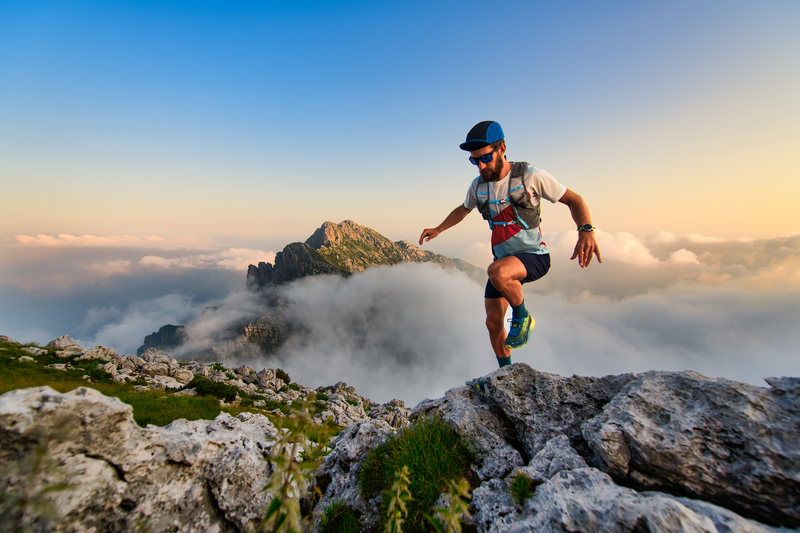
94% of researchers rate our articles as excellent or good
Learn more about the work of our research integrity team to safeguard the quality of each article we publish.
Find out more
ORIGINAL RESEARCH article
Front. Sustain. Food Syst. , 16 October 2024
Sec. Land, Livelihoods and Food Security
Volume 8 - 2024 | https://doi.org/10.3389/fsufs.2024.1391904
Introduction: Food insecurity remains a global issue and a top national priority in Ethiopia. However, it continues to pose a serious challenge in rural areas. Therefore, this study investigated household resilience to food insecurity among rural residents in Mekiet district.
Methods: A cross-sectional research design was used, focusing on two agroecological zones of Mekiet district—lowland and midland—involving 228 respondents. The study employed both quantitative and qualitative approaches. Multistage sampling techniques were employed to select the sample households, and factor analysis was employed to analyze the data.
Results and discussion: The results from the factor analysis using the TANGO approach showed that the latent variables of absorptive and adaptive capacities had factor loadings of 0.98 and 0.79, respectively. On the other hand, transformative capacity showed a negative loading (−0.78), with all variables being statistically significant. The mean household resilience capacity index was 0.51. The variables of absorptive and adaptive capacities were positively associated with household resilience capacity, while transformative capacity exhibited a strong negative association. All factor loadings were significant, exceeding the recommended absolute value of 0.364 for sample sizes of 200 or more. Additionally, these variables were also highly correlated with resilience capacity. The study recommends that resilience programs be implemented at district, community, and household levels to enhance resilience to food insecurity.
Food security emerged as a critical global issue in the mid-1970s, particularly at the World Food Conference of 1974, which was convened in response to the global food crisis (Clay, 2002). Since then, it has remained a central concern for global, national, and local communities and institutions. Achieving food security continues to be a challenge at all levels (WHO, 2019; FAO, 2020). A report by FAO, IFAD, UNICEF, WFP and WHO (2021) revealed that 768 million people faced hunger worldwide in 2020, an increase of 118 million from 2019. The report highlights that global hunger, malnutrition, and food insecurity are serious problems worldwide. In addition, more than one-third of the 282 million people in Africa experienced hunger. The report also indicates that the total number of undernourished people in sub-Saharan Africa continues to increase over time (FAO, 2020).
Ethiopia, as part of sub-Saharan Africa, is frequently cited for its persistent issues with chronic and transitory food insecurity (WHO, 2019; FAO, 2020). According to Mohamed (2017), Ethiopia’s food insecurity situation is highly linked to recurring food shortages and famine, which is often driven by recurrent droughts. More than 41% of the Ethiopian population lives below the poverty line, with over 31 million people suffering from undernutrition (Birhane et al., 2014; Welderufael, 2014; FAO, 2018).
Negash (2019) noted that the Amhara region is significantly affected by food insecurity in Ethiopia. Similarly, Kasie et al. (2017) reported that the Amhara region primarily suffers from recurrent droughts and chronic food insecurity.
Another study by Goshu (2016) highlighted that the Amhara regional state, the second most populous regional state after Oromia in Ethiopia, has the highest prevalence of food insecurity. His study focused on the determinants of rural food security in the North Wollo zone of Amhara Regional State. The North Wollo zone is categorized as food insecure. According to Aragie and Genanu (2017), average agricultural production in the North Wollo zone is sufficient for only 9 months of the year. The zone comprises 10 rural districts, with 8 of these districts, including Mekiet district (the study area), being the most drought-prone and food-insecure.
