- 1State Key Laboratory of Efficient Utilization of Arid and Semi-Arid Arable Land in Northern China, Key Laboratory of Arable Land Quality Monitoring and Evaluation, Ministry of Agriculture and Rural Affairs/Institute of Agricultural Resources and Regional Planning, Chinese Academy of Agricultural Sciences, Beijing, China
- 2TERRA Teaching and Research Centre, Gembloux Agro-Bio Tech, University of Liège, Gembloux, Belgium
- 3Heilongjiang Academy of Black Soil Conservation and Utilization, Harbin, China
- 4Institute of Agricultural Resources and Environment, Jilin Academy of Agricultural Sciences, Changchun, China
- 5Key Laboratory of Ecosystem Network Observation and Modeling, Institute of Geographic Sciences and Natural Resources Research, Chinese Academy of Sciences, Beijing, China
- 6Institute of Eco-Environment and Industrial Technology, Shanxi Agricultural University, Taiyuan, China
- 7Net Zero and Resilient Farming, Rothamsted Research, North Wyke, United Kingdom
Although Mollisols are renowned for their fertility and high-productivity, high carbon (C) losses pose a substantial challenge to the sustainable provision of ecosystem services, including food security and climate regulation. Protecting these soils with a specific focus on revitalizing their C sequestration potential emerges as a crucial measure to address various threats associated with climate change. In this study, we employed a modeling approach to assess the impact of different fertilization strategies on crop yield, soil organic carbon (SOC) stock, and C sequestration efficiency (CSE) under various climate change scenarios (baseline, RCP 2.6, RCP 4.5, and RCP 8.5). The process-based SPACSYS model was calibrated and validated using data from two representative Mollisol long-term experiments in Northeast China, including three crops (wheat, maize and soyabean) and four fertilizations (no-fertilizer (CK), mineral nitrogen, phosphorus and potassium (NPK), manure only (M), and chemical fertilizers plus M (NPKM or NM)). SPACSYS effectively simulated crop yields and the dynamics of SOC stock. According to SPACSYS projections, climate change, especially the increased temperature, is anticipated to reduce maize yield by an average of 14.5% in Harbin and 13.3% in Gongzhuling, and soybean yield by an average of 10.6%, across all the treatments and climatic scenarios. Conversely, a slight but not statistically significant average yield increase of 2.5% was predicted for spring wheat. SOC stock showed a decrease of 8.2% for Harbin and 7.6% for Gonghzuling by 2,100 under the RCP scenarios. Future climates also led to a reduction in CSE by an average of 6.0% in Harbin (except NPK) and 13.4% in Gongzhuling. In addition, the higher average crop yields, annual SOC stocks, and annual CSE (10.15–15.16%) were found when manure amendments were performed under all climate scenarios compared with the chemical fertilization. Soil CSE displayed an exponential decrease with the C accumulated input, asymptotically approaching a constant. Importantly, the CSE asymptote associated with manure application was higher than that of other treatments. Our findings emphasize the consequences of climate change on crop yields, SOC stock, and CSE in the Mollisol regions, identifying manure application as a targeted fertilizer practice for effective climate change mitigation.
1 Introduction
Mollisols, characterized by their thick, dark and well-structured surface horizon, stand out as one of the most fertile and productive soils on Earth (Durán et al., 2011). Comprising approximately 7% of the world’s ice-free land surface, these soils account for 28.6% of global farmland across all soil types (Durán et al., 2011; Liu et al., 2012; Xu et al., 2020). Their unique characteristics make Mollisols exceptionally suitable for cultivating cereal crops and establishing pasture and forage systems (Durán et al., 2011; Xu et al., 2020), playing a vital role in agricultural productivity and global food security (Liu et al., 2012). Mollisols are primarily distributed in four regions: the majority of Russia and Ukraine (45%), the central plains of North America (29%), Northeast China (10%), and a substantial portion of South America (10%) (Liu et al., 2012; Xu et al., 2020). Mollisols face challenges due to the conversion of natural grassland to croplands and consecutive planting and tillage practices. These practices have resulted in insufficient carbon (C)inputs, leading to significant soil organic C (SOC) loss in these regions (Sanford et al., 2012). Reports indicate Mollisols in Russia and Ukraine have lost approximately 15–40% of their original organic C pool, while those in America and Northeast China have seen a decline of around 50% over the past several decades (Gollany et al., 2011; Xu et al., 2020). This accelerated land degradation is often accompanied by decreases in crop yield. For example, crop yields in North America have declined by 20–40% over the past century, as a result of unsustainable cultivation practices (Posner et al., 2008). Similarly, Mollisols in Northeast China have experienced a decrease in thickness of approximately 30–50 cm, with each 1 cm reduction corresponding to a 2% reduction in crop yield (Liu H. et al., 2013). In addition, even a slight reduction in C from the substantial SOC pool (approximately 4 Pg) in Mollisols could lead to significant CO2 emissions within these regions (Cheng et al., 2010). For instance, the average C release rate from Mollisols in Northeast China ranged from 0.17 to 2.17 Tg C yr.−1, significantly surpassing the global average of 0.09–0.78 Tg C yr.−1 from the pedological C pool (Lal et al., 2007; Li et al., 2013). Therefore, strategic C sequestration in Mollisols is crucial not only for ensuring food security and promoting soil stability but also for effectively mitigating global climate change.
