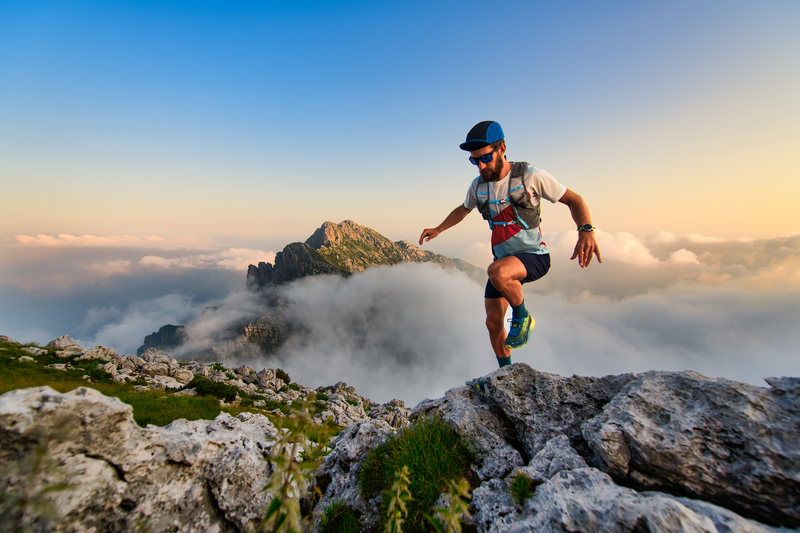
95% of researchers rate our articles as excellent or good
Learn more about the work of our research integrity team to safeguard the quality of each article we publish.
Find out more
ORIGINAL RESEARCH article
Front. Sustain. Food Syst. , 12 June 2024
Sec. Climate-Smart Food Systems
Volume 8 - 2024 | https://doi.org/10.3389/fsufs.2024.1393129
This article is part of the Research Topic Increasing Resilience through Multi-Species Intercropping, Agroforestry and Mixed Grazing Crop-Livestock Systems View all 10 articles
The agricultural productivity and sustainability in Eastern Gangetic Plain Zones of India are threatened because of the inefficiency of current production practices, shortage of resources, and socioeconomic constraints. We hypothesized the potential impact of intensified cereal systems with mung bean as a third crop within the annual cropping cycle. We assessed economic, social, and environmental indicators for intensified and current cropping system management practiced by different farm types in the region using the FarmDESIGN model. Building on a farm typology constructed for the region in our past research, we used five types of farmers: part-time (PT), well-endowed (WE), small-scale (SS) crop and livestock mix, medium-scale (MS), and resource-poor farmers (RP) in this study. The performance indicators of the 229 original cropping systems cultivated within the 43 farms varied strongly in the eight performance indicators. This variability of cropping systems performance within the farm types resulted in the absence of significant differences between the types. Compared to the original cropping systems, the intensified cropping systems with mung bean not only performed high in dietary energy (DE) production and organic matter (OM) inputs into the soil but also had high application rates of biocides and minimized losses of nitrogen (N). The intervention systems were low in labor requirement and scored at an intermediate level for crop gross margin, water use, and greenhouse gas (GHG) emissions. The ranges of areas of maize- and rice-based systems that could be replaced by intensified systems were largest for the WE and RP farm types. This was reflected in large ranges of change in the performance indicators, but no significant differences in response were found between the farm types. The intensification of maize- and rice-based systems with the proposed intervention cropping systems involving mung bean would result in increased profitability, higher DE yield, and lower requirements for labor and water as the proportion of the farms being converted increases. However, the use of biocides would increase, while the intervention cropping systems would have no significant effect on OM input, GHG emissions, and soil N losses.
In the Eastern Indo-Gangetic Plains (EIGP) of South Asia, an estimated 450 million people live in extreme poverty, with a high-density population of over 1,000 persons per square kilometer, relying on cereals for primary subsistence (Gathala et al., 2021). The Eastern Gangetic Plain (EGP) zones cover approximately 6.0 million hectares of rice–wheat (R-W) and rice–rice double-cropping systems, respectively, and the rice–maize system is emerging as an alternative profitable system in this region (Gathala et al., 2022). The IGP has a high degree of spatial variability in terms of poverty and farm size, with a clear low-to-high gradient of food insecurity from the western regions toward the EIGP (Erenstein and Thorpe, 2011). Challenges to the agricultural systems in the EIGP include low crop productivity correlated with the region’s increased poverty, farmers’ low investment capability and aversion to risk, and increasing energy and input costs, in addition to climactic variability (Gathala et al., 2022). Mixed crop-livestock systems are common as is farmer engagement in seasonal and semi-permanent migration and off-farm labor (Erenstein and Thorpe, 2011). The EIGP is one of the most vulnerable regions to climate change in India due to heat, drought, and flood risks, in addition to increasingly erratic monsoon precipitation (Gathala et al., 2020a,b).
Over the last decade, alternative cropping systems employing the principles of Conservation Agriculture (CA) have been developed through on-station (Jat et al., 2014) and on-farm validation trials conducted over 4 years across Bihar (Gathala et al., 2020a,b). Alternative technologies such as no-till and crop residue recycling have shown potential advantages in terms of resource use efficiency and yield stability to climate change and variability in a wide range of agro-ecologies (Jat et al., 2014; Rakesh et al., 2021), which could support the adaptive and productive capacity of farming systems to future climates and resource scenarios. CA-based practices have a larger potential to improve household food availability for various farm types in the EIGP than livestock interventions (Lopez-Ridaura et al., 2018). The implementation of CA interventions requires an analysis of trade-offs and synergies among productive, socio-economic, and environmental performance indicators. To exploit synergies and minimize trade-offs, decision support tools can be used to identify portfolios of practices in the IGP (Jat et al., 2019a).
