- 1Department of International Environment and Development Studies, Norwegian University of Life Science, As, Norway
- 2School of Computing, Mathematics and Engineering, Charles Sturt University, NSW, Australia
- 3Graham Centre for Agricultural Innovations, Charles Sturt University Wagga, Wagga, NSW, Australia
- 4Department of Agribusiness Management, Lilongwe University of Agriculture and Natural Resources, Lilongwe, Malawi
Household food security and income play central roles in sustainable development at the global, national, and local levels. Smallholder vegetable farming systems are widely regarded as crucial contributors to enhancing household food security and income in the developing world. Comprehensive whole-farm analysis of smallholder vegetable farming systems points out greater diversity and heterogeneity driven by interactions of socioeconomic and biophysical factors such as land profiles, land use, farm assets, enterprise income, off/non-farm activities, and household structure and expenditure. However, three distinct farm typologies exist (Resource and Livelihood Constrained Off-farm Dependent/Supplemented–RLCOD farmers, Resource and Livelihood Abundant and Commercial Oriented–RLACO farmers, and Medium Resources and Livelihoods Constrained Market Oriented–MRLCMO farmers), exhibiting significant differences and variability in structural and functional factors. The production of maize, groundnuts, soybean, and bean crops remains the primary source of livelihood for all farm typologies. Similarly, vegetable production is vital for farmers’ livelihoods in all farm types, yielding more income than food needs. However, both crop and vegetable productivity and production are still low and below the national and potential target across farm typologies. Small-scale irrigation offers one alternative solution in the context of increasing climate change. Small livestock (chickens, goats, and pigs) play a significant role in the livelihood of vegetable farmers despite their low productivity. Disparities exist across farm typologies in available arable and irrigable land, available household labor, capacity to hire additional labor, farm assets, and inputs, as well as participation in off-farm and non-labor-farm activities. While vegetable production and its proportion sold and earned income emerge as critical factors affecting household food accessibility and acquisition, additional factors such as crop production, proportion of crops sold, daily food expenditure, livestock income, and TLU significantly influence household access for different farm typologies, requiring consideration to achieve food security in vegetable farming systems.
1 Introduction
Smallholder farming systems are key in enhancing food security and income in developing countries (Guarín et al., 2020), contributing 70 to 80% of food production and about 60% of household income (Fan and Rue, 2020; Hazell, 2020; Musafiri et al., 2020). Consequently, smallholder agriculture in the developing world is viewed as a crucial pathway for maintaining agrobiodiversity for achieving sustainable food security and income (Forsythe, 2017; Guarín et al., 2020). Smallholder farmers can conserve and use agrobiodiversity in their farming practices to build farmer resilience and enhance agricultural productivity, thereby increasing food production and income, and improving ecosystem services (Thrupp, 2000). Built upon the premise that smallholder farmers represent a significant segment of the population and cultivate a considerable portion of farmland, the pathway for smallholder agriculture development is boosting productivity, stimulating rural economies, advocating for the conservation of natural resources, and facilitating access to support from state and non-state entities (Kamara et al., 2019; Hazell, 2020).
Smallholder vegetable farming systems emerge as crucial contributors to enhancing food security by diversifying diets, meeting micronutrient needs, and generating income (Rajendran et al., 2017). By enhancing the diversification and consumption of primarily vegetable-based diets improved human health can be achieved (Ali and Abedullah., 2002). Micronutrients play a key role in balancing diets and combating malnutrition, a factor associated with a significant number of premature deaths worldwide (Mlalama et al., 2022). Moreover, vegetable production has the potential to stimulate business ventures, providing avenues for employment and income. However, the global supply of vegetables appears inadequate to meet the World Health Organization’s (WHO) minimum daily requirements (400 grams per person) for approximately 55% of the global population (Lucia et al., 2019). This situation is particularly critical in sub-Saharan Africa (SSA), where approximately 956 million people fall short of the recommended minimum daily consumption. Projections indicate that by 2050, over 1 billion people in SSA may be unable to meet the minimum daily intake of vegetables (Lucia et al., 2019; Mlalama et al., 2022). Considering further projections that by 2050, the global population will need 70% more food for human consumption than is consumed today to ensure healthy societies (Cole et al., 2018), addressing constraints in both vegetable production and consumption becomes imperative. This requires a transformation of vegetable farming systems through targeted interventions and investments, aiming to increase vegetable production among smallholder farmers and promote the consumption of healthy diets (Ebert, 2017; Gelli et al., 2020).
Smallholder vegetable farmers, while sharing certain common characteristics, such as the presence of vegetable enterprises on their farms, exhibit a notable diversity and heterogeneity in terms of resource endowments, dominant patterns of farm activities, and livelihoods diversity (Dixon et al., 2001; Tittonell et al., 2010; Guarín et al., 2020). The arrangement and management of resources, along with their flows, also appear to differ among smallholder farmers (Kuivanen et al., 2016a). This heterogeneity results in varying capabilities, incentives, aspirations, and agricultural needs for achieving food security and income among smallholder farmers (Gassner et al., 2019). Given that smallholder vegetable farmers also engage in non-vegetable crops, livestock, off-farm, and non-farm enterprises, understanding the whole-farm context of diverse smallholder vegetable farm systems and how they influence sustainable food security and income becomes crucial. The utilization of farm typologies offers a method of capturing and comprehending the complexity, diversity, and heterogeneity of farming systems by classifying farms into homogeneous groups with common characteristics (Kuivanen et al., 2016a,b; Kumar et al., 2019; Shukla et al., 2019; Guarín et al., 2020; Musafiri et al., 2020). These farms can be grouped based on shared resource endowments, such as land and capital, as well as the production and livelihoods orientation of the farms (Tittonell et al., 2010; Goswami et al., 2014; Kuivanen et al., 2016a). The choice of factors for classifying farming systems depends on the objective or purpose of classification, such as understanding the adoption of technologies and practices, resource use efficiency, and food security. These factors are considered influential in understanding the specific diversity and heterogeneity among various types of smallholder farmers (Tittonell et al., 2010; Kumar et al., 2019; Shukla et al., 2019; Musafiri et al., 2020; Innazent et al., 2022).
Some studies have classified farming systems using solely structural criteria that pertain to the physical and tangible aspects of farming or exclusively functional criteria that relate to the roles and operations within farming systems (Tittonell et al., 2010; Kumar et al., 2019). In contrast, other researchers have opted to use both structural and functional criteria for classifying farming systems, aiming to provide more comprehensive insights that reflect the realities of farms and their acceptability to policymakers (Goswami et al., 2014; Kuivanen et al., 2016b; Lopez-Ridaura et al., 2018; Shukla et al., 2019; Musafiri et al., 2020). The integration of structural and functional factors in classifying smallholder farming systems allows for capturing interactions that exist between social, economic, and biophysical factors contributing to the diversity and heterogeneity of smallholder farmers (Innazent et al., 2022). The resulting farm types are conceptually valid and relevant, representative of the farming system, and easily identified among farming households (Innazent et al., 2022). These typologies can be employed for in-depth analyses, modeling, and simulation of farms (Kuivanen et al., 2016a; Innazent et al., 2022). Furthermore, the resulting farm typologies can reveal drivers and patterns of diversity, illustrating their linkages to livelihood strategies, food security, and income (Shukla et al., 2019), as well as household farm strategies, such as land use plans and farm management techniques, and participation in innovative platforms (Tittonell et al., 2010; Guillem et al., 2012). They can also help identify opportunities and constraints within each farm system, guiding the formulation of appropriate pro-poor policies and development activities, including considerations of enterprise combinations, production systems, innovations, and agricultural technologies, for economic and environmental viability and sustainability (Tittonell et al., 2010; Kuivanen et al., 2016a; Varadan et al., 2022).
In Malawi, where approximately 65% of the population is engaged in farming, and smallholder farmers account for over 75% of food consumed (Mango et al., 2018a,b), vegetables play a crucial role, being the most widely produced horticultural crops (Mwandira, 2008). Common types of exotic vegetables in Malawi include cabbage, Chinese cabbage, rape, mustard, tomato, onion, garlic, okra, carrot, green pepper, eggplant, cucumber, turnips, lettuce, cauliflower, and broccoli. These vegetables thrive throughout the country in well-drained, friable, fertile soils, under suitable environmental conditions, and with adequate soil moisture supplied through various irrigation technologies such as watering cans, buckets, treadle pumps, motorized pumps, solar pumps, gravity-lined canals, sprinklers, and micro drip irrigation equipment (Chadha et al., 2008). Vegetables hold a significant place in the Malawian diet, providing essential vitamins and minerals for good health (Chadha et al., 2008), and serving as a source of income for farmers. This income allows farmers to access farm inputs, leading to increased output and a more diversified food supply for food security (Government of Malawi, 2021a). Recognized for their potential to improve human nutrition, boost farmers’ income, and reduce poverty in rural areas of Malawi (Government of Malawi, 2016a, 2022; Benfica and Thurlow, 2017), vegetables are also identified as priority commodities to enhance export revenue (Government of Malawi, 2021b). The national goal is thus to increase vegetable production to meet domestic demand, improve the nutrition status of both rural and urban populations, and satisfying export demand (Government of Malawi, 2021a, 2022).
We adopt the Food and Agriculture Organization’s (FAO) definition of food security which refers to the “situation when all people always have physical, social, and economic access to sufficient, safe, and nutritious food, to meet their dietary needs and food preferences for an active and healthy life” (FAO, 2021a). Currently, food security is conceptualized and evaluated in food systems in terms of six dimensions: availability, accessibility, utilization, stability, agency, and sustainability (FAO, 2021b). Despite this comprehensive framework, most farming systems analyses have primarily focused on food availability, with only a limited number of studies exploring the linkages between farming systems and food accessibility (Nicholson et al., 2021). In this study, we specifically focus on the food accessibility component of food security. Food accessibility refers to an individual’s or household’s physical and economic access to food, revealing the patterns and processes of food distribution to individuals or households. This assessment incorporates considerations of physical access, economic feasibility, safety, cultural acceptability, and household acquisition (Nicholson et al., 2021).
This paper explores the diversity and heterogeneity of smallholder vegetable farming systems in Malawi, aiming to uncover patterns and diverse livelihood strategies that contribute to enhancing food security and income within the resource boundaries of farmers. The objectives of the paper are threefold: (1) to characterize vegetable farm systems (farm typologies); (2) to outline patterns and livelihood strategies within these farm typologies; and (3) to examine the impact of vegetable production on stimulating accessibility and acquisition of food and income.
Recognizing that the definition of smallholder farmers varies across countries and agroecological zones (Bakhsh et al., 2021), we define smallholder farmers as households that operate up to 15 acres of land and largely depend on family labor for their production portfolio to meet food and income security needs. This definition aligns with the criteria set forth by Malawi’s Ministry of Agriculture, Irrigation and Water Development, which categorizes farmers based on landholding sizes into three: 0 to 15 acres (Smallholder farmers), ≥15 to 50 acres (Medium scale farmers) and ≥ 50 Acres (Large scale farmers). We address the study objectives through a comprehensive whole-farm approach, analyzing the household composition and characteristics, farming and non-farming resources and activities, food security status, income strategies, and their interactions and interrelationships between components parts of farm systems. However, we acknowledge the approach’s dependance on farmer’s recall, records, and their accuracy in providing data. This approach also confines to the farm and farm systems boundaries and requires entire look of the whole system rather than its components. It also regards common elements of biophysical systems and external influences on the farms as well as common problems, relationships, and farm adjustment possibilities. Additionally, the collection of detailed, up-to-date, and consistent data in given time and resources, and aggregation of data that conceals individual farm variability in a diverse farming system, all pose limitations of the approach to the study. The subsequent sections of the paper delineate the materials and methods, followed by results, discussion, conclusion, and policy implications.
2 Materials and methods
2.1 Study area/sites
The study was conducted in six districts across Malawi’s Central and Northern regions, namely Kasungu, Dowa, Mchinji, Mzimba North, Mzimba South, and Rumphi. Mzimba South, Mzimba North, and Dowa are major vegetable-producing districts in Malawi, while Rumphi, Kasungu, and Mchinji are considered potential districts for vegetable production (Mwandira, 2008). Each district is administratively and agroecologically divided into Extension Planning Areas (EPAs). The specific EPAs chosen for this study were: Champhira in Mzimba South, Bwengu in Mzimba North, Mhuju in Rumphi, Chiosya in Mchinji, Kaluluma in Kasungu, and Mvera in Dowa (Figure 1). Figure 1 was produced with data from the Department of Land Resources Conservation of the Ministry of Agriculture. Each EPA is further divided into administrative sections. Supplementary material S1 provides the names of the sections within each EPA where the study was conducted.
