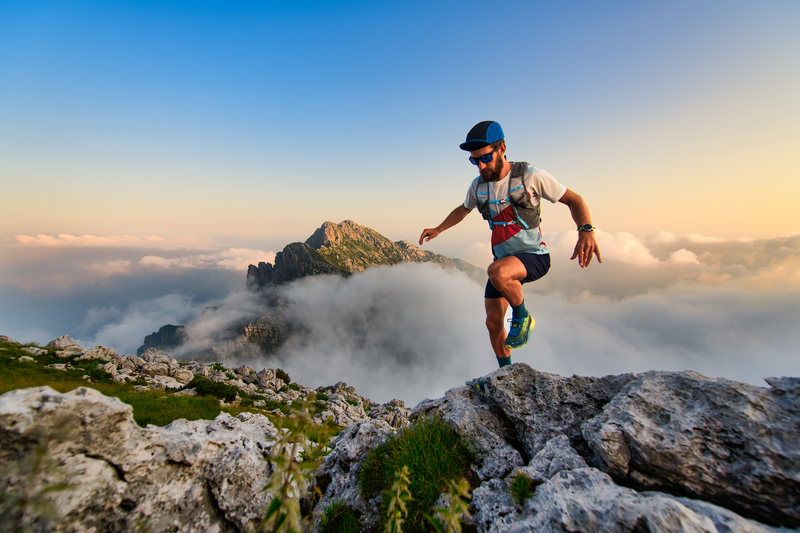
95% of researchers rate our articles as excellent or good
Learn more about the work of our research integrity team to safeguard the quality of each article we publish.
Find out more
ORIGINAL RESEARCH article
Front. Sustain. Food Syst. , 22 May 2024
Sec. Climate-Smart Food Systems
Volume 8 - 2024 | https://doi.org/10.3389/fsufs.2024.1385672
Precision livestock farming (PLF) tools are increasingly used in daily herd management to improve health, welfare, and overall production. While not intended to reduce greenhouse gas (GHG) emissions on farm, PLF tools can do so indirectly by improving overall efficiency, thereby reducing the emissions per unit of product. This work modelled the potential effects of commercially available PLF tools on whole enterprise and product emissions of two average Scottish dairy farm systems (an 8,000 L and 10,000 L herd) using the Agrecalc carbon footprinting tool. Scenarios modelled included an improvement in fertility and an improvement in fertility and yield from the introduction of an accelerometer-based sensor, and an improvement in health from introduction of an accelerometer-based sensor, with and without the use of management interventions. Use of a sensor intended to improve fertility had the largest reduction in total emissions (kg CO2e) of −1.42% for a 10,000 L farm, with management changes applied. The largest reduction in emissions from milk production (kg CO2e) of −2.31% was observed via fertility technology application in an 8,000 L farm, without management changes. The largest reduction in kg CO2e per kg fat and protein corrected milk of −6.72% was observed from an improvement in fertility and yield in a 10,000 L herd, with management changes. This study has highlighted the realistic opportunities available to dairy farmers in low and high input dairy systems to reduce their emissions through adoption of animal mounted PLF technologies.
The number of dairy farmers utilizing precision livestock farming (PLF), or agri-tech, tools in daily management of their enterprises is increasing. These include estrus detection technology, lameness detection technology, in-line milk quality sensors, and many more. Currently, the number of farmers utilizing PLF tools globally is not known, with many farmers not regarding themselves as users, despite utilizing technology daily. Often, literature focuses on adoption of a specific technology in a specific region, i.e., automatic milking system (AMS) adoption in North Holland (Floridi et al., 2013); AMS adoption in Norway (Hansen, 2015); AMS adoption in Idaho (Tejeda et al., 2020). When utilized to their fullest potential, PLF tools can help support animal management, improve animal health (Neethirajan, 2017; Le Cozler et al., 2022), welfare (Berckmans, 2014; Buller et al., 2020) and production (Marino et al., 2021). They can also help monitor or reduce greenhouse gas (GHG) emissions (Hammond et al., 2016; Gabbi et al., 2021), improve overall farm environmental efficiency (Morrone et al., 2022) and improve traceability of livestock products (Kampan et al., 2022), among other uses.
Particularly important for larger herds, PLF tools and technologies can provide more focused use of available labor. Tools are not meant to replace evaluation of stock by a trained stockperson, or the routine visual and auditory information they gather, but to aid them (Wathes et al., 2008). In comparison to existing methods, PLF technologies can allow collection of detailed information in more rapid and accurate ways, with reduced animal handling and the associated negative impacts (Hamilton et al., 2004; Slayi, 2023). Use of PLF technologies is linked to improved decision making on farm, either solely by the farmer or directed by a decision support system aligned with the technology. With increasing herd size, time pressures and poor labor availability, particularly in dairy herds, more focused use of time is important.
Mitigation of GHG emissions is an important policy objective for UK and international governments. In Scotland, agriculture is estimated to account for 20% of the GHG emissions, and significant reductions are needed to meet the emission reduction targets of 75% by 2030 and 90% by 2040 (Scottish Government, 2023). There is not a clear agreement in the literature as to whether one dairy production system provides a more “climate-friendly” alternative to another (Lorenz et al., 2019), with high milk production systems being shown to be lower emitters in some years and lower production systems in others (Toma et al., 2013). Therefore, to reduce emissions across all dairying systems, a focus on increasing efficiency in each individual system could provide opportunities to reduce undesirable outputs, including GHG emissions (Toma et al., 2013).
