- 1Department of Agricultural Economics, Wolaita Sodo University, Wolaita Sodo, Ethiopia
- 2Department of Economics, Addis Ababa University, Addis Ababa, Ethiopia
Introduction: This research investigates the impact of credit constraints on the yield of smallholder ginger farmers in southern and central Ethiopia. It addresses the importance of understanding the relationship between credit constraints and agricultural productivity in smallholder farming systems.
Methods: Employing a cross-sectional dataset from 343 randomly selected households, we utilized the endogenous switching regression model to address potential sample selection bias.
Results: The analysis in the first stage showed that livestock holding, marital status, farm size, distance to credit source, and information access are primary determinants influencing the credit constraint status of smallholder ginger farmers. In the second stage, the analysis revealed that family size, farm size, and cooperative membership significantly affected ginger yield. Moreover, the average treatment effect suggests a significant impact of credit constraints on ginger yield, with credit-constrained farmers experiencing a greater positive effect compared to credit-unconstrained farmers. These findings highlight the complex relationships between credit constraints, socioeconomic factors, and agricultural yield in the context of smallholder ginger farming.
Discussion: The implications of this research extend to informing policy decisions and intervention strategies aimed at alleviating credit constraints and enhancing the overall yield and livelihoods of smallholder ginger farmers in the studied regions. Policy recommendations include prioritizing interventions to enhance ginger yield by promoting cooperative membership, improving access to credit sources, fostering livestock ownership, and reducing the distance to credit sources. Additionally, enhancing access to information for effective mitigation of credit constraints is crucial for boosting productivity in smallholder ginger farming.
1 Introduction
In 2021, an alarming 2.3 billion people worldwide grappled with food security (Galanakis, 2020). Within this Africa, bore the brunt with over one-third, approximately 795 million people, struggling to access adequate nutrition (Bashari, 2023). This unsettling reality underscores the urgent need to address hunger and malnutrition as part of the sustainable development goals (SDGs). Goal 2, “Zero Hunger,” epitomizes this imperative, aiming to end hunger, achieve food security, improve nutrition, and promote sustainable agriculture by 2030 (Muchunguzi, 2023). To achieve the sustainable development goals (SDGs) and ensure sustainable food access, urgent action is needed (Rafael, 2017). Addressing food insecurity requires boosting agricultural production, especially in developing nations where agriculture is pivotal (Bhatt et al., 2018). Supporting farmers, the backbone of agriculture, and tackling impediments to intensification are crucial to averting the looming threat of global food insecurity (Ahmadzai et al., 2021).
Addressing the challenge of improving agricultural productivity in sub-Saharan Africa, particularly in relation to land area, stands as a critical goal for the 21st century (Jayne et al., 2021). Despite the continent’s vast agricultural potential, Africa lags behind other continents in terms of yield, with substantial variations between regions (Tian and Yu, 2019). The proposed solution to enhance productivity is grounded in the concept of the “green revolution,” which advocates for the widespread adoption of inputs such as fertilizers, irrigation, improved seeds, and technologies (Tadele, 2017; Qaim, 2020).
In Ethiopia, smallholder farmers constitute a significant portion of the population, relying heavily on agriculture for their livelihoods (Akanmu et al., 2023). Ginger, renowned globally as a versatile spice, holds significant agricultural importance in Ethiopia, both for local consumption and export, due to favorable climatic and soil conditions and a longstanding cultivation tradition (Access et al., 2012). Despite the nation’s substantial potential for ginger production, it remains confined to select regions in southern Ethiopia, with minor cultivation in southwestern Oromia and northern Amhara (Hordofa and Tolossa, 2020). In 2019, ginger production in Ethiopia surged to 445,000 metric tons, positioning the country as the second-largest ginger producer in Africa, following Nigeria, according to data from the Ethiopian Ministry of Agriculture (Kifile et al., 2023). Over the past two decades (2004–2022), Ethiopia has exported a total of 86,820 tons of ginger. Notably, the majority of this export volume, approximately 95%, occurred within the first decade (2004–2013), followed by a substantial decline in the second decade (2014–2022) (FAOSTAT, 2022).
However, ginger production in Ethiopia confronts several daunting challenges, including a high incidence of ginger diseases, suboptimal agronomic practices, limited marketable surplus due to poor quality, inadequate attention from policymakers and development practitioners, and notably, financial constraints, such as limited access to credit (Kifile et al., 2023). This financial constraint significantly impedes smallholder farmers’ ability to invest in essential inputs such as fertilizers, irrigation systems, and improved seeds, thus hampering productivity and overall yield. Without sufficient financial resources, farmers face difficulties in adopting modern agricultural practices and technologies Sekyi et al. (2020), widening the yield gap between Ethiopia and other regions. This credit constraint not only stifles agricultural productivity but also perpetuates cycles of poverty and food insecurity among smallholder farmers. As they are unable to invest in their farms, farmers remain trapped in subsistence farming practices, unable to break free from the cycle of low yields and meager incomes. Moreover, the impact of credit constraints extends beyond individual farmers to the broader economy, impeding the country’s efforts to achieve sustainable development goals, particularly SDG 2: Zero Hunger.
The Resilient Agriculture for Inclusive and Sustainable Ethiopian Food System (RAISE-FS) program supports ginger production in southern Ethiopia by fostering research and collaboration, yielding solutions such as disease management strategies, which have enhanced yields (Abate and Schaapp, 2022). Nevertheless, scaling these interventions may face limitations due to resource constraints and agricultural complexities. Several organizations, including Areka Agricultural Research Center, Stichting Wageningen Research Ethiopia, Ethiopian Institute of Agricultural Research, and Wageningen Center for Development Innovation, actively support ginger production through research and technical assistance (Kifile et al., 2023). Continuous evaluation is essential to gauge the effectiveness of these interventions.
Amidst these challenges, the role of credit constraints in aggravating the difficulties faced by smallholder ginger farmers has become increasingly apparent (Kifile et al., 2023). The scarcity of financial resources impedes farmers’ ability to adopt improved technologies, secure high-yielding varieties, and implement effective disease and pest management strategies (Sekyi et al., 2020). Consequently, there is a pressing need to investigate the socioeconomic determinants of credit constraints among ginger farmers in southern and central Ethiopia and understand the consequent impact on ginger yield.
Existing literature, represented by studies, such as Ayodele and Sambo (2014), Gobena (2016), and Ogbanje and Igboko (2019), lacks a comprehensive assessment of credit constraints in ginger farming and their productivity impact. This study aimed to investigate these factors among smallholder ginger farmers in southern and central Ethiopia. Examining the relationship between credit constraints, input adoption, productivity, and ginger yield addressed gaps in understanding the socioeconomic factors influencing ginger production.
Smallholder farmers face difficulty accessing affordable credit, hindering investment in modern inputs and technologies (Balana and Oyeyemi, 2022). Our study aimed to fill this gap by investigating the socioeconomic determinants of credit constraints among ginger farmers, shedding light on their implications for productivity. Through comprehensive research, we aimed to inform policymakers, practitioners, and stakeholders, facilitating evidence-based interventions to promote sustainable agricultural development in the region.
