- 1Department of Agricultural Extension and Education, College of Agriculture, Tarbiat Modares University (TMU), Tehran, Iran
- 2Department of Futures Study, School of Social Sciences, Imam Khomeini International University, Qazvin, Iran
Introduction: Innovation is a fundamental component of agricultural infrastructure, fostering the sector’s development and productivity. Innovation indices tailored to the farm level are benchmarks for assessing innovation within this agricultural context. Therefore, this study aims to discover, localize, and validate comprehensive innovation measurement indices at the farm level. A distinguishing feature of this research is its endeavor to discover and validate a comprehensive tool for measuring innovation at the farm level. Notably, there has been no prior research on discovering comprehensive innovation indices at the farm level, marking a novel aspect of this study.
Method and results: The current research is applied in terms of its purpose and falls into the category of mixed research (quantitative and qualitative), as well as exploratory and descriptive research. This study has been conducted in three stages: The first phase involved reviewing existing literature and extracting indices for measuring innovation. In the second phase, a tool was designed specifically to gauge innovation at the farm level. The third phase entailed face and content validation of the identified indices. This validation process was conducted quantitatively and qualitatively through surveys of subject-matter experts in Iran, and by calculating the content validity ratio (CVR) and content validity index (CVI).
To measure innovation at the farm level, 90 items across 7 dimensions (education, infrastructure, market sophistication, business sophistication, knowledge and technology outputs, creative outputs, and innovative outputs) were extracted, localized, and developed. According to the results, dimensions such as innovative outputs (product innovation, process innovation, and marketing innovation), education (farmers’ education status in technology and innovation), and infrastructure (familiarity, access, and application of Information and Communication Technology [ICT]) demonstrated high credibility based on CVR and CVI scores.
Conclusion: The utilization of innovation assessment indices can help mitigate the challenges associated with evaluating innovation status on farms, thereby enhancing their condition and increasing farmers’ profitability. Accurate and reliable data on innovation status empower farmers in market competition and improve economic performance. Attention to innovation and the use of assessment tools can facilitate the attraction of new investors to the agricultural industry. Furthermore, improving agricultural processes through innovative technologies can contribute to the preservation of natural resources and the reduction of environmental pollution.
1 Introduction
Agriculture serves as the foundation of human survival and the economic and industrial base of many countries. However, its development still faces specific challenges such as food security, imbalances in nutritional structure, and resource scarcity (Lei et al., 2023). There is a widespread belief that past technologies and experiences are no longer sufficient to address the opportunities and challenges agriculture will face in the future (Fraser and Campbell, 2019). Emerging trends in agricultural technologies and innovations enable the production of animal and plant products with higher efficiency and reduced environmental impact (Webb and Forum, 2018).
For instance, in a study conducted by Maroušek et al. (2013, 2023a), the economic and environmental benefits of producing and applying digestate biochar (derived from waste materials from the gas production process in biogas plants) were discussed. This biochar plays a significant role in wastewater treatment, reducing associated costs, improving soil quality, and controlling environmental pollution (Maroušek et al., 2023b). Additionally, Maroušek et al. (2022) demonstrated in another study that the application of silica nanoparticle technology (synthesized from coir pith using the acidic sol–gel method) enhances plant germination and growth while lowering production costs compared to traditional chemical and physical methods. In another study, they mentioned the utilization of an extractor (a device for extracting useful substances or energy-generating substances from raw materials) combined with shock pressure to extract rapeseed oil. In this method, biogas serves as an energy source to create pressure in the extractor device, leading to increased oil production and improved extraction efficiency. High pressure facilitates better and faster oil extraction and optimal utilization of renewable energy (Maroušek, 2013; Maroušek et al., 2013). These innovative methods aim to enhance farm performance, improve the quality of agricultural products, reduce production costs, increase yields, boost income, and ensure food security. From an environmental perspective, these innovations can help improve water and soil quality, reduce greenhouse gas emissions, enhance energy efficiency, and promote the efficient use of natural resources (Ogundari and Bolarinwa, 2018; Hellerstein and Vilorio, 2019; Herrero et al., 2020; Maroušek et al., 2023a,c).
Developing countries heavily rely on agriculture; however, unfortunately, productivity in this sector remains very low. Agricultural innovations play a crucial role in enabling producers to tackle the sector’s major challenges (Lema et al., 2021). A new report by the World Bank emphasizes that developing countries must significantly enhance agricultural innovation and the adoption of technology by farmers to eradicate poverty, meet the growing demand for food, and address the adverse effects of climate change (Fuglie et al., 2019). Iran is no exception. With agriculture contributing approximately 7% of GDP, employing 18% of the workforce, and accounting for 6% of non-oil exports, while also supplying about 80% of food and 80–90% of raw materials required by industries, it holds a significant position in the country’s macroeconomy (Bakhshayesh et al., 2020). In pursuit of economic development, the country has formulated a vision document aiming to establish itself as the leading knowledge-based economy in the region by 2026 (Azimi et al., 2021). One of the key areas within this knowledge-based economy framework is knowledge-based agriculture, which entails increasing productivity and maximizing the utilization of knowledge and technology in agricultural and industrial production across the country (Lainez et al., 2018). From the perspective of knowledge-based agriculture, the critical aspect is to foster innovation based on various technologies that should be accompanied by research (Lainez et al., 2018). It’s worth noting that these technologies must be economically viable, as technologies that fail to generate economic returns cannot be considered sustainable in the long term. The sustainability of technology is directly linked to its profitability, as only profitable technologies can be continually developed and prove beneficial to society and the environment over an extended period (Akbari et al., 2021; Pavolova et al., 2021).
