- 1Dr. Reddy’s Foundation, Hyderabad, India
- 2Center for Rural Development and Innovative Sustainable Technology, Indian Institute of Technology Kharagpur, Kharagpur, India
Purpose: The present study aims to identify the crucial determinants of the adoption of zero-tillage (ZT) technology in maize production in peninsular India. The study also measures the impact of ZT adoption on maize yield, income generation, and the expenses associated with different agricultural operations.
Methodology: The study used multi-stage stratified random sampling and conducted a face-to-face questionnaire survey to collect primary data from 1,189 maize farmers. Initially, the study employed probit regression analysis to identify the ZT adoption determinants. Subsequently, using the Propensity Score Matching (PSM) approach, the study measures the impact of ZT adoption over conventional tillage in terms of yield, income, and cost management. Finally, the Endogenous Switch Regression (ESR) method was implemented to mitigate unobserved heterogeneity and sample selection bias. Additionally, ESR assessed the robustness of PSM results.
Findings: The probit model identifies that variables like education, institutional credit adoption, crop insurance, visit of extension agent, landholding size, and prior experience of new technology adoption positively influence ZT adoption. The PSM and ESR approach results suggest that ZT adoption positively impacts farmers’ yield and net income while reducing cultivation costs and labor use. Results show that ZT adoption decreases the cost of land preparation, weed, pest management, and harvesting by INR 2708 acre−1, INR 167 acre−1, and INR 649 acre−1, respectively, thereby decreasing the overall cultivation cost by INR 8376 acre−1. However, seed and seed treatment costs and irrigation costs improve by INR 108 acre−1 and 176 acre−1 due to the adoption of ZT in maize cultivation. Moreover, ZT improves maize yield by 2.53 quintal acre−1 and minimises 9.56 person-days acre−1. ESR results suggest that the net return from maize cultivation is 26.1% higher for ZT adopters than conventional farmers. Additionally, ZT adopters can save 8.23 man-days acre−1, providing additional monetary benefits of INR 3259 acre−1 compared to ZT non-adopters.
Practical implications: The study findings may support policymakers in designing suitable agricultural policies to improve technology adoption and motivate small and marginal maize farmers for sustainable production.
1 Introduction
Maize is a versatile agricultural commodity cultivated across diverse agroecological regions in both rabi and kharif seasons to ensure food and nutritional security in India. India ranks fourth in total maize cultivation globally, with a recorded 22.26 million tons of production on 8.71 million hectares of arable land (GOI, 2015). Maize is predominantly used for animal feed (63%), followed by human food (23%), and industrial purposes (Yadav et al., 2014). During the preceding decade (2003–04 to 2012–13), maize cultivation grew by 1.8%, and yields increased by 4.9%, indicating an annual increase in productivity of 2.6% in India (GOI, 2015). Andhra Pradesh is one of the major states in India for maize cultivation. In 2020–21, 0.3 million hectares of the state were under maize cultivation and produced 1.8 million tonnes of maize, i.e., 6.09% of the country’s total production (GOI, 2021). Previously, maize was assessed as a potential substitute for rice under a conventional rice-wheat cropping system (Parihar et al., 2018). However, its economic viability is compromised by its comparatively lower yield and higher production cost.
Furthermore, traditional maize cultivation practices involve deep tillage to prepare seed beds, resulting in soil degradation, organic matter loss, and less water retention. Therefore, conventional maize production technologies are less water-efficient (Parihar et al., 2016; Das et al., 2018; Sayed et al., 2020), less cost-effective (Jat et al., 2014), and deteriorate soil health (Jat et al., 2013) when compared with minimum or zero tillage (ZT) practices. Additionally, studies have reported that ZT practices increase water and crop productivity while decreasing cultivation costs in rice-maize cropping systems. Reducing production expenses and eliminating detrimental environmental effects in maize production is imperative.
There are several alternatives to traditional agricultural practices aimed at mitigating the environmental footprints. Among those, soil tillage should be prioritized to improve soil health and agricultural sustainability (FAO, 2017).
Agriculture relies heavily on tillage, the most energy-intensive process (Sun et al., 2022). Reducing tillage, such as ZT,1 has environmental benefits, such as mitigating CO2 emissions, improving soil structure, and enhancing carbon sequestration (Sharma et al., 2011; Sefeedpari et al., 2012; Lal, 2019). However, there is a severe apprehension that ZT systems may also reduce crop yield due to soil compaction, difficult weed control, and stratification of organic matters (Pittelkow et al., 2015; Peixoto et al., 2020), making farmers reluctant to adopt ZT systems (Księżak et al., 2018). Zero tillage often increases soil bulk density in the top soil layer (10–20 cm), hindering crop growth by reducing air-filled pore space (Šíp et al., 2013). Arvidsson et al. (2014) and Brennan et al. (2014) also reported that reduced or no tillage had no significant effect on grain yield during the first three years, which decreased in subsequent years. Furthermore, there are contrasting findings like reduced tillage may promote the proliferation of soil-borne pathogens due to residue accumulations while sequestering carbon, enhancing soil organic matter and nitrogen cycling processes, thereby contributing to climate change mitigation and improving crop yield (Dang et al., 2018; Blanco-Canqui and Wortmann, 2020; Guo et al., 2021; Wang et al., 2021). When majority of research focusses on crop developmental aspects and benefits to the soil environment, very few delve deep into the aspects favouring or discouraging the adoption of conventional and conservation tillage practices (Hobbs et al., 2008). We also observed that the reports measuring the impact of adopting conservation tillage (i.e., zero tillage) over conventional tillage in dry farming are very scanty. There is a need to evaluate yield with the associated costs under different farm operations and net income generated for different farmer groups with varying socio-economic profiles.
Although, zero/minimum tillage offers numerous benefits, like decreased fuel expenses, diversified micro-biota, enhanced soil macro-porosity, and improved water retention (Bottinelli et al., 2017; Mitchell et al., 2017; Sultan et al., 2017; Choudhary et al., 2018; Das et al., 2018; Zhao et al., 2019), it comes with some associated costs (like herbicides, irrigation) which has direct consequences on net income of small and marginal farmers. On the other hand, conventional tilling causes soil erosion and nutrient loss, which impairs the natural resource base. Zero tillage practices decrease soil evaporation due to stubble retention (Lampurlanés et al., 2016). Improved net photosynthesis rate and transient water use leading to a more balanced relationship among yield and yield components in winter crops like maize make them a strong candidate for ZT interventions (Wang et al., 2020). Despite having several environmental, ecological, and economic benefits, ZT’s adoption in India’s winter maize production is scarce, which is not a good sign for any agricultural economy. There might be different socio-economic and demographic characteristics restricting farmers from adopting ZT in the winter season. However, we could not find any significant study identifying the critical determinants in the adoption of ZT maize in the dryland ecosystem. Also, the available information from various research (see Table 1) frequently appears to be conflicting, resulting in challenges when attempting to derive meaningful interpretations.
To fulfill the research gaps mentioned above, this research aims to identify the constraints restricting farmers from adopting no-tillage interventions in maize production in the dryland ecosystem of peninsular India. Further, the study aims to measure the impact of ZT on the yield of winter maize and the effect on the costs incurred by different farm operations. As most of the past literature compared the impact of conventional and zero tillage based on experimental environments, our study uses household-level primary data to measure the impact of ZT adoption on maize farmers’ yield, cost of cultivation, income, and labor resource utilization.
2 Conceptual framework
We have divided the study into two stages based on the research objectives. In the first stage, based on past literature surveys and focused group discussions followed by standard statistical measures, we identify the various socio-economic and demographic variables that may play critical roles in farmers’ ZT adoption decisions. Finally, 17 independent variables were considered for further analysis. The study uses probit regression analysis and identifies the determinants that positively and negatively influence farmers’ adoption decisions. In the second stage, the study measures the impact of ZT adoption based on PSM (Stage 2A) and ESR (Stage 2B) approaches. In the PSM method, we consider two groups. Those households that adopted ZT for the last three years are categorised as a ‘treated group.’ Farmers from the same village who had an opportunity to adopt ZT but did not opt for it are categorised under the ‘control group.’ This study also employs the ESR model in stage 2B to overcome the econometric problems associated with the PSM model and check the PSM outcomes’ robustness. Under this model, we compare the expected outcomes of those farmers adopting the ZT with those who do not adopt the ZT. Similarly, two hypothetical groups are created to investigate the counterfactual case (see Figure 1).
