media for various processes, including extracting natural compounds (Jeong et al., 2017). When all components of DES are of natural origin, it is called NADES, which adheres to the 12 principles of Green Chemistry (Anastas and Eghbali, 2010).
In recent years, an overwhelming number of studies have been reported in the literature concerning the utilization of NADES. These studies have demonstrated the capacity of these solvents to efficiently extract bioactive compounds, such as flavonoids, phenolic acids, anthocyanins, and alkaloids, from various plant-derived materials, mainly from residues of the agrifood industry, such as peels, hulls, seeds, pomace, and leaves. The extraction ability of this solvent has been investigated, among others, in date seed (Airouyuwa et al., 2023), olive leave (Alrugaibah et al., 2023), cocoa bean shell (Rebollo-Hernanz et al., 2021; Benítez-Correa et al., 2023), pomegranate peels, almond hulls, and elderberry pomace (Bertolo et al., 2023), buckthorn leaves (Cui et al., 2018), hazelnut skin (Mazzeo et al., 2023), mango by-products (Pal and Jadeja, 2020; Ojeda et al., 2023), sour cherry pomace (Popovic et al., 2022) and avocado peels (Posta et al., 2023).
Mathematical models can improve extraction performance and reduce capital and time costs (Hu et al., 2023). For many years, the response surface methodology (RSM) has been used for optimization in various fields of research, including the extraction of bioactive compounds using deep eutectic solvents (Qin et al., 2023; Wang et al., 2023), being a valuable tool for process optimization when independent variables have nonlinear and interactive effects on the desired response (Dean et al., 2017). However, RSM has some drawbacks regarding the range of variables of the operational process (Witek-Krowiak et al., 2014). Recently, artificial intelligence, such as Artificial Neural Networks (ANN) and Convolutional Neural Networks (CNN), has gained considerable attention in prediction and optimization (Abiodun et al., 2018; Gu et al., 2018). ANN and CNN are robust computational and mathematical modelling techniques that attempt to imitate the biological neural networks of the human brain, giving satisfactory results in fitting the data due to their ability to learn from observations and create conclusions through generalization and modelling of the behavior of very complex nonlinear processes, even when the exact nature of the relationship between input parameters and process response variables is unknown, using a limited number of experimental measurements (Musa et al., 2016; Abiodun et al., 2018; Gu et al., 2018; Rebollo-Hernanz et al., 2021).
No research has been conducted on extracting bioactive compounds from lucuma seed using natural deep eutectic solvents. Additionally, no comparison study has been found that evaluates the predictive capacity of TPC and DPPH using RSM, RNA, and CNN methodologies to extract bioactive compounds from lucuma seed.
This study aimed to optimize the extraction process of TPC from lucuma seeds using natural deep eutectic solvents as the extraction solvent. The response surface methodology was used for this purpose. Furthermore, the study aimed to compare the efficiency of RSM, RNA and CNN modelling, and statistically evaluate their estimation capabilities.
2 Materials and methods
2.1 Vegetal material
Lucuma seeds (Pouteria lucuma) seda variety was obtained from the Topara farm in Chincha, Ica, Peru, from which the dark brown pericarp was removed, then they were cut with an approximate thickness of 0.5 cm. They were dried in a tray dryer with forced airflow at 60°C to a final humidity of 8%. Finally, it was pulverized in a hammer mill (Willey Mill, CYC) and sieved using a no. 80 sieve. The obtained lucuma seed flour (LSF) was stored at −18°C until use.
2.2 Chemicals and reagents
Sodium hydroxide (CAS N° 1,310-73-2, purity = 99.99%), Folin-Ciocalteau 2 N, 2,2-diphenyl-1-picrylhydrazyl (CAS N°1898-66-4), Trolox (CAS N° 135,806–59-6, purity ≥98.0%), methanol (CAS N°67–56-1, purity ≥99.8%), anhydrous sodium carbonate (CAS N° 497–19-8, purity = 99.999%) and gallic acid (CAS N° 5,995-86-8, purity ≥98.0%) (Sigma-Aldrich, United States), lactic acid (CAS N° 79–33-4, purity = 95%), sodium acetate anhydrous (CAS N° 127–09-3, purity ≥99.0%), urea (CAS N° 57–13-6, purity ≥99.0%), glucose anhydrous (CAS N° 50–99-7, ACS, purity = 99.0%), sucrose (CAS N° 57–50-1, ACS), ammonium acetate (CAS N° 631–61-8, purity = 99.05%), glycerol (CAS N° 56–81-5, purity ≥99.5%) (PanReac AppliChem, Spain), malic acid (CAS N° 6,915-15-7, purity = 98%) (Alfa Aesar, United States) and acetone (CAS N° 67–64-1, ACS, purity = 99.5%) (Fisher Chemical, United States) were used.
2.3 Experimental methodology
This research consisted of 3 stages. In the first stage, 7 NADES were tested, of which 3 were discarded (lactic acid-sucrose, citric acid-glycerol and malic acid-glucose). The best natural deep eutectic solvent (NADES) was prepared, characterized, and selected based on its ability to extract a higher total polyphenol (TPC) than an ultrasound-assisted extraction. In the second stage, with the NADES selected, the extraction process was optimized using the response surface methodology (RSM). In the third stage, the prediction capacity of the TPC and DPPH was compared using RSM, Artificial Neural Networks (ANN), and Convolutional Neural Networks (CNN).
2.4 Preparation and selection of NADES
NADES were synthesized using the thermal method (Skulcova et al., 2018; Savi et al., 2019). Briefly, the compounds HBD (lactic acid, citric acid, and malic acid) and HBA (sodium acetate, urea, glucose, sucrose, and glycerol) were weighed in a 3:1 molar ratio (Table 1), obtaining a binary mixture, then added 20% water (w/w) to this mixture. They were then subjected to continuous stirring (250 rpm) with heating (50°C) until a transparent and homogeneous liquid was formed. Initially, seven NADES were synthesized, of which only four were homogeneous, stable, translucent, low viscosity, and did not crystallize over time. The 4 NADES (Table 1) were prepared with lactic acid (LA) as HBD with sodium acetate (SA), urean (U), glucose (G), and ammonium acetate (AA) as HBA; it was prepared in a 3:1 ratio; based on previous studies, it has been shown that it significantly reduces viscosity, increasing diffusivity (Bi et al., 2013; Karakashov et al., 2015) and facilitating the extraction of metabolites (Bakirtzi et al., 2016).
2.5 Extraction methods of bioactive compounds
2.5.1 Extraction by heating-stirring with NADES
The extract of bioactive compounds with NADES (ENADES) was obtained according to the procedure described by Savi et al. (2019) and Skulcova et al. (2018) with slight modifications. 0.2 g of LSF was weighed, and the respective NADES was immediately added in a ratio of 2:100 (w/v). The mixture was heated (60°C) and stirred (250 rpm) for 1 h in closed amber glass bottles. It was then centrifuged at 6000 rpm for 10 min at 4°C (Eppendorf, 5804R). The recovered supernatant (ENADES) was stored at −18°C until further analysis.
2.5.2 Ultrasound-assisted solvent extraction
Extractions were performed with ultrasound according to Ojeda et al. (2023) with some modifications. Two solvents were used: 70% methanol (EUMe) and water (EUW). 1 g of LSF was weighed to do this, and the respective extraction solvent (1/10, w/v) was added. It was then subjected to an ultrasonic bath (WUC-D03H, DAIHAN-brand®) for 10 min and 20 kHz at 25°C. It was then centrifuged (6,000 rpm for 10 min at 4°C), and the supernatant was recovered and stored at −18°C until further analysis.
2.6 Total polyphenol content
The rapid Folin–Ciocalteu method adapted by Medina-Remón et al. (2009) determined the TPC. Briefly, in a 96-well microplate, 15 μL of the ENADES, EUMe, or EUW extracts previously diluted with water (1:2) was added, 170 μL of Milli-Q water, 12 μL of Folin–Ciocalteau 2 N were added to each extract and stirred carefully. Then, 30 μL of 20% sodium carbonate was added and shaken. It was stored in the dark at room temperature (~20°C) for 1 h. Subsequently, 73 μL of Milli-Q water was added, and the absorbance was measured at 765 nm in the microplate reader (BertholdTech TriStar2S). The results were expressed as milligrams of gallic acid equivalent per 100 g of LSF (mg GAE/100 g LSF, DW).
2.7 Antioxidant activity
The analysis protocol for determining DPPH in microplates proposed by Prieto (2012) was used with some modifications. 250 μL of diluted DPPH solution was added to a 96-well microplate, and the initial absorbance was measured at 515 nm in a microplate reader (BertholdTech TriStar2S). Then, 2 μL of each extract (ENADES, EUMe, or EUW) previously diluted in their respective extraction solvents (1:2) was added and stored in the dark at room temperature (~20°C) for 50 min. The absorbance was measured at 515 nm. The results were expressed as millimole of Trolox per gram of dry flour (mM Trolox/ g LSF, DW).
2.8 NADES characterization
2.8.1 Density
The density measurement of the NADES was conducted at a temperature of approximately 25°C. An analytical balance was used to weigh the mass of 1 mL of NADES, with a precision of ±0.005 g/cm3. The resulting density was expressed in g/cm3 (Popovic et al., 2022).
2.8.2 Viscosity
The viscosity (kinematic and dynamic) was determined using the calibrated Cannon Ubbelohde viscometer, suspended vertically in a thermostat (Model: 14 L-SS, Equiptron, India) with a temperature stability at 40°C. The kinematic viscosity (ν) of the NADES (m2/s) was obtained by multiplying the flow time “t” (seconds) by the viscometer constant “θ” provided by Cannon (Eq. 1). In contrast, the dynamic viscosity “η” (cP) was obtained by multiplying the kinematic viscosity (ν) by their respective density values (ρ) (g/cm3) (Eq. 2) (Jangir et al., 2021).
2.8.3 Electrical conductivity and pH
The electrical conductivity (mS/cm) of the NADES was measured at 25°C using a portable conductivity meter model 470 (Jenway, London, UK). Before measurement, the NADES were allowed to stand for 30 min at 25°C. The pH of this same sample was measured with a 370 potentiometer (Jenway, London, UK) (Popovic et al., 2022).
2.8.4 FT-IR spectrum
The IR spectra of the individual compounds (HBD and HBA) and the four NADES were obtained using a Nicolet iS 10 spectrometers (Perkin Elmer, France) equipped with an attenuated total reflectance (ATR) accessory, following the methodology proposed by Villanueva et al. (2023) with slight modifications. The homogenized solid samples (~ 0.1 g) were placed directly on the surface of the ATR crystal, and the spectrum was collected in the region of 4,000–500 cm−1 with a resolution of 4 cm−1 by pressing the powder onto the crystal using a pressure clamp. In the case of NADES and lactic acid, 200 μL was deposited directly on the glass surface. Before sample measurement, a background spectrum was recorded in the air. After each analysis, the ATR surface was cleaned with 95% acetone and dried with tissue paper. The entire process, which included cleaning the ATR crystal, collecting the background, and obtaining the IR spectrum of the sample, took less than a minute.
2.9 Response surface methodology
2.9.1 Screening
The screening was carried out using a 2IV(4–1) fractional factorial design, with two levels for each factor, where the minimum and maximum levels for each factor were: water content (10 and 30%), extraction time (80 and 100 min), LSF: NADES ratio (1:100 and 8:100 w/v), and temperature (40 and 60°C). The response variable was the TPC. Furthermore, the defining relationship was I = ABCD and the main effects were aliased with three-way interactions (Heck et al., 2021).
2.9.2 Optimization using RSM
Based on the analysis results, a Box–Behnken design was created with 3 center points and 3 repetitions for each treatment, making a total of 45 runs, for optimization using RSM (Bezerra et al., 2008), where the non-significant factor (water content) was kept constant. In this case, the minimum and maximum levels for each factor were time (80 to 100 min), LSF: NADES ratio (1:100 to 8:100, w/v), and temperature (40 to 60°C), and the response variable was the TPC.
2.10 Comparison of the prediction of response surface methodology (RSM), artificial neural networks (ANN) and convolutional neural networks (CNN)
In RSM, the Box–Behnken design was used to predict the value of the dependent variables studied (Giri and Mishra, 2022), according to Eq. 3.
Where: X1, X2, X3,…, βij (i = 1, 2 … k, and j = 1, 2,…,k) are the regression coefficients for the intercept, linear, quadratic, and interactions, respectively, and k is the number of variables.
