- 1Department of Agricultural Finance and Banking, Sylhet Agricultural University, Sylhet, Bangladesh
- 2Department of Agricultural Finance and Banking, Bangladesh Agricultural University, Mymensingh, Bangladesh
- 3Department of Agricultural Statistics, Sylhet Agricultural University, Sylhet, Bangladesh
- 4Upazila Land Office (Golapganj), Sylhet, Bangladesh
Introduction: The unique characteristics of pineapples as a perennial plant, which guarantee their quick proliferation and adoption in both the tropics and subtropics, readily justify their economic significance. Although pineapple is a popular tropical fruit among Bangladeshi citizens, they continue to produce fewer pineapples than other international producers with limited export offerings. Hence, the study aimed to estimate the technological efficiency, prospects, and policies of pineapple growers in the northeastern district of Bangladesh.
Methods: One hundred respondent growers were surveyed directly to gather cross-sectional data using a multistage sampling technique. The technical efficiency scores of individual farms were calculated using the stochastic frontier model with the technical inefficiency model for identifying factors responsible for inefficiency.
Results: The technical efficiency scores range from about two-thirds to the absolute efficiency level, with a mean technical efficiency above the ninety percent level. The technical inefficiency effect model interpreted that farmers’ age and education had a significant positive impact, whereas credit, training, and family size had a significant negative impact on inefficiency.
Discussion: Findings indicated that sampled farmers may use inputs more efficiently and raise their yield by nearly one-twentieth. Therefore, the study suggests that the government should concentrate on strategies to attract young growers, as they are more capable of managing resources effectively and willing to accept technological breakthroughs. The study’s conclusions have significant policy ramifications specifically in the areas of finance, education and skills, and rural development that the Government should consider to increase farmer’s productivity and overcome various challenges while upholding national interests and ensuring the farming sector’s continued prosperity. To commercialize pineapple production and establish Bangladesh as a prominent production zone, more research and development are needed.
1 Introduction
Bangladesh’s agriculture industry is a vital economic pillar. Its GDP contribution immediately following liberation in 1971 was approximately 60 percent. We all know that it is the most significant industry in Bangladesh in terms of GDP contribution, employment opportunities, and support for people’s livelihoods. Notwithstanding its considerable potential for employment in the rural labor force, its percentage of the GDP has declined over the past 10 years, from 17 percent in 2010 to approximately 11.66 percent in 2020 (BBS, 2022; BER, 2023). Improved agricultural productivity and food and nutrition security have been made possible by the adoption of agriculture-friendly policies and strategies, despite the effects of decreasing arable land, rising population demands for food and nutrition, climate change, the Russia-Ukraine crisis, and the coronavirus epidemic. This sector overwhelmingly impacts primary macroeconomic objectives like employment generation, poverty alleviation, human resources development, and food security. To meet the future needs of the expanding population, the government is working tirelessly to adopt short-term, medium-term, and long-term action plans. One such plan is to increase the export of high-value crops like pineapple (Figure 1) to build sustainable, safe, and profitable agricultural systems that ensure food security (Shakil, 2023).
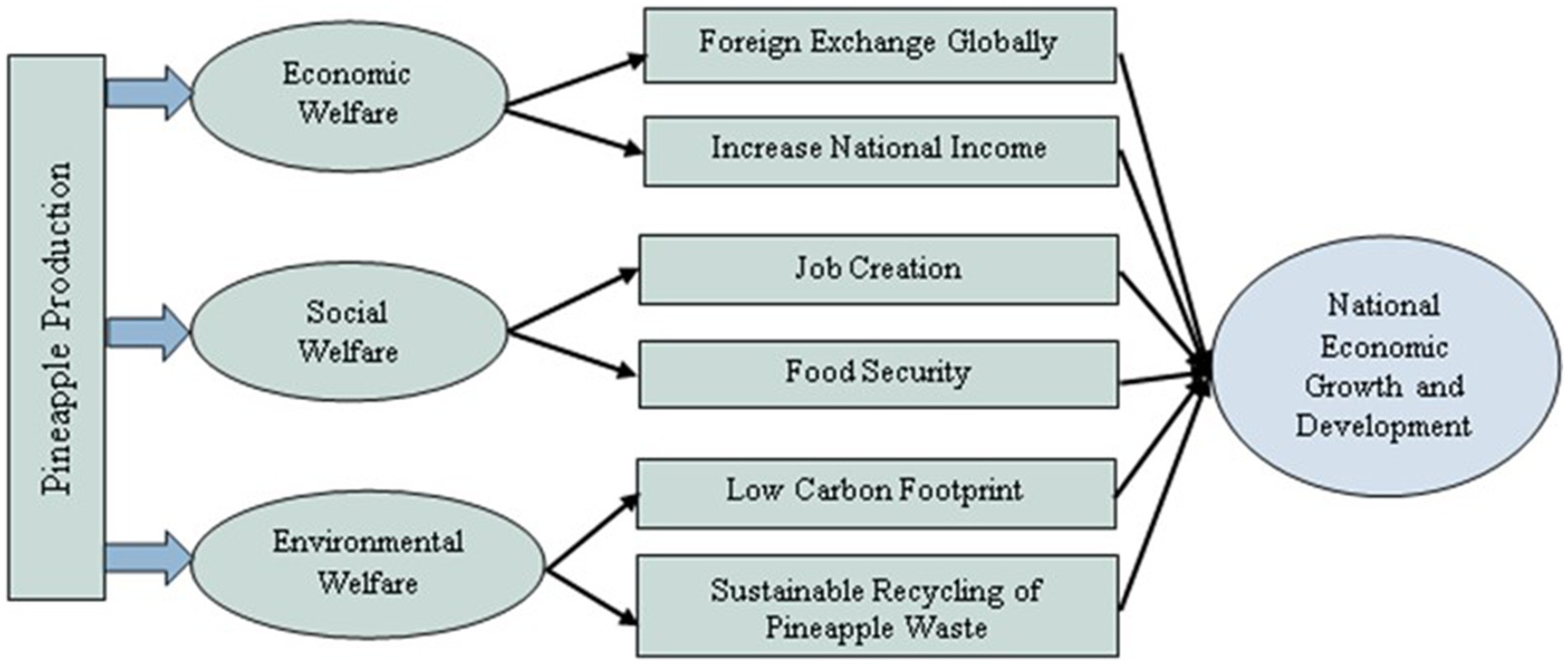
Figure 1. Safe and sustainable impacts of pineapple farming. This chart shows the favorable correlation between an economy’s overall development and pineapple production. Similar to many other fruits, pineapple production is important for the socioeconomic advancement of the populace, particularly for Bangladesh’s marginalized growers in the northeast.
Because of the success of commercial farming, fruit production in the nation has quietly undergone a revolution during the last 20 years. Bangladesh has maintained an average annual rise in fruit production of 11.5 percent over the previous 18 years, placing it among the top 10 tropical fruit-producing nations in the world (FAO, 2018; BBS, 2019). Pineapple is one of Bangladesh’s most important commercial fruit crops. Among all the fruits produced in the country, pineapple ranks 3rd in total garden area under fruit cultivation after banana and mango (BBS, 2016). Because of its chemical composition, it is the primary raw material utilized by the confectionary industries to produce food additives and domestic fruit juices (Akhilomen et al., 2015). As a significant fruit crop, pineapple is widely farmed in several districts, including Tangail, Rangamati, Mymensingh, Gazipur, Chattogram, Khagrachari, Bandarban, Moulvibazar, Sylhet, and Dhaka (Hasan et al., 2011). These districts employ a large number of women and farmers. Bangladesh’s most crucial pineapple fruit-growing areas are the Madhupur upazila of Tangail and the Sreemangal upazila of Moulvibazar. Based on an estimated 14,164 hectares of land, the nation produced 208,000 tons of fruit in 2020–21. The number of pineapples that grew in 2021–2022 increased from 0.469 million tons to 0.538 million tons, according to Department of Agricultural Extension (DAE) data. Due to the more extended winter season in 2021–22, the honey queen kind of pineapple, also known locally as Joldubi, which is grown throughout Bangladesh, particularly in the central part and the north-eastern division, has produced a higher yield than it did last year. While the yield of pineapples in 2022 was more significant than in previous years, the cost of production is also higher because more labor, water, and fertilizers are needed. Policymakers consider the pineapple industry among the top priorities for growing exports (Shakil, 2023). The agriculture department is addressing the issue of using chemicals to ripen and increase the size of pineapples.
In addition to utilizing chemical fertilizers, some avaricious growers and dealers treat immature pineapples with excessive growth hormones to get them onto the market sooner. Producers have not yet been able to lower their production risk due to the cultural practices used for pineapple growth up to that point. Nevertheless, pineapple output in Bangladesh is still far too low to meet export demands in the European Union (EU) and the subregion, despite the country’s potential for growing this fruit. There are some reasons for this low pineapple production, including the scarcity of good suckers, the producers’ inability to grasp basic principles like traceability and the crucial quality standard for fruit meant for export, and the high cost of specific inputs that have a detrimental effect on fruit quality and preservation. The fact that animals and birds consume naturally ripened crops while still in the fields presents another challenge for growers. However, animals and birds will not consume the fruits if ripening hormones are sprayed on them (Datta et al., 2023).
Furthermore, it is unfortunate that Moulvibazar lacks standards for the amounts and mixes of mineral fertilizers appropriate for pineapple crops, which would better protect the environment’s components—particularly the soils and water resources vulnerable to pollution or degradation. These standards would also be economically beneficial. Because of this, Sreemangal pineapple growers use different doses and types of mineral fertilizers according to their needs. This impacts the production costs and the quality of pineapples (Shakil, 2023) because confident growers overuse chemical fertilizers. Given that producers nowadays do not always have access to precise inputs and quality releases, knowing the efficiency level of producers allows for the definition of strategies to drive interventions (Fassinou et al., 2012). Investigating production potentials is necessary to match demand and supply with worldwide standards for pineapple, which requires improving productivity and quality. To prevent resource waste and, more importantly, to focus guidance on increasing the output of pineapple growers, the technical efficiency of pineapple producers must be evaluated.
