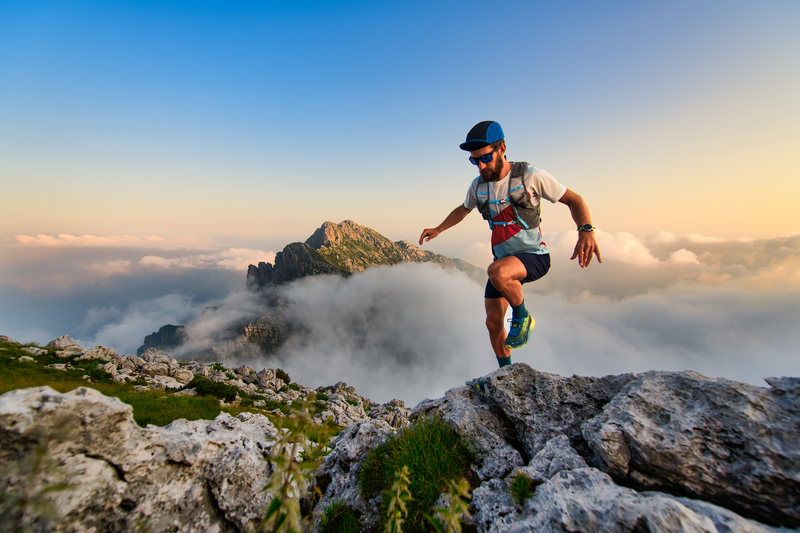
94% of researchers rate our articles as excellent or good
Learn more about the work of our research integrity team to safeguard the quality of each article we publish.
Find out more
ORIGINAL RESEARCH article
Front. Sustain. Food Syst. , 30 May 2024
Sec. Climate-Smart Food Systems
Volume 8 - 2024 | https://doi.org/10.3389/fsufs.2024.1389368
This article is part of the Research Topic Modelling Approaches for Climate Variability and Change Mitigation and Adaptation in Resource Constrained Farming Systems View all 12 articles
Climate change due to human activities is a reality and a growing threat to global food security. The trend of increasing temperature and decreasing rainfall in Iran shows climate warming, the result of climate change in Iran. Climate-smart agriculture (CSA) is an approach to developing new agricultural practices that support food security against climate change. The purpose of this study was to design a behavioral model for using CSA technologies in three phases: cognitive, motivational, and volitional, using the theories of planned behavior, self-determination, and social cognition. This research is the first to combine behavioral models to understand the application of CSA technologies. The research method in this study was quantitative and non-experimental. The statistical population included 800 wheat farmers from the city of Nazarabad in the Alborz province of Iran. A sample size of 260 people was determined using the Cochran formula. Appropriate stratified sampling was selected as the sampling method. Wheat farmers were then randomly selected in each village through proportional assignment. The research data was collected through a cross-sectional survey and a questionnaire. The models and hypotheses were tested using structural equation modeling. The statistical results confirmed the theoretical model and 15 research hypotheses. Additionally, the estimated field model explained about 62% of the variance in the behavior of using CSA technologies. Therefore, cognition, motivation, and volition effectively shape the behavior of using CSA technologies. In the cognitive phase, environmental concerns have the greatest impact on attitude toward the behavior of using CSA technologies (β = 0.714). In the motivational phase, personal norms have the most impact on the intention to use CSA technologies (β = 0.643). Finally, in the volitional phase, the intention to use CSA technologies has the highest impact on the behavior of using CSA technologies (β = 0.386). The increase in the guaranteed purchase price of wheat and paying soft and long-term loans by the policymakers will lead to an increase in the intention of wheat farmers to use CSA technologies.
The main challenge of the worldwide agricultural sector in 2050, considering the increase in population to about 10 billion people, will be the food supply (Venkatramanan and Shah, 2019). Therefore, food production will need to increase by 60% compared to the current world production (Jariwala et al., 2022). According to the Sixth IPCC Assessment Report, during the 21st century, the world climate will be warmer by 1.5°C under different greenhouse gas emissions scenarios compared to 1850–1900 (Pörtner et al., 2022). In addition, the amount of rainfall will also decrease (Ghalibaf et al., 2023). Climate change is defined as long-term changes in average temperature and rainfall patterns and other climatic factors due to natural changes or human activities in the world (Pickson et al., 2022). Climate change is a reality (Bozoglu et al., 2022) and a growing threat to global food security (Ghobadi Aliabadi et al., 2022). Climate change costs the world more than 1.2 trillion US$ and therefore destroys 1.6% of the global GDP annually (Kumar et al., 2017). The reasons for climate change and its effects are shown in Figure 1. By 2050, Iran will experience a 20% to 25% reduction in average precipitation, an increase of 2.6°C in temperature, and intense and prolonged droughts and floods (Goli et al., 2020). An investigation of fluctuations in precipitation indices (250 mm annual average) and temperature (18°C annual average) shows that climate change has warmed Iran (Ardakani et al., 2019). The average yearly temperature in Iran for each decade during the last 50 years, from 1970 to 2020, shows an increasing trend with a rate of about 0.4°C. During this period, the country’s average temperature showed an increase of about 2°C compared to the period before 1970. This increase is almost twice the average global temperature of about 1°C. Rainfall changes in Alborz province during the 2019–2021 crop year compared to the long term showed a decrease of 20.9%. The average temperature of the province has also been above normal (Iran Meteorological Organization, 2022). Nazarabad city of Alborz province is located in an arid and semi-arid climate with an average annual rainfall of 238 mm and an average annual temperature of 14.2°C (Mirkhani et al., 2020), which is the main research area.
Figure 1. The reasons for climate change and its effects. Source: Pachauri et al. (2014), Belay et al. (2017), Lipper et al. (2017), Hasan et al. (2018), Ardakani et al. (2019), Abbas (2022), Kadkhodazadeh et al. (2022), Olubukola et al. (2022), and Pörtner et al. (2022).
The wheat crop area worldwide is more than 220 million ha, with 5.7 million ha in Iran. Studies show that changes in climatic factors such as temperature will affect wheat yield (Eyni-Nargeseh et al., 2020). The agricultural sector in Iran produces about 18% of the GDP, 25% of non-oil exports, 20% of employment, and 85% of the total food supply, therefore it plays a very significant role in economic growth. Moreover, about 27% of Iran’s people live in rural areas, and their primary income source is agriculture (Jamshidi et al., 2020). The crop area of irrigated and rainfed wheat in Iran is 1,931,766 and 3,932,788 ha, its production is 8,173,673 and 5,541,585 tons, and its yield is 4,231 and 1,409 kg per ha, respectively. In Alborz province, the crop area of irrigated and rainfed wheat is 10,978 and 110 ha, its production is 63,675 and 100 tons, and its yield is 5,800 and 909.1 kg per ha, respectively. In Nazarabad city, the wheat crop area is 6,070 ha, its production is 30,263 tons, and its yield is 4985.7 kg per ha (Ministry of Iran Agriculture Jihad, 2022).
The impact of climate change on agricultural fields, along with the increasing world population, threatens to respond to the growing need for food. Climate-Smart Agriculture (CSA) is an approach to developing new agricultural practices that supports food security against climate change (Waaswa et al., 2022). CSA is a function of the environment and reduces the effects of climate change and causes carbon sequestration in the soil (Amadu, 2022). CSA is not a specific agricultural technology but identifies suitable farming methods for each specific location (Palombi and Sessa, 2013). Any method or technology that includes at least one of these goals is considered CSA (Khatri-Chhetri et al., 2016). CSA interventions is broad and includes water, soil, energy, genetic resources, livestock, forestry, fisheries management, and climate-smart crop production (Mashi et al., 2022). It introduces different methods of climate-smart crop production that have been adapted to climate change and local conditions (Brüssow et al., 2017), which is the subject of this study, as shown in Figure 2. Climate change has dramatically reduced production in many countries of the world (Datta et al., 2022). Due to the occurrence of climate change in Iran, it is necessary to use climate-smart crop production methods to achieve CSA goals.
Figure 2. Climate change effects on wheat fields, and adaptation strategies has been designed by authors (Caruana et al., 2000; Palombi and Sessa, 2013; Hasan et al., 2018; Akbari et al., 2019; Gardezi et al., 2022; Gauthier et al., 2022; Naz et al., 2022; Pickson et al., 2022; Taimour et al., 2022; Wu et al., 2022).
Over the past 20 years, the theory of planned behavior (TPB; Ajzen, 1991) and before that the theory of reasoned action (TRA; Ajzen and Fishbein, 1980) have been some of the most important and widely models used by researchers to understand and predict the relationship between attitude, intention and behavior (Small and Maseyk, 2022). TRA and TPB are social psychology models that show the beliefs and attitudes of farmers and have been used to understand and correctly predict their behavior (Asadollahpour et al., 2016). Many studies have used different applications of TRA and TPB in various structures to predict relationships between intention and behavior (Akbari et al., 2019). In addition, researchers have widely used TPB to explain the factors influencing environmental protection behaviors (Gansser and Reich, 2022), such as water conservation (Valizadeh et al., 2018), air pollution control (Xu et al., 2020), soil conservation (Tama et al., 2021), energy saving and carbon reduction (Chen, 2016), recycling (Strydom, 2018), and organic food purchase (Ahmed et al., 2021). For this reason, TPB was selected as the basis for designing the behavioral pattern of the use of CSA technologies. To develop the model, we used social cognition theory (Bandura, 1991) and self-determination theory (Deci and Ryan, 2000). The application of social cognition theory has been very useful for various researchers in investigating human behavior and the learning process (Hartley, 2022). Self-determination theory is a leading theory in human behavior motivation and is widely used in several domains (Gauthier et al., 2022). We also used two components, value for money (Caruana et al., 2000) and personal norms (Harland et al., 1999), in three phases: cognitive, motivational, and volitional. The purpose of this study was to design a behavioral pattern of using CSA technologies with emphasis on the three phases: cognitive, motivational, and volitional. The main research question is: What factors affect the wheat farmers’ behavior in using CSA technologies? Therefore, it is essential to study the farmers’ behavior in using CSA methods.