In Iran, challenges such as high water consumption in agriculture, low yield per hectare in various crops and horticulture, as well as in livestock production, and deficiencies in production technology leading to low productivity, exemplify the current state of the agricultural sector in the country (Keshavarz et al., 2021), which is not in line with the principles of a knowledge-based economy. Therefore, considering the existing situation of the agricultural sector in Iran and the significance of innovation in a knowledge-based economy, prioritizing the development of innovation in the agriculture sector as a foundational pillar of such an economy should be a top priority for policymakers and researchers in the country.
The impact of innovation on economic growth and economic superiority can be achieved in two ways: first, innovation can increase efficiency and the utilization of scarce resources, and second, it can enable the utilization of these resources in activities that generate more wealth. Therefore, given the importance of this issue, the measurement indices of innovative agriculture should be examined to assess and enhance the current status of innovation. However, despite the significance of innovation in the agricultural sector, agricultural industries are rarely the focus of innovation policies and are not perceived as underdeveloped sectors, or at least sectors in need of comprehensive research (Andrade et al., 2020). Only a few countries have attempted to identify indices of innovation in this sector, which contrasts with the attention given to the industrial and service sectors (OECD, 2018).
For instance, Bjerke and Johansson (2022) conducted a study on the innovation situation in agricultural and non-agricultural knowledge-based companies in Sweden. The indicators examined to measure innovation in companies included: human capital (employees with higher education, employees’ work experience), the company’s cooperation with other companies to develop its business, the introduction of products, services, processes, or new/improved marketing methods within the past 2 years (Bjerke and Johansson, 2022). Additionally, Buchana and Sithole (2023) presented a conceptual framework to measure innovation in agricultural knowledge-based companies in developing countries in sub-Saharan Africa. In this framework, innovation was generally divided into two categories: technological innovations (product and process innovation) and non-technological innovations (organizational innovation and marketing), with indicators related to the measurement of each category determined (Buchana and Sithole, 2023). Furthermore, Oliveira et al. (2019) considered factors such as product, process, marketing, and institutional innovation (incentives and institutional policies) to measure innovation in agriculture. Dutta et al. (2018) measured innovation in agricultural and food systems and offered a framework based on global innovation indicators. The indicators examined in this study included: (a) human capital and research, (b) market sophistication: financial markets, (c) knowledge and technology outputs, and (d) creative outputs (Dutta et al., 2018). Läpple et al. (2015) also employed a composite indicator in their study to evaluate innovation in agriculture, including components related to the acceptance of innovation, knowledge acquisition, and investment in machinery at the farm level.
Currently, there is a shortage of research in the field of measuring innovation in the agricultural sector, especially at the farm level. One of the main reasons for this is the lack of indices to measure innovation. According to studies, the indices presented in this area are either incomplete or not suitable for the farm level (Lei et al., 2023). Therefore, in addition to comprehensively identifying innovation measurement indices, it is necessary to localize these indices according to the farm level to investigate the innovation status in this field. The main challenge is the localization and development of innovation measurement indices appropriate for the farm level, followed by validation to determine their validity. To validate, the indices should undergo evaluation by several experts to create a list of items suitable for this sector with the necessary validity. The validated items are then used to measure the innovation status. In this context, the primary research question can be framed as follows: Which indices are appropriate and tailored for the agricultural sector? Additionally, how do experts evaluate the validity of these indices and their components in the context of farm conditions?
2 Research method
The method of this research is presented in detail in the following three steps:
2.1 The first step
In this step, extracting the indices of innovation was done by reviewing library resources and previous literature. The statistical population for this research step includes all articles related to this field that were published in prestigious journals between 2013 and 2023. We chose to focus on these 10 years because, considering the study’s emphasis on innovation, it’s crucial to incorporate more recent research. To search and find the articles, we used the keywords ‘innovation,’ ‘innovation in agriculture,’ ‘innovation in farm,’ ‘product innovation,’ ‘process innovation,’ ‘marketing innovation,’ ‘innovation system,’ ‘agricultural innovation system,’ ‘innovation indices,’ ‘innovation indices in agriculture’ were looked for in scientific databases such as Nature, Civilica, Ricest, Science Magazine, Elsevier, Scopus, Springer, Emerald, Web of Science, Magiran. To select the articles, 55 journals were reviewed, the most papers were published in the journals “research policy,” “agricultural systems,” “food policy,” “technology prediction, and social change,” and “innovation and development.” The selection process was as follows: at first, 302 articles were identified through the relevant title and then articles with relevant abstracts and related keywords were investigated. Furthermore, during the subsequent steps of selecting valid articles, those lacking a valid publisher were excluded from further consideration in the study. Ultimately, 32 articles and one book were included for content analysis. Figure 1 depicts the process of article selection along with the inclusion and exclusion criteria for the content analysis. The review began with the most relevant and highly cited articles, continuing until theoretical saturation was achieved.
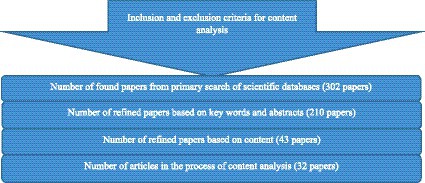
Figure 1. Steps to selecting articles and inclusion and exclusion criteria for articles in the process of content analysis.
In this part of the study, for data analysis, qualitative content analysis of documents with a deductive approach was used to extract innovation indices. Qualitative content analysis serves as a research method for subjectively interpreting textual data through systematic classification, encoding, and theme development, based on known patterns. These definitions underscore how qualitative content analysis enables researchers to subjectively interpret the authenticity and truthfulness of data while maintaining a scientific approach. The presence of a systematic encoding process ensures the objectivity of the results. Qualitative content analysis delves deeper than the words or objective content of texts, exploring both apparent and hidden themes or patterns within explicit content (Graneheim et al., 2017; Kleinheksel et al., 2020).