Although not intended to reduce GHG emissions, PLF technologies can do so indirectly by improving the efficiency of animals and therefore the enterprise. A reduction in GHG emissions from livestock could be achieved by increasing production efficiency and feed efficiency, by lowering production emissions intensity, or a combination of both (Min et al., 2022). The more efficient an animal is, the lower the emissions per unit of product, such as milk or meat (Grossi et al., 2018) across the supply chain or sector. Improving animal production efficiency through adoption of PLF technologies has the potential to maximize the profitability of average and high yielding dairy systems and therefore improves environmental and economic sustainability of the sector (Tzanidakis et al., 2023). For example, on dairy farms, utilizing PLF technology such as an estrus detection tool could reduce GHG emissions through more accurate detection of estrus, leading to more successfully timed artificial insemination (AI). More successful AI reduces days left open, reducing the number of days cows are non-productive on farm – i.e., producing emissions with no product. In beef systems, technology such as automated weigh crates can reduce GHG emissions through closer monitoring of performance and ensuring animals reach target weights quicker. By reaching targets earlier, age at slaughter can be lowered, meaning animals are on farm for less time, requiring less feed, producing less manure and therefore emitting less GHGs. However, it should be noted that commercially available systems are often not peer reviewed for their intended use, and even less so for reducing emissions. In a study of 1,111 publications relating to dairy cattle sensor technologies, only 42 studies (30 tools) met requirements for external validation (Stygar et al., 2021). The aims of this study were therefore to demonstrate the impact of the adoption of a PLF tool intended to improve fertility or health on the emissions of a high and low input dairy farm, based on currently commercially available animal-mounted systems.
Data for Scotland from the Cattle Tracing System (CTS) and the Farm Advisory Service (FAS) Farm Management Handbook (SAC Consulting Ltd, 2022) were utilized in this study. The CTS is a UK governmental database for all cattle in Great Britain for notification of births, movements, and deaths. The FAS is a Scottish Government funded advisory service which aims to provide information and resources on how to increase sustainability and profitability of farms and crofts in Scotland. Data were collated and average values were used to create a baseline dairy farm, typical of those seen in Scotland; 225 cows calving all year-round (AYR), having access to pasture and producing 8,000 L/cow/annum. Utilizing data from the Langhill Dairy Research herd (SRUC, Scotland) and the Farm Management Handbook (SAC Consulting Ltd, 2022), a second baseline dairy farm was created; 225 cow, AYR calving, fully housed herd producing 10,000 L/cow/annum. Diets were formulated utilizing input from SAC Consulting dairy nutritionists to create representative Scottish dairy total mixed rations (TMR), along with typical grazing periods. Diets were formulated to provide 17% crude protein (CP) at 70% digestibility, and comprised of predominantly grass silage plus wholecrop cereal, barley and barley straw, concentrate pellets and minerals. Grazing cows (8,000 L and 8,500 L herds) were assumed to be grazed for 152 days of a 305-day standard lactation. For all herds and scenarios, dry cows were expected to be grazed (60-day dry period). Youngstock were expected to be grazed for 340 days, and fed concentrate in late season when grass is poorer quality and less available, at a rate of 1.5 kg/head/day, as is standard in region.
Baseline farm carbon footprints and PLF technology adoption scenarios were modelled using the Agrecalc carbon footprinting tool (Agrecalc Ltd., Edinburgh). The system boundary for Agrecalc is “cradle-to-gate” meaning all emissions from dairy production from birth to farm gate are included. Agrecalc closely mirrors UK National GHG Inventory reporting and is aligned with PAS2050 carbon footprinting standards (2011). The tool uses methodologies from the latest 2019 refinements of the 2006 Intergovernmental Panel on Climate Change (IPCC) Guidelines for National Greenhouse Gas Inventories. Agrecalc employs IPCC (2019) Teir 2 country-specific emission factors (EFs) for all enteric and manure methane (CH4) emissions. IPCC (2019) Tier 2 calculations are also employed for nitrous oxide (N2O) from excreta deposited on grazing land, manure management and direct emissions from soil following fertilizer and manure application. N2O emissions from crop residues and indirect emissions arising from volatilization and leaching utilize IPCC (2019) Tier 1 methodology. EFs from energy use come from DEFRA (2012). Embedded fertilizer emissions use values form Kool et al. (2012) and imported feed from the Dutch Feedprint database (Vellinga et al., 2013). Agrecalc uses global warming potential over 100 years (GWP) published in the fourth assessment report (AR4). Therefore, CH4 has a GWP of 25 and N2O of 298 (IPCC, 2007). All emissions from co-production (e.g., meat and milk) were allocated on an economic basis. It is important to highlight, these values have been updated in the most recent assessment report (AR6; IPCC, 2022). Model outputs were expressed as both total emissions (kg CO2e) and emissions per unit of product (kg CO2e/kg fat protein corrected milk (FPCM)).
Model inputs for Agrecalc, including units of measurement, are listed in Supplementary Tables 1–3. Estimated impacts of technologies were modelled based on assumptions from validated technologies, expert opinion (SAC Consulting, Edinburgh), and direct experience of similar tools on similar Scottish farms. Scenarios modelled included: (S1) adoption of an accelerometer-based sensor for fertility (ESTRUS TECH); (S2) adoption of an accelerometer-based sensor for fertility and associated yield improvements (ESTRUS TECH + YIELD); and (S3) adoption of an accelerometer-based sensor for health detection (HEALTH TECH). Those parameters expected to be affected by introduction of technology (e.g., calving interval, replacement rate, mortality, yield) and associated feed, bedding and land requirement changes were considered in the model. Modeling was split into 3 groups with each scenario (S1-3) applied within each group. Groups were pre-determined based on the design of a wider study.