CA interventions aim to improve crop yields, enhance input use efficiency, and increase net farm incomes (Hoque et al., 2013; Dutta et al., 2020; Jat et al., 2020; Rakesh et al., 2021) while reducing greenhouse gas emissions (Sapkota et al., 2019). These interventions have been extensively tested at the field level in the EGP (Gathala et al., 2020a,b). The inclusion of legumes and CA-based management optimization can be a potential option to ensure nutritious food for the dwelling communities and the sustainability of natural resources in the IGP of India (Gora et al., 2022).
Some agronomic technologies such as zero tillage are widely promoted in the EIGP, but do not fit in all diversified farming systems due to their low and separated adoption (Singh et al., 2016), adding more challenges to cereal production in the region (Lopez-Ridaura et al., 2018). Sustainability of intensive cereal systems can be achieved through CA-based management with sustainable intensification and residue recycling in cereal-based cropping systems (Dutta et al., 2023). The inclusion of legumes in the cropping systems (Samal et al., 2017) has the potential to contribute to the accumulation of soil organic carbon resulting in better soil health, higher crop productivity, and sustainability. Crop diversification, along with the integration of oilseeds and pulses crops into sustainable intensification, has been recognized as an effective strategy for achieving the objectives of food and nutritional security, sustainable management of land and water resources (Yadav et al., 2021), and enhancing the production of dietary energy, fats, and protein (Damerau et al., 2020).
The farming system design aims to develop options for sustainable intensification by integrating diverse crops, improved practices, and technologies. The benefits are analyzed in terms of productive, socio-economic, and environmental performance indicators (Groot et al., 2012). A multi-enterprise model for sustainable production under diverse situations can not only increase system productivity, profitability, and livelihood security of farming communities but also help in resource conservation and mitigating risks and uncertainties (Cortez-Arriola et al., 2016). Various computational tools have been developed to simulate and explore alternatives that can inform the re-design of farming systems. Modeling tools have been used to precisely simulate and optimize yield, profitability, labor, and whole farm management in agriculture (Tittonell, 2014). Analysis at the farm scale by using a model as an additional tool simultaneously with empirical information supports scientists and advisors to illustrate accurately potential outcomes and to relate these to individual farm objectives. The FarmDESIGN model (Groot et al., 2012; Ditzler et al., 2019) supports iterative cycles of farm structure reconfiguration allowing the analysis of mixed crop-livestock farming systems. In the EGP, a prominent cropping sequence is rice during the kharif season, wheat during the rabi season, and fallow during the summer season. Additionally, maize intercropping with potato during the rabi season and rice during the kharif season is also practiced on a large scale. To assess the impact of short-duration mung bean (Vigna radiata) in the summer season, three alternative cropping patterns were constructed in combination with common kharif (rice and maize) and rabi (wheat and mustard) crops: rice–wheat–mung bean; maize–wheat–mung bean; and maize–mustard–mung bean. The objectives of this study were (1) to identify sustainable intensification opportunities through diversified cropping systems to increase food production by sustainably increasing the productivity of smallholder farms and (2) to assess current and intensified cropping sequence on farm performance and quantify the contribution of CA practices that enhance farm economic performance, while improving resource use efficiency and reducing negative environmental impacts of diverse farming systems at the farm level in smallholder systems of Bihar.
Bihar is located in the Eastern part of India. It has a geographic area of 94,163 km2 divided into two parts by the river Ganges that flows from west to east. Over 66% of the geographic area is cultivated. Agriculture in Bihar is characterized by low productivity, large yield gaps, high uncertainty, and instability in crop production. In the state, approximately 91% of farmers have marginal holdings, with each holding less than one hectare of land. Cropping intensity is also low (1.45%). Approximately 60% of the gross cropped area is irrigated; tube wells are the main source of irrigation followed by canal irrigation [Agriculture Census (2015–16), 2019]. The rotation of rice during the kharif season (July to November), wheat during the rabi season (November to April), and fallow during the summer season (April to June) is the dominant cropping system in the region. The climate is hot and humid in summer and cold in winter with 1,140 mm average annual rainfall, of which 70% occurs during the months of June to September. The mean annual maximum and minimum temperatures are 30°C and 19°C, respectively, with 60–95% relative humidity throughout the year.
In total, 43 farmers representing 5 farm types; [well endowed (WE), part-time (PT), medium scale (MS), small scale (SS), and resource poor (RP)], were selected randomly from different farm types of the region defined by Lopez-Ridaura et al. (2018). This typology was constructed based on structural farm properties comprising land, workforce, market integration and income, cropping patterns, use of crop residues, and farm-produced household food consumption for each farm household based on farm productions and prices.
Structured in-depth interviews were conducted to collect input data for FarmDESIGN from 10 villages of Vaishali, Samastipur, and Muzaffarpur Districts of Bihar, consisting of a total of 43 farms. The Impact LITE survey instrument (Rufino et al., 2013) was adapted to the local context and used to collect the data for the farmer’s case study. Environment, economics, farm-produced food household consumption, crop management, livestock management, and applicable intervention were entered into FarmDESIGN. All methods were carried out in accordance with relevant guidelines and regulations. All experimental protocols were approved by the research committee CIMMYT. The informed consent was obtained from all subjects and/or their legal guardian(s).