As we all know, efficiency is a comparative indicator of a company’s ability to use inputs in a production process relative to other companies in the same industry. It can encompass all pertinent production parameters and represents actual farm performance. Understanding how sound resources are being used and what opportunities there are to increase productivity with the resources and technology already in place is crucial (Ahluwalia, 1996). Even though inputs are necessary for productive production, farmers in poor nations like Bangladesh confront significant obstacles when obtaining inputs. To help better comprehend the new demands of Bangladesh’s agricultural sector, it appears imperative to ascertain the study with two specific research questions: firstly, whether the farmers are producing in a technically efficient manner. Secondly, are there any factors or lack thereof for the technical inefficiency of pineapple growers?
However, several studies in the world and Bangladesh (Bakh and Islam, 2005; Begum et al., 2010; Islam and Sumelius, 2011; Alam et al., 2012; Polas, 2013; Sarker and Alam, 2016; Razzaq et al., 2019; Phrommarat and Oonkasem, 2021) have investigated the overall efficiency along with technical efficiency in different fields. Akter et al. (2020) explored the technical efficiency of pineapple in the Tangail district. Nevertheless, the currently available literature ignores the technological efficiency level of pineapple growers in Bangladesh’s northeastern region. Studying the effectiveness of livelihood-focused interventions in that area is extremely important because of their unique ecological characteristics.
Furthermore, rice used to be the main focus of Bangladesh’s previous food policies, with the goal being rice self-sufficiency. While rice continues to play a significant role in society, it is now necessary to create regulations and other tools that encourage crop diversification to supply a variety of food products and encourage the consumption of balanced, nutrient-rich diets.
As one of the fastest-growing agricultural subsectors, pineapples (Figure 1) are high-value commodities that need to be prioritized to ensure proper use of the nutrients ingested (FAO, 2018).
Therefore, multi-sectoral interlinked interventions focused on enhancing nutritional outcomes can be designed with the assistance of a new policy that is holistic and encourages the use of a “nutrition lens” to evaluate and prioritize various choices. Furthermore, a recently implemented policy that crosses the purview of more than a dozen ministries can offer an institutional framework under a single roof for ensuring sustainable production-consumption systems (SDG 12 of United Nations global goals) in the agricultural sector. The National Food and Nutrition Security Policy (NFNSP) of Bangladesh will benefit from the study’s results, which will also likely serve as a reference for creating and carrying out the country’s eighth and ninth five-year plans (MoF, 2022).
1.1 Background for identifying clear research gap
Despite extensive research on the Technical Efficiency of Pineapple, there is a research gap in classic literature and specific context. The contextual gap emerged when we could not explore any existing studies directly related to the topic-the understanding of the technological effectiveness of Pineapple in rural farm populations within the Sylhet Division. Hence, there was an opportunity for us to investigate a completely new geographic area with its high-value fruit crop.
The bulk of the studies were completed worldwide on the efficiency of different products including pineapple which helped us generate ideas regarding study design and analysis. For instance, Oladapo et al. (2007) examined the market margin and spatial pricing efficiency of pineapple in Nigeria. In addition, the technical efficiency and its determinants in Garden egg (Solanum Spp.) production were determined by Okon et al. (2010) in Uyo Metropolis, Akwa Ibom State, while Trujillo and Iglesias (2013) and Ghimire et al. (2023) employed the stochastic frontier approach for measuring the small pineapple farmers and lentil producers’ technical efficiency in Colombia and Nepal, respectively.
However, no study has been documented on the technical efficiency of pineapple production in the northeastern part of Bangladesh to the best of the author’s knowledge. Although numerous published works were identified on the various contexts of pineapple cultivation in different areas of Bangladesh (Table 1), our attempt could help mitigate this type of contextual gap in the advanced research arena.
To improve the economic, social, and consumer welfare of rural farmers, policies must be developed to increase their output through fruit farming. Hence, the aims of this study are twofold to mitigate the current research gaps: (1) To estimate the technical efficiency of pineapple production using the Stochastic Frontier Model (SFM) and (2) To identify the factors influencing the technical efficiency level of pineapple producers. The findings will add to the body of knowledge in light of Bangladesh’s shifting agricultural landscape, make it easier for the relevant authorities to pursue the right policies, and remove obstacles to the successful implementation of sustainable food systems through the productive production of pineapples in rural areas.
2 Materials and methods
2.1 Study area: north-eastern district of Bangladesh
The northeastern part of Bangladesh (Sylhet basin; Figure 2) contains the most commercially and ecologically significant hillocks with evergreen pineapple orchards, which are referred to as pineapple villages (Jahid, 2023). Sreemangal is situated southwest of the Moulvibazar district at 24.3083°N 91.7333°E (Banglapedia, 2023). Agriculture is the communities’ primary industry as this sector occupies 30.90 percent compared to other sources of income in the study area. The Sreemangal lies 17 m above sea level where the climate was warm and temperate. In winter there is much more rainfall than in summer. The average annual temperature is 24.7°C/76.5°F in Sreemangal. The annual rainfall is 2,420 mm. Precisely, Sreemangal is notable for its extensive tea and pineapple gardens as well as its continuous rain. The presence of lush trees has enriched its dynamic vegetation. The terraced tea gardens, plantations, pineapple gardens, and evergreen hills of Sreemangal are popular tourist destinations (Seema et al., 2023).
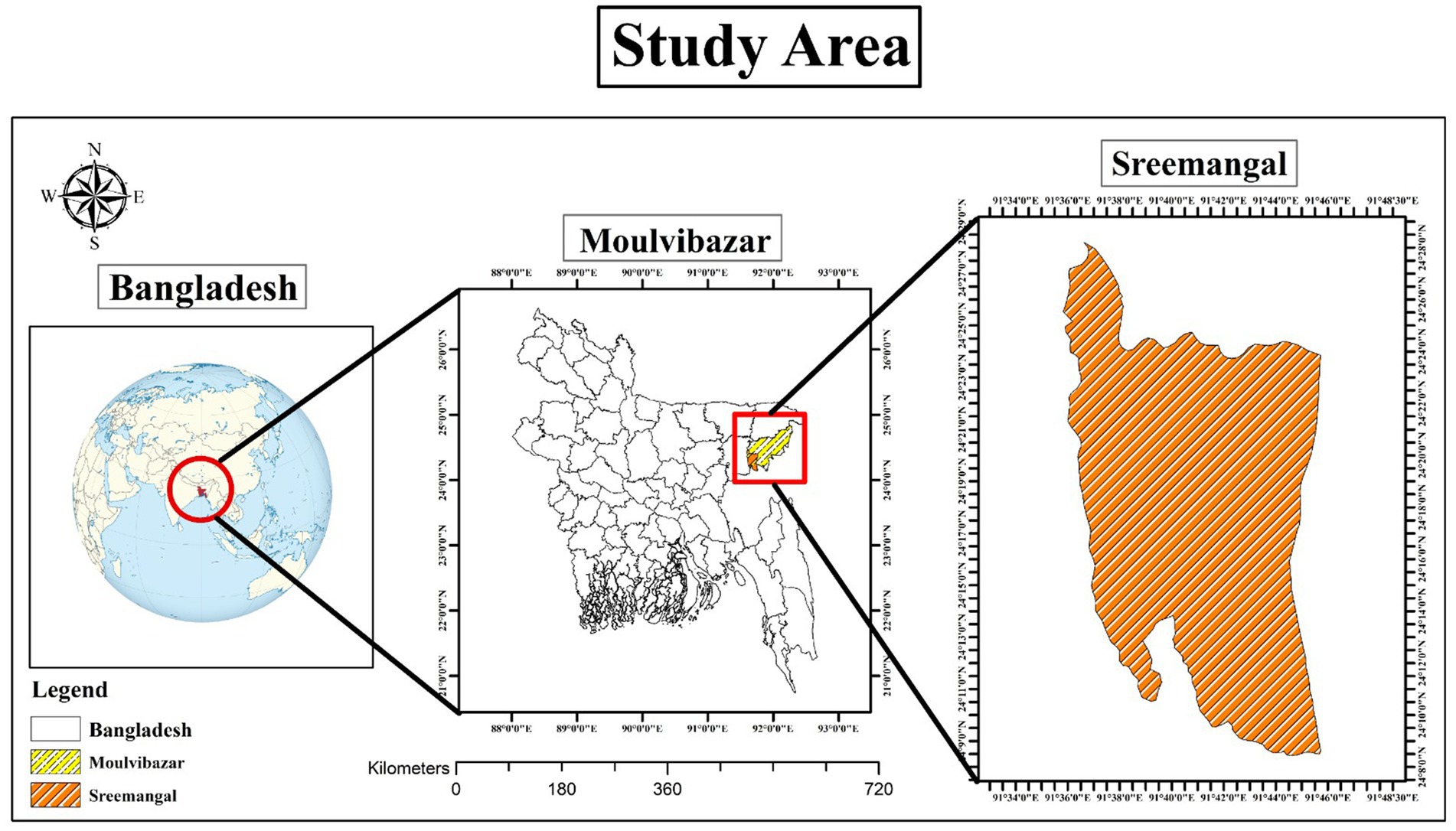
Figure 2. Location of the study area. The research area, also known as “Pineapple Village,” is depicted on the map along with its physical borders and pertinent information. Because of the favorable biological circumstances in these areas, the fruit can be profitably grown on underutilized land, hillocks, and yards.
Since the Pakistani era, this region has been well-known for growing a variety of fruits, including pineapple, in the villages of Bishamoni, Bhunabir, Ashidron, Radhanagar, Ramnagar, Balishira, Noorjahan, Doluchhara, Satgaon, and Mohajerabad (Deshwara, 2015). The district’s 1,210 hectares of land are used for pineapple cultivation. On the other hand, this season in Sreemangal, about 500 hectares of land have been planted with three different varieties of pineapples (The Daily Tribunal, 2023). Nonetheless, this high-value cash crop can be grown in the northeastern region of Bangladesh on laterite soils on hillslopes and sandy, loamy soils high in humus. According to NHB (2015), it favors soils with a pH range of 5.0–6.0. Since Sreemangal’s soil, environment, and warm, humid weather are ideal for growing pineapple, many pineapples are grown here in the upper hills. This region can help Bangladesh’s millions build a sustainable socioeconomic existence by providing jobs, wholesome food, fuel, and fodder. The study is focused on the Sreemangal Upazila (Figure 2) due to its natural richness and biodiversity.