Group 1 – Modeling of PLF technology use on a baseline average Scottish dairy farm with 225 cows calving all year-round (AYR), having access to pasture and producing 8,000 L/cow/annum, with no management changes applied, i.e., no change in percentage use of sexed semen.
Group 2 – Modeling of PLF technology use on a baseline average Scottish dairy farm with 225 cows calving AYR, having access to pasture and producing 8,000 L/cow/annum, with management changes applied, i.e., changes in percentage use of sexed semen with improved fertility.
Group 3 – Modeling of PLF technology use on a baseline 225 cow, AYR calving, fully housed herd producing 10,000 L/cow/annum, with management changes applied, i.e., changes in percentage use of sexed semen with improved fertility.
Scenario 1: S1 ESTRUS TECH – Adoption of an accelerometer-based sensor for fertility.
A summary of the input data from each scenario for groups 1–3 is provided in Tables 1–3 respectively, where variations from the baseline farm input in each group are shown in bold. The addition of an accelerometer-based collar intended to improve fertility, through earlier and more accurate detection of estrus, to the baseline dairy farm was modelled with the following assumptions. Introduction of technology resulted in:
1 A reduction in calving interval
2 A corresponding increase in calves born
3 A reduction in replacement rate
4 Associated feed and manure changes:
a Decrease in feed grown for grass silage, whole crop cereal and barley grain and associated fuel, electricity, fertilizer and crop residue emissions
b Decrease in straw purchased for feed and bedding
c Decrease in manure on farm (FYM), while slurry remained constant
5 Associated land requirement changes:
a Reduction in hectares (ha) of grass for grazing.
b Reduction in ha of grass for silage (3 cuts) and aftermath
c Reduction in ha of whole crop cereals and ha of feed spring barley
d Reduction in total land needed
Table 1. Input data for Group 1: baseline 8,000 L dairy farm with no management changes for scenario 1 (estrus technology), scenario 2 (estrus technology + yield change) and scenario 3 (health technology).
Table 2. Input data for Group 2: baseline 8,000 L dairy farm with management changes (changes to sexed semen usage), with scenario 1 (estrus technology), scenario 2 (estrus technology + yield change) and scenario 3 (health technology) applied with management changes.
Table 3. Input data for Group 3: baseline 10,000 L dairy farm with management changes (changes to sexed semen usage) with scenario 1 (estrus technology), scenario 2 (estrus technology + yield change) and scenario 3 (health technology) applied with management changes.
Scenario 2: S2 ESTRUS TECH + YIELD – Adoption of an accelerometer-based sensor for fertility and associated yield improvements.
The data was further modelled to include, in addition to assumptions 1–5 modeled in S1, a 6th assumption of an increase in milk yield, expected from the reduction in replacement rate and corresponding change in herd structure. With a reduced replacement, it was assumed that numbers of 2nd, 3rd, or higher parity cows would increase in the herd, increasing the overall yield.
Scenario 3: S3 HEALTH TECH – Adoption of an accelerometer-based sensor for health detection.
The addition of technology on farm such as an accelerometer-based sensor, intended to improve health, welfare, and production, via earlier detection of diseases and disorders, to the baseline dairy farm was modelled with the following assumptions.
1 A reduction in cow and calf mortality and associated reduction in deaths on farm
2 An increase in yield
3 A reduction in calving interval
4 A corresponding increase in calves born
5 A reduction in replacement rate
6 Associated feed and manure changes:
a Decrease in grass silage, whole crop cereal and barley grain
b Decrease in straw purchased for feed
c Decrease in bedding and purchased straw
d Decrease in manure on farm, while slurry remained constant
7 Associated land requirement changes:
a Decrease in ha of grass grazing
b Decrease in grass for silage (3 cuts) and aftermath
c Decrease in whole crop cereals
d Decrease in ha of feed spring barley
Resource use and emissions for each scenario were compared to the baseline farm for groups 1–3. For all groups and scenarios, most dairy and whole farm emissions (methane, nitrous oxide and energy use) came from enteric fermentation, followed by purchased feed, manure management and fertilizer, respectively. Baseline emissions (kg CO2e/kg FPCM) were higher for the 8,000 L grazing herd with (1.56 kg CO2e/kg FPCM) and without management changes (1.56 kg CO2e/kg FPCM; groups 1 and 2), in comparison to group 3, the 10,000 L housed herd (1.46). For each scenario, the emissions intensities were also lower for group 3, with S3 resulting in the lowest emission intensity of 1.37 kg CO2e/kg FPCM compared to 1.46 kg CO2e/kg FPCM for groups 1 and 2.
Scenario 1, ESTRUS TECH, resulted in a decrease in total emissions from farming, total emissions from milk production and kg CO2e/kg FPCM for all groups. Scenario 2, ESTRUS TECH + YIELD, resulted in an increase in total emissions from farming for all groups. However, for total emissions from milk production and kg CO2e/kg FPCM, a reduction was observed in all groups. Scenario 3, HEALTH TECH modelled as reduced mortality, improved replacement rate (reduced culling) and increased yield (reduction in sub-clinical and clinical diseases) resulted in a decrease in kg CO2e/kg FPCM for all groups. A reduction in total emissions from milk production was also observed for all groups and a reduction in total emissions from farming for group 3.