A static farm balance model (FarmDESIGN) was used to calculate flows and balances of carbon, nitrogen, phosphorus, and potassium to, through, and from a farm, the feed balance, the amount and composition of manure, labor balance, and economic results on an annual basis (Groot et al., 2012; Ditzler et al., 2019). Input data representing management options described rotations, crop groups and crops (area, yield, and destination), farm animals (species, number, weight, growth, production, and activities), feed rations, additional fertilizers, labor, equipment, and buildings. Economic calculations allowed the determination of crop and animal margins, fixed costs, operating profit, and return to labor. Information from the soil, crops, livestock, labor, income, inputs, imports, and nutrients cycling at the farm scale was used as data input in the FarmDESIGN model.
Eight indicators were selected as objectives based on the farm diagnosis performed in the region. Gross margin = crop gross margin (×1,000 INR ha−1); DE yield = dietary energy yield expressed in number of persons that can be sufficiently fed per ha; labour input = labour required for crop cultivation (h ha−1); OM input = organic matter added to the soil (kg ha−1); water use = amount of irrigation water applied (m3 ha−1); GHG emiss. = greenhouse gas emissions (Mg CO2-eq ha−1); biocide use = biocide active ingredients applied (kg ha−1); and soil N loss = loss of nitrogen (N) from the cropping system (kg ha−1).
In this study, we analyzed the objective as maximizing the gross crop margin of the farm, water saving, DE yield, and soil organic matter (SOM) input and minimizing the soil N loss, GHG emissions, use of biocides, and labor inputs while intensified cropping sequences. Soil organic matter balance, water saving and nitrogen balance, GHG emission, and biocide use function as proxies for environmental health. This selection was such that it targeted improving the social, economic, and environmental aspects of the farms and farmers’ livelihoods, by maximizing the profitability (expressed in financial terms; Indian rupees, INR), thus increasing economic performance. Minimizing the nitrogen (N) soil losses (kg ha−1 year−1) enhances the N availability of the farming system. Maximizing the SOM input (kg OM ha−1 year−1) enhances inherent soil fertility and water retention. Minimizing labor use (h ha−1) therefore improves the availability of labor for other farm operations or improves livelihood time. Minimizing GHG emissions therefore minimizes global warming and affects various aspects of climate, including surface air temperatures, precipitation, and sea levels. Maximizing DE yield makes food and nutrition available for a family on the farm. Minimizing biocide use therefore minimizes harmful effects on human health and soil and environmental health. The interactions between farm components under these eight farm objectives. Gross margin (Indian rupees INR year−1) is the farm economics indicator, calculated as the difference between gross margin and farm input costs, such as manure and fertilizer, hired labor, and general farm maintenance costs. SOM input (kg ha−1 year−1) is calculated as the difference between the added organic matter into the soil and the losses by degradation and erosion. Water requirement for irrigation (m3) measures the depletion of available water resources and the amount of water applied to each of the crops. Farm nitrogen losses (kg N ha−1 year−1) is calculated directly after excretion, during storage, and after application on the field using loss fractions. DE yield is calculated by dividing the energy content (in kilocalories) in consumed food by the weight of foods consumed at the farm. GHG emissions are calculated as emissions (N2O, CH4, and CO2) from all sources (green manure, residue applied in soil, flooding, atmospheric N fixation, symbiotic N fixation, etc.) and summed emissions expressed in CO2 equivalents from all sources. Labor input is calculated as the sum of farm labor (family labor, hired labor, and casual labor) required for crop, animal, and general management of the farm. Biocide use is calculated as the total amount of active ingredients applied (kg ha−1) on the farm.
Three alternatives to farmers’ dominant cropping sequences (rice–wheat–fallow) were used in the case study area: rice–wheat–mung bean (RWMb); maize–wheat–mung bean (MWMb); and maize–mustard–mung bean (MMuMb). The data generated from the on-station experiments at BISA farm, Pusa over 4 years were used as primary data for the study. These practices are known as climate-resilient cropping systems, which address the interconnected challenges of food security, accelerating climate change, and mitigation. These cropping systems also save input costs and water due to the no-tillage system, improve soil health due to residue retention and inclusion of mung bean (Choudhary et al., 2018), and minimize greenhouse gas emissions (Sapkota et al., 2014).
We performed an inventory of the cropping sequences at 43 farm households from 10 climate-smart villages (CSVs) in Bihar. The current practices of rice-based cultivation have substituted the cropping sequences by switching to rice–wheat–mung bean (RWMb) or maize–wheat–mung bean (MWMb). Similarly, maize-based cultivation has substituted the cropping sequences with maize–mustard–mung bean (MMuMb), regardless of other crops in the cropping sequences.
We investigated the relationships between the original cropping sequences and the alternative substitutes on the performance indicators. The correlation between original versus intensified sequences and trade-off analysis was done through plotting in the form of a scattered plot of all the performance indicators using Microsoft Office Excel 2013 version. To conduct cluster analysis and ANOVA, we used the R packages “fit lm model” (R version 3.5.2).
The performance indicators of the 229 original cropping systems cultivated within the 43 farms varied strongly in the eight performance indicators (Figure 1). This variability of cropping systems performance within the farm types resulted in the absence of significant differences between well-endowed (WE), part-time (PT), medium-scale (MS), small-scale (SS), and resource-poor (RP) farm types (Figure 2; Supplementary Table S1). Contributing individual cropping sequences on performance indicators to improve whole-farm performance, the average value of each indicator was assessed for each farm type (Figure 2). The five farms differed in terms of characteristics (land, cropping sequences, and household size) and performance indicators.