3 Methodology
3.1 Estimation strategy
This study has considered a fundamental relationship to examine the impact of ZT adoption on the cost associated with various operations, the overall cost of cultivation, yield, net income, and labor use. We assume that such outcomes are a linear function of the vector of explanatory variables () and the ZT adoption dummy variable (). Thus, the linear regression equation can be expressed as:
Where is the mean outcome (i.e., operation-wise cost, overall cost of cultivation, net return, yield, and labor use), and is a dummy variable (0 or 1) used for ZT adoption; = 1 if the ZT is adopted and = 0, otherwise. The vector expresses the farm-level socio-economic and environmental characteristics of the farm household. Based on these attributes, farm households decide whether to adopt ZT for maize production. Thus, the ZT adoption decision is a farmer’s selection rather than random assignment. The index function used to evaluate the adoption of ZT by a farmer can be represented as:
Where Ci∗ is a latent variable that articulates the difference between the advantages gained from adopting ZT () and the advantage gained from not adopting the ZT (), the household should adopt ZT if = -> 0. Using farm-level and household characteristics () as explanatory variables, the term estimates the difference between the advantages obtained from adopting ZT. Here, expresses as the error term. From Equations 1, 2, it is evident that perhaps the relationship between ZT adoption and outcome (i.e., yield, cost of cultivation, the net return, and labor use) is interdependent. Therefore, ZT adoption may help in yield, cost of cultivation, the net return, and labor use. Conversely, farmers with better yield, income, and less labor use may be better disposed toward adopting ZT. Hence, the treatment assignment is not random, with a group of adopters being systematically different. Also, the chance of selection bias increases as the unobservable factors influence error terms of both the outcome equation () and the ZT adoption equation (). Consequently, the evaluation of the outcome equation (Equation 1) with ordinary least squares (OLS) guides toward a bias estimation.
The authors employed the Heckman two-step selection method and instrumental variable approach (IV) to overcome the above-mentioned problem. However, limitations are also present in both these approaches, like (i) the Heckman approach based on the restrictive assumption of normally distributed errors, (ii) the difficulty in identifying an instrumental variable for the IV approach, and (iii) both IV and Heckman method impose a linear functional form assumption which means that the coefficient of independent variables is same for ZT adopters and non-adopters. Using the PSM approach, one can easily overcome the limitations of the discussed parametric methods. The PSM method does not require any assumption about the functional form to specify the associations between the outcome and the anticipators of the outcome.
3.1.1 The matching method
The impact of ZT adoption on different outcome variables is identified with the estimation of the average treatment effect (). is defined through a counterfactual framework by Rosenbaum and Rubin (1983). According to this framework, is defined as:
Where represents the outcome of household that adopted the ZT maize; farmers without ZT are labelled as . When we estimate the impact of ZT adoption with the help of Equation 3, the biases occur either from or , as both are observed from an individual farmer but not from the same farmer. Hence, this observation can be expressed as Equation 4:
If is the probability of identifying a farmer with (ZT adopted), the average treatment effect can be explained as:
The impact of ZT adoption identified with Equation 5 explains that the entire sample is the weighted average of the effect of ZT adoption on the ZT acceptors (treated) and non-ZT acceptors (control), and their relative frequencies weigh each of them. However, a major problem associated with this estimation is the unobserved counterfactuals, like (i.e., non-adopters had they decided to adopt) and (i.e., adopters had they decided not to adopt) which are not considered in the estimation.
The unobserved counterfactual situation leads to missing data problems. The PSM method can address this problem as it abridges each farmer’s pre-treatment attributes into a single index variable and then calculates the propensity score (PS) to match a similar individual (Rosenbaum and Rubin, 1983). The PS can be calculated as Equation 6:
Where is the vector of pre-treatment attributes and is the cumulative logistic distribution. After estimating the PS, the average treatment effect on treated (ATT) can be computed as Equation 7:
This study uses three matching approaches to determine the treatment effect (ZT adoption) on treated (yield, cost of cultivation, net return, and labor use). Along with the nearest neighbor (NN) and radius matching approach, a non-parametric kernel-based approach is also used. In the NN matching approach, matching between treated subjects and an untreated subject whose PS is nearest to the treated one. However, if multiple untreated subjects consist of a score closest to the treated subject, any one of the untreated subjects is selected randomly. Five nearest-neighbor matching is introduced in this study so that all five untreated subjects having a PS nearest to the treated one can be incorporated to minimise the bias (Austin, 2011). In such an approach, the treated subject always matched the nearest untreated ones, although their PS differ widely. Therefore, there is always a chance of poor matching in some cases. Thus, computing the treatment effect using such a matching approach can be erroneous (Caliendo and Kopeinig, 2008). The study also incorporates a radius matching (RM) approach to retrieve the risk associated with NN matching. In such a matching approach, treated subjects are matched only with untreated subjects whose PS falls within the predefined radius—the smaller the radius, the better the matching quality (Becker and Ichino, 2002). Finally, the kernel matching (KM) method (non-parametric) somehow differs from these approaches. Here, the entire sample of untreated is used to match the means of treating one (Heckman et al., 1997).
3.1.2 Endogenous switching regression
This study has also employed the endogenous switching regression (ESR) model to overcome the sample selection bias (Heckman et al., 1997), endogeneity (Hausman, 1978), and missing data problem (unobserved counterfactual situation) associated with PSM. Using the ESR model, in addition to the PSM approach, not only helps to overcome the above-mentioned econometric problems but also checks the robustness of the PSM outcomes.
The selection equation for ZT adoption under the model can be specified as follows:
Where represents a latent variable for ZT adoption, Bi is the observable counterpart ( = 1; if the farmer adopts ZT for maize cultivation and 0 otherwise), is non-stochastic observed independent variables that specify both farm and non-farm characteristics, which in turn determine the ZT adoption decision of the farm household. is the random disturbance involved in the adoption of ZT.
The study employs the model on outcomes where the farmer faces two regimes: (i) to adopt ZT and (ii) not to adopt ZT to overcome the selection bias. These two regimes can be specified as follows:
Where denotes the overall cost of cultivation, yield, net income, and labor use for regimes 1 and 2. stands for the vector of independent (exogenous) variables influencing the outcome variables.
The error term associated with Equations 8, 9a, 9b is assumed to have a trivariate normal distribution with zero means. The non-singular covariate matrix is represented as Equation 10:
Where , , and are the variance of error terms associated with the selection equation (Equation 8), outcome equation of regime 1 (Equation 9a), and the outcome equation of regime 2 (Equation 9b), while and are designated as the covariance of and , respectively. As the selection equation’s (Equation 8) error term () is correlated with the outcome equations’ (Equations 9a, 9b) error term ( and ), the anticipated measures of and conditional on the sample selection is non-zero. Therefore, complete information maximum likelihood (FIML) effectively estimates the ESR model (Lokshin and Sajaia, 2004). The FIML method utilizes both the selection and regression equation to generate consistent standard errors. With the application of trivariate normal distribution in place of the error term, we can write the likelihood logarithmic function for Equations 8, 9a, 9b as Equation 11:
Where, , = 1,2 with represents the coefficient for the correlation between the error term of the selection equation () and the error term associated with Equations 9a, 9b. The parameters related to the FIML-based ESR model can be identified using the “movestay” command in STATA.
Thus, it can be written as Equations 12, 13:
Where and are the functions of normal probability and cumulative density, respectively. So, we can represent and as Equations 14, 15:
Using the ESR framework, we can compare the expected outcomes of those farmers accepting the ZT with those who did not adopt the ZT. Similarly, two hypothetical groups were created to investigate the counterfactual case. Nevertheless, the farmers who adopted ZT in the first year chose not to continue the practice in succeeding years. Another group is represented by farmers who have not adopted ZT but have decided to adopt it.
These four conditions can be represented as:
Adopters with adoption:
Non-adopters without adoption:
Adopters had they decided not to adopt (Counterfactual):
Non-adopters had they decided to adopt (Counterfactual):
Now, we can measure the treatment effect (adopt ZT) on the treated (TT) as the difference between Equations 16a, 16c.
