- 1College of Management, Sichuan Agricultural University Chengdu, Chengdu, China
- 2Plant Production Department, College of Food and Agriculture Sciences, King Saud University, Riyadh, Saudi Arabia
- 3Department of Agricultural Extension and Rural Society, Faculty of Agriculture, Mansoura University, Mansoura, Egypt
Worldwide, the continuous advancement of off-grid solar photovoltaic irrigation seeks to improve water access, increase food production, and reduce carbon emissions and energy costs associated with fuel usage. Consequently, this enhances human resilience to climate change and contributes to the improvement of farmers’ income. This study investigates the influence of solar energy adoption on farmers’ income, drawing insights from 1,080 growers in Pakistan. It uses the logit model and propensity score matching (PSM) to address bias. Factors influencing income, including gender, education, decision-making autonomy, farm size, extension services, cooperative associations, access to credit, risk perception, market distance, and tube well availability, are identified. Findings reveal a positive correlation between solar energy adoption and increased crop farmer income. PSM analysis validates this, emphasizing the need for government and agricultural extension interventions to enhance financial accessibility for farmers facing mobility challenges. This includes subsidies for technology adoption and knowledge dissemination about digital technology. The study advocates for an accelerated adoption of solar energy to foster agricultural development in Pakistan. In resource-poor nations like Pakistan, government subsidies are crucial to offset technology costs for citizens facing challenges in affording green energy. Addressing Pakistan’s energy crisis through promoting solar energy for irrigation can amplify farmers’ income. It is imperative to promote access to this technology, particularly for water pumping, through subsidies and readily available credit facilities, given the resource limitations and small landholdings of many farmers in Pakistan.
1 Introduction
Globally, approximately 2 billion people face food insecurity, reflecting a discouraging trend in nutrition indicators (Lefore et al., 2021). Unfortunately, strategies aimed at reducing chronic hunger and enhancing livelihoods encounter limitations due to risks associated with climate change and ecological resilience. In historical contexts, irrigation has proven instrumental in significantly boosting food production. Recent studies suggest that implementing irrigation in severely food-insecure areas could pave multiple pathways to improved nutrition. Additionally, access to water for domestic and sanitation purposes is crucial for advancing nutrition and livelihoods (Passarelli et al., 2018). However, the development of water access strategies must carefully consider climate and environmental risk mitigation. The efficacy of water usage in addressing nutritional gaps and reducing poverty is now under scrutiny due to the uncertainties posed by climate change (Balasubramanya and Stifel, 2020). Amid global challenges such as climate change and food security, South Asia, particularly Pakistan, faces a pressing issue deeply intertwined with its agrarian foundation (Rasul, 2021; Khan et al., 2023). The region heavily relies on pump-lift irrigation, predominantly utilizing groundwater for crop irrigation. According to Mukherji’s findings, South Asia stands out as the world’s largest consumer of groundwater, withdrawing a staggering 210 km3 annually. This critical dependence on pumping groundwater underscores the intricate relationship between the energy sector and irrigation, commonly referred to as the “energy-irrigation” nexus (Mukherji and Shah, 2005; Ali and Behera, 2016; Batool et al., 2022).
However, this nexus does not exist in isolation; it forms a complex triad with poverty, creating what is referred to as the “energy-irrigation-poverty” nexus. Understanding the intricacies of this nexus becomes paramount, especially in the agricultural landscape of developing nations like Pakistan, as global challenges loom large. In recent years, Pakistan has confronted severe electricity shortages due to a substantial deficit in power generation and a continuous surge in energy demand across the industrial, agricultural, and domestic sectors. Recognizing energy as the central driver for the nation’s financial advancement, the growing gap between energy demand and capacity significantly hinders Pakistan’s inclusive development. This difference has led to an escalation in electricity prices, creating barriers to affordable and sufficient energy access for a significant proportion of the impoverished population (Asif, 2012). The repercussions extend beyond economic constraints, significantly impacting various sectors, particularly the agricultural domain in Pakistan.
With over 63% of Pakistan’s 180 million-strong population residing in rural areas, where agriculture and related activities form the backbone of livelihoods, the consequences are profound. The agricultural industry makes up around 22.9% of the national GDP, supporting approximately two-thirds of the population. It accounts for 50% of the nation’s exports and engages 37.4% of the workforce (Ali and Behera, 2016; Khan et al., 2020). This noteworthy contribution stems from favorable climatic conditions that empower farmers to cultivate a diverse array of economically viable fruits, crops, nuts, and vegetables of the highest export quality, positioning the sector favourably on a global scale. In Pakistan, the widespread practice of irrigating farmlands with conventional electricity-powered water pumping is crucial for cultivating staple crops like maize, wheat, and rice, ensuring food security and poverty alleviation (Ullah et al., 2023). The country has faced a severe energy crisis, leading to frequent power outages and rising electricity tariffs, particularly impacting farmers relying on pump-lift irrigation. This energy shortage hampers input applications, affecting crop production and national food security. In response, farmers are increasingly seeking reliable alternatives to electrically-driven pumps, with a notable shift towards solar energy technology.