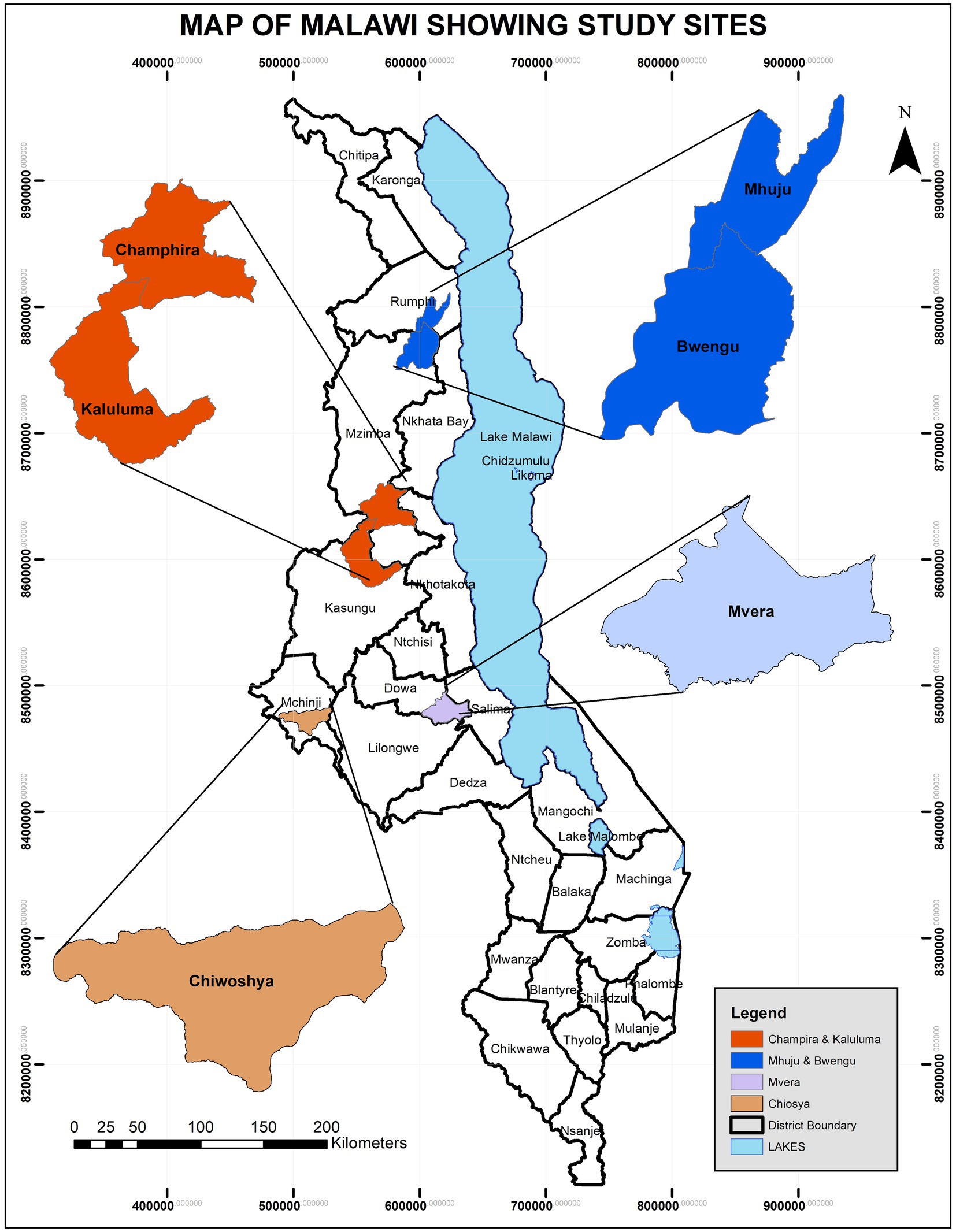
Figure 1. Study sites in the Central and Northern Regions of Malawi. Data source: Department of land resources conservation of the ministry of agriculture, irrigation and water development.
Mvera and Mhuju EPAs are situated in high-altitude areas at an elevation of 1,350 m above sea level, predominantly characterized by lithosol soils. The high-altitude areas experience higher annual rainfall, ranging between 1,000 mL and 3,000 mL, with an average temperature of 13 degrees Celsius. On the other hand, Champhira, Bwengu, Kaluluma, and Chioshya EPAs represent medium-altitude plateau areas, with elevations ranging from 750 m to 1,350 m above sea level. These areas are characterized by well-drained latosol soils on upland sites and poorly drained hydromorphic soils in rivers and streams. In the medium-altitude areas, annual rainfall ranges from 760 mL to 1,000 mL, and average temperatures fluctuate between 19 and 21 degrees Celsius. All the studied EPAs provide a suitable environment for vegetable production (Government of Malawi, 2010).
2.2 Study design
The study employed a cross-sectional household survey design, a widely adopted approach in agricultural and nutrition research for estimating the prevalence of outcomes, practices, and intervention coverage (Carletto et al., 2015). This type of survey was deemed most suitable for this study due to its cost-effectiveness, expediency, and compatibility with the study’s timeframe and budget compared to longitudinal designs. Additionally, cross-sectional surveys enable the determination of sources and drivers of diversity and heterogeneity, livelihood strategies, and food security among vegetable farmers at a specific point in time (Carletto et al., 2015). However, it is essential to acknowledge potential issues related to recall and seasonality biases, particularly if data collection occurs during a specific period of the year (Levin, 2006; Babbie, 2020).
2.3 Sampling design
The study employed a multistage sampling design. Firstly, six districts were purposively selected based on their significance in vegetable production. Secondly, we purposively selected vegetable-producing EPAs within each district, considering household dependence on agriculture and vegetables for food security and income, poverty levels, and diversity of production systems (Norwegian Church Aid, 2020). This selection process was conducted in consultation with partners, local agricultural extension agents, and project baseline reports. The third stage involved the random selection of three Sections in each EPA, as listed in Supplementary material S1. Finally, smallholder vegetable farmers were randomly chosen by the researcher from the database of smallholder vegetable producers in the Section maintained by local agricultural extension offices. This approach, incorporating random sampling and a large geographically dispersed sample, aimed to minimize potential biases in the study.
The chosen study sections include a total of 28,905 smallholder farming households, among which 13,064 (45%) are engaged in vegetable production (Supplementary material S1). The proportion of smallholder farmers producing vegetables in the study sites (0.45), total smallholder farmers in the study sites (28905), z-statistic corresponding with a 95% confidence level (z = 1.96) and confidence interval (error allowance of 5%) were used to compute the required sample size for the study using the recommended adapted statistical formula (Kothari, 2004). The calculated sample size was 376 smallholder vegetable farmers. However, to enhance accuracy, a sample size of 429 farmers was employed. This final sample size was deemed adequate for conducting a cross-sectional survey, ensuring efficient, representative, reliable, and flexible statistical analysis of the target population.
2.4 Questionnaire development and pre-testing
The questionnaire was crafted in alignment with the study objectives, the types of data needed, and the planned analysis. It included questions covering household composition and characteristics, crops, vegetables, fruit, and livestock production, as well as post-production practices. It also included household farm labor, resources (natural, physical, financial, and communal shared), off-farm and non-farm activities, and food security and nutrition. These questions were adapted from previous national and local surveys (Government of Malawi, 2017; Norwegian Church Aid, 2020). To ensure the questionnaire’s effectiveness, the initial draft underwent an expert review by study collaborators. It was also pretested with 34 farmers to assess aspects such as length, clarity, flow, and cultural appropriateness. Feedback from the pretest was incorporated into the final version of the questionnaire. The final questionnaire was then programmed into the World Bank Group’s Survey Solution Software for data collection on tablets.
2.5 Data collection
Data collection took place from October to December 2022, facilitated by a team of 12 graduate and postgraduate research assistants. To prepare the team, a comprehensive 5-day training session covered the study objectives, questionnaire content, interview techniques, and ethical considerations. Additionally, the research assistants actively participated in the two-day pretesting of the questionnaire. All members of the research assistant team had prior experience in survey work. A researcher supervised all field activities, throughout the data collection period, overseeing the research assistants and conducting regular reviews of incoming data for quality assurance. Feedback on any issues or concerns was provided to each research assistant to ensure data accuracy and integrity.
2.6 Data analysis
We employed Stata statistical software version 17 to analyze the data (StataCorp, 2021). Before the analysis, data underwent cleaning and pre-processing to identify and address errors, inconsistencies, and outlier values that could improperly influence and distort results. As a rule of thumb, outliers were defined as observations more than 1.5* Interquartile Range (IQR) below the first quartile or above the third quartile. Thus, outliers were those observations below Q1-1.5*IQR and above Q3 + 1.5*IQR. All identified outliers were removed and subjected to winsorization. Quantitative continuous variables for principal component analysis were normalized. Following the exploratory analyses, we employed two widely used multivariate statistical techniques, Principal Component Analysis (PCA) and Cluster Analysis (CA), to detect similarities and differences across several structural and functional characteristics (Guarín et al., 2020) and to categorize vegetable farming systems (Kuivanen et al., 2016b). PCA served as a dimension reduction technique for handling numerous highly correlated variables by transforming them into uncorrelated principal components that account for maximal variance (Jollife and Cadima, 2016). Only principal components meeting the Kaiser criterion (with an eigenvalue exceeding 1.00), screeplot inflection point, 60% minimum cumulative percentage of variance, and demonstrating conceptual meaning or interpretability in relation to the vegetable farming system were retained (Goswami et al., 2014; Kuivanen et al., 2016a). We used orthogonal rotation (varimax method) to identify a smaller number of highly correlated variables under each component for easy interpretation (Goswami et al., 2014).
Using the retained components from PCA, we applied a two-step clustering approach, in CA, to classify smallholder farmers into clusters of vegetable farming systems. Firstly, we conducted hierarchical agglomerative clustering using Euclidean distance and Ward’s method on scores to define the appropriate number of groups or clusters (k) based on the dendrogram (Goswami et al., 2014). We determined the optimal cluster cut-off point for the dendrogram by assessing the level of dissimilarity between clusters, where each cluster maximizes both intra-cluster similarity (homogeneity) and inter-cluster differences (heterogeneity; Kuivanen et al., 2016a; Lopez-Ridaura et al., 2019). Secondly, we used the number of clusters from the Ward’s method to perform k-means (non-hierarchical) clustering to delineate and validate an optimal number of farm types based on their meaning and relevance. The combination of hierarchical followed by k-means techniques provides robust clustering solutions (Everitt et al., 2011). Cross-tabulation and Descriptive statistics were performed to profile, characterize, and understand the structure and behavior of each cluster (Innazent et al., 2022). Additionally, we conducted a one-way analysis of variance (ANOVA) to test for significant differences across clusters and identify the variables responsible for differences among clusters (Kumar et al., 2019). ANOVA addresses issues of multiple pairwise testing (Type I error) by analyzing variance between and within groups (Seltman, 2018).
Following the construction of farm typologies, food accessibility, and acquisition were evaluated for each farm typology. While various measures of food accessibility exist, such as Household Food Security Scale (HFSS), Household Food Insecurity Access Scale (HFIAS), Food Insecurity Experience Scale (FIES), Household Hunger Scale (HHS), and Coping Strategies Index (CSI), we employed the Food Consumption Score (FCS) and Household Dietary Diversity Score (HDDS) because of their ability to capture multiple dimensions of food accessibility and acquisition, and for being less time-consuming and costly to collect data for their construction. The FCS was determined by multiplying the household consumption frequency of eight food groups (the number of days a particular food was consumed) in a week by their respective assigned weights and summing up the values. The resulting household FCS is then compared against the minimum standard score of 35 to assess food security status. The HDDS was determined using 12 equally weighted food groups (each food group assigned an equal weight of (1) over the past 12 h, 24 h, and 7 days and compared to the population distribution of scores for these groups (Kennedy et al., 2010). Supplementary material S2 provides details on the food groups and weights used in constructing these scores. One-way analysis of variance FCS and HDDS scores was conducted to test for significant differences across farm typologies (Kumar et al., 2019). Furthermore, a multiple linear regression model was employed to examine the contribution of different variables to food accessibility and acquisition in each farm typology. Specifically, each of FCS and HDDS was regressed over the combination of vegetable, crop, livestock, off-farm, non-farm, and household socioeconomic factors as predictors. Assumptions of normality, linearity, homoscedasticity, and absence of multicollinearity were checked before analysis. Bootstrapping, a resampling technique, was used to derive robust standard errors and confidence intervals where assumptions were violated (Fox and Weisberg, 2018).
2.7 Selection of variables for farmer typology construction
Generally, the purpose of constructing farm typologies guides the process of variable selection, with factors proven to affect the diversity of farmers being chosen. Additionally, the use of local expert and stakeholder knowledge is beneficial in selecting variables (Pacini et al., 2014). The selection of variables in this study was informed by the diversity in smallholder farming households in Malawi, rooted in inequalities in resource endowments, access to institutions and markets, and socioeconomic vulnerability (Makate and Mango, 2017). Households well-endowed with resources are more likely to engage in diversified enterprises for food security and income compared to less-endowed households. However, households with similar resources and opportunities do not always choose the same portfolio of farming activities due to differences in smallholder farmers’ objective functions (related to income, consumption, and other livelihoods) and socioeconomic characteristics (such as wealth, power, ethnicity, and social class; Makate and Mango, 2017). Thus, a combination of farm, off-farm, and non-farm activities, along with socioeconomic factors, constitutes major sources of diversity among smallholder farmers. We defined the diversity and heterogeneity of vegetable farming systems as a function of 27 structural and functional variables, induced and stimulated by both internal and external characteristics, respectively (Shukla et al., 2019).
3 Results
3.1 Principal component analysis and cluster analysis
The PCA yielded 11 components whose eigenvalues were above one (Figure 2), in accordance with the Kaiser criterion. These 11 components collectively explain 76.61% of the total variations in smallholder vegetable farming systems (Supplementary material S3A). To facilitate the interpretation of these 11 components, the orthogonal rotation using the Varimax Method, identified a smaller number of highly correlated variables under each component (Supplementary material S3B). All correlation coefficients between the original variables and the principal components with an absolute value greater than or equal to 0.30 were retained for interpretation.
Components 1 and 2 represent Rainfed Crop Production on Arable Land and Irrigated Vegetable Production and Income, respectively. Component 1 explains 14.9% of variations among smallholder vegetable farmers. It shows variations that exist because of differences in arable land utilization driven by the size of arable land farmed and rented, and the extent of rainfed crop production (number of crop enterprises farmed and their land allocation). Component 2 explains 10.4% of variations among vegetable farmers. This component shows that differences in land size under irrigated vegetable production, types of vegetable enterprises cultivated, areas allocated to each vegetable enterprise, extent of vegetables sold, and amount of income earned from the sales of vegetables also contribute to variations among vegetable farmers.
Components 3 and 4 are associated with Arable Land Profile and Off-farm Participation and Income, respectively. Arable land profile accounts for 6.3% of variations among smallholder vegetable farmers. This component showcases distinctions in the sizes of owned land, arable land, and arable land left uncultivated by smallholder vegetable farmers. Off-farm participation or involvement in off-farm activities, encompassing casual labor, cash for work, social cash transfer programs, public works programs, sales of honey, charcoal and firewood, and inputs for work, along with their earned income, elucidates 6.1% of variations among smallholder vegetable farming systems.
Components 5, 6 and 7 are linked with Irrigated Crop Production, Non-farm Participation and Income, and Irrigable Land Profile, respectively. Component 5 elucidates 6.1% of variations among smallholder farmers. It highlights the extent of irrigated land utilization for crop production, excluding vegetables, by examining the land allocated to various crop enterprises. The size of land allocated to crops is positively related to the size of the farmed irrigated land. Therefore, an increase in irrigated land not only augments the land allocated to vegetables but also to other crops. Component 6 explains 6% of variations among vegetable farmers and is characterized by participation in non-farm activities, including formal employment, business or petty trading, artisan work (such as building and carpentry), and non-farm value-adding activities, along with their corresponding income earnings. Component 7 accounts for 5.9% of variations among smallholder vegetable farmers. This component reveals that variations among farmers are influenced by differences in the size of the irrigable land owned and the size of irrigable land that remains unused by smallholder vegetable farmers.
