- 1New Zealand Life Cycle Management (NZLCM) Centre, Massey University, Palmerston North, New Zealand
- 2School of Exercise and Nutrition Sciences, Faculty of Health, and Centre for Agriculture and the Bioeconomy, Queensland University of Technology, Brisbane, QLD, Australia
- 3The New Zealand Institute for Plant and Food Research Ltd, Lincoln, New Zealand
Introduction: This study investigates the development and potential application of a nutritional Life Cycle Assessment (nLCA) method to rank meals, using a case study of a “toppings on toast” (ToTs) meal. Methodological issues are investigated in the context of application to support consumers to make more informed food choices at the meal level.
Methods: Fourteen selected “toppings on toast” (ToTs) commonly consumed in New Zealand (NZ) were evaluated for their climate change impacts and nutritional value using the serve size of each topping as the functional unit (FU). NZ-specific climate change values were obtained from an existing database and recent literature. Nutritional value was calculated using the NRF family of indices – specifically the NRF9.3 and NRF28.3 indices (the latter constructed for this study to include all nutrients in the selected toppings for which reference values were available) and presented in a separate midpoint nutrition impact category. The NRF and climate change scores were assigned quartile-based weights, and the weight of each index score was averaged with that of the climate change score. Based on these average values, the toppings were ranked in two ranking sets (one for each index). In a sensitivity analysis, two alternative reference units were also used (100 g and 100 kcal) to investigate how different FUs influenced the final rankings.
Results: The results showed that use of one or other NRF index affected the magnitude of the nLCA results; however, the rankings of the ToTs based on the nLCA results did not change much between the two indices. Avocado and peanut butter performed the best (top two ranks), and bacon, butter, and cheese were the poorest performers (bottom two ranks), for both the ranking sets. The toppings which did change ranks mostly moved up or down by only one position. Thus, the results of this case study suggest that the NRF9.3 index is sufficient to determine overall the best, medium, and worst performing toppings in the ToT meal context. However, the results also showed that water-soluble vitamins and unsaturated fats included in the NRF28.3 index contributed significantly to the nutritional scores for most of the toppings and were instrumental in the rank changes for the toppings which are particularly rich in these nutrients.
Discussion: Thus, for a more diverse range of toppings/meals, an expanded index including these nutrients can generate more nuanced rankings. This study contributes to the nascent but fast-growing nLCA research field, particularly within the meal context. The method used in this case study could be applied in food composition databases, restaurant menus, and websites/apps that provides recipes for meals. However, the study also highlighted the potentially significant variability in climate change and nutritional values in the toppings associated with different production practices, seasonality, and different varieties of the same product. Any future development of nLCA-based meal level rankings should address this variability and communicate it to the consumer.
1 Introduction
Agri-food systems have far-reaching environmental impacts, including significant contributions to climate change, biodiversity loss, freshwater use and pollution, and soil degradation (Vermeulen et al., 2012; Mekonnen and Hoekstra, 2014; Dudley and Alexander, 2017; Springmann et al., 2018a; Intergovernmental Science-Policy Platform on Biodiversity and Ecosystem Services (IPBES), 2019; Food and Agriculture Organization of the United Nations (FAO), 2020; Crippa et al., 2021). At the same time, much of the world’s population has nutrient-poor diets low in fresh fruits, vegetables, grains and legumes, and overconsumption of foods that increase risk of chronic disease, e.g., processed and ultra-processed foods high in sodium, saturated/trans fats, and added sugar (Springmann et al., 2018b; Clark et al., 2019; Global Nutrition Report, 2021). There are 690 million undernourished people and 11 million deaths related to poor diets around the world annually (Global Panel on Agriculture and Food Systems for Nutrition, 2020). With a global population set to peak at almost 10 billion by 2050 (Gu et al., 2021), this “diet-environment-health trilemma” of global food systems (Clark et al., 2018; Hawkins, 2019) requires urgent attention, and has led to calls for a transition to more sustainable food systems (Food and Agriculture Organization of the United Nations (FAO) and World Health Organization (WHO), 2019; Willett et al., 2019; Global Panel on Agriculture and Food Systems for Nutrition, 2020; United Nations Framework Convention on Climate Change (UNFCCC), 2023b). From a demand constraint perspective, the focus has been on changes in consumption patterns and dietary shifts (Heller et al., 2013; Meier and Christen, 2013; Hallström et al., 2015; Notarnicola et al., 2017; Willett et al., 2019). However, at the same time there are concerns about the nutritional inadequacy of some diets (vegan, vegetarian etc.) that have been widely acknowledged as being good for planetary health (Graham et al., 2019; Mazac et al., 2023). Consumers can be supported in their choices for healthy and sustainable foods by having access to easily comprehensible information about a food product’s environmental and nutritional credentials in the form of indicators and other metrics (Notarnicola et al., 2015, 2017).
Environmental Life Cycle Assessment (LCA) has been widely used for assessment of the environmental impacts of production and/or packaging, distribution, and consumption of various agri-food products (Schaubroeck et al., 2018; Clark et al., 2022), meals/menus (Calderón et al., 2018; Takacs et al., 2022) and diets (Hallström et al., 2015; Eme et al., 2019; Henriksson et al., 2021). It is recognized as one of the most informative and holistic methods to evaluate environmental impacts associated with agri-food systems (Sala et al., 2017; McAuliffe et al., 2018). The unit of analysis in an LCA is the functional unit (FU), representing the function or service provided by a product system. Food LCAs have traditionally mostly used mass- or volume-based FUs, and do not account for nutritional value (Saarinen et al., 2017; Sala et al., 2017; Sonesson et al., 2017). Yet one of the most critical functions of food is to provide nutrition to support healthy growth, development, and longevity (Willett et al., 2019; World Health Organization (WHO), 2023). This has led to development of a nascent field of study on nutritional LCA (nLCA), defined as an LCA study in which nutrition is considered the main, or one of the main, functions of food (McLaren et al., 2021).
Several nLCA studies assess the quantity (or quality-corrected quantity) of selected individual nutrients in food items and diets, including phenols, protein, fat, calcium, and energy (e.g., Martínez-Blanco et al., 2011; Oonincx and de Boer, 2012; Saarinen et al., 2017; McAuliffe et al., 2018; Berardy et al., 2019; Salazar et al., 2019). However, foods are a complex mix of many macro- and micro-nutrients that are essential for the proper functioning of the human body. Nutritional Profiling (NP) can be used to assess the nutritional value of foods more comprehensively (Drewnowski et al., 2019, 2021). In this approach, the nutritional value is expressed in the form of indices featuring nutrients to encourage, nutrients to limit, and/or a combination of both.
One particular set of indices, the Nutrient Rich Food (NRF) family of indices proposed by Drewnowski (2009) has been comprehensively tested and validated and is increasingly used in nLCA studies (see for example, Van Kernebeek et al., 2014; Doran-Browne et al., 2015; Esteve-Llorens et al., 2019; Hallström et al., 2019; Bianchi et al., 2020; Green et al., 2020, 2021; Ridoutt, 2021; Strid et al., 2021; Aceves-Martins et al., 2022; Mazac et al., 2023). An NRF index is comprised of two indices; one represents nutrients to encourage (NRn) and the other represents nutrients to avoid or limit (LIM).1 An NRF index is commonly calculated as the difference between the NRn and LIM indices. This NRF family of indices can include a variable number of nutrients and can be calculated using nutrient reference values for specific population groups, thus it is easily adapted to suit the requirements of a particular nLCA study. Several methodological choices have to be made when choosing to use an NRF (or other NP) index. These include the number of nutrients to assess, whether a specific index is chosen for each food group or whether one index is used for all food groups considered in the analysis, the reference amount to be used (e.g., mass-, energy-, or serve size-based), capping/weighting the nutrients, and energy standardization (Scarborough et al., 2010; Masset et al., 2015; Drewnowski, 2017; Saarinen et al., 2017; Hallström et al., 2019; Bianchi et al., 2020; Green et al., 2021, 2023; Strid et al., 2021; Kyttä et al., 2023). Green et al. (2020) note that methodological choices with respect to these variables can significantly alter the assessment of nutritional value of a particular food. However, there is no formal consensus on how to choose the best index-based metric to summarize this nutritional value. Moreover, case studies investigating the influence of these methodological choices in the context of nLCA studies are currently limited (see, for example, Bianchi et al., 2020).
Regarding the selection of nutrients, an index with a limited number of nutrients is sometimes considered most appropriate for nutrient profiling (Fulgoni et al., 2009; Drewnowski, 2017; Green et al., 2020; Weidema and Stylianou, 2020). However, selection of nutrients for nutritional index development should be based on sound justification (Hallström et al., 2019; Bianchi et al., 2020; Ridoutt, 2021; Strid et al., 2021) due to the risk of excluding nutrients that may have an important role to play in human health. The NRF9.3 index, comprising nine nutrients to encourage (protein, fiber, calcium, iron, magnesium, potassium, and vitamins A, C, and E) and three nutrients to limit (added sugar, sodium, and saturated fats), is the most widely used and validated NRF index to date (Van Kernebeek et al., 2014; Doran-Browne et al., 2015; Fernández-Ríos et al., 2021). However, the NRF9.3 index does not reflect the full nutritional value of a food, and this is particularly relevant for food items with specific characteristics (Kägi et al., 2012; Bianchi et al., 2020). For example, seafoods such as tuna and salmon are a rich source of Polyunsaturated Fats (PUFAs) (Coelho et al., 2016; Salazar et al., 2019), avocados are a good source of Monounsaturated Fats (MUFAs) (Guan et al., 2022), mushrooms are rich in selenium (Falandysz, 2008), and chicken is a good source of selenium and B vitamins like niacin and vitamin B6 (New Zealand Food Composition Database (NZFCD), 2022). However, none of these nutrients are included in the NRF9.3 index. In such cases, Bianchi et al. (2020) suggest that an index with a more comprehensive selection of nutrients to encourage, and/or one which is tailored to represent the nutritional profile of the foods being studied, may be more appropriate. For example, Hallström et al. (2019) and Saarinen et al. (2017) developed expanded indices to include all nutrients relevant to the food group being studied. Likewise, Vieux et al. (2013) included all nutrients they considered “key” for diet-related assessments. Another approach is to include all nutrients with formally available Daily Recommended Intake (DRI) and nutrient composition values (Fern et al., 2015; Green et al., 2021, 2023; Ridoutt, 2021).
Combined nutritional and environmental LCA studies have mostly focused on individual food items or diets; there have been relatively fewer combined studies at the meal level. Of these, several studies considered meals based on, or aligned to dietary guidelines, nutritional recommendations (e.g., configuring meals to the Lunch Plate model), or national certification standards for “healthy meals” (Virtanen et al., 2011; Saarinen et al., 2012; García-Herrero et al., 2019; Sameshima et al., 2023). Some studies assumed that the meals being considered are nutritionally adequate (Takacs et al., 2022), while others scaled the meals to have comparable nutritional and/or caloric values, or to similar quantities of foods (Davis et al., 2010; Virtanen et al., 2011; Ernstoff et al., 2019; Sameshima et al., 2023). Thus, these studies did not compare meals based on their individual calculated nutritional value; rather, the latter was kept constant, and the environmental impacts of these nutritionally comparable meals were assessed.
A review of the literature enabled the identification of 11 meal-level studies relevant to this research paper, i.e., meal-level nLCA studies as well as meal-level studies that used environmental and nutritional information to rank meals. Supplementary Table 1 shows that most of the identified studies used integrated methods of analysis.2 This is related to a common theme in the literature – the discussion around “single scores” representing the combined assessment of both environmental impacts and nutrition to facilitate informed consumer choices (Lukas et al., 2016; Sturtewagen et al., 2016; Schaubroeck et al., 2018; Mazac et al., 2023). Around half of the studies included ranking of meals based on their combined environmental impacts and nutritional value, and a variety of approaches were used to determine the nutritional value of meals. Environmental impacts were assessed in various impact categories, with climate change impacts assessed most frequently. Most of the 11 studies assessed actual meals or meals constructed with real-life data, in university, school, and worksite canteens, care homes, and restaurants (Supplementary Table 1), while only three studies (Lukas et al., 2016; Batlle-Bayer et al., 2020 and Mazac et al., 2023) evaluated theoretical/hypothetical meals. In fact, the importance of using real-life meals to account for social/cultural acceptance was noted for future research (e.g., Batlle-Bayer et al., 2020).
In summary, the literature showed that the integrated approach to combined assessments was the more frequently adopted approach in meal-level studies, and a “real life” meal focus was considered important to the analyses. Moreover, only four nLCA studies were identified that used nutritional indices to compare and rank meals. Three of these studies used the NRF9.3 index, and only Mazac et al. (2023) used the NRF approach with more than 9 nutrients to encourage. Therefore, given the outstanding methodological issues related to the use of NRF indices in nLCA studies, this research strives to develop a better understanding of the use of NRF indices in nLCA studies of meals. To do this, different methods were investigated for ranking foods within a simple meal context, using a case study of a New Zealand (NZ)-specific “toppings on toast” (ToTs) meal. The overarching aim of this study is to understand how nLCA can be used to support consumers to make informed food choices based on credible, life cycle-based nutritional and environmental information.
2 Methods
A survey was conducted to identify common food choice preferences for ToT meals (Section 2.1). The survey results were used to identify the most commonly consumed toast toppings. For the selected toppings, climate change impact scores (Section 3.1.1), nutritional value scores (Section 3.1.2), and nLCA results (Section 3.1.3) were then calculated.
2.1 Survey of preferred toast toppings
A survey was developed as an online questionnaire using Google Forms, with questions about topping preferences on toast. It was shared with the researchers’ personal and professional contacts by email and on social media. Of the 157 respondents, 94% said that they commonly consume toasted or untoasted bread with toppings as a meal or snack option and most respondents preferred to have this for breakfast or lunch. Most of the respondents were fairly evenly distributed between age groups covering 21–60-year-old people, with a smaller proportion aged younger than 20 or over 60 years of age – thus, the survey was fairly representative of the age distribution of adult NZ residents (O'Neill, 2023). Respondents indicated that the most preferred toppings were avocado, tomatoes, cheddar cheese, salmon, tuna, chicken, egg, butter, hummus, mushrooms, banana, honey, jam, nut butters, marmite/vegemite,3 and bacon. Of these, LCA data was not available for hummus and marmite/vegemite, therefore these specific toppings were excluded from further analysis.
2.2 Climate change scores
The literature on the nutrition-environment nexus and sustainable diets notes a consistent focus on climate change as the priority environmental impact category of interest (Heller et al., 2013; Hallström et al., 2015; Jones et al., 2016; Eme et al., 2019; Guo et al., 2022; Harrison et al., 2022). Given the current climate crisis (United Nations Framework Convention on Climate Change (UNFCCC), 2023a), climate change was considered for the environmental impact analysis. The impact scores, calculated using Global Warming Potential (GWP) over a 100-year timeframe (GWP100), were quantified for each of the selected toppings.
In a recent study, Drew et al. (2020) developed an NZ-specific database of LCA-based climate change impact scores for a comprehensive range of food products aligned with those listed in the New Zealand Food Composition Database. To do this, the authors first screened several available databases based on predefined criteria and then selected the one provided by Hoolohan et al. (2013) as the reference database that matched all the criteria (see Drew et al. (2020) for details on inclusion and exclusion criteria). NZ-specific LCA data was available for the production of some food products; for these products, the farming/processing-related climate change values were used in the new database (for example, tomatoes, wine, cheese, and dairy milk). For other domestically grown food items for which climate change values were unavailable, Greenhouse Gas (GHG) emissions were estimated by averaging NZ-specific farming/processing values of similar food items grown in NZ for which values were available. In cases where this was not possible, the value from the reference database (Hoolohan et al., 2013) was used as a proxy. One exception to this rule was dairy products. For some dairy products which did not have associated NZ-specific emissions data, emission values for the same dairy products in the reference database were adapted to reflect the quantity of NZ dairy milk used in them. The dairy milk emissions estimates in the reference database were based on global data obtained from Food and Agriculture Organization of the United Nations (FAO) (2010). For all the products for which post-production GHG emissions data were unavailable for NZ, values in the reference database were adapted to reflect transport, storage, and distribution within NZ to the point-of-sale. To our knowledge, this is the only comprehensive NZ-specific LCA-based GHG emissions database available currently.
Because the Hoolohan et al. (2013) dataset (used to develop the Drew et al. (2020) dataset) is ten years old and uses LCA values from even earlier years, a decision tree approach was applied to arrive at the most representative and relevant climate change values for the food items selected for this study. Such decision trees have also been used in other areas of quantitative and qualitative research (e.g., Verdinelli and Scagnoli, 2013; Dutton et al., 2015; De Smalen et al., 2021). As shown in Figure 1, the starting point for the decision flowchart was the availability of recent (<5 years old) climate change impact scores for food items that are specific to NZ. The resulting climate change scores for the chosen toast topping, including further details on calculations, are listed in the Supplementary material (Section 1, and Supplementary Table 2).