2.2 Variables of data collection, sampling technique, and sample size
Data on socioeconomic characteristics, pineapple production activities (such as input and output costs), and other farm-specific variables were collected from the farmers and analyzed using the stochastic frontier model, which was developed by Aigner et al. (1977) and adopted by Tadesse and Krishnamoorthy (1997) and Taylor and Shonkwiler (1986) were used to estimate the technical efficiency of pineapple farmers (Balogun et al., 2018). In analyzing the efficiency or inefficiency of farmers, the greatest output that can be produced from a particular set of inputs rather than the average of the actual relationship between farmers’ inputs and output is of relevance. According to the statement, given the state of technology, not all producers can use the minimal inputs necessary to generate the desired output. Producers do not continuously optimize their production functions, according to theory. Technically efficient producers are those who operate above the frontier production curve, and technically inefficient producers are those who operate below the frontier production curve.
Various works on technical efficiency in developing countries were reviewed to choose appropriate variables that best suit our research objectives before preparing the questionnaire. Among others, age (in years), Household head gender (male = 0, female = 1), Educational status (years of schooling), Occupation (1 = Farming, 2 = Business, 3 = Service, 4 = Farming + Business, 5 = Farming + Service, 6 = Others), Farming experience (in years), Family size (in Numbers), Farm category (Marginal = 1, Small =2, Medium = 3 and Large = 4), Access to credit (if taken credit = 1, otherwise = 0), Training (if received any training = 1, otherwise = 0) and Advisory services (if received any advisory services = 1, otherwise = 0) were included for technical efficiency analysis.
2.2.1 Research design
A multistage sampling procedure was used to sample 100 farmers. The first stage was the purposive selection of the Sreemangal Upazila from the other six pineapple-producing upazilas of Moulvibazar District because of its production potentiality (DS, 2011). The second stage was the random selection of 4 unions (Ashidron, Satgaon, Sindurkhan, and Sreemangal) of the upazila from 9 union parishads based on their bumper contribution to pineapple production using the lottery method. In contrast, the last stage was the random selection of a total of 100 farmers from the respective unions through the random number table method.
2.2.2 Method of data collection and sample size determination
This study collected raw data from 100 respondents employing a semi-structured questionnaire during on-farm production. The data were collected from sampled pineapple farmers about their production technology and output for 2019–20. The final questionnaire contained different study aspects, including general information, land-holding information, socio-economic aspects, problems, and probable solutions. Pre-testing was done before the final survey, which was conducted from February to April 2020. After that, a simple random sampling procedure was adopted to select the desired sample size and was calculated by using the method suggested by Cochran (1977) to calculate a representative sample for proportions as:
When,
Here, is the required sample size.
n0 is Cochran’s sample size computed for the ideal sample size.
is the size of the population.
is the selected critical value of the desired confidence level 95 percent = 1.962 = 3.8416.
is the estimated proportion of an attribute that is present in the population = 0.5, = −1 = 0.5.
is the error term 5% as we considered the confidence interval 95 percent (d = 0.05).
Therefore, = 384.14 and = 200.
Thus, based on the Cochran formula the sample size required was 200. For some limitations, the total sample size for the study was maintained at 100.
2.3 Analytical framework
The previous literature illustrates that the evaluation of technical efficiency employs two methods: a parametric approach and a non-parametric approach. The parametric approach enables econometric techniques, but the nonparametric approach is based entirely on mathematical techniques of Data Envelopment Analysis (DEA) used by Pakravan-Charvadeh and Flora (2022) and Pakravan-Charvadeh et al. (2022) in their research work identify nutrition efficiency. Previous studies have discussed and explained the advantages and shortcomings of both approaches (Battese and Coelli, 1992; Bravo-ureta and Evenson, 1994; Battese and Coelli, 1995). The econometric technique is stochastic and splits the impact of random error from the inefficiency effect. The non-parametric technique combines the errors and is known as combination inefficiency. The econometric technique is parametric and controls the impact of misspecification of practical form through inefficiency. The non-parametric technique is not so liable for this description error. However, the literature reveals that the econometric technique is commonly used to assess the technical efficiency of firms (Tchale and Sauer, 2015; Ali et al., 2019; Ndubueze-Ogarak et al., 2021). Accordingly, the econometric techniques were used in our study for Stochastic Frontier Analysis (SFA).
2.3.1 Theoretical foundations
Formalizing the yields’ response to various inputs is the primary driving force behind measuring manufacturing processes’ technical efficiency. The primary causes of the observed variances in this responsiveness are changes in the technology employed by the enterprises, variations in the efficiency levels of the production processes, and variations in the production setting. As a result, technological efficiency determines economic efficiency (Adegbite and Adeoye, 2015).
Farrell (1957) methodology was a precursor to technical efficiency modeling since it presumes the presence of an efficient production-possibility frontier (PPF). According to this concept, the PPF indicates the highest production that can be obtained from a specific combination of productive elements. The gap between each firm’s production level and the PPF’s peak level is used to calculate technical inefficiency. Consequently, it is possible to compute the technical efficiency as a percentage of the sample’s highest productive production unit.
Two different methods could be used to estimate this production function. First, there are the non-parametric approaches, which stand out for their adaptability. They are less constrictive when it comes to the parameters that are applied to the reference technology and when modeling manufacturing processes that involve many products (Trujillo and Iglesias, 2013).
Second, one can econometrically estimate a production function using parametric methods, allowing one to draw statistical conclusions from the estimation’s findings.
To apply these techniques, the dataset must be in a functional form. Using the latter approach, Aigner et al. (1977) and Meeusen and Broeck (1977) develop a stochastic production function to separate mistakes resulting from model misspecification from those that productive inefficiencies can explain. Determining the precise distribution of the error component and the production function’s functional shape is necessary for this differentiation.
The primary empirical source on the factors influencing technical efficiency in agriculture is Battese and Coelli (1995). The main hypothesis put out by these writers is the combined estimation of a model that incorporates the factors influencing agricultural production inefficiency and the efficient frontier of agricultural production.
As Coelli and Battese (1996) demonstrated Indian farmers tend to be more productive when they are older, have larger farms, and have more education. Similarly, Tian and Wan (2000) discovered that the efficiency of rice production in China is positively impacted by education, farm size, and the implementation of different cropping techniques. Similarly, Villano and Fleming (2006) found that factors including age, education level, the percentage of adults in the household, and the amount of money generated by non-farm activities influence technical efficiency in a sample of farmers in Central Luzon, the Philippines.
On the other hand, Amaza and Olayemi (2002) suggested that greater levels of technical efficiency are attained in Nigeria if education, technical support, and crop diversification all rise.
Within the same continent, Essilfie et al. (2011) measured maize growers in Ghana’s technical efficiency at the farm level; their findings indicated that variations in age, sex, years of education, family size, and farmers’ off-farm income impact technical efficiency. In Latin America, limited research was conducted on the estimation of the level of technical efficiency in agriculture using the stochastic frontier approach; however, it is noteworthy to mention the study of Benoit-Cattin and Mendez (1996) focused on a group of small coffee farmers in Guatemala for revealing their efficiency level at using the existing technology; these authors concluded that both technical assistance & credit support sustain Guatemalan coffee plantations. In Brazil, Conceicao and Araujo (2000) found the TE of a sample of commercial farmers and discovered that experience plays a significant role in explaining these farmers’ technical efficiency.
Furthermore, Richetti and Reis (2003) study looks at the economic effectiveness of the productive resources used in the State of Mato Grosso do Sul’s soybean farming. They demonstrated how the methods of transferring technology to growers of this grain are connected to technical inefficiency. According to Santos et al. (2006), there is a positive correlation between technological efficiency and the following factors in Chile: the size of the property, the distance from the main road, the age of the head of the family, and membership in a technology transfer group. Moreira et al. (2006) worked on a highly unbalanced panel dataset for a sample of small dairy farms in Southern Chile in a related investigation carried out in the same nation.
Both Taylor and Shonkwiler (1986) and Tadesse and Krishnamoorthy (1997) used the stochastic frontier model created by Aigner et al. (1977). When analyzing a farmer’s efficiency or inefficiency, what matters is not the mean of the actual relationship between the farmer’s inputs and output, but rather the highest output that can be produced with a specific set of inputs. The statement suggests that, given the state of technology, not all producers can use the minimal number of inputs needed to generate the desired quantity of output. Producers do not always optimize their production functions, according to theory. Technically efficient producers are those who operate on the production frontier; technically inefficient producers are those who operate below the frontier production curve.
Nevertheless, studies on the technical efficiency of production that employ the parametric method are scarce regarding pineapple production. Chen et al. (2001) conducted a remarkable study wherein the technical efficiency of 83 pineapple farms in China was measured. The study concluded that manpower is the most crucial aspect of production. In a similar vein, Adinya et al. (2010) established the inefficiency of Nigerian pineapple production. Their findings suggested that achieving higher production efficiency is significantly impacted by the farmers’ educational attainment.
Eventually, two types of functions namely: Cobb–Douglas and Translog dominate the technical efficiency literature. In the case of a lower sample size, the Translog specification might not be representative. The stochastic frontier production model is employed to determine respondents’ technical efficiency.
The Cobb–Douglas (CD) is the appropriate form of the frontier production function. The production technology is assumed to be characterized by the Cobb–Douglas production function since it has the advantage over other forms of production functions like the Linear and Semi-log production functions in that a logarithmic transformation provides a model that is linear in the log of input and hence, easily used for econometric studies (Coelli, 1995). This production function gave the best fit to data compared to the linear, exponential, and semi-log functional forms (Akhilomen et al., 2015).
In addition to the above, the CD functional form is mainly preferred because its coefficients directly represent the elasticity of production. It provides an adequate representation of the production process as we are interested in an efficiency measurement and not an analysis of the production structure (Taylor and Shonkwiler, 1986). Further, the CD functional form has been widely used in farm efficiency analyses. It is an adequate representation of the data (Abedullah and Bakhsh, 2006).
2.3.2 Stochastic frontier analysis
SFA, which is also known as a composed error model, was developed initially by Aigner et al. (1977) and Meeusen and Broeck (1977). Supposing an appropriate production equation, we described the stochastic production frontier Eq. 1 below:
Where Yi, yield produced by ith pineapple grower; Xi, inputs for the pineapple by ith growers; β, parameters of study; εi, collected unsystematic errors; εi = νi – ui, νi is symmetric (−∞ < νi < ∞) and shows those random errors, such as climate change or other natural disasters, which are out of the farmer’s control in the Eq. 2.
It is expected that νi is identically and independently distributed as N (0, σ2v; Gujarati, 2003). Farm-specific technical inefficiency is denoted by ui. On the other hand, it shows the gap of output (Yi) and its maximum possible output assumed by the SFA [f(Xi, β) + νi] (Aigner et al., 1977). ui arises from N (0, σ2v) and is half normally distributed below 0 (Kumbhakar and Lovell, 2000). The terms νi and ui are always independent of the input factors Xi.