The potential to sequester C in soils depends not only on the quantity of C input but also on the retention coefficient of the applied C, a concept referred to as C sequestration efficiency (i.e., CSE, △SOC/△C input) (Stewart et al., 2007; Maillard and Angers, 2014). Stewart et al. (2007) established an asymptotically increasing relationship between C inputs and changes in SOC, based on data from 14 long-term experiments conducted over 12 to 96 years of fertilization. This highlighted a decreasing CSE as the soil approached C saturation (Hassink and Whitmore, 1997; Six et al., 2002). However, our previous study (Liang et al., 2023) indicated that CSE remained nearly constant within four decades of fertilization. This was accompanied by a linear relationship between C inputs and SOC changes, as validated by eight extensive long-term experimental datasets, including the Mollisol site at Gongzhuling. Therefore, a narrow range of C input levels, corresponding to shorter fertilization durations, did not necessarily reflect the full spectrum of linear-to-asymptotic behaviors for SOC content (Stewart et al., 2007), and, consequently, the CSE characteristics. Further, examining SOC dynamics that encompasses Mollisols across a broad range of C input levels is essential and urgent to comprehensively elucidate their CSE characteristics.
Optimal fertilizer practices play a critical role in promoting SOC restoration in degraded Mollisols (Xu et al., 2020). Manure application, especially when combined with chemical fertilizers, has demonstrated the potential to enhance CSE due to the beneficial effects of additional organic C and nutrients on soil fertility and soil structure (Liang et al., 2023). However, the sustainability of this benefit under long-term fertilization conditions as soil C approaches saturation requires further investigation. In addition, most Mollisol regions experience cool, moist, and semi-humid climates (Xu et al., 2020), and the warmer and wetter future climates in these regions might significantly impact SOC sequestration by influencing crop production and soil ecological processes (Chu et al., 2017; Lin et al., 2017). Thus, predicting SOC changes and crop yields (associated with C input) in Mollisols is necessary to explore the dynamics of CSE and its response to future climate change under different fertilization strategies.
Process-based models have become essential decision support tools for addressing climate change issues in agriculture management and production, as these models enable the integration of diverse data sources and knowledge concerning the impacts of phenological and environmental variables allowing for valuable evaluations of specific hypotheses and scenarios (Smith et al., 2005). Among the widely used process-based models, the SPACSYS model stands out for its comprehensive consideration of processes in C, nitrogen (N) and phosphorus (P) cycling. Numerous studies have demonstrated its robust capabilities in accurately simulating plant growth, N and P uptake, SOC and soil total nitrogen (TN) stocks, as well as CO2 and N2O emissions across cropland and grassland in diverse regions of Europe and China (Wu et al., 2015; Perego et al., 2016; Li et al., 2017; Liang et al., 2018). Furthermore, validated using data from the Broadbalk continuous wheat experiment over a century of fertilization, the SPACSYS model has successfully captured the dynamics of SOC stock in the plow layer (Liang et al., 2024). These results confirm the SPACSYS model’s reliability in simulating SOC changes and crop yields in response to different long-term fertilization strategies and various climatic conditions. The Mollisol long-term experiments established in the 1980 s in Northeast China offer a valuable resource with well-documented and comprehensive records, detailing fertilization practices, cultivation methods, crop management, climate conditions, grain and straw yields, and soil properties. These detailed records provide a solid foundation for the modeling study and enable accurate predictions of the impacts of fertilizer management and climate change on CSE.
