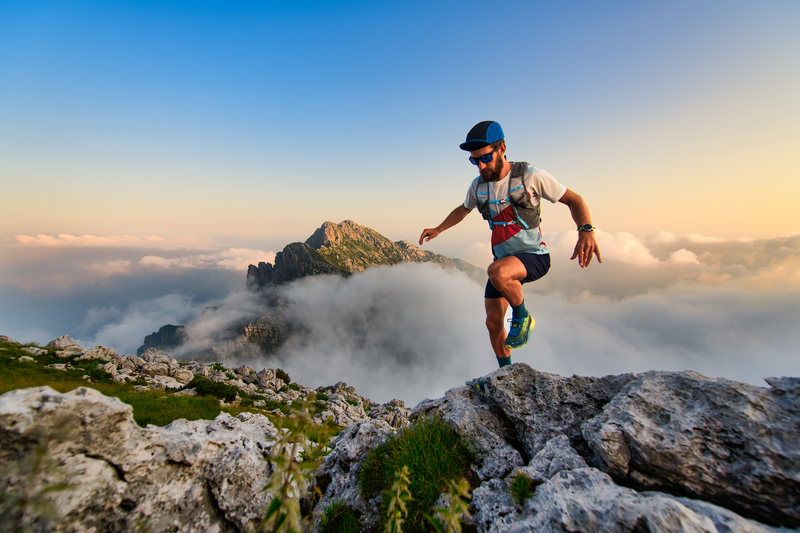
95% of researchers rate our articles as excellent or good
Learn more about the work of our research integrity team to safeguard the quality of each article we publish.
Find out more
ORIGINAL RESEARCH article
Front. Sustain. Food Syst. , 10 April 2024
Sec. Agricultural and Food Economics
Volume 8 - 2024 | https://doi.org/10.3389/fsufs.2024.1340874
A key instrument for upgrading China’s agriculture is the Internet of Things (IoT). To solve the problem of IoT technology promotion, farmers’ intentions to adopt IoT technology must be transformed into behavior, and their intentions and behaviors must be unified. The multivariate logistic model was used to analyses the factors influencing farmers’ intention to adopt IoT technology and behavioral deviation based on survey data of vegetable farmers in Jiangxi Province. The ISM model was used to investigate the relationship and hierarchy between the factors influencing farmers’ intention to adopt IoT technology and behavioral deviation. The findings revealed that first, a significant deviation exists between vegetable farmers’ IoT technology adoption intention and behavior, with 72.69% of those surveyed having the intention to adopt, but did not follow through. Second, among the 12 factors confirmed as remarkably influencing the deviation, expected return is the most superficial factor; intermediate influencing factors include vegetable price volatility, adoption cost, credit support, related knowledge and skills, and technology maturity; secondary factors include social capital, whether vegetables are registered trademarks, planting scale, planting method, and IoT related equipment supply; and age is the deepest root factor.
China has transformed its agricultural and rural areas into one of the fastest-growing countries in the world over the past 40 years of reform and opening-up, with the advancement of agricultural technology serving as one of the key driving engines (Chandio et al., 2022; Zhang et al., 2022). For three consecutive years from to 2020–2022, Document No. 1 of the central government proposed to vigorously promote the construction of the digital countryside; advance the development of smart agriculture; strengthen the construction of modern agricultural facilities; and accelerate the Internet, fifth-generation mobile communication networks, smart meteorology, and other modern agricultural information technology in the field of agriculture. Thus, it can be seen that the application and deep integration of IoT technology in the field of agriculture has received significant attention and strong support from the government, and is a way to achieve rural revitalization, agricultural modernization, and rural transformation (Hou et al., 2018).
To revitalize the countryside, industry must be prioritized. Jiangxi is a big agriculture producing province. The vegetable business is an essential pillar of Jiangxi agriculture, and it is a high-value agricultural industry that is indispensable in stabilizing the supply of “vegetable basket” items, assuring food safety, and increasing farmers’ income (Li Q. et al., 2020). In 2020, the vegetable sowing area in Jiangxi province reached 661,040 hectares, and the total output has reached 16,426,500 tons, which is nearly 20.9% higher than the total output in 2011. The total output has been growing steadily annually under the extremely slow growth of the sowing area in the past decade; hence, the role of modern information technology represented by IoT technology to improve quality and efficiency cannot be ignored. The vegetable industry in Jiangxi Province has formed five advantageous areas, namely, the suburban vegetable production area, vegetable production area for Hong Kong, vegetable production area for the coast and “one belt and one road,” specialty vegetable production area, and aquatic vegetable production area (Li L. et al., 2020). To encourage the implementation of IoT technology in other agricultural industries and the vegetable industry, the Jiangxi Provincial Government has issued policy documents such as the “Jiangxi Provincial Agricultural Rural Modernization Plan,” “Jiangxi Provincial Wisdom Agriculture City and County Construction Guidance,” and “Jiangxi Agricultural Internet of Things Construction Technical Guidance Specifications.” At present, Jiangxi province strongly implements the “123 + N” enhancement project of wisdom agriculture, and by the end of 2022, system interoperability and online data sharing will be completed to service the province’s “Three rural” wisdom agriculture service systems (Chen, 2018).
Illustration on the relevant definitions of scholars, consulting the advice of relevant experts, and considering the conditions of China’s agricultural production and operation and economic development, this project defines “adoption of Internet of Things technology” as the use of IoT technology in the whole process of agricultural production. This includes fine planting in fields, facilities, and greenhouses; agricultural products quality and safety supervision; monitoring agricultural production environmental data (such as soil temperature and humidity, nitrogen and oxygen content, environmental temperature and humidity, light intensity, CO2 concentration, etc.); providing scientific basis for crop production management; providing the best production conditions for crops; effectively improving crop yields and the overall efficiency of agriculture; thereby promoting the level of automation and intelligence in agriculture (Yu and Bai, 2022).
The adoption of agricultural IoT technology is directly influenced by vegetable farmers, and the modernization of the vegetable sector and the efficacy of IoT technology diffusion are directly correlated with the adoption behavior of these farmers. Research on the realization of intention-based behavior reveals that intention is not very effective at predicting behavior (Ma et al., 2020). Intention can only be fully integrated in behavior and have a better sustainability when adoption of the intention is congruent with conduct. This is also when applicable regulations can be most successful. As a result, it’s critical to address the bias that exists between intention and behavior and to help farmers transform their intentions about adopting technology into actual adoption behaviors (Yan et al., 2018).