More recently, the concept of resilience to Food Insecurity has emerged and gained wider attention (Constas and Barrett, 2013; Frankenberger, 2015; FAO, 2016; Smith and Frankenberger, 2018; Atara et al., 2020; Beyene et al., 2023). This approach focuses on measuring the ability of systems, such as households, to absorb the negative impacts of unpredictable shocks, rather than merely predicting the occurrence of crises (Frankenberger and Nelson, 2013). Highlighting the growing interest in this concept, Constas and Barrett (2013) noted that the rapid and widespread adoption of resilience as a framework underscores its recognition as a key solution to poverty and food insecurity. Resilience enhances a household’s capacity to maintain a certain level of well-being, such as food security, by withstanding shocks and stressors (Alinovi et al., 2010; FAO, 2014; Atara et al., 2020).
Thus, to investigate and measure household resilience to food insecurity, two widely recommended approaches are the Resilience Index Measurement and Analysis (RIMA) (FAO, 2014, 2016) and the Technical Assistance to Non-Governmental Organizations (TANGO) measurement approaches (Frankenberger, 2015; Smith and Frankenberger, 2018).
However, several empirical studies assessing household resilience to food insecurity in Ethiopia have primarily used the RIMA approach. For instance, Demeke and Tefera (2013) identified the determinants of sustainable resilience for food and nutrition security among rural farming households based on the ERHS using the RIMA approach. Similarly, Guyu and Muluneh (2015) showed household resilience to seasonal food insecurity in northwestern Ethiopia, estimating household resilience as a latent variable through the RIMA approach. More recently, studies by Atara et al. (2020) and Beyene et al. (2023) also used the RIMA approach to assess the resilience of rural households to food insecurity in Southern Ethiopia. Given the focus of previous studies on the RIMA approach, this study addresses a gap by investigating rural household resilience to food insecurity using the other recommended TANGO measurement approach.
Furthermore, TANGO International developed a significantly modified variation on RIMA, first with a program evaluation in Ethiopia by Frankenberger (2015) and subsequently in Bangladesh (Smith and Frankenberger, 2018).
The TANGO method focuses on household- and community-level capacities: absorptive, adaptive, and transformative, which are hypothesized to promote resilience.
Constas et al. (2014) define resilience as the capacity to ensure that adverse stressors and shocks do not lead to long-lasting negative developmental consequences. Since 2018, the TANGO approach has been the recommended framework for resilience analysis in USAID-funded projects (Henly-Shepard and Sagara, 2018). The TANGO resilience survey module is now systematically collected to evaluate resilience investments by USAID. Therefore, this study aimed to investigate rural household resilience to food insecurity using the TANGO approach in Mekiet district.
The study was conducted in Mekiet district, North Wollo zone, Amhara National Regional State, Northeast Ethiopia. This district is situated 656 km from the capital city, Addis Ababa, and 159 km from the regional city, Bahir Dar. The district is bordered to the south by the Dawunt and Wadila districts, to the west by the Lay Gayint district of the South Gondar zone, to the north by the Lasta and Bugna districts, and to the east by the Gazo district (Negussie, 2019). As shown in Figure 1, the district is located between 11°35‟50‟ N -12°20‟30‟ N north latitudes and 38°32‟35‟ E - 39°16‟40‟ E east longitudes. The agroecological zones of the study area are lowlands (27%), midlands (63%), and highlands.
The estimated average annual rainfall ranges from 600 mm in the lowlands to 1,120 mm in the highlands, whereas the area’s mean maximum and minimum temperatures are approximately 24.6°C and 12.1°C, respectively. The main source of livelihood for the local community is small-scale rain agriculture (MWADO, 2024).
This study employed a cross-sectional research design, in which data were gathered from households only once. The study employed a household survey as its primary research strategy, using a predominantly quantitative approach, complemented by a qualitative approach.
Multistage sampling was used to identify the study area and the sample households. Mekiet district was purposefully selected due to its status as a top drought-prone area, with more than half of its population being chronically food insecure and highly exposed to recurrent droughts. Second, the district was stratified based on agroecological zones (highlands, midlands, and lowlands). Then, in each agroecological zone, kebeles were selected using simple random sampling. However, in the study sample, kebeles were selected from two agroecological zones: midlands and lowlands. Two agroclimates were purposefully selected. As a result, a large portion of the population lives in the two agroecological zones: midland (63%) and lowland (27%). Additionally, the two agroecological zones (midlands and lowlands) have a high frequency of drought occurrence, a level of food insecurity, and a higher number of safety net program beneficiaries than highlands (MWADO, 2024). Moreover, in the two agroecological zones, there is low access to basic services such as water, distance to market, distance from the district town, education, and health services, and there is a lack of infrastructure (including roads or electricity). Proportional sampling was then applied to ensure fair distribution of kebeles and households across agroecological zones. Three sample kebeles were selected from the midland (Berekeza and Aymate) and lowland (Debrekerbie).