The general design of this research, which includes the effects of climate change on wheat fields, strategies to adapt to it, CSA goals, Climate-smart crop production methods, and behavioral theories of the subject of study to change the behavior of wheat farmers, is shown in Figure 3. In some cases, the intention of wheat farmers to use CSA technologies does not lead to the behavior of using these technologies. In other words, intention does not always lead to behavior and wheat farmers do something else. For example, the intention to use pressurized irrigation systems (drip and sprinkler irrigation) does not always lead to the behavior of using these technologies. The reason for this issue is the high cost of establishing the equipment of these technologies. This issue is explained in the voluntary phase of the research.
Figure 3. Some different methods of sustainable crop production based on CSA. Source: Palombi and Sessa (2013), Makate et al. (2018), Partey et al. (2018), Tiamiyu et al. (2018), Descheemaeker et al. (2020), and Bazzana et al. (2022).
Due to its critical position, Alborz Province is considered a crossroads of the north and west of Iran. It is limited to the Mazandaran province on the north, Tehran province on the east, Markazi province on the south, and Qazvin province on the west. The arid and semi-arid climate makes up half of the province’s total area. This province consists of six cities (Eshtehard, Savojbalagh, Taleghan, Fardis, Karaj, and Nazarabad). Its population is about 2,712,400 people (Iran Statistical Center Website, 2022). The main research site is Nazarabad city, with an area of 587 km2 and a population of 152,437 people located in two central regions (including Nazarabad city and three rural districts of Ahmadabad, Jamaleddin and Najmabad) and Tankaman regions (including Tankaman city, southern Tankaman, and northern Tankaman rural districts) with 53 villages (Alborz Agricultural Jihad Organization, 2022), see Figure 4.
Figure 4. Geographical location of the study area (Yousefi Khanghah et al., 2020).
In Iran, the government’s policy is self-sufficiency in wheat production according to global conditions. Wheat is a global strategic crop and self-sufficiency in its production is vital for Iran (Najafi Alamdarlo et al., 2019). Therefore, supporting wheat farmers is very important for the government. Researchers’ interviews with wheat farmers in the Nazarabad city of Alborz province on climate change show the main reasons for their concern about decreasing wheat yields are: reduction and strong fluctuations in rainfall, temperature increase, drought, groundwater decrement, and dry winds. Furthermore, the largest crop area of wheat and barley belongs to Nazarabad city. The authors posit that due to these conditions, it is necessary to use CSA methods to achieve CSA goals. On the other hand, the expected behavior changes of wheat farmers toward using CSA technologies to combat climate change and adapt to these conditions has not yet happened. For these reasons, the statistical population of this research was selected from the wheat farmers of Nazarabad city, Alborz province.
The research method in this study is quantitative and non-experimental, and research data were collected through a cross-sectional survey and questionnaire (Creswell, 2009). The statistical population of this study includes 800 wheat farmers from Nazarabad city in the Alborz province of Iran (Iran Statistical Center Website, 2022). Appropriate Stratified sampling was selected as the sampling method. The research area was first divided into four classes (north, south, west and east). Villages were then randomly selected in each class. The 260 participant sample size was determined using the Cochran formula as follows (Cochran, 1963; Nanjundeswaraswamy and Divakar, 2021)
n: statistical sample size.
N: the size of the statistical population.
d: allowed error equal to 0.05.
Z: standard normal probability, at the 95% confidence level is equal to 1.96.
p: The estimated proportion of an attribute that is present in the population.
q: The estimated proportion of an attribute that is not present in the population.
(p and q are assumed equal to 0.5).
Therefore,
n = 260.
Finally, wheat farmers were randomly selected in each village through proportional assignment. After a literature review, a list of CSA methods consistent with the examples provided by Palombi and Sessa (2013), Descheemaeker et al. (2020), and Qureshi et al. (2022) was prepared, and the following research items were determined.
(1) Irrigation management: The principal strategy is to adapt to climate change (Senyolo et al., 2018). Climate change will intensify the need for water and increase droughts (Nazari et al., 2018). While the amount of water consumption in Iran’s agricultural sector is high approximately 92% of available water (Momvandi et al., 2018), the use of sprinklers and drip irrigation could increase water use efficiency by about 50% to 70% and 80% to 90%, respectively (Nikolaou et al., 2020). (2) Conservation agriculture: Through biological processes, this method reduces greenhouse gases emission, increases soil carbon absorption (Descheemaeker et al., 2020), and boosts productivity and resilience to climate risks (Michler et al., 2019). (3) Integrated pests and diseases management: Climate change causes temperature fluctuations, changes in rainfall patterns, and water shortages. It also complicates controlling and combating pests and diseases (Grace et al., 2019). Therefore, it is necessary to use this method to reduce the damage of pests and diseases, and protect the environment by diminishing pesticide use resulting in less pollution of water, soil, and air. (4) Integrated weed management: These methods are necessary because Changes in climatic factors cause an increase in weeds (Bajwa et al., 2019) and the spread of new weed species in different regions (Grace et al., 2019). (5) Farm management: This method, such as crop diversification and the use of appropriate amounts of inorganic and organic fertilizers (Thornton et al., 2018), increases farm productivity, income, and adaptation to the effects of climate change (Brüssow et al., 2017). (6) Using improved seed varieties: This method, such as using high-yield seeds, increases the field income and adaptation to the effects of climate change (Aryal et al., 2018). A researcher-made questionnaire consisting of 15 sections was prepared to measure research variables in three phases (cognitive, motivational, and volitional). The variables, the number of items, and their resources (Sections 1–14) of the questionnaire are as follows:
The cognitive phase includes the following variables: Environmental beliefs with seven items (Dunlap and Van Liere, 1978; Straughan and Roberts, 1999), Environmental concerns with five items (Dunlap and Van Liere, 1978; Kilbourne and Pickett, 2008), Perceived value for money with five items (Caruana et al., 2000), Outcome expectancy with six items.
The motivational phase includes the following variables: Attitude with six items, Subjective norms with five items (Ajzen, 2002), Perceived behavioral control with five items (Venkatesh et al., 2003), Intention with six items (Ajzen, 2002), Personal norms with five items (Harland et al., 1999), High self-determination motivations with four items (Mullan and Markland, 1997), Low self-determination motivations with four items (Wilson et al., 2002).
The volitional phase includes the following variables: Action planning with four items (Scholz et al., 2008), Coping planning with four items (Scholz et al., 2008), and behavior with six items (Ajzen, 2002). The final dependent variable is behavior, and the intermediate dependent variables are intention and attitude toward behavior. All other variables are independent. Section 15 of the questionnaire measures wheat farmers’ individual and professional characteristics. All items were measured using a Likert 7-point scale (ranging from fully disagree = 1 to fully agree = 7).
The viewpoints of the supervisor, advisor, and experts were used to determine the validity of the questionnaire and the necessary corrections were made. Then, to determine the reliability of the questionnaire, a pilot test was conducted in an area outside the main research site (Hashtgerd city); these results were not applied in the final evaluation. The overall Cronbach’s alpha coefficient of the questionnaire is 0.904, with the lowest alpha coefficient of latent variables being related to the behavior of using CSA technologies (0.712) and the highest related to outcome expectancy (0.888). Cronbach’s alpha coefficient for other latent variables is as follows: Environmental beliefs = 0.776, Environmental concerns = 0.729, Perceived value for money = 0.752, Attitude = 0.786, Perceived behavioral control = 0.716, Intention = 0.762, Personal norms = 0.880, High self-determination motivations = 0.828, Low self-determination motivations = 0.880. Action planning = 0.751, Coping planning = 0.797. These results confirmed the reliability of the questionnaire. The research method is shown in Figure 5.
Based on the literature review, the theoretical model of this research was presented in three phases, cognitive, motivational, and volitional, as follows:
In this phase, the wheat farmers were studied from a cognitive viewpoint. Cognitive psychology encompasses psychological processes such as perception, thinking, knowledge representation, memory, problem-solving, and human information processing (Shuell, 1986). Cognitive models consider human action as intentional behavior, and individuals examine the characteristics of their behavior and beliefs by examining the value of behavior before intending to perform the behavior (Hagger and Chatzisarantis, 2014). Most views on attitude research focus on understanding the relationship between cognitive structures (i.e., belief), affect, and attitude (Bodur et al., 2000; Perry et al., 2022). Attitude resulting from a cognitive structure of beliefs is called behavioral belief (Gadenne et al., 2011; Hagger et al., 2022). General beliefs talk about the relationship between humans and environment; specific beliefs about environmental problems are the subject of this study. Environmental beliefs include people’s knowledge of environmental issues (Rosenthal, 2022). The results of the research conducted by Kilbourne and Pickett (2008) with the title: How materialism affects environmental beliefs, concern, and environmentally responsible behavior, showed that when beliefs about environmental problems occur, concerns will arise afterward. The results of studies conducted by Arbuckle et al. (2013) showed that farmers who believe climate change results from human activities are concerned about the effects and perform adaptive activities. Farmers who believe that nature is the cause of climate change had less concern and adaption, and there was very little likelihood of adaptive activities. The farmers who include climate change in their beliefs, concerns, and attitudes toward adaptation are more successful than those who do not. The results of studies conducted by Kwistianus et al. (2020) with the title: Environmental Concern, Attitude, and Willingness to Pay of Green Products: Case Study in Private Universities in Surabaya, Indonesia showed that Environmental concerns significantly affect attitude and Purchase Intention. Furthermore, Purchase Intention for environmentally friendly products has a positive effect on Purchase Behavior for environmentally friendly products. The results of the research conducted by Han et al. (2022) with the title: Impact of Climate Change Beliefs on Youths’ Engagement in Energy-Conservation Behavior: The Mediating Mechanism of Environmental Concerns showed that Climate change beliefs have an important influence on formation of environmental concerns; The stronger an individual’s climate change belief, the higher environmental concern.