2.3.3 Stochastic frontier model specification
The SFA model was used to estimate the technical efficiency of pineapple production. This technique specifies the effect of technical inefficiency that cannot be controlled by pineapple growers. The Cobb–Douglas Production function is suitable for estimating technical efficiency in our study, due to its advantages of easing interpretation and estimation. In addition, the elastic functional form solves the difficulty of multi-collinearity. We can express the SFA Eq. 3 for the analysis as below:
Where Yi, yield of pineapple in kilograms per ha; ith, Number of farmers up to 100; jth, Number of variables up to 8; X1, Area under pineapple cultivation (ha); X2, Quantity of seedling (piece/ha); X3, Lime (kg/ha); X4, Urea (kg/ha); X5, Triple Superphosphate (TSP) (kg/ha), X6, Muriate of Potash (MoP; kg/ha); X7, Hormone (ml/ha); X8, Human labor (man-days/ha); εi, Error (composed error term); ln, natural logarithm; β0, Intercept of the model; βj, equation parameters.
2.3.4 Estimation of the stochastic frontier model
The Maximum Likelihood Estimation (MLE) technique was employed to estimate the SFA (Greene, 2000). The basic idea of the maximum likelihood principle is to choose the parameter estimates (β, σ2ε) to maximize the probability of obtaining the data:
Where σ2v and σ2u are the variances in the equation for v and u, respectively; further, σ2ε = σ2v + σ2u, and γ = σu/σv.
The MLEs of β, γ, and σ2ε at which the value of the likelihood function is the maximum were obtained by setting the first-order partial derivatives for β, γ, and σ2ε as equal to zero and solving these non-linear equations simultaneously in the Eqs. 4, 5. It can be estimated by using a non-linear optimization algorithm to find the optimal values of the parameters.
2.3.5 Equation of technical inefficiency estimation
In the model specification of technical efficiency estimation, it is expected that random vi is normally distributed as N (0, σ2v), whereas ui is half normally distributed as N (0, σ2u).
Where Ui denotes the specific technical inefficiency of pineapple yield;
Z1 = Age of the pineapple farmer (in years).
Z2 = Education of the pineapple farmer (in years of schooling).
Z3 = Dummy variable for credit taken from any source, e.g., Banks, NGOs (Non-governmental Organizations) only for cultivating pineapple (1 for yes and 0, otherwise).
Z4 = Dummy variable for training on pineapple farming participated by the pineapple farmer (1 for yes and 0, otherwise).
Z5 = Family size in number.
δj = Parameters of the respective technical inefficiency variable to be estimated.
(j = l, 2,............... 5)
Wi = Random error term that is defined by the truncation of the normal distribution (With zero mean and variance, σw2).
2.3.6 Estimation of technical efficiency and technical inefficiency of individual pineapple growers
The following formula Eq. 7 is applied to estimate the Technical Efficiency (TE) of pineapple growers:
Where Yi, observed yield of ith pineapple grower; Yi*, frontiers yield of ith pineapple grower that is obtained; TEi, technical inefficiency of ith pineapple grower in the range of 0 to 1.
To obtain the result of Technical Inefficiency (TI) of individual pineapple growers, the Eq. 8 below was employed
Where TIi = 1- (Yi/Yi*), TIi, technical inefficiency of ith pineapple growers in the ranges of 0 to 1 Eq. 8.
2.3.7 Hypothesis testing
It would be reasonable to fit an inefficiency effect model like (Eq. 6), if the inefficiency effects are significant, stochastic, and have a particular distribution specification.
Hence, according to Battese and Coelli (1995), we ought to test the following null hypotheses:
The generalized likelihood ratio test was to be used to examine the null hypotheses. To get the log-likelihood values under the above three null hypotheses, i.e., from Eqs. (9–11), three different models were fitted. The model under the null hypothesis Eq. (9) is the traditional mean response function where output or cost is regressed up on the respective predictor variables given in stochastic production or cost frontier assuming zero inefficiencies. That is, only statistical noise makes up the random component; the inefficiency effect model was discarded. The degrees of freedom under this null hypothesis was 7, since γ = 0, implying σu2 = 0 and δj’s (j = 0,1,2, …, 5) are equal to zero. Similarly, the model under the null hypothesis Eq. (10) is also a conventional mean response function where the farm-specific variables such as age, education, credit, training on farming, and family size were also included in the model as independent variables with the input variables. In this case, only the inefficiency component (i.e., ui) is dropped from the original model which means that parameters γ, σu2 and δ0 are equal to zero. Hence, the degree of freedom under this null hypothesis was 3. Again, the model under the null hypothesis Eq. (11) is the original stochastic frontier model as it is, but the inefficiency effect model will be spilled. That is, dropping δj’s parameters (where, j = 1, 2, …, 5), the values of γ, σu2 and δ0 were to be calculated. Hence, the degrees of freedom under this null hypothesis are equal to the number of farm-specific variables dropped (i.e., 5).
Now the value of the log-likelihood function calculated at the model under H0 needed to be close to the value calculated at the original model for the null hypothesis to be accepted. A measure of this familiarity was the likelihood ratio statistic under the above null hypothesis is
Where λ follows a chi-square distribution with degrees of freedom equal to the number of parameters assumed to be zero in the null hypothesis (H0) provided that H0 is correct and L(Ho) is the log-likelihood value under H0 and L(H1) is the log-likelihood value under H1 in the Eq. (12). Additionally, the renowned Student’s t-test was used to perform individual significance tests on the parameters in both the stochastic frontier model and the inefficiency effect model.
3 Results and discussion
3.1 Stochastic production frontier estimates
Table 2 displays the findings of maximum likelihood estimates for the pineapple technical inefficiency effect model and stochastic frontier production function. We discovered that area, lime and labor input would be sequentially correct based on their coefficients values all had statistically significant negative coefficients, meaning a negative impact on technical efficiency, with the values −0.011, −0.019, and −0.088, respectively. Small farms motivated family work and large farms’ tardiness in timely completing farm operations during crucial times may be responsible for the negative correlation between area and production. This conclusion coincides with those of Samarpitha et al. (2016) where the area under rice crops negatively impacted the technical efficiency (−0.0114), suggesting that small and marginal farms were technically more efficient than their bigger counterparts. So, the integration of rural industries is a crying need to improve agricultural productivity (Ye et al., 2023). On the other hand, the case of labor input may be linked to ineffective labor management in the study area and a declining rate of return on labor. The negative coefficients of labor input indicate that every single unit increase in the amount of labor per hectare leads to the rise of farmers’ inefficiency level by 0.088 units. This might be due to the lack of workers’ specialization in pineapple farming with less labor productivity nature in the study area. To ensure the positive impact of labor input employed for certainly we ought to give more focus on labor productivity rather than the labor supplies.
Ghimire et al. (2023) also found the coefficient of labor was 0.066 and was negatively significant denoting possible negative change by 6.6% in aggregate output of lentils as a result of unit man-days increment in labor use. These unexpected outcomes would be overcome with suggestions provided by Hasan et al. (2022) and Alam et al. (2019). The acreage of pineapple production explained the levels of technical efficiency to a significant degree as reported by Trujillo and Iglesias (2013).
The primary input in the production of pineapples was seedlings (sucker), for which the elasticity was determined to be 0.998 at a 1 percent significant level. This suggests that a 1 percent increase in seedling pieces per hectare is positively connected to a 0.998 percent improvement in farm efficiency. This positive relationship with output conforms to a priori expectation that is supported by Ghimire et al. (2023) where they also observed the coefficient of seed input was positively significant indicating unit increase in seed quantity will lead to an increase in lentil production by 37.6 percent. According to Balogun et al. (2018), as a management strategy for free movement and simple weeding, poor spacing of pineapple suckers after planting may have contributed to the positive coefficient of suckers’ substantial relationship with output. Leaders in the agriculture sector risk severe repercussions if they willfully ignore seed quality, such as increased produce prices, food insecurity, and a collapse in farm revenues. Considering the significant risks involved, it makes sense to spend money on premium seeds. Otherwise, this may be the reason that most of the farmers will use local seeds that may come up with poor germination and plant vigor (Ghimire et al., 2023). Additionally, we should get ready for financial instability to cascade down the supply chain from the farm to the consumer. The implication is that this will shorten production times while improving the quality, yield, and consistency of the finished crop.
3.2 Determinants of technical inefficiency among pineapple farmers
The lower part of the table enlisted the parameters of the technical inefficiency model. A negative value of parameters of the technical inefficiency variable presages a negative impact on technical inefficiency and vice-versa. Thus, the sign of the ‘δ’ parameters in the inefficiency effect model was expected to be negative which will impact the technical efficiency positively. The sigma square (σ2) is 0.025 of the estimated models was statistically significant at a 1 percent level of probability. This indicated a good fit of the distributional form assumed for the composite error term. The gamma (γ), which measures the dominance of the inefficiency effect over random error, with a value of 0.984 at a 1 percent level of significance implies that 0.984 percent of the variation in the output was attributed to technical inefficiency. The result shows that three variables credit, training, and family size had a significant impact on technical inefficiency with a negative coefficient. In the case of credit, the coefficient −0.066 at a 10 percent level of significance describes that technical inefficiency decreases with the increase of credit availability and utilization in pineapple production. This finding was consistent with the work of Balogun et al. (2018), Haq (2013) and Amoah et al. (2014), which showed a positive association between credit and input use and farm productivity. Again, at a 1 percent statistical level, farmers who received training conversely impact the technical inefficiency with the parameter coefficient of −1.034. It describes that farmer who had taken training tend to be less inefficient than their counterparts without having training. Trained farmers could implement improved production technology and had contact with the extension agents that led to efficient production for them. The result agrees with Balogun et al. (2018) and Akhilomen et al. (2015). On the contrary, Mussa (2011) used family size in the inefficiency effect model and found a positive impact on technical efficiency that coincides with our result. The reason may be that more family members ensure the availability of more family labor and can carry out important agricultural practices timely thus improving efficiency. It follows that a farmer who interacts with extension agents more often will be able to recognize and implement new farming methods more successfully than a farmer who interacts with them less. Since efficiency and a nation’s economic development are directly correlated, over time, economic growth is increased by more efficient businesses, higher input productivity, and increased economic activity. Every gain in efficiency usually results in a decrease in the cost of manufacturing (Naseer et al., 2020). Consumer prices for goods and services might go down when production costs are low. Technical efficiency is therefore necessary for long-term economic growth.