The minimum sample size for this study was calculated using the mathematical formula suggested by Cochran (1977). The sample size was determined by considering a 95% confidence level, a desired level of precision (z = 1.96), and an estimated population proportion (p) of 10% (reflecting the 10% of the population that understands household resilience (Naing et al., 2006)). The formula used to calculate and determine the sample size is as follows:
where n = is the desired sample size, z = the desired confidence level, p = the estimated proportion of the population to be included in the sample, q = 1-p, and d is the desired level of precision or level of statistical significance or margin of error.
This calculation assumes a 5% precision level (e), commonly used in social science research, with a 95% confidence level (z = 1.96) and an estimated population proportion (p) of 10% [reflecting the 10% of the population knowledgeable about household resilience (Naing et al., 2006)]. This Cochran sample size determination formula is valid if the calculated sample size is smaller than or equal to 5% of the target population of the study (n/N ≤ 0.05).
Fortunately, the calculated sample size of this study was <5% of the study population. However, household surveys often have non-sampling (non-response) errors, mainly due to poorly defined variables, respondent bias or failure to respond, coverage errors, and researcher compiling errors. To mitigate these issues, it is recommended to oversample by 10 to 20% of the computed sample size (Naing et al., 2006). Therefore, considering a design effect of 1.5 due to the multistage sampling technique and a 10% non-response rate, the total sample size was adjusted to [(138 + 10%) × (1.5) = 228].
Thus, 228 sample household respondents were selected using a systematic random sampling technique, with the probability proportional to the size of each kebeles, applying appropriate sampling intervals (k).
There are two main types of data sources that a study can collect: primary and secondary. This study mainly utilized primary data collected from selected rural households in the study area. As this study focused on household-level resilience to food insecurity, the household was the unit of analysis. As the unit of analysis for the study is rural households, the study utilized a structured interview schedule method for data collection, in addition to utilizing key informants and conducting focus group discussions. Thus, nine key informants were interviewed, and three focus groups were interviewed from each kebeles. The number of focus group participants in one group for each kebeles was 6–10 members.
Secondary data were collected from the Agricultural Office of Mekiet district. From this office, information related to the total number of households in the district, the total number of households residing in the rural area, and general information about the infrastructure and access to social services in the district were collected. Furthermore, information related to assistance provided to poor rural households, such as social safety nets and emergency aid, was collected.
To conduct the study, the TANGO International approach was developed as a significantly modified variation in resilience index measurement analysis, first with a program evaluation in Ethiopia (Frankenberger, 2015) and subsequently in Bangladesh (Smith and Frankenberger, 2018). Since 2018, the TANGO approach has served as the basis for recommended resilience analysis under USAID-funded projects (Henly-Shepard and Sagara 2018), and the associated TANGO resilience survey module is now systematically used to evaluate resilience investments by USAID. The TANGO approach focuses on the household- and community-level capacities across three resilience capacity dimensions: absorptive, adaptive, and transformative. These dimensions are hypothesized to promote resilience. Each of these resilience capacity dimensions was estimated using observable household-level variables.
The overall household resilience capacity and its TANGO dimensions were estimated using factor analysis. First, the dimensions of resilience capacity—absorptive, adaptive, and transformative—were estimated using factor analysis (FA) of the observed variables. The procedure allows for the reduction of the set of variables used as proxy indicators for the latent variable—the dimension of interest—as a single variable. Second, the overall resilience capacity index was constructed by combining the three resilience capacity dimensions using factor analysis. Factor analysis was used to estimate a construct that was not directly observed, that is, the dimensions of the resilience capacity (Bollen, 2002).
According to Bollen (2002), the basic idea of a latent variable approach is that “there are one or more latent variables that create the association between unobserved variables.” The formal expression of this idea is as follows:
where; = observed indicator for the ith case.
= intercept term.
= factor loading for the 1st through k case.
= unique variable or error term.
To calculate an index for the latent variable, the factor analysis identifies one or more common factors that linearly reconstruct the observed variables by predicting their correlation matrix. It then calculates the factor loadings for multiple common factors. These loadings are used to identify the common factor that appeared to represent the concept being measured. Such identification takes place by examining the signs and magnitudes of the loadings. Identification is achieved by examining the signs and magnitudes of the loadings. The overall resilience capacity index is calculated as follows:
where = resilience capacity index, = absorptive capacity, = adaptive capacity, =Transformative capacity, and = is the factor analysis coefficient estimated using inter-correlations among the three resilience capacity dimensions. They are interpreted as weights assigned to the capacities in the estimation of an overall resilience capacity index, with greater weights assigned to capacities that correlate more highly with the overall index. The final factor indexes are re-scaled from 0 to 100 using a mini-max procedure according to the following expression, where Min is the minimum value of the estimated index and Max is its maximum value.