The rest of the article is organized as follows: section 2 is a literature review, section 3 presents the methodology, section 4 presents the results and discussion, and section 5 concludes this article by presenting policy implications.
2 Literature review
2.1 Theoretical approach
This study is based on the theory of utility maximization, which assumes the rational behavior of ginger producers in their activities. The choice to employ utility maximization theory over key theories, such as agricultural growth theory (Barrett et al., 2010), credit constraint theory (Jack, 2013), and socioeconomic theory (Kang and Sawada, 2008), stems from the study’s precise focus and objectives. While these key theories offer valuable insights into broader agricultural productivity dynamics, utility maximization theory provides a more targeted approach to examining the relationship between credit service constraints and agricultural yields in ginger farming. Utility maximization theory posits that ginger producers make rational decisions to maximize their utility or satisfaction, considering their preferences and constraints, particularly in obtaining the full requested amount of credit. The theoretical analysis of the factors influencing farmers’ credit utilization behavior and its impact on agricultural yield is depicted in Figure 1. This framework allows for a detailed analysis of the factors influencing farmers’ credit utilization behavior, incorporating individual preferences and strategic considerations.
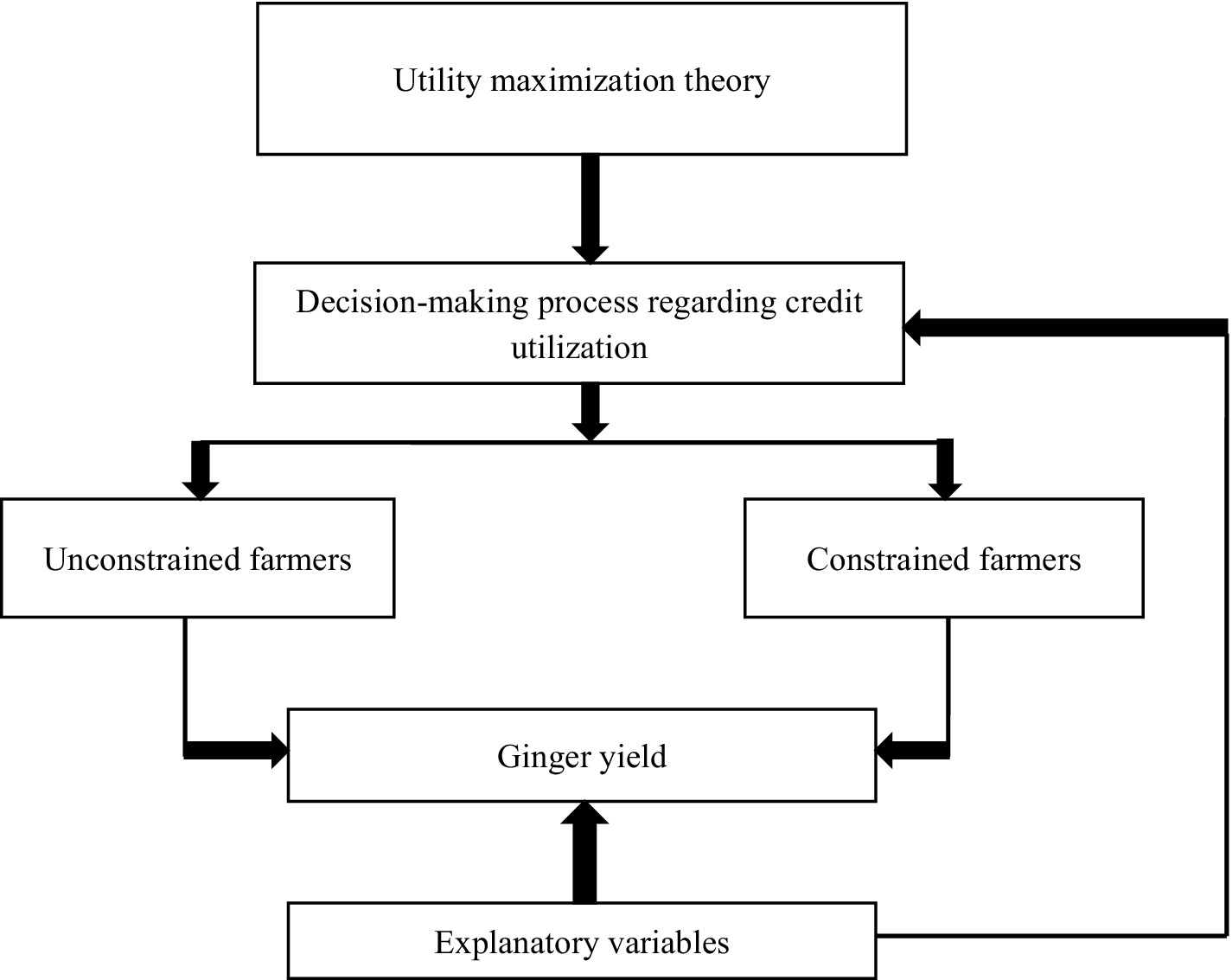
Figure 1. Conceptual framework of the study. Source: Adopted from Awunyo-Vitor (2018).
Moreover, utility maximization theory facilitates a micro-level examination of decision-making processes within the agricultural sector, complementing the broader theoretical perspectives of the key theories. By focusing on how individual decisions translate into agricultural productivity outcomes, the study aims to deepen the understanding of the mechanisms underlying farmers’ credit utilization behavior and its impact on agricultural yields, thereby enhancing the insights provided by the key theories. A farmer’s rational decision to apply for a loan should be based on the fact that the benefit of receiving the requested full amount is greater than the benefit of not obtaining it. Using the direct elicitation approach (Ali et al., 2014), only farmers who have received the entire requested amount are considered as unconstrained, while the others are considered constrained (Supplementary Appendix I).
The utility derived from credit utilization by ginger farmers is then described as follows:
With:
Equation (1) represents the utility derived from credit utilization by ginger producers, Where , is utility derived from credit utilization by ginger producers and which is a function of the set of explanatory variables that would affect the credit unconstrained ginger producers, α represents the coefficient or parameter associated with the explanatory variables affecting the credit availability for unconstrained ginger producers, and represents the error term. Equations (2) and (3) utilized to represent utility derived from credit utilization by credit unconstrained and constrained producers respectively. Similarly, the difference in expected utility from using credit is not directly observable, but what could be observed is farmers’ decision to use credit (Ali and Awade, 2019). Let denote the net marginal benefit of having access to full amount of credit (the dummy latent variable). It is defined based on the binary indicator of whether a farmer has access to the full amount of credit or not and described as follows:
Then, the utility function of a ginger farmer takes the form as follows:
Here, Equation (4) represents the net marginal benefit of having access to the full amount of credit, where a value of 1 is assigned if the farmer is credit unconstrained, and 0 if the farmer is credit constrained. Equation (5) presents the utility function of a ginger farmer incorporating the net marginal benefit term. Assuming that being credit unconstrained is derived from expected utility maximization subject to the socioeconomic characteristics of typical ginger farmers. Assuming that being credit unconstrained is derived from expected utility maximization subject to the socioeconomic characteristics of typical ginger farmers.