Equation 17 represents the impact of ZT adoption on the overall cost of cultivation, yield, net income, and labor use for those farmers that indeed adopted ZT. Similarly, the difference between the outcomes for farmers who had not opted for the ZT (Equation 16b) with its counterfactual (Non-adopters had they decided to adopt; Equation 16c) is computed as treatment effect on untreated (TU).
According to the concept of Carter and Milon (2005), we define the base heterogeneity for this study. For the group of farmers that adopt ZT, the base heterogeneity for this group can be specified as the difference between Equations 19.
Similarly, for those groups of farmers who had decided not to adopt ZT, the base heterogeneity effect can be calculated as a difference between Equations 20.
Finally, the “transitional heterogeneity” (TH) is identified simply by calculating the difference between Equations 17, 18.
3.2 Study area and sample size
India cultivated maize on 9.9 million hectares and produced 31.51 million tonnes in 2020–21. Among the non-traditional maize states in India, Andhra Pradesh (AP) stands second in terms of area under maize cultivation (0.3 million ha) and production (1.78 million tonnes). The state’s agro-climatic conditions suit maize cultivation during the kharif and rabi seasons. However, farmers mainly produce paddy (rainfed) in the kharif season and choose dryland maize farming in rabi as the crop requires less irrigation than paddy. Maize production in AP accounts for 78.61% of the total production of coarse grains. A state-wise detailed analysis shows that more than 65% of the cost is incurred as variable costs in AP. A major chunk of this cost utilized labor and machinery costs during tillage (Jat et al., 2014). Hence, we found this state most suitable as our study area. In AP, out of 81 districts, Srikakulam district stands 3rd in Maize production (0.26 million tonnes in 2019–20), and more than 80% of the farmers are involved in ZT maize production during Rabi season. Like in other districts, most farmers keep a portion of the produce for their consumption and sell it in the domestic market. Hence, we purposively select this district for a face-to-face questionnaire survey (Figure 2).
According to Census (2011), the district’s farmers number was 2,266,411. Out of the total, the number of farmers who owned land and were directly involved in agriculture was 145,576. We employed Bartlett et al. (2002) sample size calculation formula to calculate an adequate sample size for the study:
Where N = Size of the population (2,266,411), n = size of the sample population; Z = confidence interval at 95% (Z = 1.96); d = error at 5% (d = 0.05); p = proportion of target population (p = 0.5); and q = 1−p (q = 0.5). We found that 384 household data were sufficient for this study. However, we surveyed 1,189 households from 20 blocks of Srikakulam district. From these 20 blocks, we selected only those villages for the questionnaire survey where ZT maize cultivation training was provided, and some farmers have already adopted the technique and have been producing maize using the technique for the last three years. Such criteria confirm that it is farmers’ choice whether to adopt ZT in maize cultivation, and it is crucial to overcome the selection bias and present the counterfactuals. Of the 1,189 households, 801 farmers adopted the ZT method, while 388 farmers followed the conventional tillage for maize cultivation.
4 Results and discussion
4.1 Descriptive statistics
The study uses 17 independent variables and 11 dependent variables for analysis (see Appendix 1). Table 2 shows the descriptive statistics and mean difference test for adopting and non-adopting farmers of the ZT technology. The result illustrates that the adopters and non-adopters significantly differ in cost associated with seed and seed treatment, land preparation, fertiliser application, weed management, pest management, harvest, and irrigation. Moreover, farmers adopting the ZT maize achieve 1.47 quintal acre−1 more yield, INR7047 acre−1 net return, and INR3939 acre−1 less cost of cultivation than farmers without ZT adoption. This implies that the benefits are not limited to input cost cutting. The farmers can reap more direct returns in the form of improved crop yield. Adopting ZT maize also saves 9.71 man-days more than conventional maize growers. Descriptive statistics also specify that ZT adopters differ significantly in socio-economic and demographic attributes. The descriptive outcomes indicate that farmers following ZT in maize cultivation have a higher education level, membership in farmers’ organisations, farm size (acre), institutional credit, crop insurance, prior experience in mechanisation, and risk-taking attitude than conventional maize farmers. We also report that farmers who are relatively aged, have a higher household size, and reside relatively far from the marketplace prefer conventional practices, which might be due to their low risk-taking ability.
4.2 Determinants of zero-tillage adoption
The probit model ascertains the factors that influence farmers’ ZT adoption. We estimate the Variance Inflation Factor (VIF) as a statistical measure to assess the presence of multicollinearity among the independent variables. According to Appendix 2, the VIF for the independent variables is below the specified threshold of 5. This suggests that the predictors exhibit no significant correlation with each other.
The limitations of ZT technology adoption are determined using a standard probit model. Table 3 shows a statistically significant inverse correlation between age and ZT maize adoption. A one-year increase in the mean age of the population is likely to decrease the chance of ZT maize adoption by 3.8%, which is in line with the findings of Ali et al. (2014). The probit analysis supports our assertion, which is drawn from the results of descriptive statistics, that younger farmers are likelier to adopt ZT technology than their elder counterparts. A potential rationale for this phenomenon could be that younger farms exhibit greater enthusiasm for adopting innovative technologies. In comparison, relatively aged farmers tend to adhere to conventional maize cultivation techniques and are reluctant to bear the risk of adopting novel technological advancements beyond their comfort zone (Marenya and Barrett, 2007; Läpple and Van Rensburg, 2011). However, our results are contrary to those of Sodjinou et al. (2015), who reported that the age of farmers positively affects their adoption of novel farming techniques.
Nevertheless, the impact of education on the acceptability of sustainable technology like ZT is of great importance, as demonstrated by a positive and statistically significant relationship between farmers’ educational levels and the adoption of ZT technology. A one-year increase in education year in the sample means an improvement in the probability of ZT adoption by 1.4%. This can be referred to the fact that farmers are inclined to adopt new technologies when they understand the new technology. At the same time, farmers who have received more formal education may exhibit an enhanced ability to comprehend the information disseminated by agricultural experts and extension agents and make informed decisions about new technologies (Ruzzante and Bilton, 2021). Also, farmers with a formal education better predict the potential ramifications of emerging technologies on both agricultural yield and financial profitability. Consequently, there is a favourable correlation between the educational level of the household head and their inclination to embrace innovative and sustainable methods in farming, such as ZT in maize farming. This observation aligns with the results reported by Duraisamy (2002), Idrisa et al. (2012), and Huffman (2001). According to Adeoti (2008) and Nonvide (2021), farmers with higher levels of education tend to have enhanced capabilities in adapting to new challenges and effectively utilising emerging technology.
The coefficient associated with membership in farmers’ organisations demonstrates a positive and statistically significant correlation with adopting ZT technology. This result aligns with the findings of Tura et al. (2010). Conley and Udry (2001, 2010) have provided evidence to support the notion that extension services and farmers’ groups are effective channels for disseminating information among agricultural producers and improving the chance of technology adoption. The results obtained in this study are consistent with the findings published in previous studies conducted by Abdulai et al. (2011), Allagbe and Biaou (2013), Barry (2016), and Seye et al. (2016). Membership in farmers’ organisations also brings economies of scale in cultivation and marketing (Trebbin and Hassler, 2012), minimises the cost of commercialisation, and improves production efficiency (Francesconi and Heerink, 2011; Bernard and Taffesse, 2012; Fischer and Qaim, 2014). It also enables the farmers to cope with the alteration in the global value chain and prevailing market inadequacies (Meinzen-Dick et al., 2004). Group/organisation membership serves as an indicator of social capital. Social networks enable producers to exchange information and participate in peer-to-peer learning. Social organisations served as an informal form of insurance during times of crisis. If members of the group support adopting any new technology, a positive attitude toward adoption is generated in farmers’ minds, which in turn helps in technology adoption. This may be why farmers with group membership are more inclined toward ZT adoption.
The results show that household size negatively affects the adoption of ZT maize. With a 1% increase in the average household size of the population, the probability of adopting the ZT maize decreases by 2.4%. This finding is consistent with the outcome reported by Dey and Singh (2023). Household size is a proxy for labor availability (Feder et al., 1985). If the household size is large, more family labor is available, and households choose labor-intensive technologies. Conventional maize production is a labor-intensive process. It requires additional labor, particularly for land preparation, weeding, and harvest. Therefore, farm families with larger household sizes choose conventional maize farming.
Meanwhile, relatively small families tend to adopt those technologies that minimise labor requirements. ZT does not require land preparation and stubble removal from the field. The sowing process is also machine-driven. Hence, the ZT technique is a less labor-dependent process. Therefore, households with fewer members tend to adopt this technology.