The statistical requirement for the appropriateness of a good factor analysis model and its family model was checked based on the Kaiser Meyer Olkin (KMO) measures of sampling adequacy, Bartlett’s test of sphericity, the existence of multicollinearity or singularity, and factor loadings (DiStefano et al., 2009; Odum, 2011; Field, 2009; Yong and Pearce, 2013; Kaiser, 1960).
To organize the data for analysis, all quantitative datasets were coded and entered into SPSS-version 25 (due to its quality for data management) and exported into STATA version 14.1 (due to its quality for executing and using statistical models). Additionally, data from focus group discussions and key informant interviews were analyzed concurrently and thematically with the quantitative results.
The TANGO approach was constructed from the three resilience capacity dimensions of absorptive, adaptive, and transformative capacities. For this purpose, the TANGO approach adopted a factor analysis (FA) model based on observable variables to estimate the three resilience capacity dimensions and overall household resilience capacity. Therefore, each dimension of resilience capacity, namely absorptive capacity, adaptive capacity, and transformative capacity, was estimated specifically as follows.
Absorptive capacity is a latent variable that refers to the ability to minimize exposure to shocks and stresses and to recover quickly when they occur. Strengthening absorptive capacity at the community and household levels helps reduce disaster risk and enables households to absorb the impacts of shocks without experiencing permanent negative impacts on their long-term food security. Absorptive capacity consists of five observed variables: bonding social capital, asset ownership, cash savings, access to informal safety nets, and disaster preparedness and mitigation. Before estimating absorptive capacity, some of these variables were indexed and estimated through factor analysis. Finally, absorptive capacity was estimated using the observed variables.
In the estimations of absorptive capacity, two factors were retained because their eigenvalues were greater than one. The first and second factors obtained 34 and 21% of the variation. These variables explain 55% of the total variation (see Supplementary Appendix Table A1). As presented in Table 1, all statistical requirements for a good factor analysis model were tested and satisfied, as recommended by the Kaiser rule. Moreover, all five variables were found to be statistically significant, with an absolute value of factor loadings greater than 0.364. As expected, the factor loadings of all variables except the asset ownership index, disaster preparedness, and mitigation were positive and high. The same study by Sunday et al. (2021) shows that access to informal safety nets is the most important factor contributing to absorptive capacity in rural households in Uganda.
Therefore, the relative size of the factor loading for each variable has important policy implications (i.e., the higher the loading, the more important it is, and the more policy attention should be placed on it). Generally, as suggested by the statistical criteria mentioned above, factor scores can be used to estimate the ABC variable. As a result, two factors were used to estimate ABC, as their contribution was adequate. This is estimated as follows:
The value obtained from this equation was used to estimate the RCI using the TANGO approach.
Adaptive capacity is one of the latent variables of resilience capacity in the TANGO approach, which involves making positive and informed choices regarding alternative livelihood strategies. The adaptive capacity in the TANGO approach involves bridging social capital, linking social capital, livelihood diversity, human capital, and access to information. Consequently, before approximating the adaptive capacity, some of the variables were indexed and estimated using factor analysis. Finally, adaptive capacity was estimated through the aggregate indices of all five variables based on the appropriate models.
The result specifies that using factor analysis, two components were extracted as both appeared significant, based on Kaiser’s rule, in terms of explaining variations within the data (Supplementary Appendix Table A2). As shown in Table 2, the relevant statistical requirements for the good-factor model were also checked and satisfied. All five variables were found to be statistically significant, with an absolute value of loadings greater than 0.364 in terms of contribution to adaptive capacity.
Given the nature of the variables, the signs of loadings were also expected. For instance, adaptive capacity is a proxy, with five variables having a strong and positive loading, except for the human capital index. The human capital index has negative loadings. Hence, the negative loading of the variable indicates that adaptive capacity declines as the household head’s literacy status becomes poorer. Linking social capital and accessing information has higher factor loads in adaptive capacity. A similar study by Sunday et al. (2021) found that adaptive capacity, linking social capital, and access to information are the most important factors for increasing household resilience to food insecurity in Uganda.
Subsequently, the components produced were meaningful in terms of the share of total variances explained, and both were considered as the underlying latent variable for AC. Hence, the component scores obtained using the Bartlett scoring method were combined to estimate the latent variable of (AC). This is accomplished by using the relative variance explained by each component as a weight. Hence, the AC index for each household is the weighted sum of its score for each of the two components:
The result obtained from this equation was used to construct the RCI using the TANGO approach.
