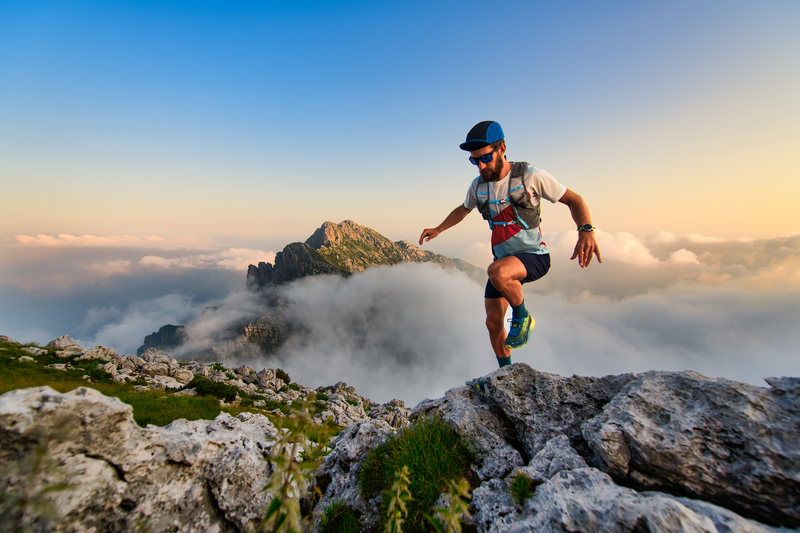
95% of researchers rate our articles as excellent or good
Learn more about the work of our research integrity team to safeguard the quality of each article we publish.
Find out more
ORIGINAL RESEARCH article
Front. Sustain. Food Syst. , 12 June 2024
Sec. Agricultural and Food Economics
Volume 8 - 2024 | https://doi.org/10.3389/fsufs.2024.1385935
This article is part of the Research Topic Strategies Of Digitalization And Sustainability In Agrifood Value Chains View all 23 articles
Digital technology plays a crucial role in advancing sustainable farming and ensuring food security, especially in developing countries. This study evaluates the impact of Internet technology usage on technical efficiency in crop productivity, using data from 600 wheat farmers in rural Pakistan. It addresses the imperative need to enhance agricultural practices within the context of sustainable food production. To achieve this, a matched sample of Internet users and non-users was formed through propensity score matching. The study employs the stochastic frontier method with sample selection adjustment, ensuring a robust evaluation of technical efficiency between these groups. The findings reveal a positive influence of Internet usage on efficiency, persisting even after mitigating self-selection bias from observed and unobserved factors. Internet users exhibit a technical efficiency score of 0.62, surpassing the 0.55 score of non-users. Quantile regression analysis exposes varying impacts of Internet usage on technical efficiency, with less efficient farmers experiencing substantial improvements. Widespread Internet adoption holds the potential to significantly enhance agricultural production for growers. The research underscores the role of promoting Internet utilization to stimulate growth and improve farming efficiency within the evolving digital economy. Policymakers are advised to promote the adoption of modern technology to enhance crop production and support economic growth.
Agricultural production in many countries, particularly in developing nations, is predominantly driven by intensive farming practices, marked by substantial input usage and consumption. This reliance on inputs leads to diminished technical efficiency (TE), which not only impedes the development of local agriculture and compromises food production and quality but also imposes significant pressure on the ecological environment (Fu and Zhu, 2023). Overusing chemical fertilizers and pesticides further exacerbates groundwater pollution, posing grave threats to drinking water and agricultural irrigation in countries such as Pakistan, Bangladesh (Huq et al., 2019), Iran (Ostad-Ali-Askari et al., 2017), and others. There is an urgent need to transition from inefficient to efficient agricultural practices to address these pressing environmental and agricultural challenges.
Enhancing agricultural productivity and efficiency is crucial for ensuring food security and lifting rural communities from poverty. However, many smallholder farmers in developing countries face significant barriers preventing them from reaping agricultural progress benefits. These obstacles include limited access to information about suppliers and markets, high transaction costs, a lack of farming expertise, and difficulty accessing credit (Fu and Zhu, 2023). Specifically, due to information disparities, smallholder farmers, especially those in rural areas, struggle to adopt technologies such as improved seeds, fertilizers, and pesticides or efficiently use available resources. Consequently, these growers experience low crop yields and incomes, undermining their livelihoods and hindering rural development (Khan et al., 2022). Therefore, reducing information gaps through modern technologies is essential to improving farm performance.
The integration of sustainable Internet technology (IT) can mitigate information asymmetry by facilitating the swift and cost-effective distribution of information. Past studies have shown that IT usage enhances farmers’ accessibility to financial and agricultural services (Fu and Zhu, 2023), strengthens their connections to input and output markets, and amplifies their engagement in income-generating endeavors like off-farm employment and social media usage on their sustainable development (Kılıçaslan and Töngür, 2019; Dvorský et al., 2023; Valaskova et al., 2024). Many nations have adopted diverse sustainable Internet-driven programs to bolster farm productivity and foster rural advancement (Ankrah et al., 2023; Zheng and Ma, 2024). These initiatives encompass models like the “Internet-Agriculture-Finance” framework, online farmer field schools, and platforms for sustainable rural e-commerce (Zheng et al., 2021; Khan et al., 2022).
Many studies have delved into the effects of integrating computers, mobile phones, and IT, on-farm performance, and farmers’ welfare (Kaila and Tarp, 2019; Leng et al., 2020). These investigations have tackled the issue of selectivity bias in technology adoption, employing a variety of methodologies, including propensity score matching (PSM), endogenous treatment regression (ETR) models, and instrumental variable (IV) approaches. For instance, Issahaku et al. (2018) utilized a PSM model and discovered that mobile phone usage significantly enhances agricultural productivity in Ghana. Similarly, Ma et al. (2020) employed an ETR model, revealing that Internet utilization notably boosts the income of households and expenditures in rural areas.
IT can influence the TE of crop production by shaping farmers’ production strategies concerning the amalgamation and application of diverse inputs, such as fertilizers, pesticides, labor, and capital assets. TE denotes the ratio of observed output to the maximum achievable output given the existing inputs (Khan et al., 2022; Liu and Liu, 2023), reflecting the effectiveness with which various agricultural inputs are utilized. Existing literature indicates that the use of these modern technologies significantly impacts farmers’ decisions regarding seed and fertilizer usage (Kaila and Tarp, 2019), and land expansion (Zheng et al., 2021). Based on our understanding, apart from the study by Mwalupaso et al. (2019) in Zambia, no prior research has investigated the influence of IT usage on the TE of crop production. Mwalupaso et al. (2019) analyzed the impact of IT usage via mobile phones on the TE of maize production in Zambia and found a significant improvement in farmers’ TE. However, a limitation of the study is its failure to address the issue of unobserved selection bias in IT usage.
This study aims to evaluate the influence of IT usage on the TE of wheat crop production in Pakistan. This study analysis is grounded in survey data collected from 600 wheat farmers across the country. Our focus specifically on wheat production in Pakistan stems from several reasons. First, we adopt a more nuanced approach to IT adoption, concentrating solely on its role in accessing information to enhance wheat crop yields, in contrast to previous studies that relied on broader indicators like overall IT investment or ownership (Battese, 1997; Ramalho et al., 2010; Zheng et al., 2021). This focused approach establishes a direct link between farmers’ IT usage and agricultural output. Second, despite the potential positive impacts of agricultural output on the economy and poverty alleviation (Ma et al., 2018; Khan et al., 2021; Zheng et al., 2021), there has been limited research in this domain, and our study aims to bridge this gap. Third, by employing QTE, policymakers can glean valuable insights into the varied impacts of IT on TE, which can inform the design of tailored and pragmatic solutions to address the specific requirements of diverse crop farmers. This research endeavors to ascertain whether IT utilization influences growers’ decisions regarding input usage, consequently augmenting crop yield efficiency and technological effectiveness in rural Pakistan.