The ANNs and CNNs were built with the data generated for the Box–Behnken design. Neural networks are a Machine Learning technology that is generally used in cases where the data has a non-linear behavior due to its great flexibility that is manifested by the different combinations that can be established with its components (nodes, layers, weights, activation function, propagation type, loss function, optimization algorithm, backpropagation, and regularization) (Erzin et al., 2008; Xi et al., 2013; Ciric et al., 2020; Rebollo-Hernanz et al., 2021; Shi et al., 2022; Alrugaibah et al., 2023). Regarding the data recorded in this research, the values of 80, 90, and 100 for the time variable, 1:100, 5:100, and 8:100 for the LSF: NADES ratio variable were used; and 40, 50 and 60 for the temperature variable (°C), to be combined and then obtain 45 values of the response variables (TPC and antioxidant activity-DPPH). These data were submitted to the NumPy, pandas, Keras, and Scikit-Learn (sklearn) libraries of the Python software, along with the functions available for constructing the ANN and CNN.
2.11 UHPLC-ESIMS/MS analyses
The composition of phenolic compounds in NADES-4 was analyzed using UHPLC-ESIMS/MS. A Bruker Impact II high-resolution mass spectrometer was employed for non-targeted analysis. Electrospray ionization (ESI) was used in both negative (−2.5 Kv) and positive (+3.2 Kv) modes, with the source temperature at 300°C for the positive mode and 250°C for the negative mode and a temperature of 400°C for desolvation gas in both modes. Sheath gas pressure was maintained at 29 psi. MS spectra were acquired within a mass range of m/z 130–1,500. Protonated [M + H]+ molecules were fragmented in a high-energy collisional dissociation (HCD) cell with different voltages maintained at 35 or 45 V. Nitrogen (N2) was used as a collision gas.
Liquid chromatography was performed using a Bruker Intensity Solo 2 C18 column (1.6 μm, 100 × 2.1 mm, Phenomenex, United States) operated at 40°C. The detection wavelengths were λ 254 and 280 nm. Mobile phases were 0.1% formic acid aqueous solution (A) and acetonitrile with 0.1% formic acid aqueous solution (B). The gradient program was: 5–95% B in 10 min. The flow rate was 0.25 mL/min, and the injection volume was 2 μL. The NADES were prepared as follows: 30 mg of each perfectly homogenized residue were weighed in a clean, dry vial, 5 mL of a MeOH-H2O mixture (8:2) was added to each vial, and the mixture was shaken in ultrasound equipment (150 W, 0.7 A) for 20 min. The resulting solutions were filtered using a hydrophilic PVDF filter (0.22 um x 25 mm, Millipore brand, United States) into an HPLC vial and then stored at −20°C until analysis by UHPLC-ESIMS/MS.
2.12 Statistical analysis
The results of the characterization and selection of the NADES were evaluated using a one-way analysis of variance. In addition, a multiple comparison test (Tukey) was carried out to assess the difference between their means (p < 0.05). The 2IV(4–1) fractional factorial design, the response surface methodology’s efficiency, and the regression coefficients’ statistical significance were evaluated with an analysis of variance. Principal Component Analysis (PCA) was used to observe how the physicochemical variables of NADES influence its behavior as an extraction solvent for total phenolic compounds. In addition, the heat map of the Pearson correlations of the variables under study was constructed. All these analyses were processed with the R software. On the other hand, the ANN and CNN were constructed with the Python software. The models were evaluated by analyzing the results of the target and output values using the coefficient of determination (R2) (Eq. 4), root mean square error (RMSE) (Eq. 5), and the mean absolute percentage error (MAPE) (Eq. 6) (Bingöl et al., 2012; Geyikçi et al., 2012).
Where n is the number of points, yi is the predicted value, ydi is the actual value, and ym is the average of the actual values.
3 Results and discussion
3.1 Characterization of natural deep eutectic solvents (NADES)
Initially, seven carboxylic acid-based natural deep eutectic solvents (CA-NADES) were synthesized. CA-NADES are known for their optimal extraction potential of bioactive compounds (Zannou et al., 2022; Airouyuwa et al., 2023). The NADES lactic acid (HBD)-sucrose (HBA), citric acid (HBD)-glycerol (HBA), and malic acid (HBD)-glucose (HBA) had high viscosity. The elevated viscosity of NADES reduces the diffusion rates of analytes, resulting in decreased mass transfer phenomena and prolonged extraction durations, thereby impacting extraction efficiency (Hikmawanti et al., 2021). Additionally, solvents with high viscosity are difficult to handle, transport from one place to another, stir, and filter. Therefore, this property plays an important role in the selection of an appropriate solvent (Negi et al., 2024). The viscosity of CA-NADES increased with the presence of the carboxylic acid functional group (–COOH), hydroxyl group (–OH), and the number of carbon atoms present in the structure of each carboxylic acid. Citric acid, possessing three carboxylic groups, and malic acid, with two, exhibited notably higher viscosity (Fuad et al., 2021; Airouyuwa et al., 2023). Lactic acid has a lower viscosity due to its weaker hydrogen bond interaction caused by the smaller molecular size (Fuad et al., 2021). In addition, the physical state of the carboxylic acid also contributes to the viscosity of the NADES (Airouyuwa et al., 2022). Citric acid and malic acid are solids at room temperature, while lactic acid is liquid; hence, the higher viscosity observed in citric and malic acid (Airouyuwa et al., 2023). This problem is often solved by adding water to reduce the viscosity (Dai et al., 2015), in this study the addition of 20% of water was not enough. However, an excessive amount of water would disrupt the intermolecular interactions (breakage of hydrogen bonds in the system) between the HBA and HBD, hence affecting their eutectic properties, which could also result in poor extraction efficiency of the NADES (Dai et al., 2015; Airouyuwa et al., 2023). For the reasons stated, these three NADES were discarded. It has been documented that sucrose, among the sugar-derived hydrogen bond donors (HBDs), displayed the highest viscosity, potentially owing to its nature as a disaccharide with a higher number of hydrogen bonds compared to glucose, which is a monosaccharide (Airouyuwa et al., 2022).
At present, only very few studies have dealt with the use of lactic acid-based deep eutectic solvents for the extraction of bioactive compounds (Bakirtzi et al., 2016; Macchioni et al., 2021; Koraqi et al., 2024). Lactic acid is a naturally occurring organic acid recognized as Generally Recognized as Safe (GRAS). Its chemical and physical attributes confer remarkable reaction versatility, characterized by its acidic nature in aqueous solutions and its bifunctional reactivity due to the presence of both carboxyl and hydroxyl functional groups (Martinez et al., 2013; Macchioni et al., 2021). On the other hand, choline chloride is frequently used as the hydrogen bond acceptor (HBA) in the formulation of natural deep eutectic because it has a good extraction performance. Nevertheless, choline chloride is comparatively costly when juxtaposed with other untapped natural alternatives, which remain underutilized (Macchioni et al., 2021). Also, considering the possible application of the extract as an active agent, the use of choline chloride is limited. For example, choline esters and salts are prohibited in cosmetic formulations (Alchera et al., 2024). For these reasons, it may be interesting to study NADES based on lactic acid, salts, and sugars.
The physicochemical properties of NADES (Table 2) are essential to establish their potential applications. Therefore, they directly influence the ability to extract compounds from different food matrices. The most studied properties are density, viscosity, electrical conductivity, pH, and surface tension (Omar and Sadeghi, 2022). Density and viscosity significantly influence NADES because they affect the mass flow of the analyte, with low viscosity values being ideal (Mitar et al., 2019; Imteyaz and Ingole, 2023). pH is essential for the solubility of bioactive compounds due to the strong interaction of hydrogen bonds between the analyte molecules and NADES (Mitar et al., 2019). Surface tension is an important physical property that reveals the impact of molecular structure on the interactions between HBD and HBA in NADES (Omar and Sadeghi, 2022). Furthermore, the structure of NADES greatly influences its physical properties, which could be successfully modified and tuned by adding water.
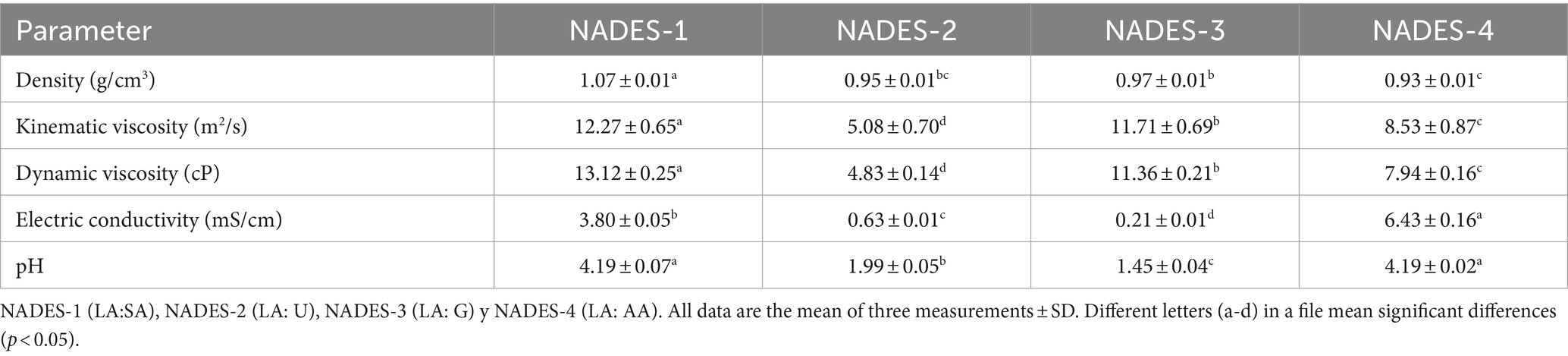
Table 2. Density (g/cm3), kinematic viscosity (m2/s), dynamic viscosity (cP), electrical conductivity (mS/cm), and pH of the NADES.
The density of the investigated NADES was in the range of 0.93 to 1.07 g/cm3. This variation in density is attributed to the molecular weight of the different components used to prepare the NADES (Fuad et al., 2021). Also, NADES’s densities depend on the molecular organization and packing, temperature, and HBA: HBD molar ratio. It is also affected by holes and vacancies within the liquid NADES’s (Saini et al., 2022). The eutectic mixture containing high molecular weight compounds such as NADES-1 (lactic acid: 90.08 g/mol and sodium acetate: 82.0343 g/mol) and NADES-3 (lactic acid: 90.08 g/mol and glucose: 180.156 g/mol) have a higher density than NADES formed by low molecular weight compounds such as NADES-2 (lactic acid: 90.08 g/mol and urea: 60.06 g/mol) and NADES-4 (lactic acid: 90.08 g/mol and ammonium acetate: 77.0825 g/mol). One of the primary hurdles in implementing NADESs is their elevated viscosity relative to conventional solvents, potentially causing limitations in mass transfer (Savi et al., 2019). The elevated viscosity observed in certain NADESs is ascribed to robust intermolecular interactions. These interactions primarily stem from the hydrogen bond network within the eutectic blend (Fuad et al., 2021), supplemented by weaker van der Waals and electrostatic forces (Saini et al., 2022). These interactions restrict molecular mobility within the mixture, thereby elevating viscosity. Studies have shown that as temperature and water content increase in NADES samples, the internal resistance of molecules diminishes, leading to a subsequent decline in viscosity (Chemat et al., 2016; Yang, 2019).
In the same way, the viscosity of the NADES system is influenced by its constituent molecular weights. Generally, NADES derived from lactic acid tend to be less viscous than NADES derived from high molecular weight compounds such as malic acid, sucrose, or glucose (Fuad et al., 2021). Viscosity has a strong relationship with conductivity; NADES with high viscosity have poor conductivity at room temperature. However, it is also affected by the increase in water, the molar ratio, and the nature of HBA and HBD (Omar and Sadeghi, 2022).
On the other hand, pH is an important characteristic that must be considered in developing NADES because it plays a crucial role in plant phenolic compounds’ extractability and subsequent stability. The extraction of polyphenols is generally performed at low pH because these compounds adopt the neutral form in acidic environments, which is the most suitable to be solubilized. However, excessive acidification could impair extraction since the profile of native polyphenols may be distorted due to the hydrolysis of simple (acyl)glycosides (Tsao, 2010). Moreover, pH is also essential in the release of non-extractable polyphenols, which, in their native form, remain attached to structural elements of the matrix (Gil-Martín et al., 2022). Also, research indicates that phenolic compounds, mainly phenolic acids, tend to exhibit greater stability under acidic conditions, while their degradation rate significantly rises under alkaline conditions (Friedman and Jürgens, 2000). In general, for a nonaqueous solvent, the pH depends on the chemical potential of hydrogen, which is affected by the presence of cations and anions. Molecular interaction, such as the hydrogen bonding of these ions with other species in the solvent, determines pH values (Fuad et al., 2021). The pH value of NADES-2 and NADES-3 was 1.99 and 1.45, respectively, indicating a very acidic level (pH range from 0 to 3.0), while for NADES-1 and NADES-4 it was 4.19, potentially be classified as slightly acidic (pH range 4.0 to 4.5) (Skulcova et al., 2018). The acidity and basicity of the hydrogen bond donor (HBD) and hydrogen bond acceptor (HBA) are known to govern the pH of the eutectic system (Omar and Sadeghi, 2022). Popovic et al. (2022) reported a pH range of 2.0 to 5.9 for NADES systems prepared based on choline chloride/malic acid and choline chloride/urea, respectively. On the other hand, Omar and Sadeghi (2022) mention that there are very acidic NADES close to 0 pH (choline chloride/citric acid) and basic NADES such as choline chloride/urea. In our work, the use of lactic acid had a direct influence on the pH of the NADES systems. In addition, the pH is also influenced by adding water (Fuad et al., 2021), mainly in NADES made from an organic acid, where the pH tends to increase (Mitar et al., 2019).
