- 1LEAF Research Unit, Terra Associated Laboratory, Instituto Superior de Agronomia, Universidade de Lisboa, Lisbon, Portugal
- 2CIRAD, UMR ART-DEV/FOFIFA, Antananarivo, Madagascar and ART-DEV, Université de Montpellier, CIRAD, CNRS, Montpellier, France
- 3Sustainable Societies Unit, School of Economics, University of Cape Town, Cape Town, South Africa
- 4CIRAD, UMR SELMET, Dakar, Senegal and SELMET, Université de Montpellier, CIRAD, INRAE, Institut Agro SupAgro, Montpellier, France
- 5CIRAD, UMR SELMET, Antsirabe, Madagascar and SELMET, Université de Montpellier, CIRAD, INRAE, Institut Agro SupAgro, Montpellier, France
Promoting smallholders’ sustainable development in Africa means addressing agronomic and economic factors but also highly relevant social influences shaping farmers’ production and affecting household well-being. Holistic, integrated analyses can help to meet this need, informing more effective policies and interventions for smallholder farming systems. The authors apply a transdisciplinary, quantitative approach to analyzing social impacts in the smallholder context, using milk-producing crop-livestock family farms in central Madagascar as a test case. First, stochastic frontier analysis is leveraged to confirm education as a social indicator linked to production efficiency. Then, linear regression is used for exploratory modeling of children’s educational outcomes. Findings from the Malagasy case emphasize the influence of rural infrastructure, parental education, chronic poverty, family planning, and crop-livestock diversification on children’s educational outcomes among one region’s farming households. Taken together, results suggest that Madagascar’s policymakers should consider comprehensive territorial planning for simultaneously promoting agricultural development and human well-being. This study illustrates how a transdisciplinary approach to social impacts analysis can integrate agronomic, economic, and social dynamics and help anticipate potential outcomes in support of smallholders’ sustainable development.
1 Introduction
Sustainable development is a multidimensional construct integrating natural resource management, economic growth, and human well-being (Helne and Hirvilammi, 2015; Lior et al., 2018). Logically, promoting sustainable agri-food systems requires holistic attention to interdependent environmental, economic, and social dynamics (UNHLPE, 2016). This need for holism is critical when addressing smallholder agriculture in developing countries, where farmers face vulnerabilities and limitations that shape their decisions, impact their production outcomes, and affect their families’ well-being (Rapsomanikis, 2015; Gassner et al., 2019).
The smallholder context is characterized by unique and specific constraints. Chronic poverty, structural inequality, environmental risk, and agronomic diversity interact to delineate farmers’ food production and farming families’ daily lives and futures (Kamara et al., 2019). For most rural African smallholders1, there is no real separation between ‘family’ and ‘farm’ for two key reasons: family members are the primary source of farm labor, and farm-level decision-making is heavily influenced by family circumstances (FAO, 2018). Acknowledging this reality is essential to Africa’s successful agricultural development because environmental and financial considerations must be integrated with familial and social dynamics (Bosc et al., 2018). So, if agricultural production, economic growth, and household well-being are inextricably intertwined for African smallholders, then we are drawn into the web of social factors that influence their development (Archer et al., 2008; Bacon et al., 2012; Fan and Rue, 2020).
In recent decades, efforts toward Africa’s sustainable development have focused on smallholder farming with only modest successes (Wiggins, 2014; Myeki et al., 2022). Climate change continues to aggravate farmers’ vulnerability (Cohn et al., 2017; Pereira, 2017), while rates of poverty, food insecurity, poor health, and undereducation have stagnated among rural households (Beegle et al., 2016; FAO, 2020). Research and policy aimed at African smallholders have emphasized technical innovation, inputs-oriented intensification, and globalized value chains (Toenniessen et al., 2008; Wise, 2021; Bassett and Munro, 2022) but those ‘Green Revolution’ type, market-oriented interventions have often failed to achieve sustainable development outcomes (Reardon, 2015; Dawson et al., 2016; Clay and Zimmerer, 2020; Wise, 2020). Given the stagnant statistics mentioned above, those failures highlight the need for more comprehensive approaches addressing the interplay between farming households’ production and their persistent social realities (Ollenburger et al., 2019; Nyambo et al., 2022; Martiarena and Temudo, 2023).
Enhancing production, increasing incomes, and improving well-being demands research approaches that integrate environmental, agronomic, economic, and social factors (Macombe, 2016; Ngetich et al., 2022). Agricultural research for development (AR4D) engages actors from across sectors to generate new technologies, build capacity, and improve policies for developing countries (FARA, 2018; IFAD, 2024). AR4D connects agricultural research activities with rural development aims to help fight poverty and hunger, promote resilience, and create better livelihoods (EC DGR, 2007). While AR4D has given attention to socio-ecological systems’ embedded interactions (Douthwaite et al., 2017), social influences are still far more often identified than quantified (Dent et al., 1995). Admittedly, measuring and modeling social impacts are, without doubt, challenging for researchers (Clift, 2003; Janker et al., 2019; Janker and Mann, 2020). New transdisciplinary approaches that holistically combine concepts, methodologies, and data across disciplines are essential for exploring social impacts and anticipating social outcomes that contribute to – or inhibit – smallholders’ sustainable development (Nelson and Coe, 2014; Froebrich et al., 2020; Manjula and Rengalakshmi, 2021).
What’s missing from the AR4D toolbox are techniques that link agronomic and economic methods and evidence to quantitative analyses of smallholders’ social realities. Life Cycle Thinking (LCT) offers a strong conceptual link to sustainable development (Pombo et al., 2019) that can help AR4D to fill this gap. LCT applies a systems-based lens that encompasses all facets of a product’s life cycle from inputs to outputs and impacts, acknowledging that changes in one part of the system are likely to affect the other parts (UNEP, 2004; Sherli and Kani, 2018). LCT’s holistic framework mirrors that of sustainable development by addressing production’s environmental, economic, and social elements (Ren and Toniolo, 2020). LCT tools, known as Life Cycle Assessments (LCA), operationalize comprehensive analyses that can support interventions’ design and improve related policymaking (Pennington et al., 2007; Sonnemann et al., 2018; Sala, 2019; García-Herrero et al., 2022). Social Life Cycle Assessment (S-LCA) is one LCT tool that can help AR4D conduct social impacts analysis (SIA) in smallholder-focused sustainability research (Petti et al., 2018; Venkatesh, 2019; Huertas-Valdivia et al., 2020).
S-LCA leverages qualitative and quantitative methods from sociology, engineering, and economics (UNEP, 2020). It examines the three causal dimensions of social effects and impacts – behaviors, socio-economic dynamics, and capitals – in the production of goods or services (UNEP, 2009). Rooted in social ecology’s view of the inherent relationship between society and the environment (Catton and Dunlap, 1978), S-LCA evolved amidst debate among engineers and social scientists around theories of ecological modernization and sustainability (Jänicke, 1985; Mol and Spaargaren, 2000). Contemporary thinking acknowledges S-LCA as a socio-political intervention that seeks to provoke social change (Sakellariou, 2016; Hobson and Lynch, 2018). It directs attention to structural inequalities, power relations among market actors, and concrete socio-economic issues embedded in production systems (Macombe, 2016). This theoretical foundation makes S-LCA appropriate for the African smallholder context, so often characterized by poverty, inequality, and uneven development.
Agri-food systems’ social dimensions are frequently under-addressed, and agri-food applications of S-LCA remain limited (Tragnone et al., 2022; Arcese et al., 2023). More recent case studies tend to focus on qualitative social performance in agri-food systems (Chen and Holden, 2017; Muthu, 2018; Frank et al., 2020; Zira et al., 2020; Aranda et al., 2021; Brenes-Peralta et al., 2021), and rarely do they focus on smallholders (Marting Vidaurre et al., 2020; Mulyasari et al., 2023; Rahmah et al., 2023). In fact, the S-LCA guidelines only incorporated smallholders as vulnerable stakeholders in 2020 (UNEP, 2020; Arcese et al., 2023). There are few examples of consequential S-LCA in agri-food systems (Feschet et al., 2013; Iofrida et al., 2019; Wei et al., 2022), where social impact pathways are quantitatively explored using causal or correlational analysis (UNEP, 2020). Consequential LCA (2024) could support smallholders’ sustainable development because it models social impacts and outcomes, generating quantitative evidence to inform social policy decision-making (Head, 2010; Mayne et al., 2018; Munda et al., 2020).
S-LCA is increasingly used as the social component of sustainability assessments, alongside Environmental Life Cycle Assessment and Economic Life Cycle Costing (Fauzi et al., 2019). However, as the youngest framework under the LCT umbrella, S-LCA is still evolving as a methodology (Pollok et al., 2021), and this presents challenges to the tool’s more widespread use (Iofrida et al., 2018; Huarachi et al., 2020). Inadequate global and national datasets are an obstacle, and there is not yet consensus on impact categories or appropriate social indicators, nor a standardized approach to the impacts analysis stage (Huertas-Valdivia et al., 2020; Larsen et al., 2022). So, despite its potential, the methodology’s immaturity impedes its standalone use, particularly for African smallholder contexts where no case studies exist yet. However, transdisciplinary approaches to AR4D can borrow methodologically from S-LCA when designing comprehensive, context-adapted social impact analyses that support smallholders’ sustainable development (Andersson and Giller, 2019).
Focusing on central Madagascar, this study applies an integrated, two-phased approach to transdisciplinary SIA. It builds from existing agronomic evidence supporting smallholders’ diversified crop-livestock production, as well as from economic analyses demonstrating unmet demand for fresh milk. In the first phase of analysis, stochastic frontier analysis (SFA) with inefficiency effects (Battese and Coelli, 1992, 1995) is applied to validate a relevant social indicator related to milk production in the regional sample. Then in the second phase, linear regression is used for exploratory modeling of educational outcomes for children among the sample’s households. The study’s findings inform rural development policy and planning for the region.
2 Context: Madagascar
Madagascar’s small-scale family farms are at the center of this study. Located in the Indian Ocean along the southeastern coast of Africa, Madagascar is an island state and former French colony with an estimated population of 28 million in 2019 (UNDESA). More than 70% of Madagascar’s land mass is arable (IFAD, 2020) and 83% of all Malagasy households are agricultural (INSTAT, 2020). Small-scale family farming predominates throughout the country, with national statistics placing 70% of agricultural production on <1.5Ha (INSTAT, 2011). Mixed crop-livestock diversification is the production norm (INSTAT, 2020). Developmentally, Madagascar’s progress has been repeatedly derailed by multiple post-independence political and economic crises, public health emergencies, and severe natural disasters (The African Development Bank Group (AfDBG), 2017). More than 80% of Malagasy people live below the international poverty line (World Bank, 2022a) and over half live in extreme poverty (IMF, 2017). Food insecurity, communicable disease, and low educational attainment are persistent challenges to development. Madagascar is ranked third highest in the world for child undernourishment (von Grebmer et al., 2021), and fewer than one-sixth of Malagasy children complete secondary school (INSTAT, 2018). Concerningly, Madagascar was recently labeled as one of the most disaster-prone countries in the world (UNOCHA, 2022), underscoring its vulnerability.
This study focuses on the highlands region of Vakinankaratra, located in central Madagascar (see Figure 1). One of the country’s main agricultural zones, Vakinankaratra has a temperate subtropical climate favorable to fruit trees, market vegetables, and staple cereals. Mixed crop-livestock production on family farms is the widespread norm. Extensive livestock grazing is commonly practiced, and dairy production is both climatically and economically viable (Naudin et al., 2019; Sourisseau et al., 2019). In fact, Vakinankarata is part of Madagascar’s “dairy triangle” where regional milk production once boomed, reaching volumes of over 40 million liters per year from some 13,000 producers until political crises crashed the sector in 2009 (Penot et al., 2016). More recently, there has been renewed growth in the Malagasy dairy industry, reflected in its inclusion in Madagascar’s 2017 National Development Strategy and the 2018 launch of the Africa-Milk program (CIRAD, 2019).