Components 8 and 9 embody Household Size and Family Labor, and Crop Income, respectively. Component 8 accounts for 5.8% of variations among farmers, highlighting household size and extent of family labor as key socioeconomic variables explaining variations among smallholder vegetable farmers. This component provides insights into household characteristics, working dynamics, and the productivity of family members. Component 9 explains 5.8% of variations among farmers. It illustrates variations attributed to differences in the proportion of crops sold and the income earned by the vegetable farmer. This component highlights distinctions in the objectives of crop farming and their alignment with market-oriented strategies.
Components 10 and 11 are related to Rented Irrigable Land and Daily Food Expenditure, and Household Farm Assets, respectively. Component 10 explains5.1% of the variations among farmers. The variations that arise due to differences in the size of the rented land and the amount of daily food expenditure. The higher the daily food expenditure, the greater the amount of land needed to be rented for irrigated production, possibly indicating higher food requirements. Component 11 is the final component that explains 4% of variations among smallholder vegetable farmers. This component reflects the differences in farm assets, their economic value, and how they influence the farming system.
These 11 components have revealed several structural and functional factors that explain a greater diversity and heterogeneity among smallholder vegetable farmers. However, the CA which maximizes both intra-group similarity and inter-group differences yields a dendrogram (Figure 3) that indicates three clusters of smallholder vegetable farm typologies that exist and are joined together as the level of dissimilarity increases. The k-means clustering method that followed in the CA confirmed three as the optimal number of clusters of farmers. It optimized the distribution of farmers into three clusters and tabulated them by sex of the household head and name of EPA (Table 1). The results indicate that more than 80% of smallholder vegetable farmers across all three farm typologies, are male-headed households, suggesting either lower participation of female-headed households in vegetable farming or the inherent gender characteristics. All three farm types are present in all EPAs (Table 1), indicating their prevalence across all geographical regions of central and northern Malawi.
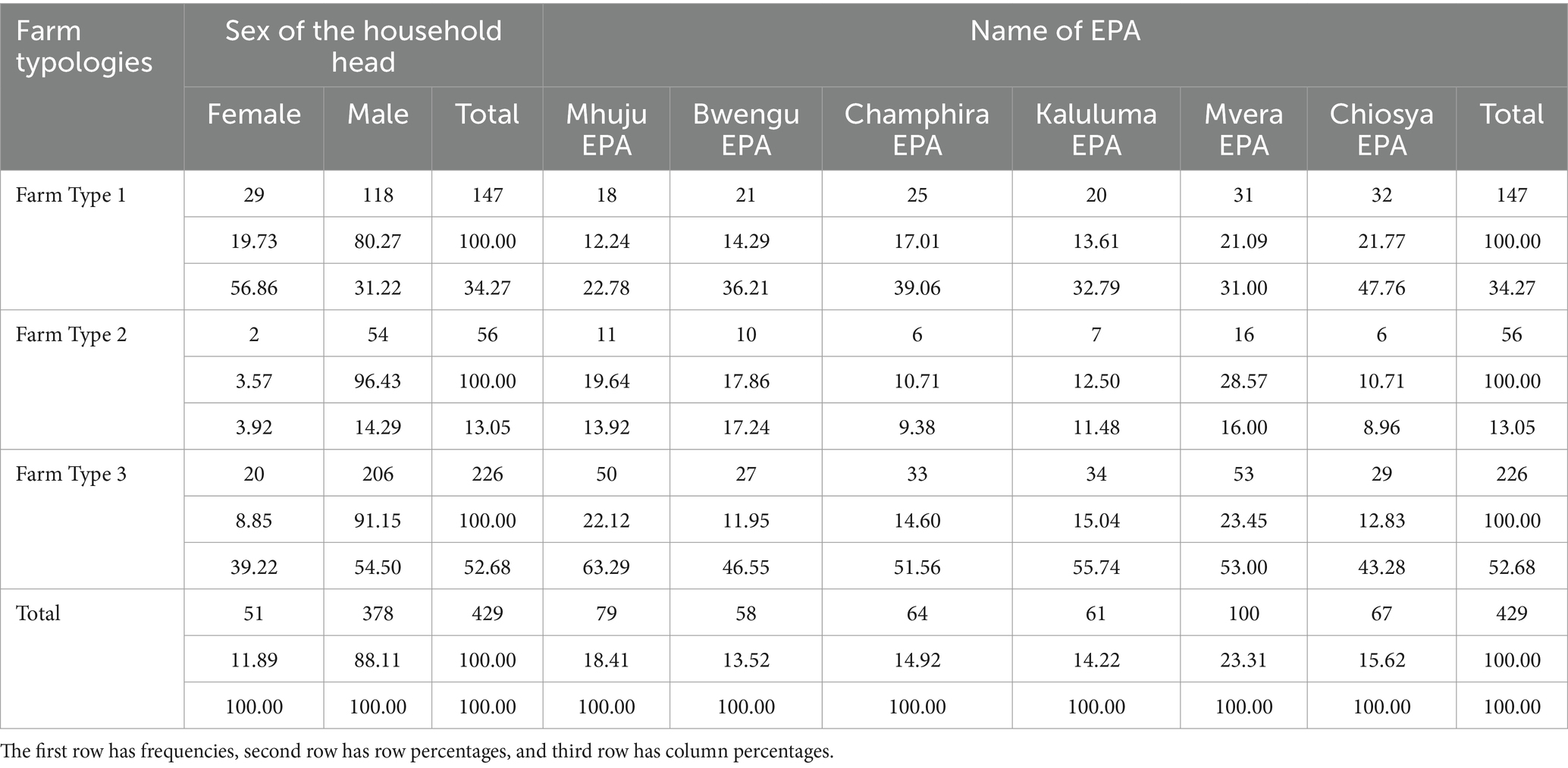
Table 1. Tabulation of farmers into three farmer types by sex of the household head and name of EPA.
3.2 Characterization of vegetable farm systems (Farm Typologies)
Table 2 displays Descriptive Statistics that show the mean value of each of the structural and functional variables used in profiling farm typologies. The table also shows the results of ANOVA that indicate significant differences in variations among the three farm types and the variables responsible for these differences.The Tukey’s post hoc testresults that followed ANOVA are also presented in Table 2 for pairwise comparisons of each variable.
Farm Type 1 represents Resource and Livelihood Constrained Off-farm Dependent (RLCOD) Farmers, constituting 34.27% of the sampled farming households. These farmers face significant constraints in resource endowments and livelihood factors, relying also on off-farm income. With an average landholding of 1.91 acres, of which 1.66 acres are arable land and 0.25 acres are irrigable land, these farmers cultivate approximately 1.5 acres of arable land, leaving only about 0.18 acres unfarmed. Regarding irrigable land, they cultivate around 0.22 acres, leaving minimal unfarmed irrigable land (0.02 acres). These farmers also have the capacity to rent about 0.1 acre of arable land and 0.02 acre of irrigable land. Rainfed crop production covers nearly all arable land (1.6 acres), while the size of irrigated land for crop production is approximately 343 m2, smaller than the land allocated to vegetable production (405 m2). However, both total crop production and vegetable production are relatively low at 1298 kgs and 373 kgs, respectively. These farmers retain 70.77% of crops and 56.39% of vegetables for food, signaling their reliance on crops rather than vegetables for food security. Similarly, the mean income from crop and vegetable production is lowest at MK160,759.30 and MK115,121.70, respectively. Despite this, the proportion of vegetables sold (44%) exceeds the proportion of crops sold (29%), indicating that most vegetables produced are sold in the market rather than consumed by the household. The mean Tropical Livestock Unit (TLU) is small (about 0.49) and the Livestock-related income is also modest(MK15,582.76). These farmers earn a higher mean monthly off-farm income (MK12,057.82) compared to non-farm income (MK10,408.16), highlighting that their livelihoods are more supplemented by off-farm activities. Additionally, these farmers have a lower mean farm asset value (MK58,802.99) and lower daily food expenditure (MK2,881.51).
Farm Type 2 comprises Resource and Livelihood Abundant and Commercial Oriented (RLACO) Farmers, constituting 13.05% of the sampled farmers. These farmers possess abundant resource endowments and livelihoods characterized by a commercial orientation. With an average landholding size of about 7 acres, including 6.14 acres of arable land and 0.86 acres of irrigated land, these farmers cultivate approximately 5.57 acres of arable land, leaving about 0.56 acres of unfarmed arable land. Regarding irrigable land, they cultivate about 0.80 acres, with minimal unfarmed irrigable land (0.06) left. These farmers also have the capacity to rent about 1.32 acres of arable land and 0.07 acres of irrigable land. Rainfed crop production covers 5.3 acres, representing almost all rainfed cultivated arable land. Irrigated crop production accounts for about 1765.73 m2, larger than the size of irrigated land allocated to vegetable production (988.16 m2). This suggests that these farmers prioritize crop production over vegetable production during irrigated farming. Both crop production and vegetable production are high at 7218 kgs and 2,721 kgs, respectively. The farmers retain 50.1% of crops and 47.8% of vegetables for food suggesting their equal emphasis on food security. Similarly, income from crop and vegetable production is substantial at MK2,337,517.00 and MK719,816.60, respectively. However, the proportion of vegetables sold (52%) is slightly higher than the proportion of crops sold (50%), indicating that these farmers produce both crops and vegetables primarily for the market rather than household consumption. These farmers have a substantial size of TLU (2.1) and high income from livestock (MK155,401.80) However, they have the lowest monthly off-farm income earnings (MK4,196.43) and the highest non-farm income earnings (about MK57,142.86). This suggests that part of their livelihood is largely supplemented by non-farm activities. They possess a mean farm asset value of MK1,595,388.00 and a daily food expenditure of about MK4,859.82.
Farmer Type 3 represents Medium Resources and Livelihoods Constrained Market-Oriented (MRLCMO) Farmers, constituting the largest proportion (52.68%) of the sampled households. These farmers are characterized as medium-resourced and livelihoods constrained but with a market-oriented production focus. They possess an average landholding size of 4.0 acres, including 3.44 acres of arable land and 0.58 acres of irrigable land. The farmers cultivate about 2.78 acres of arable land, leaving about 0.66 acres of unfarmed arable land. In terms of irrigable land, they cultivate about 0.46 acres, with 0.12 acres of unfarmed irrigable land, larger than the other types of farmers. These farmers also have the capacity to rent about 0.26 acres of arable land and 0.07 acres of irrigable land. Rainfed crop production covers most of the rainfed arable land (2.69 acres). Irrigated crop production accounts for about 784.17 m2 of land, almost the same size as the irrigated land allocated to vegetable production (723.04 m2). This suggests an equal emphasis on both crops and vegetables in their livelihoods. Crop production and vegetable production are moderate at 2614.21 kgs and 1201.56 kgs, respectively. These farmers retain 64.4% of crops and 44.0% of vegetables for food, showing their reliance on crops for food security. Similarly, income from crop and vegetable production is medium and almost equal at MK417,932.30 and MK307,517.40, respectively. However, the proportion of vegetables sold (55%) is higher than the proportion of crops sold (36%), indicating that these farmers tend to produce a greater proportion of crops for household consumption and a larger proportion of vegetables for the market. Income from livestock is MK43,163.70, with a medium number of TLU (0.7). These farmers have low monthly off-farm income earnings (MK11,692.48) but moderately high non-farm income earnings (MK44,734.51), highlighting the significant role of non-farm income in their livelihoods. The mean farm asset value is MK84,462.58, and daily food expenditure is MK4,505.67.
The ANOVA (Table 3) indicates significant differences among the three farm types (RLCOD, RLACO, and MRLCMO). While several factors exhibit significant differences across all three farm types, certain variables show no significant distinctions between RLCOD and RLACO farmers. Similarly, several factors, including unfarmed arable and irrigated land, rented irrigated land, land under irrigated vegetable production, monthly non-farm earnings, daily food expenditure, and the proportion of vegetables sold, are not statistically significant between RLACO and MRLCMO farmers. Additionally, variables such as rented arable land, livestock income, monthly off-farm earnings, household farm asset value, crop income, and tropical livestock units do not exhibit significant differences between RLCOD and MRLCMO farmers.
3.3 Resource pattern and livelihood strategies in farm systems
The analysis of land resource use in the farm typologies shows that RLACO farmers, owning the largest land size, exhibit the highest land utilization, followed by RLCOD and MRLCMO farmers (Figure 4). This trend is consistent for arable land utilization across the three farm types. However, concerning irrigable land defined as current and potential land for irrigation using smallscale irrigation methods and technologies (such as bucket, treadle pump, motorized pumps, and river diversion) with water sources from wells, dams, streams, and rivers, RLACO farmers have the highest land utilization, followed by MRLCMO and RLCOD farmers, respectively. This suggests that RLCOD farmers have a relatively higher proportion of unutilized irrigable land compared to the other farm types. Additionally, the observation that irrigated land utilization is smaller than arable land utilization for all three farm types (Figure 4) suggests greater potential for expanding production under irrigation than rainfed arable production.
The correlation among household size, family labor, and the household’s capacity to hire additional labor identifies labor constraints that affect both rainfed arable and irrigated production across all farm types. Despite the family being the major source of labor, approximately 50% of household members are unavailable to contribute to family labor across all farm types (Figure 5A). This situation appears more challenging for RLCOD farmers who also exhibit the lowest capability to hire additional labor compared to other farmer types (Figure 5B). The insufficient family labor and limited ability to hire additional labor may consequently affect the potential for expanding production and agricultural diversification within the vegetable farming systems.
The analysis of household farms shows that smallholder farmers within the vegetable farming systems possess similar types of farm assets. There is a higher proportion of farmers owning land clearing and plowing farm tools (Supplementary material S4). However, the proportion of farmers owning farm production and irrigation equipment is smaller, with the smallest ownership observed among MRLCMO and RLCOD farmers, respectively. Additionally, there is limited ownership of animal and mechanically powered equipment among the farmers. These disparities in farm assets highlight the challenges smallholder farmers face in acquiring existing and modern farm equipment and tools. Such differences can impact the type and scale of farm operations, as well as the efficiency and productivity of vegetable farmers across all farm typologies.