In the Principal Component Analysis (PCA), it is observed that the first two principal components describe 92.1% of the total variance of the data (Dim 1 = 53.0% and Dim 2 = 39.1%) (Figure 1A). The NADES-4 is the one that has the most significant relationship with the TPC variable, unlike the other NADES. NADES-1 is more related to dynamic viscosity, kinematic viscosity, and density. Contrary to the NADES-2 and NADES-3, which have little relationship with the variables studied. On the other hand, Figure 1B shows that TPC and density present a high negative correlation (r = −0.9); that is, the higher the NADES density, the lower TPC values will be obtained; similar behavior was shown by the dynamic viscosity (r = −0.7) and kinematic viscosity (−0.6). On the other hand, TPC shows a positive correlation with pH (r = 0.6) and electrical conductivity (r = 0.8). A higher TPC content will be obtained for the highest pH and electrical conductivity values studied.
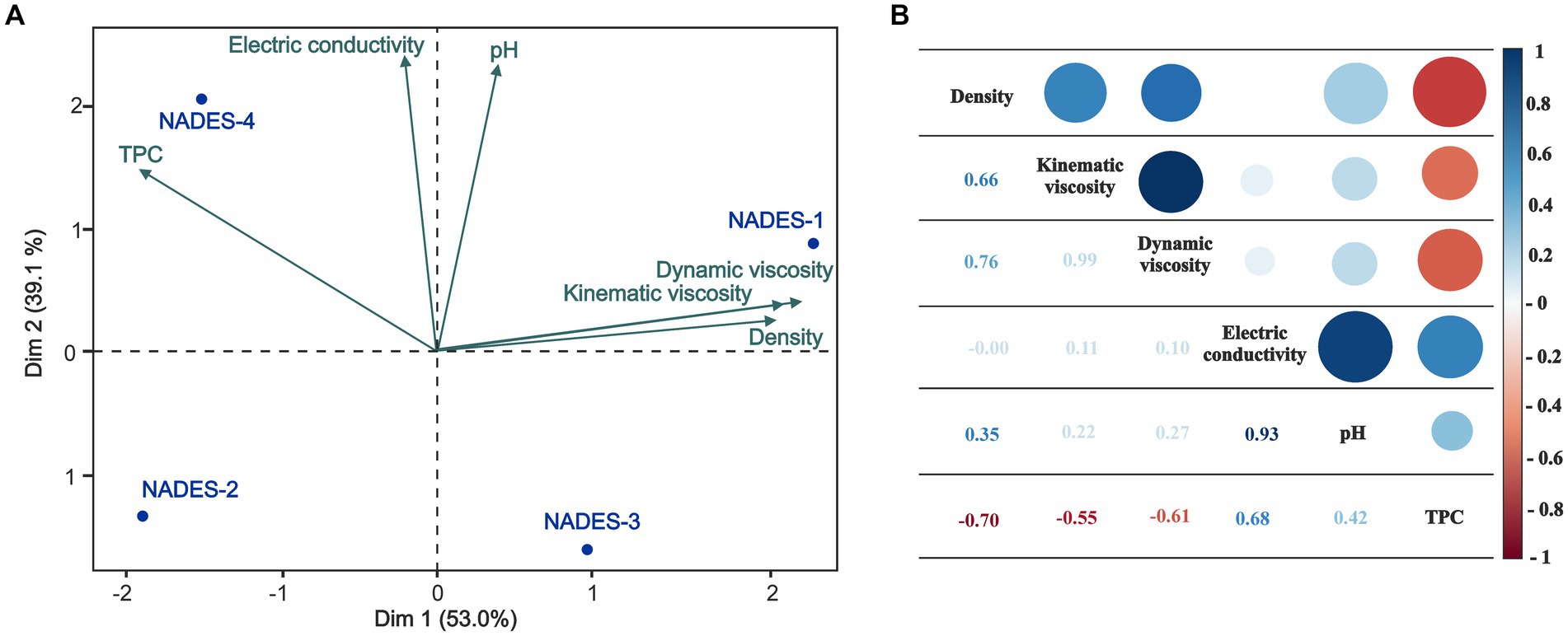
Figure 1. Influence of NADES physicochemical variables on TPC extraction. (A) Biplot of the first two principal components of the PCA. (B) Correlation between the TPC, density, kinematic viscosity, dynamic viscosity, electric conductivity, and pH.
3.2 Spectrometry (FT-IR)
IR of the NADES was performed to obtain information on the types of chemical bonds and functional groups of the prepared HBD, HBA, and the 4 NADES (Figure 2). The objective was to identify the intermolecular interactions between the functional groups of HBA and HBD. In the NADES-2 (LA: U) spectrum, the vibrational bands at 3320 cm−1 refer to the symmetric stretching of NH2 (Hayyan et al., 2015). Furthermore, the bands 1,660 cm−1, 1,609 cm−1, and 784 cm−1 are characteristic functional groups of urea, namely, C=O stretching for amide, N-H scissors band, and N-H bending band, respectively (Hayyan et al., 2015; Delgado-Mellado et al., 2018). Also, the representative peak of the carboxylic group of lactic acid (1,720 cm−1) can be observed in the 4 NADES (Macchioni et al., 2021). The peaks 1,750 and 1,690 cm−1 generally correspond to the C=O functional group of carboxylic acids. The broader peak between wave numbers 3,000 and 3,500 cm−1 indicates hydrogen bonding between the hydrogen of the carboxylic acid functional group and the nitrogen of the amino group of urea and ammonium acetate (Airouyuwa et al., 2023). Changes were also observed in the fingerprint region (Ren et al., 2018).
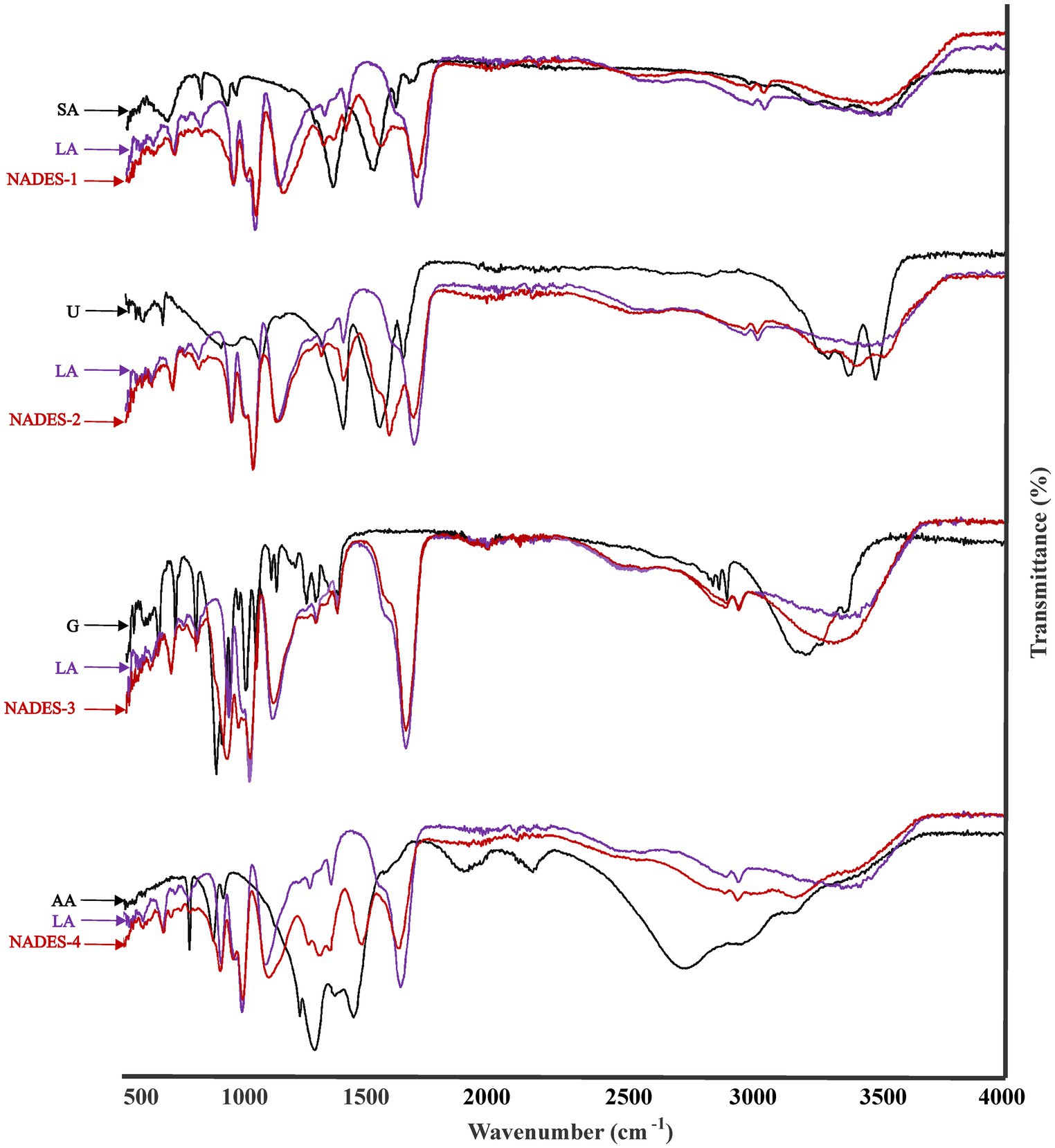
Figure 2. IR spectrometry of the HBD, HBA, and NADES under study. SA, sodium acetate; LA, lactic acid, NADES-1 (LA: SA), U: urea, NADES-2 (LA: U), G: glucose, NADES-3 (LA: G), AA: acetate ammonium and NADES-4 (LA: AA).
3.3 Comparison with conventional method and selection of NADES
Preliminarily, it is observed that the four NADES used in this study obtained different extraction capacities (Figure 3). It is known that the extraction capacity of NADES varies according to its polarity, which depends, among other factors, on the various combinations of HBD and HBA (Wang et al., 2024). These combinations generate different interactions between the target compound and NADES (Tang et al., 2021), directly affecting its solubility and extraction capacity (Zheng et al., 2022). It is also observed that they obtained higher TPC than ultrasound-assisted extractions (EUMe and EUW). Similar results were obtained by Wang et al. (2024), who studied nine NADES, of which the one that obtained the best extraction efficiency was the NADES formed by lactic acid and L-proline, compared to ultrasound-assisted extractions with 70% ethanol and water. This observation could be attributed to hydrogen bonds facilitating cell wall penetration, promoting molecular interactions between NADES and plant cellulose chains (Liu et al., 2022). Additionally, NADES has the capability to establish hydrogen bonds with phenolic compounds, which enhances their solubility (Dai et al., 2013a,b). Given the nature of polyphenols, which are considered hydrogen bond donors (HBD) (Bakirtzi et al., 2016), their interaction with acetate anions could generate strong bonds, resulting in a more stable supramolecular structure during the process of extraction, increasing the efficiency of the process (Bakirtzi et al., 2016; Mazzeo et al., 2023).