3.3 Farm efficiency level
The frequency distribution of the technical efficiency estimates for pineapple production is shown in both Table 3 and Figure 3. It is observed that technical efficiency varies from 61.57 to 99.33 percent for pineapple growers. The mean technical efficiency of pineapple farming is 94.37 percent in the study area. It indicates that on average a pineapple farmer in the study area still had the capability of increasing technical efficiency by 5.63 percent, using the available resources and technology to achieve the frontier output level. The frequency distribution of the technical efficiency implies that 85 percent of farmers had TE above 0.9, and only 4 percent is less than 0.71 (Figure 3). More precisely, in the study area, maximum farmers were found to operate at an 85 percent efficiency level. The result shows consistency with persistent heritage in the study area related to good agricultural practices, in particular for pineapple production. Eventually, a slight improvement in technical efficiency will lead to a good cost-efficient direction, which will help to enhance the farmer’s profit (Ghimire et al., 2023).
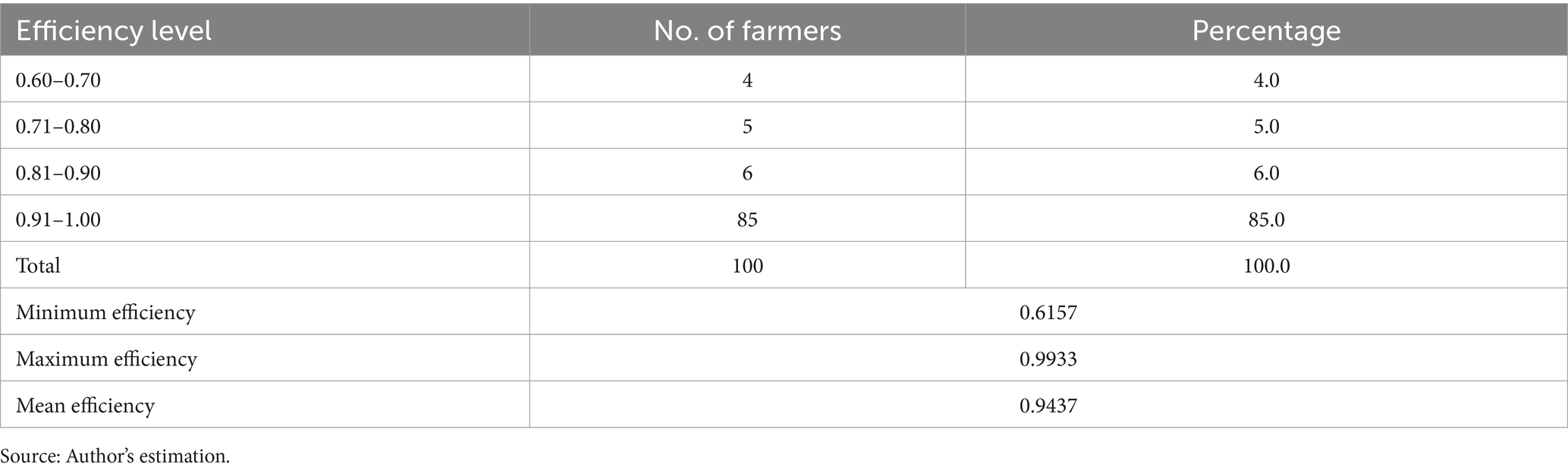
Table 3. Frequency distribution of technical efficiency estimates from C-D stochastic frontier production function.
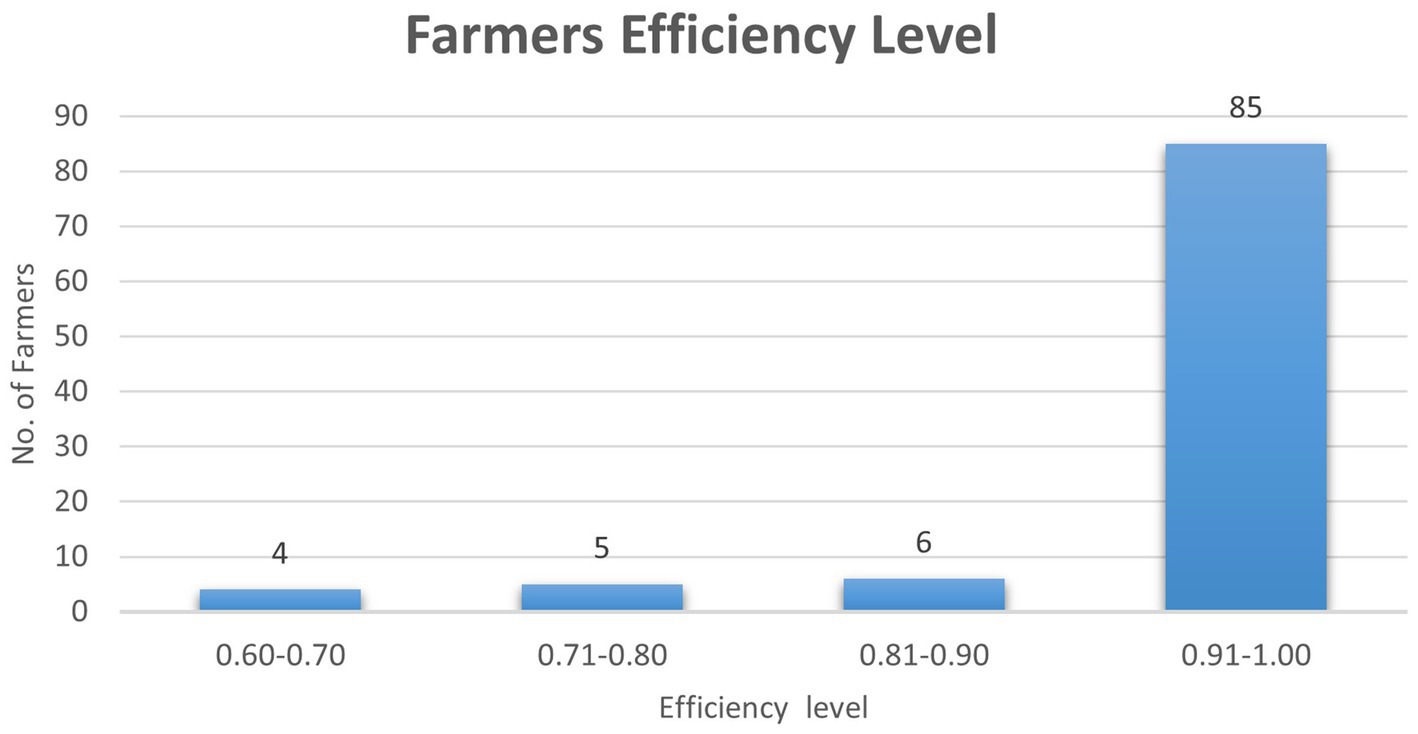
Figure 3. Technical efficiency scores of the individual pineapple farmers. The frequency distribution of technical efficiency estimates from the C-D stochastic frontier production function is demonstrated with percentages in this bar chart. Using the results, we may suggest suitable policies based on the nature of each farmer and their farm enterprise.
3.4 Tests of hypotheses on the parameters of the technical inefficiency model
Generalized likelihood-ratio tests of null hypotheses that the technical inefficiency effects are absent are presented in Table 4. The first null hypothesis, which specified that the inefficiency effects were absent from the stochastic production frontier model, was strongly rejected. The second null hypothesis, which specified that the inefficiency effects were not stochastic for the production frontier model, was also strongly rejected. The third null hypothesis, considered in Table 4, specifies that the inefficiency effects of stochastic production were not a linear function of age, education, credit, training, and family size. This null hypothesis was also strongly rejected at a 5 percent level of significance. This indicates that the joint effect of these five explanatory variables on the inefficiency of production was significant although the individual effect of one or more variables might not be statistically significant. The inefficiency effect in the stochastic production frontier was stochastic and was not uncorrelated to age, education, credit, training, and family size. Thus, it appears that, in this application, the proposed inefficiency stochastic production frontier model was a significant improvement over the corresponding stochastic frontiers which do not involve a model for the technical inefficiency effects.
3.5 Summary findings of the farm-level prospects
This study explored the future potentiality of fresh pineapples both in raw and processed form in Bangladesh (Figure 4). We are well-informed that pineapple has huge demand both locally and internationally for its several types of uses. Its consumption is increasing day by day because of its different nutritional and medicinal values (Shakil, 2023). Since exporting fresh pineapples is difficult because of their perishable nature, growers suggested that we focus on exporting canned pineapples. Besides, pineapple leaf fine fiber is already used for making different types of products (clothes, rope) in some countries due to its organic nature (Pandit et al., 2020). In the study area, most of the pineapple cultivators are mainly small and marginal-level farmers, they sell their product through trade agents at the village level, and a major share of pineapple marketing is run by this system in Bangladesh. The farmers reported that if it can be marketed through proper marketing channels then these juicy and nutritious pineapples are sure to earn huge amounts of foreign currency through export.