In this study, we aimed to elucidate the responses of crop yield and CSE to climate change in the typical Mollsiol regions of China, while proposing a sustainable strategy to enhance both crop productivity and C sequestration. Specifically, firstly we initially calibrated and validated the SPACSYS model using data from two Mollisol long-term experiments in Northeast China. Subsequently, we quantified the impacts of various future climate scenarios and fertilizer application practices on crop yield, SOC stock, and CSE within the plow layer (0–20 cm) of Mollisols. Finally, we elucidated the comprehensive CSE characteristics when a substantial amount of C has accumulated in the future.
2 Materials and methods
2.1 Study sites background information
2.1.1 Study sites and experimental design
Two typical Mollisols long-term experiments located in Northeast China at Harbin (45°40′ N, 126°35′ E) and Gongzhuling (43°30′ N, 124°48′ E) have been selected for this study. The essential background information, including the locations, initial soil properties, cropping systems and climatic conditions, is given in Table 1. For both experiments, four fertilizer treatments common were chosen, namely (1) no fertilizer (CK), (2) combinations of chemical nitrogen (N), phosphorus (P) and potassium (K) fertilizers (NPK), (3) manure application (M), and (4) chemical fertilizers (N or NPK) plus manure (NM/NPKM) (refer to Supplementary Table S1 for details). Detail information can be found in the works of Jiang et al. (2014, 2018).
2.1.2 Soil sampling and analysis
The soil samples of Gongzhuling in the plow layer (0–20 cm) were collected from 1989 to 2018 annually in approximately 15 days after harvest (n = 28 for each treatment). The soil samples of Harbin in the plow layer (0–20 cm) were collected annually from 1979 to 1988 and almost every 3 years from 1988 to 2018 in approximately 15 days after harvest (n = 23 for each treatment). The SOC content was determined using dichromate oxidation following the modified Walkley & Black method (i.e., heated for 12 min at 220°C) with a correction factor of 1.1 (Walkley and Black, 1934; Xu et al., 2016). While the factor may vary between samples with and without manure applications, a very high heating temperature of 220°C results in a nearly complete oxidation of the organic carbon, and as such, also the more recalcitrant fractions. This strongly limits the potential error introduced to the application of it across all treatments. In this context, we assumed that the error introduced in SOC measurements due to variations in the correction factor among the different treatments could be considered negligible.
2.2 Model parameterization and prediction
2.2.1 Model description
The SPACSYS model is a field-scale, weather-driven with flexible time steps (ranging from minutes to daily), employing a process-based approach that quantifies the biogeochemical processes related to C, N, and P cycling, as well as the water and heat budgets within the soil-plants-and atmosphere continuum. In the current version (6.0) the model incorporates the Farquhar method for photosynthesis (Yin and Struik, 2009) coupled with a modified stomatal conductance sub-model based on Leuning et al. (1995) and Tuzet et al. (2003). This integration enables simulation of the plant photosynthesis rates accounting for the impacts of atmospheric CO2 concentration on photosynthesis and transpiration.
Compared to other widely cited process-based simulation models, SPACSYS stands out due to its detailed representation biogeochemical processes (refer to Supplementary Table S2) and a substantial number of organic matter pools (five). Additionally, SPACSYS includes a detailed root architecture that significantly influences nutrients and water capture, comprehensive descriptions of above- and below-ground plant growth and a nutrient recycling (Wu et al., 2007). More information about SPACSYS can be found in previous articles (Wu et al., 2007, 2015; Bingham and Wu, 2011).
2.2.2 Calibration, validation, and prediction
SPACSYS was operated from the starting year of the experiments (1979 for Harbin and 1989 for Gongzhuling) until 2020 using a daily time-step. Daily weather data (maximum and minimum temperatures, precipitation, wind speed, relative humidity, and solar radiation) were obtained as inputs from the National Meteoritical Information Center.1 Agronomic management details including soil work (plowing depth and date), plant management (seeding and harvesting dates), and fertilizer application (date, amount and type) for each treatment in the Harbin and Gongzhuling long-term experiments, were sourced from records maintained by the Heilongjiang Academy of Black Soil Conservation and Utilization and Jilin Academy of Agricultural Sciences, respectively. Soil properties in the top 20 cm deep at each site (Table 1) were acquired through field surveys. Other missing properties that the model required were estimated from soil texture using pedo transfer functions (PTFs) implemented in the model. The atmospheric CO2 concentration was set from 330 to 380 ppm for Harbin and from 348 to 380 ppm for Gongzhuling, following a linear increase over the experimental period, as per IPCC (2021) guidelines. Grain yields and straw dry matter (if recorded) of maize, wheat and soybean, as well as SOC and total nitrogen (TN) stocks from the NPK and NPKM/NM treatments, were randomly chosen for the model calibration. Data from the other treatments served for model validation. Parameters related to C and N cycling, soil water movement, heat transformation, and plant photosynthesis were adopted from previous studies (Bingham and Wu, 2011; Wu et al., 2015; Liu et al., 2020). The parameters related to the manure decomposition and transformation were from our previous reports (Zhang et al., 2016; Liang et al., 2018). The built-in Multi-Objective Shuffled Complex Evolution Metropolis algorithm (MOSCEM-UA) (Vrugt et al., 2003) was applied to determine the other parameters, primarily those linked to phenology and vegetation characteristics. The optimized parameters and their values for maize, wheat, soybean, and soil C and N cycling are listed in Supplementary Tables S3, S4.