This study has a strong foundation thanks to the extensive research conducted by a number of researchers on the adoption behaviors of agricultural technology, including IoT, by individual farmers, industrial and agribusiness users, and other subjects. In general, the following elements can be essentially attributed to the factors influencing the intention to use technology. Technology has a role in the first. IoT technology adoption will be aided by perceived benefits and technical compatibility, according to research on this particular segment of the agricultural supply chain (Tu, 2018), while the implementation costs of technological factors affect the adoption of IoT technologies by vegetable producers and suppliers, where producers must plan and estimate their return on investment (Scur et al., 2023). In the cold chain logistics industry, the cost–benefit comparison of IoT adoption determines whether the cold chain logistics body can truly adopt IoT technology (Sun et al., 2020). The second is the user (organization) factor. According to a study, management support is the key factor impacting the digitalization of agri-food businesses. Support from management is essential for effective digitization because it facilitates, leads, and is prepared to take anticipated hazards (Calafat-Marzal et al., 2023). For individual characteristics, a study mainly focused on gender, age, education, income, and business characteristics (Zhang et al., 2018; Omulo et al., 2024). The third involves environmental factors. The environmental component of the user is complex and diverse, and researchers believe that it mainly focuses on factors such as policy environment, infrastructure, and external pressures. However, in reality, farmers express a strong intention to adopt but do not engage in actual adoption behaviors, and there is often a considerable degree of deviation between intention and actual behavior (Yaghoubi et al., 2019). At a glance, there are two views on the relationship between farmers’ intentions and behavior. One view is that intention is a precursor of behavior and can effectively predict behavior (Gudigantala and Bicen, 2016; Peña-García et al., 2020). The other is that although intention is a precursor of action, there are degrees of intention to transform behavior, and behavior can be detached from the intended goal, which suggests that intention and behavior are not always consistent (Zhang et al., 2020; Jenkins et al., 2021).
After combining the existing literature, it is found that from multiple theoretical perspectives, most researchers believe that the causes of will-behavior deviance can be broadly classified into individual factors (Ruiz-Palomino and Martínez-Cañas, 2021), realistic situational factors (Abadi, 2023), perceived behavioral control (Mutyebere et al., 2023), cognitive factors (Davis et al., 1989), other factors (e.g., reward and punishment policies (Zhang M. et al., 2021), and immediate context (Bahaddin et al., 2019), etc.). Therefore, deciphering the will-behavior bias and facilitating the transformation of farmers’ will to adopt technology into actual adoption behavior has become an important practical issue. Moreover, most research has been conducted on industrial enterprises; very little research has been conducted on the adoption of IoT technology by agricultural operators, and very few studies have been published on the deviations of intention and behavior of vegetable farmers. Addressing the bottlenecks of intention and behavior bias in order to better promote IoT technology.
In contradiction of this background, this study takes vegetable farmers as an entry point to explore the deviation in intention and behavior of IoT technology adoption. Whether the vegetable farmers adopt IoT technology is a rational decision-making process, not only because of their resource endowment and perceived behavioral control ability but also because of realistic contextual and technological cognitive factors. Considering that having no intention to engage in behavior rarely occurs in reality, it was not considered the research object of this study. This study focuses on the following three classifications of will-behavior relationships: negative agreement (no will without behavior), paradox (will without behavior), and positive agreement (will with behavior), in which the analysis of the will-behavior paradox (will without behavior) is the focal issue of this study. Therefore, the aim of this study is to provide empirical evidence on vegetable farmers’ intention to adopt IoT technology as well as their actual behavior. To this end, we set the following objectives: (1) to investigate the deviation between vegetable farmers’ words and behaviors regarding IoT technology adoption; (2) to reveal the factors that lead to the deviation between vegetable farmers’ intentions to adopt IoT technology and their behaviors, using positive consistency as the reference group; and (3) to analyze the correlations and hierarchical structure among these factors to provide a basis for guiding the transformation of intention to adopt to actual behaviors. The study’s findings enhance the focus of policy measures in order to eliminate obstacles to the intention to change behavior and, consequently, enable IoT technology to empower the vegetable industry, advance its modernization process, and achieve the sector’s objective of rural revitalization.
The data for this study comes from field research conducted by members of the author’s group, college students, and graduate students at Jiangxi Agricultural University from July 2019 to June 2021. Three to four towns or villages were randomly selected in 20 cities, counties, and districts where vegetable farmers were concentrated, such as Nanchang county, Jinxian county, Ruichang city, Xiushui county, Leping city, Xiangdong district, Fenyi county, Guixi city, Ganxian district, Dingnan county, Xinfeng County, Nankang District, Ga’an City, Jing’an County, Guangfeng District, Yiyang county, Wan’an county, Jinggangshan city, Linchuan district and Nancheng county. Based on this, 10–15 households were randomly selected from each township’s vegetable farmer to conduct a questionnaire survey in the form of one-on-one interviews. Author’s group distribute a total of 800 questionnaires and 679 were collected, with a recovery rate of 84.9%. Among them, 546 questionnaires were valid, with an effective rate of approximately 80.4%. The basic characteristics of the sample are shown in Table 1. The majority of vegetable farmers are male (89.2%); age is concentrated between 40 and 59 years old, and the overall education level is relatively low. From the social capital perspective, the proportion of growers with 51–100 telephone numbers is 26.7%, and the proportion of growers with more than 100 telephone numbers is as high as 58.1%, indicating that vegetable growers are richer in social capital overall, which may be due to the fact that rural society is a society of acquaintances. From the viewpoint of whether the vegetables are registered, majority (453; 83%) of vegetable farmers do not have registered trademarks while 93 (17%) have registered trademarks, which shows that most vegetables sold by the growers are not registered and lack brand certification. Regarding having a contract with the buyer, most growers do not have a contractual relationship with the buyer, with a proportion as high as 73.4% (401 people); only 26.6% (145 people) of the growers have a contract with the buyer; hence, vegetable farmers selling vegetables face greater market risks, and the awareness of signing contracts is low.