Figure 1. Relationship between performance indicators for individual cropping patterns of different farm types (closed symbols) and the three intensified cropping systems (open symbols). Indicator definitions: gross margin = crop gross margin (×1,000 INR ha−1); DE yield = dietary energy yield expressed in number of persons that can be sufficiently fed per ha; labour input = labour required for crop cultivation (h ha−1); OM input = organic matter added to the soil (kg ha−1); water use = amount of irrigation water applied (m3 ha−1); GHG emiss. = greenhouse gas emissions (Mg CO2-eq ha−1); biocide use = biocide active ingredients applied (kg ha−1); soil N loss = loss of nitrogen (N) from the cropping system (kg ha−1).
Gross margin (INR ha−1) was reported to be the highest for MS, followed by WE, PT, and RP farm types. The lowest profit was reported with the SS farm type. DE yield was reported to be the highest under WE farms, followed by RP, MS, PT, and SS farm types, respectively. The highest labor input was reported with WE farms, whereas the lowest was with PT farm types. Similarly, in the case of organic matter input, WE farm types showed the highest value, whereas PT farm types reported the lowest value. Regarding water use for irrigating the crop, WE farm types reported higher water use, whereas SS needed less water for their crops in their farms. The highest GHG emission was reported with the WE farm type, followed by SS, RP, MS, and PT, farm types, respectively. The highest biocide use was reported with PT farm types, whereas the lowest was with MS farm types. Higher N soil losses were reported with WE, followed by SS, RP, PT, and MS farm types, respectively (Figure 2; Supplementary Table S4).
Compared to the original cropping systems, the intensified cropping systems with mung bean performed well in terms of dietary energy production and organic matter inputs into the soil. However, they also had high application rates of biocides and nitrogen losses (Figure 1). The intervention systems (rice–wheat–mung bean; maize–mustard–mung bean, and maize–wheat–mung bean) were low in labor requirement and scored at an intermediate level for crop gross margin, water use, and GHG emissions.
The potential contribution of intensification of rice–wheat and maize-based cropping systems by adding mung bean and CA practices to the improvement of the performance indicators was evaluated for all potential farm types. The model-based assessment replaced ‘rice–wheat–fallow’ systems with ‘rice–wheat–mung bean’, and maize-based sequences with ‘maize–wheat–mung bean’ and ‘maize–mustard–mung bean’ cropping sequences.
The ranges of areas of maize- and rice-based systems replaced by intensified systems were the largest for the WE and RP farm types (Figure 3). This was reflected in large ranges of change in the performance indicators, but no significant differences in response were found between the farm types (Figure 4). The profit increased the most for the RP farm types, followed by SM, WE, MS, and PT farms. The DE yield increased the highest for the RP farm types, followed by WE farm types when intensified with alternative cropping sequences. Similarly, total labor inputs were minimized more for RP farm types than for WE farm types when intensified with an alternative crop sequence. The organic matter input increased the highest for the WE farm types and the lowest for RP farm types. Total water requirement increased highest for SS whereas minimum for WE farm types. In the case of GHG emissions, MS farm types emitted less GHG compared to other farms when intensified with alternative sequences. Lower biocide use was reported in PT farm types, whereas the highest use was in RP farm types, followed by SS, WE, and MS farm types, respectively. Higher N soil losses were reported for WE farm types whereas lower in PT farm types, whereas the rest of the farm showed moderate soil N losses when intensified with alternative cropping sequences (Figure 4; Supplementary Table S5).
Figure 3. Farm area and percentage of change in area to intensified rice- and maize- based cropping systems. (A) farm area; (B) area changed without intensification; (C) area changed with intensification.
Figure 4. (A–H) Change in performance indicators per farm type in response to intensification of rice- and maize-based cropping systems.
The intensification of maize- and rice-based systems with the proposed intervention cropping systems with mung bean would result in increased profitability and DE yield and lower labor and water requirements with an increasing proportion of the farm being converted (Figures 5A–C). However, also the use of biocides would increase, while the intervention cropping systems would have no significant effect on organic matter input, GHG emissions, and soil N losses (Figures 5D–H). Among biocides, the use of herbicides increases more significantly with the extensive adoption of intensification combined with CA-based practices compared to fungicides and insecticides as a proportion of farmland as a function to control the annual and perennial weeds. Average changes in farm performance, the alternative cropping system intensification would particularly increase DE yield (82%) and profitability (39%) and reduce labor requirement by 84%, while irrigation water use would decline by 28%, but biocide use would enhance by 44% and N losses by 21%. For the increase in DE yield and the reduction in labor requirement, significant differences were found between the farm types (Figures 4A,C, 5B,C): Both WE and RP farm types could achieve the largest increase in DE yield, whereas the labor reduction was stronger for RP farms that for farms of other types.
Figure 5. (A–H) Changes in performance indicators in relation to the percentage area of change to intensified rice- and maize-based cropping systems for different farm types. Colored solid lines represent different relationships per farm types, the dark blue lines indicate a significant effect of area change, and the grey dashed lines indicate a non-significant trend.