For crop yield and SOC predictions, four climate scenarios were considered, encompassing the baseline and three representative concentration pathways (RCP 2.6, RCP 4.5, and RCP 8.5; Riahi et al., 2011; van Vuuren et al., 2011). The baseline scenario involved historic meteorological data rotated between 1979 and 2020 for Harbin and between 1989 and 2020 for Gongzhuling with a constant CO2 concentration of 380 ppm. Weather data for the RCP scenarios between 2021 and 2,100 were extracted from the HadGEM2-ES model with a spatial resolution of 0.5° × 0.5° (Collins et al., 2011; Jones et al., 2011). The annual average of maximum and minimum temperatures, precipitation, and CO2 concentration under the three RCP scenarios are shown in Supplementary Figure S1 and Supplementary Table S5. The climate projections indicated warmer and wetter future conditions in the studied regions, with average increases of 0.8–3.5°C and 0.4–2.5°C observed in Harbin and Gongzhuling, respectively, when compared to the baseline. The mean annual precipitation (MAP) was projected to rise by 43–121 mm in Harbin and 137–207 mm in Gongzhuling. The RCP 8.5 scenario demonstrated the most significant alteration, nearly doubling CO2 concentrations by 2,100.
Crop varieties and field management practices were fixed as identical to those before 2021 for both sites and scenario. To simplify the assessment of future climate change on crop phenology and yield, the sowing dates were fixed on 30th April for maize, 23rd April for wheat, and 29th April for soybean in Harbin and 26th April in Gongzhuling each year in this study. Harvest dates were determined based on simulated physiological maturity. Simulations were run for each of the four treatments under the various proposed climate scenarios.
2.3 Soil carbon sequestration rate and efficiency
2.3.1 Soil organic carbon sequestration rate
Since SOC predominately undergoes changes in the plow layer (0–20 cm) in Mollisol (Liang et al., 2009), our report concentrates on SOC within this layer. The SOC sequestration rate in the plow layer of the field with fertilization (Cr, t C ha−1 yr.−1) in year t is calculated using the Equation (1) as follows:
where SOCF and SOCCK are the SOC stock in the layer for the NPK, NPKM, NM, or M treatment and the CK treatment in year t, respectively.
2.3.2 Carbon sequestration efficiency
CSE for a fertilization treatment is defined as the percentage of the SOC stock change relative to the net C input between the treatment and CK (Jiang et al., 2018). It is calculated using the Equation (2) as follows:
where CI-F and CI-CK are the annual C input rate for a fertilization treatment (NPK, NPKM, NM, or M) and CK, respectively, which are the cumulative amounts in both manure and crop residue.
2.4 Statistical analysis
We used three statistical criteria to evaluate the model performance: (1) the coefficient of determination (R2) that measures the degree of agreement between simulation and observation; (2) the root mean squared error (RMSE), providing a measure of the average deviation of the estimates from observed values; and (3) the relative error (RE) that reflects the overall difference between simulated and observed data.
Two-way analysis of variance (ANOVA) and Tukey tests (p < 0.05) were used to compare the effects of fertilizer practices and future climate scenarios on grain yield, SOC stock, C input, and CSE. All statistical analyses were conducted using SPSS 24.0 (SPSS, Inc., 2017, Chicago, United States).
3 Results
3.1 Model calibration and validation
The simulated crop yields for different treatments at both sites closely aligned with observations, demonstrating an RMSE below 24% (Supplementary Figures S2–S5; Table 2). Linear regression analysis further revealed a satisfactory correlation between the observed and simulated values (R2 > 0.54) (Figure 1), despite a tendency for the model to overestimate low observed yields and underestimate high observed ones. It was noted that SPACSYS underestimated maize grain and straw yields at Gongzhuling by an average of 16 and 20%, respectively, from 2010 to 2012 (Supplementary Figure S3).