The social cognition theory (SCT; Bandura, 1991) and the component of value for money (Caruana et al., 2000) were used to design the cognitive phase model. Social cognition theory (SCT) describes the function of psychology in the condition of triple reciprocal causality, which is as follows: (1) Cognitive and personal factors, (2) Behavior, (3) Environmental events. These factors affect each other bilaterally (Bandura, 1991; Naz et al., 2022). The two main components of SCT are outcome expectancy and self-efficacy. Outcome expectancy is an individual’s evaluation of specific behavior that leads to determining results, and self-efficacy is the judgment of an individuals’ ability to perform a certain level of performance (Bandura, 1986; Devi et al., 2022). The results of the research of Collado and Evans (2019) with the title: Outcome expectancy: A key factor to understanding childhood exposure to nature and children’s pro-environmental behavior showed that Outcome expectancy of environmental behaviors has a significant effect on attitude toward these behaviors.
The results of studies conducted by Mehra and Ratna (2014) with the title: Attitude and behavior of consumers toward organic food: an exploratory study in India, showed that the significant influencing factors on the attitude toward organic food are: perception toward organic food, health awareness, product information, value for money, access and trust. Therefore, value for money has a significant effect on attitude toward organic food. The findings of Kungumapriya and Malarmathi (2018) also show that perceived value for money is effective on attitude toward behavior Quality of service, customer satisfaction, and product value are the three factors that many companies strive to provide. Product value definitions include the following: (1) Low price, (2) Factors requested in a product, (3) Quality obtained vs. price paid, and (4) What is obtained vs. what is paid (Caruana et al., 2000). In this study, the value for money is interpreted as the perceived value for money (PVfM). We consider it the overall wheat farmer’s assessment of the benefits vs. costs (Wylde, 2022) of using CSA technologies. The results of various researches have shown that environmental concerns are effective on attitude toward behavior (De Groot and Steg, 2007; Khaola et al., 2014; Paul et al., 2016). Beliefs about environmental problems lead to concerns about these problems, and environmental concerns lead to a positive attitude toward doing a behavior. On the other hand, outcome expectancy and perceived value for money were introduced as two cognitive factors that affect human behavior. These factors lead to the formation of attitude that further shape behavioral intention. These factors are variables that affect attitude in the cognitive phase of research and were presented in the form of the following hypotheses:
H1: Environmental beliefs significantly affect environmental concerns.
H2: Environmental concerns significantly affect attitudes toward the use of CSA technologies.
H3: The outcome expectancy of using CSA technologies significantly affects attitudes toward using these technologies.
H4: The perceived value for money of using CSA technologies significantly affects attitudes toward using these technologies.
The motivation of wheat farmers was examined in this phase. Individuals begin, continue, and develop their behavior to the extent that they believe it will lead to desirable consequences (Deci and Ryan, 2000). Motivation is responsible for the decision to do the work, the duration of its sustainable continuation, and the effort to continue it (Chen et al., 2008; Vorobyova et al., 2022). Many theories have examined behavioral motivation variables to understand the behavior better. In this study, this issue is investigated by combining the theory of planned behavior (TPB; Ajzen, 1991) and self-determination theory (SDT; Deci and Ryan, 2000). The intention is at the center of the structure of many theories of motivation (Deci et al., 1991). The intention construct is the core of TRA (Ajzen and Fishbein, 1980) and TPB (Ajzen, 1991; Hagger et al., 2022). Intention represents an individual’s attempt to perform a behavior (Armitage and Conner, 2001), and it is a set of motivational factors affecting behavior (Ajzen, 1991). Behavior is described based on the ingredients of purpose, action, context, and time (Ajzen, 2002). The TPB assumes that three independent factors determine the behavioral intention: (1) Attitude toward the behavior: A person’s overall positive or negative evaluation of the performance of a specific behavior, (2) Subjective norms: Perceived social pressures for doing or not doing the behavior, and (3) Perceived behavioral control: An individual’s conception about the ease or difficulty of doing a behavior (Ajzen, 1991, 2002; Arkorful et al., 2022).
The results of the research conducted by Asrari et al. (2022) with the title: Modeling resilience behavior against climate change with food security approach showed that variables of attitude toward the behavior, subjective norms and perceived behavioral control have a positive and significant effect on behavioral intention. Furthermore, the results of the research Wang and Li (2022) with the title: Consumers’ intention to bring a reusable bag for shopping in China: Extending the theory of planned behavior it shows the same results. The results of studies conducted by Close et al. (2018) with the title: Using the theory of planned behavior to explain intention to eat a healthful diet among Southeastern United States office workers showed that variables of attitude toward the behavior, subjective norms and perceived behavioral control have a positive and significant effect on behavioral intention.
To increase the model’s predictive power, the component of personal norms (PN) was added to the model. Personal norms are a person’s belief about their moral commitment to perform a particular behavior (Harland et al., 1999). Personal norms are a feeling of commitment toward a behavior that affects the individual’s behavioral intention (Godin et al., 2008). The results of the research conducted by Roos and Hahn (2019) with the title: Understanding Collaborative Consumption: An Extension of the Theory of Planned Behavior with Value-Based Personal Norms showed that personal norms have a significant effect on intention. Furthermore, the results of the research of Yaghoubi et al. (2019) with the title: Iranian agriculture advisors’ perception and intention toward biofuel: Green way toward energy security, rural development and climate change mitigation it shows the same results. The results of the research conducted by Park and Ha (2014) also show that personal norms have a significant effect on behavioral intention. The findings of various researchers show that behavioral intention has a significant effect on behavior (De Leeuw et al., 2015; Hagger et al., 2017; Brown et al., 2018; Kungumapriya and Malarmathi, 2018; Strong et al., 2018; Sun et al., 2018).
According to TPB, with the component of personal norms added to the model, the following five hypotheses were tested in this study:
H5: Attitude toward using CSA technologies significantly affects the intention to use these technologies.
H6: Subjective norms about using CSA technologies significantly affect the intention to use these technologies.
H7: Perceived behavioral control about using CSA technologies significantly affects the intention to use these technologies.
H8: Personal norms regarding the behavior of using CSA technologies significantly affect the intention of using these technologies.
H9: Intention about the behavior of using CSA technologies significantly affects the behavior of using these technologies.
SDT was first proposed by Deci and Ryan (1991). According to this theory, different types of motivation are as follows: Intrinsic motivation, extrinsic motivation, and amotivation (Pelletier et al., 1998; Good et al., 2022). SDT states that autonomous motivation includes behaviors with volition and choice, and controlled motivation is the behaviors created by external forces (Deci and Ryan, 2008; Ojo, 2022). The main focus of the theory is the vast difference between autonomous and controlled forms of motivation (Hagger and Protogerou, 2020). Therefore, examining the effects of these two components on behavioral intentions provides a helpful perspective for developing appropriate methods for behavioral stimulation intentions. The results of studies conducted by Rahi and Ghani. (2019) with the title: Integration of expectation confirmation theory and self-determination theory in internet banking continuance intention showed that high self-determination motivations and low self-determination motivations have a significant effect on intention. The results of various researches show that high self-determination motivations has a significant effect on behavioral intention (Rump et al., 2017; Williams et al., 2019; Abdullatif and Velázquez-Iturbide, 2020). The results of the findings of Wang et al. (2019) also show that low self-determination motivations has a significant effect on behavioral intention. In this study, we combined TPB and SDT to predict the behavior more accurately (Deci and Ryan, 1991). According to SDT, this study tests the following two hypotheses:
H10: High self-determination motivations in using CSA technologies significantly affect the intention to use these methods.
H11: Low self-determination motivations in using CSA technologies significantly affect the intention to use these methods.
In this phase, wheat farmers are tested in terms of volition because they do not always behave according to their intentions. Different factors facilitate or threaten the translation of intention to action (Scholz and Lippke, 2008). After the formation of intention, people enter the volitional phase in which the intention changes into the action. While behavior can be best predicted with the help of intention, this component alone is insufficient to predict behavioral change and does not account for large amounts of unexplained behavioral variance. This phenomenon is called the intention-behavior gap (Scholz et al., 2008). Planning plays a considerable role in bridging the gap between intention and behavior for implementing health behaviors (Ziegelmann et al., 2006; Xu et al., 2022). Planning is the understanding of the mental simulation of behavior to facilitate the conditions for attainment behavior and an economical and effective method to achieve the goal. A predetermined behavior will be performed through planning (Scholz et al., 2008). There are two types of planning: action planning and coping planning. Action planning determines the when, where, and how to act, and coping planning predicts dangerous situations and the mental simulation of obstacles and readiness for successful adaptation (Sniehotta et al., 2005; Xu et al., 2022). The findings of various researchers show that behavioral intention has a significant effect on action planning and coping planning (Hagger et al., 2017; Brown et al., 2018; Strong et al., 2018; Lin et al., 2020). The results of various researches show that action planning and coping planning have a significant effect on behavior (Strong et al., 2018; Lin et al., 2020). These concepts are examined during the volitional phase of the study according to the following four hypotheses:
H12: The intention to use CSA technologies has a significant effect on action planning.
H13: The intention to use CSA technologies has a significant effect on coping planning.
H14: Action planning for the use of CSA technologies has a significant effect on the behavior of using CSA technologies.
H15: Coping planning for the use of CSA technologies has a significant effect on the behavior of using CSA technologies. Finally, the theoretical model was designed according to Figure 6.
Figure 6
Descriptive and inferential statistics were used to answer the research questions and achieve the research objectives. The mean, median, and mode were used in the descriptive statistics, and structural equation modeling was used in the inferential statistics. The statistical data analysis was done using SPSS version 26 and Amos version 24. Structural equation modeling (SEM) is a multivariate technique that is often used to test hypothetical models in behavioral sciences. A review of the literature shows its increase popularity as a research tool (Cheung and Chan, 2005). SEM allows complex phenomena to be statistically modeled and tested. Therefore, SEM technologies are the best method to confirm or reject mathematical models in a quantitative method (Schumacker and Lomax, 2004). SEM is a combination of factor analysis and path analysis. The purpose of SEM, similar to factor analysis, is to provide a summary of the relationships between variables. SEM is also similar to path analysis in that researchers can test hypothesized relationships between constructs. In SEM, researchers must evaluate multiple test statistics and a number of appropriate indicators to determine whether the model accurately determines the relationship between constructs and observable variables (Weston and Gore, 2006). In general, any SEM analysis includes the stages of model design, data collection, model estimation, model evaluation, and possibly model modification (Lei and Wu, 2007). The features of structural equation modeling are as follows: (1) Simultaneous response to a large number of statistical hypothesis tests. (2) Answering research questions and hypotheses without estimating latent variables. (3) Confirming, correcting or rejecting the presented theoretical model based on the literature review (Payendeh Najafabadi and Omidi Najafabadi, 2013).