The results of this model-based study suggest that alternative cropping sequences with crop diversification and intensification in existing rice- and maize-based sequences could be beneficial for farm types differing in endowment levels. The intensification of maize- and rice-based systems with the proposed intervention cropping systems with mung bean and mustard would result in increased profitability and DE yield and lower labor and water requirements with an increasing proportion of the farm converted. However, also the use of biocides would increase, while the intervention cropping systems would have no significant effect on organic matter input, GHG emissions, and soil N losses. In the early stages of adopting conservation agriculture (CA) practices, the use of biocides, especially herbicides to knock down the existing weeds, increases due to the transition from conventional to no-tillage systems. However, in the long term, these usage are expected to decrease (Jat et al., 2019b). Furthermore, due to financial constraints, farmers in the EGP often find themselves unable to afford inputs, particularly herbicides and existing weeds controlled by tillage and later post-emergence weeds managed by family labor. As a result, there is a reduced reliance on biocides in their current agricultural practices. In particular, WE and RP farm types could enhance DE yield, while the largest labor reduction was achievable for RP farm types. Increasing the availability of land for intensified cropping sequences would help to increase soil organic matter and decrease soil erosion while maintaining or increasing farm profit (Jat et al., 2019a). This may be due to growing the other cropping sequence options such as cash crops available for the farmers in this region. Michalscheck et al. (2018) observed that higher resource-endowed farmers had larger space to improve their objective indicators because of more options available to improve their objective indicators.
Under maize–wheat sequences, the system yield significantly increased with the addition of third crop (mung bean) in the system (Parihar et al., 2017). CA-based rice-wheat cropping sequences had a positive effect on cropping system productivity when intensified with mung bean in the system (Jat et al., 2019b). Choudhary et al. (2018) and Kumar et al. (2018) reported that the integration of mung bean in the CA-based rice–wheat system resulted in higher system productivity compared to the conventional rice–wheat system. This might be because of improved soil physical, chemical, and biological properties when added mung bean (Gathala et al., 2016; Jat, 2017; Kumar et al., 2024). In the study area, farms are very diverse to grow other cash crops, instead of cereal crops. Samal et al. (2017) reported higher rice–wheat system grain yield under CA practices intensified with mung bean compared to farmer’s practices. Rashid et al. (2019) reported that the grain yields of maize were obtained higher under rice–maize–mung bean sequences in CA-based practices than in conventional tillage-based practices. This might be due to reduced input costs such as fuel and labor, coupled with higher system productivity resulting in higher gross margins. In addition, having crop residue on the soil surface can increase soil organic matter, and total N, available P, and available S contents of soil resulting in higher crop yields and farmer income. Aryal et al. (2018) assessed that CA-based climate-smart agriculture practices (CSAPs) have clear economic and climate change adaptation benefits for farmers in Bihar, India. However, the factors that determine the adoption of these practices include crop rotations, education, social and economic capital, farmland characteristics, access to the market, extension services, and training. Gora et al. (2022) offered that CA-based technologies like system intensification, residue retention, crop rotation, and no-tillage improve productivity, profitability, and resilience to climatic risks.
The study suggests that the intensified system showed improvement in all the objective indicators but not in all cropping systems and farm types. In each selected farm, there is a diversity of cropping sequences which has potential support to improving objective variables. The improvement in objective indicators is also determined by the socio-economic condition of a farmer, availability of inputs, and crop management practices and by external factors such as climate, soil, and market. Similarly, Sierra et al. (2017) revealed that variability of farming practices at the farm-type level had a strong impact on soil organic carbon changes, which ranged from net soil organic carbon losses to carbon (C) sequestration. Comparing the current farm performance with the sets of alternative options, well-performed farms in terms of N and labor balances, whereas the largest scope for improvement was found for increasing soil organic matter balances (Cortez-Arriola et al., 2016). Sutherland et al. (2019) demonstrated that emergent patterns of land management depend upon farm types, differentiated primarily by the scale of farm size, presence of diversification activities, and types of animals present. Gitz and Meybeck (2012) indicated that climatic shocks, plant disease and pests, reductions in output prices, and increases in input prices are the types of risks that impact agricultural production systems. Therefore, intensification and improved agronomy (CA-based management) as presented here should be entrenched in adaptive as well as practical for researchers and policymakers, which could target the farm types and cropping systems for better farm input use efficiencies and potential benefits.
In this study, we assessed the potential of integration of intensified cropping systems with mung bean to improve multiple objectives for five farm types, each representing a farm type in Samastipur, Bihar, India. We found large performance variations on objective values among farms, indicating heterogeneity in the farming community, leading to differentiated potential to improve the system’s performance. These insights on the alternative intensification options per farm type help decision-makers establish policies for this sector. They also assist stakeholders and farmers in defining priorities, identifying solutions, and implementing technical interventions for specific sets of farm types. The farm-scale model suggests that there is an option to improve the overall performance of current farms, including aspects such as overall farm profitability, soil organic matter accumulation, water saving, and improving environmental and nutrition outcomes among the community in the region. However, additional innovations may be needed to further manage the vulnerability and resilience of these systems in the face of increased input costs, deteriorating soil health, and potential climatic risks in this region. These findings could be highly beneficial for the Government of Bihar in directing interventions and prioritizing investments within the 4th Agricultural Road Map (2023–2028), which has an allocated budget of US$ 20 billion.
The raw data supporting the conclusions of this article will be made available by the authors, without undue reservation.
All experimental protocols were approved by the research committee CIMMYT. The informed consent was obtained from all subjects and/or their legal guardian(s).