The study indicates a positive association between access to institutional financing and the likelihood of adopting agricultural technologies. The likelihood of ZT adoption increases by 17.1%, with the increase of institutional credit adoption by 1%. This finding is similar to the results by Tura et al. (2010) and Idrisa et al. (2012). Farmers who have obtained institutional loans are more inclined to employ agricultural technologies. Mdemu et al. (2016) and Nonvide et al. (2018) have identified a notable constraint in the use of technology due to a lack of access to formal financial services. The mentioned studies reported that financial resources could facilitate the acquisition of agricultural inputs and the implementation of innovative technologies in agriculture. The study identifies that farmers need to purchase the double-wheel marker to adopt ZT in maize production. Small and marginal farmers with limited resources find it difficult to invest money in mechanisation. However, if farmers receive financial support from formal institutions, they invest the money to purchase the required machines. This may be why farmers with access to formal credit are more inclined to adopt ZT.
The findings in Table 3 show a positive correlation between landholding size and the adoption of ZT. This implies that farmers who own larger farm sizes are more inclined to adopt ZT practices. The study findings exhibit a resemblance to Houeninvo et al. (2020), Tura et al. (2010), Ali et al. (2018), Abay et al. (2018), and Mwangi and Kariuki (2015). Furthermore, smallholder farmers may believe that using new technology may decrease their average yield, hindering their ability to attain food security and anticipated income. In contrast, relatively large landholders assign their land under the purview of both initiatives, i.e., ZT and conventional. Given the substantial scale of their farm, they possess a sense of assurance that in the event of a fall in yield resulting from using ZT, they can effectively offset any potential income losses and guarantee food security by implementing conventional maize.
A significant and positive association exists between the extension agent and ZT adoption. A 1% increase in the interaction with the extension agent improves the adoption of ZT maize by 8.3%. Extension service can be regarded as a viable alternative to formal education in promoting adopting certain practices (Feder et al., 1985; Nkamleu and Adesina, 2000). This service is found to be most effective in areas with high levels of education. Farmers learn more about the new technology by interacting with the extension agent. We observed that farmers can clear their doubts about the technology by face-to-face interaction with the extension agents. Trusted information from extension agents regarding higher production with less cultivation cost further inspires the farmers to adopt ZT in maize cultivation.
Farmers have various options available to mitigate agricultural risks. Our results indicate that adopting a specific risk management tool is always conducive to further including supplementary risk management techniques (Velandia et al., 2009). Furthermore, our findings suggest crop insurance favours adopting other risk management strategies, such as ZT, for maize production. We observed that farmers who choose to use ZT instead of conventional maize production also believe that if reduced crop output results from their lack of proficiency in implementing this new technique, crop insurance will be an extra safeguard against potential losses.
Successfully adopting new technologies in the past inspires and boosts faith in adopting smart technologies in the future (Mwombe et al., 2014). Perhaps this may be why farmers who have bought and used new technology in the past also adopt ZT maize farming. Results show that a 1% increase in the prior experience in adopting any new technology improves the chance of ZT adoption by 22.5%. Similarly, farmers who do not want to take risks follow conventional farming. However, a 1% increase in farmers’ risk-taking capacity improves the adoption of ZT maize by 12.7%.
4.3 Average impact of ZT adoption on the cost of cultivation, the net return, yield, and human resource utilization
Table 4 shows the average impact of ZT adoption on maize farmers’ cost of cultivation (at various stages of operations), yield, net income, and human resource utilization. The results further support our descriptive analysis that ZT adoption significantly decreases the cost of cultivation and improves maize yield and net income from maize production. ZT adopters are likely to achieve INR 8376 acre−1 more (average based on the matching algorithms) and save 9.5 man-days acre−1 than conventional farmers. Likewise, the yield of ZT farmers is 2.52 quintal ha−1 higher than conventional farmers. A detailed analysis of the operation-wise cost of cultivation shows that in ZT maize farming, land preparation cost, weed and pest management cost, and harvest cost are lower by INR 2708 acre−1, INR 167 acre−1, and INR 649 acre−1, respectively, than conventional farming. Nevertheless, seed and seed treatment costs and irrigation costs are higher by INR 108 acre−1 and INR 176 acre−1 for ZT adopters than non-adopters.
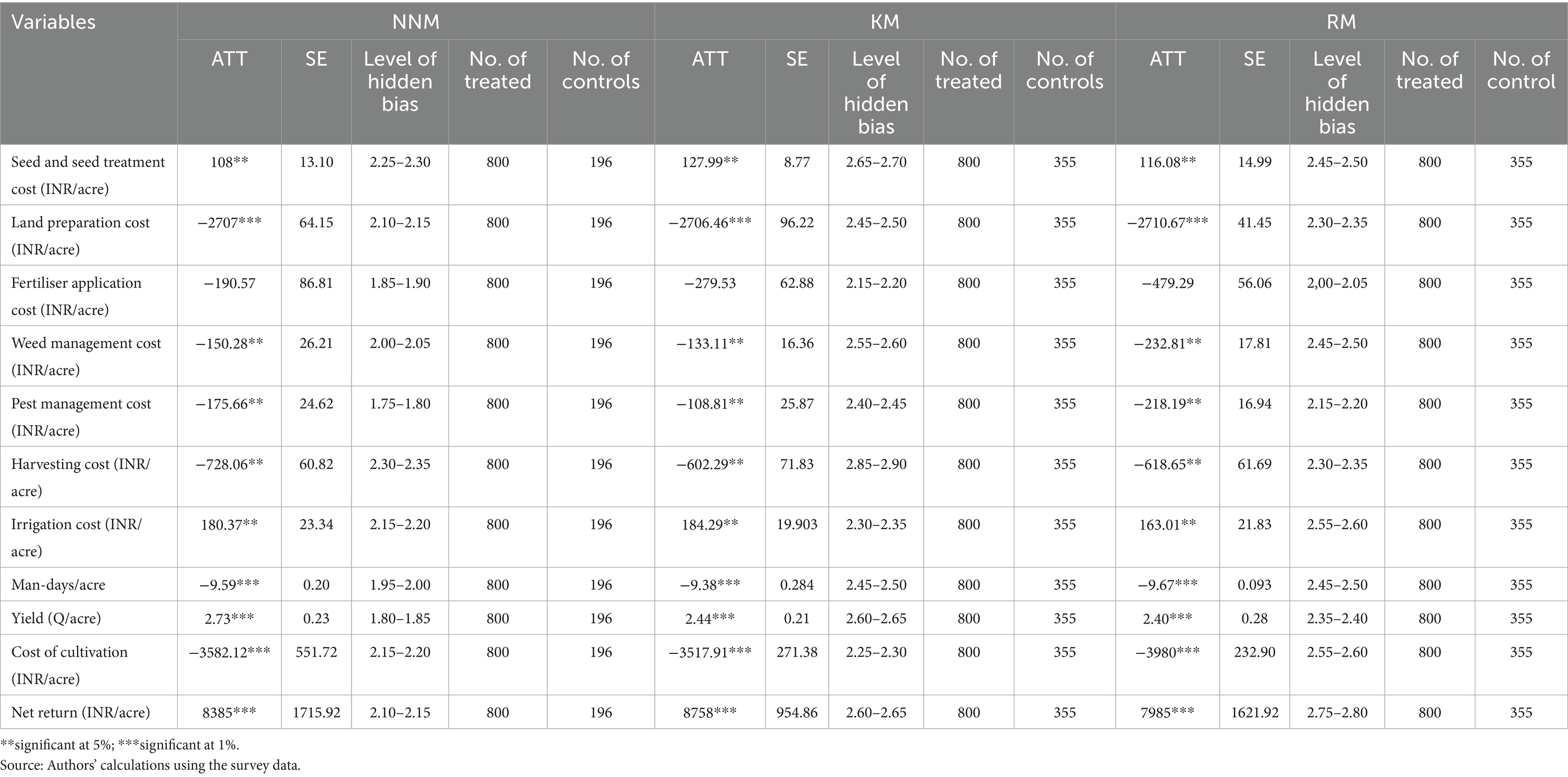
Table 4. Impact of ZT adoption on cost incurred in various farm practices, maize productivity, income, and human resource use.