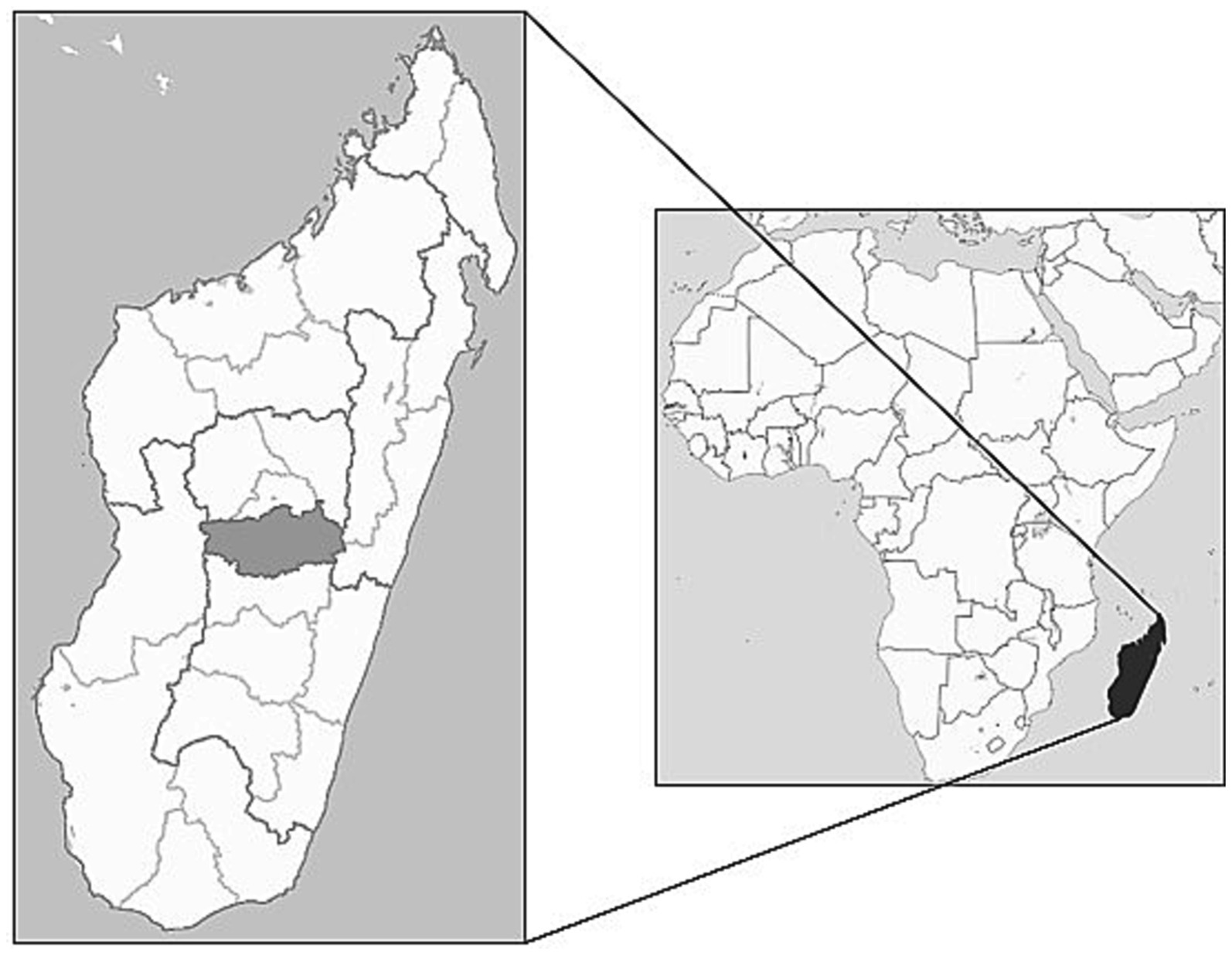
Figure 1. Map of Madagascar highlighting the central highlands region of Vakinankaratra (adapted from Sadalmelik (2008); CC A-SA 4.0).
For more than 30 years, an international AR4D consortium2 in Vakinankaratra has promoted and evidenced sustainable food system transformation for the region’s smallholder family farms. Agronomic research activities within the consortium support improved agroecological practices in Vakinankaratra’s ubiquitous crop-livestock farming systems (Andrianantoandro and Bélières, 2015; Côte et al., 2019), particularly around optimizing nutrient recycling via livestock manure management (Vall, 2020; Fanjaniaina et al., 2022). The consortium’s recent World Bank-funded value chains analyses also established domestic demand for fresh milk not yet met by farmers in the “dairy triangle,” while milk powder is being imported by Madagascar’s commercial dairies (Chatellier, 2019; Bélières and Lançon, 2020). There is a strategic developmental opportunity to support farmers’ intensified milk production in Vakinankaratra, leveraging their diversification to potentially increase incomes, improve household well-being, and augment manure resources for on-farm use (Sourisseau et al., 2019). However, family farms’ sustainable development is not assured by inputs and technical innovation alone. Without integrating SIA alongside the consortium’s agronomic and economic results, the social realities acting on farmers’ production and influencing their developmental outcomes could jeopardize the success of interventions.
From 2012 to 2022, the AR4D consortium engaged in participatory dialogues with a range of regional stakeholders, including: farmers, their spouses, and other adult members of farming households; actors from the public, private, and NGO sectors; national research institutions and international research partners. Those dialogues, which were captured by the consortiums’ various project reports, identified multiple areas of social concern3 relevant to farm-level production and household well-being. Under-education was highlighted in multiple stakeholder dialogues, which is no surprise since nearly two-thirds of Malagasy youth have not completed primary education and approximately one in five young people in Madagascar have no formal schooling at all. These figures skew even higher when poverty and rurality are taken into account (Education Policy and Data Center (EPDC), 2018; UNICEF, 2019a).
The authors reviewed the dataset and confirmed data availability for household members’ educational attainment (whereas there was no data available for many other social concerns mentioned in stakeholder dialogues) so this variable was considered for analysis. Education is widely acknowledged as a social contributor to farming households’ well-being and sustainable development (Schaafsma and Gross-Camp, 2021). It affects farmers’ decision-making and adoption of technical innovation (Fadeyi et al., 2022) and in Madagascar, research has linked higher educational outcomes with improved rural livelihoods and agricultural diversification (Neudert et al., 2015; UNICEF, 2019b). There are strong links between education and food security for rural populations (De Muro and Burchi, 2007), and education is also an evidenced indicator of intergenerational mobility4 and poverty alleviation in Africa’s developing countries (Alesina et al., 2021; Ouedraogo and Syrichas, 2021). Significant relationships have been established between African parents’ educational backgrounds and their children’s educational outcomes (Azomahou and Yitbarek, 2021; Razzu and Wambile, 2022), and research has also investigated the correlation between on-farm labor and children’s educational outcomes in African agriculture (Nkamelu and Kielland, 2006; Moyi, 2011; Asenso-Okyere et al., 2013). So, highlighted by regional stakeholders’ input and supported by the literature, education was deemed to be both relevant and context-appropriate for the study’s analyses.
3 Methods
3.1 Research design
As illustrated by Figure 2, the study’s research design mirrors the four steps of S-LCA: goal and scope definition, inventory, social impact assessment, and interpretation (UNEP, 2009, 2020). The study’s goal was to apply a context-adapted, transdisciplinary approach to SIA. Detailed, reliable technical and socio-economic data are rare in the region (indeed, in the entire country) so it was necessary to set the study’s scope considering analytical criteria that were relevant to the regional context and for which data were available. Given only farm- and household-level data availability, the study’s scope was set ‘from farm gate to farm gate’, focusing on farm-level inputs and outputs. Figure 3 illustrates how a typical crop-livestock family farm is embedded in the regional milk value chain. A range of inputs contribute to milk production and other farm outputs, although the data inventory revealed that some inputs depicted were not available for analysis (e.g., electricity, milk hygiene, land quality, volumes of purchased inputs, family health) while others were not correlated with outcome variables in this study’s analyses.
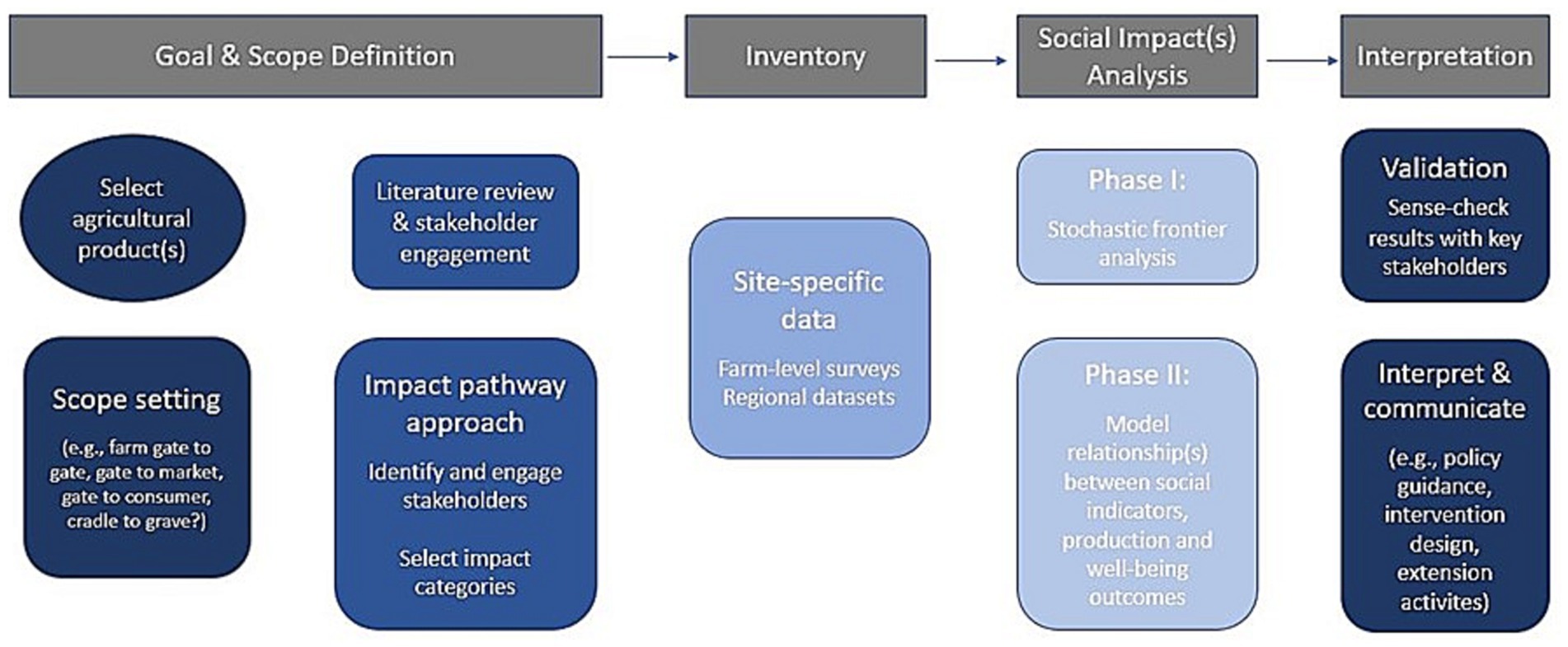
Figure 2. Diagram of the study’s context-adapted approach to social impacts analysis for smallholders’ sustainable development (adapted from UNEP, 2020).