The intention and behavior of vegetable farmers in adopting IoT technology are shown in Table 2. The outcomes show that about 49.7% vegetable farmers had the intention to adopt IoT technology, whereas only 13.6% had adopted IoT technology. Among the 271 growers who had the intention to adopt IoT technology, only 74 actually adopted IoT technology, indicating that there is a deviation between vegetable farmers’ intention to adopt IoT technology and their behavior. Hence, it is meaningful to study the deviation between vegetable farmers’ intention to adopt IoT technology and their behavior.
Nineteen variables were adopted to explore their effects on vegetable farmers’ intentions to adopt IoT technologies and behavioral deviations, including farmers’ factors, real-life situational factors, perceived behavioral control, and technological perception. The selection and description of each variable are listed in Table 3.
The explanatory variable for this study was the relationship between intention and behavior, which was generated after processing. Vegetable farmers were asked whether they intended to adopt IoT technology in their vegetable production process, and declarative intention was used as the criterion. In addition, it was found that although some vegetable farmers had not included IoT technology in their production process at the time of the survey, they were already building IoT technology or had purchased IoT-related equipment and products. Because it takes time to build and install IoT technology from the time the equipment is purchased to the time it is put into use, this group of vegetable farmers is also considered to have adoption behavior. In the empirical analysis, the relationship between intention and behavior was defined as an unordered categorical variable. This study focused on the factors impacting the paradox of intention and behavior with a positive consistent reference group.
In this study, the vegetable farmers’ factors were selected as control variables, including eight variables: gender, age, education level, social capital, number of year-round home-based farmers, total household income, whether the vegetables were registered trademarks, and whether they were a contract with the buyer. Of these, the number of people working at home all year round and the total household income were continuous variables (Mastenbroek et al., 2021; Wang et al., 2021). For the empirical analysis, the total household income was logarithmically treated (Mulokozi et al., 2020).
The core explanatory variables of this study included realistic contextual factors, perceived behavioral control, and technological perception. Among them, the real situation factors can be divided into the technology application environment, market environment, and policy environment, which vegetable farmers have to consider when adopting IoT technologies (Ma et al., 2022). The technology application environment cannot be separated from the consideration of current farmers’ planting characteristics, mainly including planting scale and planting methods (Bing, 2016; Yi et al., 2022). The market environment includes the magnitude of vegetable price fluctuations, IoT-related equipment supply, and adoption costs (Luong et al., 2016; Wu and Ma, 2020). The policy environment mainly includes credit support (Tuan et al., 2020). Perceived behavioral control mainly refers to growers’ knowledge and skills related to information technology and relevant experience in implementing information technology or information system projects (Yang et al., 2018); technological perception mainly refers to growers’ perceived ease of use of IoT technology, perceived maturity of the technology, and expectations of the benefits of adopting IoT technology for growing vegetables (Shi et al., 2022; Strong et al., 2022).
The relationship between vegetable farmers’ intention to adopt IoT technologies and their behavior is the explanatory variable in this study, which takes the form of negative agreement (no intention and behavior), deviation (intention without behavior), and positive agreement (intention with behavior). Although theoretical cases of no intention or behavior exist, they are extremely rare in reality and have not been examined in this study. This study aims to scrutinize the factors influencing the behavioral deviance of vegetable farmers’ intention to adopt IoT technologies, while capturing the role of the positive consistent variable. Therefore, this study uses a multi-categorical logistic regression model with positive consistency as the reference group to reveal the factors influencing vegetable farmers’ intention to adopt IoT technologies and behavioral deviance in the following form:
In Equation (1), is the intercept term, βi represents the estimated coefficient, represents the explanatory variable, and is the error term. The probability of bias arising from vegetable farmers’ IoT technology adoption intentions and behavior is:
In Equation (2), e is the base of the natural logarithm, which is estimated as:
In Equation (3), the event occurrence ratios are the probability occurrence ratios for Type I (negative agreement), Type II (deviations), and Type III events (positive agreement).
This study argues that influencing vegetable farmers’ IoT technology adoption decisions is a complex social activity that occurs due to the influence of multiple interacting factors, and that the ISM method can be used to analyze the interactions and correlations between the factors more effectively. The significant influencing factors that affect vegetable farmers’ intention to adopt IoT technologies and behavioral dissonance can either directly affect intention and behavioral dissonance or indirectly affect them through the interaction of factors that ultimately lead to the outcome of intention and behavioral dissonance. Specific approaches include the following.
The first step was to determine the adjacency matrix . Based on the empirical analysis, it is concluded that there are factors that influence vegetable farmers’ intention to adopt IoT technology and behavioral deviation. indicates vegetable farmers’ intention to adopt IoT technology behavioral deviation, indicates the influencing factor, and the formula for constructing the adjacency matrix is as Equation (4):
The second step is to determine the reachable matrix 1, which is obtained using Boolean operations following Equation (5), where 2 is the unit matrix and is .
In the third step, the hierarchical structure of the factors is determined. In Equation (6), the reachable matrix is divided into a reachable set and an antecedent set , both of which represent the set of factors that can be obtained from the factor in the reachable matrix . Both represent the factors in the reachable matrix. Finally, the highest level was determined by Equation (7) to be the vegetable farmers’ intention to adopt IoT technology and behavioral deviance, as well as the hierarchical structure and correlations of the influencing factors it contains.
Finally, a logical hierarchy of influencing factors affecting vegetable farmers’ intention to adopt IoT technologies and behavioral paradoxes is constructed by connecting adjacent layers and each influencing factor in the same layer with arrows.
The results of the multi-category logistic regression analysis on the factors influencing vegetable farmers’ intention to adopt IoT technologies and behavioral biases using the Stata 15.0 econometric software are shown in Table 4. The log-likelihood of the model was −265.092 and LR chi2 was 544.470, with p-values much less than 0.05, indicating that the model is significant and valid for regression analysis.
Table 4. Analysis of factors influencing vegetable farmers’ intention to adopt IoT technologies and behavioral biases.
First, for the control variables (farmer factors) on adoption intentions and behavioral biases, age passes the 5 and 10% significance levels in Models 1 and 2, respectively, with positive coefficients, indicating that age positively affects negative consistency and deviation of intentions and behavior. Generally speaking, the physical condition, learning ability, and risk attitude of growers usually change; with limited physical condition, reduced learning ability, and increased fear of risk-taking as they get older, the more reluctant they are to adopt new technologies. Even if they express an intention to adopt, they may be “too intend to do so” due to age, thus increasing the negative consistency between intention and behavior. This increases the probability of negative consistency and divergence between intentions and behavior.