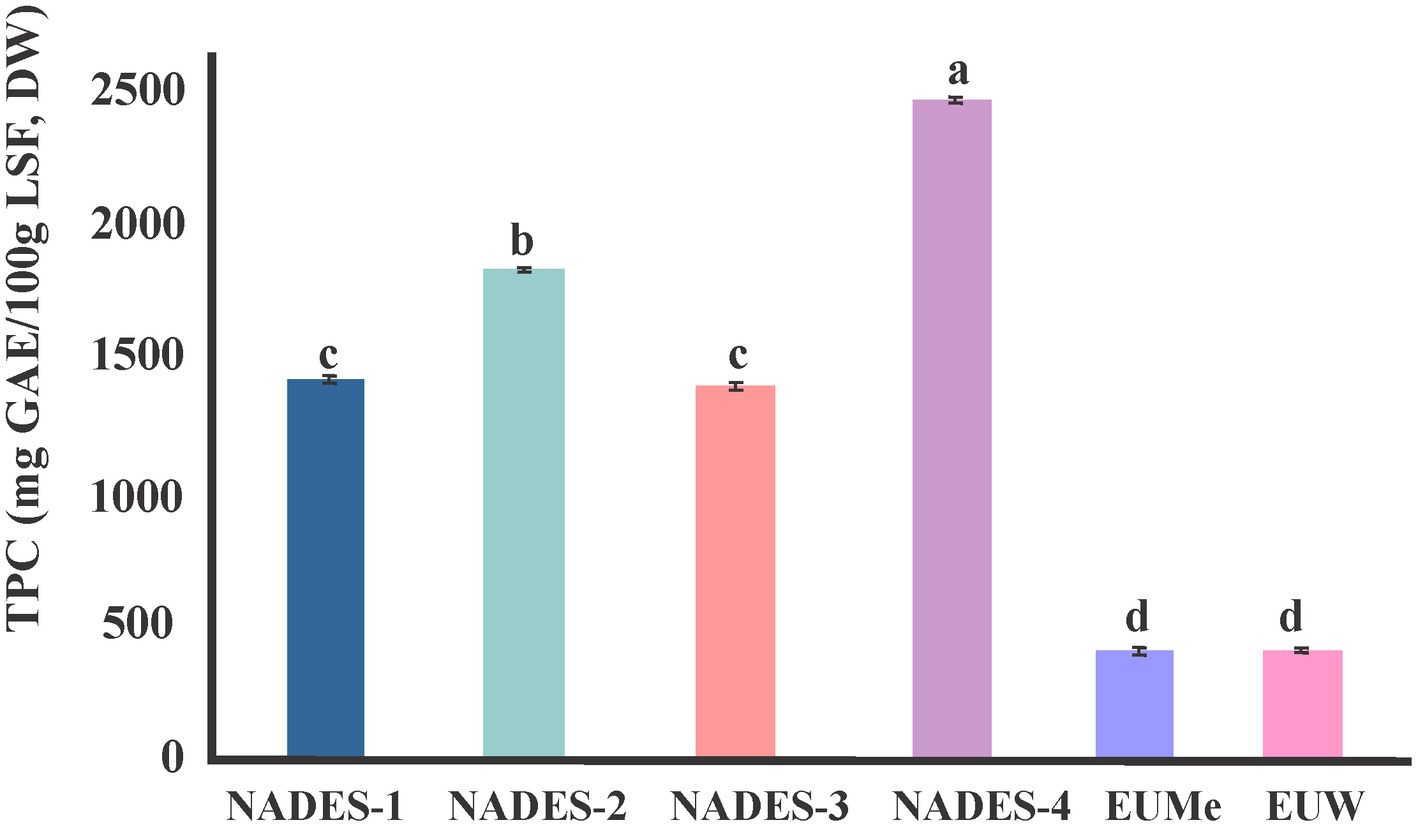
Figure 3. Total polyphenol content (mg GAE/100 g LSF, DW). NADES-1 (LA: SA), NADES-2 (LA: U), NADES-3 (LA: G), NADES-4 (LA: AA), EUMe: Ultrasound with 70% methanol, and EUW: Ultrasound with water.
The NADES-4 consisting of lactic acid and ammonium acetate (LA: AA) obtained the best TPC result (2,434.49 ± 0.18 mg GAE/100 g LSF, DW) of all the NADES studied. Therefore, it was chosen as the desired NADES for the subsequent optimization of the TPC of lucuma seed flour.
3.4 Response surface methodology
3.4.1 Screening
The analysis of variance of the fractional factorial design 2IV(4–1) (Table 3) shows that the significant factors (p-value <0.05) were the extraction time (min), extraction temperature (°C) and the relationship LSF: NADES (w/v). While it is true that adding more water to the NADES preparation can reduce its viscosity, increase its polarity, and decrease surface tension, leading to improved extraction efficiency, the research results indicate that these effects were not significant (p-value >0.05). This was demonstrated even though previous studies have shown that increasing the water content in the NADES preparation does have a positive impact on its extraction performance (Bi et al., 2013; Karakashov et al., 2015; Bakirtzi et al., 2016; Peng et al., 2016). Similar results were obtained by Koutsoukos et al. (2019) by evaluating the water content in a range of 25 to 30% (w/w) in the NADES formed by choline chloride and tartaric acid.
The formation of hydrogen bonds drives the production of NADES. The HBD and HBA components used in this study are water-soluble and hygroscopic. Due to the interactions between water molecules and NADES components, the hydrogen bond network may be altered, leading to changes in the system’s viscosity, density, and electrical conductivity (Ninayan et al., 2023). In this research, it was necessary to incorporate 20% of water (w/w) to reduce the viscosity of the NADES, thus facilitating the diffusion of polyphenols, as has been observed in previous studies (Karakashov et al., 2015; Bakirtzi et al., 2016). However, it is essential to regulate the water content since this variable can influence the chemical reactivity of NADES. Generally, a concentration higher than 50% can break the hydrogen bond between HBD and HBA (Dai et al., 2015; Moradi et al., 2023) and decrease the interactions between NADES and polyphenols, affecting the performance of extraction (Peng et al., 2016). It is known that the presence of water can complicate specific reactions by competing for hydrogen bonding sites or affecting the local concentration of reactants (Ninayan et al., 2023). Additionally, DES formed by hydrophilic HBA or HBD components could leach into water due to their solubility (Florindo et al., 2017, 2019). However, in the case of DES based on ammonium salts, only HBA is the main factor of stability in water (Tang et al., 2018).
3.4.2 Optimization
The significant factors from the screening stage were used to optimize the TPC extraction using the Box–Behnken design. In this study, the values of the regression coefficient, R2(0.803) and R2adj (0.752), were very similar, showing that even after eliminating some interaction terms, the quality of the model was maintained (R2 > R2adj) (Pal and Jadeja, 2020).
Time (p < 0.05), LSF: NADES ratio (p < 0.01), and temperature (p < 0.05) had significant effects on TPC. The quadratic effect of time and temperature was significant, unlike the effect of the LSF: NADES ratio. Furthermore, no interaction effect was significant (p > 0.05). The experimental data of the RSM model can be expressed by the following equation (coded variables): .
The LSF: NADES ratio was significant in the range of 7:100 to 9:100 (w/v), and the temperature was between 45 to 52°C (Figure 4A), obtaining the highest TPC values (> 3,500 mg GAE/100 g LSF, DW). For a time between 85 and 91 min and a LSF: NADES ratio between 7.6:100 to 8:100 (w/v), the highest TPC is obtained (> 3,600 mg GAE/100 g LSF, DW) (Figure 4B), while for a time between 81 and 94 min and a temperature between 44 and 54°C the highest TPC is obtained (> 3,200 mg GAE/100 g LSF, DW) (Figure 4C). To validate the results obtained from RSM, we performed experiments in triplicate under optimized conditions. The TPC value obtained was 3,601.51 ± 0.51 mg GAE/100 g LSF, DW. Our results show that the TPC value was higher than other agroindustrial waste such as mango peel (2,305.0 mg GAE/100 g, DW) (Ojeda et al., 2023), cocoa pod (1,500 mg GAE/100 g, DW) (Benítez-Correa et al., 2023) and cherry pomace (323.83 mg GAE/100 g, DW) (Popovic et al., 2022), but lower than avocado peel (8,290 mg GAE/100 g, DW) (Posta et al., 2023) and mango seed (6,001.0 mg GAE/100 g, DW) (Ojeda et al., 2023), where different deep eutectic solvents were used.
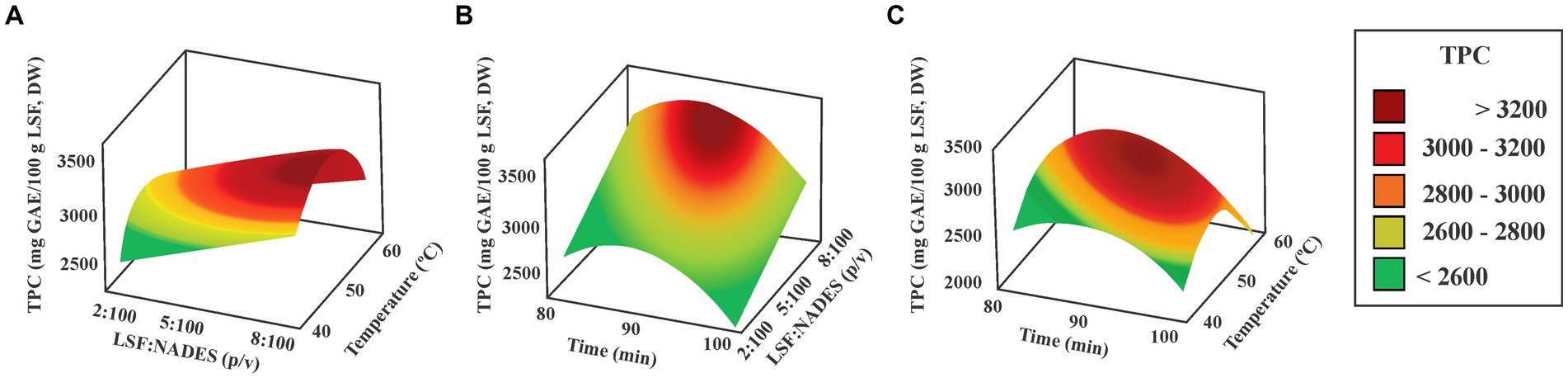
Figure 4. Effect of time (A), temperature (B), and LSF: NADES ratio (C) on the total polyphenol content (TPC) (mg GAE/100 g LSF, DW).
Notably, the experimental data for DPPH did not show an appropriate fit for the RSM model. This suggests that the RSM model’s limitations may be why it failed to represent the DPPH value accurately. The model only considers first-order, second-order, and interaction terms, whereas the DPPH value may not necessarily have these relationships. A better alternative may be to use models such as neural networks, which can handle non-linear relationships even when the nature of the relationship is unknown (Musa et al., 2016). However, Pavlić et al. (2019) found a poor fit for the case of DPPH (R2 = 0.6646) evaluating the effect of extraction time, solvent concentration, and solid–liquid ratio using artificial neural networks.
Temperature influences various fundamental physicochemical parameters for the extraction of bioactive compounds, highlighting mainly viscosity, surface tension, and electrical conductivity. The predominant factors that affect the viscosity of NADES are hydrogen bonding forces, the Van der Waals force, and electrostatic forces. As the temperature increases, the viscosity of NADES decreases due to the gradual weakening of Van der Waals forces and hydrogen bonds. Furthermore, low viscosity improves mass transfer between a solute and a solvent (Zhang et al., 2020).
In NADES systems, the surface tension is caused by strong contact between the HBA and HBD molecules (Boateng, 2022). In most DES, the surface tension decreases as the temperature increases, and this relationship is linear (Shahbaz et al., 2012; Francisco et al., 2013; Ghaedi et al., 2017). Generally, the surface tension is stable between 20 and 30°C up to a mole fraction of water of 0.8. This information is beneficial because a liquid with higher surface tension is known to have a higher extraction efficiency, as explained by Boateng (2022). However, as the temperature increases, there is enough energy to interrupt the cohesive forces, decreasing surface tension and affecting the extraction performance of bioactive compounds (Chen et al., 2019). Additionally, although the increase in temperature indeed generates a greater extraction yield of bioactive compounds due to the increase in the solubility of the lucuma seed flour in the NADES and the decrease in the viscosity of the NADES, improving the diffusivity (Bi et al., 2013; Francisco et al., 2013; Dai et al., 2013a,b; Karakashov et al., 2015), when high temperatures occur, bioactive compounds can degrade (Peng et al., 2016). On the other hand, increasing temperature facilitates molecular heating and increases kinetic energy that accelerates supramolecular movement, leading to the formation of large supramolecular molecules, which, in the process, can decompose into smaller supramolecular molecules, increasing the probability of collisions between molecules, weakening the interactions between molecules and increasing the electrical conductivity of the system; This phenomenon could favor the dissolution of bioactive compounds (Zhang et al., 2020).
Because prolonging extraction times is inadequate from an operational and economic point of view, evaluating this factor is essential in the extraction process of bioactive compounds. Time control can positively affect the extraction performance, which consists of 2 stages: first, the soluble components are solubilized from the surface of the solid matrix, followed by the mass transfer of soluble components from the plant material to the solution phase through diffusion or osmotic processes. This mass transfer process is the slowest and determines the extraction rate (Veličković et al., 2006). Furthermore, acidic NADES have a more significant dissolving effect on the cellulose of the cell wall of the plant material (Zhang et al., 2020), so a longer extraction time would cause greater diffusion of the bioactive compounds and, in parallel, the prolonged contact time of NADES with the cell wall would cause its dissolution, improving the extraction efficiency of bioactive compounds. Our study shows that the TPC increased as time and the LSF: NADES ratio increased (Figure 4B). However, exposure to prolonged time and high temperatures caused a decrease in TPC (Figure 4C) due to possible structural damage caused by prolonged thermal exposure, which could result in the decomposition of thermosensitive bioactive compounds (Oliveira et al., 2016; Peng et al., 2016).