Qualitative content analysis encompasses two main approaches: inductive and deductive. Inductive content analysis becomes essential when there’s insufficient information about a phenomenon, and the researcher aims to establish necessary background knowledge. Here, the objective of inductive research is to facilitate the emergence of research findings by identifying dominant and common themes within the data. This involves gradually summarizing collected data to ultimately uncover the main concepts and themes pertinent to the research topic. Unlike the deductive approach, which begins with a predetermined theoretical framework and tests hypotheses, inductive content analysis adopts a bottom-up, exploratory, and open approach. In this method, coding initiates without predetermined codes, allowing researchers to identify codes (main categories) through the analysis of the data set (Krippendorff, 2018; Kleinheksel et al., 2020).
Deductive content analysis, unlike inductive content analysis, relies on pre-existing research or theories to analyze data. Researchers begin with a set of predetermined categories or codes and then apply them to the data in a top-down approach for the sake of meaning-making. The use of this approach becomes necessary when the research is to test previous theories or expand them in a different context. Existing theories and literature in a field can help predict variables and the relationships between them. Hence, it is possible to guide the study process in a more structured way by taking advantage of previous views. The existing theories help us achieve the operational definition of the desired concepts. This operational definition provides the required categories of research. The operational definition is composed of implicit attributes in each variable, and these attributes must be comprehensive. The main categories representing the subject under investigation are extracted from the operational definition. While conducting qualitative content analysis with a deductive approach, the research starts with theory and theoretical framework. Defining the codes is done before and during the analysis, and extracting the codes is done from the theory or research findings. Coding in this approach is top-down, where researchers start with a set of predetermined codes (categories) and then find excerpts that fit those codes (Krippendorff, 2018; Kibiswa, 2019; Kleinheksel et al., 2020). The reason why we used this approach in the current study was to expand the theory related to global innovation indicators in the field of agriculture and at the farm level, and the coding was carried out with a set of predetermined codes (main categories) which are considered to be the global indicators of innovation (human capital and research, market sophistication, business sophistication, infrastructure, knowledge and technology outputs, creative outputs, and innovative outputs), and then related excerpts (concepts) found by reviewing previous studies were placed in the main categories (Table 1).
2.2 The second step
The second step consisted of localization of the extracted innovation indices according to the farm level and then designing primary questionnaires. This questionnaire was designed by the researcher. To design a researcher-made questionnaire, first, the global innovation indices and indices related to the innovation model of the OECD were used, then these indices were localized according to the farm level, and valid sources were used to localize the indices (Dutta et al., 2018; OECD, 2018; Lei et al., 2023). These dimensions and indices are:
1. Education (Farmers’ education status): This indicator has 16 items measured by a 5-point Likert scale and an interval scale.
2. Infrastructure: This dimension has three general indices (familiarity with the application of ICT, access to ICT, and application of ICT by farmers) and 24 items designed as a 5-point Likert scale.
3. Business sophistication: This dimension was localized at the farm level with the title of the type of inputs used and had 8 items and redesigned as a question format.
4. Market sophistication: This dimension was localized at the farm level with the title of the credit indicator for the renovation of farms and the number of credits for the preparation of new inputs and had 10 items that were designed as open question and interval scale.
5. Knowledge and Technology Outputs: This dimension was localized at the farm level with the title of production factors productivity indicator and had 12 items that were designed as open question and interval scale.
6. Creative outputs: This dimension was designed at the farm level, with 2 items (trademarks and patents) as a nominal scale.
7. Innovative outputs: This dimension was localized at the farm level with the title of product innovation indicator, process innovation, and marketing innovation and had 18 items that were designed as a nominal scale, a five-point Likert scale, and an interval scale.
2.3 The third step
At this step, the compiled indices were sent to the subject-matter experts in Iran for quantitative and qualitative validation. Surveys of subject-matter experts were conducted in two stages. In the first stage, the face validity of the questionnaire was tested. To do the initial assessment, the extracted indices were sent to 10 subject-matter experts, and after getting feedback and applying their opinions, several cases were removed and some of them were corrected. At this stage, the face validity of the questionnaire was approved. In this stage, the experts were asked whether the appearance of the questionnaire was properly designed to evaluate the researcher’s goal (identification and localization of innovation indices at the farm level). In this stage, subject-matter experts were asked about the suitability, attractiveness, and logical sequence of questions as well as the abbrevity and comprehensiveness of the questionnaire (Dehhaghi et al., 2022). After approving face validity, to determine the qualitative and quantitative content validity, the questionnaire was sent to the subject-matter experts. Subject-matter experts included university professors and subject-matter experts in the field of innovation and innovation in the agricultural sector in Iran. Criterion-based sampling method was used to select these subjects. In the criterion-based sampling method, several criteria are identified, and based on the criteria the statistical population is limited and more limited to achieve the desired sample (Creswell and Creswell, 2017). In this research, participants were included based on the following criteria: (a) holding a doctoral degree in the field of study (innovation or entrepreneurship in agriculture), (b) having published an article on the topic under investigation, (c) demonstrating accountability, and (d) possessing over 10 years of agricultural experience. A total of 24 individuals were selected as research participants to evaluate both the qualitative and quantitative content validity.
3 Content validity
Content validity refers to a process that determines to what extent the dimensions and elements of a concept can be successfully defined (Ghazali et al., 2017). The content validity indicates how much a scale or questionnaire measures all aspects of the desired construct. To determine the content validity of the questionnaire, experts’ judgment and the assessment of the coverage extent and appropriateness of the questions with the objectives of the research were used. In this study, this indicator was used to ensure whether the items related to the questionnaires were designed in the best possible way or not. One of the most widely used methods for measuring content validity was created by Lawshe in 1975. It is essentially a method of measuring agreement between assessors or referees (inter-rater agreement) about the importance of a particular item (Obilor and Miwari, 2022). In this study, to quantitatively confirm the content validity of questionnaires, Lawshe’s (1975) method was used. In Lawshe’s (1975) method, CVR and CVI were determined. To calculate this ratio, the members of the expert board judge each item in terms of the necessity and relevance of the item.