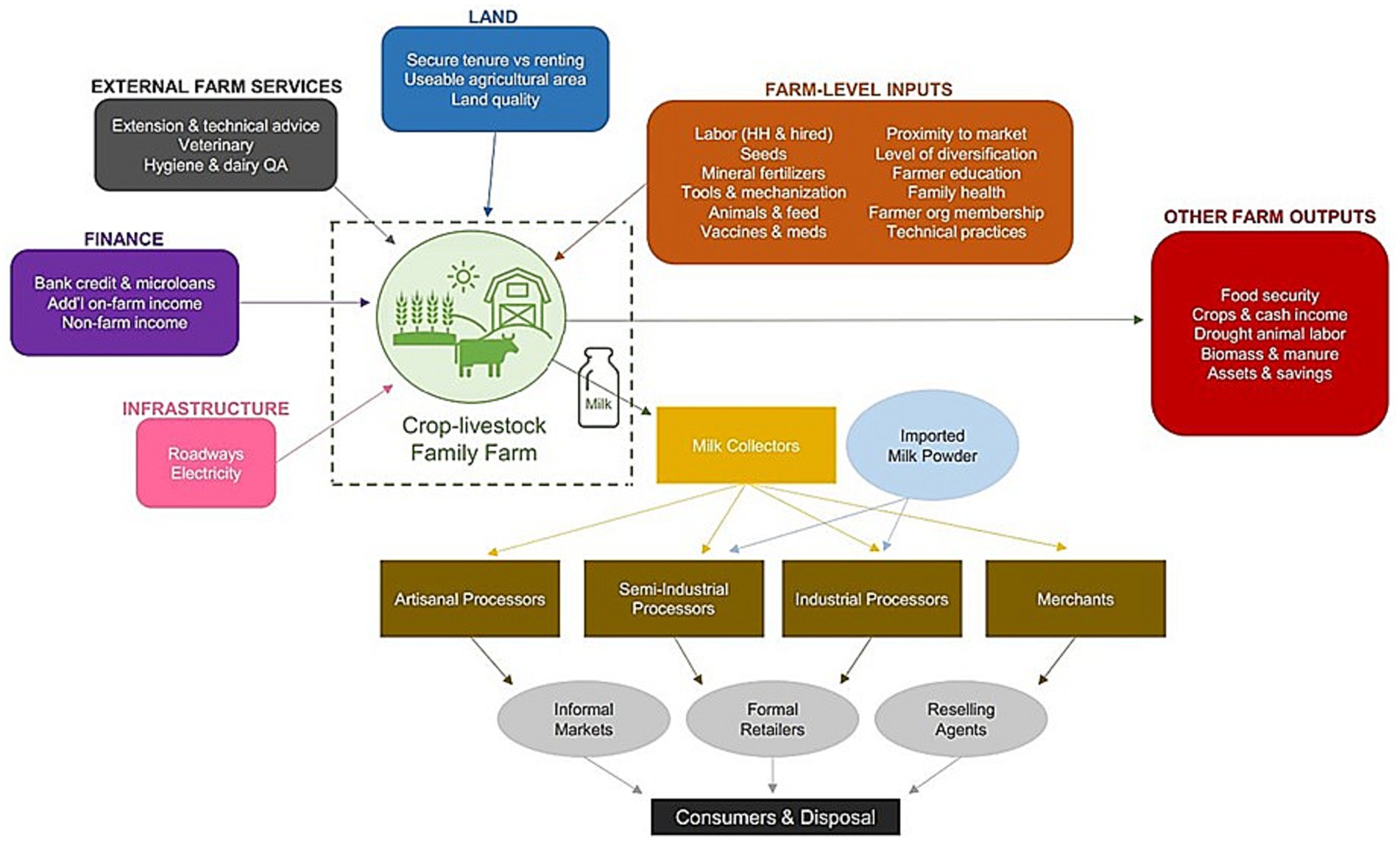
Figure 3. Diagram of the milk value chain in Vakinankaratra, Madagascar with this study’s ‘from farm gate to farm gate’ boundary delineated by the dashed line.
LCA tools typically define a functional unit of analysis in a given production system (Consequential LCA, 2024), so one liter of fresh milk was the agricultural product selected as the functional unit for Phase I’s SFA. It should be noted that SFA was used for two distinct reasons. First, the authors acknowledged the developmental opportunity identified by the AR4D consortium to intensify farmers’ milk production. There was a desire to produce evidence supporting that aim, and SFA is a commonly applied econometric technique for unpacking agricultural productivity (Hadley, 2006; Okoye et al., 2016; Conradie and Genis, 2020). SFA can help researchers and policymakers identify and quantify factors influencing farm-level efficiency. There are no existing productivity analyses of milk production in Madagascar, so it was a strategic choice to use SFA to augment the AR4D consortium’s evidence base.
Pertinent to this study, the second reason for using SFA in Phase I was simply to confirm whether education is related to milk production among the sample’s farming households. The authors had already justified the choice of education as a viable social impact variable based on stakeholders’ input and evidence in the literature. The SFA was leveraged to further validate that choice, highlighting that econometric techniques can provide additional justification for such choices when triangulated with participatory methods and existing evidence. Full results of the SFA (Thom and Conradie, 2024) are not presented in this manuscript because they focus on technical efficiency and are not integrated in Phase II’s SIA of children’s educational outcomes.
3.2 Data, sample selection, and variables for analysis
3.2.1 Data collection
The cross-sectional dataset originated from two comprehensive farm-level surveys conducted by the international AR4D consortium, which is anchored by Malagasy and French partner institutions. The first survey was conducted in late 2018 using a random sample of N = 405 representative farms from across Vakinankaratra. It aimed to typologize farms and improve interventions by analyzing the diversity of mixed crop-livestock farming systems, farmers’ management strategies, and the agroecological techniques in practice. In early 2019, the second survey was conducted and employed a randomly selected sample of N = 602 farms representing the same region. Intending to guide agricultural development policy and investment, the second survey sought to characterize milk producing farms in terms of socio-economic and technical production factors and activities. There was no duplication of individual farms in the two surveys.
Sampling for both surveys took place in two stages. The first stage was based on reasoned choice informed by a group of regional resource specialists and researchers who identified a relevant selection of districts5 and communes representative of Vakinankaratra’s agricultural diversity. Then, in two or three fokontany from each commune, farm households were drawn at random from electoral lists in the presence of administrative authorities. Questionnaires were designed with closed quantitative, qualitative, and open-ended questions and then pilot tested. Surveys were administered by trained interviewers in French and/or Malagasy (as appropriate). Data from both surveys were captured into customized Access databases, which were then audited and validated. Even though each survey had a different focus (i.e., agroecological practices in the first survey and milk production factors in the second), many of the same variables were captured since the instruments were designed by the same research team. Common variables from both surveys were identified and consolidated into a combined dataset (N = 699 farming households). All subsequent statistical analyses were conducted in Stata/SE version 16.1.
Finally, two post-analysis focus groups were conducted to solicit farmers’ own interpretations of the study’s quantitative results. Crop-livestock milk producers were invited from the Vakinankaratra region with the AR4D consortium’s assistance. Participating farmers were offered reimbursement for their transport, as well as lunch. A facilitation script was developed in English by the first author, then translated into French and Malagasy by a local researcher recommended by the AR4D consortium due to her deep familiarity with the regional production context and her fluency in English, French, and Malagasy languages. Focus groups were held on separate days, in two different villages. A total of 52 farmers (both male and female) attended. Focus groups were facilitated in Malagasy by the aforementioned local researcher, who provided real-time translation for the first author’s dialogue with the farmers throughout both interactions. Each focus group was also audio recorded for later reference. The local researcher drafted detailed synopses of the farmers’ qualitative responses to each query in the script, as well as summarized the topics and key points that emerged in dialogues. The authors analyzed the focus groups’ output using a straightforward constant comparison approach (Tumen Akyildiz and Ahmed, 2021) to connect the study’s quantitative results with farmers’ own experiential interpretations thereof.
3.2.2 Sample selection for statistical analyses
The overall sampling objective was to represent farming households by integrating the agricultural production unit and the family. The simple underlying logic is that 83% of all Malagasy households are agricultural households (INSTAT, 2020) so in Madagascar’s current developmental context, improving agricultural productivity effectively means improving living conditions and well-being for most households.
Sample selection for Phase I SFA used three selection criteria6: (1) cases that were randomly sampled, (2) mixed crop-livestock farming households in the Vakinankaratra region, and (3) farms with milking cows and/or selling milk. The resulting sub-sample of n = 147 farms includes two cases that do not own a cow but keep one in metayage, meaning they manage milk production (i.e., shelter, feed, water, and maintain animal health at their own expense) in exchange for the cow’s milk and sometimes its calves. Both cases were kept in the sub-sample because they represent a cultural production practice that is not uncommon in the region. The sub-sample also contains two outliers at the very high end of the regional milk production spectrum. Those cases were also retained because their production process does not differ in practice from the rest of the sample, and they represent a volume of production that was common in prior decades when a former President’s dairy in the region once thrived.
For the SIA in Phase II, the sub-sample above was then used to select all children in those households from ages 5–17 in alignment with the Malagasy educational system’s ages of attendance for primary to upper secondary school. The sub-sample was then further defined by the child’s relationship to the head of household, where cases were retained for that individual’s children, nieces or nephews, grandchildren, stepchildren, or entrusted children. These selection criteria produced a sub-sample of n = 248 children from 111 farming households in Vakinankaratra.
3.2.3 Dependent and predictor variables for Phase I
The outcome variable for Phase I’s SFA is liters of milk produced, captured in surveys as the total volume of milk produced on-farm in the prior year. Explanatory variables for analyzing dairy productivity with SFA typically include: farmer characteristics such as age, education, and years of experience; cow herd size and breed type; measures of labor; inputs such as feed and veterinary costs; climate data; water availability; access to markets, credit, and extension services; and household size (Masuku and Sihlongonyane, 2015; Masunda and Chiweshe, 2015; Nakanwagi and Hyuha, 2015; Hassan et al., 2018; Girma, 2019; Bahta et al., 2021). Unfortunately, the available dataset did not include climate data on temperature or rainfall, nor variables reflecting water availability or extension services, but other variables mentioned were available for testing in the frontier and the inefficiency model.
3.2.4 Dependent and predictor variables for Phase II
The first outcome variable ONPAR is a measure of children’s actual educational attainment in relation to their intended educational attainment at a given age, according to the Malagasy school system’s structure7. It was informed by the literature on ‘learning poverty’ in Africa, which describes how African children are falling far behind their international peers in terms of overall educational attainment and functional learning outcomes as they age (Agbor, 2012; Azevedo, 2020; World Bank, 2022b). Taking UNESCO’s approach to educational completion rates as a guide (UNESCO Institute of Statistics, 2024), the ONPAR index was coded as: 0 = no schooling, 1 = ≥3 years behind, 2 = on par with age or < 3 years behind. Categorization was initially validated by results of one-way analyses of variance (ANOVA) then refined through goodness-of-fit tests during modeling.
The second outcome variable EDUC_CLASS reflects children’s overall educational attainment categorized by level of schooling, where: 0 = no education, 1 = primary school, 2 = lower secondary school, 3 = upper secondary school.
The predictor variables used in the SIA were either continuous or categorical measures of: children’s age, gender, school status and on-farm agricultural activities; parents’ educational attainment; household size, distance to market and road access; farms’ total number of taxonomic animal and/or crop types produced; total value of household assets as proxy for household wealth/poverty.
Selection of predictor variables for each model started with those most highly correlated with the respective outcome variable. The Spearman’s rank correlation was chosen over Pearson where tests for normality indicated that continuous variables were normally distributed. Predictor variables for each model were selected one at a time, based on evaluation of model fit after each variable’s addition.
Descriptive statistics and definitions of predictor variables modeled with ONPAR and EDUC_CLASS can be seen in Tables 1, 2.

Table 1. Descriptive statistics and definitions of continuous predictor variables modeled with outcome variables ONPAR and EDUC_CLASS.
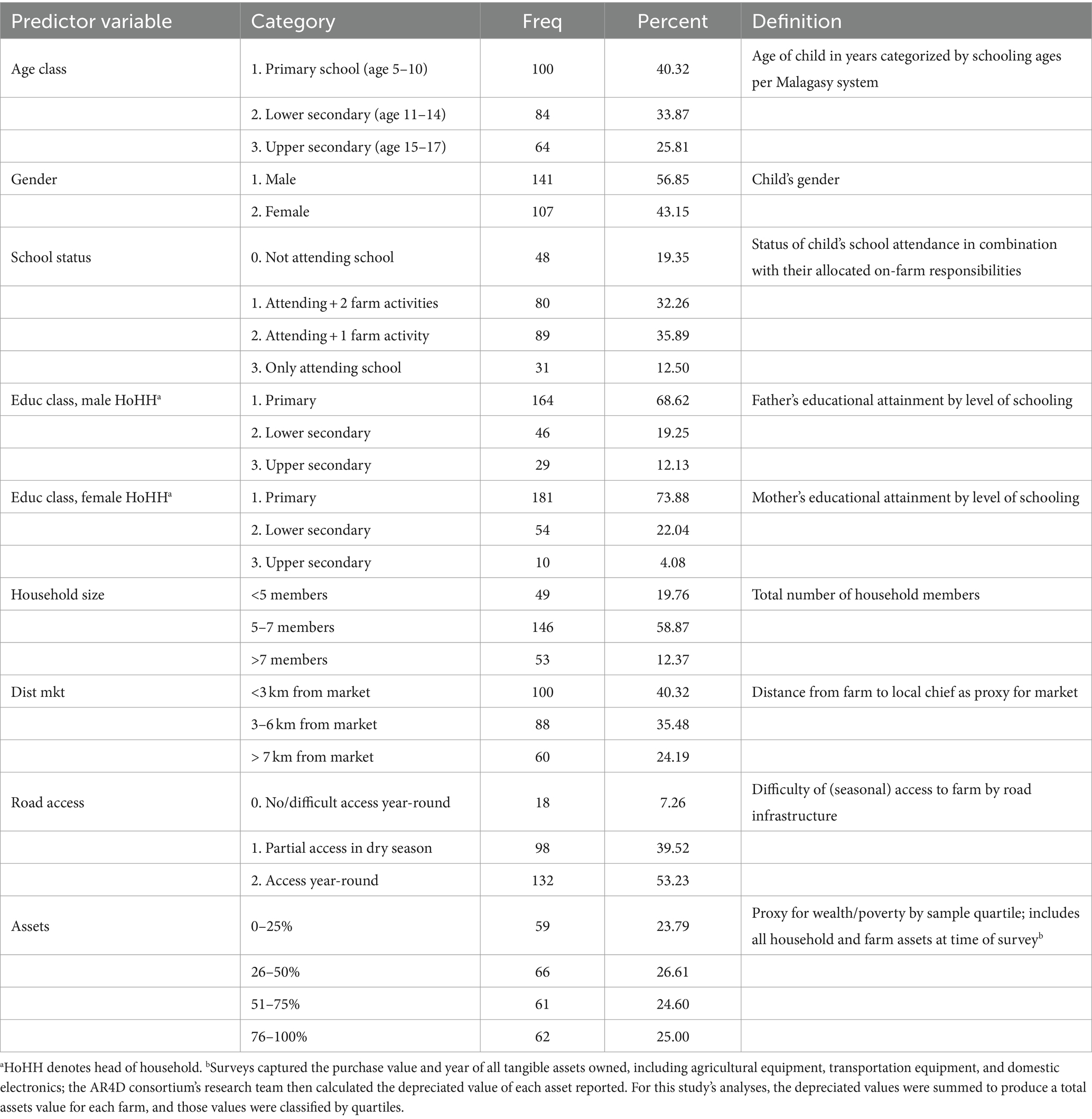
Table 2. Descriptive statistics and definitions of categorical predictor variables modeled with outcome variables ONPAR and EDUC_CLASS.