Social capital is statistically significant at 10% in both Models 1 and 2, and the coefficients are negative, suggesting that social capital negatively influences the probability of negative intention-behavior agreement and deviance. A possible reason for this is that the richer the social capital of vegetable farmers, the wider their social networks, the easier it is to access information, and the lower the transaction costs of adopting new technologies; therefore, the less likely it is that negative agreement and divergence will occur, and the more likely it is that the intentions held will be transformed into behavior.
Whether vegetables are registered is significant at the 10% level in both models, and the coefficients are negative, indicating that whether vegetables are registered has a negative effect on the negative agreement and deviation of intentions and behaviors. Growers whose vegetables (and their products) were trademarked were less likely to adopt negative consistency or deviance than those whose vegetables were unregistered. The reason for this is that registered vegetables are more likely to gain buyers’ favor and trust in the market, thereby enhancing the marketability of growers, helping to promote the sale of vegetable products, increasing the income of operators, and making them more capable of adopting IoT; this has many advantages, such as ensuring accurate production, controlled yield, and quality safety of vegetables. Hence, intention is more likely to translate into behavior, and the probability of negative alignment and divergence between intention and behavior is lower.
Second, in terms of the real-life contextual factors on adoption intention and behavioral bias, the IoT technology application environment of farmers must consider the current planting characteristics; the planting scale and planting method passed the 1 and 5% significance levels in Models 1 and 2, respectively. The scale of planting has a dampening effect on the negative agreement and deviation of intention and behavior; the larger the scale of planting, the lower the probability of negative agreement and deviation of intention and behavior. This is because larger farmers have economies of scale in the adoption of IoT technologies (Zhang M. et al., 2021; Zhang S. et al., 2021) and are more active in rural areas and affairs than smaller farmers. Consequently, they are less likely to have negative or inconsistent intentions and behaviors. Small households are often less endowed, more information-closed, and more conservative, so they are more likely to have neither the will nor the behavior to adopt. Moreover, even if they do have the will, they are reluctant to adopt IoT technology due to risk aversion, and tend to “say one thing but do another.” This is because a large field is a complex system with many uncertainties, which makes it more difficult to process and analyze information (Jianwei, 2017), as well as to apply IoT technologies.
The magnitude of vegetable price volatility passed the 5% level of significance in both Models 1 and 2, and the direction of the effect was negative in both cases. A possible explanation for this is that vegetable price volatility implies that vegetable farmers face greater market pressure and that there is more urgency to adopt new technologies in response to external pressures; this negatively influences the occurrence of negative agreements and paradoxes between intention and behavior. The supply of IoT-related equipment passed the 5% significance level in both Models 1 and 2, and the coefficients were both negative, indicating that the more adequate the supply of IoT-related equipment, the lower the probability of negative consistency and deviation of intention and behavior.
China’s agricultural IoT technology is under development and is an integrated technology that relies on various devices in the sensing, transmission, processing, and application layers; for example, the sensing layer relies on light intensity, temperature and humidity, CO2 sensors, the transmission layer relies on devices such as sensing networks and mobile networks; the processing layer relies on devices such as cloud computing and image and video processing; and the application layer relies on integrated management platform APP, monitoring and management platforms, secondary development components, and other devices (Da Xu et al., 2014). Therefore, the adequacy of the supply of IoT-related devices will directly affect whether intention can be transformed into behavior. The cost of adoption passes only the 10% significance level in Model 2, with a negative effect direction. The reason for this is that the lower the cost of IoT adoption, the more affordable the adoption cost for growers, thereby reducing the deviation from intention to behavior and tending to transform intention to behavior.
Credit support passed the 10 and 5% significance levels in Models 1 and 2, respectively, and the coefficients were both negative, indicating that growers who received credit support were less likely to have a negative will-behavior paradox than those who did not receive credit support. This is because the adoption of IoT technology requires greater capital investment, and growers who receive credit support are less likely to deviate from their behavior as their financial constraints are weakened.
Third, in terms of perceived behavioral control on adoption intentions and behavioral biases, knowledge and skills passed the 1% significance level in both Models 1 and 2 with negative coefficients, indicating that the more knowledge and skills vegetable farmers possess, the less likely they are to adopt IoT technologies with negative consistency and deviation from behavior. The possible reason for this is that growers with relevant knowledge and skills tend to be more knowledgeable about IoT technologies, more technologically literate, and have higher self-efficacy in adopting IoT technologies, which tends to transform their intentions into behaviors and reduce the divergence between intentions and behaviors.
Fourth, in terms of technological perceptions on adoption intentions and behavioral deviations, technology maturity passed the 1 and 5% significance levels in Models 1 and 2, respectively, and the coefficients were both positive. The question on technological maturity is an inverse question, with larger values representing lower technological maturity. The results of the regression analysis show that the larger the value of technological maturity, the greater the probability of negative agreement and deviation of intention and behavior, i.e., the less mature the technology perceived by farmers, the more likely it is to lead to negative agreement and deviation of intention and behavior. This is because the lower the maturity of the IoT technology, the less guaranteed the expected benefits of using it in vegetable production and management, and the greater the risk that farmers will not be able to recover the adoption cost and initial investment; hence, it is difficult for farmers to have confidence and trust in the IoT technology. Even if they express their intention to adopt it, they will not be able to bear the technological risk which will lead to a negative intention-behavior relationship.
Revenue expectations passed the 1 and 5% significance levels in Models 1 and 2, respectively, and the coefficients were both negative, indicating that the better the expected revenue, the lower the probability of negative alignment and divergence between intention and behavior. This is because growers are rational economists and will fully consider the benefits of the technology before adoption; the more positive the expected benefits of IoT technology, the higher their confidence in IoT technology adoption and the easier it is to put their adoption intentions into action.
Referring to the robustness tests of existing results (Nastis et al., 2019), we used a multivariate Probit model to test the robustness of the factors influencing vegetable farmers’ intention to adopt IoT technologies and behavioral biases; the results are in Table 5. The results of the robustness test indicate that, after replacing the regression model, the multivariate Probit model is significant and valid. The significance of the explanatory variables and the positive and negative signs of the regression coefficients are highly consistent with the multi-categorical logistic regression, showing that the empirical results of this study are more robust.