This transition addresses uncertainties in power supply and strategically meets the rising water demand for crop irrigation throughout the harvesting period. It reflects a pragmatic adaptation to the challenges posed by the energy crisis, ensuring a stable energy source for sustaining agricultural activities and contributing to the broader goal of consistent water supply for crop irrigation. The pump sets driven by alternative energy sources offer cost-effective and convenient solutions, providing farmers with greater elasticity and autonomy in watering their crops compared to traditional electrically powered pumps. The escalating costs and scarcity of traditional energy sources, such as diesel and electricity, have created substantial potential for the adoption of renewable energy-utilizing water pumps in Pakistan (Raza et al., 2020). Water energy via solar systems, in particular, is anticipated to provide a fitting solution to supply water for household usage and irrigation requirements, especially in rural regions of Pakistan where lack of grid electricity or insufficient availability.
Globally, concerted efforts are underway, both in Pakistan and elsewhere, to implement the utilization of solar energy pumps for crop irrigation (Kamran, 2018; Raza et al., 2020). The water pumping technologies through the immense efficiency of renewable energy base in Pakistan are underscored by the abundant sunlight of the country, with approximately 300 days of sun per year. Additionally, the prevalence of huge areas in rural villages that have no approach to grid connections, coupled with extensive use of groundwater for irrigation, further accentuates the suitability of renewable energy solutions. The study also delves into the contemporary shift to solar energy, positioning itself at the forefront of sustainable agricultural practices amid Pakistan’s severe energy crisis. The research provides valuable insights into the economic, environmental, and practical dimensions of renewable energy adoption, contributing significantly to discussions on inclusive development and agricultural sector resilience.
However, the widespread implementation of this renewable-energy technology for water pumping hinges on its fiscal feasibility and ecological viability (Ali and Behera, 2016; Terang and Baruah, 2023). Despite the promising prospects, the economic and environmental sustainability of this technology will play a crucial role in determining its large-scale acceptance. In addition to these factors, the dispersion of renewable water-pumping technology in remote areas of Pakistan will be significantly influenced by the intensity of awareness among farmers and various social, economic, and demographical features. The primary objective of the current study is to systematically identify the adoption of solar energy technology and its impact on farmers’ income. By delving into the drivers behind the adoption of specific technology, the research endeavors to contribute valuable insights that can inform policies and practices in the agricultural sector, especially in the context of sustainable and renewable energy usage.
The remainder of the paper is ordered as follows. The methodology and analytical framework are shown in Section 2. Part three delves into the results and discussion, while part four outlines the conclusions.
2 Theoretical framework and method
2.1 Theoretical framework
2.1.1 Adoption decision modeling and affecting issues
The adoption of technology in developing countries is often hindered by challenges such as insufficient financial resources, inefficient marketplaces, inadequate rural infrastructure, and a lack of knowledge (Alshubiri et al., 2019; Zhang et al., 2021). Despite these obstacles, estimates from Asfaw et al. (2012), Aker et al. (2016) suggest that farmers would be inclined to embrace technological advancements if they can enhance their efficiency and net income. In light of these considerations, the study employed the random utility framework proposed by Asfaw et al. (2012) to simulate the adoption of solar energy. The existing investigation assumes that farmers exhibit risk-averse behavior and choose solar energy that optimizes their utility function while considering input costs and other limitations. Let represent the utility derived by farmer from adopting solar energy and represent the utility gained by not accepting solar energy. Under this postulation, is defined as , and it is considered a positive if the utility gained from adopting modern technology exceeds the value obtained from not adopting it (Abdulai and Huffman, 2014; Deng et al., 2019; Martínez-Domínguez and Mora-Rivera, 2020; Twumasi et al., 2021; Zheng H. et al., 2021; Zhu et al., 2021). Moreover, since the actual values of these utility ratings cannot be directly observed, the study represents them in the latent variable model as functional aspects, addressing the challenge of unobservable variables.
The equation represents the relationship, where is a binary variable taking the value of one if is greater than zero (indicating the adoption of solar energy) and zero otherwise (Eq. 1). In this equation, is a vector encompassing household characteristics and technology, represents the parameter vectors to be determined, and is the error term, with following a normal distribution
The vectors represent household characteristics and technological factors, respectively. The parameter vectors need to be evaluated, and is the error term with following a normal distribution
The Eq. 2 “ ” were used to calculate the direct impact of solar energy adoption on the dependent variable, such as farmers’ income. However, this approach may yield inaccurate results since it assumes that external factors have minimal influence on the adoption of solar energy. Furthermore, the non-random nature of the treatment task, stemming from individual self-selection and planning, introduces selection bias issues. This bias arises when unobserved factors are correlated with the standard error in the adopted definition and the error term in the outcome model. In light of this consideration, the ordinary least square approach is likely to produce biased results (Ali and Abdulai, 2010; Zhang et al., 2021).
In earlier research, various econometric models have been employed to address the challenge of selection bias. These models include the instrumental variable, Heckman’s two-stage, propensity score matching (PSM), and differences in differences (DID) approaches. Heckman’s two-stage method operates under the assumption that unobserved factors are typically distributed. However, the instrumental variable approach faces constraints, particularly when attempting to incorporate at least one variable into its selection model, limiting its usefulness for outcome assessment. Additionally, this approach’s effectiveness is contingent upon the functional procedure of the result equation. Another promising method for reliable and unbiased evaluation of selection bias is the DID approach. It is worth noting that the DID approach is specifically tailored for panel data surveys (Ali and Abdulai, 2010; Khan et al., 2021; Li et al., 2021), which regrettably have not been leveraged in earlier investigations. This absence of utilization surpasses the limitations inherent in previous techniques. To address bias in the data, this study adopts the PSM procedure developed by Rosenbaum and Rubin (1983). This approach enhances the comprehensibility of the operational forms and distributional prospects used in describing the outcome equation (Becerril and Abdulai, 2010; Luo and Niu, 2019; Dohmwirth and Liu, 2020).