This article is structured into five sections. After the introduction, Section 2 reviews the literature background and presents the conceptual framework. Section 3 outlines the methodology employed. The research findings and discussions are presented in Section 4. Finally, Section 5 summarizes the conclusions and discusses policy implications, limitations, and future directions.
Information and communication technology (ICT) has seen significant advancements in recent decades across various fields. Due to its potential to transform the economy and society, extensive research has been conducted to examine its impact on various aspects (Chandio et al., 2023). Early studies concentrated on production, economic growth, and poverty reduction, and ICT was considered part of the production function alongside land, capital, and labor (Chandio et al., 2023). Numerous studies indicate that ICT positively affects employment (Atasoy, 2013), family income, and labor mobility (Hartje and Hübler, 2017). Some scholars suggest that ICT may help reshape rural economies and narrow the global development gap (Ma et al., 2020). Research on ICT has expanded to encompass various topics, including gender gap reduction (Ojo et al., 2013), entrepreneurship promotion (Afutu-Kotey et al., 2017), and financial empowerment. ICT benefits these factors by enhancing the efficiency of information generation, transmission, and access, reducing search and transaction costs, and enabling more efficient production and management systems.
Several factors influencing agricultural productivity have been identified (Issahaku et al., 2018). Over the last two decades, a significant body of literature has emphasized the role of ICT. Lio and Liu (2006) initially demonstrated the role of ICT in enhancing agricultural output in 81 countries between 1995 and 2000. Subsequent research by various scholars supported these findings. Ogutu et al. (2014) showed that widespread ICT use improves production in small-scale agriculture by addressing information asymmetry. Internet connectivity significantly boosted food production in Vietnam (Kaila and Tarp, 2019), reducing poverty in rural areas (Twumasi et al., 2021). Another study in Pakistan found that mobile phone and Internet usage increased wheat growers’ income, indicating improved marketing and sales efficiency that enhances crop profitability (Khan et al., 2022). Deng et al. (2019) also reported that Internet use enhances resource efficiency and lessens agricultural waste.
Extensive literature evidence supports the Internet’s role in agricultural production, prompting investigations into its causes. These studies highlight increased human capital and information access as key factors. Access to technical agricultural information aids growers in diversifying crops, allocating land and inputs more efficiently (Leng et al., 2020), and expanding their land holdings (Hou et al., 2019), leading to higher productivity. Additionally, addressing challenges like knowledge asymmetry and adverse selection helps farmers make better decisions and exhibit more effective management. Enhancing farmer communication and providing learning opportunities can significantly bolster social capital and information literacy and ultimately influence farmer behavior toward the adoption of more productive agricultural practices. For instance, when farmers have access to Internet-based resources, they tend to exercise greater discernment in using chemical inputs such as fertilizers and pesticides. Additionally, IT platforms have the potential to broaden the social capital of households, fostering an environment conducive to the dissemination and application of production technology (Fu and Zhu, 2023). Similarly, Deng et al. (2022) indicated that Internet use influenced rural growers’ perceptions of ecological contamination in China, suggesting that Internet access can be a valuable tool for promoting environmentally friendly agricultural expansion and mitigating environmental issues.
Furthermore, studies have examined the agriculture industry from various perspectives, analyzing the impact of technological advancements on the incomes of the agricultural sector and rural families. For instance, research suggests that using ICT could effectively reduce income inequality in rural areas (Deng et al., 2022). Moreover, Min et al. (2020), utilizing empirical data from 2008 to 2015, concluded that ICT plays a significant role in driving economic expansion and growth. The assumption that IT usage positively influences rural growers’ well-being is supported by Ma et al. (2020). Nie et al. (2021) provide support for their conclusions. Existing literature commonly acknowledges the positive impact of technology on the agriculture industry. Technology holds promise for improving the economic feasibility of biochar in conventional agriculture while also fostering contributions to the circular economy (Maroušek et al., 2023). Based on the results, this research investigates the impacts of IT on wheat crop output. While some research has focused on different Pakistani crops, most have examined the long-term consequences of climate change. Research centered on crop production differs from ours in several fundamental ways. The most recent study by Lin et al. (2022) is comparable to ours regarding topic choice. Investigators look at the key elements that will boost agricultural output, but, in contrast to our findings, they place a greater emphasis on cooperative participation.
The findings of the study suggest that cooperative contributions positively impact the overall factor efficiency of small- and medium-sized businesses. Specifically, in Pakistan, a limited number of studies have explored the influence of IT usage on crop yield, particularly among those investigating the impact of ICT on the agricultural industry. The information collected in this regard will aid in understanding how the agricultural sector, facing pressure from both demand and supply due to population growth and climate change, may address this issue.
In the following section, we elaborate on key concepts within the productivity framework to clarify the potential pathways through which IT usage can impact the TE of farms. We begin with a simple representation of the production frontier, which signifies the maximum output attainable at each input level. Productivity is quantified by the ratio of aggregated output over aggregated input (Coelli, 1995). Consequently, farms situated on the frontier are deemed technically efficient, while those below are not, as a greater output can be achieved with the same input level, or inputs can be conserved without compromising the output level. Therefore, achieving elevated TE requires either increasing the output with the current inputs or reducing the inputs without compromising the prevailing output.
Internet technology could act as a factor influencing TE for several reasons. First, IT can assist farmers in making informed decisions and guide them toward adopting suitable farming methods. Crop and vegetable farmers in less developed countries face challenges such as a lack of education and experience, restricted access to inputs, and inferior agricultural extension services. By facilitating direct, fast, and global information and idea exchange between farmers and experts and addressing these issues (Hobbs, 1996; Bozoğlu and Ceyhan, 2007; Aker, 2008; Schmidt and Wagner, 2019; Quintana-García et al., 2021; Kang et al., 2023), ICT enhances the transmission of information. Producers may also gain greater access to advice and instruction from reliable professionals.
Second, IT promotes the availability of agricultural inputs with greater quality or cheaper cost and gives information on products and services (Zhu et al., 2021). Farmers may currently acquire the up-to-date market report and are no longer confined to the few options they had previously for keeping up with factor markets. Third, IT can assist rural families in distributing labor and capital more effectively by connecting them with suppliers and consumers and enabling communication (Zanello and Srinivasan, 2014; Hou et al., 2019). Farmers may identify market trends and quickly modify production methods to account for potential risks and losses when they have immediate access to market and pricing information related to agriculture.
Balochistan, the largest province in southwestern Pakistan, covers a vast area of 347,190 square kilometers. Despite being the least populous province, it constitutes 44% of the country’s total land area. The agricultural sector in Balochistan holds significant economic potential (Shami et al., 2016; Abdullah and Ahmed, 2018). Many areas in the province are conducive to agricultural production, but the true potential has not been fully realized due to various challenges. Over 81% of farmers across the province express concerns about issues such as power and water shortages negatively impacting agriculture (Ashraf and Routray, 2013). Provinces serve as the highest administrative units in Pakistan, each with its provincial government. Districts operate as second-level administrative units within a province, while tehsils are sub-district administrative units within a district. Union councils (UCs) represent the smallest rural administrative units within a tehsil.