The outcomes shown in Table 4 are contingent upon the assumption of conditional independence and confounding. We infer from the results that when an unobserved independent variable has the potential to impact both ZT adoption and outcome variables, there is a possibility of unobserved heterogeneity arising, which might potentially modify the importance of the impact (Rosenbaum and Rubin, 1983; Becker and Caliendo, 2007). However, determining the extent of hidden bias in nonexperimental studies poses challenges due to the absence of a suitable assessment instrument. We assessed the degree to which unobserved exogenous factors impact the significance of the estimate by employing the Rosenbaum-bounds-sensitivity calculation (DiPrete and Gangl, 2004; Caliendo and Kopeinig, 2008).
Our results indicate that each ATT value is linked to a corresponding critical level of hidden bias. This number represents a significant gamma level at which one might justify the causal inference of ZT adoption. An illustration of this may be seen in the gamma value range of 2.75–2.80 for net return (in radius matching). This range suggests that if farmers possess identical baseline variables, their likelihood of ZT adoption may increase by 175–180%. However, the extent to which ZT adoption positively affects net income from maize production may be subject to scrutiny. This implies that the magnitude of the concealed bias must be sufficiently high enough to impact the results. Additionally, the study acknowledges that most exogenous factors are treated as baseline covariates that influence the treatment and dependent variables.
4.4 ESR outcomes
Unobservable variables may introduce bias into the findings identified with the PSM technique. Therefore, this study has utilized the FILM-based ESR model to address the reliability and inherent biases associated with the PSM model. Table 5 displays the average treatment effect of ZT adoption based on the ESR method for a set of dependent variables, i.e., seed and seed treatment cost, land preparation cost, fertiliser cost, irrigation cost, disease and pest management cost, cost of cultivation, yield, the net return, and farm labor required under both actual and counterfactual scenarios.
Results show that both the PSM and the ESR methods yield comparable results in evaluating the effects of ZT adoption on various outcomes. The direction and difference between ATT and ATU are statistically significant for all those variables, where ATT associated with the outcome variable in PSM are also significant. The average improvement in seed and seed treatment costs for ZT adopters is INR 52 acre−1. Results show that seed and seed treatment costs are INR 2889 acre−1 for conventional farmers while INR 2996 acre−1 for ZT adopters. In the counterfactual scenario, if the ZT adopters decide not to continue ZT adoption, their seed and seed treatment costs will decrease by INR52 acre−1. The study identifies that seed rate does not differ significantly among the ZT adopters and non-adopters, which repudiates the myth that ZT requires less seed. However, ZT adopters mostly used pre-treated seed, which may be costlier than non-treated seed used by conventional farmers. The land preparation cost is 56% less for ZT adopters (INR 2122 acre−1) than non-adopters (INR 4831 acre−1). Also, the finding illustrates that if the conventional maize farmers (non-adopters) adopt the ZT practice in the future, they can save INR 2482 acre−1 for land preparation.
Similarly, ZT adopters can save 8.9 and 6.8% of the weed and pest management cost compared to conventional maize farmers. Considering the counterfactual scenarios, the study identifies that if the ZT non-adopters become ZT adopters, they can save INR 116 acre−1 and INR 115 acre−1 for weed and pest management, respectively. The harvesting cost is 11.9% less for ZT adopters (INR 4485 acre−1) than non-adopters (INR 5091 acre−1). Also, the finding illustrates that if the conventional maize farmers (non-adopters) adopt the ZT practice in the future, they can save INR 512 acre−1 under harvesting. Unlike harvest cost, irrigation cost is relatively higher for ZT adopters (INR 1300 acre−1) than conventional farmers (INR 1225 acre−1). Under the counterfactual scenario, if ZT adopters become non-adopters in the future, they can save 2.7% on the irrigation cost. However, the ZT adopters (27.56 quintal acre−1) achieve a 9.32% additional yield compared to non-ZT adopters (25.21 quintal acre−1). Outcomes also indicate that if conventional farmers adopt ZT practice, they can gain an additional yield of 1.37 quintal acre−1. Estimating the cost of cultivation suggests that it is INR 3462 acre−1 less for ZT adopters than conventional maize growers. If ZT adopters discontinue following ZT practice, their cost of cultivation will increase by INR 2891 acre−1. Similarly, the net return from maize cultivation is 26.1% higher for ZT adopters than conventional farmers. Considering counterfactual scenarios, our study revealed that conventional farmers must adopt ZT practice, which may significantly improve their net income from maize cultivation by 18.9%. The study implies that ZT adoption is beneficial in improving farmers’ net return in many ways. For instance, labor use can be significantly saved in ZT practice. Furthermore, if we monetise the gains, zero tillage can significantly improve the livelihood of maize farmers.
5 Conclusion
Concerns regarding the sustainability of agricultural resource utilization have increased significantly. From this perspective, conservation tillage technologies and practices have emerged as a substitute for conventional tillage. However, adopting conservation tillage (i.e., zero tillage) practices in peninsular India is still limited. Therefore, this study aims to identify the critical determinants of the widespread adoption of ZT technology in maize production. The study also measures the impact of ZT adoption on maize yield, income, cost of cultivation, and labor utilization. Using the probit regression analysis, the study identifies that education, institutional credit adoption, crop insurance, visit of extension agent, landholding size, and prior experience of new technology adoption positively influence ZT adoption. The impact assessment findings indicate that adopting ZT reduced the cost associated with land preparation, weed and pest management, and harvesting by INR 2708 acre−1, INR 167 acre−1, and INR 649 acre−1, respectively.
Consequently, this results in a drop in the overall cultivation cost by INR 8376 acre−1. Implementing ZT in maize production improves seed and seed treatment costs and irrigation expenses by INR 108 acre−1 and 176 acre−1, respectively. In addition, ZT enhances maize productivity by 2.53 quintal acre−1 and reduces person-days per acre by 9.56. The ESR findings indicate that ZT adopters achieve a net return from maize farming that is 26.1% greater than conventional farmers. Furthermore, adopters of ZT have the potential to conserve 8.23 man-days acre−1 than non-adopters of ZT practice.
ZT maize cultivation is not only a soil management and restoration technique; the current study also indicates that it improves maize yield and farmers’ income. Hence, promoting such conservation tillage supports the National Mission for Sustainable Agriculture and the National Food Security Mission. However, state and central governments must strengthen their extension services in rural areas to engage a wider farming community under ZT maize production. Government-regulated extension services providers like the Agricultural Technology Managing Agency, Krishi Vigyan Kendras, Agriculture Clinics and Agriculture Business Centres, and Kisan Call Centres must motivate smallholders to adopt ZT. Also, governments need to strengthen smallholders’ institutional credit linkage by effectively implementing the KCC scheme, Agricultural Debt Waiver and Debt Relief Scheme, and Interest Subvention schemes so that farmers can get low-interest loans and support the mechanisation in agriculture. Krishi Mela can be promoted where farmers can meet with other farmers, bank officials, extension agents, service agencies, and institutions to gather information about ZT. Moreover, in collaboration with private sectors and NGOs, central and state governments may initiate learning through capacity development approaches like demonstration plots, cross visits, study tours, and Farmers Field School to improve smallholders’ capacities to adopt ZT.
The research was conducted in a representative region of peninsular India, specifically Andhra Pradesh. The study could be replicated in other agro-climatic zones to assess the superiority of ZT over conventional tillage in Maize cultivation. The scope of this study is limited to the economic advantages associated with adopting ZT. Forthcoming research may centre on environmental consequences and soil properties with far-reaching societal advantages.
Data availability statement
The raw data supporting the conclusions of this article will be made available by the authors, without undue reservation.
Ethics statement
Ethical review and approval was not required for the study on human participants in accordance with the local legislation and institutional requirements. Written informed consent from the patients/participants or patients/participants legal guardian/next of kin was not required to participate in this study in accordance with the national legislation and the institutional requirements.
Author contributions
SD: Writing – review & editing, Writing – original draft, Visualization, Validation, Supervision, Software, Resources, Project administration, Methodology, Investigation, Formal Analysis, Data curation, Conceptualization. KA: Writing – review & editing, Writing – original draft, Visualization, Validation, Supervision, Software, Resources, Project administration, Methodology, Investigation, Formal Analysis, Data curation, Conceptualization. SS: Writing – review & editing, Supervision, Project administration, Funding acquisition, Conceptualization. PS: Writing – review & editing, Supervision, Resources, Formal Analysis, Data curation, Conceptualization. SK: Writing – review & editing. PB: Writing – review & editing, Supervision, Project administration. AR: Writing – review & editing, Supervision, Resources. HK: Writing – review & editing, Formal analysis, Data curation. AC: Writing – review & editing, Visualization, Supervision, Software. HR: Writing – review & editing, Validation, Supervision. AU: Writing – review & editing, Visualization, Project administration, Conceptualization.