2.1.2 PSM method estimations
We assessed the outcome variables by comparing cases where farmers received treatment for solar energy adoption with cases where they did not measure the impact. The challenge lies in deciphering the effects of solar energy adoption when considering counterfactual findings. The calculation of the average treatment effect (ATE) in the counterfactual scenario is outlined as follows (Rosenbaum and Rubin, 1983).
Where represent the outcomes of farmers i who have adopted solar energy and farmers i who have not adopted solar energy, respectively. However, placing farmers accurately into treatment or control groups may pose a challenge, leading to potentially inaccurate estimates in the explanation provided by Eq. 3 (Dillon, 2011). Frequently, for each farmer i, only the outcome is deliberate at a given point in time. The variable D represents a dummy variable, which is equal to one for a farmer’s adopter and zero for a farmer’s non-adopter. Therefore, the discovered result is offered as follows:
Assuming the absence of selection bias, this research explores the influence of solar energy adoption on households that have embraced it, focusing on the ATT.
In this context, represents the counterfactual outcome, with denoting the household feature vector. The calculation of the ATT in the formula is imperative, as the absence of counterfactual findings introduces the potential for bias in the computation Eq. 5 (Ngango and Seungjee, 2021; Zhou et al., 2021).
The PSM method facilitates the pairing of solar energy adopters with non-adopters by aligning their distributions across various observed variables (Li et al., 2021). Commonly referred to as the probability of solar energy adoption, PSM is underpinned by two competing concepts. According to the conditional independence hypothesis (Imbens and Wooldridge, 2009), the selected outcome and state variables are independent for a typical range of observable covariates . The second hypothesis, known as the frequent sustenance stipulation, posits that 0 < Pr (D = 1X) < 1. This condition is deemed crucial as it signifies an overlapping requirement, given that adopters and non-adopters are more possible to select comparable covariates.
The examination of PSM for solar energy adoption involves employing the logit method. Subsequently, the treatment and control groups undergo matching using three distinct techniques: radius matching (RM), nearest neighbor matching (NNM), and kernel-based matching (KBM). These algorithms, commonly utilized in cross-sectional datasets, aim to assess the average effect of a treatment. The NNM estimator is employed to match each treated individual with the nearest PSM control individual (Hou et al., 2018; Zheng H. et al., 2021). In the KBM approach, each analyzed individual is matched with the weighted average of every control individual in the shared support part (Becerril and Abdulai, 2010; Hou et al., 2018; Heckman et al., n.d.). Conversely, the RM procedure within the defined PSM range (calipers) matches the treated information to the control observation (Dan et al., 2021).
Ensuring a balanced variable distribution between the treatment and control sets is crucial post-matching. The data supporting the balancing test reveals no latent variances in the variables between these two groups (Dan et al., 2021). For a comprehensive illustration of diagnostic statistics, (Sianesi, 2004) suggests a comparative approach using pseudo-R2 values before and after matching. Pseudo R2 reflects the impact of the independent variable on the likelihood of enrolling in the program. Post-matching, systematically consistent distribution of the crucial variable across adopter and non-adopter groups results in a lower pseudo R2 (Becerril and Abdulai, 2010). The covariate balancing hypothesis posits that the cumulative effect of independent variables is nullified following correlation (Ali and Abdulai, 2010). Nevertheless, the absence of systematic distinctions or noticeable bias in covariate distribution between the two groups does not automatically ensure the absence of hidden bias robustness. A thorough evaluation of the assessed ATA is imperative for bias consideration. Hence, we implemented the boundary strategy to determine the significance of unobserved factors’ influence on outcome variables, assessing whether it could substantially affect the matching technique.
2.2 Study sites and data collection
Balochistan, the largest province in Pakistan spanning 347,190 square kilometers, presents significant economic opportunities in agriculture. The region’s conducive environment for cultivating cash crops suggests a potential thriving agricultural sector (Shami et al., 2016; Abdullah and Ahmed, 2018). However, this potential faces obstacles, with water scarcity and inadequate energy infrastructure ranking among the foremost concerns. A notable 81% of farmers express apprehension about these challenges (Ashraf and Routray, 2013). To illuminate this scenario, an extensive study was conducted in Balochistan between September 2022 and February 2023 (Figure 1). The research involved the distribution of 1,080 questionnaires to crop farmers and direct interactions with growers, employing a multistage random sample technique for data collection. The research delved into the initial adoption of solar energy by farmers, focusing on five districts chosen based on their respective farming production proportions. In the second phase, ten tehsils were thoughtfully selected from the districts to ensure comprehensive coverage. These tehsils became the target for completing the planned questionnaire. Advancing to the third phase, twenty union councils were chosen from the ten tehsils, facilitating a representative sample of the population. In the fourth stage, forty villages were randomly nominated from the twenty union councils, ensuring a diverse range of perspectives. Valuable information was then collected from 1,080 growers residing in these nominated villages. The research questionnaire, designed to be comprehensive, covered various aspects. The primary section focused on collecting socioeconomic and demographic data from the contributors, whereas the subsequent sections were tailored to collect data on solar energy adoption. To ensure accuracy and clarity, detailed interviews were conducted due to the questionnaire’s complexity. A pre-testing phase was implemented to address uncertainties and refine the questionnaire, covering a wide range of information on growers’ socioeconomic aspects, solar energy adoption, and other relevant study variables. The collected data underwent meticulous editing and coding using Stata 14 to guarantee accuracy, authenticity, homogeneity, coherence, and completeness.