3.3 Statistical analysis
3.3.1 Phase I stochastic frontier approach
As discussed previously, SFA was used in Phase I to validate the choice of education as a variable relevant to production among the sample’s farming households. Stochastic frontier methods are commonly used econometric techniques for analyzing farm enterprise productivity (Hadley, 2006; Okoye et al., 2016; Conradie and Genis, 2020). They are particularly useful for agricultural contexts because they incorporate random errors to deal with the ‘noise’ of unpredictable externalities, like weather and economic shocks, and they can also cope with measurement errors (Coelli et al., 1998; Nguyen et al., 2021).
A stochastic production function with inefficiency effects first defines a ‘frontier’ of optimal production efficiency given a set of inputs (Aigner et al., 1977; Meeusen and van den Broeck, 1977; Battese and Coelli, 1992). It then identifies farm and farmer characteristics that explain inefficiency in terms of distance from the frontier (Battese and Coelli, 1995; Kumbhakar and Wang, 2010). A Cobb–Douglas production function and inefficiency model were specified in Stata/SE 16.1 using the frontier routine. As each variable was fitted, first for the production function and then for the inefficiency model, likelihood ratio (LR) tests were conducted to check goodness-of-fit using nested specifications. Lastly, predicted efficiency scores were generated for the sample using the inefficiency model. Statistics were considered significant at p ≤ 0.05.
3.3.2 Phase II regression modeling
In Phase II, regression modeling was used for exploratory SIA due to the cross-sectional nature of the dataset. Typically, measuring impacts necessitates multiple panels of data, and a second round of data collection was initially planned for the case study. However, the global pandemic impeded field travel, so analyses were limited to the single panel of data available. For this reason, impacts analyses presented in this paper are exploratory and anticipatory, rather than predictive.
A multinomial logistic regression (MLR) model was employed for statistical analyses of ONPAR using the mlogit command in Stata. MLR uses predictor variables in a regression equation to predict multiple levels of a dependent nominal variable with more than two outcome categories. While levels 1 and 2 of ONPAR have an ordinal character, MLR was chosen over an ordinal logistic regression (OLR) because in first-iteration analyses using an ordinal approach, the assumption of proportional odds was consistently violated. For MLR, the following additional assumptions must be met: independence of irrelevant alternatives; no influential outlying cases; independence of observations; no multicollinearity; and non-normally distributed variables. Multicollinearity was excluded by examining the variables’ VIF statistics, and dependence among some cases (due to the familial household nature of the dataset) was addressed using clustering in the model.
Nine observations were dropped in the MLR modeling due to missing data for fathers’ and/or mothers’ education. Outliers were explored post-estimation using Stata’s leastlikely command, which produces a list of cases with the lowest predicted probabilities of the outcome observed; five cases were considered outliers. The model was run without them to explore their potential influence, and doing so substantially improved model fit, increasing the log likelihood (LLH) by 10% and reducing Akaike’s information criteria (AIC) and Bayesian information criteria (BIC) by 8 and 6%, respectively. Therefore, the fitted MLR model excludes those cases.
Given that the outcome variable EDUC_CLASS is categorical and ranked, an OLR was utilized for its analyses using the ologit command in Stata. OLR uses predictor variables in a regression equation to predict multiple levels of a dependent ordered variable with three or more outcome levels. OLR presents similar assumptions to MLR but imposes the proportional odds assumption instead of the IIA assumption. As with the MLR analyses of ONPAR, multicollinearity was excluded using VIF statistics and independence was addressed through clustering. The gologit2 (Williams, 2006) command was employed in Stata to fit a partial proportional odds (PPO) model that met the proportional odds assumption.
Nine cases were dropped in OLR modeling due to missing data for some fathers’ education. In post-estimation, outliers were explored using the Pearson residuals predicted in binary logits using dummy outcome variables created for each level of EDUC_CLASS. Only two cases were considered as potentially influential outliers but removing them did not substantially change the model’s behavior or fit, so they were retained in the sample.
The process for fitting each model began with examination of the correlations between the respective outcome variable and the predictor variables. The Spearman’s rank correlation was chosen over Pearson because tests for normality indicated that none of the continuous variables were normally distributed. Each model was constructed one variable at a time, beginning with the most significantly correlated predictor variables and evaluating model fit after each variable’s addition through examination of:
• The LLH value and the Wald Chi-square statistic (Fox, 1997);
• McFadden’s r-squared value (McFadden, 1974); and
• AIC and BIC (Kuha, 2004).
For the MLR model, the Hausman test was applied to ensure the assumption of IIA was met (Hausman, 1978), and the Hosmer-Lemeshow test was also used for evaluating goodness-of-fit (Hosmer and Lemeshow, 1980). The OLR PPO model fit was examined using likelihood ratio tests (Peterson and Harrell 1990) comparing it with a more restrictive version of the OLR, as well as with the MLR version of the model. The Brant test was also applied to the OLR model to check that the proportional odds assumption was not violated (Brant, 1990).
Results from the MLR and OLR models are reported with a 95% confidence interval, and statistics were considered significant at p ≤ 0.10. The complexity of both models means that results can be challenging to interpret using coefficients and ratios alone, so marginal effects are presented to aid interpretation (Williams, 2012; Agresti and Tarantola, 2018).
4 Results
4.1 Validating education for social impact analysis
Results from the SFA (Thom and Conradie, 2024) confirmed that education is relevant to milk production among the sample’s crop-livestock farms. The sample was broken into terciles by the model’s predicted efficiency levels, and differences between the least and most efficient groups were explored. Farmer education differed significantly between those groups. The labor contribution of adolescents aged 12–14 also differed significantly between the least and most efficient farms, with the former using more than twice the adolescent labor as the latter. It is logical that adolescent children on the least efficient farms could experience poorer educational outcomes due to those families’ higher dependence on their labor. Both insights gleaned from the SFA confirmed that education is relevant to this production context.
4.2 Modeling educational outcomes on par with age
Interpreting MLR results requires examination of each outcome category’s predictors relative to the model’s base reference category, which is expected to change by its respective parameter estimates. The base reference category for the MLR results is ONPAR = 2, which reflects children who are educationally on par with their age or ≤ 2 years behind in schooling. Therefore, estimates and risk ratios for outcome categories 0 and 1 are interpreted relative to outcome category 2. It should be noted that the focus of interpretation here is on outcome ONPAR = 1, where children are more than 3 years behind in schooling. Results for ONPAR = 0, where children have no schooling, are not discussed.
The statistics and significant average marginal effects presented in Tables 3, 4 best illustrate the MLR model’s complex results. Girls are 10.0% less likely to be >3 years behind than boys and 13.9% more likely to be on par. Children’s increasing age makes it much more likely that they will fall behind (31.0% for adolescents and 45.8% for teenagers). Having fewer on-farm responsibilities in addition to school attendance means that those children are 10.5% more likely to be on par than their peers with additional on-farm tasks to perform. Raising more types of livestock means that children are 5.2% less likely to fall behind, while producing more crop types means they are 6.3% less likely to be on par. Having a more educated father makes a child 10.7% more likely to be educationally on par, while being part of a very large family living farthest from town makes a child 18.8 and 15.2%, respectively, more likely to fall behind. Finally, children from the poorest households are 21.7% less likely to achieve schooling on par with their age.

Table 3. Results of multinomial logistic regression (MLR) of outcome variable ONPAR (base outcome = 2).
4.3 Modeling overall educational attainment
When interpreting the results of OLR, the parameter estimates represent the change in the outcome category relative to the next level of the outcome variable. For this model, the base outcome EDUC_CLASS = 3 represents children who have attained (some or all) upper secondary school education. Estimates and odds ratios for outcome categories 0, 1 and 2 are interpreted relative to the subsequent outcome category. Just as in the MLR results presented above, it should be noted that the focus of interpretation here is on outcome categories EDUC_CLASS = 1 and = 2 and = 3, where children have obtained (some or all) primary, lower secondary or upper secondary school education. Results for EDUC_CLASS = 0, where children have no schooling, are not discussed.
The statistics and significant average marginal effects presented in Tables 5, 6 show that girls are 3.9% more likely than boys to attain lower secondary schooling. As children age, it is logical that they are more likely to attain schooling overall, but the AMEs reveal that this occurs at a decreasing rate: 4.7% for lower secondary but only 2.8% for upper secondary. The more educated a father, his child is 1.4% more likely to reach upper secondary school. The larger a child’s household size, the less likely it is that that child will advance in schooling (1.4% less likely for lower secondary and 0.9% less likely for upper secondary). Partial road access to the home makes children 2.4% more likely to reach upper secondary school, and a family farm tending more types of livestock makes children 10.0% less likely to attain primary school but 12.0% more likely to reach lower secondary. Those children in the poorest asset class are 7.5% less likely to attain lower secondary schooling and 4.1% less likely to reach upper secondary school.

Table 5. Results of ordered logistic regression (OLR) of outcome variable EDUC_CLASS (base outcome = 3).
5 Discussion
5.1 Children’s gender, parents’ education, and intergenerational mobility on family farms
Modeling revealed that girl children in the sample are more likely to be educationally on par and have better overall educational attainment, at least until lower secondary level. This suggests that perhaps boys bear the burden of a gender-based division of on-farm tasks that is more time- and labor-intensive and which keeps them out of school more often than their female peers, especially as they get older. This assumption was confirmed in post-analysis focus groups with farmers and aligns with research on agricultural child labor that confirms boys work harder than girls, in productivity terms, when performing on-farm tasks (Bandara et al., 2015; André et al., 2021). Among these farming households, boys suffer more of the educational consequences of farm labor than girls. However, the available data did not include a labor measure for girls’ domestic chores, which would surely compete with boys’ added workload and influence results if included in modeling. Farmers also commented in focus groups that boys are more likely to choose to stop schooling early to pursue wage employment, whereas girls will more often stay in school if they are allowed to do so. Those personal choice dynamics could also influence results if they could be rigorously measured for analysis.
Results also confirmed that, generally speaking, the more educated the parents, the better their children’s educational outcomes. In this sample, the more educated the father, the more likely it is that his child(ren) will continue to secondary school and stay educationally on par with their age. These results accord with the literature evidencing parental education’s positive influence on intergenerational mobility. They emphasize the importance of educating today’s children so the next generation – and perhaps their farms’ future production – can benefit from this influential relationship (Neudert et al., 2015).
5.2 Family size, poverty, and access to public services and infrastructure
The number of family members in a given household matters for children’s educational outcomes. Children from households with less than seven members are better positioned for on par educational attainment. In post-analysis focus groups, farmers expressed concerns about the difficulty of educating multiple children. Families with more children tend to remove the older ones from school so the younger ones can at least learn to read, write, and do basic math. Albeit practical in the face of limited resources, this strategy promotes a persistent cycle of undereducation to the detriment of intergenerational mobility. Farmers affirmed that family planning services offered in the Malagasy public health system could support having smaller families, but they voiced frustration that those services are too expensive. Despite farmers’ expressed willingness to use them, family planning services can only be accessed when there is ‘extra’ cash available. This is a clear constraint to moderating family size. The lack of affordable or no-cost family planning services in the Malagasy public health system therefore contributes to poor educational outcomes among children in the region’s farming households. This finding is supported by literature on social returns to family planning services in low-income countries (Joshi and Schultz, 2007; Haile and Haile, 2011; Canning and Schultz, 2012; Wouterse and Badiane, 2019).