Funding
The author(s) declare that no financial support was received for the research, authorship, and/or publication of this article.
Acknowledgments
We thank farmers from the villages of Srikakulam district for their willingness to participate in the data collection.
Conflict of interest
The authors declare that the research was conducted in the absence of any commercial or financial relationships that could be construed as a potential conflict of interest.
Publisher’s note
All claims expressed in this article are solely those of the authors and do not necessarily represent those of their affiliated organizations, or those of the publisher, the editors and the reviewers. Any product that may be evaluated in this article, or claim that may be made by its manufacturer, is not guaranteed or endorsed by the publisher.
Supplementary material
The Supplementary material for this article can be found online at: https://www.frontiersin.org/articles/10.3389/fsufs.2024.1362530/full#supplementary-material
Footnotes
1. ^Zero-till or no-till refers to a method of farming that involves minimizing soil disturbance as a substitute for conventional cultivation techniques like plowing or discing. These conventional methods include breaking up the soil and cultivating it to create a suitable seedbed. In the absence of conventional farming methods, seeds are planted in a narrow groove carved into the ground, resulting in minimal disruption to the soil. While no-till seeders are often utilized, there is a growing availability of small-scale no-till seeders that may be operated with either animal traction or small tractors. In Sub-Saharan Africa, no-till planting can be accomplished by creating a hole for each seed, such as maize, using a ‘dibble stick.’
References
Abay, K. A., Berhane, G., Taffesse, A. S., Abay, K., and Koru, B. (2018). Estimating input complementarities with unobserved heterogeneity: evidence from Ethiopia. J. Agric. Econ. 69, 495–517. doi: 10.1111/1477-9552.12244
Abdulai, A., Owusu, V. C., and Bakang, J. E. A. (2011). Adoption of safer irrigation technologies and cropping patterns: evidence from southern Ghana. Ecol. Econ. 70, 1415–1423. doi: 10.1016/j.ecolecon.2011.03.004
Adeoti, A. I. (2008). Factors influencing irrigation technology adoption and its impact on household poverty in Ghana. J. Agric. Rural. Dev. Trop. Subtrop. 109, 51–63
Ali, A., and Erenstein, O. (2013). Impact of zero tillage adoption on household welfare in Pakistan. J. Agric. Technol. 9, 1715–1729
Ali, A., Erenstein, O., and Rahut, D. B. (2014). Impact of direct rice-sowing technology on rice producers’ earnings: empirical evidence from Pakistan. Dev. Stud. Res. 1, 244–254. doi: 10.1080/21665095.2014.943777
Ali, E. B., Awuni, J. A., and Danso-Abbeam, G. (2018). Determinants of fertiliser adoption among smallholder cocoa farmers in the Western region of Ghana. Cogent Food Agric. 4:1538589. doi: 10.1080/23311932.2018.1538589
Ali, S., Tedone, L., Verdini, L., Cazzato, E., and De Mastro, G. (2019). Wheat response to no-tillage and nitrogen fertilization in a long-term Faba bean-based rotation. Agronomy 9:50. doi: 10.3390/agronomy9020050
Allagbe, M. C., and Biaou, G. (2013). Déterminants de l’adoption des variétés améliorées de riz Nerica dans les communes de Dassa-Zouméet de Glazoué au Bénin. Bull. Rech. Agron. Bénin 74, 48–59
Arvidsson, J., Etana, A., and Rydberg, T. (2014). Crop yield in Swedish experiments with shallow tillage and no-tillage 1983–2012. Eur. J. Agron. 52, 307–315. doi: 10.1016/j.eja.2013.08.002
Austin, P. C. (2011). An Introduction to Propensity Score Methods for Reducing the Effects of Confounding in Observational Studies. Multivariate Behavioral Research, 46, 399–424. doi: 10.1080/00273171.2011.568786
Barry, S. (2016). Déterminants socioéconomiques et institutionnels de l’adoption des variétés améliorées de maïs dansle Centre-Suddu Burkina Faso. Revue D’economie Théorique Et Appliquée 6, 221–238
Bartlett, J. E., Kotrlik, J. W., and Higgins, C. C. (2002). Organizational Research: Determining Appropriate Sample Size in Survey Research. Information Technology, Learning, and Performance Journal, 19, 43–50.
Becker, S. O., and Caliendo, M. (2007). Sensitivity analysis for average treatment effect. Stata J. 7, 71–83. doi: 10.1177/1536867X0700700104
Becker, S. O., and Ichino, A. (2002). Estimation of average treatment effects based on propensity scores. Stata J. 2, 358–377. doi: 10.1177/1536867X0200200403
Bernard, T., and Taffesse, A. S. (2012). Returns to scope? Smallholders’ commercialisation through multipurpose cooperatives in Ethiopia. J. Afr. Econ. 21, 440–464. doi: 10.1093/jae/ejs002
Bernstein, E. R., Posner, J. L., Stoltenberg, D. E., and Hedtcke, J. L. (2011). Organically managed no-tillage Rye–soybean systems: agronomic, economic, and environmental assessment. Agron. J. 103, 1169–1179. doi: 10.2134/agronj2010.0498
Beyaert, R. P., Schott, J. W., and White, P. H. (2002). Tillage effects on corn production in a coarse-textured soil in southern Ontario. Agron. J. 94, 767–774. doi: 10.2134/agronj2002.7670
Blanco-Canqui, H., and Wortmann, C. S. (2020). Does occasional tillage undo the ecosystem services gained with no-till? A review. Soil Tillage Res. 198:104534. doi: 10.1016/j.still.2019.104534
Bottinelli, N., Angers, D. A., Hallaire, V., Michot, D., Le Guillou, C., Cluzeau, D., et al. (2017). Tillage and fertilisation practices affect soil aggregate stability in a humic Cambisol of Northwest France. Soil Tillage Res. 170, 14–17. doi: 10.1016/j.still.2017.02.008
Brennan, J., Hackett, R., McCabe, T., Grant, J., Fortune, R. A., and Forristal, P. D. (2014). The effect of tillage system and residue management on grain yield and nitrogen use efficiency in winter wheat in a cool Atlantic climate. Eur. J. Agron. 54, 61–69. doi: 10.1016/j.eja.2013.11.009
Caliendo, M., and Kopeinig, S. (2008). Some practical guidance for the implementation of propensity score matching. J. Econ. Surv. 22, 31–72. doi: 10.1111/j.1467-6419.2007.00527.x
Calzarano, F., Stagnari, F., D’Egidio, S., Pagnani, G., Galieni, A., Di Marco, S., et al. (2018). Durum wheat quality, yield and sanitary status under conservation agriculture. Agriculture 8:140
Carter, D. W., and Milon, J. W. (2005). Price Knowledge in Household Demand for Utility Services. Land Economics, 81, 265–283. https://www.jstor.org/stable/4129668
Census. (2011). Available at: https://censusindia.gov.in/2011-Common/CensusData2011.html
Choudhary, M., Datta, A., Jat, H. S., Yadav, A. K., Gathala, M. K., Sapkota, T. B., et al. (2018). Changes in soil biology under conservation agriculture-based sustainable intensification of cereal systems in indo-Gangetic Plains. Geoderma 313, 193–204. doi: 10.1016/j.geoderma.2017.10.041
Conley, T. G., and Udry, C. R. (2001). Social learning through networks: the adoption of new agricultural technologies in Ghana. Am. J. Agric. Econ. 83, 668–673. doi: 10.1111/0002-9092.00188
Conley, T. G., and Udry, C. R. (2010). Learning about a new technology: pineapple in Ghana. Am. Econ. Rev. 100, 35–69. doi: 10.1257/aer.100.1.35
Dang, Y. P., Balzer, A., Crawford, M., Rincon-Florez, V., Liu, H., Melland, A. R., et al. (2018). Strategic tillage in conservation agricultural systems of north-eastern Australia: why, where, when and how? Environ. Sci. Pollut. Res. 25, 1000–1015. doi: 10.1007/s11356-017-8937-1
Das, T. K., Saharawat, Y. S., Bhattacharyya, R., Sudhishri, S., Bandyopadhyay, K. K., Sharma, A. R., et al. (2018). Conservation agriculture effects on crop and water productivity, profitability and soil organic carbon accumulation under a maize-wheat cropping system in the north-western indo-Gangetic Plains. Field Crop Res. 215, 222–231. doi: 10.1016/j.fcr.2017.10.021
Dey, S., and Singh, P. K. (2023). Role of market participation on smallholder vegetable farmers’ wellbeing: evidence from matching approach in eastern India. Agribusiness 39, 1217–1237. doi: 10.1002/agr.21813
DiPrete, T. A., and Gangl, M. (2004). Assessing bias in the estimation of causal effects: Rosenbaum bounds on matching estimators and instrumental variables estimation with imperfect instruments. Sociol. Methodol. 34, 271–310. doi: 10.1111/j.0081-1750.2004.00154.x
Duraisamy, P. (2002). Changes in returns to education in India, 1983–94: by gender, age-cohort and location. Econ. Educ. Rev. 21, 609–622. doi: 10.1016/S0272-7757(01)00047-4
FAO. (2017). Food and Agriculture Organization of the United Nations: Conservation Agriculture [online]. Available at: http://www.fao.org/ag/ca (Accessed August 28, 2017).