All participants were male wheat farmers with a mean age of 49.85 and Standard deviation 11.98. The mean experience of wheat farmers was 32.29, with a standard deviation 9.97. The mean monthly income of wheat farmers was 53692307.69 rials with a standard deviation 72350114.63. The mean household size of the wheat farmers was 4.37 with a standard deviation 0.99. The mean wheat crop area was about 14.20 hectares with a standard deviation 12.59. The mean yield per hectare of wheat farmers was 4.72 with a standard deviation 0.82 (Table 1).
Path analysis shows that environmental beliefs significantly increased the environmental concerns of wheat farmers (β = 0.70, p < 0.001). In addition, environmental concerns (β = 0.71, p < 0.001), outcome expectancy (β = 0.64, p < 0.001), and perceived value for money (β = 0.64, p < 0.001) also significantly increased the attitude of the wheat farmers to use CSA technologies. Therefore, hypotheses H1, H2, H3, and H4 are confirmed. In addition, attitude toward the use of CSA technologies (β = 0.44, p < 0.001), subjective norms (β = 0.55, p < 0.001), perceived behavioral control (β = 0.33, p < 0.001), personal norms (β = 0.64, p < 0.001), high self-determination motivations (β = 0.63, p < 0.001), and low self-determination motivations (β = −0.19, p < 0.01) significantly affect the intention to use CSA technologies. Therefore, hypotheses H5, H6, H7, H8, H10, H11, were confirmed. The results also show that intention (β = 0.15, p < 0.05) significantly increases action planning; therefore, H12 is approved. Moreover, intention (β = 0.39, p < 0.001) significantly increases coping planning, so H13 is also confirmed. Finally, action planning (β = 0.17, p < 0.05), coping planning (β = 0.28, p < 0.001), and intention (β = 0.38, p < 0.001) significantly influence the behavior of using CSA technologies. Therefore, hypotheses H9, H14, and H15 are also confirmed (see Table 2; Figure 7).
Figure 7. The final model of behavior to using CSA technologies (p < 0/001***, p < 0/01**, p < 0/05*).
According to the theoretical framework designed to test the behavioral pattern, 14 variables were first entered into the equation, and then a model was designed. Next, the measurement model was examined using the Maximum-Likelihood method. Finally, the model fit was evaluated using indicators (p-value cmin, X2 / DF, RMSEA, P-close, NFI, CFI, GFI, RMR). According to the appropriate criteria of these indicators, a good model fit was provided (Table 3; Payendeh Najafabadi and Omidi Najafabadi, 2013). The findings show that the estimated field model explains about 62% of the variance in the behavior of using CSA technologies. Also, a significant relationship was observed between the research variables. Therefore, cognition, motivation and volition effectively shape the behavior of using CSA technologies. In the cognitive phase, environmental concerns have the greatest impact on attitude toward the behavior of using CSA technologies (β = 0.714). In the motivational phase, personal norms have the most impact on the intention to use CSA technologies (β = 0.643). Finally, in the volitional phase, the intention to use CSA technologies has the highest impact on the behavior of using CSA technologies (β = 0.386). The most important factors affecting the behavior of using CSA technologies include the intention to use CSA technologies, coping planning, and action planning with path coefficients of 0.378, 0.276, and 0.166, respectively. Also, attitude, subjective norms, perceived behavioral control, personal norms, high self-determination motivations and low self-determination, respectively, with path coefficients (0.437, 0.549, 0.334, 0.643, 0.626, and −0.195) have an effect on the intention to use CSA technologies (Figure 8).
The proposed field model explains about 64% of the total attitude variance. Environmental concerns have the greatest impact on attitude (β = 0.714). The results show that the studied cognitive factors led wheat farmers to a positive attitude toward using CSA technologies. The findings of this research are consistent with previous research and confirm that environmental beliefs have a significant effect on environmental concerns (H1) (Kilbourne and Pickett, 2008; Han et al., 2022). Researchers’ interviews with wheat farmers in the research area show that wheat farmers’ knowledge and information regarding environmental problems such as water, soil, and air pollution have increased. The reason for this is the extensive use of agricultural inputs such as chemical fertilizers, pesticides, and herbicides to increase wheat yield. They believed that in recent years the air temperature has increased, and the amount of rainfall has also decreased. Therefore, they have understood climate change. This awareness has led them to be concerned about the environment. Furthermore, environmental concerns have had a significant effect on their attitude toward the behavior of using CSA technologies (H2) (Kwistianus et al., 2020; Wang and Li, 2022). Continuous and abundant use of chemical materials and damage to the environment, the risk of reducing agricultural productivity in the future, and decreased water and soil resources have caused concern to wheat farmers. The concern of wheat farmers about environmental problems has caused wheat farmers to develop a positive attitude toward the use of CSA technologies.
The outcome expectancy of using CSA technologies significantly affects the attitude toward using these technologies (H3) (Collado and Evans, 2019). Regarding the Outcome Expectancy of using CSA technologies, Wheat farmers have understood that using CSA technologies such as pressurized irrigation systems (drip and sprinkler irrigation) leads to a reduction in water consumption, a decrease in the cost and time of irrigation, and an increase in water use efficiency. This issue increases the quantity and quality of production, income, and food security. Moreover, it provides adaptation and builds resilience against drought, and by reducing fuel consumption for water extraction, it also effectively reduces greenhouse gases emission. This issue has led to wheat farmers’ positive attitude toward using CSA technologies to protect the environment. Therefore, outcome expectancy of using CSA technologies has led to a positive attitude toward using CSA technologies. Perceived value for money concerning the use of CSA technologies significantly affects the attitude toward using this technology (H4) (Mehra and Ratna, 2014; Hassan et al., 2022). Regarding the perceived value for money, Wheat farmers have found that the use of CSA technologies is valuable due to their high cost compared to environmentally friendly benefits, and this issue has created a positive attitude toward the use of these technologies. For example, although the cost of buying and building drip and sprinkler irrigation equipment for wheat fields is high, it is valuable compared to the environmentally friendly benefits obtained. It is suggested that information centers should be formed in the management of agricultural jihad in the studies areas. In these centers, the knowledge and awareness of wheat farmers regarding climate change and the benefits of using CSA technologies should be increased. This issue has increased the environmental beliefs and concerns of the wheat farmers and causes the effects of climate change to decrease. Moreover, as knowledge increases and is shared among wheat farmers regarding climate change, the outcome expectancy of using CSA technologies and the perceived value for money will also increase, and thus, the attitude toward the use of these technologies will be enhanced.
In the motivational phase, the proposed field model explains about 75% of the total variance of the intention to use CSA technologies and Personal norms have the most impact on the intention (β = 0.643). The findings of this research are consistent with previous researches and confirm that attitude toward using CSA technologies has a significant effect on the intention to use CSA technologies (H5) (Zhang et al., 2020). Wheat farmers have perceived that using CSA technologies to protect the environment and prevent the effects of climate change is beneficial, valuable, and necessary. Therefore, their evaluation of the use of these technologies is positive, and the intention to use these methods has increased. Subjective norms significantly affect the intention to use CSA technologies (H6) (Luc, 2020). The subjective norms of wheat farmers are influenced by other farmers, friends, family members, and agricultural extension agents. The opinions of these people motivate farmers to use CSA technologies. In other words, social pressures lead to the farmers’ intention to use these technologies against the effects of climate change.
Perceived behavioral control has a significant effect on the intention to use CSA technologies (H7) (Moon, 2021). Regarding perceived behavioral control, Wheat farmers believe that they have the necessary resources and equipment to apply CSA technology to a certain extent to reduce the effects of climate change. In other words, they have the ability to use these technologies to some extent. Therefore, perceived behavioral control leads to the intention to use these technologies. For example, wheat farmers have the necessary knowledge and ability to apply farm management.
Personal norms regarding the behavior of using CSA technologies significantly affect the behavioral intention of using these technologies (H8) (Roos and Hahn, 2019). Regarding personal norms, wheat farmers were committed to implementing CSA technologies. Despite many problems, they desired to make more regular efforts to implement these methods. This feeling of responsibility leads to the intention to implement these technologies. The use of appropriate amounts of chemical fertilizers, pesticides, and herbicides to prevent environmental pollution and the emission of greenhouse gases is a result of a personal commitment. High self-determination motivation about using CSA technologies has a significant effect on the intention of using these methods (H10) (Rahi and Ghani, 2019). Regarding the high self-determination motivation, a feeling of interest and satisfaction toward using CSA technologies has created an internal motivation in the wheat farmers. Low self-determination motivations significantly and negatively affect the intention of using these methods (H11) (Wang et al., 2019). Considering that most of the wheat farmers use conventional agriculture, the lack of suitable external rewards has reduced their intention to use CSA technologies, and this issue has led to a significant and negative effect of this motivations on intention. For example, non-payment of free loans for the implementation of pressurized irrigation systems in wheat fields leads to a decrease in the intention to use these technologies.
Intention to use CSA technologies has a significant effect on the behavior of using CSA technologies (H9) (Kwistianus et al., 2020). When wheat farmers intend to use methods such as conservation agriculture, the behavior of using these methods is also realized. In this phase, it is suggested that agricultural extension agents of agricultural jihad management in the research region should teach wheat farmers about climate change and CSA methods in short-term training courses to increase their knowledge and awareness in this regard. This will help wheat farmers find a more positive attitude toward using CSA technologies. Therefore, the intention to use CSA technologies increases in them.
It is suggested that the management of the agricultural jihad in the research region should organize social gatherings at specific time intervals and provide the necessary explanations for the wheat farmers concerning climate change and CSA technologies. In this way, the wheat farmers will gradually form subjective norms regarding the use of CSA technologies, creating an intention to use these technologies. In addition, this management should increase the number of agricultural extension agents for CSA technologies extension. This will increase the wheat farmer’s ability to combat the effects of climate change and increase their behavioral control. Therefore, the intention to use agricultural technologies among wheat farmers enhances.