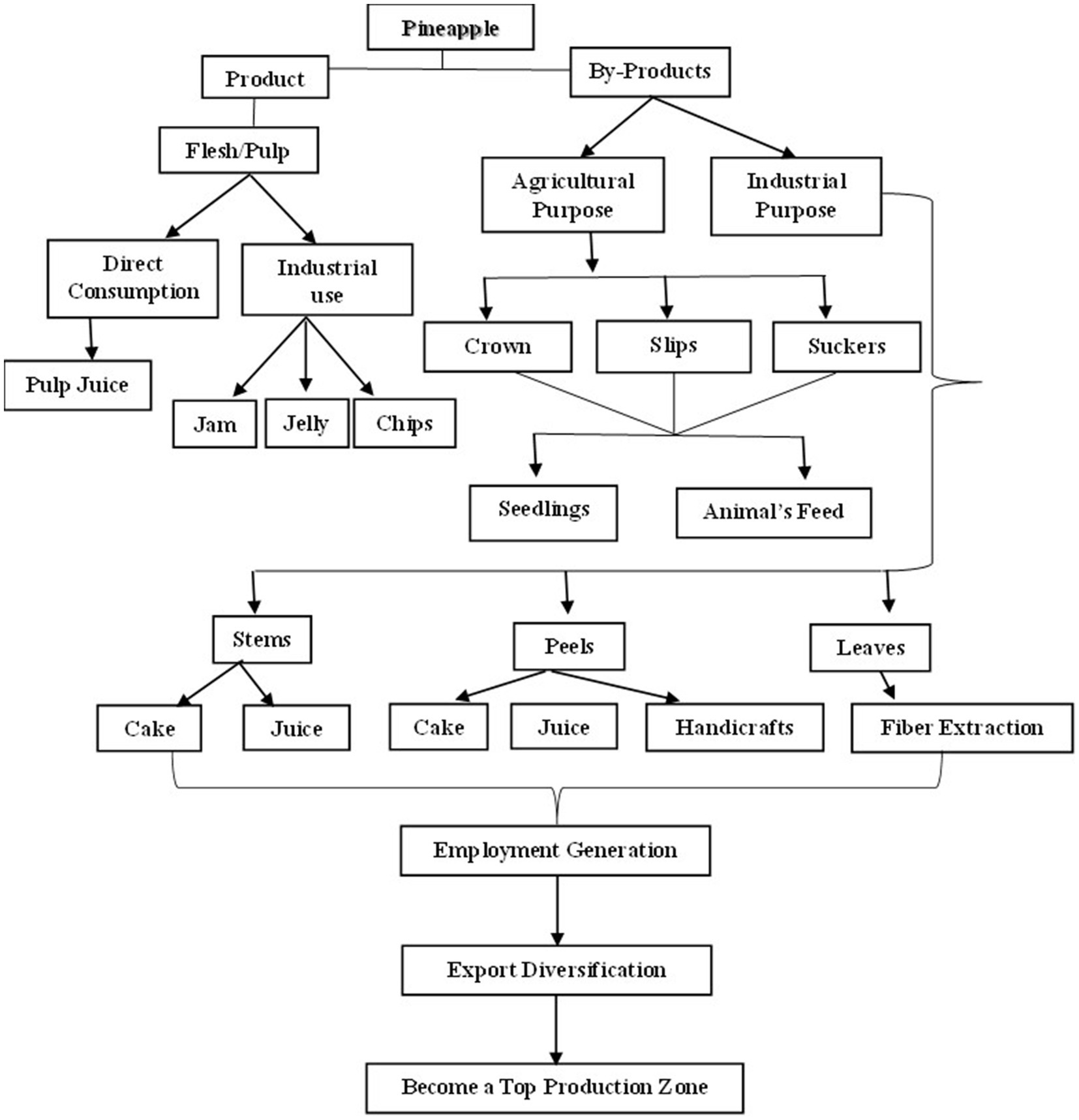
Figure 4. Potentials of a pineapple tree from growers’ perspectives. According to the farmers, there are both qualitative and quantitative advantages to a nation’s pineapple production. It raises farm families’ standards of life and produces long-term profit as it is a perennial crop. The figure depicts the direct contribution that pineapple cultivation and consumption have made to the development of a sustainable agricultural industry for the coming generations.
As pineapple production is increasing every year in Bangladesh, it will be easy to establish a potential export site by utilizing the huge amount of leaf wastage. This leaf is the main raw material for pineapple leaf fiber production. Despite having an efficiency in Bangladesh in cheap labor costs the government provides various incentives for export promotion so natural and organic pineapple leaf fiber can be used to make products rather than synthetic fiber.
It is already known that the European market is the main target of many pineapple exporting countries (Untoro et al., 2021). As Bangladesh has been given different trade facilities by the European Union till now so entering that giant market is quite easy for us. Many Bangladeshis live in different countries of the Middle East and there is a demand for local fruits. Many of the local companies of Bangladesh have strong competitiveness in those markets, strongly similar to the findings of Untoro et al. (2021). So, investing in the canned pineapple market will be beneficiary for Bangladesh. Based on the available raw materials and potential production with a low production cost, there is a high production possibility of canned pineapple and pineapple leaf fiber in the study area.
Moreover, pineapple waste which is rich in fiber can be used as an energy source as well as a good digestive feed for animals such as poultry, broilers, and cows (Figure 4). Buliah et al. (2019) also found that feeding dairy cows with pineapple waste can increase milk production due to an increase in digestion rate.
In addition, the pineapple leaf fiber extraction and weaving industry along with the high demand for canned pineapple ensures the involved people can have income and employment opportunities needed to substantially improve their lives (Figure 4). This will lead to huge potential and economic rewards for indigenous weavers. By improving the lives of their families and their communities, the country can benefit from this. Extraction of fiber, weaving, and embroidery jobs enable them to earn money that allows them to remain at home. By joining this sector, they do not need to join any hazardous and non-prestigious jobs (such as brick fields, rice mills, or domestic workers) to earn money. The qualitative field-level research findings are represented through the following chart:
Since Bangladesh has only a few actual and potential export sectors it is expected that this study will contribute to identifying another potential income and export-earning sector of Bangladesh. We hope academically that the findings of this study will encourage many entrepreneurs and established businessmen to engage in this business.
4 Conclusion and recommendations
Pineapple is one of the few fruits with adequate vitamins and improved productivity in Bangladesh. Efficiency in its production is crucial to food security, poverty abatement, and lessening vitamin-related deficiency among the masses (particularly children) in the state. The study measured the level of technical efficiency and identified the factors influencing the technical inefficiency of pineapple production using the Cobb–Douglas stochastic frontier approach. The study concludes that technical inefficiency was present in pineapple production. The average technical efficiency was estimated as just below 95% across the study area meaning that farmers had been operating their farms below the production frontier. So, the results indicated that there is still scope for a remarkable improvement in technical efficiency in pineapple production with the remaining level of input supply to obtain the optimum level of output efficiency. The efficiency model indicated that seedling is a significant primary input for pineapple farmers’ efficiency. Therefore, if maximum production is to be achieved in the pineapple industry, the quality and optimum quantity of seedlings must be ensured.
The technical inefficiency factors model revealed that the efficiency of farmers was significantly influenced by demographic and institutional factors, such as age, education, training, credit, and family size. The age and education level of farmers had a significant positive influence on technical inefficiency, this positive coefficient indicates that technical efficiencies were significantly higher for the younger farmers, compared to old age groups, and informally educated farmers. On the other hand, credit, training, and family size had a significant negative impact on technical inefficiency, which implies that technical inefficiency will be reduced significantly by increasing access to formal credit and training along with the intervention of productive family members.
Regarding the findings, the study makes the following recommendations: (a) to increase productivity and reduce unemployment and poverty in the district and the nation, the government should create an atmosphere that encourages more young people to work in the pineapple industry. This is due to the following reasons: (i) Results showed that more human work led to lower production; (ii) Youth recruitment into agriculture is crucial because they are likely to be able and willing to accept contemporary technical advancements and use resources perfectly. Farmers need to understand that increasing worker productivity is more important than limiting production with hired labor; (b) Since farmers are not receiving inputs at the government rate, public interventions may be necessary to guarantee that high-quality inputs are reasonably priced. Furthermore, farmers stated that contaminated fertilizers caused them harm. Therefore, it is important to increase public awareness of the need to maintain fertilizer quality at both the local and rural levels; (c) The government should also prioritize education and extension services by bolstering the Department of Agricultural Extension (DAE), establishing farmers’ training centers, formal and informal farmers’ education, and technical and vocational schools; (d) In addition, financial assistance on favorable terms must be given to the farmers who grow pineapples. Eventually, policymakers ought to make some necessary amendments to their policies to make production inputs available to respective farmers at the right time, in the right quantity, and at affordable prices.
It is undeniable that Bangladesh has demonstrated remarkable growth and development, particularly during periods of heightened global unpredictability. Bangladesh went from being one of the world’s poorest countries at birth in 1971 to having a lower middle class in 2015 (The World Bank, 2023). Notwithstanding these successes, the economy continues to face significant obstacles, including growing inflationary pressure, energy scarcity, a deficit in the balance of payments, and a lack of revenue. The trade deficit shrank in Fiscal Year, FY23, while the balance of payments (BoP) deficit and foreign exchange reserves decreased as a result of a contraction in the financial account deficit. In a cutthroat commercial climate, technically proficient and prosperous pineapple growers may write a wonderful tale of poverty alleviation and job creation that would guarantee even a minor contribution to reaching upper-middle-class status by 2031. To maximize productivity given available resources and technological capabilities, it will be necessary to develop human capital, a skilled labor force, effective infrastructure, and a governmental climate that encourages private investment.
The results of this study, notwithstanding its focus on Bangladesh’s northeast, will significantly impact the 12th Sustainable Development Goal (SDG 12 of UNDP) by guaranteeing a more cost-effective and sustainable agri-food production and consumption system.
5 Limitations of the study and further research
Even though this study produced some important findings, it is important to recognize several limitations when evaluating the data. First off, the current study only looked at a small number of parameters to investigate their direct impact on pineapple growers’ technical inefficiency. Future studies in this area should take into account the effects of external variables on pineapple production, such as infrastructure, government regulations, market conditions, and climate change. Second, the paper only considers pineapple production in Bangladesh’s northeastern district, which might not be enough to allow the findings to be applied more broadly. Therefore, to strengthen the relevance and applicability of the study’s findings, future studies should be conducted in this direction including a wider variety of settings and circumstances. The use of cross-sectional data, which makes it difficult to capture the effects over time, and the reliance on farmers’ self-reported measures, which could lead to inaccurate predictions, are two other limitations. Time series data and panel data (He et al., 2023) should be used in this field of study to provide an accurate picture.
Data availability statement
The raw data supporting the conclusions of this article will be made available by the authors, without undue reservation.
Ethics statement
Ethical review and approval was not required for the study on human participants in accordance with the local legislation and institutional requirements. Written informed consent from the [patients/ participants OR patients/participants legal guardian/next of kin] was not required to participate in this study in accordance with the national legislation and the institutional requirements.
Author contributions
TD: Conceptualization, Resources, Investigation, Writing – original draft, Writing – review & editing. JKS: Supervision, Writing – review & editing. MAR: Supervision, Writing – review & editing. KMMA: Writing – review & editing. KA: Formal analysis, Writing – review & editing. AC: Writing – review & editing. MSA: Visualization, Writing – review & editing, Conceptualization.
Funding
The author(s) declare that financial support was received for the research, authorship, and/or publication of this article. This research work was funded by the Ministry of Science and Technology (MoST), Government of the People’s Republic of Bangladesh. The funder has no specific role in the conceptualization, design, data collection, analysis, decision to publish, or preparation of the manuscript.