Feder, G., Just, R. E., and Zilberman, D. (1985). Adoption of agricultural innovations in developing countries: a survey. Econ. Dev. Cult. Chang. 33, 255–298. doi: 10.1086/451461
Fischer, E., and Qaim, M. (2014). Smallholder farmers and collective action: what determines the intensity of participation? J. Agric. Econ. 65, 683–702. doi: 10.1111/1477-9552.12060
Francesconi, G. N., and Heerink, N. (2011). Ethiopian agricultural cooperatives in an era of global commodity exchange: does organisational form matter? J. Afr. Econ. 20, 153–177. doi: 10.1093/jae/ejq036
GOI. (2015). Agriculture statistics at a glance 2015. Horticulture statistics division Department of Agriculture, Cooperation & Farmers Welfare Ministry of Agriculture & Farmers Welfare, Government of India.
GOI. (2021). Agricultural Statistics at a Glance, Government of India, Ministry of Agriculture and Farmers Welfare, Department of Agriculture, Cooperation & Farmers Welfare Directorate of Economics and Statistics.
Guo, L., Zhang, L., Liu, L., Sheng, F., Cao, C., and Li, C. (2021). Effects of long-term no tillage and straw return on greenhouse gas emissions and crop yields from a rice-wheat system in Central China. Agric. Ecosyst. Environ. 322:107650. doi: 10.1016/j.agee.2021.107650
Hausman, J. A. (1978). Specification tests in econometrics. Econometrica 46, 1251–1271. doi: 10.2307/1913827
Heckman, J. J., Ichimura, H., and Todd, P. E. (1997). Matching as an econometric evaluation estimator: evidence from evaluating a job training programme. Rev. Econ. Stud. 64, 605–654. doi: 10.2307/2971733
Hobbs, P. R., Sayre, K., and Gupta, R. (2008). The role of conservation agriculture in sustainable agriculture. Philos. Trans. R. Soc. B 363, 543–555. doi: 10.1098/rstb.2007.2169
Holland, J. M. (2004). The environmental consequences of adopting conservation tillage in Europe: reviewing the evidence. Agric. Ecosyst. Environ. 103, 1–25. doi: 10.1016/j.agee.2003.12.018
Houeninvo, G. H., Quenum, C. V. C., and Nonvide, G. M. A. (2020). Impact of improved maize variety adoption on smallholder farmers’ welfare in Benin. Econ. Innov. New Technol. 29, 831–846. doi: 10.1080/10438599.2019.1669331
Huffman, W. E. (2001). “Human capital: education and agriculture” In: G. L. Gardner and G. C. Rausser Eds., Handbook of agricultural economics. (North Holland Amsterdam: Elsevier) 334–381.
Idrisa, Y. L., Shehu, H., and Ngamdu, M. B. (2012). Effects of adoption of improved maize seed on household food security in Gwoza local government area of Borno state, Nigeria. Global J. Sci. Front. Res. 12, 7–12
Jat, M. L., Gathala, M. K., Saharawat, Y. S., Tetarwal, J. P., and Gupta, R. (2013). Double no-till and permanent raised beds in maize–wheat rotation of north-western indo-Gangetic plains of India: effects on crop yields, water productivity, profitability and soil physical properties. Field Crop Res. 149, 291–299. doi: 10.1016/j.fcr.2013.04.024
Jat, R. K., Sapkota, T. B., Singh, R. G., Jat, M. L., Kumar, M., and Gupta, R. K. (2014). Seven years of conservation agriculture in a rice–wheat rotation of eastern Gangetic Plains of South Asia: yield trends and economic profitability. Field Crop Res. 164, 199–210. doi: 10.1016/j.fcr.2014.04.015
Księżak, J., Bojarszczuk, J., and Staniak, M. (2018). Comparison of maize yield and soil chemical properties under maize (Zea mays L.) grown in monoculture and crop rotation. J. Elem. 23:531e543
Lal, R. (2019). Promoting “4 per thousand” and “adapting African agriculture” by south-south cooperation: conservation agriculture and sustainable intensification. Soil Tillage Res. 188, 27–34. doi: 10.1016/j.still.2017.12.015
Lampurlanés, J., Plaza-Bonilla, D., Álvaro-Fuentes, J., and Cantero-Martínez, C. (2016). Long-term analysis of soil water conservation and crop yield under different tillage systems in Mediterranean rainfed conditions. Field Crop Res. 189, 59–67. doi: 10.1016/j.fcr.2016.02.010
Läpple, D., and Rensburg, T.Van. (2011). Adoption of organic farming: are there differences between early and late adoption? Ecol. Econ., 70, 1406–1414, doi: 10.1016/j.ecolecon.2011.03.002
Lokshin, M., and Sajaia, Z. (2004). Maximum likelihood estimation of endogenous switching regression models. The Stata Journal, 3, 282–289.