Children who live fewer than seven kilometers from market/town, with at least partial road access during the dry season, go further in their schooling and are less likely to fall behind, which emphasizes the social relevance of good rural road infrastructure for reasons beyond industry and transport (Banjo et al., 2012; Hine et al., 2016). Severe poverty is a major problem for the bottom quartile of family farms, negatively impacting both agricultural production and children’s educational outcomes. This consistent finding reminds us that policies and interventions must continue to address chronic poverty among smallholders, considering this population’s needs differently from their better-off counterparts, if improved agricultural productivity and sustainable development outcomes like household well-being are to be achieved (Jayne et al., 2010).
5.3 Crop-livestock diversification and children’s education
Finally, the case study’s findings about the influence of multiple types of crop and livestock production are of particular interest. Analyses initially explored children’s educational outcomes in relation to volume of milk produced but found no significant relationship. However, analyses did reveal significant relationships between children’s education and the respective degrees of crop and livestock diversification on family farms. Producing more types of crops means that children are more likely to fall behind in school. This is likely due to the necessity of additional labor during multiple plantings and harvests throughout the year. Families must pull children out of school to help meet this need, which may be spread over days or weeks depending on the crop type(s), and lower-skill crop tasks are more often performed by younger children. Conversely, raising more types of livestock helps children stay on par with schooling and increases the likelihood that they will attain at least a lower secondary school level of education. Perhaps tending livestock is a task more often allocated to older household members and/or the volume of labor is more easily confined to pre- and post-school day timeframes. These assumptions were all validated by farmers in post-analysis workshops.
Findings suggest that children’s educational outcomes among Vakinankaratra’s family farms could benefit from a more limited amount of crop diversification but more intensified production of a wider range of livestock taxa. This is noteworthy because crop-livestock diversification is ubiquitous throughout the region and is actively promoted by AR4D to enhance resilience and buffer risk in smallholder farming systems. However, if a crop-heavy diversification mix on family farms contributes to undereducation among children, there may be ripple effects that harm intergenerational mobility and maintain the cycle of poverty. Further analyses to model different crop-livestock diversification mixes at farm-level could prove useful for guiding future AR4D and technical extension, particularly if the diversification variables were refined through evidence-based weighting.
The preceding discussion highlights how children’s educational outcomes are influenced by family farms’ resource limitations, as well as by how those limited resources are allocated. These findings underscore how, in a family farming context, the line between ‘family’ and ‘farm’ is often nonexistent. Decisions around household well-being and agricultural production are intimately interrelated and cannot be considered in isolation. So addressing the farm means addressing the family – and vice versa.
To support the duals aims of intensifying production while protecting children’s educational well-being among the region’s family farms, policy packages should take a holistic view of the production context. Given the study’s findings, Madagascar’s policymakers would do well to consider a territorial approach to family farms’ sustainable development in Vakinankaratra (Bélières et al., 2016). For example, simultaneous investment in rural infrastructusre, such as roadways and electricity, and public health services like family planning could be leveraged alongside existing agricultural interventions addressing access to markets and technical extension for the region’s smallholders. Such comprehensive policy packages are more likely to effectively promote sustainable development outcomes in family farming contexts.
5.4 Limitations
The study was limited by a lack of multiple survey panels for social impacts measurement, so modeling was exploratory rather than predictive. Future applications of this study’s approach would benefit from two or more panels of survey data for predictive impacts modeling. Additional data collection on processes and stakeholders external to the farm would also allow for the scope of the SIA to be expanded. The study’s scope was limited by data availability at farm level only, which meant that broadly analyzing social impacts across the entire regional milk production system was not possible. Relatedly, the choice of social impact indicator was limited to existing variables in the survey data. The authors’ acknowledge that education is not a novel social impact indicator, per se, but pursuing other social concerns flagged by stakeholders (e.g., food security, health outcomes, banditry) was inhibited by the lack of related variables in the dataset and the inability to undertake field travel for supplemental data collection. The dataset also lacked other variables that could have been relevant to this SIA of children’s education (e.g., detailed labor allocations across production activities, measures of domestic workload). Finally, the analysis did not encompass cultural considerations or power dynamics in household decision-making, which would have strengthened the findings.
6 Conclusion
Promoting sustainable development for African smallholders demands a holistic approach that addresses social factors influencing farmers’ production. Transdisciplinary methods can help AR4D fill this gap in the research. Integrated SIA facilitates exploration of the complex social dynamics relevant to family farms’ agricultural production. This, in turn, allows researchers and practitioners to (1) better comprehend farmers’ decision making, (2) envision potential social outcomes among farming households, and (3) generate quantitative evidence for guiding more effective sustainable development policies and interventions (Herrero et al., 2014; Ha et al., 2017; Nyambo et al., 2019).
This study’s integrated, transdisciplinary approach to SIA explored the links between children’s educational outcomes and various factors relevant to farm-level production. Findings illustrate how an SIA that builds from agronomic and economic evidence can examine such relationships and help to anticipate potential social outcomes linked to farming households’ well-being and sustainable development. This study’s approach could be applied to other smallholder production contexts, provided that similarly comprehensive survey datasets are collected and social impact(s) categories’ materiality is established through stakeholders’ substantial participation.
Data availability statement
The data analyzed in this study are subject to the following licenses/restrictions: the data analyzed in this study are available from the corresponding author, upon reasonable request and with the authors’ mutual agreement. Requests to access these datasets should be directed to AET amyethom@gmail.com.
Author contributions
AET: Conceptualization, Data curation, Formal analysis, Funding acquisition, Methodology, Validation, Visualization, Writing – original draft, Writing – review & editing. J-FB: Data curation, Investigation, Project administration, Resources, Validation, Writing – review & editing. BC: Formal analysis, Supervision, Writing – review & editing. PS: Funding acquisition, Investigation, Project administration, Resources, Writing – review & editing. MV: Funding acquisition, Resources, Writing – review & editing. DF: Funding acquisition, Project administration, Resources, Supervision, Writing – review & editing.
Funding
The author(s) declare financial support was received for the research, authorship, and/or publication of this article. This work was partially financed by national funds from the FCT – Fundação para a Ciência e a Tecnologia, I.P. (Portugal) within the scope of the following: UIDB/04129/2020 LEAF – Linking Landscape, Environment, Agriculture and Food Research Center; LA/P/0092/2020 of Associate Laboratory TERRA; doctoral research fellowship SFRH/BD/147494/2019 held by AET.
Acknowledgments
The authors would like to acknowledge the EcoAfrica and CASEF research teams in Madagascar for their generous access to project literature, survey data, and technical and logistical support throughout this study’s analyses and field missions.
Conflict of interest
The authors declare that the research was conducted in the absence of any commercial or financial relationships that could be construed as a potential conflict of interest.
Publisher’s note
All claims expressed in this article are solely those of the authors and do not necessarily represent those of their affiliated organizations, or those of the publisher, the editors and the reviewers. Any product that may be evaluated in this article, or claim that may be made by its manufacturer, is not guaranteed or endorsed by the publisher.
Footnotes
1. ^UNCTAD (2015) defines smallholders as “those who farm areas of about 2 hectares of landholding or less,” and the FAO (2018) defines a family farm as “an agricultural holding which is managed and operated by a household and where farm labor is largely supplied by that household.” Both definitions characterize African small-scale farming in general (Gollin, 2014), and they are also applicable to the farming households analyzed in this study. Therefore, these terms are used interchangeably throughout this paper.
2. ^Madagascar’s National Center for Applied Research in Rural Development (FOFIFA) and Center for Research and Rural Development in Agriculture and Livestock (FIFAMANOR) have been collaborating with the French Center for International Cooperation in Agronomic Research for Development (CIRAD) in central Madagascar for nearly 30 years, frequently accompanied by other project-specific research partners from Africa, Europe and elsewhere.
3. ^Social concerns articulated by stakeholders were: low levels of education; health; food security; family planning/size; security of livestock and other assets; rurality and access to services/infrastructure; vulnerability to economic crises (note: information sourced from unpublished project reports provided by the AR4D consortium).
4. ^According to Azomahou and Yitbarek (2021), intergenerational mobility describes the process in which socioeconomic outcomes are passed from one generation to the next. Education can be an effective indicator of socioeconomic outcomes due to its links to labor market participation, incomes, and overall economic growth. In Africa’s developmental context, intergenerational mobility through education is particularly relevant due to the persistent inequality of opportunity for children from poor and undereducated households, who are less able to move beyond their parents’ socioeconomic status (Alesina et al., 2021; Razzu and Wambile, 2022).
5. ^In Madagascar, administrative segmentation begins with provinces that are divided into regional districts, then into communes, and finally into local fokontany.
6. ^These selection criteria are justified because, at the level of Malagasy farm households, there are very diversified agricultural and non-agricultural activities, and this diversification ensures their resilience. Moreover, the simultaneous improvement of productivity and natural resource management – especially in situations where resources are limited – requires agroecological intensification. This in turn requires the strategic combination of activities, in particular integration of agriculture and livestock. Thus, there is no specialization of production at the level of farming households in Madagascar. As long as this high-risk production environment exists (e.g., climatic, diseases, pests, economic, social, and/pr political risks), then agroecological intensification via combining complementary activities seems to be the best option, at least for small and medium-sized farms. Given this scenario, milk production is a form of agroecological intensification that combines livestock breeding, cultivation of fodder, and most importantly, the production of manure, which is essential for fertilization and improving the outputs of food crops production.
7. ^In Madagascar’s schooling system, primary school should be attended between the ages of 6 to 10, lower secondary school between the ages of 11 to 14, and upper secondary school from 15 to 17 years of age (Education Policy and Data Center (EPDC), 2018).
References
Agbor, J (2012). Poverty, Inequality and Africa’s Education Crisis. Op-Ed Commentary. The Brookings Institution. Available at https://www.brookings.edu/articles/poverty-inequality-and-africas-education-crisis/. (Accessed March 2, 2024).
Agresti, A., and Tarantola, C. (2018). Simple ways to interpret effects in modeling ordinal categorical data. Statistica Neerlandica 72, 210–223. doi: 10.1111/stan.12130
Aigner, D., Lovell, C. A. K., and Schmidt, P. (1977). Formulation and estimation of stochastic frontier production function models. J. Econ. 6, 21–37. doi: 10.1016/0304-4076(77)90052-5
Alesina, A., Hohmann, S., Michalopoulos, S., and Papaioannou, E. (2021). Intergenerational mobility in Africa. Econometrica 89, 1–35. doi: 10.3982/ECTA17018
Andersson, J., and Giller, K. (2019). Doing development-oriented agronomy: rethinking methods, concepts and direction. Exp. Agric. 55, 157–162. doi: 10.1017/S0014479719000024
André, P., Delesalle, E., and Dumas, C. (2021). Returns to farm child labor in Tanzania. World Dev. 138:105181. doi: 10.1016/j.worlddev.2020.105181
Andrianantoandro, V. T., and Bélières, J.-F. (2015). L’agriculture familiale malgache entre survie et développement: Organisation des activités, diversification et différenciation des ménages agricoles de la région des Hautes Terres. Revue Tiers Monde, 221, 69–88. doi: 10.3917/rtm.221.0069
Aranda, J., Zambrana-Vásquez, D., Del-Busto, F., and Círez, F. (2021). Social impact analysis of products under a holistic approach: a case study in the meat product supply chain. Sustain. For. 13:12163. doi: 10.3390/su132112163
Arcese, G., Fortuna, F., and Pasca, M. G. (2023). The sustainability assessments of the supply chain of agri-food products: the integration of socio-economic metrics. Curr. Opin. Green Sustain. Chem. 40:100782. doi: 10.1016/j.cogsc.2023.100782
Archer, D. W., Dawson, J., Kreuter, U. P., Hendrickson, M., and Halloran, J. M. (2008). Social and political influences on agricultural systems. Renew. Agric. Food Syst. 23, 272–284. doi: 10.1017/S174217050700169X
Asenso-Okyere, K., Sarpong, D. B., Okyere, C. Y., Mensah-Bonsu, A., and Asuming-Brempong, S. (2013). Modeling the determinants of farmers’ decision on exclusive schooling and child labor in the cocoa sector of Ghana. Glob. J. Hum. Soc. Sci. Econ. 13. Available at: http://socialscienceresearch.org/index.php/GJHSS/article/viewFile/585/532
Azevedo, JP (2020) Learning Poverty: Measures and Simulations. Policy Research Working Paper No. WPS 9446. Washington, DC: World Bank Group.