Transformative capacity is the third latent variable of resilience capacity in the TANGO approach, which enables conditions that foster lasting resilience. Transformative capacities were constructed from access to formal safety nets, markets, extension services, responsibility governance, public services, and communal natural resources. Therefore, before approximating the transformative capacity, some of the variables were indices estimated through factor analysis. Finally, the transformative capacity was estimated based on the observed variables.
In the estimation of transformative capacity, three factors were retained based on their eigenvalues, each greater than one. Together, these variables explain 60.97% of the total variation (Supplementary Appendix Table A3). As presented in Table 3, all statistical requirements for a valid factor analysis model were tested and met, which is in line with the Kaiser rule. Moreover, all six variables were found to be statistically significant, with factor loadings having absolute values greater than 0.364.
As expected, the factor loadings for all variables, except access to markets and extension services, were both positive and high, indicating their significant contribution to estimating transformative capacity. Similarly, a study conducted by Asmamaw et al. (2019) showed that transformative capacity variables play a significant role in determining household resilience capacities.
The combined factor score of the three factors was used to estimate the latent variables for transformative capacity. This was accomplished by using the relative variance explained by each factor as a weight. The transformative capacity for each household was then calculated as the weighted sum of its score across the three factors. Therefore, the transformative capacity was estimated as follows:
The result obtained from this equation was further used to estimate the resilience capacity index using the TANGO approach.
Furthermore, this finding is in line with the ideas of focus group discussants. They stated that the issue of food insecurity was highly interconnected with social and public services such as road transport, extension services, health services, and electricity. In particular, during the summer season, it was not easy to move from home to the market center because the market was located far from the kebeles. Additionally, there was limited access to availability of health services, provision of livelihood activities (crop and livestock), and performing any day-to-day activities. Furthermore, households have faced problems selling and buying agricultural products for household consumption purposes at the right time. Thus, governments and local actors should address these problems by improving social services. If there are better social services in our kebeles, each household is doing and practicing any trade activities with the main source of income for agriculture, and the households can resist different shocks such as poverty and food insecurity.
The TANGO approach employs factor analysis in its second stage to estimate the overall resilience capacity index based on the results from the three resilience capacity dimensions. All statistical requirements for the goodness-of-fit of the factor analysis model were tested, and all passed the tests. Bartlett’s test of sphericity was significant (chi-square = 16.137, df = 3, p < 0.001), indicating sufficient correlation among variables to justify running the factor analysis. The KMO test of sampling adequacy was 0.500, and the determinant of the R-matrix was 0.900.
Following the Kaiser rule, two factors were retained, as their eigenvalues were greater than or equal to one. These two factors accounted for approximately 75.5% of the total variance (Supplementary Appendix Table A4). Both statistical measurements were well above the minimum required thresholds, confirming that issues such as insufficient sample size and multicollinearity are not of concern.
As presented in Table 4, absorptive capacity has a positive and high factor loading from the three latent variables and has a strong correlation with the resilience capacity index. The factor loading was 0.979, which was greater than 0.364, and the correlation coefficient was 0.127, which was significant at 0.01. This result is similar to that of Martin (2019), one of the latent variables of RCI, which had the highest factor loadings and the largest contribution to household resilience. In addition, adaptive capacities have a positive and higher factor loading, which is 0.797, and have a higher correlation coefficient with a resilience capacity index of 0.778, suggesting that these two latent variables contribute significantly to the estimation of the household resilience capacity. Similarly, a study conducted by Villoslada (2019) found that adaptive capacity has higher factor loadings and contributes to household resilience. Moreover, d’Errico et al.’s (2018) results from factor analysis show that adaptive capacity contributes the most to building resilience capacity among households in Uganda. On the other hand, transformative capacities have strong but negative factor loadings that are significant at (−780) because their absolute value is greater than the minimum required. Moreover, the implications of negative load indicated that the households did not have more access to the market, agricultural extension services, social services (road, health, school, water, and electricity), communal natural resources, and access to formal safety nets (productive safety net program and emergency aid). As a result, these households lacked the capacity to be resilient against food insecurity. The extreme negative value of the result shows the most vulnerable households, whereas the positive value shows resilient ones.
It is also possible to show the relationships between the overall household resilience capacity index and the three latent variables (absorptive, adaptive, and transformative capacities) of the TANGO approach, using two-way scatter plots. Scatter plots show the relationship between the overall RCI and the latent variables. The line drawn in a scatter plot, which is close to almost all points in the plot, is the line of best fit. If the variables are correlated, the points fall along a line or a curve. The better the correlation is, the closer the points are to the line. Hence, as shown in Figure 2, the scatter plots of resilience capacity with latent variables in the TANGO approach, adaptive capacity, and absorptive capacity have a positive correlation, while transformative capacity has a negative relationship.