The TPC based on the LSF: NADES ratio had a linear relationship (Figures 4A,B) because by increasing the proportion of solids in suspension, the driving force increases, improving the mass transfer rate and causing the solute to dissolve more easily in NADES (Lanjekar et al., 2022; Hong et al., 2023). It is known that when the solvent content is lower than that of solids, complete transfer cannot be achieved (Bener et al., 2022), and increasing the liquid–solid ratio improves the contact area between the solute and the solvent, which reduces the density of the mixture thus improving the dissolution rate (Dzah et al., 2020).
3.5 Comparison of predictive models
One crucial step in building the ANN and CNN is determining the best hyperparameter values for the network components. This is done by testing different combinations of values for the number of layers, activation function, number of nodes, regularization constant, and optimization algorithm. The optimal combination of the ANN was the following: training set 80%, cross-validation equal to 5, activation function “Rectified Linear Unit (relu),” alpha regularization constant equal to 0.0001, two layers each with twenty nodes, and Broyden-Fletcher-Goldfarb-Shanno (BFGS) optimization algorithm (Saputro and Widyaningsih, 2017). By correlating the experimental data with those predicted by RSM (Figures 5A,D), ANN (Figures 5B,E), and CNN (Figures 5C,F) for both the TPC and the antioxidant activity by DPPH, respectively, it was observed that the CNN obtained R2 values very close to 1 for DPPH in comparison to RSM and ANN, indicating a better fit of the experimental results due to the capability of processing data with several non-linear functions (Teslić et al., 2019). For TPC, both RSM and ANN showed similar R2 values; nevertheless, ANN recorded lower RMSE and MAPE values, indicating better accuracy. Several authors (Teslić et al., 2019; Yang et al., 2019; Khan et al., 2023) obtained similar results; however, Jha and Sit (2021) demonstrated results contrary to the present research.
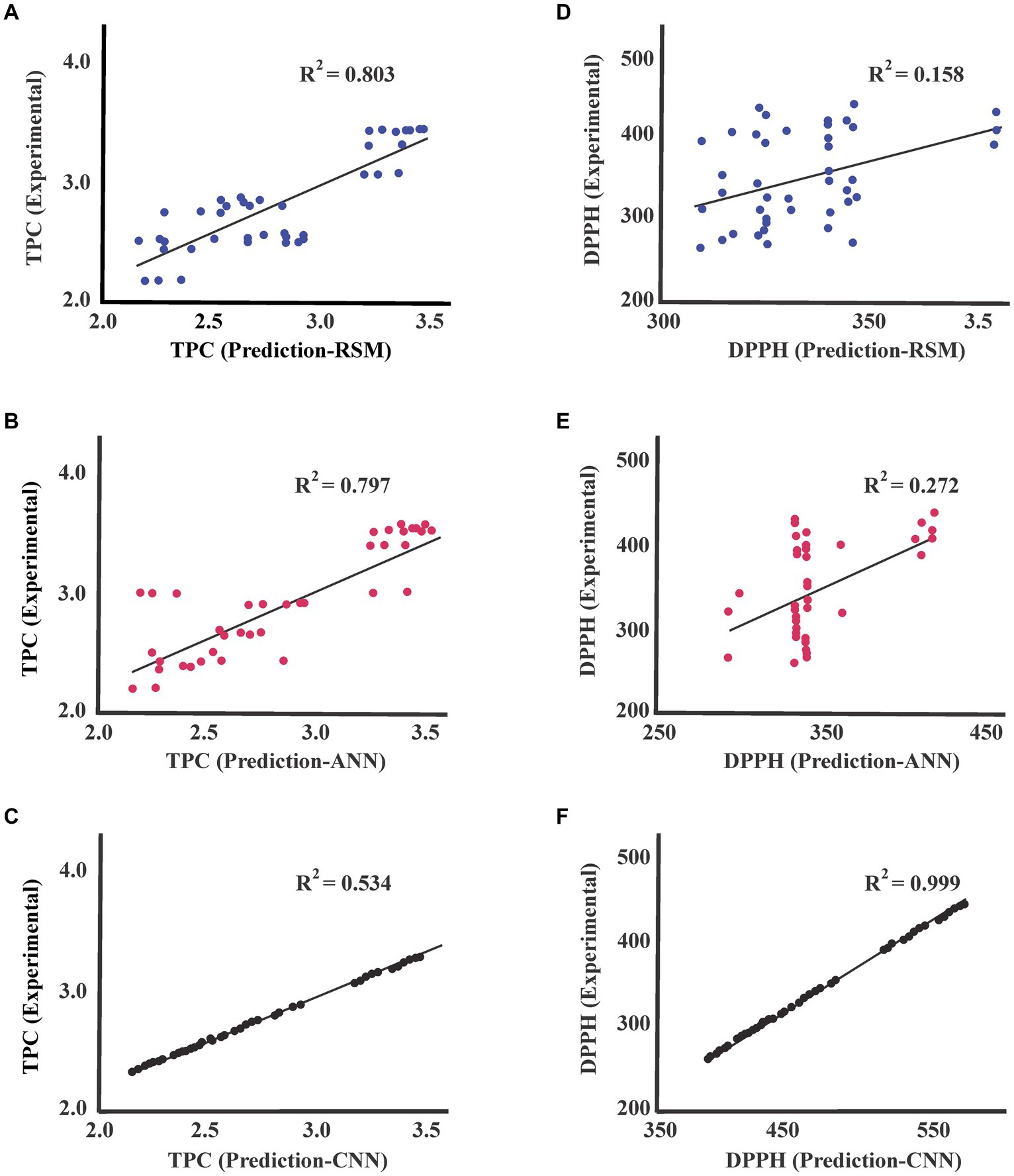
Figure 5. Scatterplot between experimental data and RSM, ANN, and CNN predictions for TPC (A–C) and DPPH (D–F).
Overall, all three models have their advantages and uniqueness, which comprise good non-linear prediction capabilities of ANN and CNN models, while RSM interprets good interaction between the variables (Khan et al., 2023). The performance of the constructed RSM, ANN, and CNN models was statistically measured using RMSE and MAPE (Table 4). Interestingly, CNN is better for DPPH data, while ANN is better for TPC data. However, both neural networks were superior to RSM in data fitting and TPC and antioxidant activity (DPPH) estimation capabilities.

Table 4. Statistical evaluation for response surface models (RSM), Artificial Neural Networks (ANN), and Convolutional Neural Networks (CNN) in the prediction of antioxidant activity (DPPH) and Total Polyphenol Content (TPC).
3.6 UHPLC-ESIMS/MS analyses of NADES-4
Based on the data from Figure 3, NADES-4 extract was selected for further polyphenol analyses. It was clear that the TPC was the highest among NADES extracts and 5-fold higher than the extract obtained by using methanol 70% with ultrasound. The total ion chromatogram obtained in positive mode is shown in Figure 6.
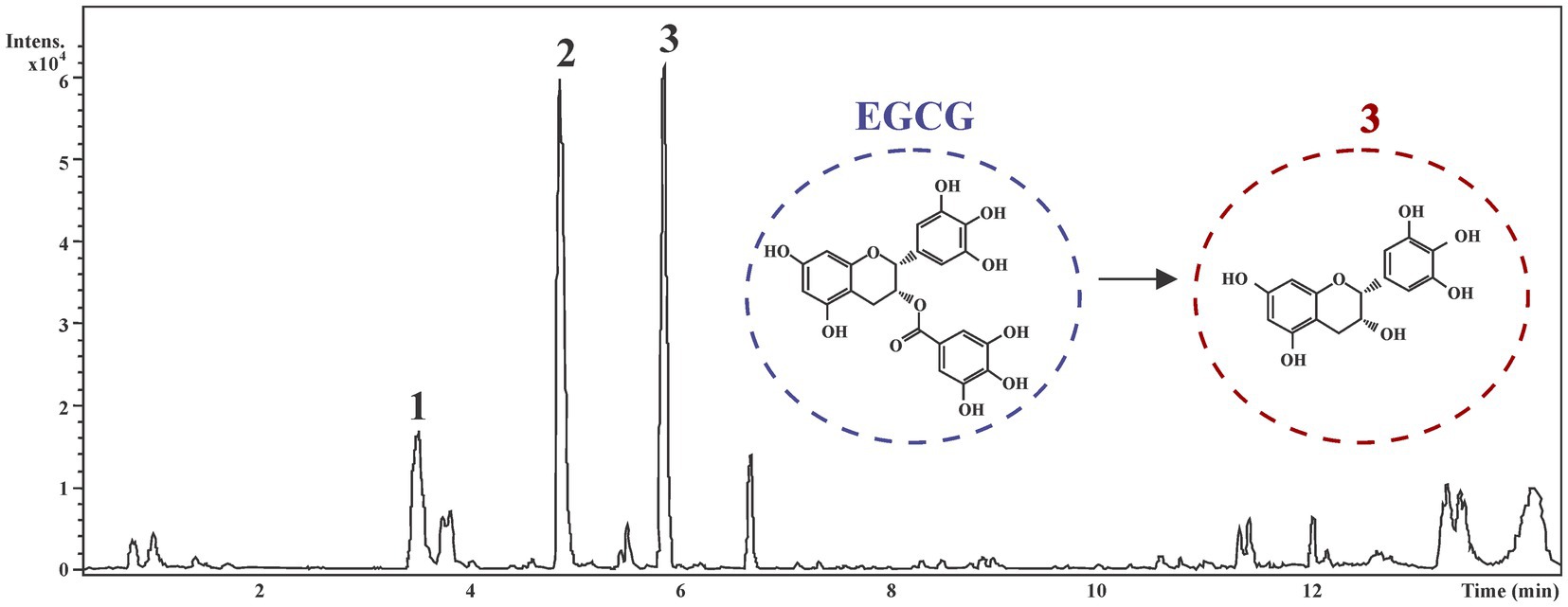
Figure 6. Total ion chromatogram obtained by UHPLC-ESIMS/MS (positive mode) of NADES-4. Suggested hydrolysis from EGCG to epigallocatechin (3) is shown.
Three compounds (1, 2, and 3) were detected as significant constituents. Compound 2 was tentatively identified as gallocatechin hexose because the ion fragment at m/z 467.1020 in negative mode (Guerrero-Castillo et al., 2019) and, the ion at m/z 491.1373 in positive mode corresponding to the adduct with sodium [M + Na]+. Compounds 1 and 3 exhibited ions at m/z 307.0618 u and 305.0614 u, in positive and negative modes, corresponding to the pseudomolecular ions [M + H]+ and [M-H]−, respectively. The presence of other fragment ions in negative mode, m/z 233.0460 and 161.0311, allowed to conclude that the chemical structure of two isomers were gallocatechin (GC) or epigallocatechin (EGC) (MW = 306 u). In contrast to the results of the methanolic extract of lucuma seeds (Inga et al., 2024), the epigallocatechin gallate (MW = 458) was not found. This suggests that the thermal effect during the extraction of lucuma seeds with NADES-4 favored the hydrolysis of EGCG to the corresponding gallocatechin isomers, thereby increasing the TPC. This suggests that the thermal effect during the extraction of lucuma seeds with NADES-4 favored the hydrolysis of EGCG to the corresponding gallocatechin isomers, such as increasing the TPC.
The presence and identification of GC or EGC could be attributed to the galloylation of catechins, which confers stability (Xiao and Högger, 2015), and to the strong interactions between the protons of hydroxyl groups of catechins and the hydroxyl group of lactic acid or the two hydroxyl groups of ammonium acetate, as the compounds GC, EGC, and NADES-4 could form a complex supramolecular matrix, resulting in higher stability (Zhou et al., 2022). These compounds are essential in the nutritional field since EGC has been shown to suppress the oxidation of low-density lipoproteins (LDL) in blood circulation systems (Nakagawa et al., 1997). Moreover, both EGC and GC exhibit potent antiproliferative and anti-inflammatory effects, much stronger than EGCG (Kürbitz et al., 2011).
4 Conclusion
In this study, after the screening of multiple NADES, the best extraction efficiencies in terms of recovery of total phenol content (2,434.49 mg GAE/100 g LSF, DW) were provided by NADES composed of lactic acid (HBD) and ammonium acetate (HBA) (1:3) compared with conventional solvents 70% methanol (379.9 mg GAE/100 g LSF, DW) and water (390.4 mg/100 g DW) ultrasound-assisted. This solvent was subsequently used to optimize the extraction process. Based on the RSM results, the optimal conditions were the following: time of 80 min, LSF: NADES ratio of 8:100 (w/v), and temperature of 52°C. Under these conditions, the recovery polyphenols was 3,601.51 ± 0.51 mg GAE/100 g LSF, DW. UHPLC–MS analysis evidenced the formation of epigallocatechin isomers from epigallocatechin gallate. Artificial Neural Networks, presented a better prediction capacity than the RSM. However, RSM has the advantage of presenting a regression equation for prediction purposes. It can also show the influence of experimental factors and their interactions on the response compared to neural networks. Therefore, integrating RSM models and neural networks in optimization processes can complement the deficiencies observed using only RSM. The study’s outputs may provide new contributions to waste valorization in terms of the proposed solvent (NADES) for extracting polyphenolic antioxidants from lucuma seed.