2.2 Conceptual framework
The conceptual framework delves into the intricate interplay between credit constraints and agricultural yield, guided by the principles of utility maximization theory and the rational decision-making process of ginger producers (Awunyo-Vitor, 2018). As highlighted by Jack (2013), credit constraints serve as formidable barriers limiting farmers’ access to essential financial resources for agricultural investments. This framework systematically outlines the crucial elements influencing farmers’ credit utilization behavior and its subsequent impact on agricultural productivity. Expected utility maximization forms the cornerstone of farmers’ decision-making, where they carefully weigh the benefits of obtaining credits against associated costs and risks (Ali and Awade, 2019). This rational approach extends to allocating agricultural inputs, such as labor, seeds, and machinery, with farmers strategically optimizing their input combinations based on expected utility from credit utilization (Barrett et al., 2008). Additionally, the adoption of innovative agricultural technologies, informed by farmers’ utility maximization decisions, plays a pivotal role in enhancing productivity and mitigating the adverse effects of credit constraints (Kang and Sawada, 2008). The socioeconomic factors, including household income and education level, further shape farmers’ utility-maximizing behavior regarding credit utilization, underscoring the broader socioeconomic context influencing agricultural outcomes (Teklewold et al., 2013). Ultimately, ginger yield emerges as the key outcome variable, reflecting the efficacy of farmers’ utility-maximizing decisions in navigating credit constraints and optimizing input allocations to achieve enhanced productivity and food security. Figure 1 below shows the conceptual framework of the study.
2.3 Analytical approach
Estimating the impact without experimental observations is a very difficult method due to selection bias and lack of counterfactual data (Wooldridge, 2003). Both unobservable characteristics of farmers and their farms can influence the decision to use or participate in any scenario, which could lead to misestimation of the impact on the outcome variables (Meskel et al., 2020). The study by Ali and Awade (2019) indicated that the characteristics of farmers who received the full amount of the requested credit service could be systematically different from those who did not receive it. This could possibly lead to the occurrence of self-selection, thus leading to a correlation of the error terms of both equations (Kim and Mokhtarian, 2023). To mitigate these challenges, researchers often employ various econometric methods. Traditional approaches include the Heckman two-step approach or instrumental variable (IV) regression. However, these methods come with assumptions, including the normal distribution of unobserved variables in the two-step procedures and the challenge of finding suitable instruments for IV regression. Additionally, both OLS and IV procedures assume a linear functional form, implying similar coefficients for control variables across credit access and non-access groups. Alternatively, propensity score matching (PSM) requires no assumption about the functional form but assumes that the yield function differs only by observable factors. However, this assumption can lead to biased estimates when yield function differs and involves unobservable factors influencing both credit access and ginger yield.
Endogenous switching regression models indeed offer a promising avenue for impact assessment studies, particularly in situations where treatment assignment is not random and individuals self-select into treatment. However, these models are not without their limitations. One major limitation is the potential for selection bias, wherein individuals who choose to participate in the treatment group may differ systematically from those who do not. To address this, endogenous switching regression models incorporate selection equations to account for the self-selection process. Another limitation is the assumption of unobserved heterogeneity, where unobserved factors influence both treatment selection and outcome. Techniques such as instrumental variables or control function approaches are often employed to mitigate this issue. Despite these limitations, the strength of endogenous switching regression models lies in their ability to simultaneously model both the selection process and the outcome equation, thus providing more robust estimates of treatment effects compared to other models (Murtazashvili and Wooldridge, 2016). This comprehensive approach allows researchers to better understand the impact of interventions while accounting for self-selection biases and unobserved heterogeneity, making it a preferable choice for impact assessment studies in complex social settings.
3 Methodology
3.1 Description of the study area
The study was conducted in two districts, Boloso-Bombe and Hadaro-Tunto, located in the Wolaita zone and Kembata-Tembaro zone, respectively. These zones were reorganized under different regional states as a result of the Ethiopian government’s reformation in 2018. The Wolaita zone is now part of southern Ethiopia, serving as an administrative and political center, while the Kembata-Tembaro zone is situated in central Ethiopia. The administrative structure comprises 22 districts in the Wolaita zone and 8 districts in the Kembata-Tembaro zone. The selected districts are known for their predominant reliance on agriculture, with ginger production being a significant contributor to the local economy.
Agriculture, especially crop production, is the main livelihood of both districts, with ginger production taking the lion’s share, followed by cereals such as maize and teff, root crops such as taro and sweet potato, and animal husbandry. Figure 2 below shows the map of the study area.
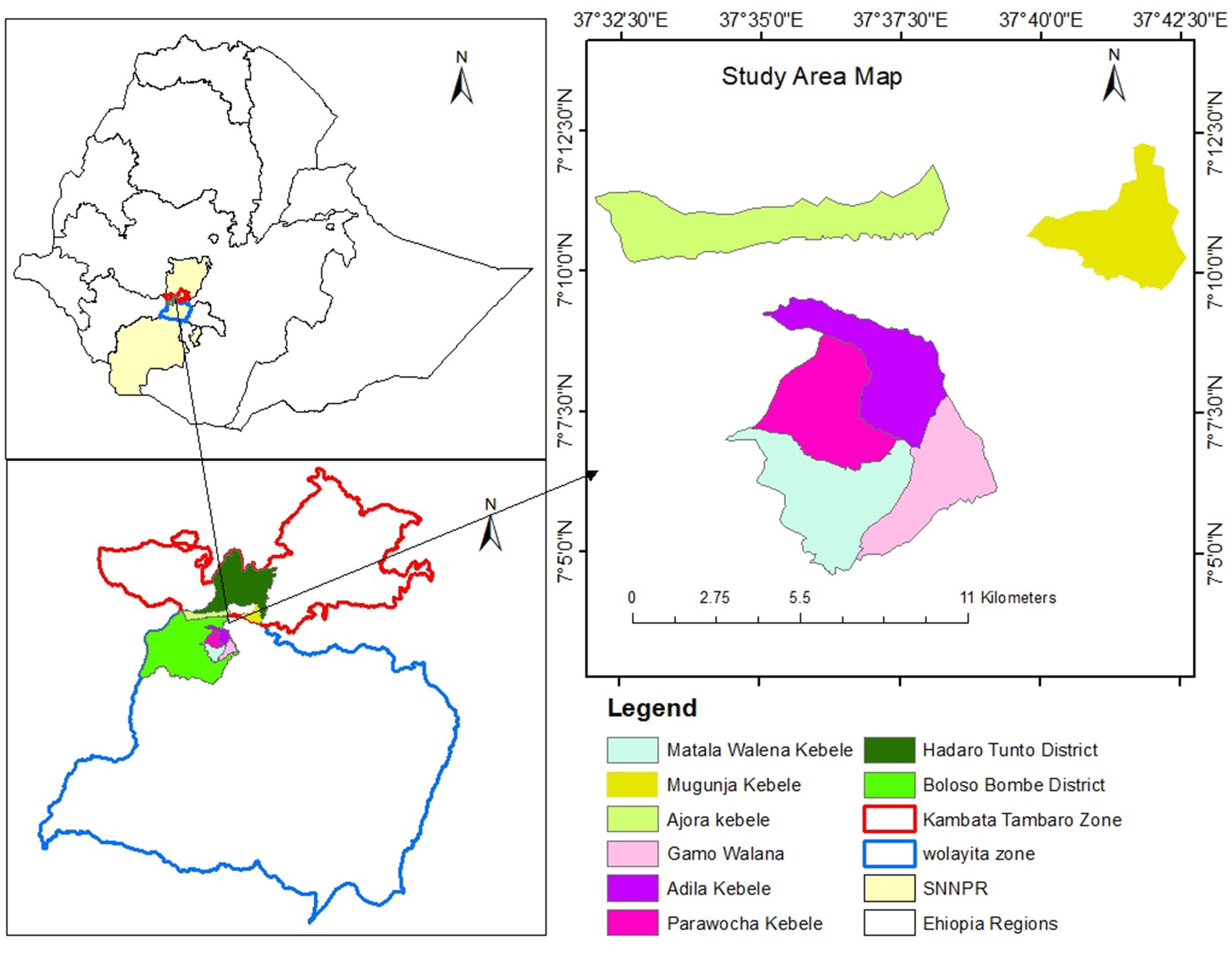
Figure 2. Map of the study area. Source: Sketched by using (ArcGIS, 2022).