Acknowledgments
The authors would like to thank the data enumerators for their invaluable support throughout this study. We are also grateful to the study area’s agricultural extension personnel for their technical expertise and administrative assistance. This work would not have been possible without the generous financial support of the MoST. Finally, we would like to thank the study participants for their kind contributions of time and insights.
Conflict of interest
The authors declare that the research was conducted in the absence of any commercial or financial relationships that could be construed as a potential conflict of interest.
Publisher’s note
All claims expressed in this article are solely those of the authors and do not necessarily represent those of their affiliated organizations, or those of the publisher, the editors and the reviewers. Any product that may be evaluated in this article, or claim that may be made by its manufacturer, is not guaranteed or endorsed by the publisher.
References
Abedullah, K., and Bakhsh, A. (2006). Technical efficiency and its determinants in potato production: evidence from Punjab. Pakistan. Lahore J. Econ. 11, 1–22. doi: 10.35536/lje.2006.v11.i2.a1
Adegbite, O., and Adeoye, I. B. (2015). Technical efficiency of pineapple production in Osun state, Nigeria. Agris Econ. Info. National Horticultural Res. Institute 7, 3–12. doi: 10.7160/aol.2015.070101
Adinya, I., Afu, S., and Ijoma, J. (2010). Economic meltdown and decline in pineapple production: determinants of production inefficiency of pineapple-based alley cropping practices in Cross River state, Nigeria. J. Animal and Plant Sci. 20, 107–116.
Ahluwalia, M. S. (1996). New economic policy and agriculture: some reflections. Indian J. Agricul. Econ. Indian Society Agricul. Econ. 51, 412–426. doi: 10.22004/ag.econ.297476
Aigner, D., Lovell, C. A. K., and Schmidt, P. (1977). Formulation and estimation of stochastic frontier production function models. J. Econ. 6, 21–37. doi: 10.1016/0304-4076(77)90052-5
Akhilomen, L. O., Bivan, G. M., Rahman, S. A., and Sanni, S. A. (2015). Economic efficiency analysis of pineapple production in Edo state, Nigeria: a stochastic frontier production approach. J. Experimental Agricu. Int. 5, 267–280. doi: 10.9734/AJEA/2015/13488
Akter, K., Majumder, S., Islam, M. A., and Sarker, B. (2020). Technical efficiency analysis of pineapple production at Madhupur Upazila of Tangail District, Bangladesh. Asian Res. J. Arts Soc. Sci. 12, 32–42. doi: 10.9734/arjass/2020/v12i230187
Alam, M. F., Khan, M. A., and Huq, A. S. M. A. (2012). Technical efficiency in tilapia farming of Bangladesh: a stochastic frontier production approach. J. European Aquaculture Society 20, 619–634. doi: 10.1007/s10499-011-9491-3
Alam, M. A., Sarker, M. A., Hoque, M. J., and Khan, M. S. H. (2019). Use of agrochemicals in pineapple farming: a case study from Madhupur Forest areas of Bangladesh. J. South Pacific Agricul. 22, 10–16.
Ali, S. M. Y., Ahiduzzaman, M., Hossain, M. M., Ali, M. A., Biswas, M. A. M., Rahman, M. H., et al. (2015). Physical and chemical characteristics of pineapples grown in Bangladesh. Int. J. Business, Soc. Scientific Res. 3, 234–246.
Ali, I., Xue-xi, H. U. O., Khan, I., Ali, H., Baz, K., and Khan, S. U. (2019). Technical efficiency of hybrid maize growers: a stochastic frontier model approach. J. Integr. Agric. 18, 2408–2421. doi: 10.1016/S2095-3119(19)62743-7
Amaza, P., and Olayemi, J. (2002). Analysis of technical inefficiency in food crop production in Gombe state. Nigeria. App. Econ. Letters 9, 51–54. doi: 10.1080/13504850110048523
Amoah, S. T., Debrah, I. A., and Abubakari, R. (2014). Technical efficiency of vegetable farmers in Peri-urban Ghana influence and effects of resource inequalities. American J. Agricul. Forestry 2, 79–87. doi: 10.11648/j.ajaf.20140203.14
Bakh, M. E., and Islam, M. S. (2005). Technical and allocative efficiency of growing wheat in northwest districts of Bangladesh. Bangladesh J. Agricul. Econ. 28, 73–83. doi: 10.22004/ag.econ.200223
Balogun, O. L., Adewuyi, S. A., Disu, O. R., Afodu, J. O., and Ayo-Bello, T. A. (2018). Profitability and technical efficiency of pineapple production in Ogun state. Nigeria. Int. J. Fruit Sci. 18, 436–444. doi: 10.1080/15538362.2018.1470594
Banglapedia (2023). National Encyclopedia of Bangladesh. Sreemangal Upazila. Accessed on 15 May, 2024. Available at: https://en.banglapedia.org/index.php/Sreemangal_Upazila
Battese, G. E., and Coelli, T. J. (1992). Frontier production functions, technical efficiency, and panel data: with application to Paddy farmers in India. J. Prod. Anal. 3, 153–169. doi: 10.1007/BF00158774
Battese, G. E., and Coelli, T. J. (1995). A model for technical inefficiency effects in a stochastic frontier production function for panel data. Empir. Econ. 20, 325–332. doi: 10.1007/BF01205442
BBS (2016). Report on the productivity survey of pineapple crop. Dhaka, Bangladesh: Bangladesh Bureau of Statistics, statistics and informatics division (SID). Ministry of Planning, government of the People’s republic of Bangladesh.
BBS (2019). Yearbook of agricultural statistics. 31st series. Dhaka, Bangladesh: Bangladesh Bureau of Statistics, statistics and informatics division (SID). Ministry of Planning, government of the People’s republic of Bangladesh.
BBS (2022). Report on the productivity survey of pineapple crop. Dhaka, Bangladesh: Bangladesh Bureau of Statistics, statistics and informatics division (SID). Ministry of Planning, government of the People’s republic of Bangladesh.
Begum, A. M., Imam, M. F., and Alam, M. A. (2010). Measurement of productivity and efficiency of potato production in two selected areas of Bangladesh: a Translog stochastic frontier analysis. Progress. Agric. 21, 233–245. doi: 10.3329/pa.v21i1-2.16780
Benoit-cattin, M., and Mendez, J. C. (1996). Determinación Paramétrico-Estocástica De La Eficiencia Técnica De La Producción De Café De Los Pequeños Productores en Guatemala. Investigación agraria. Economía 1, 117–138.
BER (2023). Agriculture, chapter seven, Bangladesh economic review, finance division, Ministry of Finance. Dhaka, Bangladesh: Government of the People’s Republic of Bangladesh.
Biswas, P., and Nishat, S. A. (2019). Production and export possibility of canned pineapple and pineapple leaf Fiber in Bangladesh. IOSR J. Business Manag. (IOSR-JBM) 21, 17–23. doi: 10.9790/487X-2109041723
Bravo-ureta, B. E., and Evenson, E. (1994). Efficiency in agricultural production: the case of peasant farmers in eastern Paraguay. Agric. Econ. 10, 27–37. doi: 10.1111/j.1574-0862.1994.tb00286.x
Buliah, N., Jamek, S., Ajit, A., and Abu, R. (2019). Production of dairy cow pellets from pineapple leaf waste. AIP Conference Proceedings 2124, 01–06. doi: 10.1063/1.5117108
Chen, T., Yang, S., and Pan, T. (2001). A study on the technical efficiency of pineapple arms in Taiwan. J. Agricul. Res. China 50, 88–97.
Coelli, T. J. (1995). Recent developments in frontier estimation and efficiency measurement. Aust. J. Agric. Econ. 39, 219–245. doi: 10.1111/j.1467-8489.1995.tb00552.x
Coelli, T. J., and Battese, G. E. (1996). Identification of factors that influence the technical efficiency of Indian farmers. Aust. J. Agric. Econ. 40, 103–128. doi: 10.1111/j.1467-8489.1996.tb00558.x
Conceicao, J. C. P. R., and Araujo, P. F. C. (2000). Fronteira de Produção Estocástica e Eficiência Técnica na Agricultura. Rev. Econ. Sociol. Rural. 38, 45–64.
Datta, T., Saha, J. K., Rahman, M. A., Chowdhury, A., Akter, M., and Gupta, A. D. (2023). The cost-benefit analysis and constraints of pineapple production in Bangladesh. Archives Agricul. Environ. Sci. 8, 397–402. https://dx.doi.org/10.26832/24566632.2023.0803018
Deshwara, M. (2015). Bangladesh: Bumper pineapple harvest in Srimangal. International Tropical Fruits Network. Available at: https://www.itfnet.org/v1/2015/07/bangladesh-bumper-pineapple-harvest-in-srimangal/
DS (2011). District statistics, Bangladesh Bureau of Statistics, statistics and informatics division, Ministry of Planning. Dhaka, Bangladesh: Government of the People’s Republic of Bangladesh.
Essilfie, F. L., Asiamah, M. T., and Nimoh, F. (2011). Estimation of farm level technical efficiency in small scale maize production in the Mfantseman municipality in the central region of Ghana: a stochastic frontier approach. J. Dev. Agric. Econ. 3, 645–654.
Farrell, M. J. (1957). The measurement of production efficiency. J. R. Stat. Soc. 120, 253–281. doi: 10.2307/2343100
Fassinou, H. V. N., Lommen, W. J. M., Van der Vorst, J., Agbossou, E. K., and Struik, P. C. (2012). Analysis of pineapple production Systems in Benin. Acta Hortic. 928, 47–58. doi: 10.17660/ActaHortic.2012.928.4
Ghimire, B., Dhakal, S. C., Marahatta, S., and Bastakoti, R. C. (2023). Technical efficiency and its determinants on lentil (Lens culinary) production in Nepal. Farming System 1, 100045–100010. doi: 10.1016/j.farsys.2023.100045
Haq, A. Z. M. (2013). The impact of agricultural extension contact on crop income in Bangladesh. Bangladesh J. Agric. Res. 38, 321–334. doi: 10.3329/bjar.v38i2.15893
Hasan, S., Ali, M., and Khalil, M. (2011). Impact of pineapple cultivation on the increased income of pineapple growers. Agriculturists 8, 50–56. doi: 10.3329/agric.v8i2.7577
Hasan, S., Hasan, S. S., Saha, S., and Islam, M. R. (2022). Identify problems and suggest possible solutions for safe pineapple production in Madhupur tract. European J. Agricul. Food Sci. 4, 68–74. doi: 10.24018/ejfood.2022.4.5.564
He, S., Yang, S., Razzaq, A., Erfanian, S., and Abbas, A. (2023). Mechanism and impact of digital economy on urban economic resilience under the carbon emission scenarios: evidence from China’s urban development. Int. J. Environ. Res. Public Health 20, 2–20. doi: 10.3390/ijerph20054454
Hossian, M. M., and Abdulla, F. (2015). A time series analysis for the pineapple production in Bangladesh. Jahangirnagar University J. Sci. 38, 49–59.