Data availability statement
The original contributions presented in the study are included in the article/supplementary material, further inquiries can be directed to the corresponding author.
Author contributions
GP-I: Data curation, Formal analysis, Investigation, Methodology, Writing – original draft, Writing – review & editing. JG-C: Formal analysis, Investigation, Methodology, Writing – review & editing. CO: Conceptualization, Formal analysis, Investigation, Methodology, Resources, Supervision, Validation, Writing – review & editing. IB-P: Data curation, Supervision, Visualization, Writing – review & editing, Writing – original draft. JC: Data curation, Software, Writing – original draft. MI: Conceptualization, Data curation, Funding acquisition, Project administration, Supervision, Visualization, Writing – original draft, Writing – review & editing, Investigation.
Funding
The author(s) declare financial support was received for the research, authorship, and/or publication of this article. This research was supported by Programa Nacional de Ciencia y Estudios Avanzados (PROCIENCIA), a non-profit organization that promotes research development in Peru (contract Nº. 162-2020).
Acknowledgments
We thank Vivero Topara (Chincha, Ica, Peru) for providing Pouteria lucuma fruits.
Conflict of interest
The authors declare that the research was conducted in the absence of any commercial or financial relationships that could be construed as a potential conflict of interest.
Publisher’s note
All claims expressed in this article are solely those of the authors and do not necessarily represent those of their affiliated organizations, or those of the publisher, the editors and the reviewers. Any product that may be evaluated in this article, or claim that may be made by its manufacturer, is not guaranteed or endorsed by the publisher.
References
Abiodun, O. I., Jantan, A., Omolara, A. E., Dada, K. V., Mohamed, N. A., and Arshad, H. (2018). State-of-the-art in artificial neural network applications: a survey. Heliyon 4:e00938. doi: 10.1016/j.heliyon.2018.e00938
Aguilar-Galvez, A., García-Ríos, D., Janampa, C., Mejía, C., Chirinos, R., Pedreschi, R., et al. (2021). Metabolites, volatile compounds and in vitro functional properties during growth and commercial harvest of Peruvian lucuma (Pouteria lucuma). Food Biosci. 40, 100882–100810. doi: 10.1016/j.fbio.2021.100882
Airouyuwa, J. O., Mostafa, H., Ranasinghe, M., and Maqsood, S. (2023). Influence of physicochemical properties of carboxylic acid-based natural deep eutectic solvents (CA-NADES) on extraction and stability of bioactive compounds from date (Phoenix dactylifera L.) seeds: An innovative and sustainable extraction technique. J. Mol. Liq. 388:122767. doi: 10.1016/j.molliq.2023.122767
Airouyuwa, J. O., Mostafa, H., Riaz, A., and Maqsood, S. (2022). Utilization of natural deep eutectic solvents and ultrasound-assisted extraction as green extraction technique for the recovery of bioactive compounds from date palm (Phoenix dactylifera L.) seeds: An investigation into optimization of process parameters. Ultrasonics Sonochemistry 91:106233. doi: 10.1016/j.ultsonch.2022.106233
Alchera, F., Ginepro, M., and Giacalone, G. (2024). Microwave-assisted extraction (MAE) of bioactive compounds from blueberry by-products using a sugar-based NADES: a novelty in green chemistry. LWT 192:115642. doi: 10.1016/j.lwt.2023.115642
Alrugaibah, M., Yagiz, Y., and Gu, L. (2023). Novel natural deep eutectic solvents as efficient green reagents to extract phenolic compounds from olive leaves and predictive modelling by artificial neural networking. Food Bioprod. Process. 138, 198–208. doi: 10.1016/j.fbp.2023.02.006
Anastas, P., and Eghbali, N. (2010). Green chemistry: principles and practice. Chem. Soc. Rev. 39, 301–312. doi: 10.1039/B918763B
Bakirtzi, C., Triantafyllidou, K., and Makris, D. P. (2016). Novel lactic acid-based natural deep eutectic solvents: efficiency in the ultrasound-assisted extraction of antioxidant polyphenols from common native Greek medicinal plants. J. Appl. Res. Med. Aromatic Plants 3, 120–127. doi: 10.1016/j.jarmap.2016.03.003
Bakun, P., Mlynarczyk, D. T., Koczorowski, T., Cerbin-Koczorowska, M., Piwowarczyk, L., Kolasiński, E., et al. (2023). Tea-break with epigallocatechin gallate derivatives–powerful polyphenols of great potential for medicine. Eur. J. Med. Chem. 261:115820. doi: 10.1016/j.ejmech.2023.115820
Bener, M., Şen, F. B., Önem, A. N., Bekdeşer, B., Çelik, S. E., Lalikoglu, M., et al. (2022). Microwave-assisted extraction of antioxidant compounds from by-products of Turkish hazelnut (Corylus avellana L.) using natural deep eutectic solvents: modeling, optimization and phenolic characterization. Food Chem. 385:132633. doi: 10.1016/j.foodchem.2022.132633
Benítez-Correa, E., Bastías-Montes, J. M., Acuña-Nelson, S., and Muñoz-Fariña, O. (2023). Effect of choline chloride-based deep eutectic solvents on polyphenols extraction from cocoa (Theobroma cacao L.) bean shells and antioxidant activity of extracts. Current research in food. Science 7:100614. doi: 10.1016/j.crfs.2023.100614
Bertolo, M. R. V., Bogusz Junior, S., and Mitchell, A. E. (2023). Green strategies for recovery of bioactive phenolic compounds from agro-indusrial wastes (pomegranate peels, almond hulls, and elderberry pomace) using natural deep eutectic solvents. ACS Food Sci. Technol. 3, 2144–2156. doi: 10.1021/acsfoodscitech.3c00367
Bezerra, M. A., Santelli, R. E., Oliveira, E. P., Villar, L. S., and Escaleira, L. A. (2008). Response surface methodology (RSM) as a tool for optimization in analytical chemistry. Talanta 76, 965–977. doi: 10.1016/j.talanta.2008.05.019
Bi, W., Tian, M., and Row, K. H. (2013). Evaluation of alcohol-based deep eutectic solvent in extraction and determination of flavonoids with response surface methodology optimization. J. Chromatogr. A 1285, 22–30. doi: 10.1016/j.chroma.2013.02.041
Bingöl, D., Hercan, M., Elevli, S., and Kılıç, E. (2012). Comparison of the results of response surface methodology and artificial neural network for the biosorption of lead using black cumin. Bioresour. Technol. 112, 111–115. doi: 10.1016/j.biortech.2012.02.084
Boateng, I. D. (2022). A critical review of emerging hydrophobic deep eutectic solvents’ applications in food chemistry: trends and opportunities. J. Agric. Food Chem. 70, 11860–11879. doi: 10.1021/acs.jafc.2c05079
Campos, D., Chirinos, R., Ranilla, L. G., and Pedreschi, R. (2018). Bioactive potential of andean fruits, seeds, and tubers. Adv. Food Nutr. Res. 84, 287–343. doi: 10.1016/bs.afnr.2017.12.005
Chemat, F., Anjum, H., Shariff, A. M., Kumar, P., and Murugesan, T. (2016). Thermal and physical properties of (choline chloride+urea+l-arginine) deep eutectic solvents. J. Mol. Liq. 218, 301–308. doi: 10.1016/j.molliq.2016.02.062
Chen, Y., Chen, W., Fu, L., Yang, Y., Wang, Y., Hu, X., et al. (2019). Surface tension of 50 deep eutectic solvents: effect of hydrogen-bonding donors, hydrogen-bonding acceptors, other solvents, and temperature. Ind. Eng. Chem. Res. 58, 12741–12750. doi: 10.1021/acs.iecr.9b00867
Chen, B. H., Hsieh, C. H., Tsai, S. Y., Wang, C. Y., and Wang, C. C. (2020). Anticancer effects of epigallocatechin-3-gallate nanoemulsion on lung cancer cells through the activation of AMP-activated protein kinase signaling pathway. Sci. Rep. 10:5163. doi: 10.1038/s41598-020-62136-2
Ciric, A., Krajnc, B., Heath, D., and Ogrinc, N. (2020). Response surface methodology and artificial neural network approach for the optimization of ultrasound-assisted extraction of polyphenols from garlic. Food Chem. Toxicol. 135:110976. doi: 10.1016/j.fct.2019.110976
Cui, Q., Liu, J. Z., Wang, L. T., Kang, Y. F., Meng, Y., Jiao, J., et al. (2018). Sustainable deep eutectic solvents preparation and their efficiency in extraction and enrichment of main bioactive flavonoids from sea buckthorn leaves. J. Clean. Prod. 184, 826–835. doi: 10.1016/j.jclepro.2018.02.295
Dai, Y., Spronsen, J., Witkamp, G. J., Verpoorte, R., and Choi, Y. H. (2013a). Natural deep eutectic solvents as new potential media for green technology. Anal. Chim. Acta 766, 61–68. doi: 10.1016/j.aca.2012.12.019
Dai, Y., Witkamp, G. J., Verpoorte, R., and Choi, Y. H. (2013b). Natural deep eutectic solvents as a new extraction media for phenolic metabolites in Carthamus tinctorius L. Anal. Chem. 85, 6272–6278. doi: 10.1021/ac400432p
Dai, Y., Witkamp, G. J., Verpoorte, R., and Choi, Y. H. (2015). Tailoring properties of natural deep eutectic solvents with water to facilitate their applications. Food Chem. 187, 14–19. doi: 10.1016/j.foodchem.2015.03.123
De Los Ángeles Fernández, M., Boiteux, J., Espino, M., Gomez, F. J., and Silva, M. F. (2018). Natural deep eutectic solvents-mediated extractions: the way forward for sustainable analytical developments. Anal. Chim. Acta 1038, 1–10. doi: 10.1016/j.aca.2018.07.059
Dean, A., Voss, D., and Draguljić, D., (Eds.) (2017). “Response surface methodology” in Design and analysis of experiments, 565–614 (Switzerland: Springer Texts in statistics).