3.2 Selection of sample size and sample size design
To ensure a robust sampling strategy, we employed a multistage sampling approach to select our sample respondents. First, we identified two agricultural zones in the study area: the Wolaita zone, comprising the Boloso-Bombe district, and the Kembata-Tembaro zone, including the Hadaro-Tunto district. These zones were chosen due to their significant ginger production activities. The two districts, Boloso-Bombe and Hadaro-Tunto, from the Wolaita and Kembata-Tembaro zones, respectively, were purposively selected in the first stage as they are known for ginger production in terms of ginger land area coverage and total ginger production in the southern and central regions, respectively (Prameela and Suseela Bhai, 2020). Moving to the second stage, we employed a quota sampling method to select kebeles within each district. Randomly, we chose 4 kebeles from the 18 kebeles in the Boloso-Bombe district and 2 kebeles from the 16 rural kebeles in the Hadaro-Tunto district. This selection was based on the proportion of ginger-producing kebeles in each district, ensuring representation across the study area. Kothari (2004) provides several sample size determination formulas based on different statistical considerations. Kothari (2004) provides several sample size determination formulas based on different statistical considerations. One such formula, commonly used in survey research, is given by Equation (6):
where n represents the sample size; Z represents the cumulative standard distribution, which corresponds to the confidence level with a value of 1.96; e is the desired precision level, as suggested by Israel (1992); a p-value of 0.5 indicates the estimated proportion of an attribute present in the population required to obtain the desired minimum level of sample size at the 95% confidence level and 5% precision; q = 1 – p; and N represents the total size of the population from which the sample is drawn.
Subsequently, within the selected kebeles, we utilized a simple random sampling technique to choose households for participation. From the 3208 households in the selected kebeles, we randomly selected 343 households. The distribution of the sample within each kebele was proportional to the number of ginger farmers. For instance, in the Boloso-Bombe district, 64 households were selected from Gamo Walalna, 29 from Matala Walana, 67 from Parawocha, and 50 from Adila. Similarly, in the Hadaro-Tunto district, 66 households were chosen from Mukurunja and 67 from Ajora. The sample size distribution is presented in Table 1.
The sampling method and sample size determination described above provided a representative and statistically valid approach to data collection in the Bolso-Bombe and Hadaro-Tunto districts to provide valuable insights into the impact of credit constraints on ginger yields in the southern and central regional states of Ethiopia.
3.3 Source of data
The data for this study were gathered through structured interviews and questionnaires administered to ginger farmers from March 2023 to June 2023. The questionnaire, which specifically targeted ginger farmers, focused exclusively on gathering information pertaining to the year 2022. In-depth interviews were conducted to ensure comprehensive data collection. Before the main data collection, a pre-test of the questionnaire was conducted to address any uncertainties. The questionnaires included sections on respondents’ socioeconomic and demographic characteristics, credit access (from formal and informal sources), farm information, such as ginger yield, and other relevant variables. A cross-sectional research design was employed to investigate the impact of credit constraints on the yield of smallholder ginger farmers in southern and central Ethiopia. Before the main data collection, a pilot survey involving five farmers from each kebele was conducted to refine the questionnaire’s clarity, comprehension, and relevance. The data collection process was facilitated by trained enumerators who possessed a deep understanding of the local context and agricultural practices specific to ginger farming. A structured questionnaire, meticulously designed to cover various aspects such as demographics, agricultural practices, credit access, credit participation, yield, and relevant variables, was administered to 343 smallholder ginger farmers.
3.4 Variable definition and prior expectation
In our study, we examined the association between credit constraint (treatment variable) and ginger yield (outcome variable). The study included 1 treatment variable, 13 independent variables, and 1 outcome variable. Credit constraint is central to the study, serving as the treatment variable that distinguishes households as either constrained or unconstrained in accessing credit resources. Ginger yield, measured in quintals per hectare, serves as the outcome variable, reflecting productivity in ginger cultivation. Formal sources of credit constraints include cooperatives, microfinance institutions, and development banks, while informal sources encompass relatives, wealthy farmers, money lenders, rotating savings and credit associations, and social networks.
Based on the previous studies, the variables included in the study are crucial determinants of credit access and household welfare, as well as agricultural productivity and efficiency. These include demographic factors such as sex, age, marital status, and education level (Economics and Library, 2017; Mukasa et al., 2017), as well as socioeconomic factors such as family size, farm size, distance to credit source, and total livestock ownership (Rada and Fuglie, 2019; Asiamah et al., 2021). Other variables, such as experience in production, extension contact, access to information, involvement in off-farm activities, and cooperative membership, further affect credit access and contribute to household welfare and food security (Awunyo-vitor et al., 2013; Ali and Deininger, 2014). To ensure clarity and accessibility, all variables used in the study are defined in Supplementary Appendix II.
The endogenous switching regression (ESR) model was employed to estimate the impact of credit constraints on ginger yield. To attain consistent, unbiased, and valid outcomes before the estimation process, multicollinearity, omitted variables, and heteroscedasticity issues were checked. The Variance Inflation Factor (VIF) values for the variables indicate that multicollinearity is not a concern, as all VIF values are below the commonly accepted threshold of 10. The mean VIF is 1.21, further confirming the absence of multicollinearity among the variables (Supplementary Appendix IV). The Breusch–Pagan heteroscedasticity insignificant test (prob > chi2 = 0.498) showed the data have no heteroscedasticity problem (Supplementary Appendix V). Similarly, the omitted variable test (ovtest) (prob > F = 0.7969) suggested that there was no omitted variable problem in the model specification (Supplementary Appendix VI).
3.5 Model specifications
The aim of the study was to understand the impact of credit constraints on the yield of smallholder ginger farmers. The ESR model consisted of two stages, the first of which used a probit model to assess credit constraints among smallholder ginger farmers. This stage aimed to determine the likelihood of farmers facing credit constraints based on various predictor variables. The probit model estimated the probability of being credit-constrained, considering socioeconomic and demographic characteristics. The model is represented by Equation (7):
Where is the aggregate distribution function of the standard normal distribution representing a vector of exogenous variables affecting the credit constraint, is a vector of exogenous variables affecting the credit constraint, and is a vector of coefficients to be estimated. Smallholder farmers were divided into two groups based on the probabilities estimated by the probit model. A common threshold (e.g., 0.5) was used to classify farmers into constrained and not constrained in the credit market.