Based on the multi-categorical logistic regression analysis, 12 factors influencing vegetable farmers’ intention to adopt IoT technologies and behavioral paradoxes were extracted. S0 denotes vegetable farmers’ intention to adopt IoT technology and behavioral dissonance, and Si(Sj) (i = 1, 2, …, 12, j = 1, 2, …, 12) is used to denote the above 12 factors that significantly affect vegetable farmers’ intention to adopt IoT technology and behavioral dissonance, as follows: S1 to S12 represent age, social capital, whether the vegetables are registered trademarks, scale of cultivation, cultivation method, price volatility of vegetables, supply of IoT-related equipment, cost of adoption, credit support, relevant knowledge and skills, technology maturity, and expectation of benefits.
Based on consultation with experts in the field of agricultural IoT and other agricultural technology adoption, the interactions and logical relationships between the factors are determined by combining the results of existing literature and theoretical analysis. If row factor i influences column factor j, it is denoted by V; if column factor j influences row factor i, then it is denoted by A; if row factor i and column factor j have no influencing relationship, then it is denoted by O. Based on this, this study identified the logical relationship between the 12 influencing factors and the interaction between vegetable farmers’ intention to adopt IoT technologies and behavioral deviance (Zhang et al., 2020; Guo et al., 2022), as detailed in Table 6.
According to the logical relationship diagram of the factors influencing IoT technology adoption intention and the behavioral paradox of vegetable farmers in Table 6, the adjacency matrix is , as shown in Figure 1. Using the Boolean algorithm and with the help of Matlab 7.0 software, the reachable matrix M is calculated, as shown in Figure 2. Subsequently, the hierarchy among the influencing factors and the factors at each level were determined to extract a skeleton matrix N of factors influencing vegetable farmers’ intention to adopt IoT technologies and behavioral paradoxes, as shown in Figure 3.
Figure 3. Skeleton matrix of factors influencing IoT technology adoption intentions and behavioral paradoxes among vegetable farmers.
Finally, based on the extracted skeleton matrix, a multilevel recursive directed graph was formed to clarify the correlations and hierarchical structure among the influencing factors. As shown in Figure 4, this study constructs a 5-level recursive directed graph, which can visually analyze the surface-level direct influencing factors, intermediate-level indirect influencing factors, and deeper-level root influencing factors of vegetable farmers’ intention to adopt IoT technologies and behavioral deviations.
From the skeleton matrix and the 5-level progressive directed graph, it can be seen that the factors influencing the paradox of growers’ intention to adopt IoT technologies and their behavior can be divided into five levels, of which Level 1 is S0, i.e., paradox of intention and behavior; Level 2 is S12, i.e., benefit expectations; Level 3 includes five factors S6, S8, S9, S10, and S11, i.e., the magnitude of vegetable price fluctuations, adoption costs, credit support, relevant knowledge and skills, and technological maturity; Tier 4 also includes five factors S7, S2, S5, S4, and S3, i.e., IoT-related equipment supply, social capital, growing practices, growing scale, and whether the vegetables are registered trademarks; and Tier 5 is S1, i.e., age. The following is a detailed discussion of the surface-level direct, middle-level indirect, and deep-rooted root factors for the paradox of vegetable farmers’ IoT technology adoption intentions and behaviors.
From the previous analysis, it can be seen that the benefit expectation factor is at the level of direct influence on the deviation of intention and behavior, and is the most superficial and direct factor that triggers the deviation of intention and behavior. As rational individuals, vegetable farmers’ primary consideration when making decisions on the adoption of IoT technologies is economic benefits, which is in line with the “economic man” hypothesis. Research has also established that benefit expectations have a strong influence on the deviation of intention and behavior (Li et al., 2021). The reason for this is that, as Britz et al. (2013) show, “as rational humans, maximizing returns is the main objective of agricultural producers” (Britz et al., 2013); therefore, expected returns are the most direct consideration for growers’ IoT technology. Only if growers perceive the expected benefits of IoT adoption to be good are they more likely to translate their adoption intentions into actual action; otherwise, the translation of adoption intentions into behavior is severely impeded.
As shown in Figure 4, the indirect influences in the middle tier are those in layers 3 and 4. First, the factors in layer 3 include the real-life contextual factors of price volatility, adoption costs, and credit support; the perceived behavioral control factors of knowledge and skills are also included, as well as the perceived maturity of the technology, all of which ultimately influence the intention to adopt and behavioral deviations by affecting expected benefits. Clearly, the technology adoption process is influenced by a complex combination of interacting factors. In general, the magnitude of vegetable price volatility directly leads to increased market risk, which in turn affects farmers’ perceptions of the expected benefits of IoT. The cost of adoption usually represents the input required to use IoT technology, and high costs affect the perception of expected benefits. Credit support reduces financial constraints, making growers more likely to recognize the economic benefits of IoT technology. The more knowledgeable and skilled growers are, the more likely they are to adopt IoT technology. Moreover, the more knowledgeable and skilled growers are, the better they understand IoT technology and the more likely they are to be optimistic about the expected benefits. The higher the maturity of the technology, the better the results achieved by IoT applications in vegetable farming and the higher the expected benefits; thus, the less likely they are to deviate from their intentions and behaviors. The factors in Level 4 include whether the vegetables are registered, social capital, planting scale, planting method, and supply of IoT-related equipment. These factors affect technological maturity in the technological perception factor in Level 3, which in turn affects the deviation of intention and behavior through expected benefits. In addition, the availability of registered trademarks for vegetables, social capital, scale of cultivation, and cultivation methods also act on benefit expectations by influencing the knowledge and skills in the perceived behavioral control factors in layer 3, which in turn influences the deviation in intention to adopt IoT technologies and behavior through benefit expectations.
The age variable is in layer 5 of the directed graph, suggesting that age is a deep-seated root cause of the deviation between vegetable farmers’ intention to adopt IoT technologies and their behavior. Age affects social capital and planting scale in layer 4, which, in turn, affects the deviation of intention and behavior through the interaction of various factors between layers. Currently, vegetable farmers in Jiangxi province are generally in the middle and old age groups, and this group has been engaged in vegetable farming in rural areas for many years. Older vegetable farmers are reluctant to adopt new technologies due to their lack of labor capacity and conservative thinking (Huang et al., 2019), cannot conduct large-scale production operations, and are unable to expand their social capital. Thus, age has a deep-rooted effect on the intention to adopt IoT technologies and behavioral dissonance.