In general, the overall results of the resilience capacity index from the stage two factor analysis suggest that household resilience is strongly dependent on absorptive and adaptive capacities. Specifically, variables such as the bonding social capital index, asset ownership index, cash savings, access to informal safety nets, and disaster preparedness and mitigation index from the absorptive, along with the bridging social capital index, linking social capital, livelihood diversity index, and access to information index from adaptive capacities of resilience, play a significant role in strengthening household resilience capacities.
These findings align with the study by Sunday et al. (2021) in Uganda, which also showed that absorptive and adaptive capacities significantly contribute to household resilience capacity. Therefore, resilience-oriented interventions should prioritize these variables to enhance the resilience capacity of households.
This study was conducted in Mekiet district, North Wollo Zone, Amhara National Regional State, Ethiopia. This study applied the technical assistance to non-governmental organization (TANGO) method to assess households’ ability to withstand food insecurity, using a factor analysis model. The study focused on three latent variables: absorptive capacity, adaptive capacity, and transformative capacity. The findings revealed that, while transformative capacity exhibited a strong negative loading (−0.78), absorptive and adaptive capacities showed positive values of 0.98 and 0.79, respectively. All factor loadings were statistically significant, with absolute values exceeding 0.364, which is recommended for sample sizes of 200 or more. Additionally, resilience capacity was found to have a strong correlation with these latent variables, highlighting the importance of absorptive and adaptive capacities in enabling households to withstand food insecurity. The study highlights the need for resilience programs to be implemented at district, community, and household levels to enhance the ability to cope with food insecurity.
Concerning TANGO’s measures of household resilience, the ability of households to withstand future food insecurity shocks and stressors is maintained. Based on this fact, the following recommendations were made:
• In TANGO’s framework, one of the latent variables, transformative capacity, showed negative factor loadings. The extreme negative value indicates that the most vulnerable households are at higher risk. Therefore, resilience policies should prioritize programs that enhance access to formal safety nets, markets, extension services, governance responsibility, public services (such as road transport, extension, market, credit, health services, and electricity), and communal natural resources.
• Resilience policies and programs should be implemented at the district, neighborhood, and household levels to enhance households’ capacity to withstand and recover from adverse events.
• This study employed a cross-sectional methodology to assess households’ ability to withstand and recover from food insecurity at a particular point in time. As a result, it is recommended that future research use longitudinal datasets to investigate fluctuations in household resilience over time in response to food insecurity.
The original contributions presented in the study are included in the article/Supplementary materials, further inquiries can be directed to the corresponding author.
The studies involving humans were approved by the Colette Browning, a health psychologist and social gerontologist with a BSc (Hons) and MSc in psychology and a PhD in public health. She is the Professor of Health Research at Federation University, Australia, Research Director of the International Primary Health Care Research Institute, Shenzhen, China, and an Honorary Professor at ANU, Australia. The studies were conducted in accordance with the local legislation and institutional requirements. Written informed consent for participation was not required from the participants or the participants’ legal guardians/next of kin because the title is published under public health.
TD: Conceptualization, Data curation, Formal analysis, Funding acquisition, Investigation, Methodology, Project administration, Resources, Software, Supervision, Validation, Visualization, Writing – original draft, Writing – review & editing. BD: Conceptualization, Data curation, Formal analysis, Funding acquisition, Investigation, Methodology, Project administration, Resources, Software, Supervision, Validation, Visualization, Writing – review & editing.
The author(s) declare that no financial support was received for the research, authorship, and/or publication of this article.
The authors are very grateful to the enumerators who collected and assisted in the data entry sample households and staff of Berekeza, Aymate, and Debrekerbie Kebelles for kindly providing the necessary information used in the study and for their willingness and patience in responding to the questionnaire at the expense of their invaluable time.
The authors declare that the research was conducted in the absence of any commercial or financial relationships that could be construed as a potential conflict of interest.
All claims expressed in this article are solely those of the authors and do not necessarily represent those of their affiliated organizations, or those of the publisher, the editors and the reviewers. Any product that may be evaluated in this article, or claim that may be made by its manufacturer, is not guaranteed or endorsed by the publisher.