3.1 Determining content validity ratio (CVR)
To determine this coefficient, the questions were given to experts in the form of a questionnaire, and they were asked to determine the appropriateness of each of the items of the questionnaire based on a three-point range of “necessary”, “useful but not necessary” and “not necessary”. After collecting expert opinions, the CVR for each item was determined using the following formula.
In this formula:
N: The total number of experts.
n: The number of experts who have chosen the “necessary” option
In the Lawshe method, the minimum acceptable CVR is determined based on the number of board members. The minimum CVR required for board members of 24 is 0.37 (Table 2) (Lawshe, 1975). Therefore, this coefficient was applied to each of the questions, and questions whose validity was less than this amount were excluded from the questionnaire, but questions that were equal to or bigger than this amount were included in the questionnaire.
3.2 Determining content validity index (CVI)
To calculate CVI, experts were asked to give their opinion based on the 4-point Likert scale, ‘Fully relevant,’ ‘requiring little change,’ ‘requiring a lot of change,’ and ‘unrelated.’ CVI was calculated for each question, and their mean was also calculated. CVI score for each phrase was calculated by dividing the number of experts agreeing to the 4 and 3 (highly relevant and requiring little change) by the total number of experts (Haghjoo et al., 2022).
The questions whose CVI was lower than 0.7 were excluded. Questionnaires whose CVI was between 0.7 and 0.79 were reviewed and corrected, and they were included in the questionnaire with a slight change, and the questions whose CVI was higher than 0.79 were confirmed (Obilor and Miwari, 2022). Finally, the questionnaire was examined in several stages in terms of the validity of the questions and their content, and necessary corrections were made.
4 Findings
To measure farm-level innovation, 90 items in 7 dimensions (education, infrastructure, market sophistication, business sophistication, knowledge and technology outputs, creative outputs, and innovative outputs) were extracted in the first stage. At this stage of the study, after investigating the face validity, CVR was evaluated. The minimum acceptable coefficient based on the number of experts in this study (24 people) is 0.37. According to the coefficients, the questions with validity less than this number were excluded from the questionnaire, and cases equal to or bigger than 0.37 were included in the questionnaire. The results are visible in Table 3. According to the results, 11 items were excluded from the questionnaire based on this coefficient. In addition, the results indicated that the average of CVR of all dimensions (education, infrastructure, market sophistication, business sophistication, knowledge and technology outputs, creative outputs, and innovative outputs) and indices related to them are more than 0.37. This shows that all dimensions and indices related to them have good content validity to measure the status of innovation at the farm level. After investigating the CVR and removing some items, CVI was also used to determine the content validity of the questionnaire. As stated in the research method, if the value of this coefficient is less than 0.7, the item is removed, if it is between 0.7 and 0.79, it should be reviewed, and if it is bigger than 0.79, it is acceptable. According to the results of Table 3, the CVI of three items of the remaining items was less than 0.7 and was excluded from the questionnaire. The coefficient of 26 items ranged from 0.7 to 0.79, so they were reviewed and the rest of the items were acceptable. Also, according to the results of CVI in Table 3, dimensions of education, infrastructure, market sophistication, knowledge and technology outputs, creative outputs, and innovative outputs and indices related to them (education status, familiarity with the application of ICT, access to ICT, application of ICT, credit for equipping and renovating farms, credit for the preparation of new inputs, productivity of production factors, trademarks and patents, product innovation, process innovation, and marketing innovation) were more than 0.79. However, some of the items related to the dimension of business sophistication needed to be revisited. In general, based on this coefficient, dimensions and indices had good content validity to measure the status of innovation at the farm level. According to the results presented in Table 4 and Chart 1, among the dimensions associated with measuring the status of innovation at the farm level, innovative outputs (product innovation, process innovation, and marketing innovation), education (farmers’ education status in technology and innovation), and infrastructure (familiarity, access, and application of ICT) exhibit high validity, as indicated by their average CVR and CVI scores. Overall, considering the total average derived from both CVI and CVR, the questionnaire demonstrates excellent validity in assessing the status of innovation at the farm level. Therefore, these indices have face and content validity for measuring the status of innovation at the farm level.
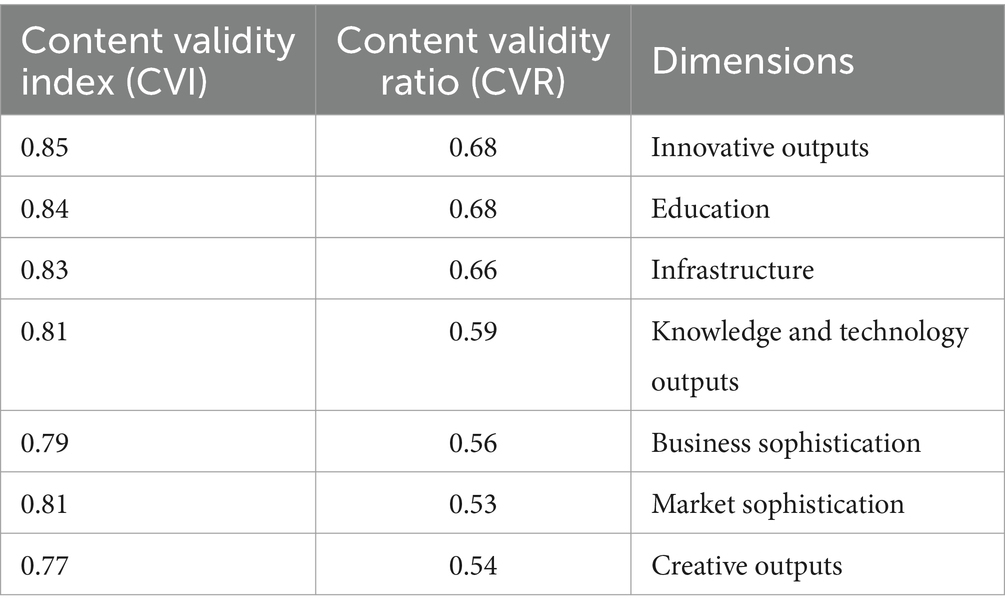
Table 4. The average CVR and CVI for dimensions of the questionnaire to assess the status of innovation at the farm level.