3 Empirical results and discussion
3.1 Main variables and their descriptions
The data presented in Table 1 provides insights into key variables. Notably, 43% of respondents in the study district accepted solar energy, with a dominant 71% being male among the sampled household heads. Respondents had an average age of about 49 years and an educational attainment of around 4 years. These statistics shed light on demographic patterns and adoption trends in the surveyed population, enriching our analytical perspective. The average family size is 6 individuals, and each family typically owns 3.21 tropical livestock units. Tube well ownership is limited to 31% of farmers, while the remaining 69% depend on borrowing water for irrigation, indicating a thriving groundwater market in Pakistan. Load-shedding statistics reveal daily outages exceeding 11 h in rural areas, severely impeding the use of modern inputs and negatively impacting agricultural areas. The study estimates that 48 growers are living in poverty. Exploring energy sources, electricity emerges as the preferred choice for most farmers, followed by solar energy. On average, each household cultivates 1.8 hectares of arable land, and the annual farmers’ income was 65,840 PKR. This comprehensive information offers valuable insights into the intricate dynamics of solar energy adoption, water management, and socio-economic conditions within the surveyed population.
3.2 Variations in household characteristics by an adoption group
Based on the insights gleaned from Table 2, there are notable distinctions in socioeconomic and farm-level characteristics between adopter growers and non-adopter producers. Specifically, the average income of wheat farmers who have accepted solar energy (105.786 PKR) surpasses that of non-adopters (78.564 PKR). Furthermore, those who have embraced solar energy exhibit significant differences in asset ownership, possessing larger quantities of both cattle and land. These outcomes underscore that, when considering demographic aspects such as age, gender, education level, and family size, the average solar energy adoption among growers is higher compared to non-adopters. Additionally, a noteworthy difference exists in the proportion of male-headed households between solar energy adopters and non-adopters. Table 2 reveals that solar energy adopters are more likely to have access to agricultural extension services, weather prediction data, credit facilities, and reliable data sources.
Crucially, farmers utilizing solar energy display a greater awareness of new technologies and a higher propensity to experiment with them compared to their non-adopting counterparts. Regarding growers’ understanding of subsidy strategies, there is no statistically significant difference between adopters and non-adopters. Another notable distinction among adopters lies in their affiliation with farmers’ organizations, with a higher proportion of adopters being members of growers’ cooperatives. According to these findings, younger growers are more likely to accept renewable energy technology for land irrigation, while experienced growers tend to adhere to conventional and non-renewable energy machinery. This divergence may be attributed to the lesser familiarity of elderly farmers with modern, renewable energy technologies.
3.3 Determinants of solar energy
Table 3 presents an analysis of the potential outcomes of a guestimate logit model evaluating factors influencing the adoption of solar energy. The results indicate that various factors significantly impact farmers’ income and the likelihood of farmers embracing solar energy. The gender-specific coefficient for solar energy is positively significant, indicating that male wheat producers exhibit a greater inclination than their female counterparts toward adopting innovative energy sources, such as solar energy. For both biogas and solar technology, the coefficients associated with access to credit are positive and substantial. This suggests that access to financial resources plays a crucial role in encouraging the adoption of energy-efficient water pumps in Pakistan. In the context of solar energy adoption in China, our findings align with those of earlier lessons (Yang et al., 2021). This underscores the empowering effect of education on farmers, enabling them to consider and implement modern technologies. Our research in specific areas indicates a higher likelihood of solar energy usage among male farmers compared to their female counterparts (Zhu et al., 2021). This observation resonates with the findings of (Nahayo et al., 2017; Zheng X. et al., 2021), highlighting that female farmers are less likely to embrace contemporary farming techniques due to limited access and control over assets. Furthermore, the size of land holdings and the impact of climate change emerge as significant factors influencing the likelihood of adopting solar-powered machinery. These results donate to a complete aware of the multifaceted determinants of solar energy adoption in agricultural contexts.
Consistent with a previous study, we observed a higher propensity for members of farmers’ cooperatives to adopt solar energy compared to non-members. This underscores the pivotal role of social capital in expediting the acceptance of technology. Our findings indicate that households receiving regular visits from agricultural extension specialists are more inclined to adopt modern solar technologies than those without such visits. The rationale behind Agri-extension networks lies in their ability to assist farmers in acquiring essential knowledge and pertinent data related to farming production (Dan et al., 2021). Access to financing is also deemed crucial in increasing the likelihood of solar energy adoption, aligning with the findings of Kim et al. (2021), who emphasized the role of credit in helping producers amass sufficient funds for contemporary technology. The results presented in Table 3 highlight that access to weather prediction information significantly enhances the likelihood of solar energy adoption. Meteorological data availability empowers producers to make more informed decisions in their agricultural activities. The swift adoption of agricultural technology in Nigeria lends support to this conclusion (Wossen et al., 2017). Furthermore, the favorable and significant climate risk coefficient suggests that farmers motivated to explore technological advancements have a higher likelihood of success. This outcome corroborates the findings of Koundouri et al. (2006) regarding the utilization of contemporary technologies in Greece. Frisk-averse farmers may address productivity uncertainties and risks by embracing modern technologies. In contrast, (Mariano et al., 2012) in the Philippines noted that risk-averse and profit-oriented growers are frequently attracted to contemporary high-yield products and farmers’ income.