For group 1, where management changes were not considered in an 8,000 L baseline herd, i.e., assessing the potential impact of technology introduction alone, there was a reduction in total emissions from farming for S1 ESTRUS TECH (−1.03%), while an increase was noted for S2 ESTRUS TECH + YIELD (+0.28%) and S3 HEALTH TECH (+0.43%). Reductions in total emissions from milk were observed for all scenarios, with the largest reduction observed for S1. Reductions were − 2.31% (S1), −0.58% (S2), and − 0.93% (S3) respectively. Reductions in emissions per kg FPCM were observed for all scenarios, with the largest reductions observed for S2 and S3. Reduction in emissions per kg FPCM were − 2.60% (S1), −6.62% (S2), and − 6.62% (S3) (Table 4).
Table 4. Total emissions from farming (kgCO2e) (including emissions from meat), total emissions from milk production (kgCO2e), and kgCO2e/kg FPCM for each scenario and percentage change relative to baseline for group 1, where management changes were not considered in an 8,000 L baseline herd, assessing the impact of technology introduction alone.
For group 2, where management changes were considered alongside technology use in a baseline 8,000 L herd, there was a reduction in total emissions from farming for S1 of only −1.22%. For scenarios S2 and S3, there was an increase in emissions of 0.07 and 0.16%, respectively. For total emissions from milk, there was a reduction observed for all scenarios of −1.77, −0.1%, and − 0.5%, respectively for S1, S2, and S3. A reduction in kg CO2e/kg FPCM was observed for all scenarios, with the largest increased observed for S3 of −6.62%, followed by S2 (−5.94%) and S1 (−1.94%) (Table 5).
Table 5. Total emissions from farming (kgCO2e) (including emissions from meat), total emissions from milk production (kgCO2e), and kgCO2e/kg FPCM for each scenario and percentage change relative to baseline for group 2, where management changes were considered alongside technology use in a baseline 8,000 L herd.
For group 3, where management changes were considered alongside introduction of technology in a 10,000 L herd, there was a reduction in total emissions from farming observed for S1 FERTILITY (−1.42%), and S3 (−0.23%), while an increase was noted forS2 (+0.31%). Reductions in total emissions from milk were observed for all scenarios, with the largest reduction observed for S1. Reductions were − 2.22, −0.82% and − 1.18%, for S1–S3, respectively. Reductions in emissions per kg FCPM were observed for all scenarios, with the largest reductions observed for S3. Reduction in emissions per kg FPCM were − 2.78% (S1), −6.72% (S2), and − 6.36% (S3) (Table 6).
Table 6. Total emissions from farming (kgCO2e) (including emissions from meat), total emissions from milk production (kgCO2e), and kgCO2e/kg FPCM for each scenario and percentage change relative to baseline for group 3, where management changes were considered alongside introduction of technology in a 10,000 L herd.
Application of PLF technologies intended to improve fertility and health and welfare, to both lower yielding grazing and higher yielding housed Scottish dairy systems, with and without management changes, resulted in reductions in total emissions from farming, emissions from milk production and emissions per kg FPCM across some scenarios. As noted by Garnsworthy (2004) in their modeling, the endpoint for consideration of GHG emissions in this model was the individual enterprise. Herd size, fertility and nutrition are all affected by management decisions made at the herd level, in comparison to policy and legislative decisions, such as emissions targets, which are made at national and international levels (Garnsworthy, 2004). Although this model is based on data from an average Scottish dairy herd (lower input, grazing 8,000 L/annum, and higher input, housed, 10,000 L/annum), results from the model could be scaled up to a national level to estimate the impact of technology adoption using the total number of cows/herds in Scotland, or average data from across all system types. However, Rotz et al. (2020) note that lower level, more detailed assessment of dairy farms allows creation of tailored mitigation strategies for individual farms which are more effective than enforcement of uniform strategies at a national level. It is also worth noting that scaling up of this modeling would require assumptions on the level of uptake of these PLF technologies. While technology is prevalent in some regions, its adoption remains slow in others, influenced by several factors such as suitability of new technologies, market availability, and uncertainties regarding return on investment (Russell and Bewley, 2013; Chavas and Nauges, 2020).
Assumptions made in this modeling are thought to be achievable for the two production systems explored. Changes achieved from proposed technology adoption were made based on expert opinion and with the advice of a senior dairy consultant, ensuring that outputs were realistic and achievable on an average farm. However, depending on the individual farm and management aspects there may be a variation in the time taken to reach these changes. Although this work is based on a number of assumptions, they were based on best available knowledge and were within the ranges found in the literature (SAC Consulting Ltd, 2022).
The most consistent reductions achieved in the current research, though not numerically largest, were observed for S1 ESTRUS TECH; introduction of a technology intended to improve fertility, across all groups. With fertility technologies being increasingly popular in dairying systems, this additional benefit (decreased GHG emissions) could further improve farmer uptake. This reduction in emissions through improvements in fertility has been noted in several studies in both dairy (Garnsworthy, 2004; Wilkinson and Garnsworthy, 2017) and beef cattle (Cullen et al., 2016; Smith et al., 2016). Garnsworthy (2004) demonstrated that improved fertility in dairy herds had the potential to reduce methane emissions by 10–11% and ammonia emissions by 9%, achieving this reduction through nutritional strategies for improving fertility. This was measured as probability of conception at each service based on parameters including estrus detection rate, conception rate to first service and to subsequent service and number of services before culling, rather than technologies. However, this large reduction in emissions achieved by Garnsworthy (2004) has been reported to be higher than achievable in practice, with emission reductions being dependent on milk quotas at the time (herd size and productivity), diet and management decisions (Bell et al., 2011). It should be noted that milk quotas have since been phased out.