3.4 Crops, vegetable and livestock production and income
Despite being a vegetable farming system, crop production serves as the primary source of livelihood for over 80% of farmers across all farm typologies. However, RLACO farmers rely most on vegetable production (61%), followed by MRLCMO farmers (45%) and RLCOD farmers (39%). This pattern is mirrored in livestock production as well (Supplementary material S5A). Maize, groundnuts, soybeans, and beans are major crop combinations across all farm types. Other crop enterprises vary among farm typologies, with RLACO farmers incorporating additional crops like tobacco and sweet potato. Rainfed crop productivity is highest for RLACO farmers (Supplementary material S5B), whereas irrigated crop productivity is highest for RLCOD farmers (Supplementary material S5C). RLACO farmers exhibit greater diversity in crop cultivation compared to RLCOD and MRLCMO. Onion, tomato, and potato are common vegetables during rainfed production across all farm typologies. Regarding vegetables, RLACO farmers exhibit the highest productivity for rainfed production (Supplementary material S5D), while MRLCMO farmers demonstrate the highest productivity under irrigation (Supplementary material S5E). RLCOD farmers demonstrate greater diversification in rainfed vegetable production, despite allocating the smallest land to them. Farmers in all typologies prioritize crops over vegetables during rainfed, allocating more land and resources to them (Figures 6A,B). However, during irrigated production, all three farm types focus more on vegetables, likely due to ease of production and the need for additional income, given their higher profitability. Major enterprises during irrigation include tomatoes, beans, potatoes, leafy-vegetables, and green maize (Figure 6C).
The greater proportions of vegetables and crops are sold rather than retained for food across all farm typologies. MRCMO farmers have the highest proportion of vegetables sold (56%), followed by RLACO (52.2%) and RLCOD (43.6%) farmers, respectively. RLACO farmers have the highest proportion (49.9%) of crops sold, followed by MRCMO (35.6%) and RLCOD (29.2%) farmers, respectively (Figure 6D). Recognizing that access to market, defined by the distance to the closest used local market, plays an important role in the proportion of crops and vegetables sold (Koppmair et al., 2017), the average distance to market for crops was 0.3 km (for RLCOD), 0.8 km (for RLACO) and 0.4 km (for MRLMCO) while the average distance to the market for vegetables was 0.2 km (for RLCOD), 0.3 km (for RLACO) and 0.3 km (for MRLCMO). This suggests that all the farmers are within the closest distance to the nearest used market of 1 km. However, local vegetable markets are closer to the farmers than local crop markets possibly due to the perishability nature of vegetables and explaining a higher proportion of vegetables sold than crops for all farm typologies. Despite more than 40% of vegetables being sold across all farm typologies, the proportion of income from vegetables is smaller than the proportion of income earned from crop sales (Supplementary material S6A). RLACO farmers, which have the highest proportion of income from crop sales, show the lowest proportion of income from vegetables.
Livestock production is another livelihood option for smallholder vegetable farmers. The mean TLU was largest for RLACO (2.1), followed by MRLCMO (0.7) and RLCOD farmers (0.49). These differences emanate from variations in the number and types of livestock species owned. While chickens, goats, and pigs are the major livestock species that farmers own across all farm typologies (Figure 7), the proportion of farmers owning goats and pigs is higher for RLCOD farmers than RLACO and MRLCMO farm types. A higher proportion of RLACO farmers own cattle compared to RLCOD and MRLCMO farmers. However, the highest livestock income is realized from cattle and pigs for RLACO farmers, while for MRLCMO and RLCOD farmers, it is from goats and pigs (Supplementary material S6B). Besides farming, smallholder vegetable farmers are also involved in off-farm and non-farm activities to support their livelihoods (Supplementary materials S7A,B). Casual labor is the primary source of off-farm activities, especially for RLCOD farmers, providing the highest monthly income for the households. On the other hand, business or petty trading is the major non-farm source of income for RLACO and MRLCMO farmers, providing the highest monthly income.
3.5 Food expenditure, food consumption scores and household dietary diversity score
The expenditure patterns on major food groups consumed exhibit similarities across farm types. However, differences exist in the daily mean amount of money spent on each food group and their FCS (Figures 8A,B). Vegetables are fourth in food group expenditures for all farm typologies, meaning that a greater proportion of household income is also spent on the purchasing of vegetables. The mean FCS for all the farm typologies is above the minimum acceptable level of 35 scores. MRLCMO has the highest mean food consumption score of 54.17, followed by RLACO (53.88) and RLCOD farmers (48.70) (Table 4). This means that the majority of smallholder farmers in all three farm typologies have adequate access to and acquisition of food, as also confirmed by the box plot of FCS (Figure 8B). However, ANOVA results (Table 4) show significant differences in mean FCS for the three farm typologies. A notable significant difference is observed between RLCOD and MRLCMO farm types. Moreover, 30.61% of RLCOD farmers, 17.86% of RLACO farmers, and 20.35% of MRLCMO farmers do not have access to food, as their food consumption scores are less than the 35-score threshold (Table 4) showing the presence of food-insecure households in each farm type. The HDDS (Table 4) indicates adequate household access to food. However, ANOVA results (Table 4) show significant differences in HDDS for the three farm types over the 12-h and 7-day periods. For the 12-h period, a significant difference exists between RLCOD and MRLCMO farm types. The situation is different for the 7-day period, where a significant difference in HDDS is observed between all farm types except between MRLCMO and RLACO farmers. There is no significant difference in HDDS across all three farm types for the 24-h period. The box plot of HDDS (Figure 8C) shows the distribution of households across farm typologies over the three periods, signaling the presence of some farmers having inadequate access to food in the farm typologies over the 12-h and 7-day periods.
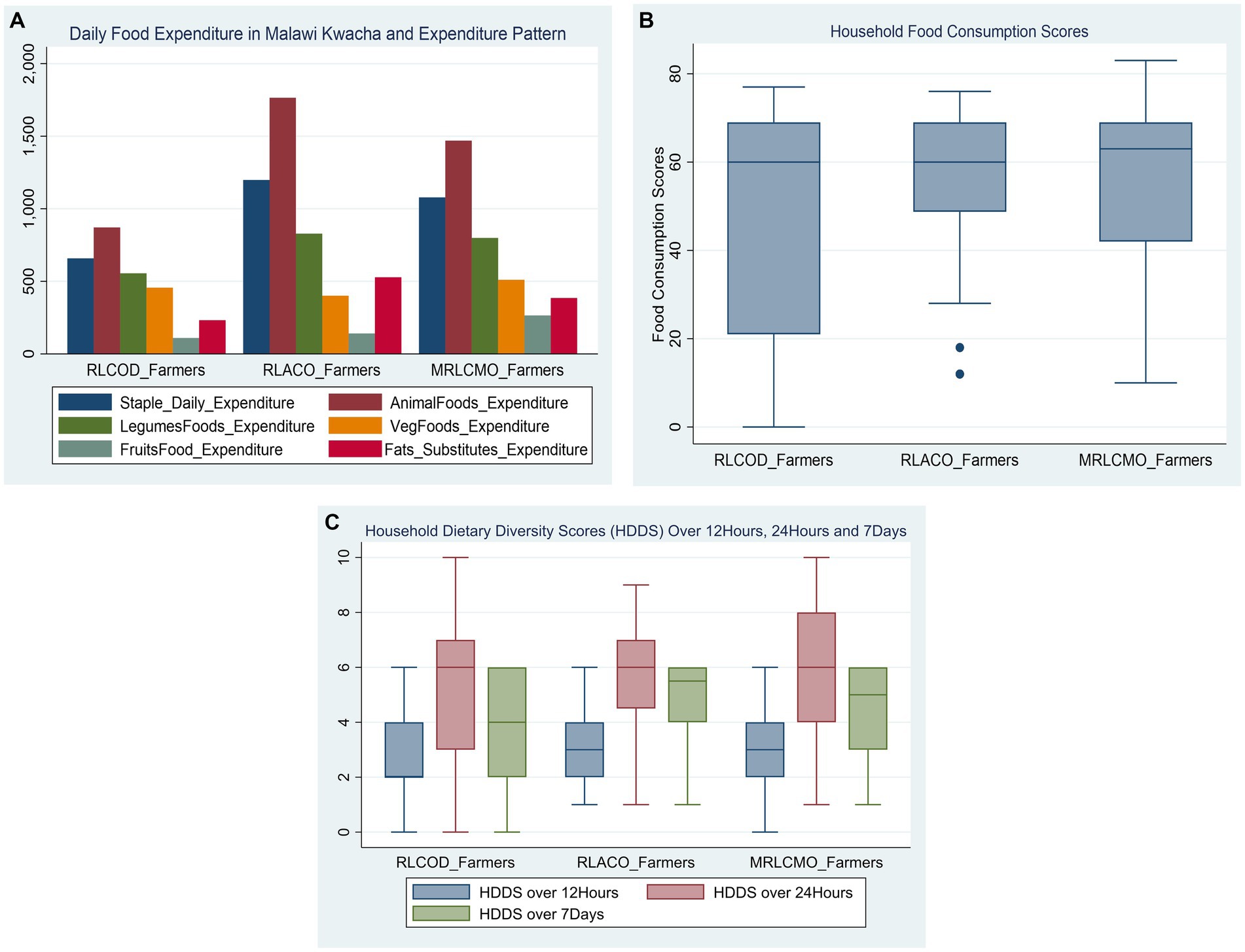
Figure 8. Household daily food expenditure, food consumption scores and household dietary diversity scores.
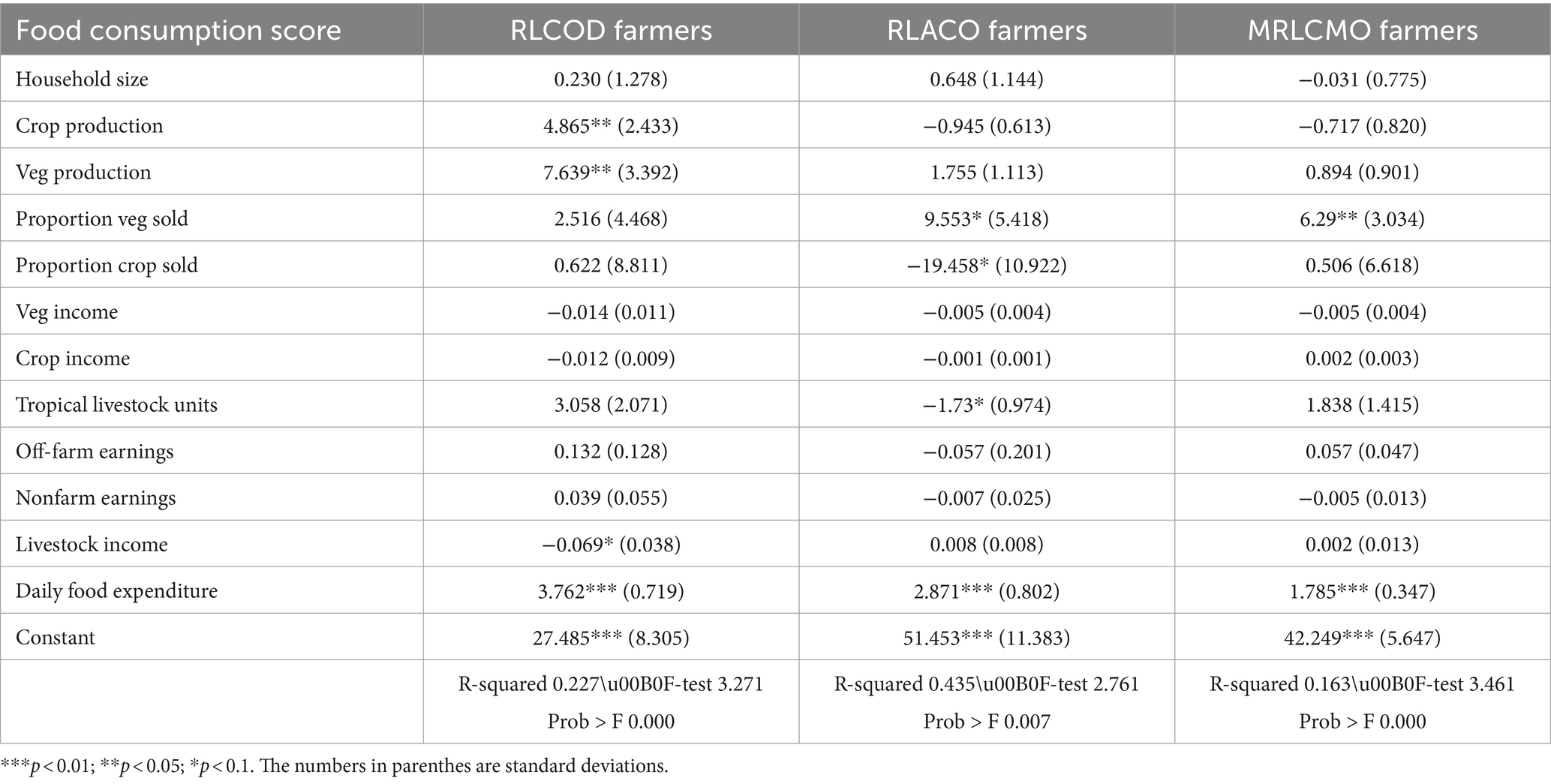
Table 4. Linear regression results of the impact of vegetable production on food consumption scores of farmers.
3.6 Multiple linear regression analysis of impact of vegetable production on food consumption scores
To explore and understand how and to what extent vegetable production impacts FCS, the multiple linear regression results for RLCOD, RLACO, and MRLCMO (Table 5) provide factors that affect household food consumption. In the case of RLCOD farmers, crop production, vegetable production, and daily food expenditure are significant and positively influence access to food consumption score. This indicates that a unit improvement in these variables will significantly enhance access to food security for these farmers. However, livestock income has a negative and significant impact on household food consumption score, suggesting that further unit increase will reduce access to food security. For RLACO farmers, daily food expenditure significantly and positively influences household food consumption score. Additionally, the proportion of vegetables sold, the proportion of crops sold, and TLU are other significant factors affecting acquisition and access to food security. However, the proportion of crops sold and livestock tropical units have negative impacts on household food consumption scores, indicating that a unit increase in these two variables will contribute to a reduction in the food consumption score of the household. For MRLCMO farmers, the proportion of vegetables sold and daily food expenditure are the only significant variables that positively influence access to food security. This implies that a unit improvement in these variables will significantly enhance access to food security for MRLCMO farmers.