Azomahou, T. T., and Yitbarek, E. (2021). Intergenerational mobility in education: is Africa different? Contemp. Econ. Policy 39, 503–523. doi: 10.1111/coep.12495
Bacon, C. M., Getz, C., Kraus, S., Montenegro, M., and Holland, K. (2012). The social dimensions of sustainability and change in diversified farming systems. Ecol. Soc. 17. doi: 10.5751/ES-05226-170441
Bahta, S., Omore, A., Baker, D., Okike, I., Gebremedhin, B., and Wanyoike, F. (2021). An analysis of technical efficiency in the presence of developments toward commercialization: evidence from Tanzania’s Milk producers. Eur. J. Dev. Res. 33, 502–525. doi: 10.1057/s41287-020-00279-8
Bandara, A., Dehejia, R., and Lavie-Rouse, S. (2015). The impact of income and non-income shocks on child labor: evidence from a panel survey of Tanzania. World Dev. 67, 218–237. doi: 10.1016/j.worlddev.2014.10.019
Banjo, G, Gordon, H, and Riverson, J (2012) Rural Transport: Improving Its Contribution to Growth and Poverty Reduction in Sub-Saharan Africa. Sub-Saharan Africa Transport Policy Program Working Paper No. 93. Washington, DC: World Bank.
Bassett, T. J., and Munro, W. (2022). Lost in translation: pro-poor development in the Green revolution for Africa. Afr. Stud. Rev. 65, 8–15. doi: 10.1017/asr.2021.99
Battese, G. E., and Coelli, T. J. (1992). Frontier production functions, technical efficiency and panel data: with application to paddy farmers in India. J. Prod. Anal. 3, 153–169. doi: 10.1007/BF00158774
Battese, G. E., and Coelli, T. J. (1995). A model for technical inefficiency effects in a stochastic frontier production function for panel data. Empir. Econ. 20, 325–332. doi: 10.1007/BF01205442
Beegle, K, Christiaensen, L, Dabalen, A, and Gaddis, I (2016) Poverty in a Rising Africa, Africa Poverty Report Overview. Washington, DC, World Bank.
Bélières, J.-F., Burnod, P., Rasolofo, P., and Sourisseau, J.-M. (2016). ““Illusion de” abondance: enjeux fonciers agricoles dans le Vakinankaratra à Madagascar” in Une Nouvelle Ruralité Émergente: Regards Croisés sur les Transformations Rurales Africaines. Eds. D. Pesche, B. Losch, and J. Imbernon (Montpellier: CIRAD-NEPAD).
Bélières, J.-F., and Lançon, F. (2020). Étude Diagnostic Relative au Potentiel de Croissance de la Chaine de Valeur Lait et Produits Dérivés (Hautes Terres–Madagascar) Antananarivo: CIRAD-CASEF.
Bosc, P. M., Sourisseau, J.-M., Bonnal, V. P., Gasselin, P., Valette, E., and Bélières, J.-F. (Eds.) (2018). Diversity of Family Farming Around the World: Existence, Transformations and Possible Futures of Family Farms. Dordrecht: Springer.
Brant, R. (1990). Assessing proportionality in the proportional odds model for ordinal logistic regression. Biometrics 46, 1171–1178. doi: 10.2307/2532457
Brenes-Peralta, L., Jiménez-Morales, M. F., Campos-Rodríguez, R., and Vittuari, M. (2021). Unveiling the social performance of selected Agri-food chains in Costa Rica: the case of green coffee, raw milk and leafy vegetables. Int. J. Life Cycle Assess. 26, 2056–2071. doi: 10.1007/s11367-021-01964-4
Canning, D., and Schultz, T. P. (2012). The economic consequences of reproductive health and family planning. Lancet 380, 165–171. doi: 10.1016/S0140-6736(12)60827-7
Catton, W. R., and Dunlap, R. E. (1978). Environmental sociology: a new paradigm. Am. Sociol. 13, 41–49.
Chatellier, V (2019) La Planète Laitière et la Place de l’Afrique de l’Ouest dans la Consommation, la Production et les Échanges de Produits Laitiers. 3 Èmes Recontres Internationales “Le LAIT, Vecteur de Développement”. Dakar: 12–13 Juin 2019.
Chen, W., and Holden, N. M. (2017). Social life cycle assessment of average Irish dairy farm. Int. J. Life Cycle Assess. 22, 1459–1472. doi: 10.1007/s11367-016-1250-2
CIRAD (2019). Africa-Milk: About the Project. Available at: https://www.africa-milk.org/ (February 14, 2024).
Clay, N., and Zimmerer, K. S. (2020). Who is resilient in Africa’s Green Revolution? Sustainable intensification and Climate Smart Agriculture in Rwanda. Land Use Policy. doi: 10.1016/j.landusepol.2020.104558
Clift, R. (2003). Metrics for supply chain sustainability. Clean Techn. Environ. Policy 5, 240–247. doi: 10.1007/s10098-003-0220-0
Coelli, TJ, Rao, DSP, and Battese, GE (1998). An Introduction to Efficiency and Productivity Analysis. Boston: Kluwer Academic Publishers.
Cohn, A. S., Newton, P., Gil, J. D. B., Kuhl, L., Samberg, L., Ricciardi, V., et al. (2017). Smallholder agriculture and climate change. Annu. Rev. Environ. Resour. 42, 347–375. doi: 10.1146/annurev-environ-102016-060946
Conradie, B., and Genis, A. (2020). Efficiency of a mixed farming system in a marginal winter rainfall area of the Overberg, South Africa, with implications for thinking about sustainability. Agrekon 59, 387–400. doi: 10.1080/03031853.2020.1828116
Consequential LCA (2024). Available at: https://consequential-lca.org/. (Accessed February 12, 2024).
Côte, F.-X., Poirier-Magona, E., Perret, S., Rapidel, B., Roudier, P., and Thirion, M.-C. (Eds.) (2019). The Agroecological Transition of Agricultural Systems in the Global South. Versailles: CIRAD.
Dawson, N., Martin, A., and Sikor, T. (2016). Green Revolution in Sub-Saharan Africa: Implications of Imposed Innovation for the Wellbeing of Rural Smallholders. World Dev. doi: 10.1016/j.worlddev.2015.10.008
De Muro, P, and Burchi, F (2007) Education for Rural People and Food Security: A Cross Country Analysis. Rome: FAO.
Dent, J. B., Edwards-Jones, G., and McGregor, M. J. (1995). Simulation of ecological, social and economic factors in agricultural systems. Agric. Syst. doi: 10.1016/0308-521X(95)00029-5
Douthwaite, B., Mur, R., Audouin, S., Wopereis, M., Hellin, J., Moussa, A. S., et al. (2017). Agricultural research for development to intervene effectively in complex systems and the implications for research organizations. KIT Working Paper 2017:12. Available at: https://agritrop.cirad.fr/584543/1/Douthwaite_al_AR4D_Research_organizations-2017_v6.pdf.
EC DGR (2007). Project Sheet ERA-ARD the Agricultural Research for Development (ARD) Dimension of the European Research Area (ERA). ERA-NET 2006: Series V. Luxembourg: European Commission, Directorate-General for Research. Publications Office of the EU.
Education Policy and Data Center (EPDC) (2018) Madagascar National Education Profile 2018 Update. Available at: https://www.epdc.org/sites/default/files/documents/EPDC_NEP_2018_Madagascar.pdf. (Accessed November 02, 2023).
Fadeyi, O. A., Ariyawardana, A., and Aziz, A. A. (2022). Factors influencing technology adoption among smallholder farmers: a systematic review in Africa. J. Agric. Rural. Dev. Trop. Subtrop. 123. doi: 10.17170/kobra-202201195569
Fan, S., and Rue, C. (2020). “The role of smallholder farms in a changing world” in The Role of Smallholder Farms in Food and Nutrition Security. eds. Y. Gomez, S. Paloma, L. Riesgo, and K. Louhichi (Cham: Springer).
Fanjaniaina, M. L., Stark, F., Ramarovahoaka, N. P., Rakotoharinaivo, J. F., Rafolisy, T., Salgado, P., et al. (2022). Nutrient flows and balances in mixed farming Systems in Madagascar. Sustain. For. 14. doi: 10.3390/su14020984
FAO (2018). FAO’s Work on Family Farming: Preparing for the Decade of Family Farming (2019–2028) to Achieve the SDGs. Available at: https://www.fao.org/3/ca1465en/CA1465EN.pdf. (Accessed March 17, 2023)
FAO (2020). Key Messages: Africa Regional Overview of Food Security and Nutrition 2019. FAO Publication No. CA7704EN/1/03.20. License: CC BY-NC-SA 3.0 IGO. Available at: http://www.fao.org/3/ca7704en/CA7704EN.pdf
FARA (2018). IAR4D. Forum for Agricultural Research in Africa. Available at: https://faraafrica.org/iar4d/ (Accessed February 10, 2024)
Fauzi, R. T., Lavoie, P., Sorelli, L., Heidari, M. D., and Amor, B. (2019). Exploring the current challenges and opportunities of life cycle sustainability assessment. Sustain. For. 11. doi: 10.3390/su11030636
Felix, K. N., Franklin, S. M., Collins, M. M., Milka, N. K., and Chris, A. S. Smallholders\u0027 coping strategies in response to climate variability in semi-arid agro-ecozones of Upper Eastern Kenya. Social Sciences \u0026amp; Humanities Open.
Feschet, P., Macombe, C., Garrabé, M., Loeillet, D., Saez, A. R., and Benhmad, F. (2013). Social impact assessment in LCA using the Preston pathway. Int. J. Life Cycle Assess. 18, 490–503. doi: 10.1007/s11367-012-0490-z
Fox, J. (1997). Applied Regression Analysis, Linear Models, and Related Methods. Thousand Oaks: Sage Publications Inc.
Frank, M., Laginess, T., and Schöneboom, J. (2020). “Social life cycle assessment in agricultural systems – U.S. corn production as a case study” in Perspectives on Social LCA. Springer Briefs in Environmental Science. eds. M. Traverso, L. Petti, and A. Zamagni (Switzerland: Springer)
Froebrich, J., Ludi, E., Bouarfa, S., Rollin, D., Jovanovic, N., Roble, M., et al. (2020). Transdisciplinary innovation in irrigated smallholder agriculture in Africa. Irrig. Drain. 69, 6–22. doi: 10.1002/ird.2400
García-Herrero, L., Brenes-Peralta, L., Leschi, F., and Vittuari, M. (2022). Integrating life cycle thinking in a policy decision tool: its application in the pineapple production in Dominican Republic. J. Clean. Prod. 360:132094. doi: 10.1016/j.jclepro.2022.132094
Gassner, A., Harris, D., Mausch, K., Terheggen, A., Lopes, C., Finlayson, R. F., et al. (2019). Poverty eradication and food security through agriculture in Africa: rethinking objectives and entry points. Outlook Agric. 48, 309–315. doi: 10.1177/0030727019888513
Girma, H. (2019). Estimation of technical efficiency of dairy farms in central zone of Tigray National Regional State. Heliyon 5:e01322. doi: 10.1016/j.heliyon.2019.e01322
Gollin, G (2014). Smallholder Agriculture in Africa: An overview and implications for policy. IIED Working Paper. IIED. Available at: https://www.iied.org/sites/default/files/pdfs/migrate/14640IIED.pdf. (Accessed September 23, 2023).
Ha, T. M., Bosch, O. J. H., Nguyen, N. C., and Trinh, C. T. (2017). System dynamics modelling for defining livelihoods strategies for women smallholder farmers in lowland and upland regions of northern Vietnam: a comparative analysis. Agric. Syst. 150, 12–20. doi: 10.1016/j.agsy.2016.09.008
Hadley, D. (2006). Patterns in Technical Efficiency and Technical Change at the Farm-level in England and Wales, 19822002. J. Agric. Econ. 57:81100. doi: 10.1111/j.1477-9552.2006.00033.x
Haile, G., and Haile, B. (2011). Child labour and child schooling in rural Ethiopia: nature and trade-off. Educ. Econ. 20, 365–385. doi: 10.1080/09645292.2011.623376
Hassan, S., Abdelaziz, H., and Ibrahimc, A. (2018). The technical efficiency of dairy farms in Sudan: a stochastic frontier approach. GPH Int. J. Agric. Res. 1, 1–14. Available at: https://gphjournal.org/index.php/ar/article/view/143. (Accessed March 2, 2024).