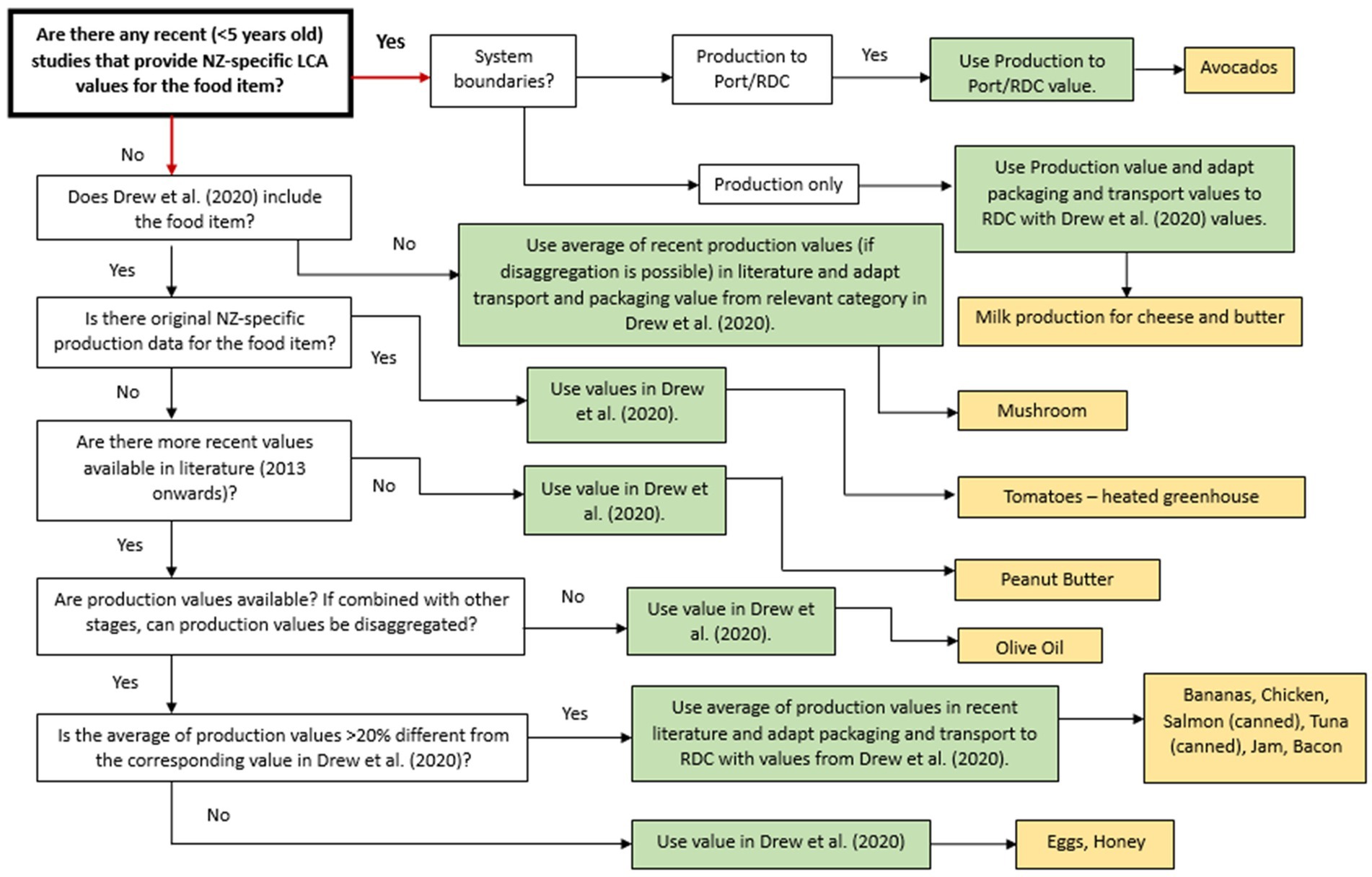
Figure 1. Decision tree to identify the climate change impact scores for the toast toppings selected for this study.
2.3 Nutritional values
2.3.1 Assignment to food group
The food group categorizations used in this study are listed in the Eating and Activity Guidelines published by the NZ Ministry of Health (2020) (see Supplementary Table 2 for a list of all toppings with the food groups they belong to in this study).4 These dietary guidelines indicate that processed foods typically high in sugar, saturated/trans fats, and/or salt (e.g., processed meat, cakes, biscuits, butter, honey and jam) should be replaced with nutrient-rich and less processed foods. The NZ dietary guidelines do not categorize these foods separately, but the Australian dietary guidelines suggest a separate category for them called “discretionary foods” (National Health and Medical Research Council, 2013a). Therefore, for this study, four toppings (bacon, butter, jam, and honey) were categorized as “discretionary foods”.
2.3.2 Nutritional score
2.3.2.1 Reference unit
This study considers different food items in a simple meal context; therefore, the portion size (which in most cases was the serve size)5 of individual toppings was selected as the appropriate reference unit (McLaren et al., 2021; Green et al., 2023). Serve sizes for most of the toppings in this study were obtained from the standard values provided in the New Zealand Food Composition Database (NZFCD) (2022) (see Supplementary Table 3 for all serve sizes used in this study). For some of the toppings, adjustments were made so that the assumed amount consumed was more realistic (for example, two rashers of bacon instead of one) guided by the NZ and Australian dietary guidelines (National Health and Medical Research Council, 2013b; Ministry of Health, 2020).
2.3.2.2 Choice of nutrients in indices
The number and type of nutrients included in published nutritional indices varies from 6 to 22 qualifying, and up to three disqualifying nutrients in NRF indices, and up to 27 qualifying and 6 disqualifying nutrients in indices using the Nutrient Balance Concept (NBC) (see Supplementary Table 4 for a list of indices identified in literature). For this study, it was decided to use the NRF family of indices and NRF9.3 was chosen as the baseline since it is the most widely used index. However, as discussed in Section 1, NRF9.3 includes a limited number of nutrients that do not represent the entire nutritional quality of all foods. Therefore, a more comprehensive nutritional index was compiled that included all the nutrients which appear at least once in the New Zealand Food Composition Database nutritional composition data for each of the 14 toppings, and for which Nutrient Reference Values (NRVs) are also available.6 This resulted in an index with 28 nutrients to encourage and 3 nutrients to limit. The selected nutrients in both indices are listed in Table 1.
NRVs for each nutrient were obtained from the combined NRV list developed for Australia and NZ by the National Medical Health and Research Council (2017). Some NRVs vary by age and sex and so the mean NRVs for adult men and women (based on the 50:50 ratio of men: women as per Stats NZ, 2023) in the NZ population were used in the analysis. The NRV value for MUFAs was obtained from Drewnowski et al. (2009) (which provided the MUFA value for a 2,000 kcal diet) and adapted to the standard NZ diet of 2,081 kcal as per the Australia New Zealand Food Standards Code [Food Standards Australia New Zealand (FSANZ), 2021]. The Upper Levels (ULs) of intake for saturated fats and sodium were also obtained from Food Standards Australia New Zealand (FSANZ) (2021). The UL for added sugar was adapted to the NZ 2,081 kcal diet from the value provided for an average 2,000 kcal diet in Fulgoni et al. (2009). Weighting and/or capping were not applied to the indices (see Section 4.1.3 for a discussion on this methodological choice).
2.3.2.3 Calculation of nutritional value
All nutritional composition data were obtained from the New Zealand Food Composition Database (NZFCD) (2022). This database considers the sugar content in honey to be both added and free, based on the definition of the two kinds of sugars provided by the United States Food and Drug Administration (Erickson and Slavin, 2015) and the World Health Organization (WHO) (2015). Since honey was studied as a topping/food item (and not as a sweetener) in this study, the sugar content in honey was not considered to be added sugar.
For each topping, the ratio of every nutrient to its NRV was calculated. These ratios can then either be summed, or their mean calculated, to arrive at the NRn or LIM score. For this study, the NRn and LIM values were calculated using the mean method (see Eqs. 1 and 2) since the toppings were being evaluated for comparison using two different indices with a different number of nutrients considered in each index. The NRF value was obtained by subtracting the LIM from the NRn.
n = number of beneficial/qualifying nutrients; Nutrienti = content of beneficial/qualifying nutrient “i” per serve of the topping (g, mg, or μg); NRVi = the nutrient reference value of beneficial/qualifying nutrient “i” (g, mg, or μg).
And
Nutrientj = content of limiting/disqualifying nutrient per serve of the topping (g or mg); NRVj = the nutrient reference value of limiting/disqualifying nutrient “j” (g or mg).
2.4 Calculation of nLCA results
For the combined nutritional and environmental analysis (nLCA), the climate change impact scores and nutritional (NRn, LIM, and NRF) scores per serve size were compiled into one table for the 14 toppings. In addition, these scores were also presented on the basis of the Energy Density (ED) of the food items per serve as recommended in McLaren et al. (2021). The scores for all the toppings within each category (climate change impact, NR9, NR28, NRF9.3, NRF28.3, and ED) were divided into quartiles and each quartile assigned a color for a visual representation of the nLCA results. In a next step, the climate change impact, NRF9.3 and NRF28.3 scores of all the toppings were assigned performance-based values from 1 (worst performance) to 4 (best performance), based on the quartiles. Then two scenarios were established – ranking set A and ranking set B. Set A consisted of the average of the above-mentioned assigned values for climate change and NRF9.3 for each of the 14 toppings. Set B used the assigned values for climate change and NRF28.3 instead of NRF9.3 (See Eqs. 3 and 4). The toppings were then ranked based on the values obtained in these two scenarios.
RAscore = ranking score for the topping for ranking set A; Vcc = quartile-based value assigned to the topping for its climate change score; V9.3 = quartile-based value assigned to the topping for its NRF9.3 score.
And
RBscore = ranking score for the topping for ranking set B; Vcc = the quartile-based value assigned to the topping for its climate change score; V28.3 = quartile-based value assigned to the topping for its NRF28.3 score.
In addition, a sensitivity analysis was undertaken using the two other commonly used reference units, mass (per 100 g) and energy (per 100 kcal), using the same method described above.
3 Results
The climate change, nutritional and nLCA results are presented in this section per serve size for the baseline scenario, followed by a comparison of the results when using a mass- or energy-based reference/functional unit.
3.1 Baseline results
3.1.1 Climate change impact scores
Figure 2 shows that the climate change impact scores are higher for the toppings that are products from animals rather than plants; canned salmon has the highest impact score with 0.42 kg CO2 eq./serve, followed closely by bacon, cheddar cheese, tuna, and chicken. Avocados and bananas had the lowest impact scores among the fresh plant-based foods with 0.07 and 0.10 kg CO2 eq./serve, respectively. Jam and honey (0.04 kg CO2 eq./serve each) had the lowest climate change impacts of all the 14 toppings.
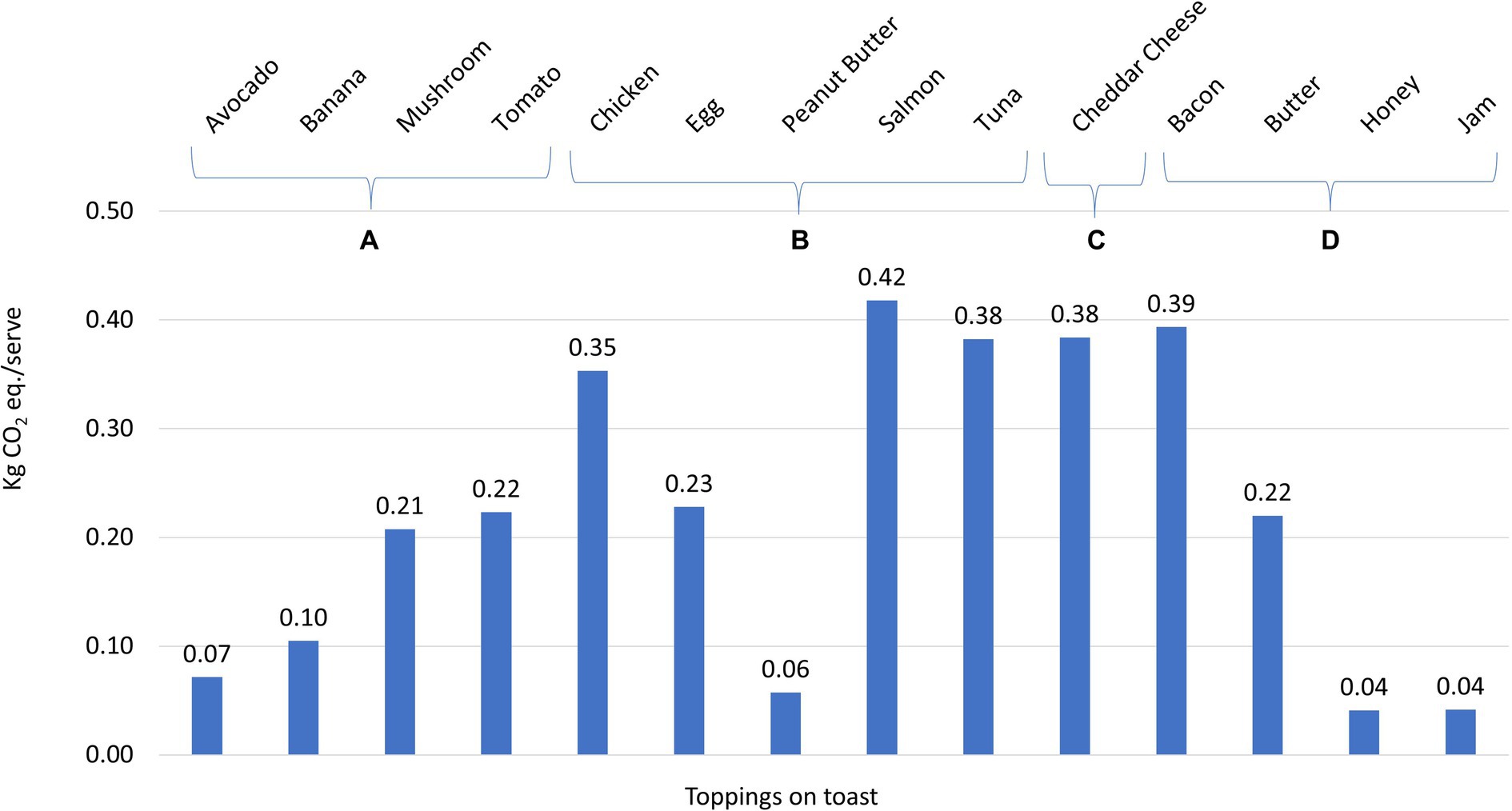
Figure 2. Climate change impact scores (kg CO2 eq./serve) for each of the 14 selected toppings representing the four food groups: (A) Vegetables and fruits; (B) Legumes, nuts, seeds, fish and other seafood, eggs, poultry, or red meat with fat removed; (C) Dairy milk and dairy milk products, mostly low and reduced fat; and (D) Discretionary foods.
3.1.2 Nutritional analysis
The NRn and LIM scores are shown in Figure 3. Butter had the highest LIM score (0.33), followed by bacon (0.25) and cheddar cheese (0.17). When considering the NR9 index, chicken and honey had the highest (0.1) and lowest (0.001) scores respectively; for the NR28 index, tuna and salmon had the highest scores (0.18 and 0.17 respectively) and jam the lowest score (0.002).