In all but one case across groups 1–3, there was an increase in total emissions from farming with S2 ESTRUS TECH + YIELD and S3 HEALTH TECH. This is likely because of the increased stock numbers due to improved fertility, reduced mortality, increased feed, and electricity consumption associated with improved yields and herd size changes. However, when considered on a per product basis (kg CO2e/kg FPCM), S2 and S3 resulted in the greatest reduction in emissions for all groups. The lower per product emissions noted for group 3 in comparison to groups 1 and 2 was expected. It has been shown that milk yield per cow influences CH4 emissions; higher milk yields require diets with higher proportions of concentrates and lower neutral detergent fiber (NDF), which would reduce CH4 emissions (Yan et al., 2000; Yates et al., 2000; Garnsworthy, 2004). Additionally, emissions associated with the feed intake to cover energy requirement for maintenance of a higher yielding cow are divided across a higher milk yield (von Soosten et al., 2020).
The difference between the percentage reduction in total emissions from milk production and per kg FPCM between groups 1 (no change in percentage use of sexed semen) and 2 (changes in percentage use of sexed semen with improved fertility) highlights the effects that other factors can have on enterprise emissions and the expected impacts of technology introduction. Differences in management and the overall mix of enterprises mean that effects of technology introduction on emissions intensity will vary greatly between farms. For example, a farm with poorer fertility that would benefit greatly from PLF introduction would be expected to show a greater corresponding reduction in emissions (Baruselli et al., 2023). This is exemplified with group 3, the higher yielding farm with poorer baseline fertility, showing greater reductions in emissions from milk production in comparison to group 2, a lower yielding herd with better fertility.
Results from Scenario 3, improvement in health, which showed a reduction in emissions intensity across all scenarios (per kg product) agreed with previous work by von Soosten et al. (2020) who showed that cows which were retained in the herd longer had reduced emissions intensity. Modeling by von Soosten et al. (2020) found that a cow with five to eight lactations could have emissions intensity 40% lower than those which left the herd after their first lactation. Therefore, monitoring of health via PLF would not only improve animal health and welfare and improve production (reduction in sub-clinical and clinical diseases which limit production), which reduces emissions, but also further reduce emissions intensities through reduced culling. This strategy would also reduce antibiotic usage on farm. As with estrus technologies, demonstration of additional benefits of technology beyond that which they were originally developed for could further improve uptake across the sector (Smith et al., 2007; Kipling et al., 2019).
The differences between total emissions from the whole farm – an increase for some scenarios – and emissions per kg FPCM noted in this study highlights that the most efficient farms (in terms of product emissions) are not always the lowest emitters (in terms of total emissions). Improved fertility and health could lead to more animals with higher liveweights, so although there is more output for emissions to be divided over, total emissions would still be increased. Moreover, if production efficiencies were increased, farmers would likely increase their stock numbers. Therefore, care must be taken that any efficiency gains are made sustainably to avoid an unintended increase in total emissions, i.e., by increasing livestock numbers across the sector as a whole.
While the initial cost of purchasing PLF systems may be unattractive to some enterprises, an improvement in financial performance could be expected through improvements in fertility, health, and the corresponding efficiency improvements if technology is exploited to its full potential (Carillo and Abeni, 2020). Previous work demonstrated that for an average yielding cow, like those in groups 1–2, the net cost of a day of delay in conception is £1.73 (at 2001 prices) when conception is delayed from 85 to 100 days post-calving, increasing to £3.55 per day if conception occurred at 146 to 175 days post-calving (Esslemont et al., 2001). For a higher yielding animal, like group 3, the net cost of one day of delay in conception was calculated at £1.68 when conception is delayed from 85 to 115 days post-calving, increasing to £4.08 per day if conception occurred between 206- and 235-days post-calving (Esslemont et al., 2001). Therefore, earlier, and more accurate detection of estrus using a PLF system could reduce net costs. Negative costs of poorer fertility and delayed conception have been shown in both grazing systems (Shalloo et al., 2014) and fully housed total mixed ration (TMR) systems (Cabrera, 2014). Similarly, high direct and indirect costs from health conditions could be reduced through earlier detection of diseases via PLF systems. A 1997 study of common production diseases and other health issues, such as mastitis, lameness, non-observed estrus, and calf mortality, estimated an average cost of £6,300 per year for a 100-cow herd in England (Kossaibati and Esslemont, 1997). Given the sharp increases in the cost of feed, fertilizer and fuel, it would be expected that all of these costs would be significantly higher in 2023. Other diseases specific studies have shown a cost of €12.10 ± 0.36 per week for a cow suffering from lameness (e.g., digital dermatitis, solar ulcer and white line disease) (Robcis et al., 2023), though did note that preventative trimming could have a larger effect than early detection.
Overall, the scenarios modelled here provide a useful indication of potential emission reductions that could be achieved through appropriate utilization of PLF technologies within typical Scottish dairy herds. It is important to note that our work did not calculate the area of land use change which would occur following the implementation of these measures. Where predictions may suggest a reduction in required cropping land or farm size, on an actual farm, the land for each crop or total land would not reduce with requirement, the farm would simply use the land for another purpose or grow surplus crop to sell. In this model, our assumption was that the land saved was not used for further production. Therefore, though the modeling is indicative of reductions which could be achieved, there would be emissions associated with additional cropping or land utilization which need to be considered in a real farm setting.