DB: Data curation, Formal analysis, Methodology, Writing – original draft. JG: Formal analysis, Software, Writing – review & editing. MJ: Conceptualization, Funding acquisition, Investigation, Project administration, Resources, Validation, Writing – review & editing. RT: Data curation, Software, Visualization, Writing – review & editing. SR: Conceptualization, Investigation, Methodology, Supervision, Visualization, Writing – review & editing. KK: Data curation, Formal analysis, Project administration, Writing – review & editing. RJ: Investigation, Resources, Supervision, Visualization, Writing – review & editing. MG: Conceptualization, Funding acquisition, Investigation, Resources, Supervision, Validation, Writing – review & editing.
The author(s) declare that no financial support was received for the research, authorship, and/or publication of this article.
We acknowledged the financial and technical support from the Indian Council of Agricultural Research (ICAR)-CA (Window 3 support). We are thankful to Global and Regional One CGIAR Initiative on Sustainable Intensification—Mixed Farming Systems (https://www.cgiar.org/initiative/mixed-farming-systems/) and Transforming Agri-Food Systems in South Asia (TAFSSA: https://www.cgiar.org/initiative/20-transforming-agrifood-systems-in-south-asia-tafssa/). We are also thankful to the Borlaug Institute of South Asia for facilitating the data on the research experiment. Furthermore, we thanked the farmers for their time and for sharing their experiences and expertise and the referees for their useful comments that helped us to improve the manuscript.
The authors declare that the research was conducted in the absence of any commercial or financial relationships that could be construed as a potential conflict of interest.
All claims expressed in this article are solely those of the authors and do not necessarily represent those of their affiliated organizations, or those of the publisher, the editors and the reviewers. Any product that may be evaluated in this article, or claim that may be made by its manufacturer, is not guaranteed or endorsed by the publisher.
The Supplementary material for this article can be found online at: https://www.frontiersin.org/articles/10.3389/fsufs.2024.1393129/full#supplementary-material
Agriculture Census (2015–16). (2019) All India report on number and area of operational holdings. Available at: https://agcensus.da.gov.in/document/agcen1516/ac_1516_report_final-220221.pdf (Accessed May 2019).
Aryal, J. P., Jat, M. L., Sapkota, T. B., Khatri-Chhetri, A., Kassie, M., Rahut, D. B., et al. (2018). Adoption of multiple climate-smart agricultural practices in the Gangetic plains of Bihar, India. Int. J. Clim. Change Strateg. Manag. 10, 407–427. doi: 10.1108/IJCCSM-02-2017-0025
Choudhary, K. M., Jat, H. S., Nandal, D. P., Bishnoi, D. K., Sutaliya, J. M., Choudhary, M., et al. (2018). Evaluating alternatives to rice-wheat system in western indo-gangetic plains: crop yields, water productivity and economic profitability. Field Crop Res. 218, 1–10. doi: 10.1016/j.fcr.2017.12.023
Cortez-Arriola, J., Groot, J. C., Rossing, W. A., Scholberg, J. M., Massiotti, R. D., Tittonell, P., et al. (2016). Alternative options for sustainable intensification of smallholder dairy farms in north-West Michoacán, Mexico. Agric. Syst. 144, 22–32. doi: 10.1016/j.agsy.2016.02.001
Damerau, K., Davis, K. F., Godde, C., Herrero, M., Springmann, M., Bhupathiraju, S. N., et al. (2020). India has natural resource capacity to achieve nutrition security, reduce health risks and improve environmental sustainability. Nat. Food. 1, 631–639. doi: 10.1038/s43016-020-00157-w
Ditzler, L., Komarek, A. M., Chiang, T.-W., Alvarez, S., Chatterjee, S. A., Timler, C., et al. (2019). A model to examine farm household trade-offs and synergies with an application to smallholders in Vietnam. Agric. Syst. 173, 49–63. doi: 10.1016/j.agsy.2019.02.008
Dutta, S. K., Laing, A. M., Kumar, S., Gathala, M. K., Singh, A. K., Gaydon, D. S., et al. (2020). Improved water management practices improve cropping system profitability and smallholder farmers’ incomes. Agri. Water Manag. 242:106411. doi: 10.1016/j.agwat.2020.106411
Dutta, S. K., Laing, A., Kumar, S., Shambhavi, S., Kumar, S., Kumar, B., et al. (2023). Sustainability, productivity, profitability and nutritional diversity of six cropping systems under conservation agriculture: A long term study in eastern India. Agric. Syst. 203:103641. doi: 10.1016/j.agsy.2023.103641
Erenstein, O., and Thorpe, W. (2011). Livelihoods and agro-ecological gradients: a meso-level analysis in the indo-Gangetic plains, India. Agric. Syst. 104, 42–53. doi: 10.1016/j.agsy.2010.09.004
Gathala, M. K., Laing, A. M., Tiwari, T. P., Timsina, J., Islam, S., Bhattacharya, P. M., et al. (2020a). Energy-efficient, sustainable crop production practices benefit smallholder farmers and the environment across three countries in the eastern Gangetic Plains, South Asia. J. Clean. Prod. 246:118982. doi: 10.1016/j.jclepro.2019.118982
Gathala, M. K., Laing, A. M., Tiwari, T. P., Timsina, J., Islam, S., Chowdhury, A. K., et al. (2020b). Enabling smallholder farmers to sustainably improve their food, energy and water nexus while achieving environmental and economic benefits. Ren. and Sus. Ener. Reviews. 120:109645. doi: 10.1016/j.rser.2019.109645
Gathala, M. K., Laing, A. M., Tiwari, T. P., Timsina, J., Rola-Ruzben, F., Islam, S., et al. (2021). Improving smallholder farmers’ gross margins and labor-use efficiency across a range of cropping systems in the eastern Gangetic Plains. World Dev. 138:105266. doi: 10.1016/j.worlddev.2020.105266
Gathala, M. K., Mahdi, S. S., Jan, R., Wani, O. A., and Parthiban, M. (2022). “Sustainable intensification in eastern Gangetic Plains of South Asia via conservation agriculture for energy, water and food security under climate smart management system” in Secondary agriculture. eds. F. A. Bahar, M. Anwar Bhat, and S. S. Mahdi (Cham: Springer).