3.4 Impact of solar energy adoption on farmers income
To assess the influence of solar energy adoption on farmers’ income, we employed the propensity score matching approach, as detailed in the methodology section. The propensity score matching technique ensures an equitable distribution of independent variables between solar energy adopter growers and non-adopter growers. Figure 2 illustrates the extent of the application of the propensity score matching method and the areas where both groups received support. Consistent with the hypothesis proposed by Caliendo and Kopeinig (2008), the density distribution of adopter growers and non-adopter growers, as determined through this approach, aligns with typical support scenarios. To ensure a proper match of observable household attributes between adopter growers and non-adopter growers, twenty-seven treated cases that were identified as unsupported were eliminated post-analysis. This step is crucial for maintaining the integrity of the comparison between the two groups.
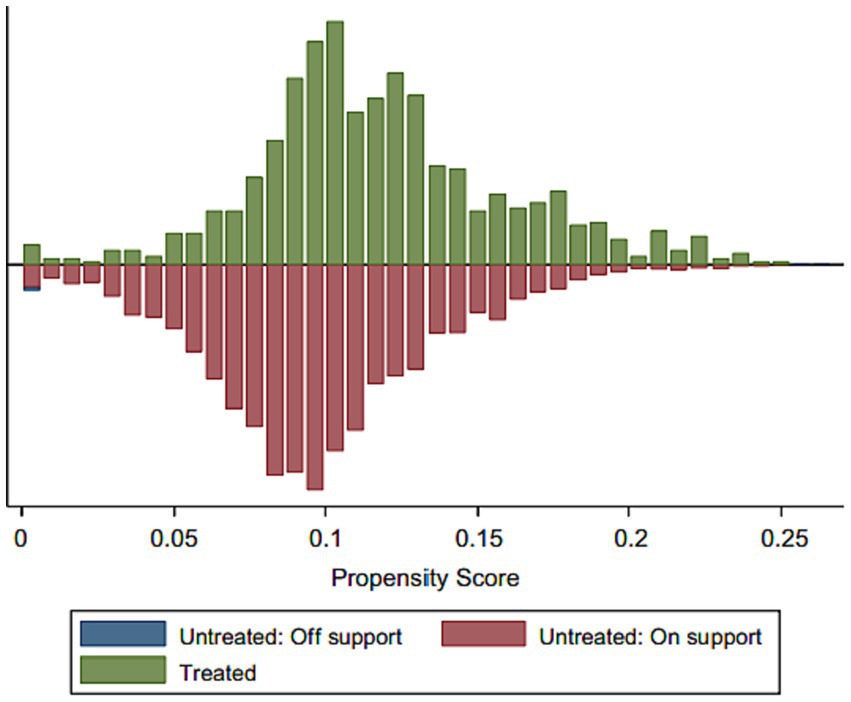
Figure 2. PSM is employed to estimate the comparability between treated and untreated groups, showcasing the robustness of the obtained results.
Table 4 provides an in-depth analysis of the ATT, illustrating the influence of adopting solar energy on farmers’ income. Three commonly used propensity score matching algorithms KBM, RNM, and RM are employed to evaluate the ATT value. The outcomes from these algorithms consistently reveal a significant impact of solar energy adoption on farmers’ income. Specifically, the adoption of solar energy is associated with an average income increase of approximately 193.38 (using KBM), 199.79 (with RNM), and 197.14 (using RM). On average, farmers adopting solar energy generate 193.38 to 199.79 more income per hectare of land compared to non-adopters. This finding is in line with Kim et al. (2021), emphasizing that the adoption of technologies significantly enhances farmers’ income.

Table 4. Propensity score matching results of sensitivity analysis and solar energy adoption impact on farmers’ income.
In Section 3, an examination of sensitivity and a test for balancing covariates were imperative to assess the strength and effectiveness of the matching procedure’s results. The outcomes, presented in Table 5, confirm the precision of the matching, revealing a significant decrease in deviance through the employed strategy. Specifically, there was a substantial reduction in bias, decreasing from 64.32% before matching to a range of 10–14% post-matching, indicating a consistent 80–87% overall reduction in bias. Furthermore, the post-matching R2 values for all techniques consistently decreased compared to pre-matching, evident in the difference between the pseudo-R2 values in the second and third columns. After the matching process, there is limited evidence suggesting a consistent change in the distribution of independent factors between adopter and non-adopter groups. Additionally, the elevated p-value from the likelihood ratio test post-matching dismisses the idea that the collective significance of descriptive variables differs between the two groups. Overall, both adopter and non-adopter farmers exhibit minimal variations in covariate distribution after matching, aligning with the findings of previous studies utilizing comprehensive covariate matching assessments. Consequently, we can assert that the proposed design of the propensity score matching analysis method effectively balances the characteristics of adopters and non-adopters.