Using itinerant extension agents and training wheat farmers about the effects of climate change on wheat fields and how to use CSA methods will lead to the creation of personal norms. This work should have very good results, especially in the communities of wheat farmers. The increment of personal norms for the use of CSA technologies increases the intention to use these technologies. The increase in the guaranteed purchase price of wheat and paying soft and long-term loans by the policymakers will lead to an increase in the intention of wheat farmers to use CSA technologies. The intention to use these technologies will cause the behavior of wheat farmers to use CSA technologies in the research area. Regional experimental farms should be established by the management of the agricultural jihad in the research area. In these farms, the use of CSA technologies will increase the high self-determination motivation of wheat farmers, increasing their intention to use CSA technology. The government should also consider the necessary incentives to increase the use of CSA technologies in wheat fields. These incentives, such as increasing the subsidized sale of agricultural inputs (chemical fertilizers, pesticides, and herbicides) will enhance the low self-determination motivation for using CSA technologies in wheat farmers. Stopping the import of wheat by the government also increases this motivation. With the enhancement of this motivation, the intention to use CSA technology will also increase among wheat farmers.
In this phase, the effective factors between the intention and behavior gap were investigated. The intention has the highest impact on the behavior of using CSA technologies (β = 0.386). The findings of this research are consistent with previous research and confirm that the intention to use CSA technologies has a significant effect on the action planning of using these technologies (H12) (Potthoff et al., 2017). When the wheat farmers intend to use the CSA technologies, this intention leads to action planning for the use of these technologies. For example, the intention to use integrated pests and disease management leads to action planning for using these methods. The intention to use CSA technologies has a significant effect on coping planning using these technologies (H13) (Strong et al., 2018). For example, the intention of wheat farmers to use integrated weed management has led to coping planning for the use of these methods. Action planning for using CSA technologies significantly affects the behavior of using CSA technologies (H14) (Brown et al., 2018). Action planning of wheat farmers has led to the behavior of using agricultural technologies. For example, when wheat farmers have planned to use high-yielding seeds, they determine the time and place of purchase and make this purchase. Coping planning for the use of CSA technologies has a significant effect on the behavior of using CSA technologies (H15) (Lin et al., 2020). Coping planning of wheat farmers has also led to the behavior of using CSA technologies. For example, when wheat farmers have decided to implement sprinkler irrigation in their fields, they have done Coping planning in this regard. In addition, they have planned to solve possible problems and obstacles in its implementation. Therefore, the wheat farmers have used coping planning to resolve the impediments and used opportunities to implement sprinkler irrigation.
In this phase, it is suggested that the management of agricultural jihad in the research area should increase the knowledge of wheat farmers about the benefits of implementing CSA technologies through the creation of social networks. This will increase their intention to implement these technologies. Increasing the wheat farmers’ intention to use CSA technologies will enhance the action and coping planning for using CSA technologies. The Agricultural Jihad Ministry and the private market sector should also provide the equipment required for CSA technologies so that wheat farmers can take the necessary action planning to use these technologies. Action planning for the use of CSA technologies by wheat farmers leads to the behavior of using CSA technologies. Training cards about how to use CSA technologies should be provided by the Agricultural Jihad Management in the research area, delivering to wheat farmers along with agricultural inputs in agricultural service centers. In this way, wheat farmers will receive the necessary knowledge on the problems and obstacles of implementing these technologies. As a result, suitable conditions are provided for coping planning. Coping planning for the use of CSA technologies by wheat farmers leads to the behavior of using these technologies.
The raw data supporting the conclusions of this article will be made available by the authors, without undue reservation.
Ethical review and approval was not required for the study on human participants in accordance with the local legislation and institutional requirements. Written informed consent from the [patients/participants OR patients/participants legal guardian/next of kin] was not required to participate in this study in accordance with the national legislation and the institutional requirements.
SK: Formal analysis, Investigation, Resources, Software, Writing – original draft, Writing – review & editing. MO: Conceptualization, Methodology, Supervision, Validation, Visualization, Writing – original draft, Writing – review & editing. SM: Conceptualization, Validation, Writing – original draft, Writing – review & editing.
The author(s) declare that no financial support was received for the research, authorship, and/or publication of this article.
The authors declare that the research was conducted in the absence of any commercial or financial relationships that could be construed as a potential conflict of interest.
All claims expressed in this article are solely those of the authors and do not necessarily represent those of their affiliated organizations, or those of the publisher, the editors and the reviewers. Any product that may be evaluated in this article, or claim that may be made by its manufacturer, is not guaranteed or endorsed by the publisher.
Abbas, S. (2022). Climate change and major crop production: evidence from Pakistan. Environ. Sci. Pollut. Res. 29, 5406–5414. doi: 10.1007/s11356-021-16041-4
Abdullatif, H., and Velázquez-Iturbide, J. Á. (2020). Relationship between motivations, personality traits and intention to continue using MOOCs. Educ. Inf. Technol. 25, 4417–4435. doi: 10.1007/s10639-020-10161-z
Ahmed, N., Li, C., Khan, A., Qalati, S. A., Naz, S., and Rana, F. (2021). Purchase intention toward organic food among young consumers using theory of planned behavior: role of environmental concerns and environmental awareness. J. Environ. Plan. Manag. 64, 796–822. doi: 10.1080/09640568.2020.1785404
Ajzen, I. (1991). The theory of planned behavior. Organ. Behav. Hum. Decis. Process. 50, 179–211. doi: 10.1016/0749-5978(91)90020-T
Ajzen, I. (2002). Constructing a TPB questionnaire: Conceptual and methodological considerations. Citeseer.
Ajzen, I., and Fishbein, M. (1980). Understanding attitudes and predicting social behavior. Englewood Cliffs, New Jersey: Prentiss-Hall.
Akbari, M., Ardekani, Z. F., Pino, G., and Maleksaeidi, H. (2019). An extended model of theory of planned behavior to investigate highly-educated Iranian consumers’ intentions towards consuming genetically modified foods. J. Clean. Prod. 227, 784–793. doi: 10.1016/j.jclepro.2019.04.246
Alborz Agricultural Jihad Organization. (2022). Available at: https://alborz.maj.ir/page-alborzmain/FA/98/form/pId15634. (Accessed March 28, 2022).
Amadu, F. O. (2022). Farmer extension facilitators as a pathway for climate smart agriculture: evidence from southern Malawi. Clim. Pol. 6, 1–6. doi: 10.1080/14693062.2022.2066060
Arbuckle, J. G., Prokopy, L. S., Haigh, T., Hobbs, J., Knoot, T., Knutson, C., et al. (2013). Climate change beliefs, concerns, and attitudes toward adaptation and mitigation among farmers in the Midwestern United States. Clim. Change 117, 943–950. doi: 10.1007/s10584-013-0707-6
Ardakani, Z., Bartolini, F., and Brunori, G. (2019). Economic modeling of climate-smart agriculture in Iran. New Medit 2019, 29–40. doi: 10.30682/nm1901c
Arkorful, V. E., Hammond, A., Lugu, B. K., Basiru, I., Sunguh, K. K., and Charmaine‐Kwade, P. (2022). Investigating the intention to use technology among medical students: an application of an extended model of the theory of planned behavior. J. Public Aff. 22:e2460. doi: 10.1002/pa.2460
Armitage, C. J., and Conner, M. (2001). Efficacy of the theory of planned behaviour: a meta‐analytic review. Br. J. Soc. Psychol. 40, 471–499. doi: 10.1348/014466601164939
Aryal, J. P., Jat, M. L., Sapkota, T. B., Khatri-Chhetri, A., Kassie, M., and Maharjan, S. (2018). Adoption of multiple climate-smart agricultural practices in the Gangetic plains of Bihar, India. Int J Clim Change Strat Manage. 10:25. doi: 10.1108/IJCCSM-02-2017-0025
Asadollahpour, A., Najafabadi, M. O., and Hosseini, S. J. (2016). Modeling behavior pattern of Iranian organic paddy farmers. Paddy Water Environ. 14, 221–229. doi: 10.1007/s10333-015-0492-0
Asrari, A., Omidi Najafabadi, M., and Farajollah Hosseini, J. (2022). Modeling resilience behavior against climate change with food security approach. J. Environ. Stud. Sci. 12, 547–565. doi: 10.1007/s13412-022-00763-z
Bajwa, A. A., Khan, M. J., Bhowmik, P. C., Walsh, M., and Chauhan, B. S. (2019). Sustainable weed management. Innov Sustain Agric, 1, 249–286. doi: 10.1007/978-3-030-23169-9_9
Bandura, A. (1986). Social foundations of thought and action. A social cognitive theory. Englewood Cliffs, NJ: Prentice Hall.
Bandura, A. (1991). Social cognitive theory of self-regulation. Organ. Behav. Hum. Decis. Process. 50, 248–287. doi: 10.1016/0749-5978(91)90022-L
Bazzana, D., Foltz, J., and Zhang, Y. (2022). Impact of climate smart agriculture on food security: an agent-based analysis. Food Policy 111:102304. doi: 10.1016/j.foodpol.2022.102304
Belay, A., Recha, J. W., Woldeamanuel, T., and Morton, J. F. (2017). Smallholder farmers’ adaptation to climate change and determinants of their adaptation decisions in the central Rift Valley of Ethiopia. Agric. Food Secur. 6, 1–13. doi: 10.1186/s40066-017-0100-1
Bodur, H. O., Brinberg, D., and Coupey, E. (2000). Belief, affect, and attitude: alternative models of the determinants of attitude. J. Consum. Psychol. 9, 17–28. doi: 10.1207/s15327663jcp0901_2
Bozoglu, M., Kilic Topuz, B., Başer, U., Shahbaz, P., and Alhas Eroğlu, N. (2022). Graduate students’ knowledge levels on climate change in the departments of agricultural economics in Turkey. J. Agric. Sci. Technol. 24, 1029–1041. Available at: https://jast.modares.ac.ir/article-23-51290-en.html.