The current study, conducted from July 2022 to March 2023, focused on the Balochistan province in Pakistan. In total, 600 questionnaires were distributed to wheat farmers using multistage random sampling techniques to collect data. The objective was to ascertain the impact of IT usage on TE in wheat crop production efficiency. The study progressed through seven phases: Pakistan was chosen in the first phase, and Balochistan became the main study area in the second phase. In the third phase, study data were categorized into five districts based on the proportion of agricultural production. The fourth phase involved choosing ten tehsils from the five districts to administer a predetermined questionnaire. In the fifth phase, 20 UCs were nominated from the selected tehsils. The sixth phase randomly monitored 20 villages from these UCs, involving 600 farmers in the seventh phase (see Figures 1, 2).
This study gathered data from wheat farmers using interviews and questionnaires. Recognizing the complexity of the questionnaire supplemented the process with in-depth interviews for a thorough understanding. To enhance reliability, we pre-tested the questionnaire before the main data collection phase. The survey questionnaire encompassed a wide array of information, including the socioeconomic profiles of the farmers, IT usage, and other relevant variables pertinent to the study objectives. Subsequently, the collected data underwent meticulous editing and coding procedures using Stata 14 software. This rigorous process aimed to ensure the accuracy, validity, uniformity, consistency, and completeness of the dataset, thus laying a robust foundation for subsequent analysis and interpretation.
The representative sample size stated above was obtained using a sample size calculation formula developed by Yamane (1973), which is considered best for a homogeneous population. The formula and the number of representative samples obtained using the Equation 1 is given by:
where n is the required sample size; N = size of the population or total number of rural households living in the study areas; and e = precision level, which is assumed to be 5%, as standard.
The variables considered in the current research investigations are displayed in Table 1. The treatment variables for IT use (indicating whether respondents use IT to find information related to crop production) are utilized to categorize farmers into treatment groups of IT users (IT) and the control group of IT non-users (NIT). Output consists of farmers’ crop sales income. Input variables refer to factors used in production. Labor measures the costs of household labor and hired labor. Households facing labor shortages may need to hire extra workers for labor-intensive crop cultivation tasks. This study efficiency assessment considers both paid and unpaid labor costs, following FAO (Lys and Cachia, 2016) methodologies. We calculate labor expenses using the formula [number of unpaid laborers * working days * daily wage]. To determine wages, we apply the principle of opportunity cost, considering the potential earnings in alternative paid employment. Recognizing lower rates of off-farm employment among experienced crop cultivators (Poon and Weersink, 2011), the current study utilizes the average regional wage for crop farming as a proxy for opportunity costs. Land denotes the total size of crop production (in hectares). Fertilizer and pesticide expenditures are included. Regarding determinants of IT use, previous studies have identified household characteristics, local conditions, and geographic attributes (Pick et al., 2015; Issahaku et al., 2018; Mwalupaso et al., 2019). The current study used different variables such as the householder’s gender, age, education, experience, certificate (professional farmer certificate), family burden ratio (the number of family members without income divided by those with income), market (distance to market), government (distance to government administration), cooperative membership, IT training, information literacy (Appendix 1 for the variable definition), social capital (frequency and quality of social contacts); [Appendix 2: this variable was adapted from Fu and Zhu (2023)], and five locational dummy variables (districts) as relevant covariates.
To investigate the impact of IT on TE, we employ a multi-step approach designed to progressively mitigate potential bias arising from both observable and unobservable factors. Initially, we present SF model results on the original (unmatched) sample, recognizing potential selection bias. Subsequently, PSM is utilized to construct a balanced sample of IT users and non-users, addressing bias related to observed characteristics. Then, Greene’s (2010) sample selection model is applied to the matched sample to rectify potential bias stemming from unobserved factors. We then compare TE scores of IT users and non-users resulting from different combinations of these correction procedures, with the most reliable outcomes derived from the sample selection SF model on the matched sample. Finally, QTE can be calculated using observed data, effectively correcting for selection bias by comparing quantiles of the outcome distribution among individuals with varying treatment values. This analytical approach facilitates an understanding of whether the influence of IT varies depending on the efficiency level within the agricultural sector.
Technical efficiency measures an individual’s capacity to maximize outcomes from specified inputs, and its assessment can employ various methodologies, such as the SF method and the data envelopment analysis model. The SF method is considered a parametric approach that incorporates symmetric variables to address statistical noise and one-sided factors to account for inefficiencies, rendering it less susceptible to measuring errors (Førsund et al., 1980; Bauer, 1990; Batiese, 1992; Bitsch, 2005). SF accurately measures efficiency but relies on specific assumptions, making it sensitive to deviations and outliers in data, which can affect its precision. Despite these limitations, SF remains a valuable tool for assessing TE in diverse economic settings. The fundamental structure of Equation 2 is outlined as follows.
where is the parameters vector to be assessed. is the production of individual. The group of is an independent input variable. signifies omitted variables, function from error, and dimension error term, and represents a non-negative ran variable capturing the inadequacy influence.
In the existing study, we employ a translog “transcendental-logarithmic” SF method as a flexible, Equation 3 functional structure by processes of production that approximate the productivity technology as follows:
TE is referred to as the ratio of the experiential output of SF outcome Equation 4 and could be computed as follows (Jondrow et al., 1982; Batiese, 1992):
The existing investigation aims to determine the average impact of IT on the TE of agricultural families. Simply comparing TE scores between IT utilizers and non-utilizers groups without accounting for variations in the initial situations of the two grower groups cannot accurately replicate the influence of IT. In 1974, Rubin introduced a counterfactual paradigm called the Rubin causal model (RCM) (Rubin, 1974). Cook et al. (2002) define the counterfactual as the likely outcome or condition of events that would occur if a particular factor, such as IT, did not exist. The primary concern is understanding how the TE of crop growers might have changed if they had not utilized IT. Although such a scenario has never been observed, the PSM method, proposed by Rosenbaum and Rubin (1983), is employed to generate a control cluster with the same identified attributes as the treatment cluster, yielding a counterfactual result. Based on the RCM, this study categorizes sample households into a treatment group of Internet technology (IT) users and a control group of non-Internet technology (NIT) users. We utilize i to represent the individual grower and to specify whether or not grower i uses IT.
In the next stage, probit regression is utilized to evaluate a farmer’s propensity score (P-score), described as the conditional probability (zi), predicting an individual’s adoption of IT based on the observed attributes zi. The covariates the current study chose to match IT users and non-users included households’ sex, education, age, experience, certificate, government distance, market distance, household burden rate, cooperative membership, training, social capital, information literacy, and position variables. Moreover, the PSM is assessed as follows in Equation 5:
Each IT utilizer is paired with a comparable non-utilizer based on the intended P-score. We explore various matching algorithms to assess the effectiveness of reducing selection bias. This research evaluates the implementation of radius, kernel matching, and nearest neighbor techniques, revealing that all three methods yield similar results regarding bias reduction. The optimal results are achieved through Gaussian kernel matching, showcasing a balanced trade-off between matching quality and sample size.
In Equation 6, we use the standardized bias “S” to assess whether the distribution of pertinent variables is balanced between the treatment and control group following matching. There should not be any substantial variations between the variables once the propensity score has been conditioned. The formula for S is as follows:
where denote the mean and variance of the covariate for each group. Usually, the standardized bias should not be greater than 10% (Rosenbaum and Rubin, 1983).
The corrected selection SF model aims to mitigate unobserved bias, particularly self-selection bias, by leveraging the PSM technique. The assumption of unconfoundedness underpins PSM, asserting that all factors influencing both acceptance choices and outcome variables are adequately accounted for. Failure to consider the association between unobservable elements impacting outcomes and those influencing the selection method can lead to biased and inconsistent estimators with traditional regression procedures (Greene, 2010; Lai, 2015; Bravo-Ureta et al., 2021; Vrachioli et al., 2021). Consequently, the selection bias stemming from unobservable variables is rectified using the selection-corrected SF model.