Gathala, M. K., Timsina, J., Islam, M. S., Krupnik, T. J., Bose, T. R., Islam, N., et al. (2016). Productivity, profitability, and energetics: a multi-criteria assessment of farmers’ tillage and crop establishment options for maize in intensively cultivated environments of South Asia. Field Crop Res. 186, 32–46. doi: 10.1016/j.fcr.2015.11.008
Gitz, V., and Meybeck, A. (2012). Risks, vulnerabilities, and resilience in a context of climate change. FAO/OECD workshop: Building resilience for adaptation to climate change in the agricultural sector, Rome, Italy, 23–24 April. Available at: http://typo3.fao.org/fileadmin/templates/agphome/documents/faooecd/gitz.pdf
Gora, M. K., Kumar, S., Jat, H. S., Kakraliya, S. K., Choudhary, M., Dhaka, A. K., et al. (2022). Scalable diversification options delivers sustainable and nutritious food in indo-Gangetic plains. Sci. Rep. 12:14371. doi: 10.1038/s41598-022-18156-1
Groot, J. C. J., Oomen, G. J. M., and Rossing, W. A. H. (2012). Multi-objective optimization and design of farming systems. Agric. Syst. 110, 63–77. doi: 10.1016/j.agsy.2012.03.012
Hoque, M. A., Gathala, M. K., Timsina, J., Ziauddin Md, A. T. M., Hossain, M., and Krupnik, T. J. (2013). Reduced tillage and crop diversification can improve productivity and profitability of rice-based rotations of the eastern Gangetic Plains. Field Crop Res. 291:10879. doi: 10.1016/j.fcr.2022.108791
Jat, M. L. (2017). “Climate smart agriculture in intensive cereal-based systems: scalable evidence from indo-Gangatic Plains” in Agriculture under climate change: Threats, strategies and policies. eds. V. V. Belavadi, N. Nataraja Karaba, and N. R. Gangadharappa (New Delhi: Allied Publishers Pvt Ltd), 147–154.
Jat, H. S., Datta, A., Choudhary, M., Sharma, P. C., and Jat, M. L. (2020). Conservation agriculture: factors and drivers of adoption and scalable innovative practices in indo-Gangetic plains of India. A review. Int. J. Agric Sustain. 19, 40–55. doi: 10.1080/14735903.2020.1817655
Jat, H. S., Kumar, P., Sutaliya, J. M., Kumar, S., Choudhary, M., Singh, Y., et al. (2019a). Conservation agriculture based sustainable intensification of basmati rice-wheat system in north-West India. Arch. Agro. Soil Sci. 65, 1370–1386. doi: 10.1080/03650340.2019.1566708
Jat, R. K., Sapkota, T. B., Singh, R. G., Jat, M. L., Kumar, M., and Gupta, R. K. (2014). Seven years of conservation agriculture in a rice–wheat rotation of eastern Gangetic Plains of South Asia: yield trends and economic profitability. Field Crop Res. 164, 199–210. doi: 10.1016/j.fcr.2014.04.015
Jat, R. K., Singh, R. G., Kumar, M., Jat, M. L., Parihar, C. M., Bijarniya, D., et al. (2019b). Ten years of conservation agriculture in a rice–maize rotation of eastern Gangetic Plains of India: yield trends, water productivity and economic profitability. Field Crop Res. 232, 1–10. doi: 10.1016/j.fcr.2018.12.004
Kumar, V., Jat, H. S., Sharma, P. C., Balwinder-Singh,, Gathala, M. K., Malik, R. K., et al. (2018). Can productivity and profitability be enhanced in intensively managed cereal systems while reducing the environmental footprint of production? Assessing sustainable intensification options in the breadbasket of India. Agric. Ecosy. Envn. 252, 132–147. doi: 10.1016/j.agee.2017.10.006
Kumar, K., Parihar, C. M., Sena, D. R., Godara, S., Patra, K., Sarkar, A., et al. (2024). Modelling the growth, yield and N dynamics of wheat for decoding the tillage and nitrogen nexus in 8-years long-term conservation agriculture-based maize-wheat system. Front. Sustain. Food Syst. 8:1321472. doi: 10.3389/fsufs.2024.1321472
Lopez-Ridaura, S., Frelat, R., van Wijk, M. T., Valbuena, D., Krupnik, T. J., and Jat, M. L. (2018). Climate smart agriculture, farm household typologies and food security. Agric. Syst. 159, 57–68. doi: 10.1016/j.agsy.2017.09.007
Michalscheck, M., Groot, J. C. J., Kotu, B., Hoeschle-Zeledon, I., Kuivanen, K., Descheemaeker, K., et al. (2018). Model results versus farmer realities. Operationalizing diversity within and among smallholder farm systems for a nuanced impact assessment of technology packages. Agric. Syst. 162, 164–178. doi: 10.1016/j.agsy.2018.01.028
Parihar, C. M., Jat, S. L., Singh, A. K., Ghosh, A., Rathore, N. S., Kumar, B., et al. (2017). Effects of precision conservation agriculture in a maize-wheat-mungbean rotation on crop yield, water-use and radiation conversion under a semiarid agro-ecosystem. Agric. Water Manag. 192, 306–319. doi: 10.1016/j.agwat.2017.07.021
Rakesh, S., Sarkar, D., Sinha, A. K., Shikha,, Mukhopadhyay, P., Danish, S., et al. (2021). Carbon mineralization rates and kinetics of surfaced applied and incorporated rice and maize residues in Entisol and Inceptisol soil types. Sustainability. 13:7212. doi: 10.3390/su13137212
Rashid, M., Timsina, J., Islam, N., and Islam, S. (2019). Tillage and residue management effects on productivity, profitability and soil properties of a rice-maize-Mungbean system in Bangladesh. J. Crop Improv. 33, 683–710 doi: 10.1080/15427528.2019.1661056
Rufino, M. C., Quiros, C., Boureima, M., Desta, S., Douxchamps, S., Herrero, M., et al. (2013). Developing generic tools for characterizing agricultural systems for climate and global change studies (IMPACTlite – phase 2). Report to CCAFS. Copenhagen, Denmark: CGIAR Research Program on Climate Change, Agriculture and Food Security (CCAFS).