Based on a total of 546 survey data of vegetable farmers in Jiangxi Province, a multi-categorical logistic model was used to reveal the factors affecting vegetable farmers’ IoT technology adoption intention and behavioral deviation, and an ISM model was used to analyze the correlations and hierarchical structure between the influencing factors. The specific findings are as follows:
1. The relationship between vegetable farmers’ intention to adopt IoT technology and their adoption behavior was generally “strong intention, weak behavior.” Nearly half (49.7%) of the growers expressed their intention to adopt, but as many as 36.1% showed a deviation between intention and behavior (intention without behavior); this indicates that there is a deviation between the intention and behavior of vegetable farmers in adopting IoT technologies.
2. The factors affecting the adoption of IoT technologies by vegetable farmers are not only farmers’ factors but also real situation factors, perceived behavioral control, and technology awareness factors. In addition to age and technological maturity, social capital, whether the vegetables are registered trademarks, planting scale, planting method, price fluctuation of vegetables, IoT-related equipment supply, adoption cost, credit support, related knowledge and skills, and expectation of profit all negatively affect intention and behavioral dissonance.
3. The ISM model was used to analyze the hierarchical structure and correlation between the significant influences of vegetable farmers’ intention to adopt IoT technology and behavioral deviance. The results showed that the hierarchical structure of the factors affecting vegetable farmers’ intention to adopt IoT technology and behavioral deviance can be divided into a 5-level recursive directed graph. The secondary influences are social capital, whether the vegetables are registered trademarks, the scale of cultivation, cultivation methods, and the supply of IoT-related equipment; the deepest root factor is age.
To conclude, we propose a number of recommendations. First, the government should use various forms of medium- and long-term vocational training for young vegetable farmers to accelerate the process of professionalization of new vegetables and to form a stable team of young professional vegetable farmers. Second, land transfer policies and vegetable scale management subsidies must be improved, and a standardized rural land transfer market should be established to promote vegetable scale management. There must also be a registration of vegetable trademarks, building and maintaining of quality vegetable regional brands, an enhancement of vegetable market competitiveness to stabilize sales, and a formation of a complete and stable vegetable production and marketing chain. Third, the government should improve the supply of IoT-related equipment and support further optimization of agricultural IoT technology, while increasing credit policy support to reduce the cost of IoT technology adoption. Fourth, it is imperative to reinforce the training and publicity of IoT technology through various means, such as media publicity, public opinion guidance, and acquaintance review, to enhance vegetable farmers’ awareness of IoT technology.
It is undeniable that we must take this study’s limitations into account. Initially, the study’s data was limited to vegetable farmers in Jiangxi province, China. This is because different economic development regions have different resource endowments. However, in the future, we may think about comparing data from other regions or even the entire nation in order to support China’s rural transformation and modernization of agriculture. Second, it should be noted that this study only looked at the hierarchical structure of influencing factors that affect vegetable farmers’ intention to adopt IoT technology and behavioral deviation. More research is needed to determine which specific factors, like risk preference and government regulation (Zhang et al., 2023; Yang et al., 2024), can close this gap.
The raw data supporting the conclusions of this article will be made available by the authors, without undue reservation.
LL: Conceptualization, Data curation, Formal analysis, Funding acquisition, Project administration, Resources, Writing – original draft. MZ: Data curation, Formal analysis, Investigation, Methodology, Software, Writing – original draft, Writing – review & editing. AC: Methodology, Software, Supervision, Validation, Visualization, Writing – review & editing. YL: Validation, Visualization, Writing – review & editing.
The author(s) declare financial support was received for the research, authorship, and/or publication of this article. This work was supported by the National Natural Science Foundation of China’s “Smart Planting Willingness and Behavior of Large and Specialized Vegetable Farms: Transformation Mechanisms, Spillover Effects and Policy Design” (No. 72263016); the National Natural Science Foundation of China’s “Internet of Things Technology Adoption Behavior, Diffusion Effect and Guiding Policy for Large and Specialized Vegetable Farms” (No. 71863018).
The authors would like to acknowledge the financial support by the National Natural Science Foundation of China (Grant No. 72263016 and No. 71863018).
The authors declare that the research was conducted in the absence of any commercial or financial relationships that could be construed as a potential conflict of interest.
All claims expressed in this article are solely those of the authors and do not necessarily represent those of their affiliated organizations, or those of the publisher, the editors and the reviewers. Any product that may be evaluated in this article, or claim that may be made by its manufacturer, is not guaranteed or endorsed by the publisher.
Abadi, B. (2023). Impact of attitudes, factual and causal feedback on farmers’ behavioral intention to manage and recycle agricultural plastic waste and debris. J. Clean. Prod. 424:138773. doi: 10.1016/j.jclepro.2023.138773
Bahaddin, B., Weinberg, S., Luna-Reyes, L. F., and Andersen, D. (2019). Building a bridge to behavioral economics: countervailing cognitive biases in lifetime saving decisions. Syst. Dyn. Rev. 35, 187–207. doi: 10.1002/sdr.1631
Bing, F., The research of IOT of agriculture based on three layers architecture. In: 2016 2nd international conference on cloud computing and internet of things (CCIOT), IEEE, (2016), pp. 162–165.