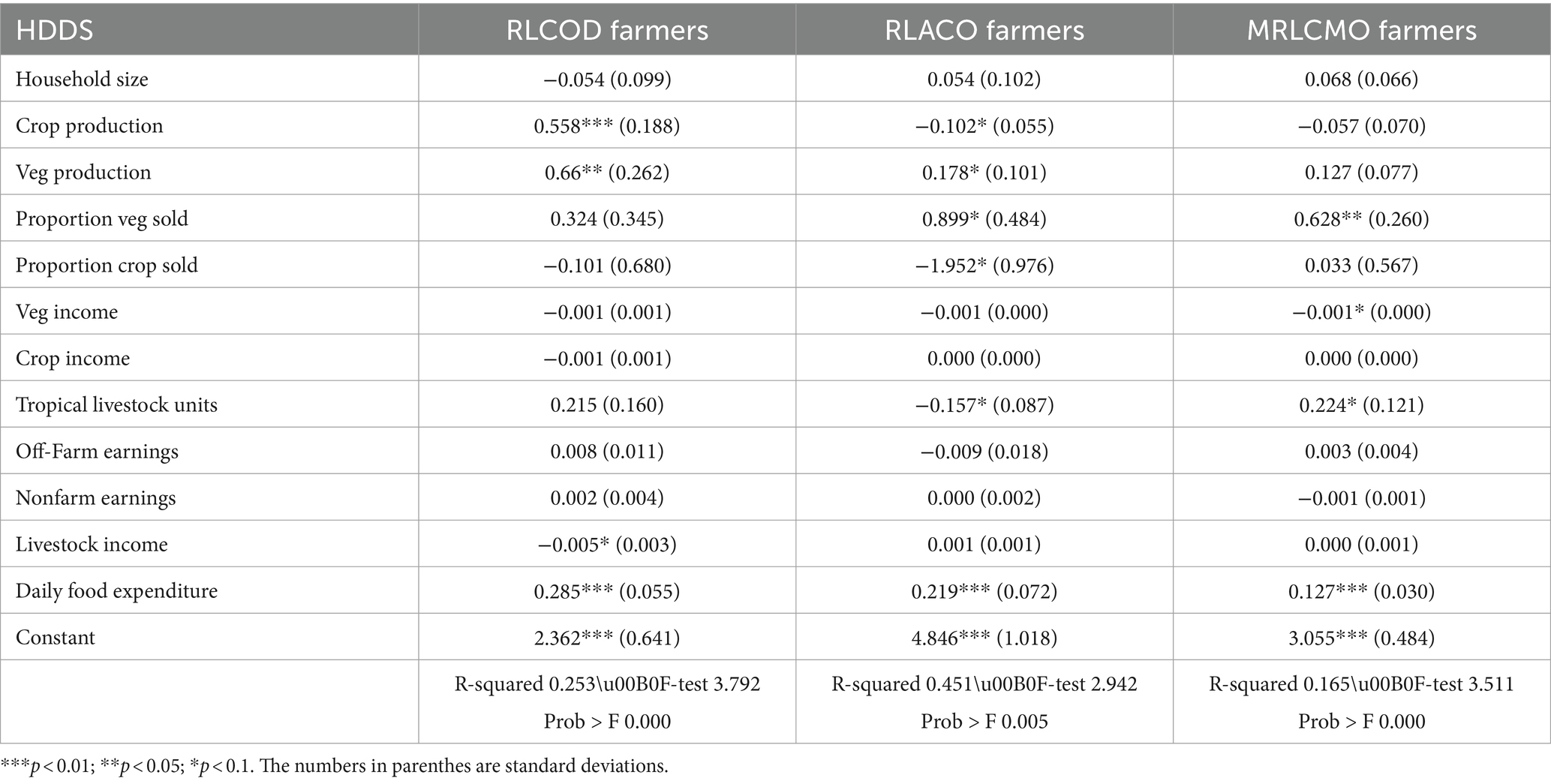
Table 5. Linear regression results of the impact of vegetable production on household dietary diversity scores.
3.7 Linear regression results of the impact of vegetable production on household dietary diversity scores
The multiple linear regression results of the impact of vegetable production on HDDS (Table 5) also show the factors that affect household dietary diversity. Just like on FCS, crop production, vegetable production, livestock income, and daily food expenditure are significant factors that influence household or individual access to the quantity and quality of food consumed over the past 7 days for RLCOD farmers. While all other significant factors positively influence household access to food, livestock income negatively affects access to food security for this type of farmers. This means that a unit increase in income from livestock reduces household access to food security. Unlike in the food consumption score regression results, more factors are significant that influence household dietary diversity for RLACO farmers. Besides the proportions of vegetables and crops sold and daily food expenditure, vegetable and crop production also significantly influence household dietary diversity score for RLACO farmers. However, a unit increase in crop production, proportion of crops sold, and TLU negatively affect the household dietary diversity score. This implies that a further unit increase in these variables will significantly reduce these farmers’ household access to food security. For MRLCMO, the proportion of vegetables sold, vegetable income, TLU, and daily food expenditure are significant variables that influence access to food security. A unit improvement in the proportion of vegetables sold, TLU, and daily food expenditure will significantly improve household dietary diversity score.
4 Discussion
4.1 Smallholder vegetable farm systems (farm typologies)
Studies on smallholder farming systems in the SSA region recognize the existence of diverse and heterogeneous smallholder farms, influenced by a range of socioeconomic and biophysical factors. These factors include demographic characteristics, wealth, resource endowments (land, labor, and capital), farm production practices and orientations, and livelihoods strategies (Tittonell et al., 2010; Makate and Mango, 2017; Chideya-Phiri, 2018; Dunjana et al., 2018; Kamau et al., 2018; Bakhsh et al., 2021). Our PCA findings confirm a significant amount of diversity and heterogeneity among smallholder vegetable farms, as evidenced by the large number and types of principal components required to capture most of the variations among smallholder farmers. Understanding the drivers of this diversity and heterogeneity is crucial for developing interventions, policies, and strategies to support and shape the future of smallholder vegetable farming (Kamau et al., 2018). The retained 11 principal components show that socio-economic and biophysical factors, along with their interactions, play a crucial role in driving the transformation of vegetable farming systems and influencing farm typologies within and across landscapes.
Land Profile and Use, which elucidates the sizes and types of arable and irrigable land owned and unfarmed by farmers (components on arable and irrigable land profiles), plays a central role in the observed heterogeneity among smallholder vegetable farmers. They highlight the role of access to land resources in shaping the diversity and scale of farm enterprises operated by farmers. The smallholder farmers’ use of arable and irrigable land for rainfed and irrigated crop and vegetable production, along with variations in crop and vegetable production (components on rainfed crop production on arable land, irrigated vegetable production, and irrigated crop production), highlight the economic importance of crop and vegetable farm enterprises, as well as rainfed and irrigated production, in creating diversity and heterogeneity within smallholder vegetable farming systems.
Farm assets and Enterprises income are additional drivers of diversity and heterogeneity among smallholder vegetable farmers. The component associated with household farm assets marks the importance of the types and value of farm assets in influencing the heterogeneity of smallholder vegetable farmers. It also reflects differences in physical farming technologies owned and used by smallholder vegetable farmers. The variations in the extent of vegetables and crops sold and the income derived highlight significant economic differences between crops and vegetables (components on irrigated vegetable production and income and crop income). This underscores the reliance of smallholder vegetable farmers not only on vegetables for income but also on non-vegetable crops.
Household structure, Expenditure and Off/Non-farm activities are additional drivers of diversity and heterogeneity in vegetable farming systems. The components that relate to household size and family labor, rented irrigable land, and daily food expenditure, respectively, outline how household characteristics and their consumption patterns shape farming practices and the targeting of interventions. For instance, larger households have more labor for farming but with higher food costs and land rental costs, possibly to support diversified food and income strategies. Moreover, smallholder farmers’ participation in off/non-farm activities and their derived income show that they do not solely rely on agricultural production for their livelihoods but also engage in different off/non-farm activities. Their engagement contributes to the observed diversity and heterogeneity among smallholder vegetable farmers. As such, policies and development interventions addressing issues surrounding these drivers of diversity and heterogeneity would enhance smallholder vegetable production and consumption.
The CA that classified the diverse and heterogeneous smallholder vegetable farms into homogeneous groups with common socio-economic and biophysical characteristics indicates the existence of three farm typologies. These findings align precisely with the three farm types proposed by Dunjana et al. (2018): “(i) resource-endowed, commercial oriented farms, (ii) medium resourced and (iii) resource constrained farms practizing subsistence and income oriented production”. However, based on purpose and criteria of construction, the classification of smallholder farmers have yielded about 2 to 5 farm typologies (Tittonell et al., 2010; Kamau et al., 2018; Bakhsh et al., 2021; Ismael et al., 2021; Innazent et al., 2022). The observation that RLCOD and MRLCMO farmers constitute the largest proportions of vegetable farmers (34.27 and 52.68% respectively), while RLACO constitutes the smallest proportion (13.05%) of vegetable farmers, is consistent with findings from other studies in the region (Bakhsh et al., 2021; Innazent et al., 2022).
Despite significant differences in farm typologies (ANOVA Results), certain structural and functional factors are similar between farm types (Tukey’s post hoc analysis results). For instance, unfarmed arable and irrigable land, as well as rented irrigable land, are significantly different only between RLCOD and MRLCMO farmers. The land under irrigated vegetable production, monthly non-farm income, and daily food expenditure are not statistically different between RLACO and MRLCMO farmers. Similarly, TLU, livestock income, monthly off-farm earnings, household farm assets, and income from sales of crops are not significantly different between RLCOD and MRLCMO farmers, while the proportion of vegetables sold is not significantly different between RLCOD and RLACO farmers. These differences and similarities seem to play a fundamental role in influencing the structure and functioning of smallholder vegetable farming systems. Recognizing and understanding these interconnections provides an empirical basis for understanding the typologies to leverage and develop likely informed agricultural policies, interventions, and pathways that can address the unique needs of each farmer type and enhance overall sector productivity and sustainability.
4.2 Land, labor and farm assets resources for farm typologies
Land resources remain crucial for agricultural production and investments among smallholder farmers across SSA, impacting food security and livelihoods (Olatunbosun Benjamin, 2020). In Malawi, the average smallholder landholding sizes (0.70 ha) are not only small but also shrinking over time with an increase in population growth (Chirwa and Matita, 2011; Muyanga et al., 2020). While the average landholding size for RLCOD farmers (0.77 ha) aligns with the national average (0.70 ha), the average landholding sizes for RLACO (2.83 ha) and MRLCMO (1.62 ha) seem to contrast with the national average, possibly because the study focuses only on vegetable producers in the central and northern regions, where landholdings tend to be larger than in the south. The larger landholding sizes of smallholder vegetable producers in these areas suggest significant opportunities to increase agricultural production on both arable and irrigable land across all farm typologies. However, their land resource utilization shows that MRLCMO farmers have the largest sizes of unfarmed arable and irrigable land compared to RLCOD and RLACO, despite owning and managing medium-sized land resources. This provides MRLCMO farmers, who constitute more than half of smallholder vegetable producers, with greater opportunities to increase and expand crop and vegetable production on both arable and irrigable land than other types of farms. The smaller utilization of irrigated land compared to arable land for all three farm typologies confirms the low (25.5%) utilization of irrigation farming in Malawi (Government of Malawi, 2016b), revealing potential for expanding production through irrigated production rather than rainfed arable production. Efforts to expand crop and vegetable production should prioritize expanding irrigated production and addressing associated constraints, such as labor and capital, rather than expanding cultivated arable land under rainfed production.
Labor is another important resource for smallholder farmers, particularly in contexts where farm mechanization is limited. Despite the availability of other sources of labor such as communal work practices and hired help, family labor remains the primary source for smallholder farming households in Malawi, accounting for more than 60% of total labor used in production (Takane, 2008; Chirwa and Matita, 2011; De Janvry et al., 2020). Our analysis of labor across the three farm typologies also indicates that RLACO farmers utilize more family labor compared to RLCOD and MRLCMO farmers. This could be attributed to larger household sizes and the necessity to manage more extensive farmed landholding sizes (Kudadze et al., 2019). With approximately 50% of household members being unavailable to provide family labor for production across all farm typologies, there is a need to hire additional labor, especially during peak times of farm operations. However, most households face challenges in hiring labor, as evidenced by other studies where about 10–30% were able to do so (Takane, 2008; Chirwa and Matita, 2011; De Janvry et al., 2020), impacting the potential expansion of crop and vegetable production, as well as the diversity of farm enterprises. This presents significant challenges for RLCOD farmers, who have the least ability to hire labor. Agricultural interventions that are not only less labor-intensive but also enhance labor productivity and the ability to hire labor among smallholder vegetable farmers, especially RLCOD farmers, need to be prioritized.
Besides land and labor, household farm assets are crucial resources that reflect the capital of smallholder farmers. These assets incorporate producer goods and basic infrastructure essential for carrying out farming activities, including farm inputs, tools, wells, equipment, and irrigation canals. While the findings indicate that all farm typologies possess similar land-clearing and plowing farm tools, differences emerge in the types, quantities, and value of the most important farm assets. These differences reflect variations in farm production orientations, objectives, and purchasing power. Understanding these differences across farm typologies is essential for shifting policies and interventions to maximize the use of existing farm assets while promoting the acquisition of modern farm inputs, tools, and equipment to enhance agricultural production quality and efficiency. The highest farm asset value for RLACO farmers appears to enable them to cultivate more land compared to the other two types of farmers, as noted by other studies (Matita et al., 2022). However, the limited ownership of modern farm production and irrigation equipment, as well as animal and mechanically powered farm tools across farm typologies, seems to impact the choice of farm enterprises, the scale of farm enterprise production, and farm enterprise productivity.
4.3 Agricultural production practices and livelihoods for farm typologies
Smallholder vegetable farmers across all farm typologies employ multiple livelihood strategies. Crop production, which includes a combination of maize, groundnuts, soybeans, and beans, remains the primary source of livelihood for all three farm typologies, highlighting the economic importance of the cereal-legume value chain to smallholder farmers (Branca et al., 2021). Maize, being main staple food crop in Malawi, is cultivated by every farmer and accounts for more than 50% of the area planted and 70% of calories consumed (Government of Malawi, 2018; Branca et al., 2021; Kansanga et al., 2021). This significant allocation of land to maize by smallholder vegetable farmers is consistent across all farm typologies. However, the continued dominance of maize as a food security crop contributes to low dietary diversity (25%), a carbohydrate-based diet, and high levels of undernutrition at both household and national levels (Government of Malawi, 2018), emphasizing the need for policy shifts, development interventions, and awareness campaigns toward increased agricultural diversity, which has been found to enhance dietary diversity, income and wealth (Bellon et al., 2020; Singh et al., 2020; Fatch et al., 2023). The production of grain legumes (groundnuts, soybean, and beans) by all farm typologies demonstrates significant potential to benefit farmers with varying resource endowments— from resource-constrained to medium and high-resource-endowed farmers (Franke et al., 2014). Therefore, crop diversification that includes grain legumes is imperative. Our findings indicate that RLACO farmers exhibit more diversified crop combinations compared to RLCOD and MRLCMO farmers, likely facilitated by their greater abundance of owned resources. This highlights the need for increased agricultural diversification efforts among RLCOD and MRLCMO farmers, who together constitute about 85% of smallholder vegetable farmers. However, specific crop enterprises may be associated with particular farm types. For example, RLACO farmers may include tobacco as an additional crop. Successful crop diversification thus requires consideration of other factors such as social organizations, resource endowments, land characteristics, and transaction costs, as echoed by other studies (Rehima et al., 2013; Asante et al., 2018; Kankwamba et al., 2018).