Delgado-Mellado, N., Larriba, M., Navarro, P., Rigual, V., Ayuso, M., García, J., et al. (2018). Thermal stability of choline chloride deep eutectic solvents by TGA/FTIR-ATR analysis. J. Mol. Liq. 260, 37–43. doi: 10.1016/j.molliq.2018.03.076
Della Via, F. I., Shiraishi, R. N., Santos, I., Ferro, K. P., Salazar-Terreros, M. J., Franchi Junior, G. C., et al. (2021). (−)-Epigallocatechin-3-gallate induces apoptosis and differentiation in leukaemia by targeting reactive oxygen species and PIN1. Sci. Rep. 11:9103. doi: 10.1038/s41598-021-88478-z
Dini, I. (2011). Flavonoid glycosides from Pouteria obovata (R. Br.) fruit flour. Food Chem. 124, 884–888. doi: 10.1016/j.foodchem.2010.07.013
Dzah, C. S., Duan, Y., Zhang, H., Wen, C., Zhang, J., Chen, G., et al. (2020). The effects of ultrasound assisted extraction on yield, antioxidant, anticancer and antimicrobial activity of polyphenol extracts: a review. Food Biosci. 35:100547. doi: 10.1016/j.fbio.2020.100547
Erzin, Y., Rao, B. H., and Singh, D. N. (2008). Artificial neural network models for predicting soil thermal resistivity. Int. J. Therm. Sci. 47, 1347–1358. doi: 10.1016/j.ijthermalsci.2007.11.001
Florindo, C., Branco, L. C., and Marrucho, I. M. (2017). Development of hydrophobic deep eutectic solvents for extraction of pesticides from aqueous environments. Fluid Phase Equilib. 448, 135–142. doi: 10.1016/j.fluid.2017.04.002
Florindo, C., Branco, L. C., and Marrucho, I. M. (2019). Quest for green-solvent design: from hydrophilic to hydrophobic (deep) eutectic solvents. ChemSusChem 12, 1549–1559. doi: 10.1002/cssc.201900147
Francisco, M., Van Den Bruinhorst, A., Zubeir, L. F., Peters, C. J., and Kroon, M. C. (2013). A new low transition temperature mixture (LTTM) formed by choline chloride+ lactic acid: characterization as solvent for CO2 capture. Fluid Phase Equilib. 340, 77–84. doi: 10.1016/j.fluid.2012.12.001
Friedman, M., and Jürgens, H. S. (2000). Effect of pH on the stability of plant phenolic compounds. J. Agric. Food Chem. 48, 2101–2110. doi: 10.1021/jf990489j
Fuad, F. M., Nadzir, M. M., and Harun, A. (2021). Hydrophilic natural deep eutectic solvent: A review on physicochemical properties and extractability of bioactive compounds. J. Mol. Liq. 339:116923. doi: 10.1016/j.molliq.2021.116923
Fuentealba, C., Gálvez, L., Cobos, A., Olaeta, J. A., Defilippi, B. G., Chirinos, R., et al. (2016). Characterization of main primary and secondary metabolites and in vitro antioxidant and antihyperglycemic properties in the mesocarp of three biotypes of Pouteria lucuma. Food Chem. 190, 403–411. doi: 10.1016/j.foodchem.2015.05.111
García-Ríos, D., Aguilar-Galvez, A., Chirinos, R., Pedreschi, R., and Campos, D. (2020). Relevant physicochemical properties and metabolites with functional properties of two commercial varieties of Peruvian Pouteria lucuma. J. Food Proc. Preserv. 44:e14479. doi: 10.1111/jfpp.14479
Geyikçi, F., Kılıç, E., Çoruh, S., and Elevli, S. (2012). Modelling of lead adsorption from industrial sludge leachate on red mud by using RSM and ANN. Chem. Eng. J. 183, 53–59. doi: 10.1016/j.cej.2011.12.019
Ghaedi, H., Ayoub, M., Sufian, S., Shariff, A. M., and Lal, B. (2017). The study on temperature dependence of viscosity and surface tension of several Phosphonium-based deep eutectic solvents. J. Mol. Liq. 241, 500–510. doi: 10.1016/j.molliq.2017.06.024
Gil-Martín, E., Forbes-Hernández, T., Romero, A., Cianciosi, D., Giampieri, F., and Battino, M. (2022). Influence of the extraction method on the recovery of bioactive phenolic compounds from food industry by-products. Food Chem. 378:131918. doi: 10.1016/j.foodchem.2021.131918
Giri, A. K., and Mishra, P. C. (2022). Optimization of different process parameters for the removal efficiency of fluoride from aqueous medium by a novel bio-composite using box-Behnken design. J. Environ. Chem. Eng. 11:109232. doi: 10.1016/j.jece.2022.109232
Gómez-Maqueo, A., Bandino, E., Hormaza, J. I., and Cano, M. P. (2020). Characterization and the impact of in vitro simulated digestion on the stability and bioaccessibility of carotenoids and their esters in two Pouteria lucuma varieties. Food Chem. 316:126369. doi: 10.1016/j.foodchem.2020.126369
Gu, J., Wang, Z., Kuen, J., Ma, L., Shahroudy, A., Shuai, B., et al. (2018). Recent advances in convolutional neural networks. Pattern Recogn. 77, 354–377. doi: 10.1016/j.patcog.2017.10.013
Guerrero-Castillo, P., Reyes, S., Robles, J., Simirgiotis, M. J., Sepulveda, B., Fernandez-Burgos, R., et al. (2019). Biological activity and chemical characterization of Pouteria lucuma seeds: A possible use of an agricultural waste. Waste Manag. 88, 319–327. doi: 10.1016/j.wasman.2019.03.055
Hayyan, M., Abo-Hamad, A., AlSaadi, M. A., and Hashim, M. A. (2015). Functionalization of graphene using deep eutectic solvents. Nanoscale Res. Lett. 10, 1–26. doi: 10.1186/s11671-015-1004-2
Heck, M. A., Melková, I., Posten, C., Decker, E. L., and Reski, R. (2021). Medium optimization for biomass production of three peat moss (Sphagnum L.) species using fractional factorial design and response surface methodology. Biores. Technol. Rep. 15:100729. doi: 10.1016/j.biteb.2021.100729
Hikmawanti, N. P. E., Ramadon, D., Jantan, I., and Mun’im, A. (2021). Natural deep eutectic solvents (NADES): phytochemical extraction performance enhancer for pharmaceutical and nutraceutical product development. Plan. Theory 10:2091. doi: 10.3390/plants10102091
Hong, S. M., Kamaruddin, A. H., and Nadzir, M. M. (2023). Sustainable ultrasound-assisted extraction of polyphenols from Cinnamomum cassia bark using hydrophilic natural deep eutectic solvents. Process Biochem. 132, 323–336. doi: 10.1016/j.procbio.2023.07.026
Hu, R. S., Yu, L., Zhou, S. Y., Zhou, H. F., Wan, H. T., and Yang, J. H. (2023). Comparative study on optimization of NADES extraction process by dual models and antioxidant activity of optimum extraction from chuanxiong-Honghua. LWT Food Sci. Technol. 184:114991. doi: 10.1016/j.lwt.2023.114991
Imteyaz, S., and Ingole, P. P. (2023). Comparison of physicochemical properties of choline chloride-based deep eutectic solvents for CO2 capture: Progress and outlook. J. Mol. Liq. 376:121436. doi: 10.1016/j.molliq.2023.121436
Inga, M., Aguilar-Galvez, A., and Campos, D. (2021). Postharvest maturation of Pouteria lucuma: effect of storage conditions on physicochemical components, metabolites and antioxidant and hypoglycemic capacity. Scientia Agropecuaria 12, 411–419. doi: 10.17268/sci.agropecu.2021.045
Inga, M., Betalleluz-Pallardel, I., Puma-Isuiza, G., Cumpa-Arias, L., Osorio, C., Valdez-Arana, J. D. C., et al. (2024). Chemical analysis and bioactive compounds from agrifood by products of peruvian crops. Front. Sustain. Food Syst 8:1341895. doi: 10.3389/fsufs.2024.1341895
Inga, M., García, J. M., Aguilar-Galvez, A., Campos, D., and Osorio, C. (2019). Chemical characterization of odour-active volatile compounds during lucuma (Pouteria lucuma) fruit ripening. CyTA J. Food 17, 494–500. doi: 10.1080/19476337.2019.1593248
Jangir, A. K., Sethy, P., Verma, G., Bahadur, P., and Kuperkar, K. (2021). An inclusive thermophysical and rheology portrayal of deep eutectic solvents (DES) for metal oxides dissolution enhancement. J. Mol. Liq. 332:115909. doi: 10.1016/j.molliq.2021.115909
Jeong, K. M., Ko, J., Zhao, J., Jin, Y., Han, S. Y., and Lee, J. (2017). Multi-functioning deep eutectic solvents as extraction and storage media for bioactive natural products that are readily applicable to cosmetic products. J. Clean. Prod. 151, 87–95. doi: 10.1016/j.jclepro.2017.03.038
Jha, A. K., and Sit, N. (2021). Comparison of response surface methodology (RSM) and artificial neural network (ANN) modelling for supercritical fluid extraction of phytochemicals from Terminalia chebula pulp and optimization using RSM coupled with desirability function (DF) and genetic algorithm (GA) and ANN with GA. Ind. Crop. Prod. 170:113769. doi: 10.1016/j.indcrop.2021.113769
Karakashov, B., Grigorakis, S., Loupassaki, S., and Makris, D. P. (2015). Optimisation of polyphenol extraction from Hypericum perforatum (St. John’s wort) using aqueous glycerol and response surface methodology. J. Appl. Res. Med. Aromatic Plants 2, 1–8. doi: 10.1016/j.jarmap.2014.11.002
Khan, K., Johari, M. A. M., Amin, M. N., Khan, M. I., and Iqbal, M. (2023). Optimization of colloidal nano-silica based cementitious mortar composites using RSM and ANN approaches. Results Eng. 20:101390. doi: 10.1016/j.rineng.2023.101390
Koraqi, H., Aydar, A. Y., Pandiselvam, R., Qazimi, B., Khalid, W., Petkoska, A. T., et al. (2024). Optimization of extraction condition to improve blackthorn (Prunus spinosa L.) polyphenols, anthocyanins and antioxidant activity by natural deep eutectic solvent (NADES) using the simplex lattice mixture design method. Microchem. J. 200:110497. doi: 10.1016/j.microc.2024.110497
Koutsoukos, S., Tsiaka, T., Tzani, A., Zoumpoulakis, P., and Detsi, A. (2019). Choline chloride and tartaric acid, a natural deep eutectic solvent for the efficient extraction of phenolic and carotenoid compounds. J. Clean. Prod. 241:118384. doi: 10.1016/j.jclepro.2019.118384
Kürbitz, C., Heise, D., Redmer, T., Goumas, F., Arlt, A., Lemke, J., et al. (2011). Epicatechin gallate and catechin gallate are superior to epigallocatechin gallate in growth suppression and anti-inflammatory activities in pancreatic tumor cells. Cancer Sci. 102, 728–734. doi: 10.1111/j.1349-7006.2011.01870.x
Lanjekar, K. J., Gokhale, S., and Rathod, V. K. (2022). Utilization of waste mango peels for extraction of polyphenolic antioxidants by ultrasound-assisted natural deep eutectic solvent. Biores. Technol. Rep. 18:101074. doi: 10.1016/j.biteb.2022.101074
Liu, Y., Zhe, W., Zhang, R., Peng, Z., Wang, Y., Gao, H., et al. (2022). Ultrasonic-assisted extraction of polyphenolic compounds from Paederia scandens (Lour.) Merr. Using deep eutectic solvent: optimization, identification, and comparison with traditional methods. Ultrason. Sonochem. 86:106005. doi: 10.1016/j.ultsonch.2022.106005
Macchioni, V., Carbone, K., Cataldo, A., Fraschini, R., and Bellucci, S. (2021). Lactic acid-based deep natural eutectic solvents for the extraction of bioactive metabolites of Humulus lupulus L.: supramolecular organization, phytochemical profiling and biological activity. Sep. Purif. Technol. 264:118039. doi: 10.1016/j.seppur.2020.118039
Makris, D. P. (2018). Green extraction processes for the efficient recovery of bioactive polyphenols from wine industry solid wastes–recent progress. Curr. Opin. Green Sustain. Chem. 13, 50–55. doi: 10.1016/j.cogsc.2018.03.013
Martinez, F. A. C., Balciunas, E. M., Salgado, J. M., González, J. M. D., Converti, A., and de Souza Oliveira, R. P. (2013). Lactic acid properties, applications and production: A review. Trends Food Sci. Technol. 30, 70–83. doi: 10.1016/j.tifs.2012.11.007
Mazzeo, L., Bertino, A., De Paola, L., Gallo, V., Della Posta, S., Fanali, C., et al. (2023). Equilibrium and fixed-bed kinetics study on hazelnut skin polyphenols extraction using choline chloride–lactic acid-based NADES and water as solvents. Sep. Purif. Technol. 327:124896. doi: 10.1016/j.seppur.2023.124896
Medina-Remón, A., Barrionuevo-González, A., Zamora-Ros, R., Andres-Lacueva, C., Estruch, R., Martínez-González, M. Á., et al. (2009). Rapid Folin–Ciocalteu method using microtiter 96-well plate cartridges for solid phase extraction to assess urinary total phenolic compounds, as a biomarker of total polyphenols intake. Anal. Chim. Acta 634, 54–60. doi: 10.1016/j.aca.2008.12.012
MIDAGRI. (2023). SIEA—BI. Available at: https://siea.midagri.gob.pe/portal/siea_bi/index.html
Mišan, A., Nađpal, J., Stupar, A., Pojić, M., Mandić, A., Verpoorte, R., et al. (2020). The perspectives of natural deep eutectic solvents in Agri-food sector. Crit. Rev. Food Sci. Nutr. 60, 2564–2592. doi: 10.1080/10408398.2019.1650717