Brown, D. J., Hagger, M. S., Morrissey, S., and Hamilton, K. (2018). Predicting fruit and vegetable consumption in long-haul heavy goods vehicle drivers: application of a multi-theory, dual-phase model and the contribution of past behaviour. Appetite 121, 326–336. doi: 10.1016/j.appet.2017.11.106
Brüssow, K., Faße, A., and Grote, U. (2017). Implications of climate-smart strategy adoption by farm households for food security in Tanzania. Food Secur. 9, 1203–1218. doi: 10.1007/s12571-017-0694-y
Caruana, A., Money, A. H., and Berthon, P. R. (2000). Service quality and satisfaction–the moderating role of value. Eur. J. Mark. 34, 1338–1353. doi: 10.1108/03090560010764432
Chen, M. F. (2016). Extending the theory of planned behavior model to explain people's energy savings and carbon reduction behavioral intentions to mitigate climate change in Taiwan–moral obligation matters. J. Clean. Prod. 112, 1746–1753. doi: 10.1016/j.jclepro.2015.07.043
Chen, J. S., Prebensen, N., and Huan, T. C. (2008). Determining the motivation of wellness travelers. Anatolia 19, 103–115. doi: 10.1080/13032917.2008.9687056
Cheung, M. W. L., and Chan, W. (2005). Meta-analytic structural equation modeling: a two-stage approach. Psychol. Methods 10:40. doi: 10.1037/1082-989X.10.1.40
Close, M. A., Lytle, L. A., Chen, D. G., and Viera, A. J. (2018). Using the theory of planned behavior to explain intention to eat a healthful diet among Southeastern United States office workers. Nutr Food Sci 48, 365–374. doi: 10.1108/NFS-06-2017-0123
Collado, S., and Evans, G. W. (2019). Outcome expectancy: a key factor to understanding childhood exposure to nature and children's pro-environmental behavior. J. Environ. Psychol. 61, 30–36. doi: 10.1016/j.jenvp.2018.12.001
Creswell, J. W. (2009). Research designs: qualitative, quantitative, and mixed methods approaches. United States: Sage publications.
Datta, A., Nayak, D., Smith, J. U., Sharma, P. C., Jat, H. S., Yadav, A. K., et al. (2022). Climate smart agricultural practices improve soil quality through organic carbon enrichment and lower greenhouse gas emissions in farms of bread bowl of India. Soil Res 60, 455–469. doi: 10.1071/SR21031
De Groot, J., and Steg, L. (2007). General beliefs and the theory of planned behavior: the role of environmental concerns in the TPB. J. Appl. Soc. Psychol. 37, 1817–1836. doi: 10.1111/j.1559-1816.2007.00239.x
De Leeuw, A., Valois, P., Ajzen, I., and Schmidt, P. (2015). Using the theory of planned behavior to identify key beliefs underlying pro-environmental behavior in high-school students: implications for educational interventions. J. Environ. Psychol. 42, 128–138. doi: 10.1016/j.jenvp.2015.03.005
Deci, E. L., and Ryan, R. M. (1991). “A motivational approach to self-integration in personality” in Nebraska symposium on motivation. ed. R. Dienstbier, Perspectives on motivation, vol. 38 (Lincoln: University of Nebraska Press), 237–288.
Deci, E. L., and Ryan, R. M. (2000). The “what” and “why” of goal pursuits: human needs and the self-determination of behavior. Psychol. Inq. 11, 227–268. doi: 10.1207/S15327965PLI1104_01
Deci, E. L., and Ryan, R. M. (2008). Facilitating optimal motivation and psychological well-being across life's domains. Can. Psychol. 49:14. doi: 10.1037/0708-5591.49.1.14
Deci, E. L., Vallerand, R. J., Pelletier, L. G., and Ryan, R. M. (1991). Motivation and education: the self-determination perspective. Educ. Psychol. 26, 325–346. doi: 10.1080/00461520.1991.9653137
Descheemaeker, K., Reidsma, P., and Giller, K. E. (2020). “Climate-smart crop production: understanding complexity for achieving triple-wins” in Climate change and agriculture (Cambridge, UK: Burleigh Dodds Science Publishing), 275–318.
Devi, B., Pradhan, S., Giri, D., and Baxodirovna, N. L. (2022). Concept of social cognitive theory and its application in the field of medical and nursing education: framework to guide research. J Posit Schl Psychol. 6, 5161–5168,
Dunlap, R. E., and Van Liere, K. D. (1978). The “new environmental paradigm”. J. Environ. Educ. 9, 10–19. doi: 10.1080/00958964.1978.10801875
Eyni-Nargeseh, H., Deihimfard, R., Rahimi-Moghaddam, S., and Mokhtassi-Bidgoli, A. (2020). Analysis of growth functions that can increase irrigated wheat yield under climate change. Meteorol. Appl. 27, 1–14. doi: 10.1002/met.1804
Gadenne, D., Sharma, B., Kerr, D., and Smith, T. (2011). The influence of consumers’ environmental beliefs and attitudes on energy saving behaviors. Energy Policy 39, 7684–7694. doi: 10.1016/j.enpol.2011.09.002
Gansser, O. A., and Reich, C. S. (2022). Influence of the new ecological paradigm (NEP) and environmental concerns on pro-environmental behavioral intention based on the theory of planned behavior (TPB). J. Clean. Prod. 14:134629. doi: 10.1016/j.jclepro.2022.134629
Gardezi, M., Michael, S., Stock, R., Vij, S., Ogunyiola, A., and Ishtiaque, A. (2022). Prioritizing climate‐smart agriculture: An organizational and temporal review. Wiley Interdiscip. Rev. Clim. Chang. 13:e755. doi: 10.1002/wcc.755
Gauthier, A. J., Guertin, C., and Pelletier, L. G. (2022). Motivated to eat green or your greens? Comparing the role of motivation towards the environment and for eating regulation on ecological eating behaviours–a self-determination theory perspective. Food Qual. Prefer. 99:104570. doi: 10.1016/j.foodqual.2022.104570
Ghalibaf, M. B., Gholami, M., and Ahmadi, S. A. (2023). Climate change, food system, and food security in Iran. J. Agric. Sci. Technol. 25, 1–17. doi: 10.52547/jast.25.1.1
Ghobadi Aliabadi, S., Agahi, H., and Farhadian, H. (2022). Farmers' perceptions of climate change risk: comparing the accuracy of farmers’ perceptions with meteorological data in Kermanshah township. J. Agric. Sci. Technol. 24, 811–823,
Godin, G., Bélanger-Gravel, A., Eccles, M., and Grimshaw, J. (2008). Healthcare professionals' intentions and behaviours: a systematic review of studies based on social cognitive theories. Implement. Sci. 3, 1–12. doi: 10.1186/1748-5908-3-36
Goli, I., Omidi Najafabadi, M., and Lashgarara, F. (2020). Where are we standing and where should we be going? Gender and climate change adaptation behavior. J. Agric. Environ. Ethics 33, 187–218. doi: 10.1007/s10806-020-09822-3
Good, V., Hughes, D. E., Kirca, A. H., and McGrath, S. (2022). A self-determination theory-based meta-analysis on the differential effects of intrinsic and extrinsic motivation on salesperson performance. J. Acad. Market Sci. 50, 586–614. doi: 10.1007/s11747-021-00827-6
Grace, M. A., Achick, T. F. E., Bonghan, B. E., Bih, M. E., Ngo, N. V., Ajeck, M. J., et al. (2019). An overview of the impact of climate change on pathogens, pest of crops on sustainable food biosecurity. Int J Ecotoxicol Ecobiol 4, 114–124. doi: 10.11648/j.ijee.20190404.15
Hagger, M. S., and Chatzisarantis, N. L. D. (2014). An integrated behavior-change model for physical activity. Exerc. Sport Sci. Rev. 42, 62–69. doi: 10.1249/JES.0000000000000008
Hagger, M. S., Cheung, M. W., Ajzen, I., and Hamilton, K. (2022). Perceived behavioral control moderating effects in the theory of planned behavior: a meta-analysis. Health Psychol. 41, 155–167. doi: 10.1037/hea0001153
Hagger, M. S., and Protogerou, C. (2020). “Self‐determination theory and autonomy support to change healthcare behavior” in The Wiley handbook of healthcare treatment engagement: Theory, research, and clinical practice. Eds. A. Hadler, S. Sutton, and L. Osterberg. John Wiley & Sons Ltd. 141–158.
Hagger, M. S., Trost, N., Keech, J. J., Chan, D. K., and Hamilton, K. (2017). Predicting sugar consumption: application of an integrated dual-process, dual-phase model. Appetite 116, 147–156. doi: 10.1016/j.appet.2017.04.032
Han, P., Tong, Z., Sun, Y., and Chen, X. (2022). Impact of climate change beliefs on youths’ engagement in energy-conservation behavior: the mediating mechanism of environmental concerns. Int. J. Environ. Res. Public Health 19:7222. doi: 10.3390/ijerph19127222
Harland, P., Staats, H., and Wilke, H. A. M. (1999). Explaining environmental intention and behavior by personal norms and the theory of planned behavior. J. Appl. Soc. Psychol. 29, 2505–2528. doi: 10.1111/j.1559-1816.1999.tb00123.x
Hartley, K. (2022). “Smartphone-induced digital distractions: using social cognitive theory and self-regulated learning to frame the challenge” in Digital distractions in the college classroom. (Hershey, Pennsylvania: IGI Global), 189–203.
Hasan, M. K., Desiere, S., D’Haese, M., and Kumar, L. (2018). Impact of climate-smart agriculture adoption on the food security of coastal farmers in Bangladesh. Food Secur. 10, 1073–1088. doi: 10.1007/s12571-018-0824-1
Hassan, H. M., Quader, M. S., and Aktar, S. (2022). The role of perceived value and Green consumption attitude on purchase intention of eco-bag: a study on young consumers. J Pengurusan 65:3. doi: 10.17576/pengurusan-2022-65-03
Iran Meteorological Organization. (2022). Available at: https://ndc.irimo.ir/far/wd/10211 (Accessed October 29, 2021).
Iran Statistical Center Website. (2022). Available at: https://www.amar.org.ir/english/Iran-Statistical-Yearbook (Accessed March 27, 2022).