After classifying smallholder farmers into treatment groups (unconstrained) and control groups (constrained) based on their predicted probabilities of constraint condition, the second stage estimated the outcome equation for each group separately:
For the treatment group (T = 0):
For the control group (T = 1):
Where Y0 and Y1 denote potential outcomes for the treatment (Equation 8a) and control groups (Equation 8b), respectively; X represents a vector of exogenous variables influencing the yield; β1 and β2 are vectors of coefficients to be estimated; and U0 and U1 are the error terms assumed to be normally distributed.
To account for potential endogeneity in the credit constraint equation, instrumental variables were introduced. The instrumental variables were variables that correlated with the endogenous explanatory variable (credit constraint) but did not correlate with the error term in the outcome equation. The instrumental variables isolated exogenous variability in the credit constraint conditions. The Pearson correlation analysis assessed instrumental validity, unveiling a significant positive correlation between distance to credit source and credit constraint (treatment variable), indicating a robust link to credit constraint. Conversely, no significant correlation was detected between distance to credit source and yield (outcome variable), implying minimal influence on yield (Supplementary Appendix III). Therefore, following the studies by Shiferaw et al. (2014) and Khonje et al. (2015), distance to credit source was used as an instrument in this study.
Understanding the treatment effect is essential in research and policy-making to gauge the impact of interventions and assess their outcomes. This study focused on the Average Treatment Effect on the Treated (ATT), a key measure calculated by examining the conditional expectations of individuals who have access to credit.
Let T be a binary variable indicating whether each unit received the treatment or not (credit access), where T = 1 for treated (credit unconstrained) and T = 0 for untreated (credit constrained). the average value of ginger yield for units that received treatment, was calculated using Equation (9):
Where is the number of treated units, is the ginger yield for unit , and is an indicator function that equals 1 if (unit is treated) and 0 otherwise.
The average ginger yield for untreated , that is, the average value of the ginger yield for units that did not receive treatment, was calculated using Equation (10):
Where is the number of untreated units, is the ginger yield for unit , and is an indicator function that equals 1 if (unit is untreated) and 0 otherwise.
The average treatment effect (ATE) was computed by taking the difference between the average yield for the treated group and the average yield for the untreated group, as shown in Equation (11):
The ATE represents the average change in ginger yield due to the treatment. If the treatment had a positive effect, the ATE would be positive, indicating an increase in the ginger yield. Conversely, if the treatment had a negative effect, the ATE would be negative.
4 Results and discussion
4.1 Demographic and socioeconomic characteristics of the sampled households
The demographic and socioeconomic characteristics of the sampled households, such as age, sex, marital status, education level, family size, farm size, total livestock holding, experience, number of extension services, information access, off-farm activity, distance to credit source, and cooperative membership, were included in the analysis as control variables (Table 2). The mean age of the sampled household head was about 47 years. The mean adult equivalent of the sampled household was 4, which is less than the national mean of 4.6 (UN-DESA, 2017). This may be due to migration, which is increasingly perceived as the only way out of poverty in the study area.
The average land devoted to ginger farming was about 1.1 hectares, and the average yield was 127.12 quintals. The mean livestock holding was found to be 4.92. The data showed that approximately 60% of farmers were not members of any cooperative organizations.
4.2 Sources of credit and reason for credit constraints
Of the 343 farmers surveyed, 277 (80.76%) had applied for credit for ginger production in the last 12 months and 66 (19.24%) had not applied for a credit. The main reasons why farmers did not apply for credit (Figure 3) included 79 (23.03%) of farmers saying they did not want to take on debt, 18 (5.25%) of potential loan applicants fearing rejection or a risky hire, and 48 (13.99%) saying they did not need credit because they had sufficient funds. Ultimately, 35 (10.2%) were rejected, 152 (44.31%) felt the potential credit was too expensive (high interest rate), and 11 (3.21%) were of the opinion that they did not have sufficient credit collateral.
As the survey output showed, approximately 58.6% of credit came from informal sources and 41.4% from formal sources. Among the 343 farmers, 66 (19.24%) did not request for credit. From those requested credit, 142 (41.4%) received financing from microfinance institutions and 90 (26.4%) from friends and neighbors. Approximately 26 (7.58%) borrowed from wealthy farmers for their agricultural activities, while 19 (5.54%) received credit from money lenders (Figure 4).
4.3 Determinants of credit constraints
The first stage of the econometric model, utilizing the endogenous switching regression (ESR) approach, focused on estimating the factors influencing credit constraints among households, is shown in Table 3. As expected, 2 of the 13 variables introduced into the model, namely, total livestock ownership and marital status, had a negative association with credit constraints, while the farm size, distance to credit source, and information access had a positive association.
Total livestock ownership (TLU) emerged as a significant negative predictor of credit constraints, indicating that households with higher TLU were less likely to face credit limitations. This finding resonates with the research by Chapoto and Aboagye (2017), who found that livestock ownership serves as a valuable asset that can be leveraged for credit access in rural agricultural contexts. The presence of livestock provides collateral for loans, thereby reducing the risk perceived by lenders and enhancing creditworthiness.
Marital status also demonstrated a negative association with credit constraints, suggesting that households headed by married individuals were less likely to experience credit limitations. This aligns with the findings of a study by Johnson (2021), which highlighted the role of household structure in accessing credit. This may be due to married couples often benefit from shared financial resources and joint decision-making, which may enhance their ability to secure credit.
Conversely, factors such as farm size, distance to credit sources, and informational access were positively correlated with credit constraints. Large farm sizes were found to increase the likelihood of facing credit constraints, consistent with the findings of the research by Brown et al. (2020), suggesting that larger farms may require greater financial resources for investment and operational expenses, leading to heightened credit needs and potential constraints in accessing credit. The estimated coefficient of the distance to credit source variable was positive and statistically significant at the 1% level. This implies that the farther away farmers are from potential lenders, the more likely they are to not apply for credit. The result is consistent with the study by Mukasa et al. (2017), who found a negative association between the distance to potential lenders and the likelihood of obtaining credit.
Similarly, limited access to credit sources and information was found to exacerbate credit constraints among households, aligning with the conclusions drawn by Raghunathan et al. (2023), who highlighted the significance of access to information in facilitating credit access and fostering economic growth.
4.4 Impact of credit constraints on ginger yield and its average treatment effects
The full information maximum likelihood (FIML) estimations of the second stage ESR for ginger yield are presented in Table 4. It presents the determinants of ginger yield for two types of farmers (with and without credit constraints). The likelihood ratio test was statistically significant at the 1% level, showing the interdependence of the three models and indicating that they should not be separately estimated. Furthermore, the Wald test significance value confirmed the joint significance of the error correlation coefficients in both equations, thereby providing further evidence of endogeneity.