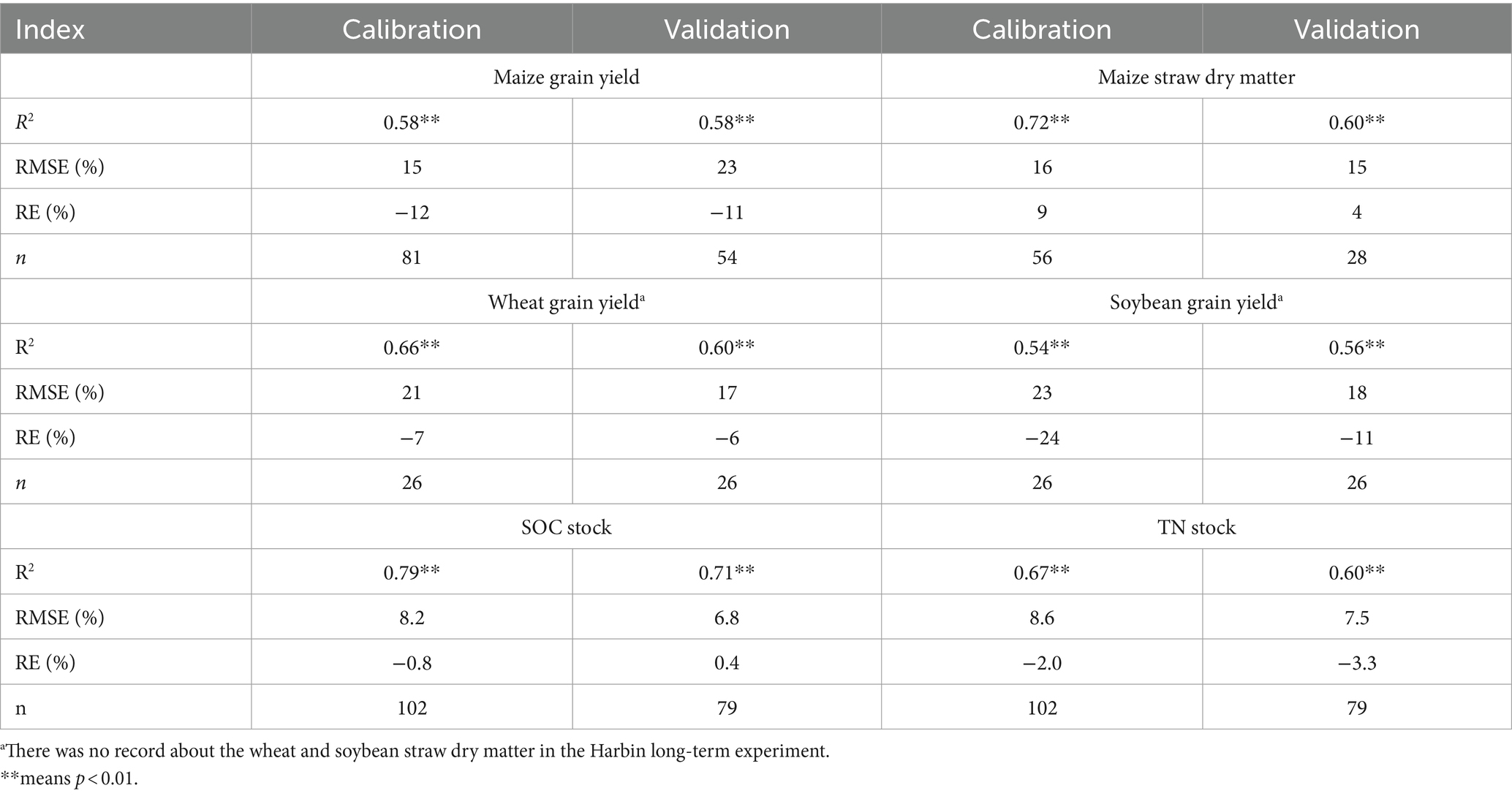
Table 2. Statistical criteria of model performance for crop grain yield, straw dry matter, and SOC and TN stocks.