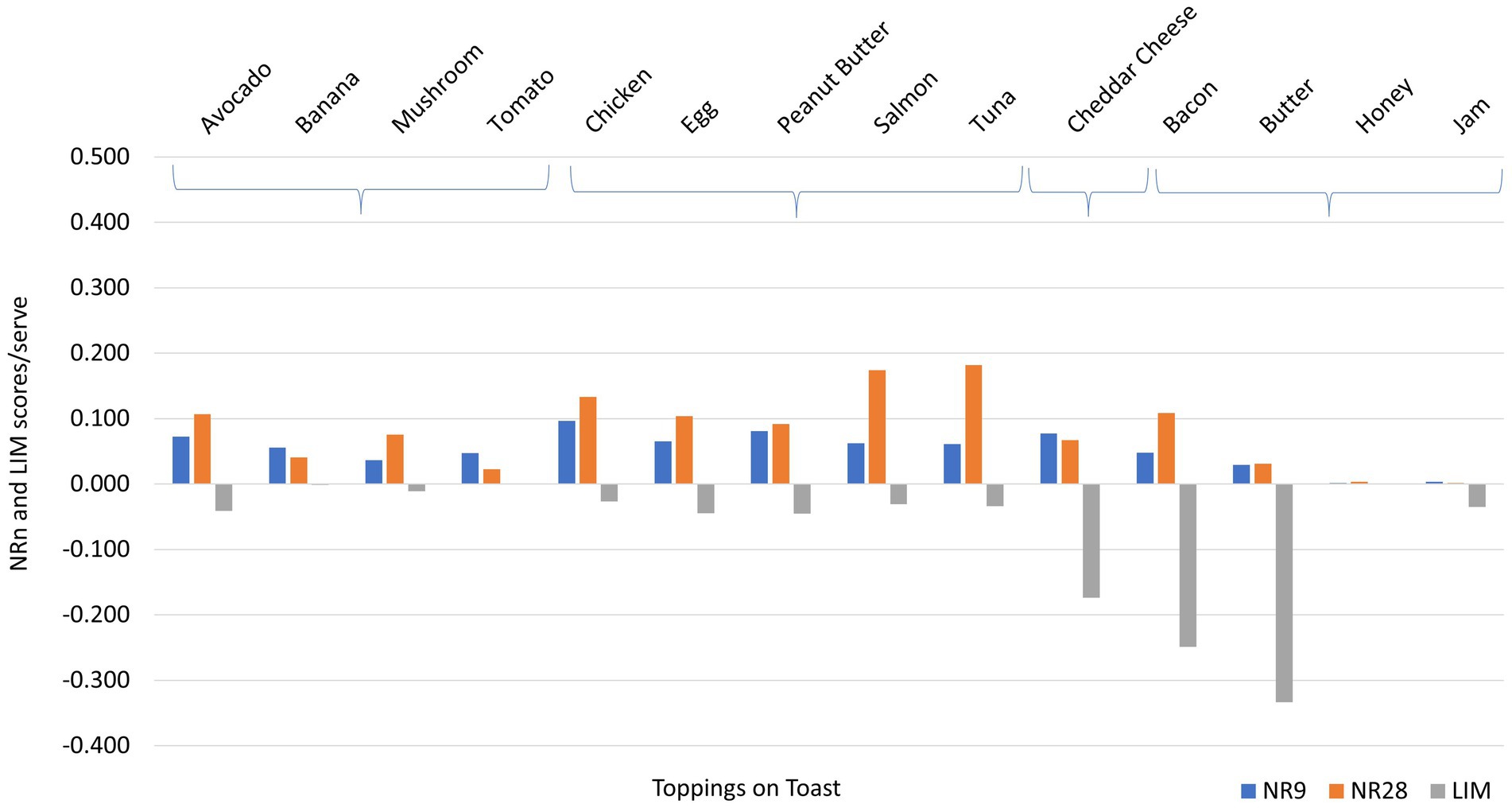
Figure 3. NRn and LIM values for 9 and 28 qualifying and 3 disqualifying nutrients for each of the 14 toppings representing the four food groups: (A) Vegetables and fruits; (B) Legumes, nuts, seeds, fish and other seafood, eggs, poultry, or red meat with fat removed; (C) Dairy milk and dairy milk products, mostly low and reduced fat; and (D) Discretionary foods.
The NR28 scores were higher than the NR9 ones for most of the toppings. The largest change (%) from the NR9 to NR28 score was noted in tuna (nearly 200% higher) (Figure 4). This was followed by salmon, bacon, honey, and mushrooms – all of which showed >100% increase from the NR9 score. The exceptions to this trend were tomatoes, bananas, cheddar cheese, and jam. The lower NR28 scores (relative to NR9) for these four toppings can be attributed to the presence of relatively smaller quantities of other nutrients (not included in NR9), which reduced the mean value of the nutrient to NRV ratios used to calculate the NRn indices. To illustrate, the largest increase from NR9 to NR28 scores was noted for tuna (as mentioned above) and the largest decrease for tomato. For tuna, the nutrients common to both indices contributed only 11% to the total NR28 score; for tomato, 66% of the contribution to the total NR28 score was from the common nutrients (see Supplementary Table 5).
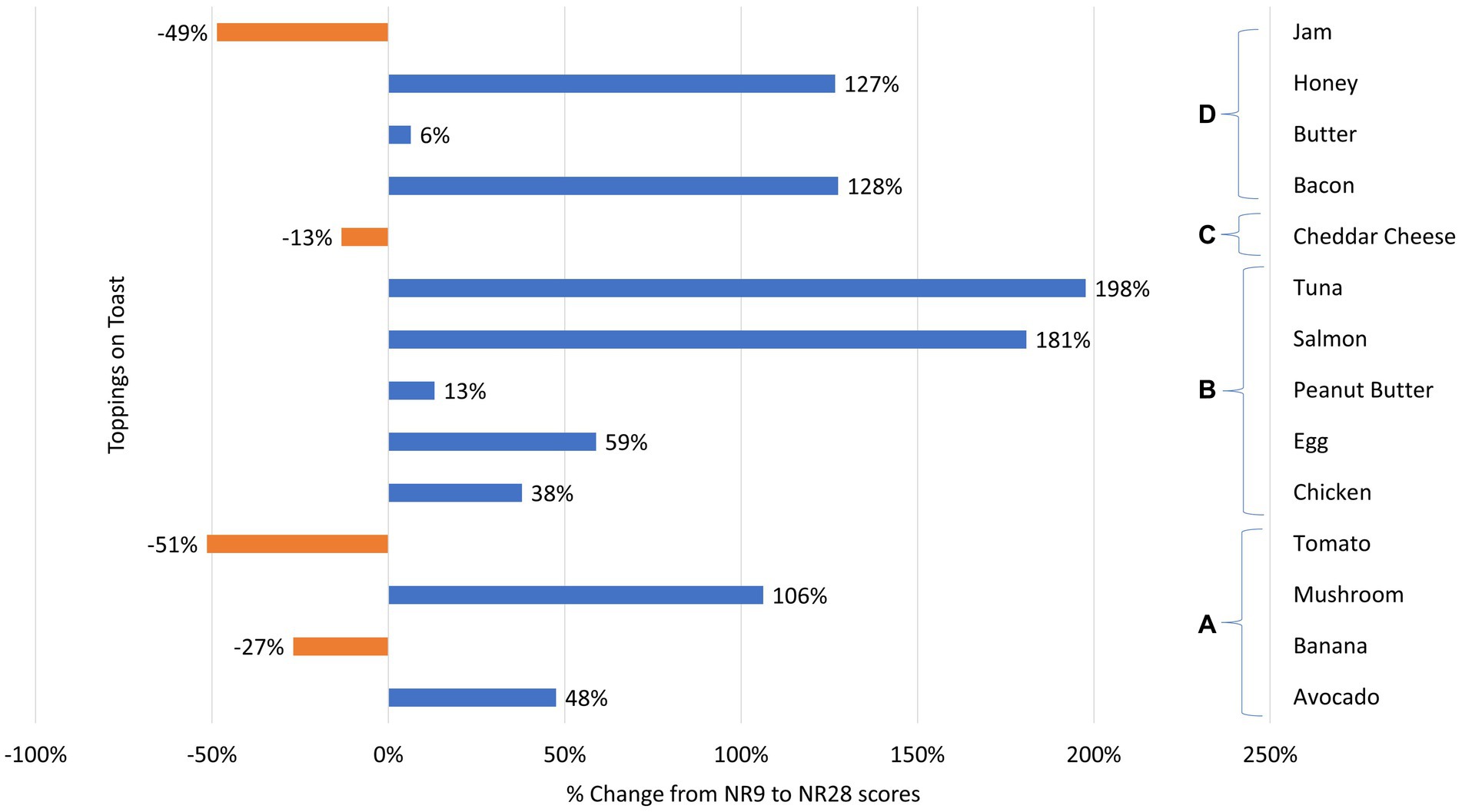
Figure 4. Change (%) from NR9 to NR28 scores of the 14 toppings representing the four food groups: (A) Vegetables and fruits; (B) Legumes, nuts, seeds, fish and other seafood, eggs, poultry, or red meat with fat removed; (C) Dairy milk and dairy milk products, mostly low and reduced fat; and (D) Discretionary foods.
The overall trend of the majority of the toppings displaying higher NR28 scores compared to NR9 can be attributed to the inclusion of a larger number of water-soluble vitamins (as well as minerals and unsaturated fats to a lesser extent) in the NR28 index (see contribution analysis in Figures 5, 6). Minerals, fat-soluble vitamins, and proteins were the main contributors to the NR9 score of most of the toppings (accounting for 38, 23, and 22% to the total score on average), with water-soluble vitamins accounting for only 9% of the total score on average. There was no contribution of dietary fiber to the total NR9 scores of the animal-based toppings, but it accounted for 9–24% of the total NR9 score for all the plant-based toppings, with the highest contribution in avocados (24%). However, when using the NR28 index, the average contribution of water-soluble vitamins to the total NR28 score increased to 39%, while the average contribution of fat-soluble vitamins and proteins dropped to 10 and 4% of the total score, respectively. Mineral contribution also decreased, but to a lesser extent (28% of total), while unsaturated fat, now represented in the NR28 index, accounted for 17% of the total score on average. Average dietary fiber contribution was the lowest at 3% of the total NR28 score.
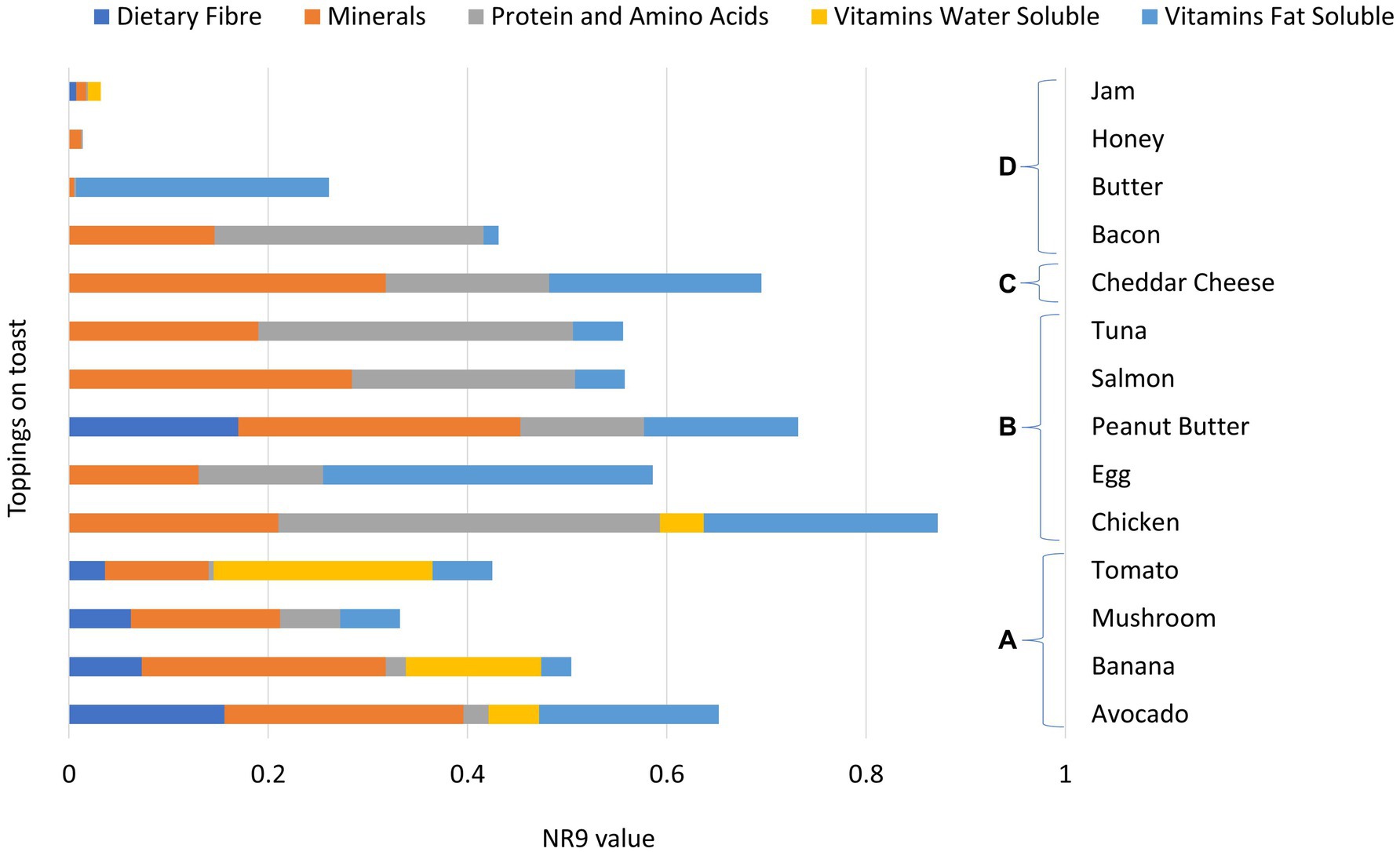
Figure 5. Contribution of nutrient categories to NR9 values for each of the 14 selected toast toppings representing the four food groups: (A) Vegetables and fruits; (B) Legumes, nuts, seeds, fish and other seafood, eggs, poultry, or red meat with fat removed; (C) Dairy milk and dairy milk products, mostly low and reduced fat; and (D) Discretionary foods.
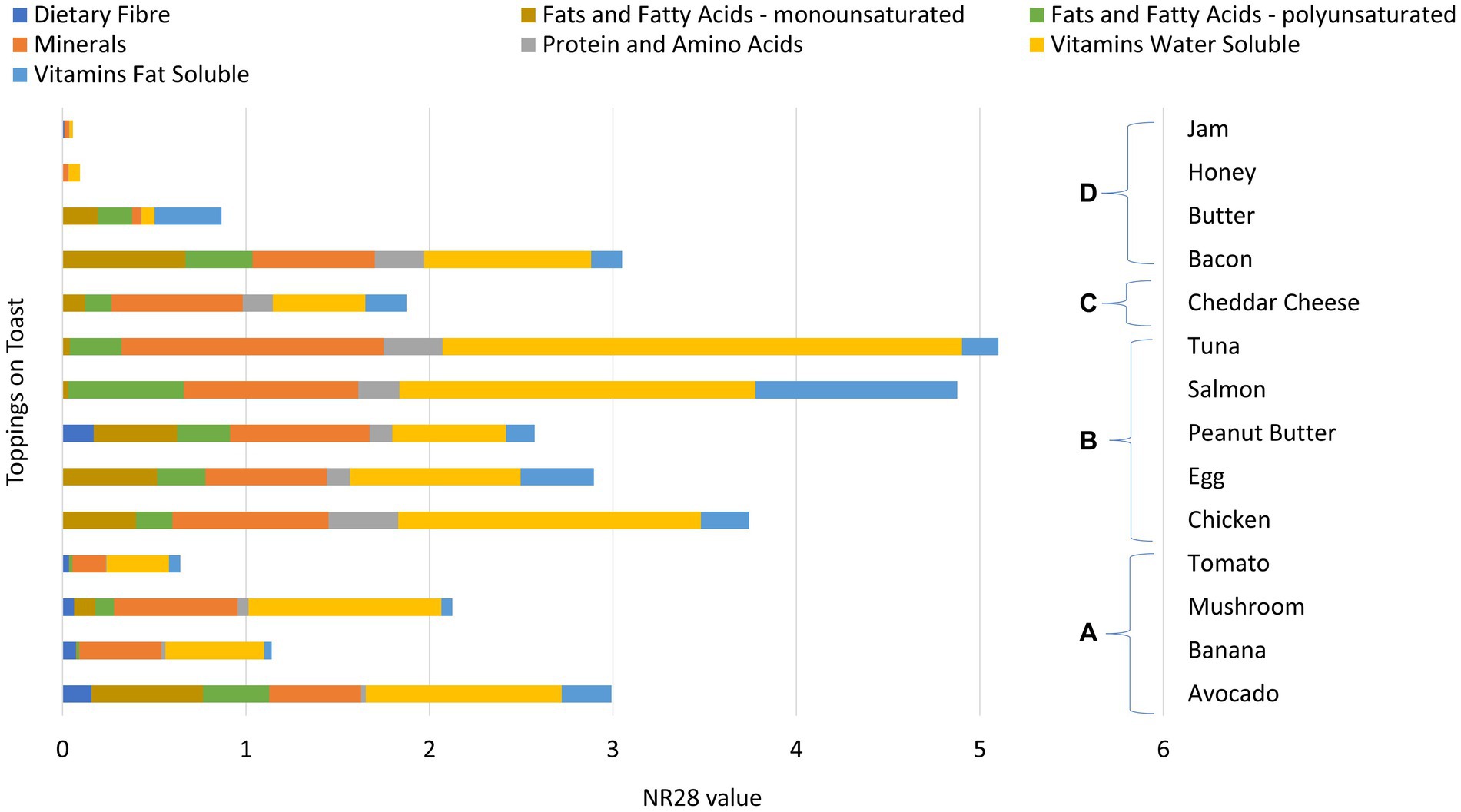
Figure 6. Contribution of nutrient categories to the NR28 values for each of the 14 selected toast toppings representing the four food groups: (A) Vegetables and fruits; (B) Legumes, nuts, seeds, fish and other seafood, eggs, poultry, or red meat with fat removed; (C) Dairy milk and dairy milk products, mostly low and reduced fat; and (D) Discretionary foods.