The SF method with sample selection comprises three key formulas.
(i) Selection Equation:
The selection equation, denoted as Di, captures the likelihood of adopting IT to access crop production information. Here, hi represents a vector of individual factors influencing farmers’ choices, y denotes the corresponding coefficients, and ei represents the normalized error term. The outcome variable Di is binary, taking a value of 1 if Di > 0 (indicating adoption of IT) and 0 otherwise in Equation 7.
(ii) Frontier Equation:
The frontier equation calculates the outcome variable based on the selected production technology set. It accounts for two probable sets of production technologies, represented by vectors . These technologies are influenced by the variables v1i, u1i, v2i, & u2i v1i,. and represent the production functions corresponding to the selected technologies. When , the outcome is determined by and when , it is determined by . The variables vi, v1i, and v2i represent the symmetric errors associated with the frontier Equation 8.
(iii) Symmetrical Errors in Corrected Selection SF Model
The equation presented represents the distribution of three symmetrical errors in the corrected selection SF model. These errors denoted as , , and which are crucial in understanding and addressing biases in the model. They are constrained to be uncorrelated with the explanatory variable vectors and are treated as a set of bivariate normal random vectors to compute the likelihood function for Eqs 7 and 8.
The equation is as follows:
where ρ1, ρ2, and ρ12 represent correlation coefficients between errors, with ρ1 and ρ2 indicating correlations between ei and v1i and v2i, respectively. ρ12 signifies the correlation between v1i and v2i. The parameters and denote variances of and , reflecting their variability. The covariance σv12 and σv2 illustrates how changes in one error relate to changes in the other, indicating their joint variability (Eq. 9). Understanding these parameters is crucial for accurate modeling and interpretation of error behavior.
A two-step technique is employed to calculate this equation system (Greene, 2010; Lai, 2015). First, the selection equation (Eq. 7) is estimated using the probit model to determine likelihood estimators ρ1 and ρ2. Then, using these estimators, the frontier model (Eq. 8) is measured. The latent components v1i or v2i influencing Yi are connected to unobservable feature ei that affects the selection method, provided that either ρ1 or ρ2 is non-zero. In the absence of non-zero values for ρ1 or ρ2, the endogenous self-selection bias arising from unobserved variables can be feasibly neglected.
The equation represents the computation of the QTE. QTEτ denotes the QTE at a specific quantile level, denoted by τ. However, QτIT represents the quantile of the outcome distribution for individuals who received the treatment (IT stands for “With Treatment”). QτNIT represents the quantile of the outcome distribution for individuals who did not receive the treatment (NIT stands for “No Treatment”) (Eq. 10).
Table 1 presents the means and standard deviations for both the combined treatment (IT users) and control (IT non-users) groups. The IT group includes 310 observations, while the NIT group comprises 290. On average, household heads in the IT group are approximately 48 years old, compared to 53 years old in the NIT group. This age difference aligns with research indicating that older individuals are less likely to innovate and utilize the Internet for entertainment (Nguyen et al., 2023). Additionally, Internet users tend to have higher levels of education, possibly due to lower-educated individuals lacking IT skills or facing difficulties with comprehensive texts (Močnik and Širec, 2010). Penard et al. (2015) also demonstrate that younger and better educated individuals are more inclined to the Internet. Regarding gender, approximately 84% of households in the IT group are male-headed, which is approximately 5% higher than in the NIT group. Moreover, farmers affiliated with cooperatives are more likely to use the Internet, possibly because growers find it easier to understand and utilize modern technologies. Furthermore, farmers with greater farming experience are more inclined to adopt IT. These findings collectively emphasize the need for targeted interventions and support mechanisms to promote the widespread adoption of digital tools in agriculture, ultimately fostering enhanced TE and productivity in food production.
The results of the selection-corrected SF and conventional SF models are presented in Table 2 for both the IT users and non-users groups, utilizing the entire samples. One exhibits statistical significance at the 1 % level, indicating the need to consider the SF method with corrective selection. The conventional SF model is also refuted by the likelihood ratio (LR) tests conducted under both regimes. The first-order constants can be interpreted as outcome elasticities computed at the sample mean by categorizing all variables based on their geometric values before calculation. This interpretation holds because all variables are segmented using their geometric means, as estimated previously (Orea, 2002). IT users display an output elasticity of 0.30, signifying that a 1% increase in fertilizer usage will result in a 0.3% boost in output. For growers utilizing IT, land has the most significant impact on agricultural output, with an output elasticity of 0.48, as per findings from earlier research on vegetable productivity in Sri Lanka (Padmajani et al., 2014). Reduced yields in vegetable cultivation may be attributed to growers using excessive amounts of chemicals to mitigate the risk of crop loss due to illness and pests. In the case of IT non-users, land size has the largest elasticity (0.30), while fertilizer and other inputs contribute approximately 0.26 and 0.23, respectively, to production. Compared to labor, pesticides exhibit a lower production elasticity of 0.1%. Our estimates align with previous investigations (Dong et al., 2019).
The cumulative fractional productivity elasticities for the IT user and non-user groups sum to approximately 1, indicating a consistently sized regression that remains robust to the subsequent results (Shrestha et al., 2016). Standard TE scores for the unmatched are presented in Table 3 for both the conventional and selection-corrected SF approaches. In the conventional SF model, IT users exhibit an average TE score of 0.62, while the non-users group has a score of 0.57. The selection-corrected SF method is anticipated to yield slightly higher TE scores. When measuring unobservable bias, the TE value for the non-users group increases by 0.03, whereas it only increases by 0.01 for IT users. The assessment of unobserved bias reveals that the two-group frontier TE values in the selection-corrected SF demonstrate a positive effect of utilizing IT on crop producers’ TE.
This study used PSM to decrease the observed bias between IT users and non-users. After calculating P-scores and matching, Table 4 shows that the standardized biases of variables greatly decrease after matching, with all absolute values lowered to less than 10%, demonstrating the success of the matching procedure. To verify the trustworthiness of matching, we must analyze the covariate balance between IT and non-IT groups. Table 4 shows how the unmatched sample fails to attain a covariate balance. However, following matching, standardized bias is significantly decreased, with absolute levels. Furthermore, the t-test findings fail to reject the null hypothesis that there are no systematic distributional differences between the two groups, confirming the efficacy of the matching procedure.
The Probit model marginal effects and constants in Table 5 elucidate how various factors impact growers’ decisions to use IT for information gathering. Gender exerts a strong and favorable influence on IT use, suggesting that male growers are more inclined to use IT for information gathering than their female counterparts. Conversely, the age of respondents has a substantial and adverse effect on the choice to utilize IT, indicating that older growers are less likely to adopt IT, consistent with the belief that senior farmers may possess lower IT abilities. Despite the general trend, experienced farmers, while more knowledgeable about technology adoption, are also more likely to employ IT for agriculture-related information (Okello et al., 2012; Paustian and Theuvsen, 2017). Family members residing near government facilities or with access to IT-related training in town exhibit a greater motivation to adopt IT, as they tend to be more open to positive initiatives to improve farming and assist producers (Kiiza and Pederson, 2012). Furthermore, participation in agricultural cooperatives, which often promote IT adoption and disseminate information through IT channels, increases the likelihood of IT utilization (Abdul-Rahaman and Abdulai, 2018). As highlighted in earlier research (Aker, 2011), a higher information literacy score is crucial for IT adoption and optimizing available resources. In particular, cooperative membership and certificate ownership can influence TE, potentially biasing the outcome. To address this issue of endogeneity, Wooldridge’s (2015) two-stage control function model is employed. The coefficients of the generalized residuals for the certificate and cooperative variables, presented in Table 4 as predictions from the initial phase of the control function, indicate that cooperation and certification are indeed endogenous in the IT selection model, with both associated coefficients being statistically significant.