Additionally, while improved efficiency leads to reduced emission per kg of product, improved efficiency can also encourage farmers to increase herd sizes to increase their yields. However, in addition to increasing cow numbers leading to an increase in total farm emissions, it is of specific importance in Scotland due to limitations in its dairy processing capacity. In 2021, it was estimated that Scotland had a surplus of 240 million liters of milk (16% of production) that was transported to England for processing [AHDB (Agriculture and Horticulture Development Board), 2021]. Therefore, while increased efficiency and reduced emissions intensity may push farmers toward larger, higher yielding herds, the emissions associated with the haulage of this milk across the border also need to be considered. This current surplus, potential increase in future milk surplus, and the drive for higher efficiency across the entire processing chain may be a useful driving force for increasing the processing capacity in Scotland.
This study is the first of its kind to demonstrate the realistic emissions reductions possible for Scottish dairy farmers if they adopt and utilize PLF technologies for improvement of farm management and efficiency. Although this modeling was based on average Scottish farm data, a similar modeling framework could be used across European dairying systems to evaluate the effects of implementing PLF technologies on emissions. However, it must be noted that changes are farm specific and the extent to which the reduction in GHG emissions could be achieved varies greatly between individual farms and systems. Despite the indicative nature of this modeling, it is a useful tool to demonstrate to industry and farmers that PLF tools can not only improve an animal’s fertility, health, or production as intended, but that they can have a positive impact on total emissions and product emissions, by improving efficiency and resilience in some scenarios and enterprises.
To further encourage adoption of technologies which improve animal health, welfare, and production, while also reducing emissions, more demonstration of additional benefits beyond those that the technology is intended for is needed. In Scotland, we must also consider infrastructure limits, processing capacity and associated transport emissions when considering herd sizes and the positive impacts PLF could have on Scottish dairying emissions. Additionally, to be cost-effective and accessible, future PLF systems need to integrate multiple data streams into one system, reducing the need for multiple systems with increased expenditure.
The raw data supporting the conclusions of this article will be made available by the authors, without undue reservation.
HF: Conceptualization, Data curation, Formal analysis, Investigation, Methodology, Visualization, Writing – original draft, Writing – review & editing. JBo: Conceptualization, Data curation, Investigation, Methodology, Writing – review & editing. LM: Writing – original draft, Writing – review & editing. JBe: Data curation, Investigation, Writing – review & editing. C-AD: Conceptualization, Funding acquisition, Writing – review & editing. RD: Conceptualization, Funding acquisition, Writing – review & editing.
The author(s) declare that financial support was received for the research, authorship, and/or publication of this article. This work was funded by the Scottish Government Rural Affairs and the Environment Portfolio Strategic Research Program RD2.3.9 and Defra funding through FACCE ERA-GAS, ERANET SUSan and ICT-AGRI ERANET in the GrASTech project.
Thank you to Lorna MacPherson of SAC Consulting for providing expert opinion on dairy farm management in Scotland.
JBe was employed by Agrecalc Ltd.
The remaining authors declare that the research was conducted in the absence of any commercial or financial relationships that could be construed as a potential conflict of interest.
All claims expressed in this article are solely those of the authors and do not necessarily represent those of their affiliated organizations, or those of the publisher, the editors and the reviewers. Any product that may be evaluated in this article, or claim that may be made by its manufacturer, is not guaranteed or endorsed by the publisher.
The Supplementary material for this article can be found online at: https://www.frontiersin.org/articles/10.3389/fsufs.2024.1385672/full#supplementary-material
AHDB (Agriculture and Horticulture Development Board) (2021). Scottish Milk balance. Available at: https://ahdb.org.uk/news/scottish-milk-balance
Baruselli, P. S., de Abreu, L. A., de Paula, V. R., Carvalho, B., Gricio, E. A., Mori, F. K., et al. (2023). Applying assisted reproductive technology and reproductive management to reduce CO2-equivalent emission in dairy and beef cattle: a review. Anim. Reprod. 20:e20230060. doi: 10.1590/1984-3143-AR2023-0060
Bell, M. J., Wall, E., Russell, G., Simm, G., and Stott, A. W. (2011). The effect of improving cow productivity, fertility, and longevity on the global warming potential of dairy systems. J. Dairy Sci. 94, 3662–3678. doi: 10.3168/jds.2010-4023
Berckmans, D. (2014). Precision livestock farming technologies for welfare management in intensive livestock systems. Sci. Tech. Rev. Off. Int. des Epizooties 33, 189–196. doi: 10.20506/rst.33.1.2273
Buller, H., Blokhuis, H., Lokhrst, K., Silberberd, M., and Veisser, I. (2020). Animal welfare Management in a Digital World. Animals 10:1779. doi: 10.3390/ani10101779
Cabrera, V. E. (2014). Economics of fertility in high-yielding dairy cows on confined TMR systems. Animal 8, 211–221. doi: 10.1017/s1751731114000512
Carillo, F., and Abeni, F. (2020). An estimate of the effects from precision livestock farming on a productivity index at farm level some evidences from a dairy farms’ sample of lombardy. Animals 10:1718. doi: 10.3390/ani10101781
Chavas, J.-P., and Nauges, C. (2020). Uncertainty, learning, and technology adoption in agriculture. Appl. Econ. Perspect. Pol. Adopt. Agric. Innov. 42, 42–53. doi: 10.1002/aepp.13003
Cullen, B. R., Eckard, R. J., Timms, M., and Phelps, D. G. (2016). The effect of earlier mating and improving fertility on greenhouse gas emissions intensity of beef production in northern Australian herds. Rangeland J. 38, 283–290. doi: 10.1071/RJ15063
DEFRA (2012). Guidelines to Defra greenhouse gas (GHG) conversion factors for company reporting. Food and Rural Affairs, London, UK: Department for Environment.