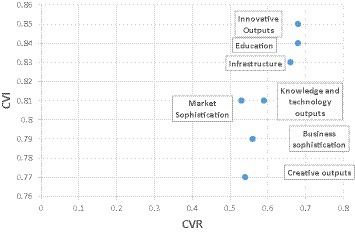
CHART 1. The average CVR and CVI for dimensions of the questionnaire to assess the status of innovation at the farm level.
5 Discussion and conclusion
Based on the results, the validity of indices measuring the innovation status at the farm level, the indices of innovative outputs (product innovation, process innovation, and marketing innovation), education (the status of farmers’ education in the field of innovation and technology), and the infrastructure indicator (familiarity, access, and application of ICT) had the highest CVR and CVI among the extracted indices. In other words, from the viewpoint of experts, these indices are the most essential and most relevant indices for measuring the status of innovation at the farm level. These findings are consistent with the findings of Dutta et al. and Buchana and Sithole, which indicate the importance of these indices in measuring the status of innovation at the farm level (Van Rijn et al., 2012; Dutta et al., 2018; Buchana and Sithole, 2023). Regarding the importance of the education indicator in measuring the status of innovation in the farm, it can be said that the development of innovation in the agricultural sector requires knowledge and expertise appropriate to such activities. The issue of lack of expertise is even concerned with the ability to use and work with smart hardware in the agricultural sector. Therefore, to improve farmers’ skills and increase their awareness, it is necessary to hold educational courses in the field of innovation and new technologies. Regarding the importance of the indicator of familiarity, access, and application of ICT infrastructures, it can be said that ICT plays a role in the development of new products and are key enabler of innovation. These infrastructures facilitate the exchange of information about innovations and new technologies and transfer them to farmers, eliminate barriers to access to new knowledge, supply new inputs, access to national and international markets to sell innovative products, and establish close relationships between businesses, suppliers, customers, and competitors, which are known as important sources of ideas for innovation. ICT generally facilitates innovation through diffusion processes, usage practices, and commercial success, and helps to solve techno-economic issues (Dvorský et al., 2023; Kliestik et al., 2023). Indices of innovative outputs (product, process, marketing, and organization) are also important indices for measuring innovation. In other words, indices of production of a new product, new or improved modes of production, new marketing or new sales methods to increase the attractiveness of agricultural goods and services, and entry into new markets are among the important indices considered for measuring innovation at the farm level. Also, in the farmers’ education status indicator, three items (technical-educational consultations for farm participatory courses, inviting international professors to train, and sending the farmers abroad for education) were excluded because their CVR and CVI were low. The reason for the low score of these items in their view is that the experts believed that these items are not very appropriate to the conditions of education of Iranian farmers and are not implemented. Therefore, no necessity and importance lie behind the measurement of these items. In the infrastructure dimension, six items (familiarity with remote sensing systems in agricultural activities, familiarity with the application of radio wave identification systems, access to remote sensing systems, access to radio wave identification systems, application of remote sensing systems, application of radio wave identification systems in agricultural activities) were eliminated due to lack of necessity or lack of tight relation with the desired dimension. According to experts, these items also had low scores for measuring the status of innovation at the farm level, so they were excluded from the questionnaire, and the reason for the low score of these items in their views was that these facilities and infrastructure are used in a very limited level or not used at all at the level of agricultural farms in Iran. Therefore, there is no need to measure these items. In the innovative output dimension, five items (turnover due to the introduction of new product for the market, turnover due to the introduction of new crops for the farm, turnover of non-new crops and products with little change, newness of at least one innovation in the Middle East, and newness of at least one innovation at the world level) were eliminated due to lack of necessity or lack of tight relation with the desired dimension. The reason for the low score of these items in the opinion of experts and consequently the elimination of the items has been that in their opinion, these items are not suitable for measuring the status of innovation because farmers do not answer financial questions accurately. The two items “newness of at least one innovation in the Middle East” and “newness of at least one innovation at the world level” were also excluded because they are not very related to the state of farm-level innovation.
Overall, this research has addressed fundamental questions related to its objectives, resulting in the identification, localization, and validation of key indices concerning the innovation status at the farm level. It is important to note that the identified indices are deemed valid based on the analyses and calculations conducted. They can be utilized at regional, national, and international levels to assess innovation status on farms and inform planning and policy-making processes. The results indicate that these indices possess both content and face validity, making them suitable for measuring innovation status at the farm level. Indeed, the dimensions and indices introduced facilitate accurate and comprehensive measurement of farm innovation, providing farmers with valuable insights for innovation development, thereby enhancing outputs and economic productivity. Furthermore, this research has consolidated scattered and fragmented knowledge and tools for measuring farm innovation in an integrated manner. Additionally, the qualitative nature of the indices and their ease of assessment contribute to the questionnaire’s strengths, making it suitable and understandable for farmers. Moreover, these indices can be adapted by other researchers to examine innovation in other agricultural sectors, such as greenhouses. It is also likely that the dimensions and indices provided in this research can be generalized for measuring farm-level innovation in countries beyond Iran, although further validation by future researchers will be necessary. Given that one of the objectives of this research phase was to assess farm innovation status using the developed indices, utilizing the results of this questionnaire can help mitigate challenges in reviewing current innovation status and its development. Therefore, it is recommended that experts, planners, and decision-makers in the agriculture sector utilize these indices, which have been endorsed by subject-matter experts.