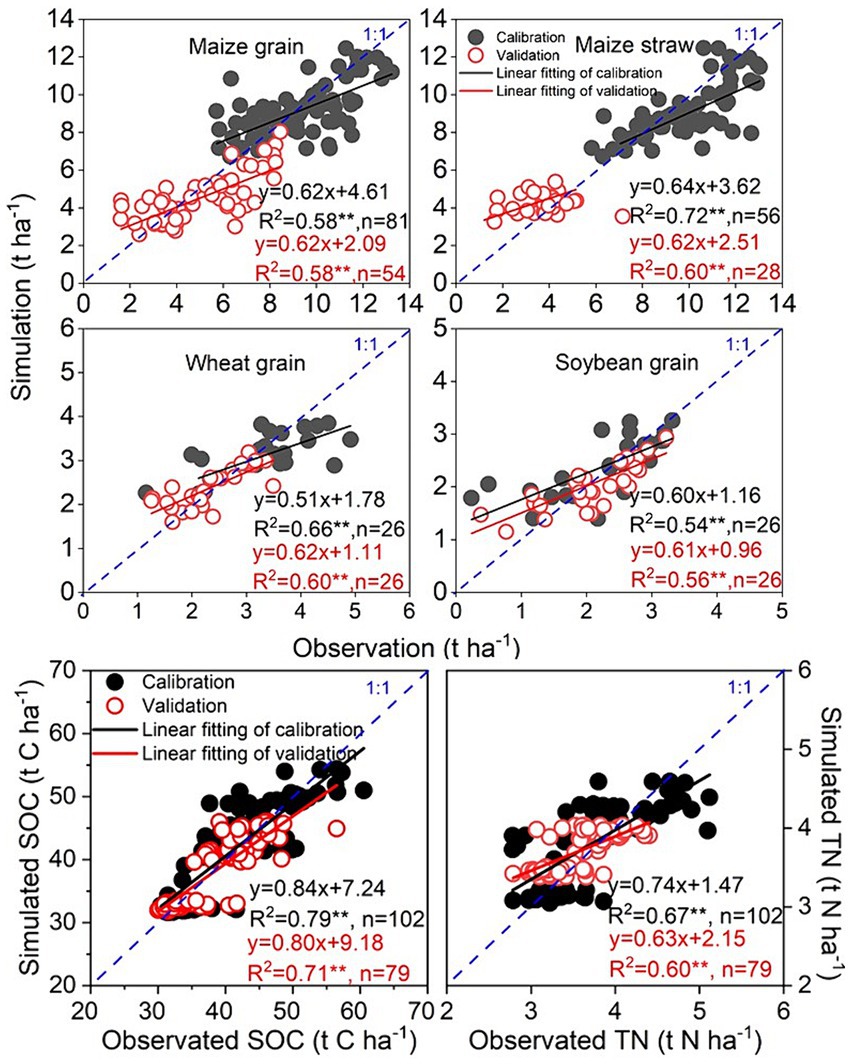
Figure 1. Comparison between simulated and observed grain yields and straw dry matter of maize, wheat and soybean for model calibration and validation. Note: wheat and soybean were only planted in Harbin, and data on straw dry matter for all crops in Harbin are unavailable.
Moreover, SPACSYS effectively simulated SOC and TN stocks, with R2 values ranging from 0.60 to 0.79 and RMSE ranging from 6.8 to 8.2% (Figure 1; Table 2). This favorable agreement with measurements is particularly evident in the first decade of the simulation period (Supplementary Figures S4, S5). In general, the model exhibited a 20% underestimation of SOC stock and a 37% underestimation of TN stock during model validation (Figure 1). However, it overestimated TN stock by an average of 7.3% for CK, 10.2% for NPK, 8.5% for NM, and 9.0% for M after 2000 in Harbin (Supplementary Figure S4).
3.2 Crop yield and SOC stock
Average yields of wheat, maize, and soybean from 2021 to 2,100 under each climate scenario with different fertilizer practices are shown in Figure 2. Notably, a slight but not statistically significant average yield increase of 2.5% was predicted for spring wheat (p < 0.05). Under all climate scenarios, there was also no significant difference (p < 0.05) between the NPK and NPKM/NM fertilizer treatments for maize (1005–12,101 vs. 1,112–12,573 kg ha−1), wheat (2729–5,548 vs. 2,727–5,287 kg ha−1), and soybean (975–4,314 vs. 1,024–4,350 kg ha−1) at both sites. However, the yields for these treatments were significantly higher than those for the M and CK treatments (p < 0.05). Compared with the baseline, the maize yield decreased by an average of 14.5 and 13.3% for Harbin and Gongzhuling, respectively, across all RCP scenarios and under all considered fertilizer practices (Table 3). The decreases in maize yield under the RCP scenarios were ranked as follows: RCP 8.5 (20.6–40.8%) > RCP 4.5 (5.5–25.4%) > RCP 2.6 (0.1–7.8%). The soybean yield decreased by 10.6% on average in Harbin, with the highest decline under RCP 8.5 (p < 0.05) (Table 3).