The parameter estimate findings for the selection-corrected SF and conventional methods for the matched samples are presented in Table 6. The returns to scale and output elasticities of both models do not differ significantly from the unsampled dataset. The results from the selection-corrected SF method indicate that the coefficient of the sample selection bias variable ρ1 for the IT group is statistically different from zero, consistent with a random sample. The current research, which examines traditional SF for IT users, once again highlights questions related to selection bias. The significant value of ρ2, indicating a selection bias of IT non-users in SF, is unsupported by any empirical evidence.
According to the findings in Table 3, IT users had average TE values after matching 0.61 in conventional SF and 0.62 in selection-adjusted SF, respectively, compared to 0.55 for IT non-users in both indicators. In the matched sample, our analysis reveals that the TE variance among the IT users’ and non-users’ groups is larger than in the mismatched group, increasing from 0.03 to 0.07. Consequently, if selection bias induced by both apparent and unobserved factors is disregarded, the mean TE variance between users and non-users may be understated. This result aligns with research conducted in past studies. There are a few factors to consider regarding the TE mean score. First, existing results are consistent with recent studies (Dong et al., 2019; Liang et al., 2019), with an average value of approximately 0.062. However, compared with neighboring countries’ crop growers globally, including Vietnam, where growers had an average productivity value of 0.74 (Nguyen et al., 2021, 2023), or India, where the score is 0.77 (Murthy et al., 2009), Pakistani crop growers seem to have inferior TE values. One probable explanation for this disparity is Pakistan’s land tenure structure, which may not be as favorable to efficient crop-growing techniques as in other nations. Farmers’ capacity to make investments in land resources and increase TE is constrained by land utilization or transfer limitations.
Second, crop growers often have fewer effective scores than other crop producers in Pakistan. For instance, the TE values for fruit growers and crop farms were determined to be 0.83 and 0.9, respectively. One of the causes of this mismatch is the labor-intensive nature of farming operations, which includes activities such as hand weeding, several harvests, and various insect management (Stringer et al., 2009). Additionally, compared to certain other crops, vegetables are more sensitive to environmental conditions such as temperature variations, water availability, and soil health (Tripathi et al., 2016). Furthermore, the progress of farmers’ TE may be hampered by a lack of institutional and socioeconomic assistance, including cooperative help and extension services (Hongyun et al., 2020; Zheng et al., 2021). These outcomes imply a requirement for more empirical research as the efficiency impacts can be country or crop-specific. It is also important to keep in mind that the efficiency score amount can be impacted by various productivity evaluation techniques and variable settings (Madau, 2012, 2015).
Understanding the diverse impact of IT on TE is crucial for developing effective agricultural development strategies. To achieve this, we utilize the residualized quantile regression (RQR) model as suggested (Nascimento et al., 2019; Borgen et al., 2021; Korkmaz et al., 2021), providing a flexible method to assess treatment effects across the distribution of results. In the existing study, the RQR model is calculated in two steps. First, to decompose the variation in the treatment variable into two different mechanisms, one that can be described through the examined control variable and one that is orthogonal to a control variable the treatment variable (IT) is adjusted for the control variable using ordinary least squares. The residualized treatment variable is regressed in the second phase using the minimal absolute deviation approach. Finally, QTE can be calculated using observed data while correcting for selection bias by comparing quantiles (τ) of the outcome distribution for individuals with different treatment values of equation QTE.
The outcomes in Table 7 shed light on this investigation. Apart from the 90th quantile, coefficients demonstrate a positive and statistically significant correlation between IT usage and TE, mirroring our prior findings. In particular, there is a marginal uptick in the coefficient for IT treatment from the 10th to the 25th percentile. The most substantial impact manifests at the 25th percentile, showcasing a coefficient of 0.116, suggesting that IT adoption notably enhances farm efficiency at lower distribution quantiles (Zheng et al., 2021). These results imply that embracing IT offers more significant advantages to farms initially operating at lower efficiency levels, as they possess greater potential for enhancement. Conversely, at the 90th percentile, the effect is statistically insignificant, hinting that IT utilization holds less sway over the most efficient farms. This may be attributed to their already optimized production processes, possibly extensively leveraging other information channels.
Enhancing the TE of agricultural production remains a pressing concern in Pakistan, reflecting challenges encountered by numerous developing nations. A recent study delves into this issue by drawing insights from a sample of 600 farmers in rural Pakistan, aiming to discern the impact of IT utilization on the TE of crop production. The study employs the SF and PSM models to mitigate biases stemming from observed and unobserved factors. The research findings underscore that, when accounting for these biases, the disparity in TE between IT users and non-users holds both financial and scientific significance. This suggests that integrating IT into wheat crop production can yield positive outcomes for rural areas. Further exploration through the QTE method reveals a nuanced relationship between IT adoption and TE. The most pronounced effects are observed among the least efficient farmhouses, gradually diminishing in significance toward the median and ultimately becoming non-significant for farmhouses achieving maximum yield. This nuanced perspective highlights the varying impacts of IT on TE across different efficiency levels in the context of wheat crop production in rural areas.
The findings of the study have some important policy implications. First, emphasizing the positive impact of IT usage on crop production efficiency underscores the need for policymakers to invest in rural IT infrastructure and reduce access costs to promote technology adoption in rural areas. Second, tailored policies promoting emerging technologies should consider the diverse characteristics of smallholder farmers, with efforts to enhance access to information through various channels, including traditional agricultural services, farmers’ organizations, and digital platforms. Third, policymakers can establish technical training centers to provide advisory services, improve rural education opportunities, and facilitate technology adoption among farmers. Finally, while IT adoption is crucial, policymakers should diversify support mechanisms by collaborating with financial institutions, research bodies, cooperatives, and agricultural enterprises to offer financial, technological, and production support.
This study has some limitations. First, its focus only on wheat production may restrict the applicability of findings to other crops due to differences in agricultural extension services. Second, the small sample size limited to one province may compromise the representativeness of the results. Finally, using cross-sectional data prevents exploration of the dynamic impact of IT usage on TE over time. Future research should aim to address the limitations of the study. First, expanding the scope of crops studied will provide a more comprehensive understanding of the impact of IT on agriculture beyond wheat production. Second, increasing the sample size and considering multiple provinces can enhance the representativeness and generalizability of the results. Finally, utilizing longitudinal or panel data analysis techniques can facilitate the exploration of the dynamic effects of IT usage on TE.
The original contributions presented in the study are included in the article/Supplementary material, further inquiries can be directed to the corresponding authors.
Ethical review and approval was not required for the study on human participants in accordance with the local legislation and institutional requirements. Written informed consent from the [patients/participants OR patients/participants legal guardian/next of kin] was not required to participate in this study in accordance with the national legislation and the institutional requirements.
BA: Writing – review & editing, Writing – original draft, Methodology, Formal analysis, Data curation, Conceptualization. ZZ: Writing – review & editing, Visualization, Supervision, Resources, Project administration, Investigation, Funding acquisition. XJ: Writing – review & editing, Supervision, Project administration, Conceptualization. HG: Writing – review & editing, Visualization, Validation, Project administration, Methodology, Investigation, Funding acquisition. NK: Writing – review & editing, Writing – original draft. YY: Supervision, Writing – review & editing.
The author(s) declare that no financial support was received for the research, authorship, and/or publication of this article.
The authors declare that the research was conducted in the absence of any commercial or financial relationships that could be construed as a potential conflict of interest.