The Supplementary material for this article can be found online at: https://www.frontiersin.org/articles/10.3389/fsufs.2024.1391904/full#supplementary-material
Alinovi, L., D’errico, M., Mane, E., and Romano, D. (2010). Livelihoods strategies and household resilience to food insecurity: an empirical analysis to Kenya. Eur. Rep. Dev., 1–52. Available at: http://erd.eui.eu/media/2010/Romano%20et%20al_Kenya%20paper%20r15.pdf
Aragie, T., and Genanu, S. (2017). Level and determinants of food security in north wollo zone (Amhara region–Ethiopia). J. Food Secur. 5, 232–247. doi: 10.12691/jfs-5-6-4
Asmamaw, M., Mereta, S. T., and Ambelu, A. (2019). Exploring households’ resilience to climate change-induced shocks using climate resilience index in Dinki watershed, central highlands of Ethiopia. PLoS One 14:e0219393. doi: 10.1371/journal.pone.0219393
Atara, A., Tolossa, D., and Denu, B. (2020). Analysis of rural households’ resilience to food insecurity: does livelihood systems/choice/matter? The case of Boricha woreda of sidama zone in southern Ethiopia. Environ. Dev. 35:100530. doi: 10.1016/j.envdev.2020.100530
Beyene, F., Senapathy, M., Bojago, E., and Tadiwos, T. (2023). Rural household resilience to food insecurity and its determinants: Damot Pulasa district, southern Ethiopia. J. Agricul. Food Res. 11:100500. doi: 10.1016/j.jafr.2023.100500
Birhane, T., Shiferaw, S., Hagos, S., and Mohindra, K. S. (2014). Urban food insecurity in the context of high food prices: a community based cross sectional study in Addis Ababa, Ethiopia. BMC Public Health 14, 1–8. doi: 10.1186/1471-2458-14-680
Bollen, K. A. (2002). Latent variables in psychology and the social sciences. Annu. Rev. Psychol. 53, 605–634. doi: 10.1146/annurev.psych.53.100901.135239
Clay, E. (2002). Food security: Concepts and measurement, paper for FAO expert consultation on trade and food security: Conceptualising the linkages Rome, 11–12 July 2002. Available at: https://www.researchgate.net/publication/285667097_Food_security_concepts_and_measurement_trade_reforms_and_food_security_conceptualizing_the_linkages_Rome
Constas, M., and Barrett, C. (2013). “Principles of resilience measurement for food insecurity: metrics, mechanisms, and implementation plans” in Expert consultation on resilience measurement related to food security (Rome: Food and Agricultural Organization and World Food Program).
Constas, M., Frankenberger, T., and Hoddinott, J. (2014). Resilience measurement principles: Toward an agenda for measurement design. Food Security Information Network, Resilience Measurement Technical Working Group, Technical Series, 1. Available at: https://www.researchgate.net/publication/282575446_A_common_analytical_model_for_resilience_measurement
d’Errico, M., Romano, D., and Pietrelli, R. (2018). Household resilience to food insecurity: evidence from Tanzania and Uganda. Food Secur. 10, 1033–1054. doi: 10.1007/s12571-018-0820-5
Demeke, M., and Tefera, N. (2013). Sustainable resilience for food and nutrition security: The case of farming rural households in Ethiopia. Rome, Italy: Agricultural Development and Economics Division (ESA), Food and Agricultural Organization (FAO), 1–32.
DiStefano, C., Zhu, M., and Mindrila, D. (2009). Understanding and using factor scores: considerations for the applied researcher. Pract. Assess. Res. Eval. 14:20. doi: 10.7275/da8t-4g52
FAO (2014). Resilience index measurement and analysis (RIMA) model. Rome, Italy: Food and Agriculture Organization of the United Nations.
FAO (2016). RIMA-II,Resilience index measurement and analysis—II. Food and agriculture Organization of the United Nations. Rome, Italy.
FAO (2018). The impact of disasters and crises on agriculture and food security. Report. Available at: https://openknowledge.fao.org/handle/20.500.14283/i8656en
FAO (2020). The state of food security and nutrition in the world (SOFI) Rome, Italy : FAO, 320. Available at: https://openknowledge.fao.org/handle/20.500.14283/ca9692en
FAO, IFAD, UNICEF, WFP and WHO (2021). The state of food security and nutrition in the world: Building resilience for peace and food security. doi: 10.4060/cb4474en
Field, A. (2009). Discopering statistics using SPSS, thrid edition. Available at: https://www.google.com.et/books/edition/Discovering_Statistics_Using_SPSS/a6FLF1YOqtsC?hl=en
Frankenberger, T. (2015). Ethiopia pastoralist areas resilience improvement and market expansion (PRIME) project impact evaluation report of the interim monitoring. Westat, Rockville, MD: Technical report.
Frankenberger, T., and Nelson, S. (2013). “Background paper for the expert consultation on resilience measurement for food security” in TANGO international–expert consultation on resilience measurement related to food security, sponsored by the food and agricultural organization and world food Programme (Rome, Italy). Available at: https://www.researchgate.net/publication/372572410_Background_Paper_for_the_Expert_Consultation_on_Resilience_Measurement_for_Food_Security
Goshu, M. T. (2016). Determinants of rural food security and child Nutrition. India: Aligarh Muslim University Aligarh.