Data availability statement
The original contributions presented in the study are included in the article/Supplementary material, further inquiries can be directed to the corresponding author.
Ethics statement
Ethical review and approval was not required for the study on human participants in accordance with the local legislation and institutional requirements. Written informed consent from the [patients/participants OR patients/participants legal guardian/next of kin] was not required to participate in this study in accordance with the national legislation and the institutional requirements.
Author contributions
EF: Conceptualization, Data curation, Formal analysis, Investigation, Methodology, Project administration, Resources, Software, Visualization, Writing – original draft. SC: Conceptualization, Methodology, Supervision, Validation, Writing – review & editing. MN: Conceptualization, Data curation, Resources, Validation, Visualization, Writing – review & editing.
Funding
The author(s) declare that no financial support was received for the research, authorship, and/or publication of this article.
Conflict of interest
The authors declare that the research was conducted in the absence of any commercial or financial relationships that could be construed as a potential conflict of interest.
Publisher’s note
All claims expressed in this article are solely those of the authors and do not necessarily represent those of their affiliated organizations, or those of the publisher, the editors and the reviewers. Any product that may be evaluated in this article, or claim that may be made by its manufacturer, is not guaranteed or endorsed by the publisher.
Supplementary material
The Supplementary material for this article can be found online at: https://www.frontiersin.org/articles/10.3389/fsufs.2024.1365687/full#supplementary-material
References
Akbari, M., Loganathan, N., Tavakolian, H., Mardani, A., and Štreimikienė, D. (2021). The dynamic effect of micro-structural shocks on private investment behavior. Acta Montan. Slovaca 26, 1–7. doi: 10.46544/ams.v26i1.01
Andrade, D., Pasini, F., and Scarano, F. R. (2020). Syntropy and innovation in agriculture. Curr. Opin. Environ. Sustain. 45, 20–24. doi: 10.1016/j.cosust.2020.08.003
Ariza, C., Rugeles, L., Saavedra, D., and Guaitero, B. (2014). Measuring innovation in agricultural firms: a methodological approach. Electron. J. Knowl. Manag. 11, 185–198.
Azimi, N. A., Samandar Ali Eshtehardi, M., and Fakhremoosavi, E. (2021). The efficiency of the National Innovation System of Iran and selected countries. QJER 21, 117–149.
Bakhshayesh, M., Keshavarz, A., and Shariatmadar, M. H. Agricultural Economics Report. National Center for Agricultural and Water Strategic Studies (2020). Available at: http://awnrc.com
Bencoova, B., Grosos, R., Gomory, M., Bacova, K., and Michalkova, S. (2021). Use of biogas plants on a national and international scale. Acta Montan. Slovaca 26, 139–148. doi: 10.46544/AMS.v26i1.12
Bjerke, L., and Johansson, S. (2022). Innovation in agriculture: an analysis of Swedish agricultural and non-agricultural firms. Food Policy 109:102269. doi: 10.1016/j.foodpol.2022.102269
Buchana, Y., and Sithole, M. M. (2023). Towards a conceptual framework for measuring innovation in the agricultural sector in sub-Saharan developing countries. Afr. J. Sci. Technol. Innov. Dev. 15, 272–282. doi: 10.1080/20421338.2022.2072794
Cavdar, S. C., and Aydin, A. D. (2015). An empirical analysis of technological development and innovation indicators. Procedia Soc. Behav. Sci. 195, 1486–1495. doi: 10.1016/j.sbspro.2015.06.449
Chang-Muñoz, E., Mercado-Caruso, N., Gazabon, D. O., Segarra-Oña, M., and Osorio, S. N. (2022). Product or process innovation? The dilemma for exporting SMEs in emerging economies: the case of the Colombian Caribbean. Procedia Comput. Sci. 198, 620–625. doi: 10.1016/j.procs.2021.12.296
Cirera, X., and Muzi, S. (2020). Measuring innovation using firm-level surveys: evidence from developing countries. Res. Policy 49:103912. doi: 10.1016/j.respol.2019.103912
Creswell, J. W., and Creswell, J. D. (2017). Research design: Qualitative, quantitative, and mixed methods approaches. 4th Edition, California: Sage publications.
Crowley, F. (2017). Product and service innovation and discontinuation in manufacturing and service firms in Europe. Eur. J. Innov. Manag. 20, 250–268. doi: 10.1108/EJIM-03-2016-0027
Cruz-Cázares, C., Bayona-Sáez, C., and García-Marco, T. (2013). You can’t manage right what you can’t measure well: technological innovation efficiency. Res. Policy 42, 1239–1250. doi: 10.1016/j.respol.2013.03.012
Dehhaghi, S., Choobchian, S., Ghobadian, B., and Farhadian, H. (2022). Policy model of renewable energy development in Iran’s agriculture sector. J. Renew. Energy Environ. 9, 93–106. doi: 10.30501/jree.2022.311064.1273
Dutta, S., Lanvin, B., and Wunsch-Vincent, S. The Global Innovation Indicator, Measuring Innovation in Agriculture and Food Systems. (2018). Available at: https://www.wipo.int/edocs/pubdocs/en/wipo_pub_gii_2017-annex4.pdf
Dvorský, J., Bednarz, J., and Blajer-Gołębiewska, A. (2023). The impact of corporate reputation and social media engagement on the sustainability of SMEs: perceptions of top managers and the owners. Equilib. Q. J. Econ. Econ. Policy 18, 779–811. doi: 10.24136/eq.2023.025
Dziallas, M., and Blind, K. (2019). Innovation indicators throughout the innovation process: an extensive literature analysis. Technovation 80-81, 3–29. doi: 10.1016/j.technovation.2018.05.005
Fraser, E. D., and Campbell, M. (2019). Agriculture 5.0: reconciling production with planetary health. One Earth 1, 278–280. doi: 10.1016/j.oneear.2019.10.022
Fuglie, K., Gautam, M., Goyal, A., and Maloney, W. F. (2019). Harvesting prosperity: Technology and productivity growth in agriculture. Washington, DC, USA: World Bank Publications.