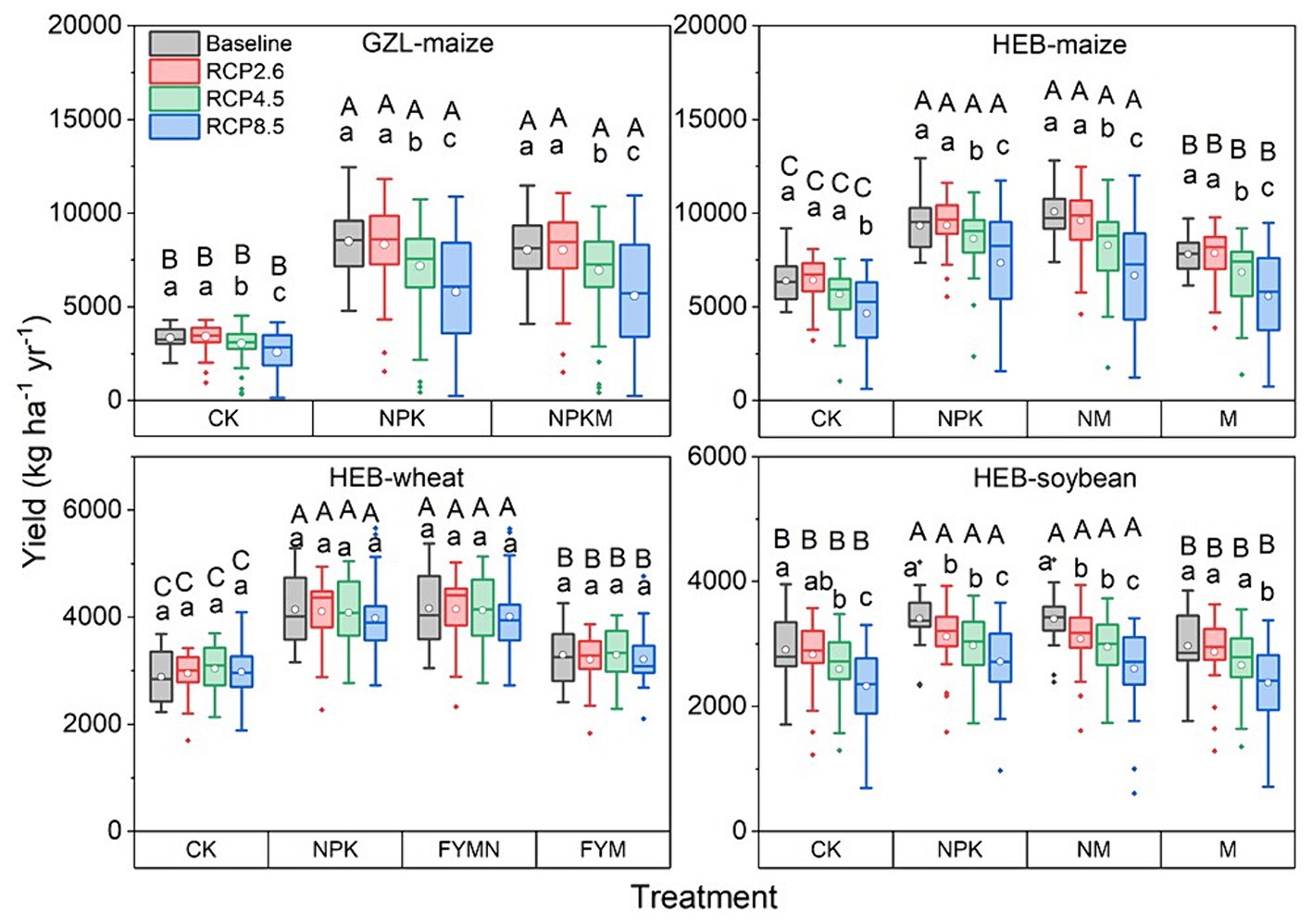
Figure 2. Boxplots of simulated grain yields of maize, wheat and soybean with various fertilizer practices under future climate scenarios between 2021 and 2,100 in Harbin (HEB) and Gongzhuling (GZL). Different lowercase letters over the boxes indicate significant difference (p < 0.05) under different climate scenarios for a specific fertilizer treatment, and different capital letters over the boxes indicate significant difference (p < 0.05) among different fertilizer treatments under a specific climate scenario.