Esslemont, R. J., Kossabati, M. A., and Allcock, J. (2001). Economics of fertility in dairy cows. BSAP Occas. Publ. 26, 19–29. doi: 10.1017/S0263967X00033565
Floridi, M., Bartolini, F., Peerlings, J., Polman, N., and Viaggi, D. (2013). Modelling the adoption of automatic milking systems in North Holland. Agri 9, 63–66. doi: 10.22004/ag.econ.149226
Gabbi, A. M., Kolling, G. J., Fischer, V., Pereira, L. G. R., Tomich, T. R., Machado, F. S., et al. (2021). Use of infrared thermography to estimate enteric methane production in dairy heifers. Quant. InfraRed Thermogr. J. 19, 187–195. doi: 10.1080/17686733.2021.1882075
Garnsworthy, P. C. (2004). The environmental impact of fertility in dairy cows: a modelling approach to predict methane and ammonia emissions. Anim. Feed Sci. Technol. 112, 211–223. doi: 10.1016/j.anifeedsci.2003.10.011
Grossi, G., Goglio, P., Vitali, A., and Williams, A. G. (2018). Livestock and climate change: impact of livestock on climate and mitigation strategies. Anim. Front. 9, 69–76. doi: 10.1093/af/vfy034
Hamilton, D. N., Ellis, M., Bertol, T. M., and Miller, K. D. (2004). Effects of handling intensity and live weight on blood acid-base status in finishing pigs. J. Anim. Sci. 82, 2405–2409. doi: 10.2527/2004.8282405x
Hammond, K. J., Waghorn, G. C., and Hegarty, R. S. (2016). The GreenFeed system for measurement of enteric methane emission from cattle. Anim. Prod. Sci. 56, 181–189. doi: 10.1071/AN15631
Hansen, B. J. (2015). Robotic milking-farmer experiences and adoption rate in Jæren, Norway. J. Rural. Stud. 41, 109–117. doi: 10.1016/j.jrurstud.2015.08.004
IPCC (2007). Task force on National Greenhouse gas Inventories. Available at: https://www.ipcc-nggip.iges.or.jp/public/2006gl/
IPCC (2019). IPCC 2019 refinement to the 2006 IPCC guidelines for National Greenhouse gas inventories
IPCC (2022). Climate change 2022: impacts, adaptation and vulnerability. Contribution of Working Group III to the Sixth Assessment Report of the Intergovernmental Panel on Climate Change.
Kampan, K., Tsusaka, T. W., and Anal, A. K. (2022). Adoption of Blockchain Technology for Enhanced Traceability of livestock-based products. Sustain. For. 14:13148. doi: 10.3390/su142013148
Kipling, R. P., Taft, H. E., Chadwick, D. R., Styles, D., and Moorby, J. (2019). Implementation solutions for greenhouse gas mitigation measures in livestock agriculture: a framework for coherent strategy. Environ. Sci. Pol. 101, 232–244. doi: 10.1016/j.envsci.2019.08.015
Kool, A., Marinussen, M., and Blonk, H. (2012). LCI data for the calculation tool feedprint for greenhouse gas emissions of feed production and utilization GHG emissions of N, P and K fertilizer production. Netherlands, Blonk Consultants.
Kossaibati, M. A., and Esslemont, R. J. (1997). The costs of production diseases in dairy herds in England. Vet. J. 154, 41–51. doi: 10.1016/s1090-0233(05)80007-3
Le Cozler, Y., Allain, C., Fisher, A., Caillot, A., Depuille, L., Xavier, C., et al. (2022). “Chapter 18: the use of 3D imaging technology in animal management, with a special emphasis on ruminant production” in Practical precision livestock farming (Wageningen Academic), 319–350.
Lorenz, H., Reinsch, T., Hess, S., and Taube, F. (2019). Is low-input dairy farming more climate friendly? A meta-analysis of the carbon footprints of different systems. J. Clean. Prod. 211, 161–170. doi: 10.1016/j.jclepro.2018.11.113
Marino, R., Petrera, F., Speroni, M., Rutigliano, T., Galli, A., and Abeni, F. (2021). Unraveling the relationship between Milk yield and quality at the test day with rumination time recorded by a PLF technology. Animals 11:1583. doi: 10.3390/ani11061583
Min, B.-R., Lee, S., Junh, H., Miller, D. N., and Chen, R. (2022). Enteric methane emissions and animal performance in dairy and beef cattle production: strategies, opportunities, and impact of reducing emissions. Animals 12:948. doi: 10.3390/ani12080948
Morrone, S., Dimauro, C., Gambella, F., and Cappai, M. G. (2022). Industry 4.0 and precision livestock farming (PLF): an up to date overview across animal productions. Sensors 22:4319. doi: 10.3390/s22124319
Neethirajan, S. (2017). Recent advances in wearable sensors for animal health management. Sens. Bio-Sens. Res. 12, 15–29. doi: 10.1016/j.sbsr.2016.11.004
Robcis, R., Ferchiou, A., Berrada, M., Ndaiye, Y., Herman, N., Lhermie, G., et al. (2023). Cost of lameness in dairy herds: an integrated bioeconomic modeling approach. Journal of Dairy Science 106, 2519–2534.
Rotz, C. A., Stour, R. C., Holly, M. A., and Kleinman, P. J. A. (2020). Regional environmental assessment of dairy farms. J. Dairy Sci. 103, 3275–3288. doi: 10.3168/jds.2019-17388
Russell, R. A., and Bewley, J. N. (2013). Characterization of Kentucky dairy producer decision-making behavior. J. Dairy Sci. 96, 4751–4758. doi: 10.3168/jds.2012-6538
SAC Consulting Ltd (2022). Dairying in the farm management handbook 2022/23, Alastair Beattie (editor), SAC consulting. Available from the Farm Advisory Service www.sruc.ac.uk/fmh
Scottish Government (2023). Open government action plan 2021 to 2025 - commitment 4: climate change, published online July 2023.