García-Granero, E. M., Piedra-Muñoz, L., and Galdeano-Gómez, E. (2018). Eco-innovation measurement: a review of firm performance indicators. J. Clean. Prod. 191, 304–317. doi: 10.1016/j.jclepro.2018.04.215
Ghazali, N., Nordin, M. S., Hashim, S., and Hussein, S. Measuring content validity: students’ self-efficacy and meaningful learning in massive open online course (MOOC) scale. In International Conference on Education in Muslim Society (ICEMS 2017) (2017) (pp. 128–133). Banten, Indonesia: Atlantis Press.
Graneheim, U. H., Lindgren, B. M., and Lundman, B. (2017). Methodological challenges in qualitative content analysis: a discussion paper. Nurse Educ. Today 56, 29–34. doi: 10.1016/j.nedt.2017.06.002
Haghjoo, R., Choobchian, S., Morid, S., and Abbasi, E. (2022). Development and validation of management assessment tools considering water, food, and energy security nexus at the farm level. Environ. Sustain. Ind. 16:100206. doi: 10.1016/j.indic.2022.100206
Hellerstein, D., and Vilorio, D. Agricultural resources and environmental indicators, indices; EIB-208 ; U.S. Department of Agriculture, Economic Research Service: Washington, DC, USA. (2019).
Herrero, M., Thornton, P. K., Mason-D’Croz, D., Palmer, J., Benton, T. G., Bodirsky, B. L., et al. (2020). Innovation can accelerate the transition towards a sustainable food system. Nat. Food 1, 266–272. doi: 10.1038/s43016-020-0074-1
Hu, R., Skea, J., and Hannon, M. J. (2018). Measuring the energy innovation process: an indicator framework and a case study of wind energy in China. Technol. Forecast. Soc. Chang. 127, 227–244. doi: 10.1016/j.techfore.2017.09.025
Islam, N., Wang, Q., Marinakis, Y., and Walsh, S. (2022). Family enterprise and technological innovation. J. Bus. Res. 147, 208–221. doi: 10.1016/j.jbusres.2022.04.004
Ivanov, C. I., and Avasilcăi, S. (2014). Measuring the performance of innovation processes: a balanced scorecard perspective. Procedia Soc. Behav. Sci. 109, 1190–1193. doi: 10.1016/j.sbspro.2013.12.610
Janger, J., Schubert, T., Andries, P., Rammer, C., and Hoskens, M. (2017). The EU 2020 innovation indicator: a step forward in measuring innovation outputs and outcomes? Res. Policy 46, 30–42. doi: 10.1016/j.respol.2016.10.001
Keshavarz, A., Fakari Sardehayi, B., Beiki, A., Khosravi, A., Farsi, M., Malekiyan, R., et al. Challenges of the country's agricultural sector. Tehran: Agricultural Research, Education and Extension Organization, Agricultural Education Publication; (2021).
Kibiswa, N. K. (2019). Directed qualitative content analysis (DQlCA): a tool for conflict analysis. Qual. Rep. 24, 2059–2079. doi: 10.46743/2160-3715/2019.3778
Kleinheksel, A. J., Rockich-Winston, N., Tawfik, H., and Wyatt, T. R. (2020). Demystifying content analysis. Am. J. Pharm. Educ. 84:7113. doi: 10.5688/ajpe7113
Kliestik, T., Nica, E., Durana, P., and Popescu, G. H. (2023). Artificial intelligence-based predictive maintenance, time-sensitive networking, and big data-driven algorithmic decision-making in the economics of the industrial internet of things. Oeconomia Copernicana 14, 1097–1138. doi: 10.24136/oc.2023.033
Krippendorff, K. (2018). Content analysis: an introduction to its methodology (5th ed). California, CA: Sage Publications.