Crop production appears to be the predominant activity in rainfed farming, covering a greater significant proportion of arable land across all farm typologies. Despite RLACO showing higher crop production and its respective earned income, followed by MRLCOMO and RLCOD, their production and productivity levels remain below national and potential targets across all farm typologies (Government of Malawi, 2018, 2021a). This underscores the need to enhance productivity to achieve higher crop output. Given that rainfed crop productivity is highest for RLACO farmers, while irrigated crop productivity is highest for RLCOD farmers, initiatives aimed at increasing land under rainfed production for RLACO farmers and land under irrigated production for RLCOD farmers could stimulate existing crop production. Additionally, increasing irrigated crop productivity for RLACO farmers and enhancing rainfed crop productivity for RLCOD farmers could further boost overall crop production for both farm typologies. The lower proportion of crops retained for food and the highest proportion of crops sold for RLACO farmers signify that their primary production purpose is largely for the market. In contrast, RLCOD farmers primarily produce more for home consumption, with the highest proportion of crops retained for food and the lowest proportion of crops sold.
Vegetable production serves as the primary source of farming livelihoods for RLACO farmers, followed by MRLCMO and RLCOD farmers, respectively. This indicates the importance of implementing targeted initiatives to enhance vegetable production and its contribution to farmers’ livelihoods, particularly among MRLCMO and RLCOD. Common vegetables produced during rainfed cultivation across all farm typologies include onions, tomatoes, and potatoes. During irrigated production, tomatoes, beans, potatoes, and leafy vegetables are the predominant choices among farmers (Chadha et al., 2008; Mwandira, 2008). Despite differences in vegetable production across the three farm typologies their productivity remains below the national and potential targets (Government of Malawi, 2018, 2021a). Implementing policies and interventions to enhance productivity would consequently contribute to increased production, especially with an increase in the land allocated to vegetables. Currently, RLACO farmers, with the highest rainfed productivity, and MRLCMO farmers, with the highest irrigated productivity, are the farmers with the greatest potential to increase vegetable production. This can be achieved by RLACO farmers expanding land under rainfed vegetable production and improving irrigated vegetable productivity. Similarly, for MRLCMO farmers, increasing both irrigated land and rainfed crop productivity could boost vegetable production. Despite RLCOD farmers having the smallest land allocations for vegetable production, they exhibit more diversity in vegetable production during rainfed cultivation compared to other farm types due to the possible production of vegetables on non-irrigable land during rainfed. Furthermore, the tendency of smallholder farmers in vegetable farming systems to prioritize crops over vegetables during rainfed production, as evidenced by a greater allocation of land to crops, could be attributed to limited production factors, particularly chemicals for pest and disease control in vegetable cultivation during rainfed periods (Dunjana et al., 2018; Gebru et al., 2019). The allocation of more land to vegetables than crops during irrigated production suggests that vegetable production is perceived as more cost-effective and profitable, resulting in increased income. The different allocations of available land resources to crops and vegetables could be attributed to differences in priorities and production objectives (Chideya-Phiri, 2018). The observation that more than 40% of produced vegetables are sold across all farm typologies underscores that vegetables are predominantly cultivated for income, contributing approximately 35% of smallholder farmers’ income in Malawi, rather than for household consumption (Chagomoka et al., 2014; Kudadze et al., 2019).
Boosting crop and vegetable productivity among smallholder farmers across the three farm typologies is crucial in the context of climate change. Climate change projections for Malawi indicate a continued increase in frequency and severity of climate change extremes, including droughts, floods, and outbreaks of crop pests and diseases by 2050, posing serious negative impacts on productivity, production, and subsequent food security, nutrition, and income (AFRICAP, 2022). These impacts are particularly concerning for Malawi, where agricultural diversification and dietary diversity are low and carbohydrate-based. However, improved smallholder irrigation offers a potential alternative that can enhance the adaptation and resilience of the vegetable farming system and reduce its vulnerability (Nhamo et al., 2016; Kudadze et al., 2019). Small-scale irrigation enables farmers to intensify production, and expand production and profitability potential, resulting in productivity growth (Shah et al., 2013; Xie et al., 2014). With the support of the implementation of favorable policies and strategies on climate change, irrigation is projected to increase yields by 10% or more (AFRICAP, 2022). Irrigation production also increases and maintains the frequency of crop and vegetable production throughout the year, thereby enhancing production and income (Nhamo et al., 2016; Mango et al., 2018a,b).
Similar to vegetables, livestock production serves as a significant source of farming livelihoods for RLACO farmers, followed by MRLCMO and RLCOD farmers, respectively. Common livestock species across farm typologies include chickens, goats, and pigs, indicating the economic importance of small stock in the livelihoods of the majority of vegetable farmers, as opposed to cattle, which are predominantly owned by RLACO farmers. While there has been an increase in all livestock species owned by farmers across the farm typologies, as confirmed by the national livestock census, the government asserts that productivity is low due to inadequate parasite and disease control, feeding, and housing management (Government of Malawi, 2021c). Apart from RLACO farmers with an average TLU of 2.10, the TLU for RLCOD (0.49) and MRLCMO (0.70) were far below the national average (1.4) (Government of Malawi, 2018), possibly due to the small number of cattle owned. This calls for policies and interventions that stimulate and support parasite and disease control, feeding, and housing management of small-stock while promoting cattle to capable households in RLCOD and MRLCMO farm typologies. Despite livestock contributing about 16 to 50% of household annual income (Government of Malawi, 2021c) and the commonalities in livestock species owned across farm typologies, RLACO farmers generate the highest livestock income from the sale of cattle and pigs, whereas MRLCMO and RLCOD farmers derive the highest income from the sale of goats and pigs. These findings highlight the distinct contributions of various livestock species to the livelihoods of different farm typologies, with certain species playing a more significant role for specific farm types.
In addition to farming, off-farm and non-farm activities play a crucial role in supporting the livelihoods of smallholder vegetable farmers. RLCOD farmers primarily supplement their income through off-farm activities like casual labor, while RLACO and MRLCMO farmers rely more on non-farm sources such as business and petty trading, artisan work, and formal employment. The dependence of smallholder farmers on off- and nonfarm initiatives reflects resource limitations for RLCOD and a shift in livelihoods strategies or non-agricultural diversification (Fatch et al., 2021) for the RLACO and MRLCMO farmers that influences farmers’ decision making, agricultural practices, priorities, and labor resources (Crowley and Carter, 2000; Tittonell et al., 2010).
4.4 Food security and impact of vegetable production within farm typologies
The adequate access and acquisition of food, as measured by FCS exceeding the minimum acceptable level of 35, indicate that the majority of smallholder vegetable farmers are food secure across all farm typologies. These findings are consistent with previous studies (Ngema, 2017; Mango et al., 2018a,b; Fite et al., 2022), which also used FCS and found more than 50% of households were food secure. However, the ANOVA findings reveal a significant difference, particularly between RLCOD and MRLCMO farm types. MRLCMO farmers exhibit the highest mean FCS, followed by RLACO and RLCOD farmers, respectively. Additionally, the box plot of FCS confirms the existence of some food-insecure households in all farm typologies, with scores falling below the 35-score threshold. RLCOD has the highest proportion of food-insecure households, followed by MRLCMO and RLACO, respectively. Similarly, the mean HDDS for 12 h, 24 h, and 7 days demonstrate adequate household access to the quantity and quality of food for all farm typologies. However, the ANOVA findings reveal significant differences in HDDS over the 12-h and 7-day periods. For the 12-h period, a significant difference exists between RLCOD and MRLCMO farm typologies. For the 7-day period, a significant difference in HDDS is observed between all farm types except MRLCMO and RLACO farmers. The box plot of HDDS also illustrates the presence of some farmers without access to the quantity and quality of food across all farm typologies over the 12-h, 24-h, and 7-day periods. The presence of food-insecure households in all farm typologies among smallholder vegetable farmers indicates the influence of various factors on their access and acquisition of food, necessitating specific policy support and development interventions tailored to each typology.
Some studies indicate that FCS is positively influenced by several factors including crop diversification, livestock ownership, asset ownership, access to credit, and education level (Mango et al., 2018a,b; Amao et al., 2023). Conversely, other studies have found that factors such as livestock income, landholding size, crop cultivation, farmer experience, access to irrigation technology, and group membership positively influence FCS, while drought and infertile land have a negative impact on FCS (Reincke et al., 2018; Nkomoki et al., 2019; Acheampong et al., 2022; Ingutia and Sumelius, 2022). Our findings of the multiple linear regression analysis reveal crop production, vegetable production, and daily food expenditure as significant and positive factors influencing FCS among RLCOD farmers. In contrast, livestock income significantly and negatively influences food security for RLCOD farmers. This might be attributed to the selling of livestock, which reduces household consumption of the animal food group contributing to food security, while the income earned is allocated to other non-food expenses. For RLACO farmers, the proportion of vegetables sold and daily food expenditure are significant and positively influencing factors for FCS. However, the proportion of crops sold and tropical livestock units negatively influence household access and acquisition to food security. This could be due to the reduction in food consumption of those crops and the increased cost of managing diversified livestock, at the expense of household food consumption. The proportion of vegetables sold and daily food expenditure are the only significant variables that positively influence access to food security for MRLCMO farmers. These findings suggest that policies and interventions aimed at promoting the positive and significant factors while mitigating the impact of negative factors for each typology have the potential to enhance food accessibility and acquisition. Similar conclusions have been drawn in other studies (Kabunga et al., 2014; Reincke et al., 2018; Nkomoki et al., 2019; Acheampong et al., 2022).
Studies on HDDS highlight several significant factors positively influencing dietary diversity, including crop diversity, crop income, vegetable production, size of land owned, food expenditure, gender, education level, access to credit, proportion of crops sold, annual income, and contact with extension workers (Ochieng et al., 2017; Rajendran et al., 2017; Singh et al., 2020; Cordero-Ahiman et al., 2021; Sambo et al., 2022). Conversely, household size has been found to have a negative influence on HDDS (Ngema, 2017). Other factors affecting HDDS include participation in irrigation schemes, ownership of small livestock, wealth, and on-farm production (Muchenje and Mushunje, 2013; Gupta et al., 2020; Bandyopadhyay et al., 2021). Similar to these studies, multiple linear regression analysis identifies crop production, vegetable production, livestock income, and daily food expenditure as significant factors influencing household HDDS for RLCOD farmers. The negative influence of livestock income on food security for RLCOD farmers is in contrast with studies, may be the selling of livestock reduces household consumption of the animal food group, while the income earned from livestock is utilized for other non-food group expenses. The HDDS for RLACO farmers is influenced by the proportion of vegetables sold, proportion of crops sold, daily food expenditure, TLU, vegetable production, and crop production. However, crop production, proportion of crops sold, and TLU negatively affect the HDDS. Since RLACO farmers cultivate the largest tract of land and have the smallest unfarmed land, a further increase in crop production comes at the expense of household food security. The highest proportion of crops sold reduces the quantity and quality of food consumed. Moreover, a higher TLU increases the cost of managing diversified livestock at the expense of household food consumption diversity. For MRLCMO, the proportion of vegetables sold, TLU, and daily food expenditure are the significant variables influencing access to food security. However, a unit increase in vegetable income reduces household access to food security, possibly due to the use of income earned from vegetables on other non-food expenses and reduction in the amount of vegetables consumed.
These findings underscore the importance of key factors specific to each farm typology in food security intervention initiatives aimed at enhancing household food security status. It is clear that, in addition to vegetable production, various other factors significantly impact household acquisition and access to food security, underscoring the need for a comprehensive consideration of these factors to effectively achieve food security in vegetable farming systems in the developing world.
5 Conclusion
This study has undertaken a comprehensive characterization of smallholder vegetable farm systems, delineating their resource patterns and livelihood strategies, and assessing the impact of vegetable production on enhancing accessibility and acquisition of food and income. The results reveal the presence of three distinct farm types: RLCOD farmers (the second-largest proportion), RLACOfarmers (the least proportion), and MRLCMO farmers (the largest proportion). While commonalities in resources and livelihood strategies exist among the three farm typologies, significant differences and variability are evident, particularly in land resource, labor availability, farm assets, crop, vegetable, and livestock production and income, as well as off-farm and non-farm income. Despite a majority of farmers achieving food security, all three farm typologies still harbor food-insecure households, with RLCOD having the highest proportion of food-insecure households, followed by MRLCMO and RLACO. Vegetable production emerges as a crucial factor in enhancing food security and income for farmers across all farm typologies. However, it is important to note that, in addition to vegetable production, other factors significantly influence household access to food.
6 Policy implications
The observed diversity and heterogeneity among smallholder vegetable farmers, driven by interactions of socioeconomic and biophysical factors such as land profiles and use, farm assets, enterprise income, off/non-farm activities, and household structure and expenditure, imply diverse and varying needs within the vegetable value chain. This necessitates multiple interventions tailored to the characteristics and requirements of each farm type to effectively promote vegetable production and consumption. The identification of three distinct vegetable farm types, which share certain structural and functional factors, underscores the importance of two types of agricultural interventions. These interventions should focus on both commonalities and differences among farm typologies to enhance the structure and functioning of vegetable farming systems for increased productivity and sustainability. Additionally, addressing gender imbalances in vegetable farming, particularly between the youthful male and female-headed households, requires deliberate policy support targeting female-headed households while saclling up vegetable farming interventions.
The production of maize, groundnuts, soybean, and bean crops remains crucial for the livelihood of all vegetable farmers, contributing to both food security and income generation. This highlights the ongoing necessity for agricultural policy support and interventions for these crops. However, the significant allocation of land to maize production indicates that food security is still heavily reliant on staple crop production and consumption. This underscores the urgency for increased policy reforms in food security, nutrition, dietary diversity, and agricultural diversification efforts, particularly in light of the growing impact of climate change. Moreover, efforts to expand and diversify crop production must consider factors such as available arable and irrigable land, household labor availability, capacity to hire additional labor, as well as farm assets and inputs specific to each farm typology. This holistic approach is crucial for enhancing overall productivity and production efficiency. Recognizing the vital role of vegetables in the farmers’ livelihoods, as evidenced by their significant allocations of land and proportions retained for food consumption and income generation, there is a pressing need for deliberate policy and development interventions. These interventions should aim not only to increase vegetable production but also to promote consumption for improved dietary diversity across all farm typologies. Similarly, chickens, goats, and pigs play significant roles in the livelihood of vegetable farmers and require increased targeted policy and development support tailored to specific farm types. This comprehensive approach is essential for sustainable agricultural development and improved livelihoods in vegetable farming communities.