In the credit constraint and yield equations, the error correlation coefficients were positive and negative values for credit-constrained and credit-unconstrained farmers, respectively, and were significant at the 1% level only for those with access to the full amount of credit. This implies that those with access to the requested full amount of credit will have above-average yields compared to their counterparts. The insignificance of the covariance estimates for credit-constrained households indicates that when credit is not used for ginger production, there is no difference in ginger yield between credit-constrained and random households caused by unobservable factors.
Regarding family size, the analysis reveals a significant positive relationship with ginger yield for both credit-constrained and unconstrained farmers. Specifically, the coefficients suggest that larger family sizes are associated with higher yields, with statistical significance at the 1% level for credit-constrained farmers and at the 5% level for unconstrained farmers. This implies that family members may help as labor or financially. This finding aligns with recent empirical works of literature, such as the study by Jiang et al. (2022), which revealed that family labor influences agricultural productivity in large farmer households in central China. This underscores the need for tailored policy interventions. However, this finding contrasts with research by Atakli and Agbenyo (2020), who suggest that family size influences agricultural productivity. Larger households may face difficulties accessing equipment, potentially affecting productivity.
Farm size exhibits a negative effect on ginger yield for both credit-constrained and unconstrained farmers, with the impact being more pronounced for the latter group. The coefficients suggest that as farm size increases, ginger yield decreases, with a stronger negative effect observed among unconstrained farmers. This result is consistent with the empirical evidence by Ali et al. (2014) but is dissimilar to Rada and Fuglie (2019) and Sheng and Chancellor (2019). However, the findings in empirical research, such as Atakli and Agbenyo (2020) and Sanchez (2021), underscore the complexity of the relationship between farm size and productivity and suggest that policy interventions should prioritize fostering and improving markets rather than relying solely on farm size as an indicator of productivity.
Household marital status was estimated to have a positive impact on ginger yield among unconstrained farmers. This positive relationship was consistent with the empirical findings of EEA and ESSP of IFPRI (2019), suggesting that promoting marriage and family stability could have positive implications for society’s welfare. Recent studies, such as the research by Doss and Quisumbing (2020), have highlighted the importance of household dynamics in agricultural productivity. Married individuals often benefit from shared labor and resources within the household, leading to more efficient farming practices. This finding underscores the significance of considering familial structures in agricultural policies and interventions to enhance yield. The gender of the household head was estimated to have a negative and significant association with ginger yield. Households headed by a man had, on average, lower yields per hectare than households headed by a woman. Recent research, such as Ali et al. (2014) and Abdisa et al. (2024), have highlighted the disparities between male-headed and female-headed households in agricultural productivity.
The significant positive coefficient for TLU among credit-constrained farmers suggests that livestock ownership positively impacts ginger yield for this group. Recent research, such as a study by Otieno et al. (2021), has emphasized the multifaceted benefits of livestock integration in smallholder farming systems. Livestock provide not only additional income but also valuable organic fertilizer, which enhances soil fertility and crop yields. Similarly, the significant positive coefficient for cooperative membership indicates its beneficial impact on ginger yield among credit-constrained farmers. Research by Abebaw and Haile (2013) and Markelova et al. (2009) support this, emphasizing cooperatives’ role in providing resources, technical assistance, and market opportunities, thereby enhancing productivity. Overall, cooperative participation is vital for improving agricultural productivity, especially for credit-constrained farmers, but effective governance and supportive policies are essential for maximizing their potential.
The estimated average treatment effect (ATT), which describes the impact of credit constraints on ginger yield, is shown in Table 5.
The mean outcome was estimated using ESRM, where the outcome equations are in the form of logarithms. Therefore, the prediction was logarithmic. The results showed that the lack of credit constraints had a positive and significant impact on ginger yield. The average treatment effect (ATT) was positive and varied statistically between 0 and 10% (Table 5). This result implies that farmers without credit constraints have higher yields than farmers with constraints. The ATT value of 0.81 indicates that, on average, ginger farmers without credit constraints achieve higher yields compared to those facing credit constraints.
5 Conclusion and policy implications
This study evaluated and provided further empirical evidence on the determinants of credit constraints and their impact on ginger yields of smallholder farmers in southern and central Ethiopia. The survey findings highlight a substantial reliance on informal sources for agricultural credit, with formal sources contributing a smaller share. Microfinance institutions emerge as the primary formal source, followed by borrowing from friends and neighbors. The findings from this study reveal that credit constraints have a significant impact on ginger yield in Ethiopia. This was influenced mainly by farmland production capacity, family size, and social inclusion. Furthermore, self-sufficiency, high loan rejection by banks, and low credit risk among farmers primarily account for not accessing credit, and the primary determinants of credit constraints are farmer’s proximity to credit, livestock holding, access to credit information, and marital status.
Based on the research findings, agricultural policies should prioritize targeted interventions addressing determinants of credit constraints. Initiatives promoting cooperative membership and improving access to credit sources are crucial for enhancing ginger yield. Moreover, fostering livestock ownership and cooperative participation among credit-constrained farmers can alleviate credit limitations and boost productivity. Policymakers should also focus on reducing distance to credit sources and enhancing access to information to mitigate credit constraints effectively. Policymakers, agricultural extension services, and financial institutions can leverage these insights to formulate effective strategies for supporting smallholder ginger farmers in southern and central Ethiopia.
While this study provides valuable insights into credit constraints and their impact on ginger yields among smallholder farmers in southern and central Ethiopia, it is crucial to recognize certain limitations. The study’s limitations include its narrow focus on the Southern and Central regions of Ethiopia, limiting the broader applicability of findings to diverse geographical and cultural contexts, the reliance on survey data introduces potential biases, and the cross-sectional design limits causal inference between credit constraints and ginger yields. Additionally, the study lacks details on the specific terms of the loans and information on the size of the loans obtained by farmers, which could further inform the nuanced relationship between credit access and agricultural outcomes. Future research endeavors employing longitudinal data and incorporating detailed loan-related parameters can offer a more comprehensive understanding of the evolving relationships.
Despite these limitations, the findings offer a foundational understanding of credit constraints in smallholder ginger farming, informing future policy strategies to support farmers in similar contexts. Employing robust methodologies enriches academic understanding and guides interventions.
Data availability statement
The original contributions presented in the study are included in the article/Supplementary material, further inquiries can be directed to the corresponding author.
Ethics statement
Ethical review and approval was not required for the study on human participants in accordance with the local legislation and institutional requirements. Written informed consent from the (patients/participants OR patients/participants legal guardian/next of kin) was not required to participate in this study in accordance with the national legislation and the institutional requirements.
Author contributions
FT: Conceptualization, Data curation, Formal analysis, Methodology, Writing – original draft. BS: Data curation, Investigation, Supervision, Writing – review & editing. AS: Investigation, Methodology, Supervision, Writing – review & editing. AH: Data curation, Investigation, Methodology, Supervision, Writing – review & editing.
Funding
The author(s) declare that no financial support was received for the research, authorship, and/or publication of this article.
Acknowledgments
The authors extend their gratitude to the study participants for their cooperation and valuable contributions.
Conflict of interest
The authors declare that the research was conducted in the absence of any commercial or financial relationships that could be construed as a potential conflict of interest.