Shalloo, L., Cromie, A., and McHugh, N. (2014). Effect of fertility on the economics of pasture-based dairy systems. Animal 8, 222–231. doi: 10.1017/S1751731114000615
Slayi, M. (2023). Avoidance-related behavioral and blood-based physiological responses of Nguni and Boran cattle subjected to routine handling activities post relocation. Front. Vet. Sci. 10:1188505. doi: 10.3389/fvets.2023.1188505
Smith, P., Martino, D., Cai, Z., Gwary, D., Janzen, H., Kumar, P., et al. (2007). Policy and technological constraints to implementation of greenhouse gas mitigation options in agriculture. Agric. Ecosyst. Environ. 118, 6–28. doi: 10.1016/j.agee.2006.06.006
Smith, S. A., Popp, M. A., Keeton, D. R., West, C. P., Coffey, K. P., Nalley, L. L., et al. (2016). Economic and greenhouse gas emission response to pasture species composition, stocking rate, and weaning age by calving season, farm size, and pasture fertility. Agric. Resour. Econ. Rev. 45, 98–123. doi: 10.1017/age.2016.11
Stygar, A. H., Gomez, Y., Berteselli, G. V., Dalla Costa, E., Canali, E., Niemi, J. K., et al. (2021). A systematic review on commercially available and validated sensor Technologies for Welfare Assessment of dairy cattle. Front. Vet. Sci. 8:634338. doi: 10.3389/fvets.2021.634338
Tejeda, H. A., Chahine, M., Du, X., Lu, L., and Westerhold, A. (2020). Investigating factors affecting adoption of automated milking systems (AMS) in Idaho. Western Econ. For. 18, 24–39,
Toma, L., March, M., Stott, A. W., and Roberts, D. J. (2013). Environmental efficiency of alternative dairy systems: a productive efficiency approach. J. Dairy Sci. 96, 7014–7031. doi: 10.3168/jds.2013-6911
Tzanidakis, C., Simitzis, P., and Panagakis, P. (2023). Precision livestock farming (PLF) systems: improving sustainability and efficiency of animal production. Sustain. Cases Stud. Using Operat. Res. Manag. Sci. Methods 333, 285–337. doi: 10.1007/978-3-031-16620-4_15
Vellinga, T. V., Blonk, H., Marinussen, M., Van Zeist, W. J., De Boer, I. J. M., and Starmans, D. (2013). Methodology used in FeedPrint: a tool quantifying greenhouse gas emissions of feed production and utilization. Wageningen UR Livestock Research 674: https://edepot.wur.nl/254098, 285–337,
von Soosten, D., Meyer, U., Flachowsky, G., and Dänicke, S. (2020). Dairy cow health and greenhouse gas emissions intensity. Dairy 1, 20–29. doi: 10.3390/dairy1010003
Wathes, C. M., Kristensen, H. H., Aertsc, J. M., and Berkmans, D. (2008). Is precision livestock farming an engineer’s daydream or nightmare, an animal’s friend or foe, and a farmer’s panacea or pitfall? Comput. Electron. Agric. 64, 2–10. doi: 10.1016/j.compag.2008.05.005
Wilkinson, J. M., and Garnsworthy, P. C. (2017). Impact of diet and fertility on greenhouse gas emissions and nitrogen efficiency of milk production. Livestock 22, 140–144. doi: 10.12968/live.2017.22.3.140
Yan, T., Agnew, R. E., Gordon, F. J., and Porter, M. G. (2000). Prediction of methane energy output in dairy and beef cattle offered grass silage-based diets. Livest. Prod. Sci. 64, 253–263. doi: 10.1016/S0301-6226(99)00145-1
Keywords: carbon footprint, dairy, emissions, precision livestock farming, modeling
Citation: Ferguson HJ, Bowen JM, McNicol LC, Bell J, Duthie C-A and Dewhurst RJ (2024) The impacts of precision livestock farming tools on the greenhouse gas emissions of an average Scottish dairy farm. Front. Sustain. Food Syst. 8:1385672. doi: 10.3389/fsufs.2024.1385672
Received: 26 February 2024; Accepted: 03 May 2024;
Published: 22 May 2024.
Edited by:
Leonard Rusinamhodzi, International Institute of Tropical Agriculture (IITA), NigeriaReviewed by:
Ilker Kilic, Bursa Uludağ University, TürkiyeCopyright © 2024 Ferguson, Bowen, McNicol, Bell, Duthie and Dewhurst. This is an open-access article distributed under the terms of the Creative Commons Attribution License (CC BY). The use, distribution or reproduction in other forums is permitted, provided the original author(s) and the copyright owner(s) are credited and that the original publication in this journal is cited, in accordance with accepted academic practice. No use, distribution or reproduction is permitted which does not comply with these terms.
*Correspondence: H. J. Ferguson, aG9sbHkuZmVyZ3Vzb25Ac3J1Yy5hYy51aw==
Disclaimer: All claims expressed in this article are solely those of the authors and do not necessarily represent those of their affiliated organizations, or those of the publisher, the editors and the reviewers. Any product that may be evaluated in this article or claim that may be made by its manufacturer is not guaranteed or endorsed by the publisher.
Research integrity at Frontiers
Learn more about the work of our research integrity team to safeguard the quality of each article we publish.