Britz, W., Ferris, M., and Kuhn, A. (2013). Modeling water allocating institutions based on multiple optimization problems with equilibrium constraints. Environ. Model Softw. 46, 196–207. doi: 10.1016/j.envsoft.2013.03.010
Calafat-Marzal, C., Sánchez-García, M., Marti, L., and Puertas, R. (2023). Agri-food 4.0: drivers and links to innovation and eco-innovation. Comput. Electron. Agric. 207:107700. doi: 10.1016/j.compag.2023.107700
Chandio, A. A., Sethi, N., Dash, D. P., and Usman, M. (2022). Towards sustainable food production: what role ICT and technological development can play for cereal production in Asian–7 countries? Comput. Electron. Agric. 202:107368. doi: 10.1016/j.compag.2022.107368
Chen, X. (2018). Forty years of rural reform in China: retrospect and future prospects. China Agricult. Econ. Rev. 11, 460–470. doi: 10.1108/CAER-08-2018-0162
Da Xu, L., He, W., and Li, S. (2014). Internet of things in industries: a survey. IEEE Trans. Industr. Inform. 10, 2233–2243. doi: 10.1109/TII.2014.2300753
Davis, F. D., Bagozzi, R. P., and Warshaw, P. R. (1989). User acceptance of computer technology: a comparison of two theoretical models. Manag. Sci. 35, 982–1003. doi: 10.1287/mnsc.35.8.982
Gudigantala, N., and Bicen, P. (2016). An examination of antecedents of conversion rates of e-commerce retailers. Manag. Res. Rev. 39, 82–114. doi: 10.1108/MRR-05-2014-0112
Guo, H., Zhao, W., Pan, C., Qiu, G., Xu, S., and Liu, S. (2022). Study on the influencing factors of farmers’ adoption of conservation tillage technology in black soil region in China: a logistic-ISM model approach. Int. J. Environ. Res. Public Health 19:7762. doi: 10.3390/ijerph19137762
Hou, J., Huo, X., and Yin, R. (2018). Does computer usage change farmers’ production and consumption? Evidence from China. China Agricult. Econ. Rev. 11, 387–410. doi: 10.1108/CAER-09-2016-0149
Huang, X., Lu, Q., Wang, L., Cui, M., and Yang, F. (2019). Does aging and off-farm employment hinder farmers’ adoption behavior of soil and water conservation technology in the loess plateau? Int. J. Clim. Change Strat. Manag. 12, 92–107. doi: 10.1108/IJCCSM-04-2019-0021
Jenkins, J. L., Durcikova, A., and Nunamaker, J. F. (2021). Mitigating the security intention-behavior gap: The moderating role of required effort on the intention-behavior relationship. J. Assoc. Inform. Sys. 22:1. doi: 10.17705/1jais.00660
Jianwei, W. (2017). Development model research of Chinese agriculture IoT. J. Agric. Sci. Technol. 19:10. doi: 10.13304/j.nykjdb.2016.758
Li, L., Hu, J., and Teng, Y. (2020). Protection and renewal of traditional villages in Jiangxi Province under the background of rural revitalization——taking Futang Village as an example, In: E3S Web of Conferences, EDP Sciences, 05039. doi: 10.1051/e3sconf/202019405039
Li, C., Shi, Y., Khan, S. U., and Zhao, M. (2021). Research on the impact of agricultural green production on farmers’ technical efficiency: evidence from China. Environ. Sci. Pollut. Res. 28, 38535–38551. doi: 10.1007/s11356-021-13417-4
Li, Q., Xu, G., Wei, J., Lu, H., Nie, Y., and Chen, X. (2020). Research on current situation, problems and countermeasures of vegetable industry development of Jiangxi Province. Acta Agricult. Jiangxi 32, 125–130. doi: 10.19386/j.cnki.jxnyxb.2020.02.23
Luong, N. C., Hoang, D. T., Wang, P., Niyato, D., Kim, D. I., and Han, Z. (2016). Data collection and wireless communication in internet of things (IoT) using economic analysis and pricing models: a survey. IEEE Commun Surv Tutor 18, 2546–2590. doi: 10.1109/COMST.2016.2582841
Ma, W., Grafton, R. Q., and Renwick, A. (2020). Smartphone use and income growth in rural China: empirical results and policy implications. Electron. Commer. Res. 20, 713–736. doi: 10.1007/s10660-018-9323-x
Ma, Q., Zheng, S., and Deng, P. (2022). Impact of internet use on farmers’ organic fertilizer application behavior under the climate change context: the role of social network. Land 11:1601. doi: 10.3390/land11091601
Mastenbroek, A., Sirutyte, I., and Sparrow, R. (2021). Information barriers to adoption of agricultural technologies: willingness to pay for certified seed of an open pollinated maize variety in northern Uganda. J. Agric. Econ. 72, 180–201. doi: 10.1111/1477-9552.12395
Mulokozi, D. P., Mmanda, F. P., Onyango, P., Lundh, T., Tamatamah, R., and Berg, H. (2020). Rural aquaculture: assessment of its contribution to household income and farmers’ perception in selected districts, Tanzania. Aquacult. Econ. Manag. 24, 387–405. doi: 10.1080/13657305.2020.1725687
Mutyebere, R., Twongyirwe, R., Sekajugo, J., Kabaseke, C., Kagoro-Rugunda, G., Kervyn, M., et al. (2023). Does the farmer’s social information network matter? Explaining adoption behavior for disaster risk reduction measures using the theory of planned behavior. Int. J. Disaster Risk Reduct. 92:103721. doi: 10.1016/j.ijdrr.2023.103721
Nastis, S. A., Mattas, K., and Baourakis, G. (2019). Understanding farmers’ behavior towards sustainable practices and their perceptions of risk. Sustain. For. 11:1303. doi: 10.3390/su11051303
Omulo, G., Daum, T., Koller, K., and Birner, R. (2024). Unpacking the behavioral intentions of ‘emergent farmers’ towards mechanized conservation agriculture in Zambia. Land Use Policy 136:106979. doi: 10.1016/j.landusepol.2023.106979
Peña-García, N., Gil-Saura, I., Rodríguez-Orejuela, A., and Siqueira-Junior, J. R. (2020). Purchase intention and purchase behavior online: a cross-cultural approach. Heliyon 6:e04284. doi: 10.1016/j.heliyon.2020.e04284
Ruiz-Palomino, P., and Martínez-Cañas, R. (2021). From opportunity recognition to the start-up phase: the moderating role of family and friends-based entrepreneurial social networks. Int. Entrep. Manag. J. 17, 1159–1182. doi: 10.1007/s11365-020-00734-2
Scur, G., da Silva, A. V. D., Mattos, C. A., and Gonçalves, R. F. (2023). Analysis of IoT adoption for vegetable crop cultivation: multiple case studies. Technol. Forecast. Soc. Chang. 191:122452. doi: 10.1016/j.techfore.2023.122452
Shi, Y., Siddik, A. B., Masukujjaman, M., Zheng, G., Hamayun, M., and Ibrahim, A. M. (2022). The antecedents of willingness to adopt and pay for the IoT in the agricultural industry: an application of the UTAUT 2 theory. Sustain. For. 14:6640. doi: 10.3390/su14116640