Publisher's note
All claims expressed in this article are solely those of the authors and do not necessarily represent those of their affiliated organizations, or those of the publisher, the editors and the reviewers. Any product that may be evaluated in this article, or claim that may be made by its manufacturer, is not guaranteed or endorsed by the publisher.
Supplementary material
The Supplementary material for this article can be found online at: https://www.frontiersin.org/articles/10.3389/fsufs.2024.1334799/full#supplementary-material
References
Abdisa, T., Mehare, A., and Wakeyo, M. B. (2024). Analyzing gender gap in agricultural productivity: evidence from Ethiopia. J. Agric. Food Res. 15:100960. doi: 10.1016/j.jafr.2023.100960
Abebaw, D., and Haile, M. G. (2013). The impact of cooperatives on agricultural technology adoption: empirical evidence from Ethiopia. Food Policy 38, 82–91. doi: 10.1016/j.foodpol.2012.10.003
Abate, L., and Schaap, M. (2022). Resilient Agriculture for Inclusive and Sustainable Ethiopian Food Systems Baseline Report 2022. Stichting Wageningen Research Ethiopia. doi: 10.18174/632638
Access, O., Achparaki, M., Thessalonikeos, E., Tsoukali, H., Mastrogianni, O., Zaggelidou, E., et al. (2012). We are Intech open, the world’ s leading publisher of open Access books built by scientists, for scientists TOP 1%. Intech 395, 116–124. doi: 10.1016/j.colsurfa.2011.12.014
Ahmadzai, H., Tutundjian, S., and Elouafi, I. (2021). Policies for sustainable agriculture and livelihood in marginal lands: a review. Sustainability 13, 1–18. doi: 10.3390/su13168692
Akanmu, A. O., Akol, A. M., Ndolo, D. O., Kutu, F. R., and Babalola, O. O. (2023). Agroecological techniques: adoption of safe and sustainable agricultural practices among the smallholder farmers in Africa. Front. Sustain. Food Syst. 7:1143061. doi: 10.3389/fsufs.2023.1143061
Ali, E., and Awade, N. E. (2019). Credit constraints and soybean farmers’ welfare in subsistence agriculture in Togo. Heliyon 5, 1–24. doi: 10.1016/j.heliyon.2019.e01550
Ali, D. A., and Deininger, K. (2014). Causes and implications of credit rationing in rural Ethiopia: the importance of zonal variation. J. Afr. Econ. 23, 493–527. doi: 10.1093/jae/eju008
Ali, D. A., Deininger, K., and Duponchel, M. (2014). Credit constraints and agricultural productivity: evidence from rural Rwanda. J. Dev. Stud. 50, 649–665. doi: 10.1080/00220388.2014.887687
Asiamah, T. A., Steel, W. F., and Ackah, C. (2021). Heliyon determinants of credit demand and credit constraints among households in Ghana. Heliyon 7:e08162. doi: 10.1016/j.heliyon.2021.e08162
Atakli, B. A., and Agbenyo, W. (2020). Nexus between financial inclusion, gender and agriculture productivity in Ghana. Theor. Econ. Lett. 10, 545–562. doi: 10.4236/tel.2020.103035
Awunyo-Vitor, D. (2018). Theoretical and conceptual framework of Access to financial services by farmers in emerging economies: implication for empirical analysis. Acta Univ. Sapient. Econ. Bus. 6, 43–59. doi: 10.1515/auseb-2018-0003
Awunyo-vitor, D., Al-hassan, R. M., and Sarpong, D. B. (2013). Agricultural credit rationing in Ghana: What do formal lenders look for? Agric. Fin. Rev. 74, 364–378. doi: 10.1108/AFR-01-2013-0004
Ayodele, T. J., and Sambo, B. E. (2014). Ginger (Zingiber officinale roscoe) production efficiency and constraints among small scale farmers in southern Kaduna, Nigeria. J. Agric. Sci. 6:141. doi: 10.5539/jas.v6n8p141
Balana, B. B., and Oyeyemi, M. A. (2022). Agricultural credit constraints in smallholder farming in developing countries: evidence from Nigeria. World Dev. Sustain. 1:100012. doi: 10.1016/j.wds.2022.100012
Barrett, C. B., Carter, M. R., and Ikegami, M. (2008). poverty traps and social protection. 804, 44. doi: 10.2139/ssrn.1141881,
Barrett, C. B., Carter, M. R., and Timmer, C. P. (2010). Acentury-long perspective on agricultural development. Am. J. Agric. Econ. 92, 447–468. doi: 10.1093/ajae/aaq005
Bashari, M. (2023). Emerging challenges in agriculture and food science vol. Benthem Press International. 8.
Bhatt, S., Lee, J., Deutsch, J., Ayaz, H., Fulton, B., and Suri, R. (2018). From food waste to value-added surplus products (VASP): consumer acceptance of a novel food product category. J. Consum. Behav. 17, 57–63. doi: 10.1002/cb.1689
Brown, W. M., Ferguson, S. M., and Viju-Miljusevic, C. (2020). Farm size, technology adoption and agricultural trade reform: evidence from Canada. J. Agric. Econ. 71, 676–697. doi: 10.1111/1477-9552.12372
Chapoto, T., and Aboagye, A. Q. Q. (2017). African innovations in harnessing farmer assets as collateral. Afr. J. Econ. Manag. Stud. 8, 66–75. doi: 10.1108/AJEMS-03-2017-144
Doss, C. R., and Quisumbing, A. R. (2020). Understanding rural household behavior: beyond Boserup and Becker. Agric. Econ. 51, 47–58. doi: 10.1111/agec.12540
Economics, A., and Library, D. (2017). This document is discoverable and free to researchers across the globe due to the work of AgEcon search. Help Ensure Sustain,
EEA and ESSP of IFPRI. (2019). Proceedings of the Sixteenth International Conference on the Ethiopian Economy. Proceedings of the Sixteenth International Conference on the Ethiopian Economy: Volume I.
FAOSTAT. (2022). Statistical Year Book : World Food and Agriculture, 2022. In World Food and Agriculture – Statistical Yearbook 2022 (Vol. 2022).