Jamshidi, O., Asadi, A., Kalantari, K., Movahhed Moghaddam, S., Dadrass Javan, F., Azadi, H., et al. (2020). Adaptive capacity of smallholder farmers toward climate change: evidence from Hamadan province in Iran. Clim. Dev. 12, 923–933. doi: 10.1080/17565529.2019.1710097
Jariwala, H., Santos, R. M., Lauzon, J. D., Dutta, A., and Wai Chiang, Y. (2022). Controlled release fertilizers (CRFs) for climate-smart agriculture practices: a comprehensive review on release mechanism, materials, methods of preparation, and effect on environmental parameters. Environ. Sci. Pollut. Res. 28, 1–29. doi: 10.1007/s11356-022-20890-y
Kadkhodazadeh, M., Valikhan Anaraki, M., Morshed-Bozorgdel, A., and Farzin, S. (2022). A new methodology for reference evapotranspiration prediction and uncertainty analysis under climate change conditions based on machine learning, multi criteria decision making and Monte Carlo methods. Sustain. For. 14:2601. doi: 10.3390/su14052601
Khaola, P. P., Potiane, B., and Mokhethi, M. (2014). Environmental concern, attitude towards green products and green purchase intentions of consumers in Lesotho. Ethiop J Environ Stud Manage 7, 361–370. doi: 10.4314/ejesm.v7i4.3
Khatri-Chhetri, A., Aggarwal, P. K., Joshi, P. K., and Vyas, S. (2016). Farmers' prioritization of climate-smart agriculture (CSA) technologies. Agr. Syst. 151, 184–191. doi: 10.1016/j.agsy.2016.10.005
Kilbourne, W., and Pickett, G. (2008). How materialism affects environmental beliefs, concern, and environmentally responsible behavior. J. Bus. Res. 61, 885–893. doi: 10.1016/j.jbusres.2007.09.016
Kumar, A., Kumar, S., Kumar, R., Kumar, R., and Imran, M. (2017). Impact of climate change on plant diseases and their management strategies. J Pharmacogn Phytochem 6, 779–781.
Kungumapriya, A., and Malarmathi, K. (2018). The impact of service quality, perceived value, customer satisfaction in calculative commitment and customer loyalty chain in Indian mobile telephone sector. IOSR J. Bus. Manag. 20, 72–82.
Kwistianus, H., Hatane, S. E., and Rungkat, N. (2020). Environmental concern, attitude and willingness to pay of Green products: Case study in private universities in Surabaya, Indonesia. (Doctoral dissertation, Petra Christian University). Available at: http://repository.petra.ac.id/id/eprint/19029.
Lei, P. W., and Wu, Q. (2007). Introduction to structural equation modeling: issues and practical considerations. Educ. Meas. Issues Pract. 26, 33–43. doi: 10.1111/j.1745-3992.2007.00099.x
Lin, C. Y., Imani, V., Majd, N. R., Ghasemi, Z., Griffiths, M. D., Hamilton, K., et al. (2020). Using an integrated social cognition model to predict COVID‐19 preventive behaviours. Br. J. Health Psychol. 25, 981–1005. doi: 10.1111/bjhp.12465
Lipper, L., McCarthy, N., Zilberman, D., Asfaw, S., and Branca, G. (2017). Climate smart agriculture: Building resilience to climate change. Netherland: Springer Nature.
Luc, P. T. (2020). Outcome expectations and social entrepreneurial intention: integration of planned behavior and social cognitive career theory. J Asian Fin Econ Bus 7, 399–407. doi: 10.13106/jafeb.2020.vol7.no6.399
Makate, C., Makate, M., and Mango, N. (2018). Farm household typology and adoption of climate-smart agriculture practices in smallholder farming systems of southern Africa. African journal of science. Technology. Innov. Dev. 10, 421–439. doi: 10.1080/20421338.2018.1471027
Mashi, S. A., Inkani, A. I., and Obaro, D. O. (2022). Determinants of awareness levels of climate smart agricultural technologies and practices of urban farmers in Kuje, Abuja, Nigeria. Technol. Soc. 70:102030. doi: 10.1016/j.techsoc.2022.102030
Mehra, S., and Ratna, P. A. (2014). Attitude and behaviour of consumers towards organic food: an exploratory study in India. Int. J. Bus. Excell. 7, 677–699. doi: 10.1504/IJBEX.2014.065503
Michler, J. D., Baylis, K., Arends-Kuenning, M., and Mazvimavi, K. (2019). Conservation agriculture and climate resilience. J. Environ. Econ. Manag. 93, 148–169. doi: 10.1016/j.jeem.2018.11.008
Ministry of Iran Agriculture Jihad. (2022). Available at: https://amar.maj.ir/page-amar/FA/65/form/pId11583. (Accessed March 28, 2022).
Mirkhani, R., Vaezi, A., and Rezaei, H. (2020). Using soil properties to estimate the irrigated wheat yield in agricultural lands of Nazarabad region in Alborz Province. Iran J Soil Water Res 51, 1227–1237. doi: 10.22059/ijswr.2020.292897.668404
Momvandi, A., Omidi Najafabadi, M., Hosseini, J. F., and Lashgarara, F. (2018). The identification of factors affecting the use of pressurized irrigation systems by farmers in Iran. Water 10:1532. doi: 10.3390/w10111532
Moon, S. J. (2021). Investigating beliefs, attitudes, and intentions regarding green restaurant patronage: an application of the extended theory of planned behavior with moderating effects of gender and age. Int. J. Hosp. Manag. 92:102727. doi: 10.1016/j.ijhm.2020.102727
Mullan, E., and Markland, D. (1997). Variations in self-determination across the stages of change for exercise in adults. Motiv. Emot. 21, 349–362. doi: 10.1023/A:1024436423492
Najafi Alamdarlo, H., Riyahi, F., and Vakilpoor, M. H. (2019). Wheat self-sufficiency, water restriction and virtual water trade in Iran. Netw. Spat. Econ. 19, 503–520. doi: 10.1007/s11067-018-9383-4
Nanjundeswaraswamy, T. S., and Divakar, S. (2021). Determination of sample size and sampling methods in applied research. Proc Engineer Sci 3, 25–32. doi: 10.24874/PES03.01.003
Naz, S., Jamshed, S., Nisar, Q. A., and Nasir, N. (2022). “Green HRM, psychological green climate and pro-environmental behaviors: an efficacious drive towards environmental performance in China” in Key topics in health, nature, and behavior. (Cham: Springer), 95–110.
Nazari, B., Liaghat, A., Akbari, M. R., and Keshavarz, M. (2018). Irrigation water management in Iran: implications for water use efficiency improvement. Agric Water Manag 208, 7–18. doi: 10.1016/j.agwat.2018.06.003
Nikolaou, G., Neocleous, D., Christou, A., Kitta, E., and Katsoulas, N. (2020). Implementing sustainable irrigation in water-scarce regions under the impact of climate change. Agronomy 10:1120. doi: 10.3390/agronomy10081120
Ojo, A. O. (2022). Motivational factors of pro-environmental behaviors among information technology professionals. Rev. Manag. Sci. 16, 1853–1876. doi: 10.1007/s11846-021-00497-2
Olubukola, G., Oyewole, A., and Ahmed, A. (2022). Climate-Smart Agricultural Practices at Oyo State-Nigeria. South Asian J. 1, 1–7.
Pachauri, R. K., Allen, M. R., Barros, V. R., Broome, J., Cramer, W., Christ, R., et al. (2014). Climate change 2014: synthesis report. In: Contribution of Working Groups I, II and III to the fifth assessment report of the Intergovernmental Panel on Climate Change. IPCC, 2014.
Palombi, L., and Sessa, R. (2013). Climate-smart agriculture: Sourcebook. Rome: Food and Agriculture Organization of the United Nations.
Partey, S. T., Zougmoré, R. B., Ouédraogo, M., and Campbell, B. M. (2018). Developing climate-smart agriculture to face climate variability in West Africa: challenges and lessons learnt. J. Clean. Prod. 187, 285–295. doi: 10.1016/j.jclepro.2018.03.199
Paul, J., Modi, A., and Patel, J. (2016). Predicting green product consumption using theory of planned behavior and reasoned action. J. Retail. Consum. Serv. 29, 123–134. doi: 10.1016/j.jretconser.2015.11.006
Payendeh Najafabadi, A. T., and Omidi Najafabadi, M. (2013). Confirmatory structured analysis. Tehran, Iran: Publishing Center of Shahid Beheshti University of Iran.
Pelletier, L. G., Tuson, K. M., Green‐Demers, I., Noels, K., and Beaton, A. M. (1998). Why are you doing things for the environment? The motivation toward the environment scale (mtes) 1. J. Appl. Soc. Psychol. 28, 437–468. doi: 10.1111/j.1559-1816.1998.tb01714.x
Perry, L. R., Moorhouse, T. P., Jacobsen, K., Loveridge, A. J., and Macdonald, D. W. (2022). More than a feeling: cognitive beliefs and positive—but not negative—affect predict overall attitudes toward predators. Conserv Sci Pract 4:e584. doi: 10.1111/csp2.584
Pickson, R. B., He, G., and Boateng, E. (2022). Impacts of climate change on rice production: evidence from 30 Chinese provinces. Environ. Dev. Sustain. 24, 3907–3925. doi: 10.1007/s10668-021-01594-8
Pörtner, H. O., Roberts, D. C., Adams, H., Adler, C., Aldunce, P., Ali, E., et al. (2022). Climate change 2022: Impacts, adaptation and vulnerability. Geneva, Switzerland: IPCC Sixth Assessment Report.