All claims expressed in this article are solely those of the authors and do not necessarily represent those of their affiliated organizations, or those of the publisher, the editors and the reviewers. Any product that may be evaluated in this article, or claim that may be made by its manufacturer, is not guaranteed or endorsed by the publisher.
The Supplementary material for this article can be found online at: https://www.frontiersin.org/articles/10.3389/fsufs.2024.1385935/full#supplementary-material
Abdullah, R., and Ahmed, N. (2018). Political status of Balochistan from 1971 to 1988. Pakistan Study Centre 8, 325–334.
Abdul-Rahaman, A., and Abdulai, A. (2018). Do farmer groups impact on farm yield and efficiency of smallholder farmers? Evidence from rice farmers in northern Ghana. Food Policy 81, 95–105. doi: 10.1016/j.foodpol.2018.10.007
Afutu-Kotey, R. L., Gough, K. V., and Owusu, G. (2017). Young entrepreneurs in the mobile telephony sector in Ghana: from necessities to aspirations. J. Afr. Bus. 18, 476–491. doi: 10.1080/15228916.2017.1339252
Aker, J. C. (2008) Does digital divide or provide? The impact of cell phones on grain markets in Niger. Center for Global Development working paper.
Aker, J. C. (2011). Dial “a” for agriculture: a review of information and communication technologies for agricultural extension in developing countries. Agric. Econ. 42, 631–647. doi: 10.1111/j.1574-0862.2011.00545.x
Ankrah, M. T., Asante, D., Wang, P., Ntiamoah, E. B., and Jiang, Y. (2023). Can the use of the internet improve fish farmers' financial performance? Evidence from Ghana. Mar. Policy 149:105494. doi: 10.1016/j.marpol.2023.105494
Ashraf, M., and Routray, J. K. (2013). Perception and understanding of drought and coping strategies of farming households in north-West Balochistan. Int. J. Disaster Risk Reduct. 5, 49–60. doi: 10.1016/j.ijdrr.2013.05.002
Atasoy, H. (2013). The effects of broadband internet expansion on labor market outcomes. ILR Rev. 66, 315–345. doi: 10.1177/001979391306600202
Batiese, G. E. (1992). Frontier production functions and technical efficiency: a survey of empirical applications in agricultural economics. Agric. Econ. 7, 185–208. doi: 10.1111/j.1574-0862.1992.tb00213.x
Battese, G. E. (1997). A note on the estimation of cobb-Douglas production functions when some explanatory variables have zero values. J. Agric. Econ. 48, 250–252. doi: 10.1111/j.1477-9552.1997.tb01149.x
Bauer, P. W. (1990). Recent developments in the econometric estimation of frontiers. J. Econ. 46, 39–56. doi: 10.1016/0304-4076(90)90046-V
Bitsch, V. (2005). Qualitative research: a grounded theory example and evaluation criteria. J. Agribus. 23, 75–91. doi: 10.22004/ag.econ.59612
Borgen, N. T., Haupt, A., and Wiborg, Ø. N. (2021) A new framework for estimation of unconditional quantile treatment effects: The Residualized quantile regression (RQR) model. doi: 10.31219/osf.io/4vquh
Bozoğlu, M., and Ceyhan, V. (2007). Measuring the technical efficiency and exploring the inefficiency determinants of vegetable farms in Samsun province, Turkey. Agric. Syst. 94, 649–656. doi: 10.1016/j.agsy.2007.01.007
Bravo-Ureta, B. E., González-Flores, M., Greene, W., and Solís, D. (2021). Technology and technical efficiency change: evidence from a difference in differences selectivity corrected stochastic production frontier model. Am. J. Agric. Econ. 103, 362–385. doi: 10.1111/ajae.12112
Chandio, A. A., Gokmenoglu, K. K., Khan, I., Ahmad, F., and Jiang, Y. (2023). Does internet technology usage improve food production? Recent evidence from major rice-producing provinces of China. Comput. Electron. Agric. 211:108053. doi: 10.1016/j.compag.2023.108053
Coelli, T. J. (1995). Recent developments in frontier modelling and efficiency measurement. Aust. J. Agric. Econ. 39, 219–245. doi: 10.1111/j.1467-8489.1995.tb00552.x
Cook, T. D., Campbell, D. T., and Shadish, W. (2002). Experimental and quasi-experimental designs for generalized causal inference. Boston, MA: Houghton Mifflin.
Deng, X., Song, Y., He, Q., Xu, D., and Qi, Y. (2022). Does internet use improve farmers’ perception of environmental pollution? Evidence from rural China. Environ. Sci. Pollut. Res. 29, 44832–44844. doi: 10.1007/s11356-022-19076-3
Deng, X., Xu, D., Zeng, M., and Qi, Y. (2019). Does internet use help reduce rural cropland abandonment? Evidence from China. Land Use Policy 89:104243. doi: 10.1016/j.landusepol.2019.104243
Dong, Y., Mu, Y., and Abler, D. (2019). Do farmer professional cooperatives improve technical efficiency and income? Evidence from small vegetable farms in China. J. Agric. Appl. Econ. 51, 591–605. doi: 10.1017/aae.2019.22
Dvorský, J., Bednarz, J., and Blajer-Gołębiewska, A. (2023). The impact of corporate reputation and social media engagement on the sustainability of SMEs: perceptions of top managers and the owners. Equilibrium 18, 779–811. doi: 10.24136/eq.2023.025
Førsund, F. R., Lovell, C. K., and Schmidt, P. (1980). A survey of frontier production functions and of their relationship to efficiency measurement. J. Econ. 13, 5–25. doi: 10.1016/0304-4076(80)90040-8
Fu, Y., and Zhu, Y. (2023). Internet use and technical efficiency of grain production in China: a bias-corrected stochastic frontier model. Humanit. Soc. Sci. Commun. 10, 1–13.
Greene, W. (2010). A stochastic frontier model with correction for sample selection. J. Prod. Anal. 34, 15–24. doi: 10.1007/s11123-009-0159-1
Hartje, R., and Hübler, M. (2017). Smartphones support smart labour. Appl. Econ. Lett. 24, 467–471. doi: 10.1080/13504851.2016.1203054
Hobbs, J. E. (1996). A transaction cost approach to supply chain management. Supply Chain Manag. Int. J. 1, 15–27. doi: 10.1108/13598549610155260
Hongyun, Z., Ma, W., and Li, G. (2020) Does internet use improve technical efficiency of banana production in China?