Considering that food accessibility and acquisition among vegetable farmers are influenced not only by vegetable production but also by various other factors, it becomes imperative for development practitioners, both state and non-state actors, to adopt a holistic approach. This approach should include interventions aimed at expanding vegetable production, as well as promoting on-farm, off-farm, and non-farm enterprises. It should take into account available resources, interactions, and economic and climatic conditions. Such a comprehensive strategy is essential for supporting smallholder vegetable farmers in achieving sustainable food security and income.
Data availability statement
The raw data supporting the conclusions of this article will be made available by the authors, without undue reservation.
Ethics statement
The studies involving humans were approved by Norwegian Center for Research Data (NSD). The studies were conducted in accordance with the local legislation and institutional requirements. The participants provided their written informed consent to participate in this study.
Author contributions
WN: Writing – review & editing, Writing – original draft, Visualization, Validation, Software, Project administration, Methodology, Investigation, Formal analysis, Data curation, Conceptualization. RI: Writing – review & editing, Validation, Formal analysis. MB: Writing – review & editing, Validation, Methodology, Formal analysis, Conceptualization. SSG: Writing – review & editing, Validation, Methodology, Conceptualization. LE: Writing – review & editing, Resources, Project administration, Funding acquisition. SRG: Writing – review & editing. SD: Writing – review & editing, Writing – original draft, Validation, Supervision, Resources, Project administration, Methodology, Investigation, Funding acquisition, Conceptualization.
Funding
The author(s) declare that financial support was received for the research, authorship, and/or publication of this article. The study is an integral part of the Sustainable Food Systems for Rural Agriculture Transformation and Resilience (TRANSFORM) and Sustainable Food Systems in Malawi (FOODMA) programs grant number MWI-19/0018. These initiatives aim to contribute to achieving sustainable food security and household income, recognizing the diversity and heterogeneity among smallholder farmers. The programs are implemented as a consortium involving Lilongwe University of Agriculture and Natural Resources (LUANAR), Norwegian University of Life Sciences (NMBU), Development Fund of Norway (DF), and Norwegian Church Aid (NCA). The Royal Norwegian Embassy (RNE) in Malawi provided crucial financial support for these programs. The overarching goal of these programs is to contribute to sustainable food systems by promoting agricultural transformation for enhanced food and nutrition security, as well as increasing household farm incomes for all smallholder farmers in Central and Northern Malawi.
Acknowledgments
We express our sincere gratitude to the farmers in the study districts for their invaluable consent, time, and active participation, which formed the basis for the data presented in this paper. Additionally, we extend our appreciation to the dedicated staff and field extension workers from the Ministry of Agriculture and Food Security for their invaluable assistance throughout the implementation of the fieldwork. Your contributions have played a crucial role in the success of this study.
Conflict of interest
The authors declare that the research was conducted in the absence of any commercial or financial relationships that could be construed as a potential conflict of interest.
Publisher’s note
All claims expressed in this article are solely those of the authors and do not necessarily represent those of their affiliated organizations, or those of the publisher, the editors and the reviewers. Any product that may be evaluated in this article, or claim that may be made by its manufacturer, is not guaranteed or endorsed by the publisher.
Supplementary material
The Supplementary material for this article can be found online at: https://www.frontiersin.org/articles/10.3389/fsufs.2024.1387912/full#supplementary-material
SUPPLEMENTARY MATERIAL S1 | Farming households producing vegetables in the study sections.
SUPPLEMENTARY MATERIAL S2 | Food groups and their weights for fcs and hdds.
SUPPLEMENTARY MATERIAL S3A | Principal components and their variations.
SUPPLEMENTARY MATERIAL S3B | Variable loadings in rotated principal components.
SUPPLEMENTARY MATERIAL S4 | Proportion of farmers owning most important farm assets.
SUPPLEMENTARY MATERIAL S5A | Smallholder vegetable farmers farming livelihood strategies.
SUPPLEMENTARY MATERIAL S5B | Rainfed crop productivity.
SUPPLEMENTARY MATERIAL S5C | Irrigated crop productivity.
SUPPLEMENTARY MATERIAL S5D | Rainfed vegetable productivity.
SUPPLEMENTARY MATERIAL S5E | Irrigated vegetable productivity.
SUPPLEMENTARY MATERIAL S6A | Proportion of vegetables and crops income over total farm income.
SUPPLEMENTARY MATERIAL S6B | Livestock species mean income in malawi kwacha.
SUPPLEMENTARY MATERIAL S7A | Mean monthly earnings from non-farm sources.
SUPPLEMENTARY MATERIAL S7B | Mean monthly off-farm earnings in malawi kwacha.
References
Acheampong, P. P., Obeng, E. A., Opoku, M., Brobbey, L., and Sakyiamah, B. (2022). Does food security exist among farm households? Evidence from Ghana. Agric. Food Secur. 11, 1–13. doi: 10.1186/s40066-022-00362-9
AFRICAP. (2022). Agricultural and food system resilience: Increasing capacity and advising policy Programme. AFRICAP.
Ali, M., and Abedullah, A. (2002). Nutritional and economic benefits of enhanced vegetable production and consumption. J. Crop. Prod. 6, 145–176. doi: 10.1300/J144v06n01_09
Amao, I. O., Ogunniyi, A. I., Mavrotas, G., and Omotayo, A. O. (2023). Factors affecting food security among households in Nigeria: the role of crop diversity. Sustainability (Switzerland) 15:534. doi: 10.3390/su15118534
Asante, B. O., Villano, R. A., Patrick, I. W., and Battese, G. E. (2018). Determinants of farm diversification in integrated crop-livestock farming systems in Ghana. Renew. Agric. Food Syst. 33, 131–149. doi: 10.1017/S1742170516000545
Bakhsh, S. Y., Feil, J. H., and Yazdan Bakhsh, S. (2021). Typology of small-scale farmers in southern Africa and implications for policy design. 61st Annual Conference, Berlin, Germany, September 22-24, 2021 317092, German Association of Agricultural Economists (GEWISOLA).
Bandyopadhyay, A., Haile, B., and Azzarri, C., and Somé, J. (2021). Analyzing the drivers of household dietary diversity: evidence from burkina faso. Food Nutr Bull. 42, 530–550. doi: 10.1177/03795721211029092
Bellon, M. R., Kotu, B. H., Azzarri, C., and Caracciolo, F. (2020). To diversify or not to diversify, that is the question. Pursuing agricultural development for smallholder farmers in marginal areas of Ghana. World Dev. 125:104682. doi: 10.1016/j.worlddev.2019.104682
Branca, G., Cacchiarelli, L., D’amico, V., Dakishoni, L., Lupafya, E., Magalasi, M., et al. (2021). Cereal-legume value chain analysis: a case of smallholder production in selected areas of Malawi. Agriculture (Switzerland) 11:1217. doi: 10.3390/agriculture11121217
Carletto, C., Jolliffe, D., and Banerjee, B. (2015). From tragedy to renaissance: improving agricultural data for better policies. J. Dev. Stud. 51, 133–148. doi: 10.1080/00220388.2014.968140
Chadha, M. L., Oluoch, M. O., Saka, A. R., Mtukuso, A. P., and Daudi, A. T. (2008). Vegetable Research and Development in Malawi, review and planning workshop proceedings, September 23-24, 2003, Lilongwe, Malawi. Review Literature And Arts Of The Americas, Publication No. 08-705, p. 116.
Chagomoka, T., Afari-Sefa, V., and Pitoro, R. (2014). Value chain analysis of traditional vegetables from Malawi and Mozambique. Int. Food Agribus. Manage. Rev. 17, 57–83.
Chideya-Phiri, G. (2018). The diversity of smallholder farmers and their adoption of the sustainable intensification practices in Malawi [Doctoral Thesis]. Western Sydney University.
Chirwa, E. W., and Matita, M. (2011). From subsistence to smallholder commercial farming in Malawi: a case of NASFAM commercialisation initiatives.
Cole, M. B., Augustin, M. A., Robertson, M. J., and Manners, J. M. (2018). The science of food security. NPJ Sci. Food 2:14. doi: 10.1038/s41538-018-0021-9
Cordero-Ahiman, O. V., Vanegas, J. L., Franco-Crespo, C., Beltrán-Romero, P., and Quinde-Lituma, M. E. (2021). Factors that determine the dietary diversity score in rural households: the case of the paute river basin of azuay province, ecuador. Int J Environ Res Public Health. 18, 1–16. doi: 10.3390/ijerph18042059
Crowley, E. L., and Carter, S. E. (2000). Agrarian change and the changing relationships between toil and soil in Maragoli, Western Kenya (1900-1994). Hum. Ecol. 28, 383–414. doi: 10.1023/A:1007005514841
De Janvry, A., Duquennois, C., and Sadoulet, E. (2020). Labor Calendars and Rural Poverty: A case study for Malawi. Available at:http://iresearch.worldbank.org/PovcalNet.
Dixon, J. A., Gibbon, D. P., and Gulliver, A. (2001). Farming systems and poverty: improving farmers’ livelihoods in a changing world. Rome, Italy: Food and Agriculture Organization of the United Nations and World Bank.
Dunjana, N., Zengeni, R., and Wuta, M. (2018). Typological characterisation of farms in a smallholder food-cash crop production system in Zimbabwe – opportunities for livelihood sustainability. J. Agric. Rural. Dev. Trop. Subtrop. 119, 11–22.
Ebert, A. W. (2017). Vegetable production, diseases, and climate change. Front. Econ. Global. 17, 103–124. doi: 10.1108/S1574-871520170000017008
Everitt, B. S., Landau, S., Leese, M., and Stahl, D. (2011). Cluster analysis. 5th Edn. New York: John Wiley and Sons.
Fan, S., and Rue, C. (2020). The Role of Smallholder Farms in a Changing World. The Role of Smallholder Farms in Food and Nutrition Security, 13–28. doi: 10.1007/978-3-030-42148-9_2
Fatch, P., Masangano, C., Hilger, T., Jordan, I., Mambo, I., Kamoto, J. F. M., et al. (2021). Holistic agricultural diversity index as a measure of agricultural diversity: a cross-sectional study of smallholder farmers in Lilongwe district of Malawi. Agric. Syst. 187:102991. doi: 10.1016/j.agsy.2020.102991
Fatch, P., Masangano, C., Jordan, I., Hilger, T., Kalimbira, A., Glas, M. G., et al. (2023). Agricultural diversity linkage to income, wealth, diets and nutrition: case of Lilongwe district in Malawi. Sci. Afr. 19:e01569. doi: 10.1016/j.sciaf.2023.e01569
Fite, M. B., Tura, A. K., Yadeta, T. A., Oljira, L., and Roba, K. T. (2022). Factors associated with food consumption score among pregnant women in Eastern Ethiopia: a community-based study. Journal of Health, Population and Nutrition, 41. doi: 10.1186/s41043-022-00286-x
Forsythe, L. (2017). The impact of staple crop value chain participation on the livelihoods of smallholder farmers in Nigeria and Malawi: Changes in poverty, gender relations, and food security. [Doctoral Thesis]. London: University of Greenwish.
Fox, J., and Weisberg, S. (2018). “Bootstrapping regression models in R” in An R companion to applied regression. 3rd ed (Newcastle upon Tyne: SAGE).
Franke, A. C., Van den Brand, G. J., and Giller, K. E. (2014). Which farmers benefit most from sustainable intensification? An ex-ante impact assessment of expanding grain legume production in Malawi. Eur. J. Agron. 58, 28–38. doi: 10.1016/j.eja.2014.04.002
Gassner, A., Harris, D., Mausch, K., Terheggen, A., Lopes, C., Finlayson, R. F., et al. (2019). Poverty eradication and food security through agriculture in Africa: rethinking objectives and entry points. Outlook Agric. 48, 309–315. doi: 10.1177/0030727019888513
Gebru, K. M., Leung, M., Rammelt, C., Zoomers, A., and van Westen, G. (2019). Vegetable business and smallholders’ food security: Empirical findings from Northern Ethiopia. Sustainability (Switzerland), 11. doi: 10.3390/su11030743
Gelli, A., Donovan, J., Margolies, A., Aberman, N., Santacroce, M., Chirwa, E., et al. (2020). Value chains to improve diets: diagnostics to support intervention design in Malawi. Glob. Food Secur. 25:100321. doi: 10.1016/j.gfs.2019.09.006
Goswami, R., Chatterjee, S., and Prasad, B. (2014). Farm types and their economic characterization in complex agro-ecosystems for informed extension intervention: study from coastal West Bengal, India. Agric. Food Econ. 2, 1–24. doi: 10.1186/s40100-014-0005-2
Government of Malawi (2010). A catalogue of agricultural Technologies in Malawi, Ministry of Agriculture and food security. Malawi: Government of Malawi.
Government of Malawi (2017). Fourth Integrated Household Survey, 2016/2017: Household Questionnaire. Malawi: Government of Malawi.
Government of Malawi (2018). National Agricultural Investment Plan (NAIP): Prioritised and Coordinated Agricultural Transformation Plan for Malawi. Malawi: Government of Malawi.
Government of Malawi (2021a). Guide to agriculture production and natural resources Management in Malawi. Agricultural Communication Branch, Department of Agricultural Extension Services. Malawi: Government of Malawi.
Government of Malawi (2022). Malawi National Horticulture Policy (2020–2030) draft, Ministry of Agriculture and Food, Security. Malawi: Government of Malawi.
Guarín, A., Rivera, M., Pinto-Correia, T., Guiomar, N., Šūmane, S., and Moreno-Pérez, O. M. (2020). A new typology of small farms in Europe. Glob. Food Sec. 26:100389. doi: 10.1016/j.gfs.2020.100389
Guillem, E. E., Barnes, A. P., Rounsevell, M. D. A., and Renwick, A. (2012). Refining perception-based farmer typologies with the analysis of past census data. J. Environ. Manag. 110, 226–235. doi: 10.1016/j.jenvman.2012.06.020
Gupta, S., Sunder, N., and Pingali, P. L. (2020). Market access, production diversity, and diet diversity: evidence from India. Food and Nutrition Bulletin, 41, 167–185. doi: 10.1177/0379572120920061
Hazell, P. (2020). “Importance of smallholder farms as a relevant strategy to increase food security” in The Role of Smallholder Farms in Food and Nutrition Security. ed. P. Hazell (Berlin: Springer), 29–43.