Samal, S. K., Rao, K. K., Poonia, S. P., Kumar, R., Mishra, J. S., Prakash, V., et al. (2017). Evaluation of long-term conservation agriculture and crop intensification in rice-wheat rotation of indo-Gangetic Plains of South Asia: carbon dynamics and productivity. Eur. J. Agron. 90, 198–208. doi: 10.1016/j.eja.2017.08.006
Sapkota, T. B., Majumdar, K., Jat, M. L., Kumar, A., Bishnoi, D. K., McDonald, A. J., et al. (2014). Precision nutrient management in conservation agriculture-based wheat production of Northwest India: profitability: nutrient use efficiency and environmental footprint. Field Crop Res. 155, 233–244. doi: 10.1016/j.fcr.2013.09.001
Sapkota, T. B., Vetter, S. H., Jat, M. L., Sirohi, S., Paresh, B. S., Singh, R., et al. (2019). Cost-effective opportunities for climate change mitigation in Indian agriculture. Sci. Total Environ. 655, 1342–1354. doi: 10.1016/j.scitotenv.2018.11.225
Sierra, J., Causeret, F., and Chopin, P. A. (2017). Framework coupling farm typology and biophysical modelling to assess the impact of vegetable crop-based systems on soil carbon stocks. Application in the Caribbean. Agric. Sys. 153, 172–180. doi: 10.1016/j.agsy.2017.02.004
Singh, V. K., Dwivedi, B. S., Singh, S. K., Majumdar, K., Jat, M. L., Mishra, R. P., et al. (2016). Soil physical properties, yield trends and economics after five years of conservation agriculture-based rice-maize systemin North-Western India. Soil Tillage Res. 155, 133–148. doi: 10.1016/j.still.2015.08.001
Sutherland, L.-A., Barlagne, C., and Barnes, A. P. (2019). ‘Hobby farming’: towards a typology of non-commercial farming. Agric. Hum. Values 36, 475–493. doi: 10.1007/s10460-019-09930-5
Tittonell, P. (2014). Livelihood strategies, resilience and transformability in African agroecosystems. Agric. Syst. 126, 3–14. doi: 10.1016/j.agsy.2013.10.010
Yadav, G., Jat, H. S., Raju, R., Yadav, R. K., Singh, S. K., Chaudhari, S. K., et al. (2021). Enterprise mix diversification: an option for ecologically sustainable food and nutritional security of small holders in indo-Gangetic plains. Int. J. Agric. Sustain. 20, 1–11. doi: 10.1080/14735903.2021.1912978
Keywords: cropping system management, FarmDESIGN model, dietary energy yield, organic matter inputs, farm types, GHG emission
Citation: Bijarniya D, Groot JCJ, Jat ML, Toorop RA, Lopez-Ridaura S, Kalvania KC, Jat RK and Gathala MK (2024) Holistic analysis of cropping diversity and intensity implications for productive, environmental, and nutritional performance of smallholder farms in Bihar, India. Front. Sustain. Food Syst. 8:1393129. doi: 10.3389/fsufs.2024.1393129
Received: 28 February 2024; Accepted: 27 May 2024;
Published: 12 June 2024.
Edited by:
Alexandra Jacquelyn Burgess, University of Nottingham, United KingdomReviewed by:
Theodor Curt Achilles Friedrich, FAO, ItalyCopyright © 2024 Bijarniya, Groot, Jat, Toorop, Lopez-Ridaura, Kalvania, Jat and Gathala. This is an open-access article distributed under the terms of the Creative Commons Attribution License (CC BY). The use, distribution or reproduction in other forums is permitted, provided the original author(s) and the copyright owner(s) are credited and that the original publication in this journal is cited, in accordance with accepted academic practice. No use, distribution or reproduction is permitted which does not comply with these terms.
*Correspondence: Mahesh K. Gathala, bS5nYXRoYWxhQGNnaWFyLm9yZw==; Mangi L. Jat, bWFuZ2lsYWwuamF0QGljcmlzYXQub3Jn
Disclaimer: All claims expressed in this article are solely those of the authors and do not necessarily represent those of their affiliated organizations, or those of the publisher, the editors and the reviewers. Any product that may be evaluated in this article or claim that may be made by its manufacturer is not guaranteed or endorsed by the publisher.
Research integrity at Frontiers
Learn more about the work of our research integrity team to safeguard the quality of each article we publish.