Hou, J., Huo, X., and Yin, R. (2019). Does computer usage change farmers’ production and consumption? Evidence from China. China Agric. Econ. Rev. 11, 387–410. doi: 10.1108/CAER-09-2016-0149
Huq, M. E., Fahad, S., Shao, Z., Sarven, M. S., Al-Huqail, A. A., Siddiqui, M. H., et al. (2019). High arsenic contamination and presence of other trace metals in drinking water of Kushtia district, Bangladesh. J. Environ. Manage. 242, 199–209. doi: 10.1016/j.jenvman.2019.04.086
Issahaku, H., Abu, B. M., and Nkegbe, P. K. (2018). Does the use of mobile phones by smallholder maize farmers affect productivity in Ghana? J. Afr. Bus. 19, 302–322. doi: 10.1080/15228916.2017.1416215
Jondrow, J., Lovell, C. K., Materov, I. S., and Schmidt, P. (1982). On the estimation of technical inefficiency in the stochastic frontier production function model. J. Econ. 19, 233–238. doi: 10.1016/0304-4076(82)90004-5
Kaila, H., and Tarp, F. (2019). Can the internet improve agricultural production? Evidence from Viet Nam. Agric. Econ. 50, 675–691. doi: 10.1111/agec.12517
Kang, S., Ait Sidhoum, A., Frick, F., Sauer, J., and Zheng, S. (2023). The impact of information and communication technology on the technical efficiency of smallholder vegetable farms in Shandong of China. Q Open 3:qoad017. doi: 10.1093/qopen/qoad017
Khan, N., Ray, R. L., Kassem, H. S., Ihtisham, M., AbdullahAsongu, S. A., et al. (2021). Toward cleaner production: can mobile phone technology help reduce inorganic fertilizer application? Evidence using a national level dataset. Land 10:1023. doi: 10.3390/land10101023
Khan, N., Ray, R., Kassem, H., Khan, F., Ihtisham, M., and Zhang, S. (2022). Does the adoption of Mobile internet technology promote wheat productivity? Evidence from rural farmers. Sustain. For. 14:7614. doi: 10.3390/su14137614
Khan, N., Ray, R. L., Kassem, H. S., and Zhang, S. (2022). Mobile internet technology adoption for sustainable agriculture: evidence from wheat farmers. Appl. Sci. 12:4902. doi: 10.3390/app12104902
Khan, N., Ray, R. L., Zhang, S., Osabuohien, E., and Ihtisham, M. (2022). Influence of mobile phone and internet technology on income of rural farmers: evidence from Khyber Pakhtunkhwa Province, Pakistan. Technol. Soc. 68:101866. doi: 10.1016/j.techsoc.2022.101866
Kiiza, B., and Pederson, G. (2012). ICT-based market information and adoption of agricultural seed technologies: insights from Uganda. Telecommun. Policy 36, 253–259. doi: 10.1016/j.telpol.2012.01.001
Kılıçaslan, Y., and Töngür, Ü. (2019). ICT and employment generation: evidence from Turkish manufacturing. Appl. Econ. Lett. 26, 1053–1057. doi: 10.1080/13504851.2018.1529391
Korkmaz, M. Ç., Chesneau, C., and Korkmaz, Z. S. (2021). On the arcsecant hyperbolic normal distribution. Properties, quantile regression modeling and applications. Symmetry 13:117. doi: 10.3390/sym13010117
Lai, H.-P. (2015). Maximum likelihood estimation of the stochastic frontier model with endogenous switching or sample selection. J. Prod. Anal. 43, 105–117. doi: 10.1007/s11123-014-0410-2
Leng, C., Ma, W., Tang, J., and Zhu, Z. (2020). ICT adoption and income diversification among rural households in China. Appl. Econ. 52, 3614–3628. doi: 10.1080/00036846.2020.1715338
Liang, Y., Jing, X., Wang, Y., Shi, Y., and Ruan, J. (2019). Evaluating production process efficiency of provincial greenhouse vegetables in China using data envelopment analysis: a green and sustainable perspective. PRO 7:780. doi: 10.3390/pr7110780
Lin, B., Wang, X., Jin, S., Yang, W., and Li, H. (2022). Impacts of cooperative membership on rice productivity: evidence from China. World Dev. 150:105669. doi: 10.1016/j.worlddev.2021.105669
Lio, M., and Liu, M. C. (2006). ICT and agricultural productivity: evidence from cross-country data. Agric. Econ. 34, 221–228. doi: 10.1111/j.1574-0864.2006.00120.x
Liu, L., and Liu, K. (2023). Can digital technology promote sustainable agriculture? Empirical evidence from urban China. Cogent Food Agric. 9:2282234. doi: 10.1080/23311932.2023.2282234
Lys, P., and Cachia, F. (2016). Handbook on agricultural cost of production statistics. Food and Agriculture Organization of the United Nations: Rome, Italy. Available at: https://openknowledge.fao.org/handle/20.500.14283/ca6411en
Ma, W., Nie, P., Zhang, P., and Renwick, A. (2020). Impact of internet use on economic well-being of rural households: evidence from China. Rev. Dev. Econ. 24, 503–523. doi: 10.1111/rode.12645
Ma, W., Renwick, A., Yuan, P., and Ratna, N. (2018). Agricultural cooperative membership and technical efficiency of apple farmers in China: an analysis accounting for selectivity bias. Food Policy 81, 122–132. doi: 10.1016/j.foodpol.2018.10.009
Madau, F. A. (2012). Technical and scale efficiency in the Italian citrus farming: A comparison between stochastic frontier analysis (SFA) and data envelopment analysis (DEA) models. Available at: https://mpra.ub.uni-muenchen.de/41403/
Madau, F. A. (2015). Technical and scale efficiency in the Italian citrus farming: comparison between SFA and DEA approaches. Agric. Econ. Rev. 16, 15–27. doi: 10.22004/ag.econ.253696
Maroušek, J., Minofar, B., Maroušková, A., Strunecký, O., and Gavurová, B. (2023). Environmental and economic advantages of production and application of digestate biochar. Environ. Technol. Innovation 30:103109. doi: 10.1016/j.eti.2023.103109
Min, S., Liu, M., and Huang, J. (2020). Does the application of ICTs facilitate rural economic transformation in China? Empirical evidence from the use of smartphones among farmers. J. Asian Econ. 70:101219. doi: 10.1016/j.asieco.2020.101219
Močnik, D., and Širec, K. (2010). The determinants of internet use controlling for income level: cross-country empirical evidence. Inf. Econ. Policy 22, 243–256. doi: 10.1016/j.infoecopol.2010.01.002
Murthy, D. S., Sudha, M., Hegde, M., and Dakshinamoorthy, V. (2009). Technical efficiency and its determinants in tomato production in Karnataka, India: data envelopment analysis (DEA) approach. Agric. Econ. Res. Rev. 22, 215–224. doi: 10.22004/ag.econ.57399
Mwalupaso, G. E., Wang, S., Rahman, S., Alavo, E. J.-P., and Tian, X. (2019). Agricultural informatization and technical efficiency in maize production in Zambia. Sustain. For. 11:2451. doi: 10.3390/su11082451
Mwalupaso, G. E., Wang, S., Xu, Z., and Tian, X. (2019). Towards auspicious agricultural informatization—implication of farmers’ behavioral intention apropos of mobile phone use in agriculture. Sustain. For. 11:6282. doi: 10.3390/su11226282
Nascimento, A. C., Nascimento, M., Azevedo, C., Silva, F., Barili, L., Vale, N., et al. (2019). Quantile regression applied to genome-enabled prediction of traits related to flowering time in the common bean. Agronomy 9:796. doi: 10.3390/agronomy9120796
Nguyen, T. T., Do, M. H., Rahut, D., Nguyen, V. H., and Chhay, P. (2023). The impact of internet use on the performance of agricultural cooperatives in Vietnam.
Nguyen, T. T., Nguyen, T. T., and Grote, U. (2023). Internet use and agricultural productivity in rural Vietnam. Rev. Dev. Econ. 27, 1309–1326. doi: 10.1111/rode.12990
Nguyen, T. T., Tran, V. T., Nguyen, T.-T., and Grote, U. (2021). Farming efficiency, cropland rental market and income effect: evidence from panel data for rural Central Vietnam. Eur. Rev. Agric. Econ. 48, 207–248.