Ingutia, R., and Sumelius, J. (2022). Determinants of food security status with reference to women farmers in rural Kenya. Scientific African, 15. doi: 10.1016/j.sciaf.2022.e01114
Innazent, A., Jacob, D., Bindhu, J. S., Joseph, B., Anith, K. N., Ravisankar, N., et al. (2022). Farm typology of smallholders integrated farming systems in southern Coastal Plains of Kerala, India. Sci. Rep. 12:333. doi: 10.1038/s41598-021-04148-0
Ismael, F., Mbanze, A. A., Ndayiragije, A., and Fangueiro, D. (2021). Understanding the dynamic of rice farming systems in southern Mozambique to improve production and benefits to smallholders. Agronomy 11:1018. doi: 10.3390/agronomy11051018
Jollife, I. T., and Cadima, J. (2016). Principal component analysis: a review and recent developments. Philos. Trans. R. Soc. A Math. Phys. Eng. Sci. 374:20150202. doi: 10.1098/rsta.2015.0202
Kabunga, N., Ghosh, S., and Griffiths, J. K.. (2014). Can Smallholder Fruit and Vegetable Production Systems Improve Household Food Security and Nutritional Status of Women? Evidence from Rural Uganda.
Kamara, A., Conteh, A., Rhodes, E. R., and Cooke, R. A. (2019). The relevance of smallholder farming to African agricultural growth and development. Afr. J. Food Agric. Nutr. Dev. 19, 14043–14065. doi: 10.18697/ajfand.84.BLFB1010
Kamau, J. W., Stellmacher, T., Biber-Freudenberger, L., and Borgemeister, C. (2018). Organic and conventional agriculture in Kenya: a typology of smallholder farms in Kajiado and Murang’a counties. J. Rural. Stud. 57, 171–185. doi: 10.1016/j.jrurstud.2017.12.014
Kankwamba, H., Kadzamira, M., and Pauw, K. (2018). How diversified is cropping in Malawi? Patterns, determinants and policy implications. Food Secur. 10, 323–338. doi: 10.1007/s12571-018-0771-x
Kansanga, M. M., Kangmennaang, J., Bezner Kerr, R., Lupafya, E., Dakishoni, L., and Luginaah, I. (2021). Agroecology and household production diversity and dietary diversity: evidence from a five-year agroecological intervention in rural Malawi. Soc. Sci. Med. 288:113550. doi: 10.1016/j.socscimed.2020.113550
Kennedy, G., Berardo, A., Papavero, C., Horjus, P., Ballard, T., Dop, M., et al. (2010). Proxy measures of household food consumption for food security assessment and surveillance: comparison of the household dietary diversity and food consumption scores. Public Health Nutr. 13, 2010–2018. doi: 10.1017/S136898001000145X
Koppmair, S., Kassie, M., and Qaim, M. (2017). Farm production, market access and dietary diversity in Malawi. Public Health Nutr. 20, 325–335. doi: 10.1017/S1368980016002135
Kothari, C. R. (2004). Research methodology: Methods and Techniques. New Delhi: New Age International (P) Ltd.
Kudadze, S., Imoru, A. J., and Adzawla, W. (2019). Farmers’ perception on irrigation farming and the factors influencing access to and size of irrigable lands in northern region, Ghana. Asian Food Sci. J. 1, 1–14. doi: 10.9734/afsj/2019/v8i329994
Kuivanen, K. S., Alvarez, S., Michalscheck, M., Adjei-Nsiah, S., Descheemaeker, K., Mellon-Bedi, S., et al. (2016a). Characterising the diversity of smallholder farming systems and their constraints and opportunities for innovation: a case study from the northern region, Ghana. Wageningen J. Life Sci. 78, 153–166. doi: 10.1016/j.njas.2016.04.003
Kuivanen, K. S., Michalscheck, M., Descheemaeker, K., Adjei-Nsiah, S., Mellon-Bedi, S., Groot, J. C. J., et al. (2016b). A comparison of statistical and participatory clustering of smallholder farming systems - a case study in northern Ghana. J. Rural. Stud. 45, 184–198. doi: 10.1016/j.jrurstud.2016.03.015
Kumar, S., Craufurd, P., Haileslassie, A., Ramilan, T., Rathore, A., and Whitbread, A. (2019). Farm typology analysis and technology assessment: an application in an arid region of South Asia. Land Use Policy 88:104149. doi: 10.1016/j.landusepol.2019.104149
Levin, K. A. (2006). Study design III: cross-sectional studies. Evid. Based Dent. 7, 24–25. doi: 10.1038/sj.ebd.6400375
Lopez-Ridaura, S., Barba-Escoto, L., Reyna, C., Hellin, J., Gerard, B., and van Wijk, M. (2019). Food security and agriculture in the Western Highlands of Guatemala. Food Secur. 11, 817–833. doi: 10.1007/s12571-019-00940-z
Lopez-Ridaura, S., Frelat, R., van Wijk, M. T., Valbuena, D., Krupnik, T. J., and Jat, M. L. (2018). Climate smart agriculture, farm household typologies and food security: an ex-ante assessment from Eastern India. Agric. Syst. 159, 57–68. doi: 10.1016/j.agsy.2017.09.007
Lucia, S., Mason-D, A. D., Bogard, J. R., Herrero, M., Mason-D, D., Bogard, J. R., et al. (2019). Gaps between fruit and vegetable production, demand, and recommended consumption at global and national levels: an integrated modelling study. Articles Lancet Planet Health 3:11.
Makate, C., and Mango, N. (2017). Diversity amongst farm households and achievements from multi-stakeholder innovation platform approach: lessons from Balaka Malawi. Agric. Food Secur. 6, 1–15. doi: 10.1186/s40066-017-0115-7
Mango, N., Makate, C., Mapemba, L., and Sopo, M. (2018a). The role of crop diversification in improving household food security in Central Malawi. Agric. Food Secur. 7:160. doi: 10.1186/s40066-018-0160-x
Mango, N., Makate, C., Tamene, L., Mponela, P., and Ndengu, G. (2018b). Adoption of small-scale irrigation farming as a climate-smart agriculture practice and its influence on household income in the Chinyanja triangle, southern Africa. Land 7:49. doi: 10.3390/land7020049
Matita, M., Chirwa, E. W., Kaiyatsa, S., Mazalale, J., Chimombo, M., Msofi Mgalamadzi, L., et al. (2022). Determinants of smallholder farmers’ livelihood trajectories. Evidence from rural Malawi. Agrekon 61, 399–411. doi: 10.1080/03031853.2022.2107030
Mlalama, K. N., Matemu, A., Kosia, E., Canavan, C. R., Bellows, A. L., Blakstad, M., et al. (2022). Factors influencing the sustainability of homestead vegetable production intervention in Rufiji, Tanzania: a cross-sectional mixed methods study. PLoS Glob. Public Health 2:e0000531. doi: 10.1371/journal.pgph.0000531
Muchenje, A., and Mushunje, V. (2013). Determinants of rural household dietary diversity: the case of Amatole and Nyandeni districts, South Africa. Int. J. Dev. Sustain. 2, 2233–2247.
Musafiri, C. M., Macharia, J. M., Ng'etich, O. K., Kiboi, M. N., Okeyo, J., Shisanya, C. A., et al. (2020). Farming systems’ typologies analysis to inform agricultural greenhouse gas emissions potential from smallholder rain-fed farms in Kenya. Sci. Afr. 8:e00458. doi: 10.1016/j.sciaf.2020.e00458
Muyanga, M., Nyirenda, Z., Lifeyo, Y., and Burke, W. J. (2020). The future of smallholder farming in Malawi.
Mwandira, C. (2008). The status of the vegetable industry in Malawi: Current and future prospects. Books.Google.Com. Available at: https://books.google.com/books?hl=en&lr=&id=vW9WWrjNSXsC&oi=fnd&pg=PA11&dq=The+status+of+the+vegetable+industry+in+Malawi+Current+and+future+prospects)&ots=mbv4FKOPEZ&sig=hM6mfq5MIjaV5nYflUKrDxL097s.
Ngema, P. Z. (2017). Factors affecting the food security status and participation in food security interventions Programmes in Maphumulo, [Master’s Thesis]. South Africa: University of Zululand.
Nhamo, L., Matchaya, G., Nhemachena, C., and Van Koppen, B. (2016). The impact of investment in smallholder irrigation schemes on irrigation expansion and crop productivity in Malawi. Afr. J. Agric. Resour. Econ. 11, 141–153.
Nicholson, C. F., Stephens, E. C., Jones, A. D., Kopainsky, B., Parsons, D., and Garrett, J. (2021). Food security outcomes in agricultural systems models: current status and recommended improvements. Agric. Syst. 188:103028. doi: 10.1016/j.agsy.2020.103028
Nkomoki, W., Bavorová, M., and Banout, J. (2019). Factors associated with household food security in Zambia. Sustainability (Switzerland) 11:2715. doi: 10.3390/su11092715
Norwegian Church Aid. (2020). Final report baseline study for sustainable food Systems for Rural Agriculture Transformation and Resilience (TRANSFORM) program. Norwegian Church Aid.
Ochieng, J., Afari-Sefa, V., Lukumay, P. J., and Dubois, T. (2017). Determinants of dietary diversity and the potential role of men in improving household nutrition in Tanzania. PLoS ONE, 12. doi: 10.1371/journal.pone.0189022
Olatunbosun Benjamin, E. (2020). Smallholder agricultural investment and productivity under contract farming and customary tenure system: a malawian perspective. Land 9:277. doi: 10.3390/LAND9080277
Pacini, G. C., Colucci, D., Baudron, F., Righi, E., Corbeels, M., Tittonell, P., et al. (2014). Combining multi-dimensional scaling and cluster analysis to describe the diversity of rural households. Exp. Agric. 50, 376–397. doi: 10.1017/S0014479713000495
Rajendran, S., Afari-Sefa, V., Shee, A., Bocher, T., Bekunda, M., dominick, I., et al. (2017). Does crop diversity contribute to dietary diversity? Evidence from integration of vegetables into maize-based farming systems. Agric. Food Secur. 6, 1–13. doi: 10.1186/s40066-017-0127-3
Rehima, M., Belay, K., Dawit, A., and Rashid, S. (2013). Factors affecting farmers’ crops diversification: evidence from SNNPR, Ethiopia. Int. J. Agric. Sci. 3, 558–565.
Reincke, K., Vilvert, E., Fasse, A., Graef, F., Sieber, S., and Lana, M. A. (2018). Key factors influencing food security of smallholder farmers in Tanzania and the role of cassava as a strategic crop. Food Secur. 10, 911–924. doi: 10.1007/s12571-018-0814-3
Sambo, T. A., Oguttu, J. W., and Mbombo-Dweba, T. P. (2022). Analysis of the dietary diversity status of agricultural households in the Nkomazi Local Municipality, South Africa. Agric. Food Secur, 11. doi: 10.1186/s40066-022-00387-0
Seltman, H. J. (2018). Experimental design and analysis. Washington, DC: Carnegie Mellon University.
Shah, T., Verma, S., and Pavelic, P. (2013). Understanding smallholder irrigation in sub-Saharan Africa: results of a sample survey from nine countries. Water Int. 38, 809–826. doi: 10.1080/02508060.2013.843843
Shukla, R., Agarwal, A., Gornott, C., Sachdeva, K., and Joshi, P. K. (2019). Farmer typology to understand differentiated climate change adaptation in Himalaya. Sci. Rep. 9:20375. doi: 10.1038/s41598-019-56931-9
Singh, S., Jones, A. D., DeFries, R. S., and Jain, M. (2020). The association between crop and income diversity and farmer intra-household dietary diversity in India. Food Secur. 12, 369–390. doi: 10.1007/s12571-020-01012-3
Takane, T. (2008). Labor use in smallholder agriculture in Malawi: six village case studies. Afr. Study Monogr. 29, 183–200.
Thrupp, A. L. (2000). Linking agricultural biodiversity and food security: The valuable role of agrobiodiversity for sustainable agriculture. Available at: www.wri.org.
Tittonell, P., Muriuki, A., Shepherd, K. D., Mugendi, D., Kaizzi, K. C., Okeyo, J., et al. (2010). The diversity of rural livelihoods and their influence on soil fertility in agricultural systems of East Africa - a typology of smallholder farms. Agric. Syst. 103, 83–97. doi: 10.1016/j.agsy.2009.10.001
Varadan, R. J., Mamidanna, S., Kumar, S., Ahmed, S. Z., and Jaisankar, I. (2022). Technology, infrastructure and enterprise trade-off: Strengthening smallholder farming systems in Tamil Nadu State of India for sustainable income and food security. Outlook Agric. doi: 10.1177/00307270221077380
Keywords: farm typologies, vegetable farming system, food security, food accessibility, household income, smallholder vegetable farmer, Malawi
Citation: Nzima WM, Ip RHL, Bhatti MA, Godfrey SS, Eik LO, Gondwe SR and Divon SA (2024) Diversity and heterogeneity of smallholder vegetable farming systems and their impact on food security and income in Malawi. Front. Sustain. Food Syst. 8:1387912. doi: 10.3389/fsufs.2024.1387912
Edited by:
Albie F. Miles, University of Hawaii–West Oahu, United StatesReviewed by:
Erin Anders, Michigan State University, United StatesSreenath Dixit, International Crops Research Institute for the Semi-Arid Tropics, India
Emmanuel Junior Zuza, Royal Agricultural University, United Kingdom
Zakariya Yahya Algamal, University of Mosul, Iraq
Copyright © 2024 Nzima, Ip, Bhatti, Godfrey, Eik, Gondwe and Divon. This is an open-access article distributed under the terms of the Creative Commons Attribution License (CC BY). The use, distribution or reproduction in other forums is permitted, provided the original author(s) and the copyright owner(s) are credited and that the original publication in this journal is cited, in accordance with accepted academic practice. No use, distribution or reproduction is permitted which does not comply with these terms.
*Correspondence: Wisdom Madede Nzima, d2lzZG9tLm1hZGVkZS5uemltYUBubWJ1Lm5v
†Present Address: Ryan H. L. Ip, Department of Mathematical Sciences, Auckland University of Technology, Auckland, New Zealand
‡ORCID: Wisdom Madede Nzima, https://orcid.org/0000-0001-9259-6494
Shai André Divon, https://orcid.org/0000-0003-2949-457X