Hausman, J. (1978). Specification tests in econometrics. Econometrica 46, 1251–1271. doi: 10.2307/1913827
Head, B. (2010). Reconsidering evidence-based policy: key issues and challenges. Polic. Soc. 29, 77–94. doi: 10.1016/j.polsoc.2010.03.001
Helne, T., and Hirvilammi, T. (2015). Wellbeing and sustainability: a relational approach. Sustain. Dev. 23, 167–175. doi: 10.1002/sd.1581
Herrero, M., Thornton, P. K., Bernués, A., Baltenwick, I., Vervoort, J., van de Steeg, J., et al. (2014). Exploring future changes in smallholder farming systems by linking socio-economic scenarios with regional and household models. Glob. Environ. Chang. 24, 165–182. doi: 10.1016/j.gloenvcha.2013.12.008
Hine, J., Abedin, M., Stevens, R. J., Airey, T., and Anderson, T. (2016). Does the Extension of the Rural Road Network Have a Positive Impact on Poverty Reduction and Resilience for the Rural Areas Served? If So How, and If Not Why Not? A Systematic Review. London: EPPI-Centre, Social Science Research Unit, UCL Institute of Education.
Hobson, K., and Lynch, N. (2018). Ecological modernization, techno-politics and social life cycle assessment: a view from human geography. Int. J. Life Cycle Assess. 23, 456–463. doi: 10.1007/s11367-015-1005-5
Hosmer, D. W. Jr., and Lemeshow, S. (1980). Goodness-of-fit tests for the multiple logistic regression model. Commun. Stat. Theory Methods 9, 1043–1069. doi: 10.1080/03610928008827941
Huarachi, D. A. R., Piekarski, C. M., Puglieri, F. N., and de Francisco, A. C. (2020). Past and future of social life cycle assessment: historical evolution and research trends. J. Clean. Prod. 264:121506. doi: 10.1016/j.jclepro.2020.121506
Huertas-Valdivia, I., Ferrari, A. M., Settembre-Blundo, D., and García-Muiña, F. E. (2020). Social life-cycle assessment: a review by bibliometric analysis. Sustain. For. 12. doi: 10.3390/su12156211
IFAD (2020). Madagascar. Country Page. Available at: https://www.ifad.org/en/web/operations/country/id/madagascar#anchor-0. (Accessed 5 June 2024).
IFAD (2024). Agricultural Research for Development. IFAD. Available at: https://ioe.ifad.org/de/web/guest/agricultural-research-for-development. (Accessed February 10, 2024)
IMF (2017). Republic of Madagascar: Economic Development Document. Country Report No. 17/225. Washington, DC: International Monetary Fund.
INSTAT (2011). Deuxieme Recensement General de la Population et de l’Habitation. Rapport Thematique sur les Resultats du RGPH-2, Theme 16: Menages Agricoles a Madagascar. Antananarivo: INSTAT Madagascar.
INSTAT (2020). Troisieme Recensement General de la Population et de l’Habitation. Rapport Thematique sur les Resultats du RGPH-3, Theme 16: Menages Agricoles a Madagascar. Antananarivo: INSTAT Madagascar.
Iofrida, N., De Luca, A. I., Silveri, F., Falcone, G., Stillitano, T., Gulisano, G., et al. (2019). Psychosocial risk factors’ impact pathway for social life cycle assessment: an application to citrus life cycles in South Italy. Int. J. Life Cycle Assess. 24, 767–780. doi: 10.1007/s11367-018-1482-4
Iofrida, N., Strano, A., Gulisano, G., and de Luca, A. I. (2018). Why social life cycle assessment is struggling in development? Int. J. Life Cycle Assess. 23, 201–203. doi: 10.1007/s11367-017-1381-0
Jänicke, M. (1985). Preventive Environmental Policy as Ecological Modernization and Structural Policy. Discussion Paper 85-2. Berlin: International Institute for Environment and Society.
Janker, J., and Mann, S. (2020). Understanding the social dimension of sustainability in agriculture: a critical review of sustainability assessment tools. Environ. Dev. Sustain. 22, 1671–1691. doi: 10.1007/s10668-018-0282-0
Janker, J., Mann, S., and Rist, S. (2019). Social sustainability in agriculture – a system-based framework. J. Rural. Stud. 65, 32–42. doi: 10.1016/j.jrurstud.2018.12.010
Jayne, T. S., Mather, D., and Mghenyi, E. (2010). Principal challenges confronting smallholder agriculture in sub-Saharan Africa. World Dev. 38, 1384–1398. doi: 10.1016/j.worlddev.2010.06.002
Joshi, S, and Schultz, TP (2007) Family Planning as an Investment in Development: Evaluation of a Program’s Consequences in Matlab, Bangladesh. Yale University Economic Growth Center Discussion Paper No. 951. Available at: https://ssrn.com/abstract=962938. (Accessed December 09, 2022).
Kamara, A., Conteh, A., Rhodes, E. R., and Cooke, R. A. (2019). The relevance of smallholder farming to African agricultural growth and development. Afr. J. Food Agric. Nutr. Dev. 19, 14043–14065. doi: 10.18697/ajfand.84.BLFB1010
Kuha, J. (2004). AIC and BIC: comparisons of assumptions and performance. Sociol. Methods Res. 33, 188–229. doi: 10.1177/0049124103262065
Kumbhakar, S. C., and Wang, H.-J. (2010). Estimation of technical inefficiency in production frontier models using cross-sectional data. Indian Econ. Rev. 45, 7–77. Available at: https://www.jstor.org/stable/29793967. (Accessed March 2, 2024).
Larsen, V. G., Tollin, N., Sattrup, P. A., Birkved, M., and Holmboe, T. (2022). What are the challenges in assessing circular economy for the built environment? A literature review on integrating LCA, LCC and S-LCA in life cycle sustainability assessment, LCSA. J. Build. Eng. 50:104203. doi: 10.1016/j.jobe.2022.104203
Lior, N., Radovanović, M., and Filipović, S. (2018). Comparing sustainable development measurement based on different priorities: sustainable development goals, economics, and human well-being—Southeast Europe case. Sustain. Sci. 13, 973–1000. doi: 10.1007/s11625-018-0557-2
Macombe, C. (Ed.) (2016). Social LCA Researcher School Book: Social Evaluation of the Life Cycle, Application to the Agriculture and Agri-Food Sectors (FruiTrop Thema Collection). Montpellier: CIRAD.
Manjula, M., and Rengalakshmi, R. (2021). Making research collaborations: learning from processes of transdisciplinary engagement in agricultural research. Rev. Dev. Change 26, 25–39. doi: 10.1177/09722661211007589
Martiarena, M. L., and Temudo, M. P. (2023). Endogenous learning and innovation in African smallholder agriculture: lessons from Guinea-Bissau. J. Agric. Educ. Ext., 1–19. doi: 10.1080/1389224X.2023.2169480
Marting Vidaurre, N. A., Vargas-Carpintero, R., Wagner, M., Lask, J., and Lewandowski, I. (2020). Social aspects in the assessment of biobased value chains. Sustain. For. 12. doi: 10.3390/su12239843
Masuku, M. B., and Sihlongonyane, M. D. (2015). A stochastic frontier approach to technical efficiency analysis of smallholder dairy farmers in Swaziland. J. Econ. Sustain. Dev. 9, 126–136. Available at: https://api.semanticscholar.org/CorpusID:73601065 (Accessed March 2, 2024).
Masunda, S., and Chiweshe, A. R. (2015). A stochastic frontier analysis on farm level technical efficiency in Zimbabwe: a case of Marirangwe smallholder dairy farmers. J. Dev. Agric. Econ. 7, 237–242. doi: 10.5897/JDAE2014.0630
Mayne, R., Green, D., Gujit, I., Walsh, M., English, R., and Cairney, P. (2018). Using evidence to influence policy: Oxfam’s experience. Palgrave Commun. 4. doi: 10.1057/s41599-018-0176-7
McFadden, D. (1974). “Conditional logit analysis of qualitative choice behavior” in Frontiers in Econometrics. ed. P. Zarembka (New York: Academic Press).
Meeusen, W., and van den Broeck, J. (1977). Efficiency estimation from cobb-Douglas production functions with composed error. Int. Econ. Rev. 18, 435–444. doi: 10.2307/2525757
Mol, A. P. J., and Spaargaren, G. (2000). Ecological modernization theory in debate: a review. Environ. Politics 9, 17–49. doi: 10.1080/09644010008414511
Moyi, P. (2011). Child labor and school attendance in Kenya. Educ. Res. Rev. 6, 26–35. Available at: https://academicjournals.org/journal/ERR/article-full-text-pdf/AB077E84306. (Accessed March 2, 2024).
Mulyasari, G., Djarot, I. N., Sasongko, N. A., and Putra, A. S. (2023). Social-life cycle assessment of oil palm plantation mallholders in Bengkulu province, Indonesia. Heliyon. 9:e19123. doi: 10.1016/j.heliyon.2023.e19123
Munda, G., Albrecht, D., Becker, W., Havari, E., Listorti, G., Ostlaender, N., et al. (2020). “Chapter 18 – the use of quantitative methods in the policy cycle” in Science for Policy Handbook. eds. V. Šucha and M. Sienkiewicz (Amsterdam: Elsevier)
Muthu, S. S. (Ed.) (2018). Social Life Cycle Assessment: Case Studies from Agri and Food Sectors. Singapore: Springer Nature.
Myeki, L. W., Bahta, Y. T., and Matthews, N. (2022). Exploring the growth of agricultural productivity in Africa: a Färe-Primont index approach. Agriculture 12. doi: 10.3390/agriculture12081236
Nakanwagi, T., and Hyuha, T. (2015). Technical efficiency of Milk producers in cattle corridor of Uganda: Kiboga District case. Mod. Econ. 6, 846–856. doi: 10.4236/me.2015.67079
Naudin, K., Autfray, P., Dusserre, J., Penot, É., Raboin, L.-M., Raharison, T., et al. (2019). “Agroecology in Madagascar: from the plant to the landscape” in The Agroecological Transition of Agricultural Systems in the Global South, Agricultures et Défis du Monde Collection, AFD, Éditions Quæ. eds. F.-X. Côte, E. Poirier-Magona, S. Perret, B. Rapidel, P. Roudier, and M.-C. Thirion (Versailles: CIRAD).
Nelson, R., and Coe, R. (2014). Transforming Research and Development practice to support Agroecological intensification of smallholder farming. J. Int. Aff. 67, 107–127. Available at: https://www.proquest.com/scholarly-journals/transforming-research-development-practice/docview/1522799634/se-2. (Accessed March 2, 2024).
Neudert, R., Goetter, J. F., Andriamparany, J. N., and Rakotoarisoa, M. (2015). Income diversification, wealth, education and well-being in rural South-Western Madagascar: results from the Mahafaly region. Dev. South. Afr. 32, 758–784. doi: 10.1080/0376835X.2015.1063982
Ngetich, F. K., Mairura, F. S., Musafiri, C. M., Kiboi, M. N., and Shisanya, C. A. (2022). Smallholders’ coping strategies in response to climate variability in semi-arid agro-ecozones of Upper Eastern Kenya. Social Sciences & Humanities Open. doi: 10.1016/j.ssaho.2022.100319
Nguyen, BH, Sickles, RC, and Zelenyuk, V (2021) Efficiency Analysis with Stochastic Frontier Models Using Popular Statistical Softwares. Working Paper Series No. WP09/2021. CEPA (Centre for Efficiency and Productivity Analysis), School of Economics, University of Queensland, Australia.
Nkamelu, G. B., and Kielland, A. (2006). Modeling farmers’ decisions on child labor and schooling in the cocoa sector: a multinomial logit analysis in cote d’Ivoire. Agric. Econ. 35, 319–333. doi: 10.1111/j.1574-0862.2006.00165.x
Nyambo, D. G., Luhanga, E. T., and Yonah, Z. Q. (2019). A review of characterization approaches for smallholder farmers: towards predictive farm typologies. Sci. World J. 2019, 1–9. doi: 10.1155/2019/6121467
Nyambo, P., Nyambo, P., Mavunganidze, Z., and Nyambo, V. (2022). “Sub-Saharan Africa smallholder farmers agricultural productivity: risks and challenges” in Food Security for African Smallholder Farmers. Sustainability Sciences in Asia and Africa Book Series. eds. H. A. Mupambwa, A. D. Nciizah, P. Nyambo, B. Muchara, and N. N. Gabriel (Singapore: Springer).