With respect to the three toppings with the largest LIM scores (bacon, butter, and cheese), sodium was the main contributor to the LIM score for bacon (70%), while saturated fatty acids contributed the most to the LIM scores for butter and cheese (95 and 75% respectively) (Figure 7). Added sugar was present in only one topping (jam) and accounted for almost its entire LIM value.
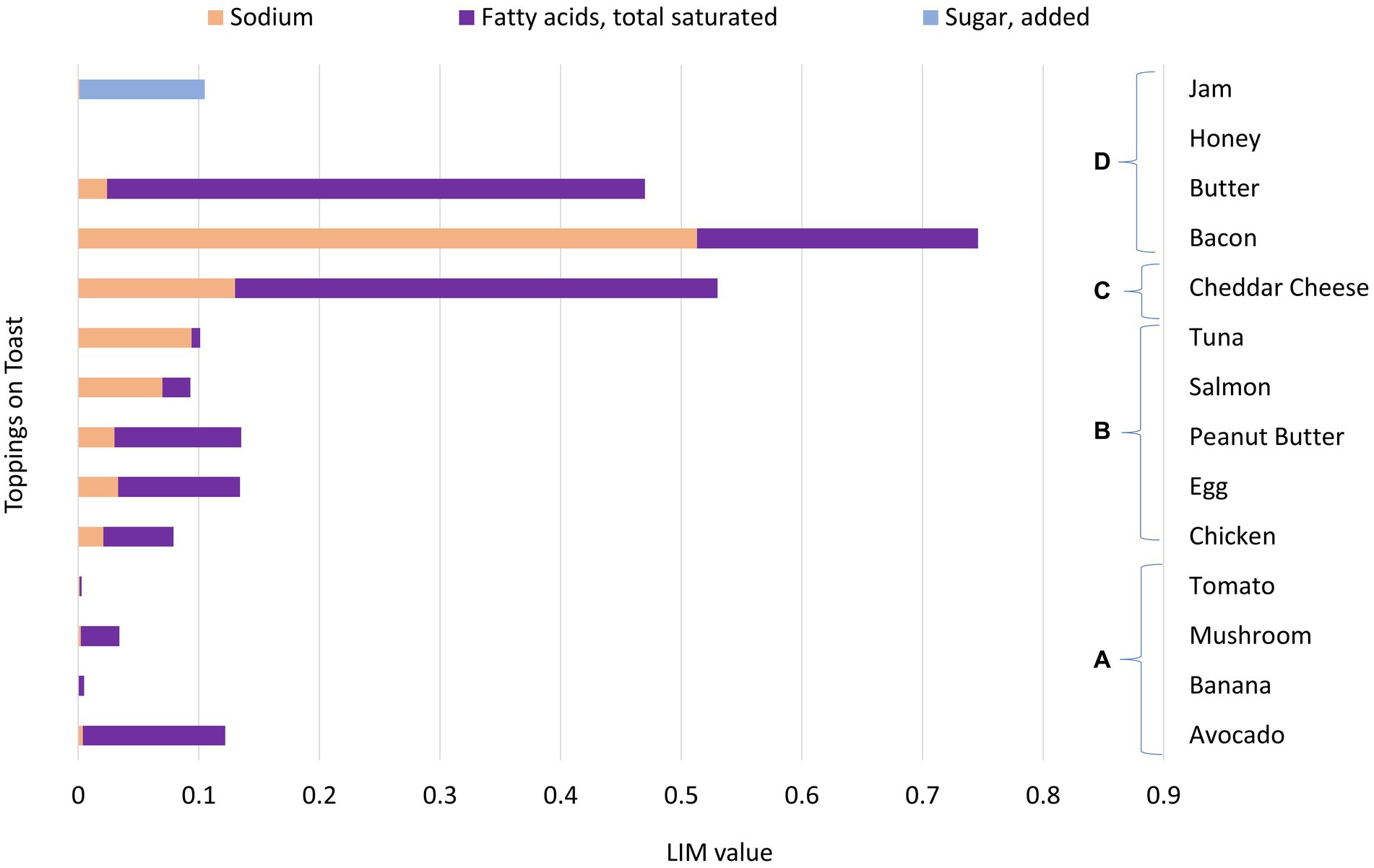
Figure 7. Contribution of the three disqualifying nutrients to the LIM scores for each of the 14 selected toast toppings representing the four food groups: (A) Vegetables and fruits; (B) Legumes, nuts, seeds, fish and other seafood, eggs, poultry, or red meat with fat removed; (C) Dairy milk and dairy milk products, mostly low and reduced fat; and (D) Discretionary foods.
Butter, bacon, cheddar cheese and jam had negative scores for both the NRF9.3 and NRF28.3 indices (Figure 8). This is explained by the relatively high LIM scores for butter, bacon, and cheese, compared to the other toppings. In the case of jam, the low NRn value combined with the higher LIM value resulted in its negative NRF score. The NRF28.3 values for most of the toppings were higher than their NRF9.3 scores. Of these, the largest difference between the NRF9.3 and NRF28.3 indices was for salmon and tuna with the NRF28.3 scores being ~5 times more than the NRF9.3 value for both. This general trend of higher scores for the NRF28.3 index was also seen in the NRn comparisons in Figure 3 as mentioned earlier (where the NR28 scores were higher than the NR9 scores for most of the toppings). As with the NRn scores, the exceptions to this trend were banana, tomato, cheddar cheese, and jam.
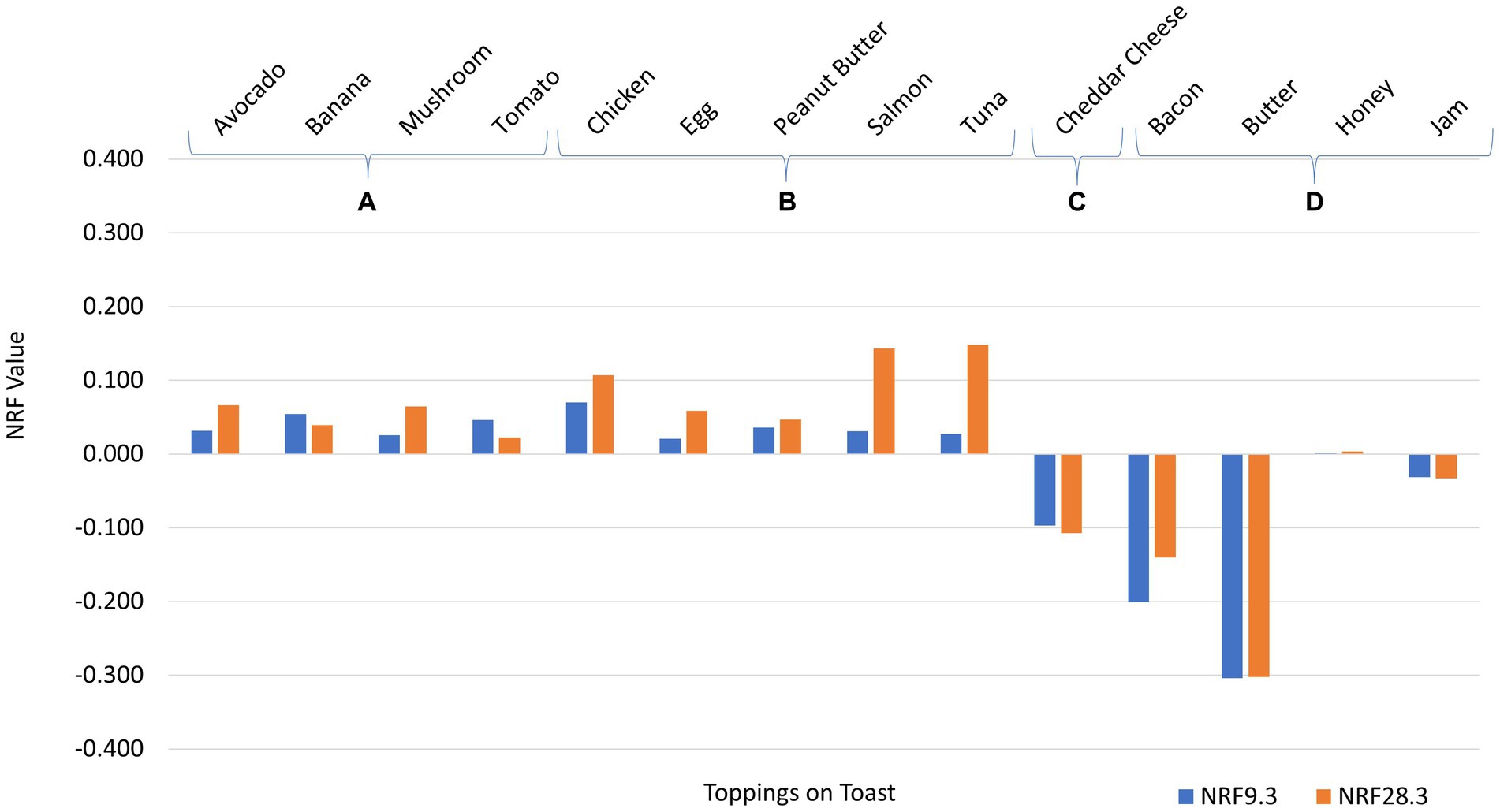
Figure 8. NRF9.3 and NRF28.3 values for all the 14 selected toast toppings representing the four food groups: (A) Vegetables and fruits; (B) Legumes, nuts, seeds, fish and other seafood, eggs, poultry, or red meat with fat removed; (C) Dairy milk and dairy milk products, mostly low and reduced fat; and (D) Discretionary foods.
3.1.3 nLCA scores
The nLCA results for the toppings are presented in Table 2. This table effectively represents the climate change impact scores alongside a separate midpoint nutrition impact category comprising the NRF scores. For the ranking process, a weighting value from 1 (worst) to 4 (best) was assigned to each of the quartiles for climate change, and the NRF9.3 and NRF28.3 scores. Only the NRF values were used in this part of the analysis because they include both nutrients to limit and encourage. Two ranking sets were then calculated: set A is the average of the toppings’ climate change and NRF9.3 quartile ranking weights, and set B is the average of the toppings’ climate change and NRF28.3 quartile ranking weights (Table 3). Peanut butter and avocado ranked in the top two positions (best scores) in both ranking sets A and B. Cheddar cheese and bacon rank lowest and butter second to last (worst scores) in both ranking sets. Overall, the ranking of the toppings does not change significantly between the two ranking sets – in fact, all the toppings just move up or down one position between the ranking sets except tomato, which changes by two positions.
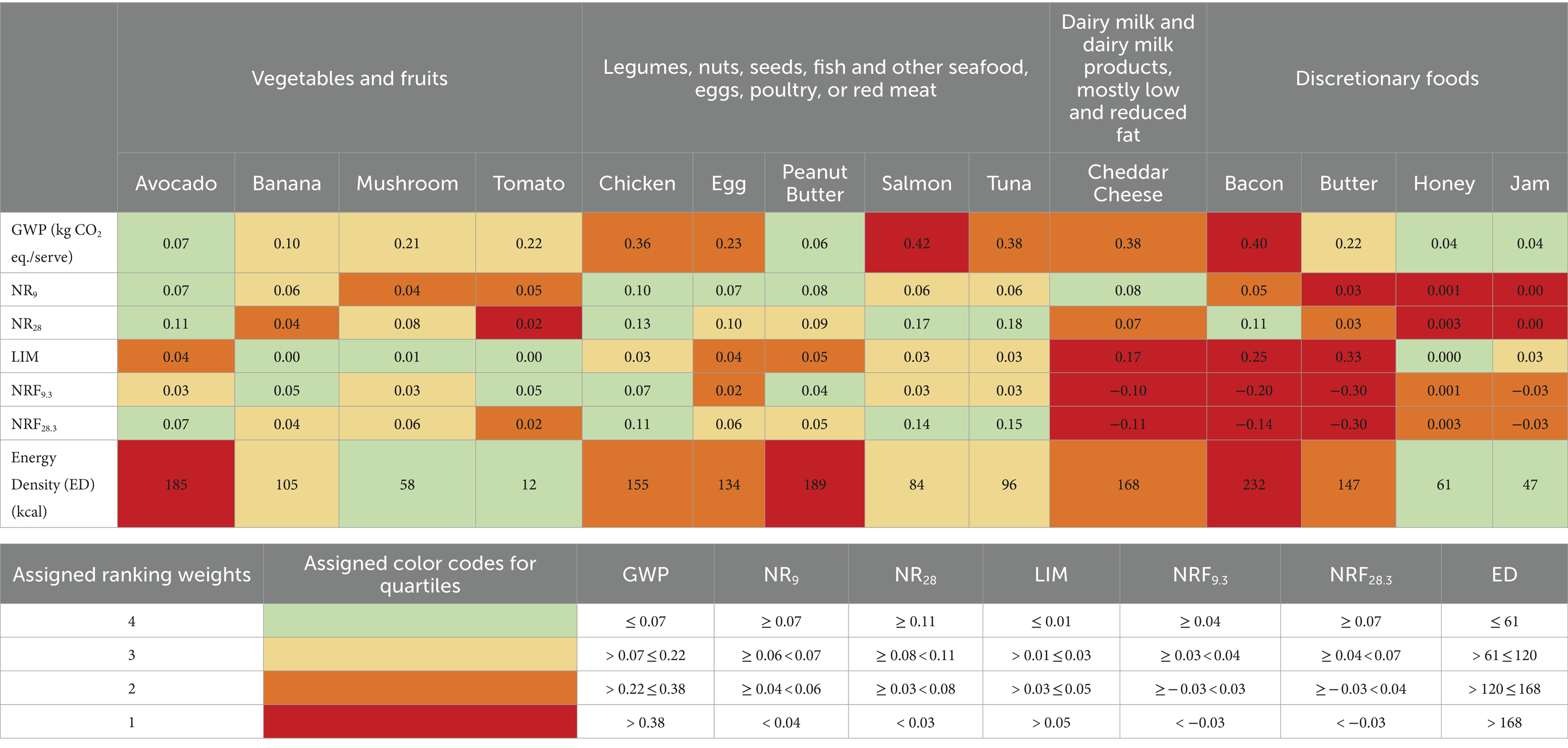
Table 2. nLCA results presented per serve for the environmental (climate change impact) and nutritional values (NRn, LIM, NRF, and Energy Density) for each of the 14 selected toast toppings, color-coded by the quartile in which each score is categorized.
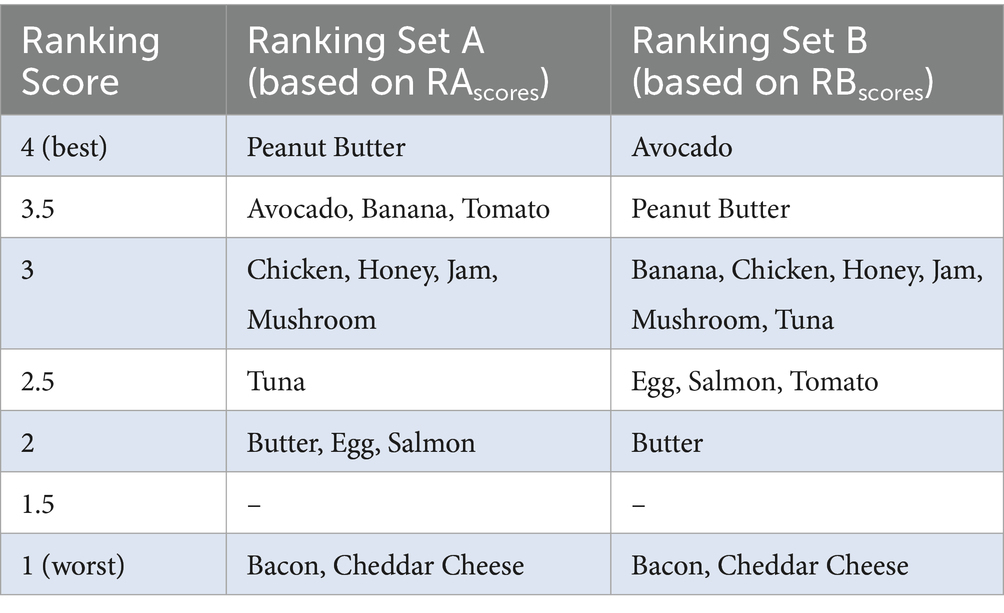
Table 3. Ranking sets A and B for toppings, based on assigned values for quartile-categorized scores for climate change, NRF9.3 and NRF28.3 (RAscores and RBscores).