Mitar, A., Panić, M., Prlić Kardum, J., Halambek, J., Sander, A., Zagajski Kučan, K., et al. (2019). Physicochemical properties, cytotoxicity, and antioxidative activity of natural deep eutectic solvents containing organic acid. Chem. Biochem. Eng. Q. 33, 1–18. doi: 10.15255/CABEQ.2018.1454
Moradi, M., Pazuki, G., and Mahmoudabadi, S. Z. (2023). A comprehensive study of the water stability of eutectic solvents using COSMO-SAC. J. Mol. Liq. 387:122605. doi: 10.1016/j.molliq.2023.122605
Musa, K. H., Abdullah, A., and Al-Haiqi, A. (2016). Determination of DPPH free radical scavenging activity: application of artificial neural networks. Food Chem. 194, 705–711. doi: 10.1016/j.foodchem.2015.08.038
Nakagawa, K., Okuda, S., and Miyazawa, T. (1997). Dose-dependent incorporation of tea catechins, (−)-epigallocatechin-3-gallate and (−)-epigallocatechin, into human plasma. Biosci. Biotechnol. Biochem. 61, 1981–1985. doi: 10.1271/bbb.61.1981
Negi, T., Kumar, A., Sharma, S. K., Rawat, N., Saini, D., Sirohi, R., et al. (2024). Deep eutectic solvents: preparation, properties, and food applications. Heliyon 10:e28784. doi: 10.1016/j.heliyon.2024.e28784
Ninayan, R., Levshakova, A. S., Khairullina, E. M., Vezo, O. S., Tumkin, I. I., Ostendorf, A., et al. (2023). Water-induced changes in choline chloride-carboxylic acid deep eutectic solvents properties. Colloids Surf. A Physicochem. Eng. Asp. 679:132543. doi: 10.1016/j.colsurfa.2023.132543
Ojeda, G. A., Vallejos, M. M., Sgroppo, S. C., Sánchez-Moreno, C., and De Ancos, B. (2023). Enhanced extraction of phenolic compounds from mango by-products using deep eutectic solvents. Heliyon 9:e16912. doi: 10.1016/j.heliyon.2023.e16912
Oliveira, G., Oliveira, A. E., Da Conceição, E. C., and Leles, M. I. (2016). Multiresponse optimization of an extraction procedure of carnosol and rosmarinic and carnosic acids from rosemary. Food Chem. 211, 465–473. doi: 10.1016/j.foodchem.2016.05.042
Omar, K. A., and Sadeghi, R. (2022). Physicochemical properties of deep eutectic solvents: A review. J. Mol. Liq. 360:119524. doi: 10.1016/j.molliq.2022.119524
Pal, C. B. T., and Jadeja, G. C. (2020). Microwave-assisted extraction for recovery of polyphenolic antioxidants from ripe mango (Mangifera indica L.) peel using lactic acid/sodium acetate deep eutectic mixtures. Food Sci. Technol. Int. 26, 78–92. doi: 10.1177/1082013219870010
Pavlić, B., Kaplan, M., Bera, O., Olgun, E. O., Canli, O., Milosavljević, N., et al. (2019). Microwave-assisted extraction of peppermint polyphenols–artificial neural networks approach. Food Bioprod. Process. 118, 258–269. doi: 10.1016/j.fbp.2019.09.016
Peng, X., Duan, M. H., Yao, X. H., Zhang, Y. H., Zhao, C. J., Zu, Y. G., et al. (2016). Green extraction of five target phenolic acids from Lonicerae japonicae Flos with deep eutectic solvent. Sep. Purif. Technol. 157, 249–257. doi: 10.1016/j.seppur.2015.10.065
Poole, C. F., and Poole, S. K. (2010). Extraction of organic compounds with room temperature ionic liquids. J. Chromatogr. A 1217, 2268–2286. doi: 10.1016/j.chroma.2009.09.011
Popovic, B. M., Micic, N., Potkonjak, A., Blagojevic, B., Pavlovic, K., Milanov, D., et al. (2022). Novel extraction of polyphenols from sour cherry pomace using natural deep eutectic solvents–ultrafast microwave-assisted NADES preparation and extraction. Food Chem. 366:130562. doi: 10.1016/j.foodchem.2021.130562
Posta, D. S., Gallo, V., Ascrizzi, A. M., Gentili, A., De Gara, L., Dugo, L., et al. (2023). Development of a green ultrasound-assisted procedure for the extraction of phenolic compounds from avocado peel with deep eutectic solvents. Green Analyt. Chem. 7:100083. doi: 10.1016/j.greeac.2023.100083
Prieto, J. M. (2012). Procedure: preparation of DPPH radical, and antioxidant scavenging assay. DPPH Microplate Protocol, 7–9
Qin, G., Zhang, F., Ren, M., Chen, X., Liu, C., Li, G., et al. (2023). Eco-friendly and efficient extraction of polyphenols from Ligustrum robustum by deep eutectic solvent assisted ultrasound. Food Chem. 429:136828. doi: 10.1016/j.foodchem.2023.136828
Rebollo-Hernanz, M., Cañas, S., Taladrid, D., Segovia, Á., Bartolomé, B., Aguilera, Y., et al. (2021). Extraction of phenolic compounds from cocoa shell: modeling using response surface methodology and artificial neural networks. Sep. Purif. Technol. 270:118779. doi: 10.1016/j.seppur.2021.118779
Ren, H., Lian, S., Wang, X., Zhang, Y., and Duan, E. (2018). Exploiting the hydrophilic role of natural deep eutectic solvents for greening CO2 capture. J. Clean. Prod. 193, 802–810. doi: 10.1016/j.jclepro.2018.05.051
Saini, A., Kumar, A., Panesar, P. S., and Thakur, A. (2022). Potential of deep eutectic solvents in the extraction of value-added compounds from agro-industrial by-products. Appl. Food Res. 2:100211. doi: 10.1016/j.afres.2022.100211
Saputro, D. R. S., and Widyaningsih, P. (2017). Limited memory Broyden-fletcher-Goldfarb-Shanno (L-BFGS) method for the parameter estimation on geographically weighted ordinal logistic regression model (GWOLR). AIP conference proceedings 1868, 1–9. doi: 10.1063/1.4995124
Savi, L. K., Carpiné, D., Waszczynskyj, N., Ribani, R. H., and Haminiuk, C. W. I. (2019). Influence of temperature, water content and type of organic acid on the formation, stability and properties of functional natural deep eutectic solvents. Fluid Phase Equilib. 488, 40–47. doi: 10.1016/j.fluid.2019.01.025
Shahbaz, K., Mjalli, F. S., Hashim, M. A., and AlNashef, I. M. (2012). Prediction of the surface tension of deep eutectic solvents. Fluid Phase Equilib. 319, 48–54. doi: 10.1016/j.fluid.2012.01.025
Shi, C. F., Yang, H. T., Chen, T. T., Guo, L. P., Leng, X. Y., Deng, P. B., et al. (2022). Artificial neural network-genetic algorithm-based optimization of aerobic composting process parameters of Ganoderma lucidum residue. Bioresour. Technol. 357:127248. doi: 10.1016/j.biortech.2022.127248
Skulcova, A., Russ, A., Jablonsky, M., and Sima, J. (2018). The pH behavior of seventeen deep eutectic solvents. Bioresources 13, 5042–5051. doi: 10.15376/biores.13.3.5042-5051
Smith, E. L., Abbott, A. P., and Ryder, K. S. (2014). Deep eutectic solvents (DESs) and their applications. Chem. Rev. 114, 11060–11082. doi: 10.1021/cr300162p
Sugihara, N., Kuroda, N., Watanabe, F., Choshi, T., Kamishikiryo, J., and Seo, M. (2017). Effects of Catechins and their related compounds on cellular accumulation and efflux transport of Mitoxantrone in Caco-2 cell monolayers. J. Food Sci. 82, 1224–1230. doi: 10.1111/1750-3841.13680
Tang, W., An, Y., and Row, K. H. (2021). Emerging applications of (micro) extraction phase from hydrophilic to hydrophobic deep eutectic solvents: opportunities and trends. TrAC Trends Anal. Chem. 136:116187. doi: 10.1016/j.trac.2021.116187
Tang, W., Dai, Y., and Row, K. H. (2018). Evaluation of fatty acid/alcohol-based hydrophobic deep eutectic solvents as media for extracting antibiotics from environmental water. Anal. Bioanal. Chem. 410, 7325–7336. doi: 10.1007/s00216-018-1346-6
Teslić, N., Bojanić, N., Rakić, D., Takači, A., Zeković, Z., Fišteš, A., et al. (2019). Defatted wheat germ as source of polyphenols—optimization of microwave-assisted extraction by RSM and ANN approach. Chem. Eng. Proc. Proc. Intens. 143:107634. doi: 10.1016/j.cep.2019.107634
Threlfall, R., Morris, R., and Meullenet, J. (2007). Product development and nutraceutical analysis to enhance the value of dried fruit. J. Food Qual. 30, 552–566. doi: 10.1111/j.1745-4557.2007.00142.x
Tsao, R. (2010). Chemistry and biochemistry of dietary polyphenols. Nutrients 2, 1231–1246. doi: 10.3390/nu2121231
Veličković, D. T., Milenović, D. M., Ristić, M. S., and Veljković, V. B. (2006). Kinetics of ultrasonic extraction of extractive substances from garden (Salvia officinalis L.) and glutinous (Salvia glutinosa L.) sage. Ultrasonics Sonochemistry 13, 150–156. doi: 10.1016/j.ultsonch.2005.02.002
Villanueva, E., Glorio-Paulet, P., Giusti, M. M., Sigurdson, G. T., Yao, S., and Rodríguez-Saona, L. E. (2023). Screening for pesticide residues in cocoa (Theobroma cacao L.) by portable infrared spectroscopy. Talanta 257:124386. doi: 10.1016/j.talanta.2023.124386
Wang, W., An, M., Zhao, G., Wang, Y., Yang, D., Zhang, D., et al. (2023). Ultrasonic-assisted customized natural deep eutectic solvents extraction of polyphenols from Chaenomeles speciosa. Microchem. J. 193:108952. doi: 10.1016/j.microc.2023.108952
Wang, W., Pan, Y., Zhao, J., Wang, Y., Yao, Q., and Li, S. (2024). Development and optimization of green extraction of polyphenols in Michelia alba using natural deep eutectic solvents (NADES) and evaluation of bioactivity. Sustain. Chem. Pharm. 37:101425. doi: 10.1016/j.scp.2023.101425
Wen, Y., Zhao, R., Gupta, P., Fan, Y., Zhang, Y., Huang, Z., et al. (2019). The epigallocatechin gallate derivative Y6 reverses drug resistance mediated by the ABCB1 transporter both in vitro and in vivo. Acta Pharm. Sin. B 9, 316–323. doi: 10.1016/j.apsb.2018.10.001
Witek-Krowiak, A., Chojnacka, K., Podstawczyk, D., Dawiec, A., and Bubała, K. (2014). Application of response surface methodology and artificial neural network methods in modelling and optimization of biosorption process. Bioresour. Technol. 160, 150–160. doi: 10.1016/j.biortech.2014.01.021
Xi, J., Xue, Y., Xu, Y., and Shen, Y. (2013). Artificial neural network modeling and optimization of ultrahigh pressure extraction of green tea polyphenols. Food Chem. 141, 320–326. doi: 10.1016/j.foodchem.2013.02.084
Xiao, J., and Högger, P. (2015). Stability of dietary polyphenols under the cell culture conditions: avoiding erroneous conclusions. J. Agric. Food Chem. 63, 1547–1557. doi: 10.1021/jf505514d
Yang, Z. (2019). Natural deep eutectic solvents and their applications in biotechnology. Appl. Ionic Liq. Biotech. 168:67. doi: 10.1007/10_2018_67
Yang, Q. Q., Gan, R. Y., Zhang, D., Ge, Y. Y., Cheng, L. Z., and Corke, H. (2019). Optimization of kidney bean antioxidants using RSM & ANN and characterization of antioxidant profile by UPLC-QTOF-MS. LWT Food Sci. Technol. 114:108321. doi: 10.1016/j.lwt.2019.108321
Zannou, O., Pashazadeh, H., Galanakis, C. M., Alamri, A. S., and Koca, I. (2022). Carboxylic acid-based deep eutectic solvents combined with innovative extraction techniques for greener extraction of phenolic compounds from sumac (Rhus coriaria L.). J. Appl. Res. Med. Arom. Plants 30:100380. doi: 10.1016/j.jarmap.2022.100380
Zhang, H., Lang, J., Lan, P., Yang, H., Lu, J., and Wang, Z. (2020). Study on the dissolution mechanism of cellulose by ChCl-based deep eutectic solvents. Materials 13:278. doi: 10.3390/ma13020278
Zheng, B., Yuan, Y., Xiang, J., Jin, W., Johnson, J. B., Li, Z., et al. (2022). Green extraction of phenolic compounds from foxtail millet bran by ultrasonic-assisted deep eutectic solvent extraction: optimization, comparison and bioactivities. LWT-Food Sci. Technol. 154:112740. doi: 10.1016/j.lwt.2021.112740
Keywords: polyphenols, natural antioxidants, gallocatechin, green chemistry, circular economy
Citation: Puma-Isuiza G, García-Chacón JM, Osorio C, Betalleluz-Pallardel I, Chue J and Inga M (2024) Extraction of phenolic compounds from lucuma (Pouteria lucuma) seeds with natural deep eutectic solvents: modelling using response surface methodology and artificial neural networks. Front. Sustain. Food Syst. 8:1401825. doi: 10.3389/fsufs.2024.1401825
Edited by:
Jiahao Yu, Zhejiang University of Technology, ChinaReviewed by:
Nishant Rachayya Swami Hulle, Tezpur University, IndiaZhijian Tan, Chinese Academy of Agricultural Sciences, China
Copyright © 2024 Puma-Isuiza, García-Chacón, Osorio, Betalleluz-Pallardel, Chue and Inga. This is an open-access article distributed under the terms of the Creative Commons Attribution License (CC BY). The use, distribution or reproduction in other forums is permitted, provided the original author(s) and the copyright owner(s) are credited and that the original publication in this journal is cited, in accordance with accepted academic practice. No use, distribution or reproduction is permitted which does not comply with these terms.
*Correspondence: Marianela Inga, bWFyaWFuZWxhaW5nYUBsYW1vbGluYS5lZHUucGU=