We delved deeper into hidden biases arising from unobservable factors using the Rosenbaum boundary sensitivity examination approach. The critical results regarding hidden bias for the three matching approaches are detailed across seven columns in Table 4. The outcomes from RM and KBM suggest that, at a 5% significance level, the measured hidden bias value was 3.75. This finding indicates that solar energy adoption is not responsive to unobserved variations, significantly enhancing the reliability of using solar energy to predict the average treatment impact of growers’ profits. Furthermore, the study’s outcomes exhibit resilience against concealed bias and align with the conditional fairness assumption inherent in the propensity score matching technique.
4 Conclusion
In modern agricultural practices, the study reveals a significant interconnection between energy and irrigation, with groundwater abstraction being the primary source. This relationship plays a pivotal role in contributing to agricultural yields, farm revenue, and rural employment. However, the adverse effects of Pakistan’s energy challenges on the agricultural sector are evident, prompting farmers reliant on groundwater to explore alternative energy sources for pumping. Drawing on data from 1,080 growers and utilizing the logit model, the study identifies key factors influencing solar energy adoption. Education, gender, agricultural extension services, farm size, cooperative membership, risk and credit awareness, and tube well ownership are highlighted as crucial determinants of adoption decisions. The subsequent PSM analysis validates that the adoption of solar energy significantly enhances income, emphasizing its potential impact on the economic well-being of crop farmers.
5 Policy implications
Given the identified factors influencing solar energy adoption and its positive impact on income, policy implications emerge. Government and agricultural extension interventions are deemed necessary to enhance financial accessibility for growers facing mobility challenges. Subsidies for technology adoption and knowledge dissemination about digital technology are recommended to encourage solar energy adoption among farmers. Effective extension services and support for farmer cooperatives are highlighted as key strategies to address barriers to adoption. The study underscores the strategic importance of raising farmers’ education levels to bolster solar energy adoption. The policy recommendations advocate for urgent implementation of regulations ensuring universal access to digitalization and a strategic increase in adoption through public-private collaboration, carrying significant implications for Pakistan’s agricultural landscape.
6 Limitations and future research directions
Despite the potential benefits, the study acknowledges certain limitations. The affordability of renewable energy-powered water pumps poses a hurdle, particularly for marginal and small-scale farmers, necessitating targeted financial support from the government. In suggesting future research directions, the study identifies potential avenues for further investigation. This includes broadening the scope beyond solar energy adoption to explore additional antecedents such as eco-friendliness concerns and financial sanctions. The examination of variables like dedication and participation concerning farm household characteristics and green innovation willingness represents a promising research direction. The study emphasizes the importance of expanding the sample size to validate findings and conducting analogous studies in diverse emerging countries to enhance result generalizability. Furthermore, the suggestion to diversify data collection methods, including semi-structured questionnaires and online interview techniques, is made to provide deeper insights into the intricate dynamics of solar energy technology adoption and its implications.
Data availability statement
The raw data supporting the conclusions of this article will be made available by the authors, without undue reservation.
Author contributions
NK: Writing – original draft, Writing – review & editing, Conceptualization, Data curation, Formal analysis, Funding acquisition, Investigation, Methodology, Project administration, Resources, Software, Supervision, Validation, Visualization. RK: Writing – review & editing. SZ: Conceptualization, Data curation, Formal analysis, Funding acquisition, Investigation, Methodology, Project administration, Resources, Software, Supervision, Validation, Visualization, Writing – original draft, Writing – review & editing. KE: Writing – review & editing, Funding acquisition, Investigation, Project administration. HK: Writing – review & editing, Methodology.
Funding
The author(s) declare financial support was received for the research, authorship, and/or publication of this article. This research was supported by the researchers supporting project number (RSPD2024R952), King Saud University, Riyadh, Saudi Arabia.
Acknowledgments
We sincerely appreciate HK for dedicating his valuable time and expertise to meticulously edit and refine the English language of this paper, significantly contributing to its overall quality.
Conflict of interest
The authors declare that the research was conducted in the absence of any commercial or financial relationships that could be construed as a potential conflict of interest.
Publisher’s note
All claims expressed in this article are solely those of the authors and do not necessarily represent those of their affiliated organizations, or those of the publisher, the editors and the reviewers. Any product that may be evaluated in this article, or claim that may be made by its manufacturer, is not guaranteed or endorsed by the publisher.
References
Abdulai, A., and Huffman, W. (2014). The adoption and impact of soil and water conservation technology: an endogenous switching regression application. Land Econ. 90, 26–43. doi: 10.3368/le.90.1.26
Abdullah, R., and Ahmed, N. (2018). Political status of Balochistan from 1971 to 1988. Pakistan Study Centre 8, 325–334.