3.2 Comparison of results using other reference units
The nLCA results for the 14 toppings are presented for each of the three FUs in Table 4. When using different FUs, the climate change results for some toppings differ markedly. In particular, for tomato the climate change result is 8.5 times higher when using the 100 kcal rather than serve size as the reference unit. The topping with the highest climate change result is different for each reference unit: salmon based on serve size, butter per 100 g, and tomato per 100 kcal. Overall, vegetables, fruits and protein-rich foods perform better nutritionally and environmentally across all three reference units, whereas cheese and toppings that are considered discretionary foods have the lowest scores.
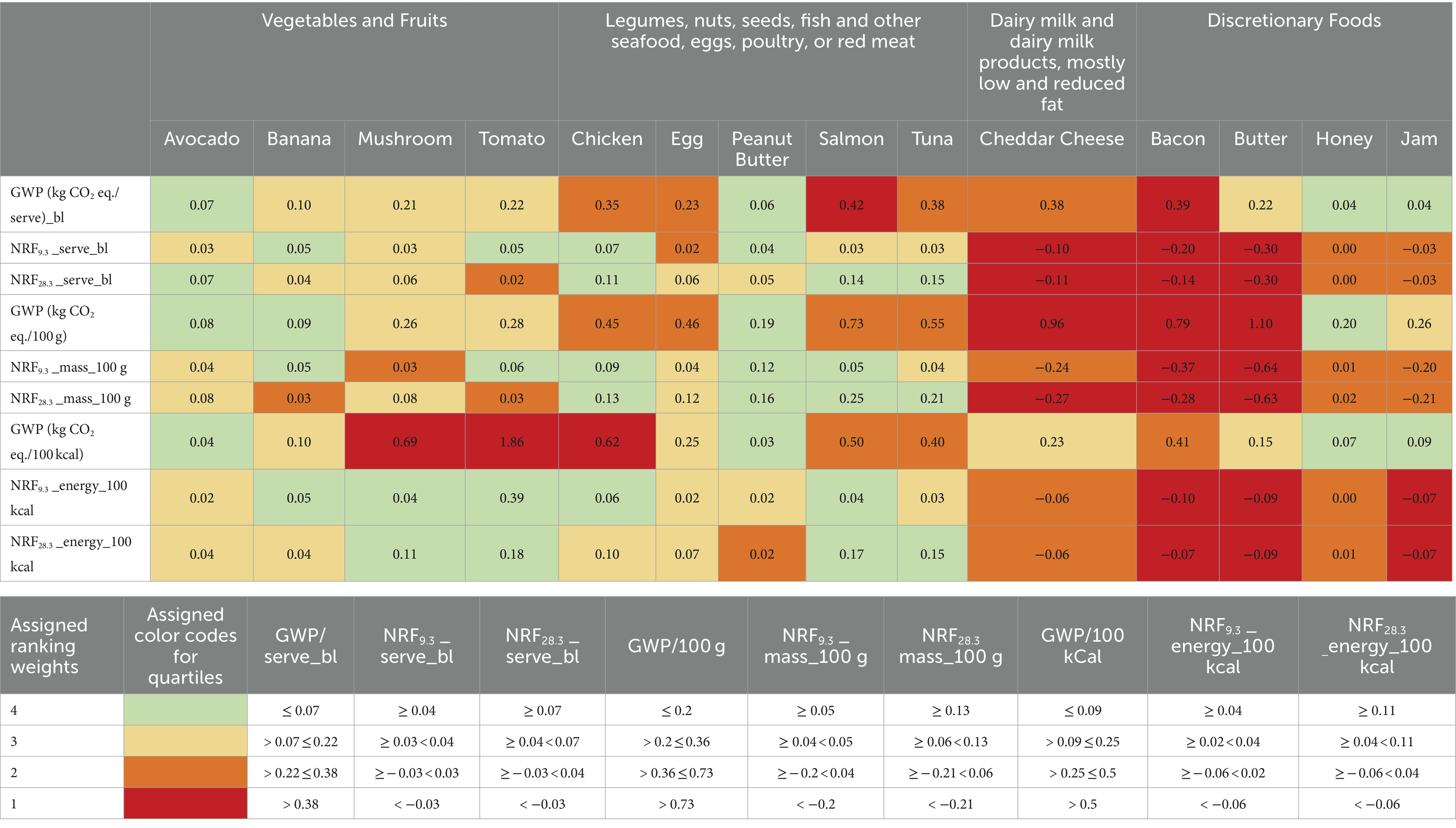
Table 4. nLCA results for all 14 selected toast toppings, with respect to three reference units – mass, energy, and serve size (bl stands for baseline) color-coded by the quartile in which each score is categorized.
In a subsequent step, the toppings were ranked using the combined climate change impact and nutritional scores as per Section 3.1.3. Two ranking sets (A and B) were assessed for the two additional FUs – mass (100 g) and energy (100 kcal). These ranking sets are presented in Table 5 along with the baseline ranking set (i.e., per serve size). Avocado, peanut butter and banana appear in the top two ranks for the three FUs in ranking set A. While tomato is ranked second per serve and per 100 g in ranking set A, it moves down two ranks with the 100 kcal FU. For ranking set B, avocado and peanut butter appear in the top two ranks for all three scenarios. Comparing across the two ranking sets, peanut butter and avocado are in the first two ranks, and butter, bacon, and cheddar cheese are in the last two ranks, across all three scenarios in both ranking sets. The change in the ranking position of food items between ranking set A and B is outlined in Supplementary Table 6 for each topping across the three FUs. The toppings which change ranks between the two ranking sets for each FU, move up or down by only one place with two exceptions. The first exception is mushroom which moves down two places in Scenario B per 100 g, and the second is tomato which moves down two places in scenario B per serve size and per 100 g.
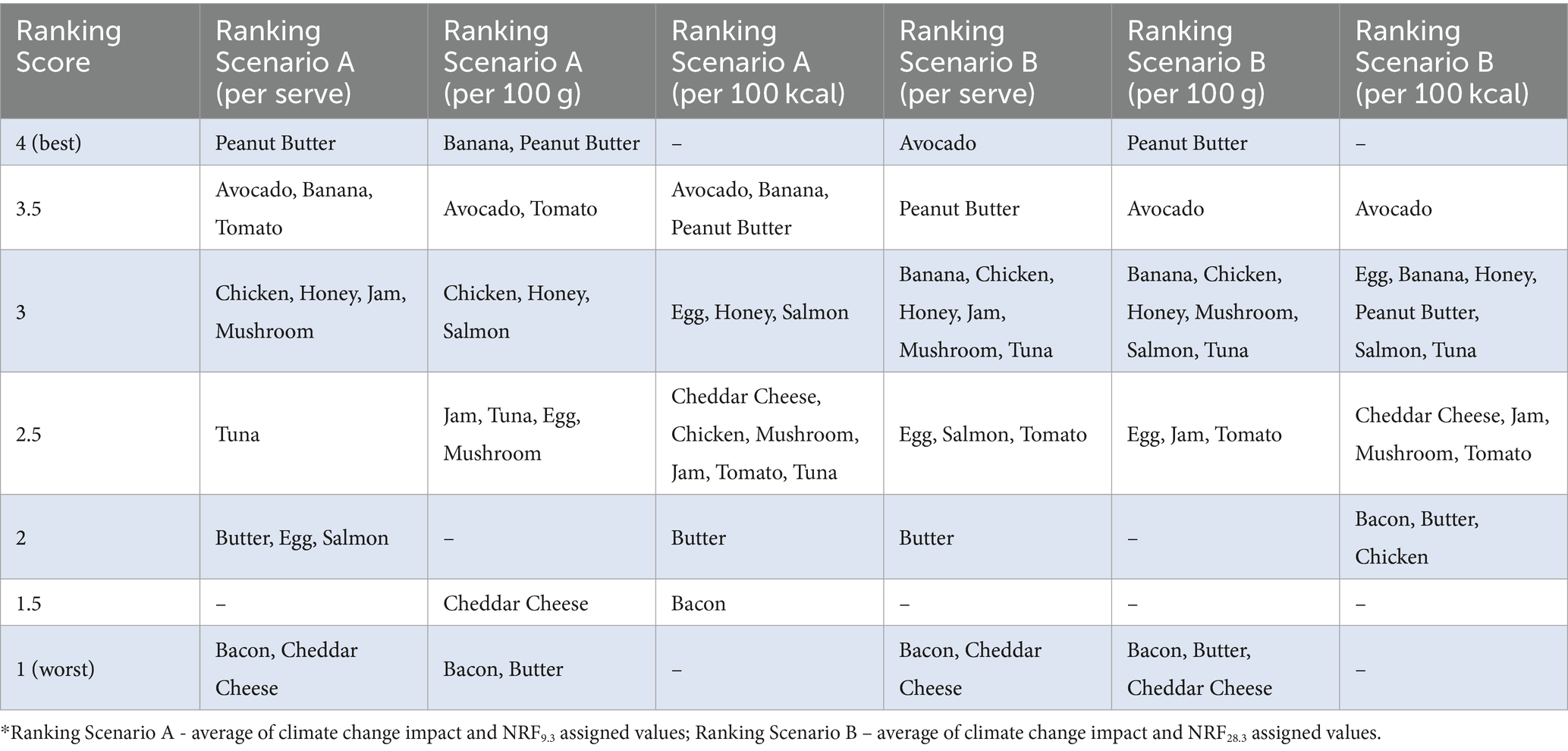
Table 5. Toppings ranked as per the two ranking scenarios*, across three FUs (serve, mass and energy).
4 Discussion
4.1 Methodological choices
4.1.1 Nutrients to consider in indices
The baseline results show that the inclusion of different numbers of nutrients in a scoring system changes the nLCA results for food items in a meal context (Table 2). However, this is not sufficient to change the ranking of the toppings more than one place in the two ranking systems used in this study (Table 3). Tomato is an exception – it changes from second to fourth place in the ranking using NRF9.3 and NRF28.3 index, respectively. This occurs because the NRn score for tomato is significantly lower (>50%) for 28 compared to 9 nutrients to encourage. This is due to the presence of Vitamin C in tomato in significant proportions in both indices and relatively smaller contributions by the additional nutrients in the NR28 index. Interestingly, the difference between the NR9 and NR28 indices for jam was very similar to that for tomato – its NR28 score was 49% lower than its NR9 score, however, its ranking did not change between the ranking sets. This could be a result of its significantly lower climate change impact score (82%), and higher LIM score (34%) relative to tomato. Thus, this study shows that, as some foods contain certain nutrients (included in the NR9 index) in more significant proportions than others (for example, calcium in cheese, dietary fibre in the plant-based toppings, and protein in most of the animal-based ones), these nutrients contribute significantly to the NR9 score of these toppings. However, using an expanded index can “dilute” the total score because of the additional nutrients (not included in NR9) in the expanded index which only make very small contributions to the total score.
The nutrient contribution analyses (Figures 5–7) offer some interesting insights. Unlike the NR9 index, where the proteins and fat-soluble vitamins contribute more to the total score than water-soluble vitamins, the latter usually dominate the NR28 index relative to the proteins and fat-soluble vitamins. Thus, water-soluble vitamins gain significance (from the perspective of proportional contribution to the total nutritional value) when an expanded index is used. This has implications for diets deficient in micronutrients, especially in Low- and Middle-Income Countries (LMICs). In particular, “priority micronutrients”7 include two water-soluble vitamins (folate and vitamin B12) (Beal and Ortenzi, 2022), neither of which are included in the NR9 index. Thus, it is recommended to include these in nLCA studies when a more nuanced approach is needed to study a population whose diet is lacking in these essential micronutrients (Katz-Rosene et al., 2023).
Secondly, most toppings in this case study contain unsaturated fats and these contributed markedly to the NR28 values of several toppings. A number of national and international nutritional guidelines encourage dietary substitution of trans and saturated fats with foods containing MUFAs and PUFAs (especially omega 3 and 6) (National Health and Medical Research Council, 2013a; Ministry of Health, 2020; World Health Organization (WHO), 2023) due to their reported positive impacts on human health (Mozaffarian et al., 2010; Ravaut et al., 2021). Green et al. (2020) also notes that not accounting for healthy fats penalizes foods that are rich in them. Although the rankings did not change significantly in this study when including additional nutrients in the NRF28.3 index, the presence of MUFAs and PUFAs was instrumental in moving some of the toppings up a rank, e.g., avocados, tuna and eggs. Therefore, if food composition data on MUFAs and PUFAs is available, it might be useful to include them in studies focused on comparing/ranking foods within meals or meals themselves.
Overall, the results of this study align with the views of other authors who note that limiting the number of nutrients in nutritional profiling algorithms can be sufficient to obtain a representative understanding of the nutritional value of food items or diets (as mentioned in Section 1). The rankings obtained in this study did not change much between indices; for example, the top two best and worst performing foods were the same in both. However, with specific food items that are significantly richer in certain nutrients not included in indices with fewer nutrients to encourage, it could be useful to expand the index to include them, as this does affect the final rankings to some extent. For example, avocados (rich in MUFAs) ranked second when using the NRF9.3 index but moved up a rank to become the best performing topping with NRF28.3. It is also important to note that these findings are specific to this study and could change if other toppings were considered (because the rankings are relative within the studied toppings). Thus, overall, the choice of nutrients considered for the index will also depend on the diversity of the food items/meals being studied – a more diverse range of foods could benefit from an expanded index, to allow for a better representation of the nutritional (and consequently nLCA) value of those foods.
4.1.2 Choice of functional unit
While choice of FU influences the nLCA values, it does not appear to alter the topping rankings significantly (Tables 4, 5).
Food-related LCA studies usually use mass-based FUs; however, when assessing food choices within a meal context, it is preferable to use serve size rather than equal mass-based units as it reflects more realistic consumption and hence nutrient intakes at the meal or diet level (Masset et al., 2014; Grigoriadis et al., 2021; Jolliet, 2022). The only challenge is that, unlike foods within the same food group that are likely to have similar serve sizes as they are commonly standardized by the amount of dietary energy provided in a serve (see, for example, Hallström et al. (2019) who studied seafood), serve sizes are generally not standardized across different food items from different food groups within meals.8 Moreover, while standardized serve sizes can be useful for comparative studies like the current study, the actual amounts/portions consumed may vary substantially between people and for different consumption situations (for instance, a small snack versus a meal or component of a dish). One solution to variable serve- and portion sizes is using an energy-based metric energy either on a 100 kcal basis or standardized to the recommended daily energy intake (usually ~2,000 kcal). Energy-based FUs account for variable water quantities or calorific densities of different food items, and therefore can make it possible to calculate nutritional value of food independent of portion or serve size (Drewnowski, 2005; Fern et al., 2015; Schaubroeck et al., 2018). Although some argue in favor of using such energy-based metrics (see for example, Green et al., 2023), serve size is still the most representative of actual amounts consumed in one sitting, and therefore more pertinent to the meal context. In fact, Bianchi et al. (2020) suggest that if standardized serve sizes are developed in future, that are also aligned with international standards, then it should be considered a “preferred choice” for FU selection in nLCA studies.
4.1.3 Weighting and capping
Most nLCA studies exclude a specific weighting process, i.e., they weight all the nutrients equally due to a lack of scientific consensus on the appropriate criteria to use for this purpose (Drewnowski, 2017; Green et al., 2020). Some authors recommend weighting of nutrients in an index using the distance-to-target approach, i.e., dividing the DRI by the average intake of that nutrient (Hallström et al., 2019; Bianchi et al., 2020; Ridoutt, 2021; Strid et al., 2021). Some data is available in the NZ Nutrition Survey of 2011 (University of Otago and Ministry of Health, 2011) for the Estimated Prevalence of Inadequate Intake (EPII) of certain nutrients in NZ adults and could potentially be used to develop a scarcity-weighted index. However, using >10-year-old data would probably not be adequately representative of the current situation. Moreover, the survey contains EPII data for only 12 nutrients and excludes, for example, Vitamin D, folate, and iodine. However, if more up-to-date data were available, this would be an interesting extension to the analysis.