Marenya, P. P., and Barrett, C. B. (2007). Household-level determinants of adoption of improved natural resources management practices among smallholder farmers in western Kenya. Food Policy 32, 515–536. doi: 10.1016/j.foodpol.2006.10.002
Mdemu, M. V., Mziray, N., Bjornlund, H., and Kashaigili, J. J. (2016). Barriers to and opportunities for improving productivity and profitability of the Kiwere and Magozi irrigation schemes in Tanzania. Int. J. Water Resour. Dev. 33, 725–739. doi: 10.1080/07900627.2016.1188267
Meinzen-Dick, R., DiGregorio, M., and McCarthy, N. (2004). Methods for studying collective action in rural development? Agric. Syst. 82, 197–214. doi: 10.1016/j.agsy.2004.07.006
Mitchell, J. P., Shrestha, A., Mathesius, K., Scow, K. M., Southard, R. J., Haney, R. L., et al. (2017). Cover cropping and no-tillage improve soil health in an arid irrigated cropping system in California’s San Joaquin Valley, USA. Soil Tillage Res. 165, 325–335. doi: 10.1016/j.still.2016.09.001
Mwangi, M., and Kariuki, S. (2015). Factors determining adoption of new agricultural technology by smallholder farmers in developing countries. J. Econ. Sustain. Dev. 6, 208–216
Mwombe, S. O. L., Mugivane, F. I., Adolwa, I. S., and Nderitu, J. H. (2014). Evaluation of information and communication technology utilization by small holder Banana farmers in Gatanga District, Kenya. J. Agric. Educ. Ext. 20, 247–261. doi: 10.1080/1389224X.2013.788454
Nkamleu, G. B., and Adesina, A. A. (2000). Determinants of chemical input use in peri urban lowland systems: bivariate probit analysis in Cameroon. Agric. Syst. 63, 111–121. doi: 10.1016/S0308-521X(99)00074-8
Nonvide, G. M. A. (2021). Adoption of agricultural technologies among rice farmers in Benin. Rev. Dev. Econ. 25, 2372–2390. doi: 10.1111/rode.12802
Nonvide, G. M. A., Sarpong, D. B., Kwadzo, T. M. G., Anim-Somuah, H., and Amoussouga Gero, F. (2018). Farmers’ perceptions of irrigation and constraints on rice production in Benin: a stakeholder-consultation approach. Int. J. Water Resour. Dev. 34, 1001–1021. doi: 10.1080/07900627.2017.1317631
Parihar, C. M., Jat, S. L., Singh, A. K., Kumar, B., Rathore, N. S., Jat, M. L., et al. (2018). Energy auditing of long-term conservation agriculture based irrigated intensive maize systems in semi-arid tropics of India. Energy 142, 289–302. doi: 10.1016/j.energy.2017.10.015
Parihar, C. M., Jat, S. L., Singh, A. K., Kumar, B., Yadvinder-Singh Pradhan, S., Pooniya, V., et al. (2016). Conservation agriculture in irrigated intensive maize-based systems of North-Western India: effects on crop yields, water productivity and economic profitability. Field Crop Res. 193, 104–116. doi: 10.1016/j.fcr.2016.03.013
Peixoto, D. S., Silva, L., Melo, L. B. B., Azevedo, R. P., Araujo, B. C. L., Carvalho, T. S., et al. (2020). Occasional tillage in no-tillage systems: a global meta-analysis. Sci. Total Environ. 745:140887. doi: 10.1016/j.scitotenv.2020.140887
Pittelkow, C. M., Liang, X., Linquist, B. A., van Groenigen, K. J., Lee, J., Lundy, M. E., et al. (2015). Productivity limits and potentials of the principles of conservation agriculture. Nature 517, 365–368. doi: 10.1038/nature13809
Rao, S. M. M. V., Lakshmana, K., and Roy, G. S. (2022). Zero tillage method of maize cultivation is a boon to the farmers in Vizianagaram District of north coastal zone of Andhra Pradesh. Prog. Res. Int. J. 11, 6504–6507
Rosenbaum, P. R., and Rubin, D. B. (1983). The central role of the propensity score in observational studies for causal effects. Biometrika 70, 41–55. doi: 10.1093/biomet/70.1.41
Ruzzante, S., and Bilton, A. (2021). Adoption of agricultural technology in the developing world: a meta-analysis of the empirical literature. World Dev. 146:105599. doi: 10.1016/j.worlddev.2021.105599
Sayed, A., Sarker, A., Kim, J.-E., Rahman, M., and Mahmud, G. A. (2020). Environmental sustainability and water productivity on conservation tillage of irrigated maize in red brown terrace soil of Bangladesh. J. Saudi Soc. Agric. Sci. 19, 276–284. doi: 10.1016/j.jssas.2019.03.002
Sefeedpari, P., Rafiee, S., Komleh, S. H. P., and Ghahderijani, M. (2012). A source-wise and operation-wise energy use analysis for corn silage production, a case study of Tehran province, Iran. Int. J. Sustain. Built Environ. 1, 158–166. doi: 10.1016/j.ijsbe.2013.03.001
Seye, B., Arouna, A., Sall, S. N., and Ndiaye, A. A. (2016). Déterminants de l’adoption des semences certifiées devariétés améliorées du riz au Bénin. J. Rech. Sci. Univ. Lomé 18, 23–33
Sharma, P., Abrol, V., and Sharma, R. K. (2011). Impact of tillage and mulch management on economics, energy requirement and crop performance in maize–wheat rotation in rainfed subhumid inceptisols, India. Eur. J. Agron. 34, 46–51. doi: 10.1016/j.eja.2010.10.003
Šíp, V., Vavera, R., Chrpová, J., Kusá, H., and Růžek, P. (2013). Winter wheat yield and quality related to tillage practice, input level and environmental conditions. Soil Tillage Res. 132, 77–85. doi: 10.1016/j.still.2013.05.002
Sodjinou, E., Glin, L. C., Nicolay, G., Tovignan, S., and Hinvi, J. (2015). Socio-economic determinants of organic cotton adoption in Benin, West Africa. Agric. Food Econ. 3, 1–22. doi: 10.1186/s40100-015-0030-9
Sultan, D., Tsunekawa, A., Haregeweyn, N., Adgo, E., Tsubo, M., Meshesha, D. T., et al. (2017). Analysing the runoff response to soil and water conservation measures in a tropical humid Ethiopian highland. Phys. Geogr. 38, 423–447. doi: 10.1080/02723646.2017.1302869
Sun, J., Wang, Z., Du, Y., Zhang, E., Gan, H., Sun, D., et al. (2022). Optimised tillage improves yield and energy efficiency while reducing carbon footprint in winter wheat-summer maize rotation systems. Sci. Total Environ. 820:153278. doi: 10.1016/j.scitotenv.2022.153278
Trebbin, A., and Hassler, M. (2012). Farmers’ producer companies in India: a new concept for collective action? Environ. Plann. 44, 411–427. doi: 10.1068/a44143
Tura, M., Aredo, D., Tsegaye, W., La Rovere, R., Girma, T., Mwangi, W., et al. (2010). Adoption and continued use of improved maize seeds: case study of Central Ethiopia. Afr. J. Agric. Res. 5, 2350–2358
Velandia, M., Rejesus, R. M., Knight, T. O., and Sherrick, B. J. (2009). Factors affecting farmers utilisation of agricultural risk management tools: the case of crop insurance, forward contracting, and spreading sales. J. Agric. Appl. Econ. 41, 107–123. doi: 10.1017/S1074070800002583
Wang, H., Guo, Q., Li, X., Li, X., Yu, Z., Li, X., et al. (2020). Effects of long-term no-tillage with different straw mulching frequencies on soil microbial community and the abundances of two soil-borne pathogens. Appl. Soil Ecol. 148:103488. doi: 10.1016/j.apsoil.2019.103488
Wang, J. B., Yan, C. R., Liu, E. K., Chen, B. Q., and Zhang, H. H. (2015). Effects of long-termno-tillage with straw mulch on photosynthetic characteristics of flag leauesand dry matter accumulation and translocation of winter wheat in dryland. J. Plant Nutr. Fertil. 21, 296–305
Wang, Q., Cao, X., Wang, C., Li, H., He, J., and Lu, C. (2021). Research progress of no/minimum tillage corn seeding technology and machine in northeast black soil regions of China. Trans. Chin. Soc. Agric. Mach. 52, 1–15
Yadav, G. S., Saha, P., Babu, S., Das, A., Layek, J., and Debnath, C. (2018). Effect of no-till and Raised-bed planting on soil moisture conservation and productivity of summer maize (Zea mays) in eastern Himalayas. Agric. Res. 7, 300–310. doi: 10.1007/s40003-018-0308-8
Yadav, O. P., Karjagi, C. G., Jat, S. L., and Dhillon, B. S. (2014). Overview of maize improvement in India. Indian Farming 64, 5–11
Keywords: conservation tillage, impact assessment, economic analysis, propensity score matching (PSM) approach, endogenous switch regression, peninsular India
Citation: Dey S, Abbhishek K, Saraswathibatla S, Singh PK, Kuntamalla S, Bommaraboyina PR, Raj A, Kaliki H, Choubey AK, Rongali HB and Upamaka A (2024) Impact of zero tillage maize production on yield, income, and resource utilization in peninsular India: an action-based quasi-experimental research. Front. Sustain. Food Syst. 8:1362530. doi: 10.3389/fsufs.2024.1362530
Edited by:
Wioletta Żukiewicz-Sobczak, Calisia University, PolandReviewed by:
Nester Mashingaidze, One Acre Fund, RwandaBiswajit Pramanick, Dr. Rajendra Prasad Central Agricultural University, India
Copyright © 2024 Dey, Abbhishek, Saraswathibatla, Singh, Kuntamalla, Bommaraboyina, Raj, Kaliki, Choubey, Rongali and Upamaka. This is an open-access article distributed under the terms of the Creative Commons Attribution License (CC BY). The use, distribution or reproduction in other forums is permitted, provided the original author(s) and the copyright owner(s) are credited and that the original publication in this journal is cited, in accordance with accepted academic practice. No use, distribution or reproduction is permitted which does not comply with these terms.
*Correspondence: Kumar Abbhishek, YWJiaGlzaGVrLmtAZHJyZWRkeXNmb3VuZGF0aW9uLm9yZw==; a3VtYXIuYWJoaTYwMkBnbWFpbC5jb20=