Guyu, D. F., and Muluneh, W.-T. (2015). Wild foods (plants and animals) in the green famine belt of Ethiopia: do they contribute to household resilience to seasonal food insecurity? For. Ecosyst. 2, 1–12. doi: 10.1186/s40663-015-0058-z
Henly-Shepard, S., and Sagara, B. (2018). “Resilience measurement practical guidance note series: an overview” in Produced by Mercy Corps as part of the resilience evaluation (Analysis and Learning (REAL) Associate Award). Available at: https://www.resiliencelinks.org/system/files/download-count/documents/2019-08/gn_overview_final_0.pdf
Kaiser, H. F. (1960). The application of electronic computers to factor analysis. Educ. Psychol. Meas. 20, 141–151. doi: 10.1177/001316446002000116
Kasie, T., Agrandio, A., Adgo, E., and Garcia, I. (2017). Household resilience to food insecurity: Shock exposure, livelihood strategies and risk response options: The case of tach-Gayint District, Amhara region. Ethiopia: University of Jaume I.
Martin, S. (2019). Somalia resilience recurrent monitoring survey (RMS) report. Washington, DC: The Resilience Evaluation, Analysis and Learning (REAL) Associate Award. Available at: https://www.resiliencelinks.org/resources/reports/somalia-resilience-recurrent-monitoring-survey-rms-report-0
Mohamed, A. A. (2017). Food security situation in Ethiopia: a review study. Int. J. Health Econ. Policy 2, 86–96. doi: 10.11648/j.hep.20170203.11
MWADO (2024). Mekiet Woreda Adminstration office: Descriptions of the study district, Mekiet, North Wollo. Ethiopia: Amhara.
Naing, L., Winn, T., and Rusli, B. (2006). Practical issues in calculating the sample size for prevalence studies. Arch. Orofac. Sci. 1, 9–14.
Negussie, S. (2019). Adoption of bread wheat technology packages: A case of Meket District in north Wollo zone of Amhara region. Ethiopia. Available at: https://www.researchgate.net/publication/350787700_Adoption_of_Bread_Wheat_Technology_Packages_in_North_Wollo_Zone_of_Amhara_Region_Ethiopia_The_Case_of_Meket_District
Odum, M. (2011). Factor scores, Structure and Communality Coefficients: A Primer. Available at: https://files.eric.ed.gov/fulltext/ED516588.pdf
Smith, L. C., and Frankenberger, T. R. (2018). Does resilience capacity reduce the negative impact of shocks on household food security? Evidence from the 2014 floods in northern Bangladesh. World Dev. 102, 358–376. doi: 10.1016/j.worlddev.2017.07.003
Sunday, N., Kahunde, R., Atwine, B., Adelaja, A., and Kappiaruparampil, J. (2021). How specific resilience pillars mitigate the impact of drought on food security: Evidence from Uganda 15, 111–131. doi: 10.1007/s12571-022-01313-9
Villoslada, S. C. (2019). Development resilience: A comparison of popular measurement methods : Cornell University. doi: 10.7298/s04f-w487
Welderufael, M. (2014). Determinants of households vulnerability to food insecurity in Ethiopia: econometric analysis of rural and urban households. J. Econ. Sustain. Dev. 5, 70–79. Available at: https://www.researchgate.net/publication/336613599_Determinants_of_Households_Vulnerability_to_Food_Insecurity_in_Ethiopia_Econometric_analysis_of_Rural_and_Urban_Households
WHO (2019). The state of food security and nutrition in the world 2019: Safeguarding against economic slowdowns and downturns : Food & Agriculture Org.
Keywords: resilience, food insecurity, rural household, Mekiet, Ethiopia
Citation: Dessie TA and Demsie BS (2024) Rural household resilience to food insecurity in Mekiet district, Ethiopia. Front. Sustain. Food Syst. 8:1391904. doi: 10.3389/fsufs.2024.1391904
Received: 23 April 2024; Accepted: 26 September 2024;
Published: 16 October 2024.
Edited by:
Valentin Marian Antohi, Dunarea de Jos University, RomaniaReviewed by:
Adrian Turek, University of Agronomic Sciences and Veterinary Medicine, RomaniaCopyright © 2024 Dessie and Demsie. This is an open-access article distributed under the terms of the Creative Commons Attribution License (CC BY). The use, distribution or reproduction in other forums is permitted, provided the original author(s) and the copyright owner(s) are credited and that the original publication in this journal is cited, in accordance with accepted academic practice. No use, distribution or reproduction is permitted which does not comply with these terms.
*Correspondence: Tadsual Asfaw Dessie, dGFkc3VhbGFzZmF3MzBAZ21haWwuY29t
Disclaimer: All claims expressed in this article are solely those of the authors and do not necessarily represent those of their affiliated organizations, or those of the publisher, the editors and the reviewers. Any product that may be evaluated in this article or claim that may be made by its manufacturer is not guaranteed or endorsed by the publisher.
Research integrity at Frontiers
Learn more about the work of our research integrity team to safeguard the quality of each article we publish.