Capping refers to restricting the “good” nutrients to 100% of their RDI to avoid over-counting their benefits if there are large amounts in the studied food items. Capping has been applied in several nLCA studies (e.g., Arsenault et al., 2012; Van Kernebeek et al., 2014; Doran-Browne et al., 2015; Drewnowski et al., 2015; Hallström et al., 2019; Batlle-Bayer et al., 2020; Green et al., 2021), but there is currently no consensus on its use (McLaren et al., 2021; Kyttä et al., 2023). Some researchers suggest that, within the diet (i.e., at the food item or meal-level), nutrients should be left uncapped (Hallström et al., 2018; Mazac et al., 2023), because generally diets comprise a diverse range of foods and if a food has lower levels of a particular nutrient, this is compensated by other foods with higher quantities of that nutrient. Green et al. (2023) recommends that nutrients should be capped in a total diet-level study because it represents a complete set of nutrients and therefore, these should not exceed RDIs. Green et al. (2023) also suggest that consuming a particular nutrient in excess of its recommended value not only provides no additional benefits but can even be harmful to health. However, while this may be true for some nutrients, other nutrients may actually have beneficial health impacts when present in concentrations higher than the RDI. For example, certain vitamins, minerals like selenium, PUFAs like omega 3, and dietary fibre have been noted to lower the risk of chronic health issues like heart disease, cancer, and degenerative cognitive issues like Alzheimer’s when consumed at levels above the RDI (National Medical Health and Research Council, 2017). As the current study was focused on a single meal and none of the nutrients in the studied food items exceeded their RDIs per serve, this was not a relevant consideration.
4.1.4 Other choices
Other methodological choices made in this study that affected the ranking of the different meals include:
Quantile-based ranking: Toppings were categorized into quartiles based on their nutritional and climate change impact scores. Other studies have used quintiles (e.g., Bianchi et al., 2020; Strid et al., 2021; Green et al., 2023). The choice of such quantiles will depend upon the number of alternatives considered and the range of the results. One challenge with quantile-based ranking is that the results may have to be re-calculated if toppings are excluded or added. However, this is only required if their values are very different from the range of values in the rest of the sample.
Weighting of climate change impacts and nutritional value: These two aspects were weighted equally. However, weighting in LCA implies value judgments and equal weighting may not be representative of how much importance consumers place on nutritional versus climate change impacts. One way to address this is to offer the consumer the option to use their own value judgements. For example, on a website or app employing this method, a tab could be included where the consumer could input their own preference-based weights, and the final scores would be calculated accordingly. This weighting process could potentially also be extended to additional environmental impacts if more environmental impact categories were included in the analysis.
Bioavailability of nutrients: Nutrient bioavailability refers to the “fraction of an ingested nutrient that becomes available for use and storage in the body” (Melse-Boonstra, 2020). Bioavailability is an important aspect of nutritional studies because it explains how the consumption of a nutrient translates into actual health effects. Protein bioavailability has been studied with respect to its quality, i.e., the digestibility of the different amino acids (using for example, the DIAAS score) within plant- and animal-based proteins (Bailey and Stein, 2019; Adhikari et al., 2022), linking protein quality and food sustainability (Moughan, 2021) and incorporating protein quality in nLCA studies (Sonesson et al., 2017; Berardy et al., 2019; McAuliffe et al., 2022). Animal-sourced foods are generally found to contain protein and some essential micronutrients in more bioavailable forms than plant-based foods (Beal et al., 2023). For example, iron is present in food as heme and non-heme iron; however, heme iron is only found in animal-based foods and is more bioavailable than non-heme iron. Similarly, zinc and calcium are generally less bioavailable from plant-based compared to animal-derived foods due to the presence of antinutrients like phytates and oxalates in plants (although the concentrations of these will vary with each food) (Maares and Haase, 2020; Melse-Boonstra, 2020; Shkembi and Huppertz, 2022; Yusuf, 2023). Thus, bioavailability of nutrients is an important consideration for future studies as micronutrient inadequacy (and consequent deficiency) continues to be of major global concern, especially in vulnerable population groups such as women of reproductive age (Beal, 2024) or people in developing countries (Zhang et al., 2016). Further, “food matrix” and “meal effects” can also influence nutrient bioavailability and associated nutritional/health impacts (McLaren et al., 2021). Ideally, this would be integrated into the scoring system; however, given the lack of available data on this aspect, it remains a topic requiring further research.
Choice of environmental impacts: As mentioned in Section 2.2, climate change was selected as the environmental impact indicator for this current study. However, this is an obvious limitation, particularly as food systems are associated with a wide range of environmental impacts. Future research should include other impact categories of particular significance to food systems, such as biodiversity loss, soil quality, land use change, and water use and pollution.
4.2 Data choices
For any single food item, the environmental impacts may be quite variable. This variability may be due to production practices, different varieties of the same product, seasonality and variable harvest times, packaging type and size, method of storage and length of storage before consumption, distance transported from farm/industry/packhouse gate to the end consumer, and end-of-life management practices. For example, with respect to production practices, tomatoes may be grown in heated greenhouses, passive or unheated greenhouses, or in the open field; eggs can be obtained from chickens in different housing conditions (caged, barn, free range); jam can be made from fresh fruit or from a semi-finished product using any one of three processes – freezing, drying, or via aseptic treatment; honey can be manufactured using stationary or migratory beehives; and salmon can be farmed in land-based/sea-based aquaculture systems or be wild caught (see Supplementary Table 7). The impact scores for these production methods can be markedly different for a food item, e.g., tomatoes grown in an actively heated greenhouse can have a climate change impact score of up to 2.5 kg CO2 eq./kg compared to 0.3 kg CO2 eq./kg when grown outside in the open field. Similarly, farmed salmon generally has been reported to have higher impacts (2.2–6.4 kg CO2 eq./kg salmon) than wild caught salmon (0.8–1.2 kg CO2 eq./kg salmon).
Production practices can also result in nutritional variability in the same type of food item – for example, farmed salmon contains more PUFAs than wild-caught ones (Colombo and Mazal, 2020). Crop farming systems can also have a significant impact on the nutritional quality of the food. For example, crops grown organically can have different nutrient compositions from conventionally grown crops (Mditshwa et al., 2017). Similarly, Montgomery et al. (2022) showed that crops grown via the regenerative method had increased levels of micronutrients and phytochemicals. The study also found that pork from animals in a regenerative farm had an improved fatty acid profile, including higher levels of omega-3 fatty acids. In addition to production practices, several other factors can impact the nutritional profile of crops, including genotype, climate, soil properties (such as soil pH and organic matter content), geographical factors like elevation, external predatory and disease stressors, as well as post-harvest handling, processing, and storage methods (Hornick, 1992).
In addition to production practices, different varieties of the same product can also have variable environmental impacts. For example, canned pink salmon (one of the toast toppings in this study) is commonly consumed in NZ, but NZ also produces Chinook or King salmon, which is consumed (less commonly) as fresh, hot or cold smoked fillets. The climate change impact of NZ King Salmon was recently calculated at 8.2 kg CO2 eq./kg edible flesh (thinkstep-ANZ, 2023); this value is 30% higher than the canned pink salmon value used in this study. This could be at least partly due to the production practice-related variability mentioned earlier – NZ King salmon is farmed, whereas the latter is most likely wild caught. For button mushrooms, this study used a climate change impact of 2 kg CO2 eq./kg mushroom at shed gate. Tongpool and Pongpat (2013) calculated a similar value for shiitake mushrooms, but Ueawiwatsakul et al. (2014) calculated a value of 4 kg CO2 eq./kg sajor-caju mushrooms at shed gate.
Nutritional content of a food can also vary with seasonality. For example, avocados can remain unharvested longer than other fruits as they ripen only after harvest (Wang et al., 2012). In NZ, avocados are often “left on the tree” to be harvested as per market requirements from September through April. As the fruit’s water content decreases, the dry matter increases as the season progresses, leading to increased concentrations for most of the nutrients (see Supplementary Table 8) and changing the nutritional value per serve size.
4.3 Future directions
The current study looked at food items within a single meal context and demonstrated that overall rankings do not change significantly when an expanded index is used in place of a limited one. This finding should be tested by undertaking additional case studies on more diverse samples of simple and/or composite meals.
Regarding application areas, in addition to helping consumers make more informed food choices, the nLCA-based method in this study could also be used to help restauranteurs or catering services to identify nutrition-poor and high environmental impact meals in their menus and change them accordingly to offer more nutritionally and environmentally sustainable options. With respect to home cooked meals, there are several existing websites and apps to help consumers make healthier food choices as per nutritional recommendations (e.g., Avenue et al., 2012; Plate My Meal, 2023; U.S. Department of Agriculture, 2023) as well as those offering a wide range of recipes to help consumers plan and cook meals at home (e.g., All Recipes, 2023). The nLCA method developed in this study could be used to rank meals on existing or new websites and apps, as well as food composition databases and even national food-based dietary guidelines.
Meals can either be home cooked or obtained outside the home from restaurants/cafés, institutional canteens, catered services, or ready meals. In the case of cooked food obtained away from home, or even home delivered meal kits, information about the food items with respect to the variables mentioned in Section 4.1.5 would, in most cases, be available to the service providers (for example, a restaurant would know whether it is sourcing free-range or factory farmed eggs, button or shiitake mushrooms, early or late season avocados, etc.). However, in the case of home-cooked meals based on recipes provided in apps or on websites, it is left to the consumer to source the meal components/ingredients. In this case, the variability mentioned in Section 4.2 can be communicated to the consumer by providing nutritional and environmental score ranges. To this end, it might be useful to investigate the extent of the influence of the abovementioned variables on nLCA-based meal rankings in future studies. This could help streamline the factors which have the largest impacts on individual food items, those which affect majority of the food items, and finally those which influence the nLCA-based meal-level rankings.
With respect to other avenues for future research, weighting the nutrients in a food or meal according to their relative importance (for example, by the average intake of specific nutrients in the target population’s diet) is meaningful if directly relevant to the population of interest and should be considered, especially in studies with a focus on specific population groups based on geographical location, age, gender, reproductive status, or socioeconomic variables (Bianchi et al., 2022). For example, regional weighting factors based on nutritional deficiencies/scarcity were applied to nutrient indices in studies conducted in Peru (Avadí and Fréon, 2015), Australia (Ridoutt, 2021) and Sweden (Hallström et al., 2019). Future research might also consider how such nLCA-based meal rankings change when considering the cost of food and affordability, as they have a direct bearing on consumer purchasing decisions (Headey and Alderman, 2019; Hirvonen et al., 2020).
Overall, this study showed that using an expanded nutritional index does not necessarily result in higher NRF scores and also does not alter the final rankings of the toppings in a ToT meal significantly. However, some of the toppings which have high proportions of nutrients not included in the NRF9.3 index move up a rank in the baseline scenario (e.g., avocados that are high in MUFAs, and eggs, which contain a large amount of PUFAs, MUFAs, and selenium). Thus, while the NRF9.3 index can generally identify the best, medium, and worst performing foods in this meal context, an expanded index could produce more nuanced rankings. More case studies are needed to understand how an expanded index would influence a larger and more diverse range of meals or foods within a meal context. In addition, investigations into the variability of different factors related to assessment of foods and meals (e.g., bioavailability of nutrients, target population nutrient deficiencies, agricultural production practices, seasonality, etc.) can offer more resolution to this nLCA ranking method, which can then be developed further for integration into new or existing tools for improved consumer decision-making. Moreover, although this study takes a primarily consumption-oriented perspective to sustainable nutrition, increased consumer demand for low impact, nutrient-rich meals could also drive systemic change in farming/production practices in the long-term.
Data availability statement
The raw data supporting the conclusions of this article will be made available by the authors, without undue reservation.
Author contributions
ShM: Writing – review & editing, Writing – original draft, Methodology, Investigation, Formal analysis, Data curation, Conceptualization. SaM: Writing – review & editing, Supervision, Methodology, Conceptualization. JP: Writing – review & editing, Methodology, Conceptualization. CL: Writing – review & editing, Methodology, Conceptualization.
Funding
The author(s) declare that no financial support was received for the research, authorship, and/or publication of this article.
Conflict of interest
The authors declare that the research was conducted in the absence of any commercial or financial relationships that could be construed as a potential conflict of interest.
Publisher’s note
All claims expressed in this article are solely those of the authors and do not necessarily represent those of their affiliated organizations, or those of the publisher, the editors and the reviewers. Any product that may be evaluated in this article, or claim that may be made by its manufacturer, is not guaranteed or endorsed by the publisher.
Supplementary material
The Supplementary material for this article can be found online at: https://www.frontiersin.org/articles/10.3389/fsufs.2024.1363565/full#supplementary-material
Footnotes
1. ^The NRn index is calculated as the sum or mean of the ratio of beneficial/qualifying nutrients relative to their associated reference values (e.g., Recommended Dietary Intake, RDI). The LIM index is expressed as the sum or mean of the ratio of disqualifying nutrients to their associated reference values (e.g., Upper Limit (UL) of intake).
2. ^In combined environmental and nutritional assessments such as nLCA studies, these two aspects can be assessed in parallel or in an integrated single score approach. With respect to integrated assessments in nLCA studies, the environmental impact of food is calculated relative to a unit of its nutritional value (e.g., Doran-Browne et al., 2015; González-García et al., 2018; Berardy et al., 2019; Chapa et al., 2020; Green et al., 2021; Strid et al., 2021).
3. ^Yeast spreads commonly consumed in the UK and Commonwealth countries, particularly Australia and New Zealand (Rozin and Siegal, 2003; Vriesekoop et al., 2022).
4. ^The fourth group in the guidelines is Grains – Grains (bread, rice, pasta, cereals), which in this study is toast and remains constant throughout the study.
5. ^The terms “serve size” and “portion size” are sometimes used interchangeably in literature to represent the quantity of food typically consumed by an individual, however they mean different things (Spanos et al., 2015). Serve size refers to the quantified (measured) value of a food product found on nutrition labels (National Heart Lung and Blood Institute, 2023), whereas portion size refers to the actual quantity that an individual consumes in one sitting for a meal or snack (The Academy of Nutrition and Dietics, 2023).
6. ^Biotin, molybdenum, and fluoride were excluded as there were no values available for these in the food composition data of the 14 toppings. Published NRVs were unavailable for chloride and sulfur. Some bioactive phytochemicals, like flavonoids and carotenoids, and food additives were excluded from the study because of lack of both available NRVs and standardized composition data.
7. ^These include six micronutrients which diets in LMICs are most frequently deficient in – iron, zinc, folate, vitamin B12, vitamin A, and calcium.
8. ^An exception to this is the U.S. Reference Amount Customarily Consumed (RACC) – a metric developed and mandated by the U.S. Food and Drug Administration (FDA) to roughly standardize actual serve sizes for a food product (Drewnowski, 2017; Berardy et al., 2019; Grigoriadis et al., 2021).
References
Aceves-Martins, M., Bates, R. L., Craig, L. C. A., Chalmers, N., Horgan, G., Boskamp, B., et al. (2022). Food-level analysis to identify dietary choices with the highest nutritional quality and lowest greenhouse gas emissions and price. Front. Nutr. 9, 1–11. doi: 10.3389/fnut.2022.851826
Adhikari, S., Schop, M., de Boer, I. J. M., and Huppertz, T. (2022). Protein quality in perspective: a review of protein quality metrics and their applications. Nutrients 14:947. doi: 10.3390/nu14050947
All Recipes . (2023). Allrecipes-About Us. Available at: https://www.allrecipes.com/about-us-6648102 (Accessed October 13, 2023).