Lainez, M., González, J. M., Aguilar, A., and Vela, C. (2018). Spanish strategy on bioeconomy: towards a knowledge-based sustainable innovation. New Biotechnol. 40, 87–95. doi: 10.1016/j.nbt.2017.05.006
Läpple, D., Renwick, A., and Thorne, F. (2015). Measuring and understanding the drivers of agricultural innovation: evidence from Ireland. Food Policy 51, 1–8. doi: 10.1016/j.foodpol.2014.11.003
Lawshe, C. H. (1975). A quantitative approach to content validity. Pers. Psychol. 28, 563–575. doi: 10.1111/j.1744-6570.1975.tb01393.x
Lei, X., Li, J., Li, H., Yan, J., Li, P., Guo, Y., et al. (2023). Regional assessment at the province level of agricultural science and technology development in China. Agriculture 13:389. doi: 10.3390/agriculture13020389
Lema, R., Kraemer-Mbula, E., and Rakas, M. (2021). Innovation in developing countries: examining two decades of research. Innov. Dev. 11, 189–210. doi: 10.1080/2157930X.2021.1989647
Luo, Q., Miao, C., Sun, L., Meng, X., and Duan, M. (2019). Efficiency evaluation of green technology innovation of China's strategic emerging industries: an empirical analysis based on Malmquist-data envelopment analysis index. J. Clean. Prod. 238:117782. doi: 10.1016/j.jclepro.2019.117782
Maroušek, J. (2013). Use of continuous pressure shockwaves apparatus in rapeseed oil processing. Clean Techn. Environ. Policy 15, 721–725. doi: 10.1007/s10098-012-0549-3
Maroušek, J., Itoh, S., Higa, O., Kondo, Y., Ueno, M., Suwa, R., et al. (2013). Pressure shockwaves to enhance oil extraction from Jatropha curcas L. Biotechnol. Biotechnol. Equip. 27, 3654–3658. doi: 10.5504/BBEQ.2012.0143
Maroušek, J., Maroušková, A., Gavurová, B., and Minofar, B. (2023a). Techno-economic considerations on cement substitute obtained from waste refining. J. Clean. Prod. 412:137326. doi: 10.1016/j.jclepro.2023.137326
Maroušek, J., Maroušková, A., Periakaruppan, R., Gokul, G. M., Anbukumaran, A., Bohatá, A., et al. (2022). Silica nanoparticles from coir pith synthesized by acidic sol-gel method improve germination economics. Polymers 14:266. doi: 10.3390/polym14020266
Maroušek, J., Minofar, B., Maroušková, A., Strunecký, O., and Gavurová, B. (2023b). Environmental and economic advantages of production and application of digestate biochar. Environ. Technol. Innov. 30:103109. doi: 10.1016/j.eti.2023.103109
Maroušek, J., Strunecký, O., and Maroušková, A. (2023c). Insect rearing on biowaste represents a competitive advantage for fish farming. Rev. Aquac. 15, 965–975. doi: 10.1111/raq.12772
Obilor, E. I., and Miwari, G. U. (2022). Content validity in educational assessment. Int. J. Innov. Educ. Res. 10, 57–69.
Ogundari, K., and Bolarinwa, O. D. (2018). Agricultural innovations, production, and household welfare in africa, working paper series N° 294. Abidjan, Côte d’Ivoire: African Development Bank.
Oliveira, M. D., Gomes da Silva, F., Ferreira, S., Teixeira, M., Damásio, H., Dinis Ferreira, A., et al. (2019). Innovations in sustainable agriculture: case study of Lis Valley Irrigation District, Portugal. Sustain. For. 11:331. doi: 10.3390/su11020331
Pavolova, H., Bakalár, T., Kyšeľa, K., Klimek, M., Hajduova, Z., and Zawada, M. (2021). The analysis of investment into industries based on portfolio managers. Acta Montan. Slovaca 26, 161–170. doi: 10.46544/AMS.v26i1.14
Ramayah, T., Soto-Acosta, P., Kheng, K. K., and Mahmud, I. (2020). Developing process and product innovation through internal and external knowledge sources in manufacturing Malaysian firms: the role of absorptive capacity. Bus. Process. Manag. J. 26, 1021–1039. doi: 10.1108/BPMJ-11-2019-0453
Saavedra, D., Rugeles, L., and Guaitero, B. How to ask Colombian farmers for innovation: a methodological approach. In 10th Global Network for the Economics of Learning, Innovation, and Competence Building Systems (GLOBELICS) International Conference, Hangzhou, China, November (2012) (pp. 9–11).
Taques, F. H., López, M. G., Basso, L. F., and Areal, N. (2021). Indicators used to measure service innovation and manufacturing innovation. J. Innov. Knowl. 6, 11–26. doi: 10.1016/j.jik.2019.12.001
van Galen, M. A., and Poppe, K. J. (2013). Innovation monitoring in the Agri-food business is in its infancy. EuroChoices 12, 28–29. doi: 10.1111/1746-692X.12016
Van Rijn, F., Bulte, E., and Adekunle, A. (2012). Social capital and agricultural innovation in sub-Saharan Africa. Agric. Syst. 108, 112–122. doi: 10.1016/j.agsy.2011.12.003
Vochozka, M., Horak, J., Krulický, T., and Pardal, P. (2020). Predicting future Brent oil price on global markets. Acta Montan. Slovaca 25, 375–392. doi: 10.46544/AMS.v25i3.10
Vochozka, M., Rowland, Z., Suler, P., and Marousek, J. (2020). The influence of the international Price of oil on the value of the EUR/USD exchange rate. J. Compet. 12, 167–190. doi: 10.7441/joc.2020.02.10
Webb, P., and Forum, W. E. Innovation with a purpose: The role of technology innovation in accelerating food systems transformation. World Economic Forum: Colony, Switzerland. (2018).
Keywords: content validity index, content validity ratio, product innovation, process innovation, marketing innovation
Citation: Farsani ED, Choobchian S and Naghani MS (2024) Comprehensive identification, localization, and validation of innovation measurement indices at the farm level in Iran. Front. Sustain. Food Syst. 8:1365687. doi: 10.3389/fsufs.2024.1365687
Edited by:
Christian Bux, University of Bari Aldo Moro, ItalyReviewed by:
Janpriy Sharma, University of Trento, ItalyEloy Hontoria, Polytechnic University of Cartagena, Spain
Stanislav Škapa, Brno University of Technology, Czechia
Copyright © 2024 Farsani, Choobchian and Naghani. This is an open-access article distributed under the terms of the Creative Commons Attribution License (CC BY). The use, distribution or reproduction in other forums is permitted, provided the original author(s) and the copyright owner(s) are credited and that the original publication in this journal is cited, in accordance with accepted academic practice. No use, distribution or reproduction is permitted which does not comply with these terms.
*Correspondence: Shahla Choobchian, c2hjaG9vYmNoaWFuQG1vZGFyZXMuYWMuaXI=