Aker, J. C., Ghosh, I., and Burrell, J. (2016). The promise (and pitfalls) of ICT for agriculture initiatives. Agric. Econ. 47, 35–48. doi: 10.1111/agec.12301
Ali, A., and Abdulai, A. (2010). The adoption of genetically modified cotton and poverty reduction in Pakistan. J. Agric. Econ. 61, 175–192. doi: 10.1111/j.1477-9552.2009.00227.x
Ali, A., and Behera, B. (2016). Factors influencing farmers’ adoption of energy-based water pumps and impacts on crop productivity and household income in Pakistan. Renew. Sust. Energ. Rev. 54, 48–57. doi: 10.1016/j.rser.2015.09.073
Alshubiri, F., Jamil, S. A., and Elheddad, M. (2019). The impact of ICT on financial development: empirical evidence from the Gulf cooperation council countries. Int. J. Eng. Business Manag. 11:184797901987067. doi: 10.1177/1847979019870670
Asfaw, S., Shiferaw, B., Simtowe, F., and Lipper, L. (2012). Impact of modern agricultural technologies on smallholder welfare: evidence from Tanzania and Ethiopia. Food Policy 37, 283–295. doi: 10.1016/j.foodpol.2012.02.013
Ashraf, M., and Routray, J. K. (2013). Perception and understanding of drought and coping strategies of farming households in north-West Balochistan. Int. J. Disaster Risk Reduct. 5, 49–60. doi: 10.1016/j.ijdrr.2013.05.002
Asif, M. Energy crisis in Pakistan: origins, challenges, and sustainable solutions. OUP Catalogue. (2012)
Balasubramanya, S., and Stifel, D. (2020). Water, agriculture & poverty in an era of climate change: why do we know so little? Food Pol. 93:101905. doi: 10.1016/j.foodpol.2020.101905
Batool, K., Zhao, Z., Atif, F., and Dilanchiev, A. (2022). Nexus between energy poverty and technological innovations: a pathway for addressing energy sustainability. Front. Environ. Sci. 10:888080. doi: 10.3389/fenvs.2022.888080
Becerril, J., and Abdulai, A. (2010). The impact of improved maize varieties on poverty in Mexico: a propensity score-matching approach. World Dev. 38, 1024–1035. doi: 10.1016/j.worlddev.2009.11.017
Caliendo, M., and Kopeinig, S. (2008). Some practical guidance for the implementation of propensity score matching, vol. 22, 31–72.
Dan, Y., Zhang, H.-W., LIU, Z.-M., and Qiao, Z. (2021). Do cooperatives participation and technology adoption improve farmers' welfare in China? A joint analysis accounting for selection bias 20, 1716–1726.
Deng, X., Xu, D., Zeng, M., and Qi, Y. (2019). Does internet use help reduce rural cropland abandonment? Evidence from China. Land Use Policy 89:104243. doi: 10.1016/j.landusepol.2019.104243
Dillon, A. (2011). Do differences in the scale of irrigation projects generate different impacts on poverty and production? J. Agric. Econ. 62, 474–492. doi: 10.1111/j.1477-9552.2010.00276.x
Dohmwirth, C., and Liu, Z. Does cooperative membership matter for women’s empowerment?, Evidence from South Indian dairy producers. (2020). 12. 133–150
Heckman, J. J., Ichimura, H., and Todd, P. E. (1997) Matching as an econometric evaluation estimator: evidence from evaluating The Review of Economic Studies, 64, 605–654. doi: 10.2307/2971733
Hou, J., Huo, X., and Yin, R. (2018). Does computer usage change farmers’ production and consumption? Evid. China 11, 387–410. doi: 10.1108/CAER-09-2016-0149
Imbens, G. W., and Wooldridge, J. M. (2009). Recent developments in the econometrics of program evaluation. J. Econ. Lit. 47, 5–86. doi: 10.1257/jel.47.1.5
Kamran, M. (2018). Current status and future success of renewable energy in Pakistan. Renew. Sust. Energ. Rev. 82, 609–617. doi: 10.1016/j.rser.2017.09.049
Khan, A., Khwaja, A., and Jawed, A. Navigating civic spaces during a pandemic. Pakistan report. (2020)
Khan, N., Ma, J., Zhang, H., and Zhang, S. (2023). Climate Change impact on sustainable agricultural growth: insights from rural areas. Atmos 14:1194. doi: 10.3390/atmos14081194
Khan, N., Ray, R. L., Kassem, H. S., Ihtisham, M., Asongu, S. A., Ansah, S., et al. (2021). Toward cleaner production: can mobile phone technology help reduce inorganic fertilizer application? Evid. Natl. Level Dataset. 10:1023. doi: 10.3390/land10101023
Kim, J., Park, J. C., and Komarek, T. (2021). The impact of Mobile ICT on national productivity in developed and developing countries. Inf. Manag. 58:103442. doi: 10.1016/j.im.2021.103442
Koundouri, P., Nauges, C., and Tzouvelekas, V. (2006). Technology adoption under production uncertainty: theory and application to irrigation technology, vol. 88, 657–670.
Lefore, N., Closas, A., and Schmitter, P. (2021). Solar for all: a framework to deliver inclusive and environmentally sustainable solar irrigation for smallholder agriculture. Energy Pol. 154:112313. doi: 10.1016/j.enpol.2021.112313
Li, X., Guo, H., Jin, S., Ma, W., and Zeng, Y. (2021). Do farmers gain internet dividends from E-commerce adoption? Evidence from China. Food Pol. 101:102024. doi: 10.1016/j.foodpol.2021.102024
Luo, X., and Niu, C. E-commerce participation and household income growth in Taobao villages. (2019)
Mariano, M. J., Villano, R., and Fleming, E. (2012). Factors influencing farmers’ adoption of modern rice technologies and good management practices in the Philippines, vol. 110, 41–53.
Martínez-Domínguez, M., and Mora-Rivera, J. (2020). Internet adoption and usage patterns in rural Mexico. Technol. Soc. 60:101226. doi: 10.1016/j.techsoc.2019.101226
Mukherji, A., and Shah, T. (2005). Groundwater socio-ecology and governance: a review of institutions and policies in selected countries. Hydrogeol. J. 13, 328–345. doi: 10.1007/s10040-005-0434-9
Nahayo, A., Omondi, M. O., Zhang, X.-H., LI, L.-Q., PAN, G.-X., and Joseph, S. (2017). Factors influencing farmers’ participation in crop intensification program in Rwanda, vol. 16, 1406–1416.