Arsenault, J. E., Fulgoni, V. L., Hersey, J. C., and Muth, M. K. (2012). A novel approach to selecting and weighting nutrients for nutrient profiling of foods and diets. J. Acad. Nutr. Diet. 112, 1968–1975. doi: 10.1016/j.jand.2012.08.032
Avadí, A., and Fréon, P. (2015). A set of sustainability performance indicators for seafood: direct human consumption products from Peruvian anchoveta fisheries and freshwater aquaculture. Ecol. Indic. 48, 518–532. doi: 10.1016/j.ecolind.2014.09.006
Bailey, H. M., and Stein, H. H. (2019). Can the digestible indispensable amino acid score methodology decrease protein malnutrition. Anim. Front. Rev. Magaz. Anim. Agric. 9, 18–23. doi: 10.1093/af/vfz038
Batlle-Bayer, L., Bala, A., Roca, M., Lemaire, E., Aldaco, R., and Fullana-i-Palmer, P. (2020). Nutritional and environmental co-benefits of shifting to “planetary health” Spanish tapas. J. Clean. Prod. 271:122561. doi: 10.1016/j.jclepro.2020.122561
Beal, T. (2024). Environmentally protective diets may come with trade-offs for micronutrient adequacy. Am. J. Clin. Nutr. doi: 10.1016/j.ajcnut.2024.01.028
Beal, T., Gardner, C. D., Herrero, M., Iannotti, L. L., Merbold, L., Nordhagen, S., et al. (2023). Friend or foe? The role of animal-source foods in healthy and environmentally sustainable diets. J. Nutr. 153, 409–425. doi: 10.1016/j.tjnut.2022.10.016
Beal, T., and Ortenzi, F. (2022). Priority micronutrient density in foods. Front. Nutr. 9:566. doi: 10.3389/fnut.2022.806566
Berardy, A., Johnston, C. S., Plukis, A., Vizcaino, M., and Wharton, C. (2019). Integrating protein quality and quantity with environmental impacts in life cycle assessment. Sustain. For. 11:10. doi: 10.3390/su11102747
Bianchi, M., Hallström, E., Parker, R. W. R., Mifflin, K., Tyedmers, P., and Ziegler, F. (2022). Assessing seafood nutritional diversity together with climate impacts informs more comprehensive dietary advice. Commun. Earth and Environ. 3:1. doi: 10.1038/s43247-022-00516-4
Bianchi, M., Strid, A., Winkvist, A., Lindroos, A.-K., Sonesson, U., and Hallström, E. (2020). Systematic evaluation of nutrition indicators for use within food LCA studies. Sustain. For. 12:21. doi: 10.3390/su12218992
Calderón, L. A., Herrero, M., Laca, A., and Díaz, M. (2018). Environmental impact of a traditional cooked dish at four different manufacturing scales: from ready meal industry and catering company to traditional restaurant and homemade. Int. J. Life Cycle Assess. 23, 811–823. doi: 10.1007/s11367-017-1326-7
Chapa, J., Farkas, B., Bailey, R. L., and Huang, J.-Y. (2020). Evaluation of environmental performance of dietary patterns in the United States considering food nutrition and satiety. Sci. Total Environ. 722:137672. doi: 10.1016/j.scitotenv.2020.137672
Clark, M., Hill, J., and Tilman, D. (2018). The diet, health, and environment trilemma. Annu. Rev. Environ. Resour. 43, 109–134. doi: 10.1146/annurev-environ-102017-025957
Clark, M. A., Springmann, M., Hill, J., and Tilman, D. (2019). Multiple health and environmental impacts of foods. Proc. Natl. Acad. Sci. U.S.A. 116, 23357–23362. doi: 10.1073/pnas.1906908116
Clark, M., Springmann, M., Rayner, M., Scarborough, P., Hill, J., Tilman, D., et al. (2022). Estimating the environmental impacts of 57,000 food products. Proc. Natl. Acad. Sci. U.S.A. 119:e2120584119. doi: 10.1073/pnas.2120584119
Coelho, C. R. V., Pernollet, F., and van der Werf, H. M. G. (2016). Environmental life cycle assessment of diets with improved omega-3 fatty acid profiles. PLoS One 11:e0160397. doi: 10.1371/journal.pone.0160397
Colombo, S. M., and Mazal, X. (2020). Investigation of the nutritional composition of different types of salmon available to Canadian consumers. J. Agric. Food Res. 2:100056. doi: 10.1016/j.jafr.2020.100056
Crippa, M., Solazzo, E., Guizzardi, D., Monforti-Ferrario, F., Tubiello, F. N., and Leip, A. (2021). Food systems are responsible for a third of global anthropogenic GHG emissions. Nat. Food 2, 198–209. doi: 10.1038/s43016-021-00225-9
Davis, J., Sonesson, U., Baumgartner, D. U., and Nemecek, T. (2010). Environmental impact of four meals with different protein sources: case studies in Spain and Sweden. Food Res. Int. 43, 1874–1884. doi: 10.1016/j.foodres.2009.08.017
De Smalen, A. W., Chan, Z. X., Abreu Lopes, C., Vanore, M., Loganathan, T., and Pocock, N. S. (2021). Developing an evidence assessment framework and appraising the academic literature on migrant health in Malaysia: a scoping review. BMJ Open 11:e041379. doi: 10.1136/bmjopen-2020-041379
Doran-Browne, N. A., Eckard, R. J., Behrendt, R., and Kingwell, R. S. (2015). Nutrient density as a metric for comparing greenhouse gas emissions from food production. Clim. Chang. 129, 73–87. doi: 10.1007/s10584-014-1316-8
Drew, J., Cleghorn, C., Macmillan, A., and Mizdrak, A. (2020). Healthy and climate-friendly eating patterns in the New Zealand context. Environ. Health Perspect. 128:017007. doi: 10.1289/EHP5996
Drewnowski, A. (2005). Concept of a nutritious food: toward a nutrient density score. Am. J. Clin. Nutr. 82, 721–732. doi: 10.1093/ajcn/82.4.721
Drewnowski, A. (2009). Defining nutrient density: development and validation of the nutrient rich foods index. J. Am. Coll. Nutr. 28, 421S–426S. doi: 10.1080/07315724.2009.10718106
Drewnowski, A. (2017). Uses of nutrient profiling to address public health needs: from regulation to reformulation. Proc. Nutr. Soc. 76, 220–229. doi: 10.1017/S0029665117000416
Drewnowski, A., Amanquah, D., and Gavin-Smith, B. (2021). Perspective: how to develop nutrient profiling models intended for global use: a manual. Adv. Nutr. 12, 609–620. doi: 10.1093/advances/nmab018
Drewnowski, A., Dwyer, J., King, J. C., and Weaver, C. M. (2019). A proposed nutrient density score that includes food groups and nutrients to better align with dietary guidance. Nutr. Rev. 77, 404–416. doi: 10.1093/nutrit/nuz002
Drewnowski, A., Maillot, M., and Darmon, N. (2009). Should nutrient profiles be based on 100 g, 100 kcal or serve size? Eur. J. Clin. Nutr. 63, 898–904. doi: 10.1038/ejcn.2008.53
Drewnowski, A., Rehm, C. D., Martin, A., Verger, E. O., Voinnesson, M., and Imbert, P. (2015). Energy and nutrient density of foods in relation to their carbon footprint. Am. J. Clin. Nutr. 101, 184–191. doi: 10.3945/ajcn.114.092486
Dudley, N., and Alexander, S. (2017). Agriculture and biodiversity: a review. Biodiversity 18, 45–49. doi: 10.1080/14888386.2017.1351892
Dutton, M. T., Lopez, I. A., Brown, C. P., and Simmons, J. V. (2015). Interdisciplinary synergy in the teaching of applied community-based research methods. Pedag. Health Promot. 1, 158–163. doi: 10.1177/2373379915580583
Eme, P. E., Douwes, J., Kim, N., Foliaki, S., and Burlingame, B. (2019). Review of methodologies for assessing sustainable diets and potential for development of harmonised indicators. Int. J. Environ. Res. Public Health 16:1184. doi: 10.3390/ijerph16071184
Erickson, J., and Slavin, J. (2015). Total, added, and free sugars: are restrictive guidelines science-based or achievable? Nutrients 7, 2866–2878. doi: 10.3390/nu7042866
Ernstoff, A., Tu, Q., Faist, M., Del Duce, A., Mandlebaum, S., and Dettling, J. (2019). Comparing the environmental impacts of meatless and meat-containing meals in the United States. Sustain. For. 11:22. doi: 10.3390/su11226235
Esteve-Llorens, X., Darriba, C., Moreira, M. T., Feijoo, G., and González-García, S. (2019). Towards an environmentally sustainable and healthy Atlantic dietary pattern: life cycle carbon footprint and nutritional quality. Sci. Total Environ. 646, 704–715. doi: 10.1016/j.scitotenv.2018.07.264
Falandysz, J. (2008). Selenium in edible mushrooms. J. Environ. Sci. Health Environ. Carcinog. Ecotoxicol. Rev. 26, 256–299. doi: 10.1080/10590500802350086
Fern, E. B., Watzke, H., Barclay, D. V., Roulin, A., and Drewnowski, A. (2015). The nutrient balance concept: a new quality metric for composite meals and diets. PLoS One 10:e0130491. doi: 10.1371/journal.pone.0130491
Fernández-Ríos, A., Laso, J., Campos, C., Ruiz-Salmón, I., Hoehn, D., Cristóbal, J., et al. (2021). Towards a water-energy-food (WEF) nexus index: a review of nutrient profile models as a fundamental pillar of food and nutrition security. Sci. Total Environ. 789:147936. doi: 10.1016/j.scitotenv.2021.147936
Food and Agriculture Organization of the United Nations (FAO) . (2010). Greenhouse gas emissions from the dairy sector: A life cycle assessment. Rome: FAO
Food and Agriculture Organization of the United Nations (FAO) . (2020). The State of Food and Agriculture 2020: Overcoming water challenges in agriculture. Rome: FAO.
Food and Agriculture Organization of the United Nations (FAO) and World Health Organization (WHO) . (2019). Sustainable healthy diets—Guiding principles. Rome: FAO
Food Standards Australia New Zealand (FSANZ) . (2021). Australia New Zealand Food Standards Code – Standard 1.2.8, Nutrition information requirements. Rome: FAO Available at: https://www.foodstandards.gov.au/food-standards-code (Accessed September 5, 2023).
Fulgoni, V. L. III, Keast, D. R., and Drewnowski, A. (2009). Development and validation of the nutrient-rich foods index: a tool to measure nutritional quality of foods. J. Nutr. 139, 1549–1554. doi: 10.3945/jn.108.101360
García-Herrero, L., De Menna, F., and Vittuari, M. (2019). Food waste at school. The environmental and cost impact of a canteen meal. Waste Manag. 100, 249–258. doi: 10.1016/j.wasman.2019.09.027
Global Nutrition Report . (2021). Global Nutrition Report: The state of global nutrition. Available at: https://globalnutritionreport.org/reports/2021-global-nutrition-report/health-and-environmental-impacts-of-diets-worldwide/
Global Panel on Agriculture and Food Systems for Nutrition . (2020). Foresight 2.0. Future food systems: For people, planet, and prosperity. Global Panel on Agriculture and Food Systems for Nutrition. Available at: https://foresight.glopan.org/
González-García, S., Esteve-Llorens, X., Moreira, M. T., and Feijoo, G. (2018). Carbon footprint and nutritional quality of different human dietary choices. Sci. Total Environ. 644, 77–94. doi: 10.1016/j.scitotenv.2018.06.339
Graham, F., Russell, J., Holdsworth, M., Menon, M., and Barker, M. (2019). Exploring the relationship between environmental impact and nutrient content of sandwiches and beverages available in Cafés in a UK University. Sustain. For. 11:190. doi: 10.3390/su11113190
Green, A., Nemecek, T., Chaudhary, A., and Mathys, A. (2020). Assessing nutritional, health, and environmental sustainability dimensions of Agri-food production. Glob. Food Sec. 26:100406. doi: 10.1016/j.gfs.2020.100406
Green, A., Nemecek, T., and Mathys, A. (2023). A proposed framework to develop nutrient profiling algorithms for assessments of sustainable food: the metrics and their assumptions matter. Int. J. Life Cycle Assess. 28, 1326–1347. doi: 10.1007/s11367-023-02210-9
Green, A., Nemecek, T., Smetana, S., and Mathys, A. (2021). Reconciling regionally-explicit nutritional needs with environmental protection by means of nutritional life cycle assessment. J. Clean. Prod. 312:127696. doi: 10.1016/j.jclepro.2021.127696
Grigoriadis, V., Nugent, A., and Brereton, P. (2021). Working towards a combined measure for describing environmental impact and nutritive value of foods: a review. Trends Food Sci. Technol. 112, 298–311. doi: 10.1016/j.tifs.2021.03.047
Gu, D., Andreev, K., and Dupre, M. E. (2021). Major trends in population growth around the world. China CDC Week. 3, 604–613. doi: 10.46234/ccdcw2021.160
Guan, V. X., Neale, E. P., and Probst, Y. C. (2022). Consumption of avocado and associations with nutrient, food and anthropometric measures in a representative survey of Australians: a secondary analysis of the 2011–2012 National Nutrition and physical activity survey. Br. J. Nutr. 128, 932–939. doi: 10.1017/S0007114521003913
Guo, A., Bryngelsson, S., Strid, A., Bianchi, M., Winkvist, A., and Hallström, E. (2022). Choice of health metrics for combined health and environmental assessment of foods and diets: a systematic review of methods. J. Clean. Prod. 365:132622. doi: 10.1016/j.jclepro.2022.132622
Hallström, E., Bergman, K., Mifflin, K., Parker, R., Tyedmers, P., Troell, M., et al. (2019). Combined climate and nutritional performance of seafoods. J. Clean. Prod. 230, 402–411. doi: 10.1016/j.jclepro.2019.04.229
Hallström, E., Carlsson-Kanyama, A., and Börjesson, P. (2015). Environmental impact of dietary change: a systematic review. J. Clean. Prod. 91, 1–11. doi: 10.1016/j.jclepro.2014.12.008
Hallström, E., Davis, J., Woodhouse, A., and Sonesson, U. (2018). Using dietary quality scores to assess sustainability of food products and human diets: a systematic review. Ecol. Indic. 93, 219–230. doi: 10.1016/j.ecolind.2018.04.071
Harrison, M. R., Palma, G., Buendia, T., Bueno-Tarodo, M., Quell, D., and Hachem, F. (2022). A scoping review of indicators for sustainable healthy diets. Front. Sustain. Food Syst. 5:263. doi: 10.3389/fsufs.2021.822263
Hawkins, I. W. (2019). The diet, health and environment trilemma In: ‘Environmental nutrition - Connecting health and nutrition with environmentally sustainable diets’. Ed. S. Joan (UK, USA: Elsevier), 151–171.
Headey, D. D., and Alderman, H. H. (2019). The relative caloric prices of healthy and unhealthy foods differ systematically across income levels and continents. J. Nutr. 149, 2020–2033. doi: 10.1093/jn/nxz158
Heller, M. C., Keoleian, G. A., and Willett, W. C. (2013). Toward a life cycle-based, diet-level framework for food environmental impact and nutritional quality assessment: a critical review. Environ. Sci. Technol. 47, 12632–12647. doi: 10.1021/es4025113
Henriksson, P. J. G., Cucurachi, S., Guinée, J. B., Heijungs, R., Troell, M., and Ziegler, F. (2021). A rapid review of meta-analyses and systematic reviews of environmental footprints of food commodities and diets. Glob. Food Sec. 28:100508. doi: 10.1016/j.gfs.2021.100508
Hirvonen, K., Bai, Y., Headey, D., and Masters, W. A. (2020). Affordability of the EAT–lancet reference diet: a global analysis. Lancet Glob. Health 8, e59–e66. doi: 10.1016/S2214-109X(19)30447-4
Hoolohan, C., Berners-Lee, M., McKinstry-West, J., and Hewitt, C. N. (2013). Mitigating the greenhouse gas emissions embodied in food through realistic consumer choices. Energy Policy 63, 1065–1074. doi: 10.1016/j.enpol.2013.09.046
Hornick, S. (1992). Factors affecting the nutritional quality of crops. Am. J. Altern. Agric. 7, 63–68. doi: 10.1017/S0889189300004471
Intergovernmental Science-Policy Platform on Biodiversity and Ecosystem Services (IPBES) (2019). Global assessment report on biodiversity and ecosystem services of the intergovernmental science-policy platform on biodiversity and ecosystem services. Bonn, Germany: IPBES secretariat
Jolliet, O. (2022). Integrating dietary impacts in food life cycle assessment. Front. Nutr. 9, 1–7. doi: 10.3389/fnut.2022.898180
Jones, A. D., Hoey, L., Blesh, J., Miller, L., Green, A., and Shapiro, L. F. (2016). A systematic review of the measurement of sustainable diets. Adv. Nutr. 7, 641–664. doi: 10.3945/an.115.011015
Kägi, T., Zschokke, M., and Dinkel, F. (2012). “Nutrient based functional unit for meals” in 8th international conference on LCA in the Agri-food sector (Rennes, France)
Katz-Rosene, R., Ortenzi, F., McAuliffe, G. A., and Beal, T. (2023). Levelling foods for priority micronutrient value can provide more meaningful environmental footprint comparisons. Commun. Earth Environ. 4:287. doi: 10.1038/s43247-023-00945-9
Kyttä, V., Kårlund, A., Pellinen, T., Pietiläinen, O., Tuomisto, H. L., Kolehmainen, M., et al. (2023). Product-group-specific nutrient index as a nutritional functional unit for the life cycle assessment of protein-rich foods. Int. J. Life Cycle Assess. 28, 1672–1688. doi: 10.1007/s11367-023-02217-2
Lukas, M., Rohn, H., Lettenmeier, M., Liedtke, C., and Wiesen, K. (2016). The nutritional footprint – integrated methodology using environmental and health indicators to indicate potential for absolute reduction of natural resource use in the field of food and nutrition. J. Clean. Prod. 132, 161–170. doi: 10.1016/j.jclepro.2015.02.070
Maares, M., and Haase, H. (2020). A guide to human zinc absorption: general overview and recent advances of in vitro intestinal models. Nutrients 12:762. doi: 10.3390/nu12030762
Martínez-Blanco, J., Antón, A., Rieradevall, J., Castellari, M., and Muñoz, P. (2011). Comparing nutritional value and yield as functional units in the environmental assessment of horticultural production with organic or mineral fertilization. Int. J. Life Cycle Assess. 16, 12–26. doi: 10.1007/s11367-010-0238-6
Masset, G., Soler, L. G., Vieux, F., and Darmon, N. (2014). Identifying sustainable foods: the relationship between environmental impact, nutritional quality, and prices of foods representative of the French diet. J. Acad. Nutr. Diet. 114, 862–869. doi: 10.1016/j.jand.2014.02.002
Masset, G., Vieux, F., and Darmon, N. (2015). Which functional unit to identify sustainable foods? Public Health Nutr. 18, 2488–2497. doi: 10.1017/S1368980015000579
Mazac, R., Järviö, N., and Tuomisto, H. L. (2023). Environmental and nutritional life cycle assessment of novel foods in meals as transformative food for the future. Sci. Total Environ. 876:162796. doi: 10.1016/j.scitotenv.2023.162796
McAuliffe, G. A., Takahashi, T., Beal, T., Huppertz, T., Leroy, F., Buttriss, J., et al. (2022). Protein quality as a complementary functional unit in life cycle assessment (LCA). Int. J. Life Cycle Assess. 28, 146–155. doi: 10.1007/s11367-022-02123-z
McAuliffe, G. A., Takahashi, T., and Lee, M. R. F. (2018). Framework for life cycle assessment of livestock production systems to account for the nutritional quality of final products. Food Energy Secur. 7:e00143. doi: 10.1002/fes3.143
McLaren, S., Berardy, A., Henderson, A., Holden, N., Huppertz, T., Jolliet, O., et al. (2021). Integration of environment and nutrition in life cycle assessment of food Items: opportunities and challenges.