Gobena, G. K. (2016). Does poor Access to rural credit constrain smallholder cash crop production participation? Evid. Wolaita Zone 7, 1–7,
Hordofa, T. S., and Tolossa, T. T. (2020). Cultivation and postharvest handling practices affecting yield and quality of major spices crops in Ethiopia: a review. Cogent Food Agric. 6:1788896. doi: 10.1080/23311932.2020.1788896
Jack, K. (2013). Constraints on the adoption of agricultural technologies in developing countries. J. Agric. Technol. Adopt. Initiat. 15, 250–260,
Jayne, T., Fox, L., Fuglie, K., Adelaja, A., Anderson, P., Ash, J., et al. (2021). Agricultural productivity growth, resilience, and economic transformation in sub-Saharan Africa implications for USAID. United States Agency for International Development/Board for International Food and Agricultural Development/Association of Public and Land-Grant Universities, 104. Available at: https://creativecommons.org/licenses/by/4.0. The full report can be accessed electronically at: https://www.usaid.gov/bifad/
Jiang, X., Zhong, S., and Huang, C. (2022). Blessing or curse? The impacts of non-agricultural part-time work of the large farmer households on agricultural labor productivity. Vilnius 28, 26–48. doi: 10.3846/tede.2021.15134
Johnson, S. W. (2021). Determinants of Access to credit: evidence from a fragile conflict-affected setting. Eur. J. Dev. Res. 33, 1475–1492. doi: 10.1057/s41287-020-00295-8
Kang, S. J., and Sawada, Y. (2008). Credit crunch and household welfare, the case of the korean financial crisis. Jpn. Econ. Rev. 59, 438–458. doi: 10.1111/j.1468-5876.2008.00429.x
Khonje, M., Manda, J., Alene, A. D., and Kassie, M. (2015). Analysis of adoption and impacts of improved maize varieties in eastern Zambia. World Dev. 66, 695–706. doi: 10.1016/j.worlddev.2014.09.008
Kifile, A., Bekele, A., Tefera, T., Alemu, D., Koomen, I., and Diro, M. (2023). Importance of ginger in Ethiopia: Recent trends and challenges. Stichting Wageningen Research Ethiopia.
Kim, S. H., and Mokhtarian, P. L. (2023). A note on the sample selection (switching regression) model and treatment effects for a log-transformed outcome variable, in the context of residential self-selection. Transportation, 1–35. doi: 10.1007/s11116-023-10384-2
Markelova, H., Meinzen-dick, R., Hellin, J., and Dohrn, S. (2009). Collective action for smallholder market access. Food Policy 34, 1–7. doi: 10.1016/j.foodpol.2008.10.001
Meskel, T. W., Ketema, M., Haji, J., and Zemedu, L. (2020). Welfare impact of Moringa market participation in southern Ethiopia. Sustain. Agric. Res. 9:98. doi: 10.5539/sar.v9n3p98
Muchunguzi, S. (2023). Participatory management of natural resources in Africa: an imperative policy direction for sustainable development. Manag. Environ. Q. Int. J. 34, 704–720. doi: 10.1108/MEQ-06-2022-0170
Mukasa, A. N., Simpasa, A. M., Salami, A. O., et al. (2017). Credit constraints and farm productivity: Micro-level evidence from smallholder farmers in Ethiopia. Working paper series no. 247. African Development Bank Group. 247, 1–40, Available at: www.afdb.org/
Murtazashvili, I., and Wooldridge, J. M. (2016). A control function approach to estimating switching regression models with endogenous explanatory variables and endogenous switching. J. Econ. 190, 252–266. doi: 10.1016/j.jeconom.2015.06.014
Ogbanje, E. C., and Igboko, B. (2019). Causality between credit to agriculture and ginger production in Nigeria (1991-2015). J. Agric. Vet. Sci. 12, 1–08. doi: 10.9790/2380-1203020108
Otieno, E. O., Kiboi, M. N., Gian, N., Muriuki, A., Musafiri, C. M., and Ngetich, F. K. (2021). Uptake of integrated soil fertility management technologies in heterogeneous smallholder farms in sub-humid tropics. Environ. Chall. 5:100394. doi: 10.1016/j.envc.2021.100394
Prameela, T. P., and Suseela Bhai, R. (2020). Bacterial wilt of ginger (Zingiber officinale Rosc.) incited by Ralstonia pseudosolanacearum - a review based on pathogen diversity, diagnostics and management. J. Plant Pathol. 102, 709–719. doi: 10.1007/s42161-020-00487-5
Qaim, M. (2020). Role of new plant breeding technologies for food security and sustainable agricultural development. Appl. Econ. Perspect. Policy 42, 129–150. doi: 10.1002/aepp.13044
Rada, N. E., and Fuglie, K. O. (2019). New perspectives on farm size and productivity. Food Policy 84, 147–152. doi: 10.1016/j.foodpol.2018.03.015
Rafael, P. (2017). Food security and the 2015–2030 sustainable development goals: From human to planetary health. Curr. Dev. Nutr. 1:e000513. doi: 10.3945/cdn.117.000513
Raghunathan, K., Kumar, N., Gupta, S., Thai, G., Scott, S., Choudhury, A., et al. (2023). Scale and sustainability: the impact of a Women’s self-help group program on household economic well-being in India. J. Dev. Stud. 59, 490–515. doi: 10.1080/00220388.2022.2154151
Sekyi, S., Domanban, P. B., and Honya, G. K. (2020). The impact of informal credit on rural agricultural productivity in the savannah ecological zone of Ghana. Afr. J. Econ. Manag. Stud. 11, 301–315. doi: 10.1108/AJEMS-03-2019-0121
Sheng, Y., and Chancellor, W. (2019). Exploring the relationship between farm size and productivity: evidence from the Australian grains industry. Food Policy 84, 196–204. doi: 10.1016/j.foodpol.2018.03.012
Shiferaw, B., Kassie, M., Jaleta, M., and Yirga, C. (2014). Adoption of improved wheat varieties and impacts on household food security in Ethiopia. Food Policy 44, 272–284. doi: 10.1016/j.foodpol.2013.09.012
Tadele, Z. (2017). Raising crop productivity in Africa through intensification. Agronomy 7, 1–30. doi: 10.3390/agronomy7010022
Teklewold, H., Kassie, M., and Shiferaw, B. (2013). Adoption of multiple sustainable agricultural practices in rural Ethiopia. J. Agric. Econ. 64, 597–623. doi: 10.1111/1477-9552.12011
Tian, X., and Yu, X. (2019). Crop yield gap and yield convergence in African countries. Food Secur. 11, 1305–1319. doi: 10.1007/s12571-019-00972-5
Wooldridge, J. M. (2003). Further results on instrumental variables estimation of average treatment effects in the correlated random coefficient model. Econ. Lett. 79, 185–191. doi: 10.1016/S0165-1765(02)00318-X
UN-DESA. (2017). World Population Ageing 2017. United Nations, 1–124, http://www.un.org/en/development/desa/population/publications/pdf/ageing/WPA2017_Report.pdf
Keywords: credit constraint, yield, ginger producers, Southern Ethiopia, Central Ethiopia
Citation: Tilore FG, Shano BK, Shirko AT and Hawitibo AL (2024) Effect of credit constraint on yield: the case of ginger producers in southern and central Ethiopia. Front. Sustain. Food Syst. 8:1334799. doi: 10.3389/fsufs.2024.1334799
Edited by:
Ruishi Si, Xi'an University of Architecture and Technology, ChinaReviewed by:
Martinson Ankrah Twumasi, Sichuan Agricultural University, ChinaOlabisi Damilola Omodara, Obafemi Awolowo University, Nigeria
Copyright © 2024 Tilore, Shano, Shirko and Hawitibo. This is an open-access article distributed under the terms of the Creative Commons Attribution License (CC BY). The use, distribution or reproduction in other forums is permitted, provided the original author(s) and the copyright owner(s) are credited and that the original publication in this journal is cited, in accordance with accepted academic practice. No use, distribution or reproduction is permitted which does not comply with these terms.
*Correspondence: Fetagn Gizachew Tilore, ZmV0YWdudGlsb3JlQGdtYWlsLmNvbQ==