Ngango, J., and Seungjee, H. (2021). Adoption of small-scale irrigation technologies and its impact on land productivity: evidence from Rwanda. J. Integr. Agric. 20, 2302–2312. doi: 10.1016/S2095-3119(20)63417-7
Passarelli, S., Mekonnen, D., Bryan, E., and Ringler, C. (2018). Evaluating the pathways from small-scale irrigation to dietary diversity: evidence from Ethiopia and Tanzania. Food Secur. 10, 981–997. doi: 10.1007/s12571-018-0812-5
Rasul, G. (2021). A framework for addressing the twin challenges of COVID-19 and climate change for sustainable agriculture and food security in South Asia. Front. Sustainable Food Syst. 5:679037. doi: 10.3389/fsufs.2021.679037
Raza, M. Y., Wasim, M., and Sarwar, M. S. (2020). Development of renewable energy technologies in rural areas of Pakistan. Energy Sources 42, 740–760. doi: 10.1080/15567036.2019.1588428
Rosenbaum, P. R., and Rubin, D. B. (1983). The central role of the propensity score in observational studies for causal effects, vol. 70, 41–55.
Shami, S. H., Ahmad, J., Zafar, R., Haris, M., and Bashir, S. (2016). Evaluating wind energy potential in Pakistan’s three provinces, with proposal for integration into national power grid. Renew. Sust. Energ. Rev. 53, 408–421. doi: 10.1016/j.rser.2015.08.052
Sianesi, B. (2004). Statistics. An evaluation of the Swedish system of active labor market programs in the 1990s, vol. 86, 133–155.
Terang, B., and Baruah, D. C. (2023). Techno-economic and environmental assessment of solar photovoltaic, diesel, and electric water pumps for irrigation in Assam. India. Energy Pol. 183:113807. doi: 10.1016/j.enpol.2023.113807
Twumasi, M. A., Jiang, Y., Zhou, X., Addai, B., Darfor, K. N., Akaba, S., et al. (2021). Increasing Ghanaian fish farms’ productivity: does the use of the internet matter? Mar. Policy 125:104385. doi: 10.1016/j.marpol.2020.104385
Ullah, I., Khan, N., Dai, Y., and Hamza, A. (2023). Does solar-powered irrigation system usage increase the technical efficiency of crop production? New insights from rural areas. Energies 16:6641. doi: 10.3390/en16186641
Wossen, T., Abdoulaye, T., Alene, A., Feleke, S., Ricker-Gilbert, J., Manyong, V., et al. (2017). Productivity and welfare effects of Nigeria’s e-voucher-based input subsidy program, vol. 97, 251–265.
Yang, L., Lu, H., Wang, S., and Li, M. (2021). Mobile internet use and multidimensional poverty: evidence from a household survey in rural China, vol. 158, 1065–1086.
Zhang, F., Sarkar, A., and Wang, H. (2021). Does internet and information technology help farmers to maximize profit: a cross-sectional study of apple farmers in Shandong, China. Land 10:390. doi: 10.3390/land10040390
Zheng, X., Lu, H., and Change, S. (2021). Does ICT change household decision-making power of the left-behind women? A Case from China. Technol. Forecast. Soc. Change 166:120604. doi: 10.1016/j.techfore.2021.120604
Zheng, H., Ma, W., Wang, F., and Li, G. (2021). Does internet use improve technical efficiency of banana production in China? Evidence from a selectivity-corrected analysis. Food Pol. 102:102044. doi: 10.1016/j.foodpol.2021.102044
Zhou, J., Lan, H., Zhao, C., and Zhou, J. (2021). Haze pollution levels, spatial spillover influence, and impacts of the digital economy: empirical evidence from China. Sustain. For. 13:9076. doi: 10.3390/su13169076
Zhu, X., Hu, R., Zhang, C., and Shi, G. (2021). Does internet use improve technical efficiency? Evidence from apple production in China. Technol. Forecast. Soc. Chang. 166:120662. doi: 10.1016/j.techfore.2021.120662
Keywords: solar energy, adoption, logit model, crop farmers, income, Pakistan
Citation: Khan N, Elhindi KM, Kassem HS, Kazim R and Zhang S (2024) Unveiling the nexus between solar energy adoption and crop farmer income: evidence from Pakistan. Front. Sustain. Food Syst. 8:1364040. doi: 10.3389/fsufs.2024.1364040
Edited by:
Isabelle Piot-Lepetit, INRAE Occitanie Montpellier, FranceReviewed by:
Mohammad Chhiddikur Rahman, Bangladesh Rice Research Institute, BangladeshMartinson Ankrah Twumasi, Sichuan Agricultural University, China
Copyright © 2024 Khan, Elhindi, Kassem, Kazim and Zhang. This is an open-access article distributed under the terms of the Creative Commons Attribution License (CC BY). The use, distribution or reproduction in other forums is permitted, provided the original author(s) and the copyright owner(s) are credited and that the original publication in this journal is cited, in accordance with accepted academic practice. No use, distribution or reproduction is permitted which does not comply with these terms.
*Correspondence: Shemei Zhang, emhhbmdzaGVtZWlAMTYzLmNvbQ==