Potthoff, S., Presseau, J., Sniehotta, F. F., Johnston, M., Elovainio, M., and Avery, L. (2017). Planning to be routine: habit as a mediator of the planning-behaviour relationship in healthcare professionals. Implement. Sci. 12, 1–10. doi: 10.1186/s13012-017-0551-6
Qureshi, M. R. N. M., Almuflih, A. S., Sharma, J., Tyagi, M., Singh, S., and Almakayeel, N. (2022). Assessment of the climate-smart agriculture interventions towards the avenues of sustainable production–consumption. Sustain. For. 14:8410. doi: 10.3390/su14148410
Rahi, S., and ABDGhani, M. (2019). Integration of expectation confirmation theory and self-determination theory in internet banking continuance intention. J Sci Technol Policy Manage 10, 533–550. doi: 10.1108/JSTPM-06-2018-0057
Roos, D., and Hahn, R. (2019). Understanding collaborative consumption: an extension of the theory of planned behavior with value-based personal norms. J. Bus. Ethics 158, 679–697. doi: 10.1007/s10551-017-3675-3
Rosenthal, S. (2022). Information sources, perceived personal experience, and climate change beliefs. J. Environ. Psychol. 81:101796. doi: 10.1016/j.jenvp.2022.101796
Rump, M., Esdar, W., and Wild, E. (2017). Individual differences in the effects of academic motivation on higher education students’ intention to drop out. Eur J High Educ 7, 341–355. doi: 10.1080/21568235.2017.1357481
Scholz, U., and Lippke, S. (2008). Social-cognitive predictors of physical exercise adherence: three longitudinal studies in rehabilitation. Am Psychol Assoc 27:S54. doi: 10.1037/0278-6133.27.1(Suppl.).S54
Scholz, U., Schüz, B., Ziegelmann, J. P., Lippke, S., and Schwarzer, R. (2008). Beyond behavioural intentions: planning mediates between intentions and physical activity. Br. J. Health Psychol. 13, 479–494. doi: 10.1348/135910707X216062
Schumacker, R. E., and Lomax, R. G. (2004). A beginner's guide to structural equation modeling. Oxfordshire: Psychology press.
Senyolo, M. P., Long, T. B., Blok, V., and Omta, O. (2018). How the characteristics of innovations impact their adoption: an exploration of climate-smart agricultural innovations in South Africa. J. Clean. Prod. 172, 3825–3840. doi: 10.1016/j.jclepro.2017.06.019
Shuell, T. J. (1986). Cognitive conceptions of learning. Rev. Educ. Res. 56, 411–436. doi: 10.3102/00346543056004411
Small, B., and Maseyk, F. J. (2022). Understanding farmer behaviour. N. Z. J. Ecol. 46, 1–11. doi: 10.20417/nzjecol.46.20
Sniehotta, F. F., Scholz, U., Schwarzer, R., Fuhrmann, B., Kiwus, U., and Völler, H. (2005). Long-term effects of two psychological interventions on physical exercise and self-regulation following coronary rehabilitation. Int. J. Behav. Med. 12, 244–255. doi: 10.1207/s15327558ijbm1204_5
Straughan, R. D., and Roberts, J. A. (1999). Environmental segmentation alternatives: a look at green consumer behavior in the new millennium. J. Consum. Mark. 16, 558–575. doi: 10.1108/07363769910297506
Strong, C., Lin, C. Y., Jalilolghadr, S., Updegraff, J. A., Broström, A., and Pakpour, A. H. (2018). Sleep hygiene behaviours in Iranian adolescents: an application of the theory of planned behavior. J. Sleep Res. 27, 23–31. doi: 10.1111/jsr.12566
Strydom, W. F. (2018). Applying the theory of planned behavior to recycling behavior in South Africa. Recycling 3:43. doi: 10.3390/recycling3030043
Sun, H., Teh, P. L., and Linton, J. D. (2018). Impact of environmental knowledge and product quality on student attitude toward products with recycled/remanufactured content: implications for environmental education and green manufacturing. Bus. Strateg. Environ. 27, 935–945. doi: 10.1002/bse.2043
Taimour, H., Farhadian, H., and Saadvandi, M. (2022). Farmers’ agreement to apply and willingness to pay for climate-smart agricultural Technologies at the Farm Level. J. Agric. Sci. Technol. 24, 779–791.
Tama, R. A., Ying, L., Yu, M., Hoque, M. M., Adnan, K. M., and Sarker, S. A. (2021). Assessing farmers’ intention towards conservation agriculture by using the extended theory of planned behavior. J. Environ. Manage. 280:111654. doi: 10.1016/j.jenvman.2020.111654
Thornton, P. K., Whitbread, A., Baedeker, T., Cairns, J., Claessens, L., Baethgen, W., et al. (2018). A framework for priority-setting in climate smart agriculture research. Agr. Syst. 167, 161–175. doi: 10.1016/j.agsy.2018.09.009
Tiamiyu, S. A., Ugalahi, U. B., Eze, J. N., and Shittu, M. A. (2018). Adoption of climate smart agricultural practices and farmers’ willingness to accept incentives in Nigeria. Int J Agric Environ Res 4, 198–205.
Valizadeh, N., Hayati, D., Rezaei-Moghaddam, K., and Karimi-Gougheri, H. (2018). Application of the planned behavior theory in ethical analysis of water conservation behavior. Bioethics J. 8, 33–48.
Venkatesh, V., Morris, M. G., Davis, G. B., and Davis, F. D. (2003). User acceptance of information technology: toward a unified view. MIS Q. 1, 425–478. doi: 10.2307/30036540
Venkatramanan, V., and Shah, S. (2019). “Climate smart agriculture technologies for environmental management: the intersection of sustainability, resilience, wellbeing and development” in Sustainable green technologies for environmental management (Singapore: Springer), 29–51.
Vorobyova, K., Alkadash, T. M., and Nadam, C. (2022). Investigating beliefs, attitudes, and intentions regarding strategic decision-making process: an application of theory planned behavior with moderating effects of overconfidence and confirmation biases. Specialusis Ugdymas 1, 367–381.
Waaswa, A., Oywaya Nkurumwa, A., Mwangi Kibe, A., and Ngeno Kipkemoi, J. (2022). Climate-smart agriculture and potato production in Kenya: review of the determinants of practice. Clim. Dev. 14, 75–90. doi: 10.1080/17565529.2021.1885336
Wang, B., and Li, Y. (2022). Consumers’ intention to bring a reusable bag for shopping in China: extending the theory of planned behavior. Int. J. Environ. Res. Public Health 19:3638. doi: 10.3390/ijerph19063638
Wang, X., Lin, X., and Spencer, M. K. (2019). Exploring the effects of extrinsic motivation on consumer behaviors in social commerce: revealing consumers’ perceptions of social commerce benefits. Int. J. Inf. Manag. 45, 163–175. doi: 10.1016/j.ijinfomgt.2018.11.010
Weston, R., and Gore, P. A. Jr. (2006). A brief guide to structural equation modeling. Couns. Psychol. 34, 719–751. doi: 10.1177/0011000006286345
Williams, L. A., Sun, J., and Masser, B. (2019). Integrating self‐determination theory and the theory of planned behaviour to predict intention to donate blood. Transfus. Med. 29, 59–64. doi: 10.1111/tme.12566
Wilson, P. M., Rodgers, W. M., and Fraser, S. N. (2002). Examining the psychometric properties of the behavioral regulation in exercise questionnaire. Meas. Phys. Educ. Exerc. Sci. 6, 1–21. doi: 10.1207/S15327841MPEE0601_1
Wu, L., Zhu, Y., and Zhai, J. (2022). Understanding waste management behavior among university students in China: environmental knowledge, personal norms, and the theory of planned behavior. Front. Psychol. 12:771723. doi: 10.3389/fpsyg.2021.771723
Wylde, E. (2022). Value for Money of social assistance in fragile contexts: considerations, evidence, and research priorities. Available at: https://opendocs.ids.ac.uk/opendocs/handle/20.500.12413/17205
Xu, Z., Shan, J., Li, J., and Zhang, W. (2020). Extending the theory of planned behavior to predict public participation behavior in air pollution control: Beijing, China. J. Environ. Plan. Manag. 63, 669–688. doi: 10.1080/09640568.2019.1603821
Xu, K., Yan, X., and Newman, M. W. (2022). Understanding People’s experience for physical activity planning and exploring the impact of historical records on plan creation and execution. In CHI Conference on Human Factors in Computing Systems (pp. 1–15).
Yaghoubi, J., Yazdanpanah, M., and Komendantova, N. (2019). Iranian agriculture advisors' perception and intention toward biofuel: Green way toward energy security, rural development and climate change mitigation. Renew. Energy 130, 452–459. doi: 10.1016/j.renene.2018.06.081
Yousefi Khanghah, S., Azarnivand, H., Chahoki, Z., Jafari, M., and Naseri, H. R. (2020). The effect of amendments on the physical and chemical properties of soil in salt-land of Nazarabad, Alborz Province. Desert 25, 123–130. doi: 10.22059/jdesert.2020.78172
Zhang, Y., Xiao, C., and Zhou, G. (2020). Willingness to pay a price premium for energy-saving appliances: role of perceived value and energy efficiency labeling. J. Clean. Prod. 242:118555. doi: 10.1016/j.jclepro.2019.118555
Keywords: climate change, climate-smart agriculture, theory of planned behavior, self-determination theory, social cognition theory
Citation: Khademi Noshabadi SM, Omidi Najafabadi M and Mirdamadi SM (2024) Uptake of climate-smart agricultural technologies and practices: a three-phase behavioral model. Front. Sustain. Food Syst. 8:1389368. doi: 10.3389/fsufs.2024.1389368
Received: 21 February 2024; Accepted: 25 April 2024;
Published: 30 May 2024.
Edited by:
Priscilla Ntuchu Kephe, Potsdam Institute for Climate Impact Research (PIK), GermanyReviewed by:
Raffaella Pergamo, Council for Agricultural and Economics Research (CREA), ItalyCopyright © 2024 Khademi Noshabadi, Omidi Najafabadi and Mirdamadi. This is an open-access article distributed under the terms of the Creative Commons Attribution License (CC BY). The use, distribution or reproduction in other forums is permitted, provided the original author(s) and the copyright owner(s) are credited and that the original publication in this journal is cited, in accordance with accepted academic practice. No use, distribution or reproduction is permitted which does not comply with these terms.
*Correspondence: M. Omidi Najafabadi, bS5vbWlkaUBzcmJpYXUuYWMuaXI=
Disclaimer: All claims expressed in this article are solely those of the authors and do not necessarily represent those of their affiliated organizations, or those of the publisher, the editors and the reviewers. Any product that may be evaluated in this article or claim that may be made by its manufacturer is not guaranteed or endorsed by the publisher.
Research integrity at Frontiers
Learn more about the work of our research integrity team to safeguard the quality of each article we publish.