Strong, R., Wynn, J. T., Lindner, J. R., and Palmer, K. (2022). Evaluating Brazilian agriculturalists’ IoT smart agriculture adoption barriers: understanding stakeholder salience prior to launching an innovation. Sensors 22:6833. doi: 10.3390/s22186833
Sun, L., Zhao, Y., Sun, W., and Liu, Z. (2020). Study on supply chain strategy based on cost income model and multi-access edge computing under the background of the internet of things. Neural Comput. Applic. 32, 15357–15368. doi: 10.1007/s00521-019-04125-9
Tu, M. (2018). An exploratory study of internet of things (IoT) adoption intention in logistics and supply chain management: a mixed research approach, the. Int. J. Logist. Manag. 29, 131–151. doi: 10.1108/IJLM-11-2016-0274
Tuan, D. M., Ha, N. X., and Xuyen, N. H. (2020). Germany’s policies on developing IOT (internet of things) technology market and lessons for Vietnam. Eur. Sci. Rev. 11-12, 72–78. doi: 10.29013/ESR-20-11.12-72-78
Wang, W., Lan, Y., and Wang, X. (2021). Impact of livelihood capital endowment on poverty alleviation of households under rural land consolidation. Land Use Policy 109:105608. doi: 10.1016/j.landusepol.2021.105608
Wu, F., and Ma, J. (2020). Evolution dynamics of agricultural internet of things technology promotion and adoption in China. Discret. Dyn. Nat. Soc. 2020, 1–18. doi: 10.1155/2020/1854193
Yaghoubi, J., Yazdanpanah, M., and Komendantova, N. (2019). Iranian agriculture advisors' perception and intention toward biofuel: green way toward energy security, rural development and climate change mitigation. Renew. Energy 130, 452–459. doi: 10.1016/j.renene.2018.06.081
Yan, B., Jin, Z., Liu, L., and Liu, S. (2018). Factors influencing the adoption of the internet of things in supply chains. J. Evol. Econ. 28, 523–545. doi: 10.1007/s00191-017-0527-3
Yang, Y., Choi, J. N., and Lee, K. (2018). Theory of planned behavior and different forms of organizational change behavior. Soc. Behav. Personal. Int. J. 46, 1657–1671. doi: 10.2224/sbp.6832
Yang, C., Liang, X., Xue, Y., Zhang, Y., and Xue, Y. (2024). Can government regulation weak the gap between green production intention and behavior? Based on the perspective of farmers' perceptions. J. Clean. Prod. 434:139743. doi: 10.1016/j.jclepro.2023.139743
Yi, X., Sauer, J., and Yin, C. (2022). Explaining farmers’ reluctance to adopt green manure cover crops planting for sustainable agriculture in Northwest China[J]. J. Integr. Agricul. 21, 3382–3394. doi: 10.1016/j.jia.2022.09.005
Yu, H., and Bai, X. (2022). Strengthen or weaken? Research on the influence of internet use on agricultural green production efficiency, Frontiers in environmental. Science 10:2088. doi: 10.3389/fenvs.2022.1018540
Zhang, H., Chandio, A. A., Yang, F., Tang, Y., Ankrah Twumasi, M., and Sargani, G. R. (2022). Modeling the impact of climatological factors and technological revolution on soybean yield: evidence from 13-major provinces of China. Int. J. Environ. Res. Public Health 19:5708. doi: 10.3390/ijerph19095708
Zhang, Y., Halder, P., Zhang, X., and Qu, M. (2020). Analyzing the deviation between farmers' land transfer intention and behavior in China's impoverished mountainous area: a logistic-ISM model approach. Land Use Policy 94:104534. doi: 10.1016/j.landusepol.2020.104534
Zhang, S., Hu, D., Lin, T., Li, W., Zhao, R., Yang, H., et al. (2021). Determinants affecting residents’ waste classification intention and behavior: a study based on TPB and ABC methodology. J. Environ. Manag. 290:112591. doi: 10.1016/j.jenvman.2021.112591
Zhang, Y.-J., Jin, Y.-L., and Zhu, T.-T. (2018). The health effects of individual characteristics and environmental factors in China: evidence from the hierarchical linear model. J. Clean. Prod. 194, 554–563. doi: 10.1016/j.jclepro.2018.05.163
Zhang, W., Khan, A., Luo, Y., Qi, T., and Zhao, M. (2023). How do risk preferences influence forage planting behaviors among farmers in the agro-pastoral ecotone of China? Front. Sustain. Food Syst 7:1252626. doi: 10.3389/fsufs.2023.1252626
Zhang, M., Wang, X., Feng, H., Huang, Q., Xiao, X., and Zhang, X. (2021). Wearable internet of things enabled precision livestock farming in smart farms: a review of technical solutions for precise perception, biocompatibility, and sustainability monitoring. J. Clean. Prod. 312:127712. doi: 10.1016/j.jclepro.2021.127712
Keywords: IoT technology adoption, vegetable farmers, logistic-ISM model, China, sustainable vegetables production, intention, behaviour
Citation: Li L, Zhang M, Chandio AA and Liu Y (2024) Investigating the intention and behavior of vegetable farmers to adopt IoT technology: survey—based evidence from China. Front. Sustain. Food Syst. 8:1340874. doi: 10.3389/fsufs.2024.1340874
Received: 19 November 2023; Accepted: 25 March 2024;
Published: 10 April 2024.
Edited by:
Athula Naranpanawa, Griffith University, AustraliaReviewed by:
Josef Abrham, Czech University of Life Sciences Prague, CzechiaCopyright © 2024 Li, Zhang, Chandio and Liu. This is an open-access article distributed under the terms of the Creative Commons Attribution License (CC BY). The use, distribution or reproduction in other forums is permitted, provided the original author(s) and the copyright owner(s) are credited and that the original publication in this journal is cited, in accordance with accepted academic practice. No use, distribution or reproduction is permitted which does not comply with these terms.
*Correspondence: Abbas Ali Chandio, YWxpY2hhbmRpb0BzaWNhdS5lZHUuY24=
†ORCID: Lianying Li, https://orcid.org/0000-0003-4847-3491
Disclaimer: All claims expressed in this article are solely those of the authors and do not necessarily represent those of their affiliated organizations, or those of the publisher, the editors and the reviewers. Any product that may be evaluated in this article or claim that may be made by its manufacturer is not guaranteed or endorsed by the publisher.
Research integrity at Frontiers
Learn more about the work of our research integrity team to safeguard the quality of each article we publish.