Islam, K. M. Z., and Sumelius, J. (2011). Technical, economic and allocative efficiency of microfinance borrowers and non-borrowers: evidence from peasant farming in Bangladesh. Eur. J. Soc. Sci. 18, 361–377.
Jahid, A. M. (2023). Early pineapple harvest benefits farmers. The Daily Star, April 30. Available at: https://www.thedailystar.net/business/news/early-pineapple-harvest-benefits-farmers-2086133
Kumbhakar, S. C., and Lovell, C. A. K. (2000). Stochastic frontier analysis. Cambridge, UK: Cambridge University Press.
Meeusen, W., and Broeck, J. V. D. A. (1977). Technical efficiency and dimension of the firm: some results on the use of frontier production functions. Empir. Econ. 2, 109–122. doi: 10.1007/BF01767476
MoF (2022). Bangladesh National Food and nutrition security policy, plan of action; 2021–2030, food planning and monitoring unit (FPMU), ministry of food. Dhaka, Bangladesh: Government of the People’s Republic of Bangladesh.
Moreira, V., Bravo-Ureta, B., Carrillo, B., and Vazquez, J. (2006). Technical efficiency measures for small dairy farms in southern Chile: a stochastic frontier analysis with unbalanced panel data. Archivos de Medicina Veterinaria 38, 25–32. doi: 10.4067/S0301-732X2006000100004
Mussa, E. C. (2011). Economic efficiency of smallholder major crops production in the central highlands of Ethiopia. MS Thesis, Agricultural and Applied Economics Department, Egerton University, Kenya.
Naseer, M. A. R., Ashfaq, M., and Razzaq, A. (2020). Comparison of water use efficiency, profitability and consumer preferences of different Rice varieties in Punjab, Pakistan. Paddy Water Environ. 18, 273–282. doi: 10.1007/s10333-019-00780-9
Ndubueze-Ogarak, M. E., Adeyoola, O. A., and Nwigwe, C. A. (2021). Determinants of technical efficiency of small-holders yam farmers in Nigeria. Rev. Agricul. App. Econ. 24, 13–20. doi: 10.15414/raae.2021.24.01.13-20
NHB (2015). Pineapple. National Horticulture Board, Ministry of Agriculture and farmers welfare. Societies registration act 2005, Gurugram, government of India. Available at: https://nhb.gov.in/PDFViwer.aspx?enc=3ZOO8K5CzcdC/Yq6HcdIxKoWjriAwu4k1X9JJJcK0OA=
Okon, U. E., Enete, A. A., and Bassey, N. E. (2010). Technical efficiency and its determinants in garden egg (Solanum spp) production in Uyo Metropolis, Akwa Ibom state. J. Field Actions 1, 1–6.
Oladapo, M. O., Momoh, S., Yusuf, S., and Awoyinka, Y. (2007). Marketing margin and spatial pricing efficiency of pineapple in Nigeria. Asian J. Market. 1, 14–22. doi: 10.3923/ajm.2007.14.22
Pakravan-Charvadeh, M. R., and Flora, C. B. (2022). Sustainable food consumption pattern with emphasis on socioeconomic factors to reduce food waste. Int. J. Environ. Sci. Technol. 19, 9929–9944. doi: 10.1007/s13762-022-04186-9
Pakravan-Charvadeh, M. R., Flora, C. B., and Emrouznejad, A. (2022). Impact of socio-economic factors on nutrition efficiency: an application of data envelopment analysis. Frontiers in nutrition. Sec. Nutrition Sustain. Diets 9, 1–11. doi: 10.3389/fnut.2022.859789
Pandit, P., Pandey, R., Singha, K., Shrivastava, S., Gupta, V., and Jose, S. (2020). Pineapple leaf fibre: Cultivation and production. Singapore: Green Energy and Technology. Springer, 1–20.
Phrommarat, B., and Oonkasem, P. (2021). Sustainable pineapple farm planning based on eco-efficiency and income risk: a comparison of conventional and integrated farming systems. Appl. Ecol. Environ. Res. 19, 2701–2717. doi: 10.15666/aeer/1904_27012717
Polas, M. A. B. (2013). Profitability and technical efficiency of maize production study in some selected areas of Natore District, MS thesis, Department of Agricultural Finance, Bangladesh agricultural university, Mymensingh-2202, Bangladesh.
Razzaq, A., Qing, P., Naseer, M. A. R., Abid, M., Anwar, M., and Javed, I. (2019). Can the informal groundwater markets improve water use efficiency and equity? Evidence from a semi-arid region of Pakistan. Sci. Total Environ. 666, 849–857. doi: 10.1016/j.scitotenv.2019.02.266
Richetti, A., and Reis, R. P. (2003). The soybean production frontier and economic efficiency in Mato Grosso do Sul, Brazil. Rev. Econ. Sociol. Rural. 41, 153–168. doi: 10.1590/S0103-20032003000100003
Samarpitha, A., Vasudev, N., and Suhasini, K. (2016). Technical, economic, and allocative efficiencies of Rice farms in Nalgonda District of Telangana state. Econ. Aff. 61, 365–374. doi: 10.5958/0976-4666.2016.00047.4
Santos, J., Foster, W., Ortega, J., and Ramifrez, E. (2006). Estudio de la Eficiencia Técnica de Productores de Papas en Chile: El rol del Programa de Transferencia Tecnológica de INDAP. Economía Agraria 10, 119–132.
Sarker, J. R., and Alam, M. F. (2016). Efficiency and economics in cotton production of Bangladesh. J. Agricul. Environ. Int. Develop. 110, 325–348. doi: 10.12895/jaeid.20162.494
Seema, S. A., Banik, J., and Nandi, R. (2023). Diversity of homestead plants in Sreemangal Upazilla. Bangladesh. Asian Plant Res. J. 9, 1–12. doi: 10.9734/aprj/2022/v9i230199
Shakil, M. (2023). Better pineapple yield brings cheers for farmers. The Daily Star, May 11. Available at: https://www.thedailystar.net/business/economy/news/better-pineapple-yield-brings-cheers-farmers-3316671
Tadesse, B., and Krishnamoorthy, S. (1997). Technical efficiency in Paddy farms of Tamil Nadu: an analysis based on farm size and ecological zone. Agric. Econ. 16, 185–192. doi: 10.1111/j.1574-0862.1997.tb00453.x
Taylor, T. G., and Shonkwiler, J. S. (1986). Alternative stochastic specifications of the frontier production function in the analysis of agricultural credit programs and technical efficiency. J. Dev. Econ. 21, 149–160. doi: 10.1016/0304-3878(86)90044-1
Tchale, H., and Sauer, J. (2015). The efficiency of maize farming in Malawi. A bootstrapped Translog frontier. Cahiers d Economie et Sociologie Rurales 82-83, 33–56.
The Daily Tribunal (2023). Sreemangal pineapple going to Country’s other areas. April 08. Available at: https://www.dailytribunal24.com/country/division/58820
The World Bank (2023). The World Bank in Bangladesh, October 04. Available at: https://www.worldbank.org/en/country/bangladesh/overview
Tian, W. M., and Wan, G. H. (2000). Technical efficiency and its determinants in China's grain production. J. Prod. Anal. 13, 159–174. doi: 10.1023/A:1007805015716
Trujillo, J. C., and Iglesias, W. J. (2013). Measurement of the technical efficiency of small pineapple farmers in Santander, Colombia: a stochastic frontier approach. Rev. Econ. Sociol. Rural. 51, s049–s062. doi: 10.1590/S0103-20032013000600003
Uddin, M. T., Roy, S. S., and Dhar, A. R. (2022). Financial profitability and value chain analysis of pineapple in Tangail. Bangladesh. World Food Policy 8, 126–143. doi: 10.1002/wfp2.12039
Untoro, A. W., Waluyati, L. R., and Darwanto, D. H. (2021). Export competitiveness of Indonesia canned pineapple in European Union market, advances in economics, business and management research, Atlantis press international B. V, proceedings of 1st international conference on sustainable agricultural socio-economics, agribusiness, and rural development (ICSASARD), 199, 53–59.
Villano, R., and Fleming, E. (2006). Technical inefficiency and production risk in Rice farming: evidence from Central Luzon Philippines. Asian Econ. J. 20, 29–46. doi: 10.1111/j.1467-8381.2006.00223.x
Keywords: Cobb–Douglas (C-D) production function, stochastic frontier analysis, technical efficiency, sustainable pineapple farming, future prospects and economic policies
Citation: Datta T, Saha JK, Rahman MA, Adnan KMM, Akter K, Chowdhury A and Alamgir MS (2024) Examining technical efficiency, prospects, and policies of farmers: data from a developing nation’s pineapple production. Front. Sustain. Food Syst. 8:1383948. doi: 10.3389/fsufs.2024.1383948
Edited by:
Wioletta Żukiewicz-Sobczak, Calisia University, PolandReviewed by:
Abdur Rouf Sarkar, Bangladesh Rice Research Institute (BRRI), BangladeshMohammad Reza Pakravan-Charvadeh, Lorestan University, Iran
Amar Razzaq, Huanggang Normal University, China
Swati Anindita Sarker, Jiangsu University, China
Matthew Ayeni, Landmark University, Nigeria
Copyright © 2024 Datta, Saha, Rahman, Adnan, Akter, Chowdhury and Alamgir. This is an open-access article distributed under the terms of the Creative Commons Attribution License (CC BY). The use, distribution or reproduction in other forums is permitted, provided the original author(s) and the copyright owner(s) are credited and that the original publication in this journal is cited, in accordance with accepted academic practice. No use, distribution or reproduction is permitted which does not comply with these terms.
*Correspondence: Md. Shah Alamgir, c2FsYW1naXIuYWZiQHNhdS5hYy5iZA==