Nie, P., Ma, W., and Sousa-Poza, A. (2021). The relationship between smartphone use and subjective well-being in rural China. Electron. Commer. Res. 21, 983–1009. doi: 10.1007/s10660-020-09397-1
Ogutu, S. O., Okello, J. J., and Otieno, D. J. (2014). Impact of information and communication technology-based market information services on smallholder farm input use and productivity: the case of Kenya. World Dev. 64, 311–321. doi: 10.1016/j.worlddev.2014.06.011
Ojo, A., Janowski, T., and Awotwi, J. (2013). Enabling development through governance and mobile technology. Gov. Inf. Q. 30, S32–S45. doi: 10.1016/j.giq.2012.10.004
Okello, J., Kirui, O., Njiraini, G., and Gitonga, Z. (2012). Drivers of use of information and communication technologies by farm households: The case of smallholder farmers in Kenya. doi: 10.5539/JAS.V4N2P111
Orea, L. (2002). Parametric decomposition of a generalized Malmquist productivity index. J. Prod. Anal. 18, 5–22. doi: 10.1023/A:1015793325292
Ostad-Ali-Askari, K., Shayannejad, M., and Ghorbanizadeh-Kharazi, H. (2017). Artificial neural network for modeling nitrate pollution of groundwater in marginal area of Zayandeh-Rood River, Isfahan, Iran. KSCE J. Civ. Eng. 21, 134–140. doi: 10.1007/s12205-016-0572-8
Padmajani, M., Aheeyar, M., and Bandara, M. (2014). Assessment of pesticide usage in up-country vegetable farming in Sri Lanka. Colombo, Sri Lanka: Hector Kobbekaduwa Agrarian Research and Training Institute.
Paustian, M., and Theuvsen, L. (2017). Adoption of precision agriculture technologies by German crop farmers. Precis. Agric. 18, 701–716. doi: 10.1007/s11119-016-9482-5
Penard, T., Poussing, N., Mukoko, B., and Piaptie, G. B. T. (2015). Internet adoption and usage patterns in Africa: evidence from Cameroon. Technol. Soc. 42, 71–80. doi: 10.1016/j.techsoc.2015.03.004
Pick, J. B., Sarkar, A., and Johnson, J. (2015). United States digital divide: state level analysis of spatial clustering and multivariate determinants of ICT utilization. Socio Econ. Plan. Sci. 49, 16–32. doi: 10.1016/j.seps.2014.09.001
Poon, K., and Weersink, A. (2011). Factors affecting variability in farm and off-farm income. Agric. Finance Rev. 71, 379–397. doi: 10.1108/00021461111177639
Quintana-García, C., Benavides-Chicón, C. G., and Marchante-Lara, M. (2021). Does a green supply chain improve corporate reputation? Empirical evidence from European manufacturing sectors. Ind. Mark. Manag. 92, 344–353. doi: 10.1016/j.indmarman.2019.12.011
Ramalho, E. A., Ramalho, J. J., and Henriques, P. D. (2010). Fractional regression models for second stage DEA efficiency analyses. J. Prod. Anal. 34, 239–255. doi: 10.1007/s11123-010-0184-0
Rosenbaum, P. R., and Rubin, D. B. (1983). The central role of the propensity score in observational studies for causal effects. Biometrika 70, 41–55. doi: 10.1093/biomet/70.1.41
Rubin, D. B. (1974). Estimating causal effects of treatments in randomized and nonrandomized studies. J. Educ. Psychol. 66, 688–701. doi: 10.1037/h0037350
Schmidt, C. G., and Wagner, S. M. (2019). Blockchain and supply chain relations: a transaction cost theory perspective. J. Purch. Supply Manag. 25:100552. doi: 10.1016/j.pursup.2019.100552
Shami, S. H., Ahmad, J., Zafar, R., Haris, M., and Bashir, S. (2016). Evaluating wind energy potential in Pakistan's three provinces, with proposal for integration into national power grid. Renew. Sust. Energ. Rev. 53, 408–421. doi: 10.1016/j.rser.2015.08.052
Shrestha, R. B., Huang, W.-C., Gautam, S., and Johnson, T. G. (2016). Efficiency of small scale vegetable farms: policy implications for the rural poverty reduction in Nepal. Agric. Econ. 62, 181–195. doi: 10.17221/81/2015-AGRICECON
Stringer, R., Sang, N., and Croppenstedt, A. (2009). Producers, processors, and procurement decisions: the case of vegetable supply chains in China. World Dev. 37, 1773–1780. doi: 10.1016/j.worlddev.2008.08.027
Tripathi, A., Tripathi, D. K., Chauhan, D., Kumar, N., and Singh, G. (2016). Paradigms of climate change impacts on some major food sources of the world: a review on current knowledge and future prospects. Agric. Ecosyst. Environ. 216, 356–373. doi: 10.1016/j.agee.2015.09.034
Twumasi, M. A., Jiang, Y., Zhou, X., Addai, B., Darfor, K. N., Akaba, S., et al. (2021). Increasing Ghanaian fish farms’ productivity: does the use of the internet matter? Mar. Policy 125:104385. doi: 10.1016/j.marpol.2020.104385
Valaskova, K., Nagy, M., and Grecu, G. (2024). Digital twin simulation modeling, artificial intelligence-based internet of manufacturing things systems, and virtual machine and cognitive computing algorithms in the industry 4.0-based Slovak labor market. Oecon. Copernic. 15, 95–143. doi: 10.24136/oc.2814
Vrachioli, M., Stefanou, S. E., and Tzouvelekas, V. (2021). Impact evaluation of alternative irrigation technology in Crete: correcting for selectivity bias. Environ. Resour. Econ. 79, 551–574. doi: 10.1007/s10640-021-00572-y
Wooldridge, J. M. (2015). Control function methods in applied econometrics. J. Hum. Resour. 50, 420–445. doi: 10.3368/jhr.50.2.420
Zanello, G., and Srinivasan, C. S. (2014). Information sources, ICTs and price information in rural agricultural markets. Eur. J. Dev. Res. 26, 815–831. doi: 10.1057/ejdr.2014.1
Zheng, H., and Ma, W. (2024). Economic benefits of internet use for smallholder wheat farmers. Appl. Econ. 56, 398–413. doi: 10.1080/00036846.2023.2167928
Zheng, H., Ma, W., Wang, F., and Li, G. (2021). Does internet use improve technical efficiency of banana production in China? Evidence from a selectivity-corrected analysis. Food Policy 102:102044. doi: 10.1016/j.foodpol.2021.102044
Keywords: sustainable food system, internet technology, technical efficiency, rural areas, Pakistan, propensity score matching
Citation: Ahmad B, Zhao Z, Jile X, Gultaj H, Khan N and Yunxian Y (2024) Exploring the influence of internet technology adoption on the technical efficiency of food production: insight from wheat farmers. Front. Sustain. Food Syst. 8:1385935. doi: 10.3389/fsufs.2024.1385935
Received: 14 February 2024; Accepted: 21 May 2024;
Published: 12 June 2024.
Edited by:
Isabelle Piot-Lepetit, INRAE Occitanie Montpellier, FranceReviewed by:
Francesco Bozzo, University of Bari Aldo Moro, ItalyCopyright © 2024 Ahmad, Zhao, Jile, Gultaj, Khan and Yunxian. This is an open-access article distributed under the terms of the Creative Commons Attribution License (CC BY). The use, distribution or reproduction in other forums is permitted, provided the original author(s) and the copyright owner(s) are credited and that the original publication in this journal is cited, in accordance with accepted academic practice. No use, distribution or reproduction is permitted which does not comply with these terms.
*Correspondence: Zixiang Zhao, emhhb3ppeGlhbmdAamlzdS5lZHUuY24=; Xing Jile, eGluZ2ppbGVAamlzdS5lZHUuY24=; Humaira Gultaj, aHVtYWlyYWd1bHRhakBnbWFpbC5jb20=
Disclaimer: All claims expressed in this article are solely those of the authors and do not necessarily represent those of their affiliated organizations, or those of the publisher, the editors and the reviewers. Any product that may be evaluated in this article or claim that may be made by its manufacturer is not guaranteed or endorsed by the publisher.
Research integrity at Frontiers
Learn more about the work of our research integrity team to safeguard the quality of each article we publish.