Okoye, B. C., Abass, A., Bachwenkiz, B., Asumugha, G., Alenkhe, B., and Ranaivoson, R. (2016). Differentials in technical efficiency among smallholder cassava farmers in Central Madagascar: A Cobb Douglas stochastic frontier production approach. Cogent Economics & Finance. doi: 10.1080/23322039.2016.1143345
Ollenburger, M., Crane, T., Descheemaeker, K., and Giller, K. E. (2019). Are farmers searching for an African Green revolution? Exploring the solution space for agricultural intensification in southern Mali. Exp. Agric. 55, 288–310. doi: 10.1017/S0014479718000169
Ouedraogo, R, and Syrichas, N (2021). Intergenerational Social Mobility in Africa Since 1920. IMF Working Paper No. 2021/215. Available at: https://www.imf.org/en/Publications/WP/Issues/2021/08/06/Intergenerational-Social-Mobility-in-Africa-Since-1920-463400. (Accessed September 18, 2023)
Pennington, D., Wolf, M., Bersani, R., and Pretato, U. (2007). Overcoming barriers to the broader implementation of life cycle thinking in business and public administration. Int. J. Life Cycle Assess. 12, 458–460. doi: 10.1065/lca2007.07.355
Penot, É., Duba, G., Salgado, P., and Dugué, P. (2016). Capacités d’adaptation des exploitations laitières des hautes terres de la province du Vakinankaratra à Madagascar: impacts de la crise de 2009. Rev. Elev. Med. Vet. Pays Trop. 69, 19–31. doi: 10.19182/remvt.31168
Pereira, L. (2017). Climate Change Impacts on Agriculture Across Africa. In Oxford Research Encyclopedia of Environmental Science. Oxford: Oxford University Press.
Peterson, B., and Harrell, F. E. Jr. (1990). Partial proportional odds models for ordinal response variables. J. R. Stat. Soc. Ser. C 39, 205–217.
Petti, L., Serreli, M., and Di Cesare, S. (2018). Systematic literature review in social life cycle assessment. Int. J. Life Cycle Assess. 23, 422–431. doi: 10.1007/s11367-016-1135-4
Pollok, L., Spierling, S., Endres, H.-J., and Grote, U. (2021). Social life cycle assessments: a review on past development, advances and methodological challenges. Sustainability 13:10286. doi: 10.3390/su131810286
Pombo, O., Rivela, B., and Neila, J. (2019). Life cycle thinking toward sustainable development policy-making: the case of energy retrofits. J. Clean. Prod. 206, 267–281. doi: 10.1016/j.jclepro.2018.09.173
Rahmah, D. M., Purnomo, D., Filianty, F., Ardiansah, I., Pramulya, R., and Noguchi, R. (2023). Social life cycle assessment of a coffee production management system in a rural area: a regional evaluation of the coffee industry in West Java, Indonesia. Sustain. For. 15. doi: 10.3390/su151813834
Rapsomanikis, G. (2015). The Economic Lives of Smallholder Farmers: An Analysis Based on Household Data from Nine Countries. Rome: FAO.
Razzu, G., and Wambile, A. (2022). Four decades of intergenerational educational mobility in sub-Saharan Africa. J. Dev. Stud. 58, 931–950. doi: 10.1080/00220388.2021.2008366
Reardon, T. (2015). The hidden middle: the quiet revolution in the midstream of agrifood value chains in developing countries. Oxf. Rev. Econ. Policy 31, 45–63. doi: 10.1093/oxrep/grv011
Ren, J., and Toniolo, S. (2020). Life Cycle Sustainability Assessment for Decision-Making: Methodologies and Case Studies. Amsterdam: Elsevier.
Sadalmelik,. (2008). Map of Madagascar with Vakinankaratra Region highlighted. Available at: https://commons.wikimedia.org/w/index.php?curid=3607410.LicensedunderCreativeCommonsAttribution-ShareAlike4.0International,3.0Unported,2.5Generic,2.0Genericand1.0Generic. (Accessed February 14, 2024).
Sakellariou, N. (2016). A historical perspective on the engineering ideologies of sustainability: the case of SLCA. Int. J. Life Cycle Assess. 23, 445–455. doi: 10.1007/s11367-016-1167-9
Sala, S. (2019). “Life cycle assessment and evaluation of solutions towards sustainable development goals” in Encyclopedia of the UN Sustainable Development Goals. eds. W. Leal Filho, A. Azul, L. Brandli, P. Özuyar, and T. Wall (Cham: Springer)
Schaafsma, M., and Gross-Camp, N. (2021). Towards capturing human well-being-nature relationships in poverty assessments in rural Malawi and Rwanda. Case Stud. Environ. 5:1425104. doi: 10.1525/cse.2021.1425104
Sherli, M. C., and Kani, M. (2018). Need of life cycle thinking for effective utilization of resources based on developed and developing countries: a scenario for future generation. Environ. Sci. Pollut. Res. 25, 7280–7286. doi: 10.1007/s11356-018-1375-x
Sonnemann, G., Gemechu, E. D., Sala, S., Schau, E. M., Allacker, K., Pant, R., et al. (2018). “Life cycle thinking and the use of LCA in policies around the world” in Life Cycle Assessment. eds. M. Hauschild, R. Rosenbaum, and S. Olsen (Cham: Springer).
Sourisseau, J.-M., Bélières, J.-F., Marzin, J., Salgado, P., and Maraux, F. (2019). “The drivers of agroecology in sub-Saharan Africa: an illustration from the Malagasy highlands” in The Agroecological Transition of Agricultural Systems in the Global South, Agricultures et Défis du Monde Collection, AFD, Éditions Quæ. eds. F.-X. Côte, E. Poirier-Magona, S. Perret, B. Rapidel, P. Roudier, and M.-C. Thirion (Versailles: CIRAD).
The African Development Bank Group (AfDBG) (2017). Madagascar 2017–2021 Country Strategy Paper. Available at: https://www.afdb.org/fileadmin/uploads/afdb/Documents/Boards-Documents/Madagascar_-_2017-2021_Country_Strategy_Paper.pdf. (Accessed March 2, 2024).
Thom, A., and Conradie, B. (2024). Modelling the Efficiency of Milk Production on Crop-Livestock Family Farms in Central Madagascar Manuscript Submitted for Publication.
Toenniessen, G., Adesina, A., and DeVries, J. (2008). Building an alliance for a green revolution in Africa. Ann. N. Y. Acad. Sci. 1136, 233–242. doi: 10.1196/annals.1425.028
Tragnone, B. M., D’Eusanio, M., and Petti, L. (2022). The count of what counts in the Agri-food social life cycle assessment. J. Clean. Prod. 354:131624. doi: 10.1016/j.jclepro.2022.131624
Tumen Akyildiz, S., and Ahmed, K. H. (2021). An overview of qualitative research and focus group discussion. Int. J. Acad. Res. Educ. 7, 1–15. doi: 10.17985/ijare.866762
UNCTAD (2015). The Role of Smallholder Farmers in Sustainable Commodities Production and Trade. Report by the UNCTAD Secretariat to the 62nd Session of the Trade and Development Board. Available at: https://unctad.org/system/files/official-document/tdb62d9_en.pdf. (Accessed March 30, 2023).
UNEP (2004). Why Take A Life Cycle Approach? United Nations Environmental Program. Available at: https://sustainabledevelopment.un.org/content/documents/846Why_take_a_life_cycle_approach_EN.pdf. (Accessed October 16, 2023).
UNEP (2009). Guidelines for Social Life Cycle Assessment of Products. Nairobi: United Nations Environment Programme.
UNEP (2020). Guidelines for Social Life Cycle Assessment of Products and Organizations. Nairobi: United Nations Environment Programme.
UNESCO Institute of Statistics (2024). Completion Rate (Primary Education, Lower Secondary Education, Upper Secondary Education). UIS Glossary. UNESCO. Available at: https://uis.unesco.org/en/glossary-term/completion-rate-primary-education-lower-secondary-education-upper-secondary-education. (Accessed February 09, 2024)
UNHLPE (2016). Sustainable agricultural development for food security and nutrition: what roles for livestock? A report by the High Level Panel of Experts on Food Security and Nutrition of the Committee on World Food Security, Rome. Available at: https://www.fao.org/3/a-i5795e.pdf. (Accessed January 21, 2023)
UNICEF (2019a). Madagascar Education Sectoral and OR+ (Thematic) Report: January – December 2018. Available at: https://open.unicef.org/sites/transparency/files/2020-06/Madagascar-TP4-2018.pdf. (Accessed January 22, 2023)
UNICEF (2019b). Madagascar Education Investment Case Executive Summary. Antananarivo: UNICEF. Available at: https://www.unicef.org/esa/sites/unicef.org.esa/files/2019-04/Investment-Case-for-Education-in-Madagascar-Summary-%282016%29.pdf. (Accessed February 11, 2024)
UNOCHA (2022). Madagascar: Humanitarian Snapshot – May 2022. UNOCHA. Available at: https://reliefweb.int/report/madagascar/madagascar-humanitarian-snapshot-may-2022. (Accessed January 22, 2023).
Vall, E. (2020). Emergence of intensive and agro-ecological dairy farming systems. Technical document produced by the Africa-Milk Project. Available at: https://www.africa-milk.org/content/download/4516/33229/version/2/file/Result1.Syst%C3%A8mesdeproductiondelaitvfENG-2.pdf. (Accessed February 1, 2024).
Venkatesh, G. (2019). Critique of selected peer-reviewed publications on applied social life cycle assessment: focus on cases from developing countries. Clean Techn. Environ. Policy 21, 413–430. doi: 10.1007/s10098-018-1644-x
von Grebmer, K., Bernstein, J., Wiemers, M., Schiffer, T., Hanano, A., Towey, O., et al. (2021). Global Hunger Index: Hunger and Food Systems in Conflict Settings. Bonn/Dublin: Welthungerhilfe and Concern Worldwide.
Wei, J., Cui, J., Xu, Y., Li, J., Lei, X., Gao, W., et al. (2022). Social life cycle assessment of major staple grain crops in China. Agriculture 12. doi: 10.3390/agriculture12040535
Wiggins, S. (2014). Presidential address African agricultural development: lessons and challenges. J. Agric. Econ. 65, 529–556. doi: 10.1111/1477-9552.12075
Williams, R. (2006). Generalized ordered logit/partial proportional odds models for ordinal dependent variables. Stata J. 6, 58–82. doi: 10.1177/1536867X0600600104
Williams, R. (2012). Using the margins command to estimate and interpret adjusted predictions and marginal effects. Stata J. 12, 308–331. doi: 10.1177/1536867X1201200209
Wise, T. (2020). Failing Africa’s Farmers: An Impact Assessment of the Alliance for a Green Revolution in Africa. Working Paper No. 20–01. Medford, Global Development and Environment Institute, Tufts University.
Wise, T. A. (2021). Old Fertilizer in New Bottles: Selling the Past as Innovation in Africa’s Green Revolution. Working Paper No. 21–01. Medford: Global Development and Environment Institute, Tufts University.
World Bank (2022a). Poverty and Equity Brief, Africa Eastern and Southern: Madagascar. Available at: https://databankfiles.worldbank.org/public/ddpext_download/poverty/987B9C90-CB9F-4D93-AE8C-750588BF00QA/current/Global_POVEQ_MDG.pdf. (Accessed March 2, 2024).
World Bank (2022b) The State of Global Learning Poverty: 2022 Update. The World Bank, UNESCO, UNICEF, USAID, FSDO, and Bill and Melinda Gates Foundation. Available at: https://www.unicef.org/media/122921/file/StateofLearningPoverty2022.pdf. (Accessed February 09, 2024).
Wouterse, F., and Badiane, O. (2019). The role of health, experience and educational attainment in agricultural production: evidence from smallholders in Burkina Faso. Agric. Econ. 50, 421–434. doi: 10.1111/agec.12500
Keywords: rural development, smallholders, agri-food systems, policy guidance, sustainable development, social impacts analysis, AR4D
Citation: Thom AE, Bélières J-F, Conradie B, Salgado P, Vigne M and Fangueiro D (2024) Exploring social indicators in smallholder food systems: modeling children’s educational outcomes on crop-livestock family farms in Madagascar. Front. Sustain. Food Syst. 8:1356985. doi: 10.3389/fsufs.2024.1356985
Edited by:
Jonathan Steinke, Humboldt University of Berlin, GermanyReviewed by:
Custodio Efraim Matavel, Leibniz Institute for Agricultural Engineering and Bioeconomy (ATB), GermanyMaya Moore, Columbia University, United States
Copyright © 2024 Thom, Bélières, Conradie, Salgado, Vigne and Fangueiro. This is an open-access article distributed under the terms of the Creative Commons Attribution License (CC BY). The use, distribution or reproduction in other forums is permitted, provided the original author(s) and the copyright owner(s) are credited and that the original publication in this journal is cited, in accordance with accepted academic practice. No use, distribution or reproduction is permitted which does not comply with these terms.
*Correspondence: Amy E. Thom, amyethom@gmail.com