Mditshwa, A., Magwaza, L. S., Tesfay, S. Z., and Mbili, N. (2017). Postharvest quality and composition of organically and conventionally produced fruits: a review. Sci. Hortic. 216, 148–159. doi: 10.1016/j.scienta.2016.12.033
Meier, T., and Christen, O. (2013). Environmental impacts of dietary recommendations and dietary styles: Germany as an example. Environ. Sci. Technol. 47, 877–888. doi: 10.1021/es302152v
Mekonnen, M. M., and Hoekstra, A. Y. (2014). Water footprint benchmarks for crop production: a first global assessment. Ecol. Indic. 46, 214–223. doi: 10.1016/j.ecolind.2014.06.013
Melse-Boonstra, A. (2020). Bioavailability of micronutrients from nutrient-dense whole foods: zooming in on dairy, vegetables, and fruits. Front. Nutr. 7:101. doi: 10.3389/fnut.2020.00101
Ministry of Health . (2020). Eating and activity guidelines for New Zealand adults—2020. Available at: https://www.health.govt.nz/publication/eating-and-activity-guidelines-new-zealand-adults
Montgomery, D. R., Biklé, A., Archuleta, R., Brown, P., and Jordan, J. (2022). Soil health and nutrient density: preliminary comparison of regenerative and conventional farming. PeerJ 10:e12848. doi: 10.7717/peerj.12848
Moughan, P. J. (2021). Population protein intakes and food sustainability indices: the metrics matter. Glob. Food Sec. 29:100548. doi: 10.1016/j.gfs.2021.100548
Mozaffarian, D., Micha, R., and Wallace, S. (2010). Effects on coronary heart disease of increasing polyunsaturated fat in place of saturated fat: a systematic review and meta-analysis of randomized controlled trials. PLoS Med. 7:e1000252. doi: 10.1371/journal.pmed.1000252
National Health and Medical Research Council . (2013a). Educator guide - information for nutrition educators. Available at: https://catalogue.nla.gov.au/catalog/6775249
National Health and Medical Research Council . (2013b). Eat for health. Australian dietary guidelines. Providing the scientific evidence for healthier Australian diets. Available at: https://www.eatforhealth.gov.au/guidelines/guidelines
National Heart Lung and Blood Institute . (2023). Serve sizes and portions. Available at: https://www.nhlbi.nih.gov/health/educational/wecan/eat-right/distortion.htm (Accessed October 7, 2023).
National Medical Health and Research Council . (2017). Nutrient reference values (NRVs) for Australia and New Zealand including recommended dietary intakes. Available at: https://www.nhmrc.gov.au/about-us/publications/nutrient-reference-values-australia-and-new-zealand-including-recommended-dietary-intakes
New Zealand Food Composition Database (NZFCD) . (2022). New Zealand food composition database online search. The New Zealand Institute for Plant and Food Research Limited and Ministry of Health. Available at: https://www.foodcomposition.co.nz/search
Notarnicola, B., Sala, S., Anton, A., McLaren, S. J., Saouter, E., and Sonesson, U. (2017). The role of life cycle assessment in supporting sustainable Agri-food systems: a review of the challenges. J. Clean. Prod. 140, 399–409. doi: 10.1016/j.jclepro.2016.06.071
Notarnicola, B., Tassielli, G., Renzulli, P. A., and Giudice, A. L. (2015). “Life cycle assessment in the Agri-food sector: an overview of its key aspects, international initiatives, certification, labelling schemes and methodological issues” in Life cycle assessment in the Agri-food sector. eds. B. Notarnicola, R. Salomone, L. Petti, P. A. Renzulli, R. Roma, and A. K. Cerutti (Switzerland: Springer International Publishing)
O'Neill, A . (2023). Age structure in New Zealand 2022. Available at: https://www.statista.com/statistics/436395/age-structure-in-new-zealand/ (Accessed September 13, 2023).
Oonincx, D., and de Boer, I. (2012). Environmental impact of the production of mealworms as a protein source for humans—a life cycle assessment. PLoS One 7:e51145. doi: 10.1371/journal.pone.0051145
Plate My Meal . (2023). Plate my meal – plate my meal. Available at: https://en.platemymeal.com/
Ravaut, G., Légiot, A., Bergeron, K.-F., and Mounier, C. (2021). Monounsaturated fatty acids in obesity-related inflammation. Int. J. Mol. Sci. 22:330. doi: 10.3390/ijms22010330
Ridoutt, B. (2021). An alternative nutrient rich food index (NRF-ai) incorporating prevalence of inadequate and excessive nutrient intake. Food Secur. 10:156. doi: 10.3390/foods10123156
Rozin, P., and Siegal, M. (2003). Vegemite as a marker of national identity. Gastronomica 3, 63–67. doi: 10.1525/gfc.2003.3.4.63
Saarinen, M., Fogelholm, M., Tahvonen, R., and Kurppa, S. (2017). Taking nutrition into account within the life cycle assessment of food products. J. Clean. Prod. 149, 828–844. doi: 10.1016/j.jclepro.2017.02.062
Saarinen, M., Kurppa, S., Virtanen, Y., Usva, K., Mäkelä, J., and Nissinen, A. (2012). Life cycle assessment approach to the impact of home-made, ready-to-eat and school lunches on climate and eutrophication. J. Clean. Prod. 28, 177–186. doi: 10.1016/j.jclepro.2011.11.038
Sala, S., Anton, A., McLaren, S. J., Notarnicola, B., Saouter, E., and Sonesson, U. (2017). In quest of reducing the environmental impacts of food production and consumption. J. Clean. Prod. 140, 387–398. doi: 10.1016/j.jclepro.2016.09.054
Salazar, M. B., Cai, H., Bailey, R., and Huang, J. Y. (2019). Defining nutritionally and environmentally healthy dietary choices of omega-3 fatty acids. J. Clean. Prod. 228, 1025–1033. doi: 10.1016/j.jclepro.2019.04.359
Sameshima, H., Akamatsu, R., Hayashi, F., and Takemi, Y. (2023). Estimation of greenhouse gas emissions from Japanese healthy meals with different protein sources. Front. Sustain. Food Syst. 7:198. doi: 10.3389/fsufs.2023.1232198
Scarborough, P., Arambepola, C., Kaur, A., Bhatnagar, P., and Rayner, M. (2010). Should nutrient profile models be ‘category specific’ or ‘across-the-board’? A comparison of the two systems using diets of British adults. Eur. J. Clin. Nutr. 64, 553–560. doi: 10.1038/ejcn.2010.31
Schaubroeck, T., Ceuppens, S., Luong, A. D., Benetto, E., De Meester, S., Lachat, C., et al. (2018). A pragmatic framework to score and inform about the environmental sustainability and nutritional profile of canteen meals, a case study on a university canteen. J. Clean. Prod. 187, 672–686. doi: 10.1016/j.jclepro.2018.03.265
Shkembi, B., and Huppertz, T. (2022). Calcium absorption from food products: food matrix effects. Nutrients 14:180. doi: 10.3390/nu14010180
Sonesson, U., Davis, J., Flysjö, A., Gustavsson, J., and Witthöft, C. (2017). Protein quality as functional unit – a methodological framework for inclusion in life cycle assessment of food. J. Clean. Prod. 140, 470–478. doi: 10.1016/j.jclepro.2016.06.115
Spanos, S., Kenda, A. S., and Vartanian, L. R. (2015). Can serving-size labels reduce the portion-size effect? A pilot study. Eat. Behav. 16, 40–42. doi: 10.1016/j.eatbeh.2014.10.007
Springmann, M., Clark, M., Mason-D’Croz, D., Wiebe, K., Bodirsky, B. L., Lassaletta, L., et al. (2018a). Options for keeping the food system within environmental limits. Nature 562, 519–525. doi: 10.1038/s41586-018-0594-0
Springmann, M., Wiebe, K., Mason-D’Croz, D., Sulser, T. B., Rayner, M., and Scarborough, P. (2018b). Health and nutritional aspects of sustainable diet strategies and their association with environmental impacts: a global modelling analysis with country-level detail. Lancet Planet. Health 2, e451–e461. doi: 10.1016/S2542-5196(18)30206-7
Stats, NZ . (2023). National Population Estimates: at 30 June 2023. Available at: https://www.stats.govt.nz/information-releases/national-population-estimates-at-30-june-2023/:text=at30June2020233A37.1and39.0yearsrespectively (Accessed September 13, 2023).
Strid, A., Hallström, E., Sonesson, U., Sjons, J., Winkvist, A., and Bianchi, M. (2021). Sustainability indicators for foods benefiting climate and health. Sustain. For. 13:17. doi: 10.3390/su13073621
Sturtewagen, L., De Soete, W., Dewulf, J., Lachat, C., Lauryssen, S., Heirman, B., et al. (2016). Resource use profile and nutritional value assessment of a typical Belgian meal, catered or home cooked, with pork or Quorn™ as protein source. J. Clean. Prod. 112, 196–204. doi: 10.1016/j.jclepro.2015.09.006
Takacs, B., Stegemann, J. A., Kalea, A. Z., and Borrion, A. (2022). Comparison of environmental impacts of individual meals—does it really make a difference to choose plant-based meals instead of meat-based ones? J. Clean. Prod. 379:134782. doi: 10.1016/j.jclepro.2022.134782
The Academy of Nutrition and Dietics . (2023). Serve size vs portion size: is there a difference? Available at: https://www.eatright.org/health/wellness/nutrition-panels-and-food-labels/serving-size-vs-portion-size-is-there-a-difference (Accessed October 4, 2023).
thinkstep-ANZ . (2023). LCA of assessment of New Zealand-farmed King salmon. Available at: https://www.thinkstep-anz.com/resrc/case-studies/lca-nz-farmed-king-salmon/
Tongpool, R., and Pongpat, P. (2013). Analysis of shiitake environmental performance via life cycle assessment. Int. J. Environ. Sci. Dev. 4, 552–557. doi: 10.7763/IJESD.2013.V4.412
U.S. Department of Agriculture . (2023). My plate. Available at: https://www.myplate.gov/ (Accessed December 4, 2023).
Ueawiwatsakul, S., Mungcharoen, T., and Tongpool, R. (2014). Life cycle assessment of Sajor-caju mushroom (Pleurotus Sajor-caju) from different sizes of farms in Thailand. Int. J. Environ. Sci. Dev. 5, 435–439. doi: 10.7763/IJESD.2014.V5.523
United Nations Framework Convention on Climate Change (UNFCCC) . (2023a). Climate action and COP28 - What is the global stock take? Available at: https://unfccc.int/ (Accessed December 2, 2023).
United Nations Framework Convention on Climate Change (UNFCCC) . (2023b). Embracing sustainable agriculture: leaders unite in the UAE declaration, prioritizing resilient food systems and climate action. Available at: https://www.cop28.com/en/food-and-agriculture (Accessed December 1, 2023).
University of Otago and Ministry of Health . (2011). A focus on nutrition: key findings of the 2008/09 New Zealand adult nutrition survey. Available at: https://www.health.govt.nz/publication/focus-nutrition-key-findings-2008-09-nz-adult-nutrition-survey
Van Kernebeek, H. R. J., Oosting, S. J., Feskens, E. J. M., Gerber, P. J., and De Boer, I. J. M. (2014). The effect of nutritional quality on comparing environmental impacts of human diets. J. Clean. Prod. 73, 88–99. doi: 10.1016/j.jclepro.2013.11.028
Verdinelli, S., and Scagnoli, N. I. (2013). Data display in qualitative research. Int J Qual Methods 12, 359–381. doi: 10.1177/160940691301200117
Vermeulen, S. J., Campbell, B. M., and Ingram, J. S. I. (2012). Climate change and food systems. Annu. Rev. Environ. Resour. 37, 195–222. doi: 10.1146/annurev-environ-020411-130608
Vieux, F., Soler, L.-G., Touazi, D., and Darmon, N. (2013). High nutritional quality is not associated with low greenhouse gas emissions in self-selected diets of French adults. Am. J. Clin. Nutr. 97, 569–583. doi: 10.3945/ajcn.112.035105
Virtanen, Y., Kurppa, S., Saarinen, M., Katajajuuri, J.-M., Usva, K., Mäenpää, I., et al. (2011). Carbon footprint of food – approaches from national input–output statistics and a LCA of a food portion. J. Clean. Prod. 19, 1849–1856. doi: 10.1016/j.jclepro.2011.07.001
Vriesekoop, F., Russell, C., Tziboula-Clarke, A., Jan, C., Bois, M., Farley, S., et al. (2022). The iconisation of yeast spreads—love them or hate them. Beverages 8:15. doi: 10.3390/beverages8010016
Wang, M., Zheng, Y., Khuong, T., and Lovatt, C. J. (2012). Effect of harvest date on the nutritional quality and antioxidant capacity in ‘Hass’ avocado during storage. Food Chem. 135, 694–698. doi: 10.1016/j.foodchem.2012.05.022
Weidema, B. P., and Stylianou, K. S. (2020). Nutrition in the life cycle assessment of foods—function or impact? Int. J. Life Cycle Assess. 25, 1210–1216. doi: 10.1007/s11367-019-01658-y
Willett, W., Rockström, J., Loken, B., Springmann, M., Lang, T., Vermeulen, S., et al. (2019). Food in the Anthropocene: the EAT–lancet commission on healthy diets from sustainable food systems. Lancet 393, 447–492. doi: 10.1016/S0140-6736(18)31788-4
World Health Organization (WHO) . (2015). Guideline: sugars intake for adults and children. Geneva: World Health Organization.
World Health Organization (WHO) . (2023). Total fat intake for the prevention of unhealthy weight gain in adults and children: WHO guideline. Geneva: World Health Organization.
Yusuf, E. H. (2023). Comparison of life cycle assessments and nutritional contents of soy protein and wheat protein (seitan) based vegan bacon products for human and environmental health. J. Sci. Food Agric. 103, 3315–3321. doi: 10.1002/jsfa.12507
Keywords: nLCA, life cycle assessment, nutrition, climate change, meals
Citation: Majumdar S, McLaren SJ, van der Pols JC and Lister CE (2024) An nLCA approach to support consumer meal decisions: a New Zealand case study of toppings on toast. Front. Sustain. Food Syst. 8:1363565. doi: 10.3389/fsufs.2024.1363565
Edited by:
Som Dutt, Central Potato Research Institute (ICAR), IndiaReviewed by:
Marina Mefleh, American University of Rome, ItalyClaudia Terezia Socol, University of Oradea, Romania
Copyright © 2024 Majumdar, McLaren, van der Pols and Lister. This is an open-access article distributed under the terms of the Creative Commons Attribution License (CC BY). The use, distribution or reproduction in other forums is permitted, provided the original author(s) and the copyright owner(s) are credited and that the original publication in this journal is cited, in accordance with accepted academic practice. No use, distribution or reproduction is permitted which does not comply with these terms.
*Correspondence: Shreyasi Majumdar, cy5tYWp1bWRhckBtYXNzZXkuYWMubno=