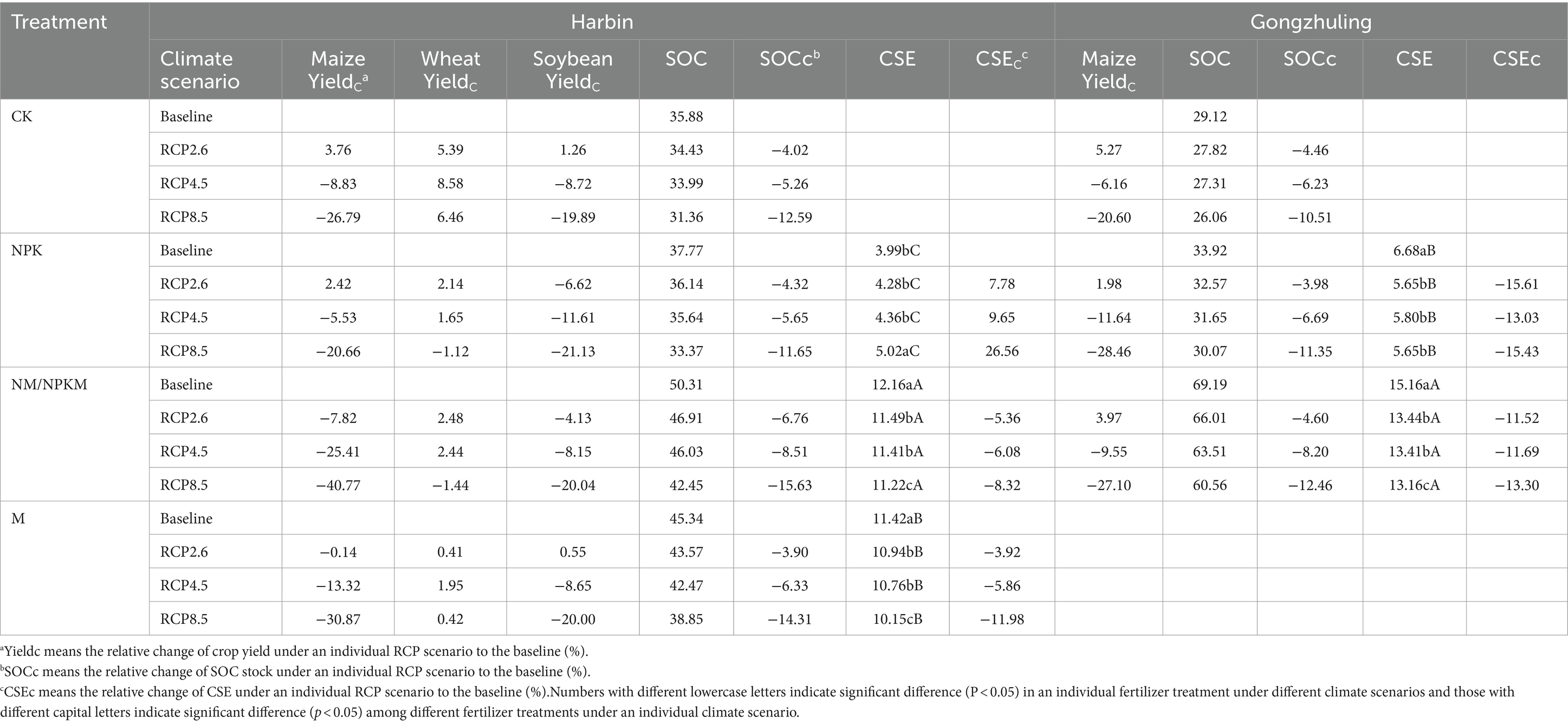
Table 3. Relative change of crop yield (%) and average annual CSE (%) between 2021 and 2,100, annual SOC stock in 2100 (t C ha-1), and their relative changes (%) with each fertilizer treatments under RCP climate scenarios compared with the baseline.
SOC stock decreased by 8.2% in Harbin and 7.6% in Gongzhuling by 2,100 across all RCP scenarios and fertilizer treatments when compared with the baseline (Figure 3; Table 3). The decline under RCP 8.5 (10.5–15.6%) was significantly higher than those under RCP 4.5 (5.3–8.5%) and RCP 2.6 (3.9–6.8%). The ranking of SOC stocks in 2100 was generally as follows: baseline > RCP 2.6 > RCP 4.5 > RCP 8.5. In addition, manure amendment resulted in the highest SOC stock among the treatments, with the values of 38.9–50.3 t C ha−1 in Harbin and 60.6–69.2 t C ha−1 in Gonghzuling, respectively (p < 0.05) (Table 3).
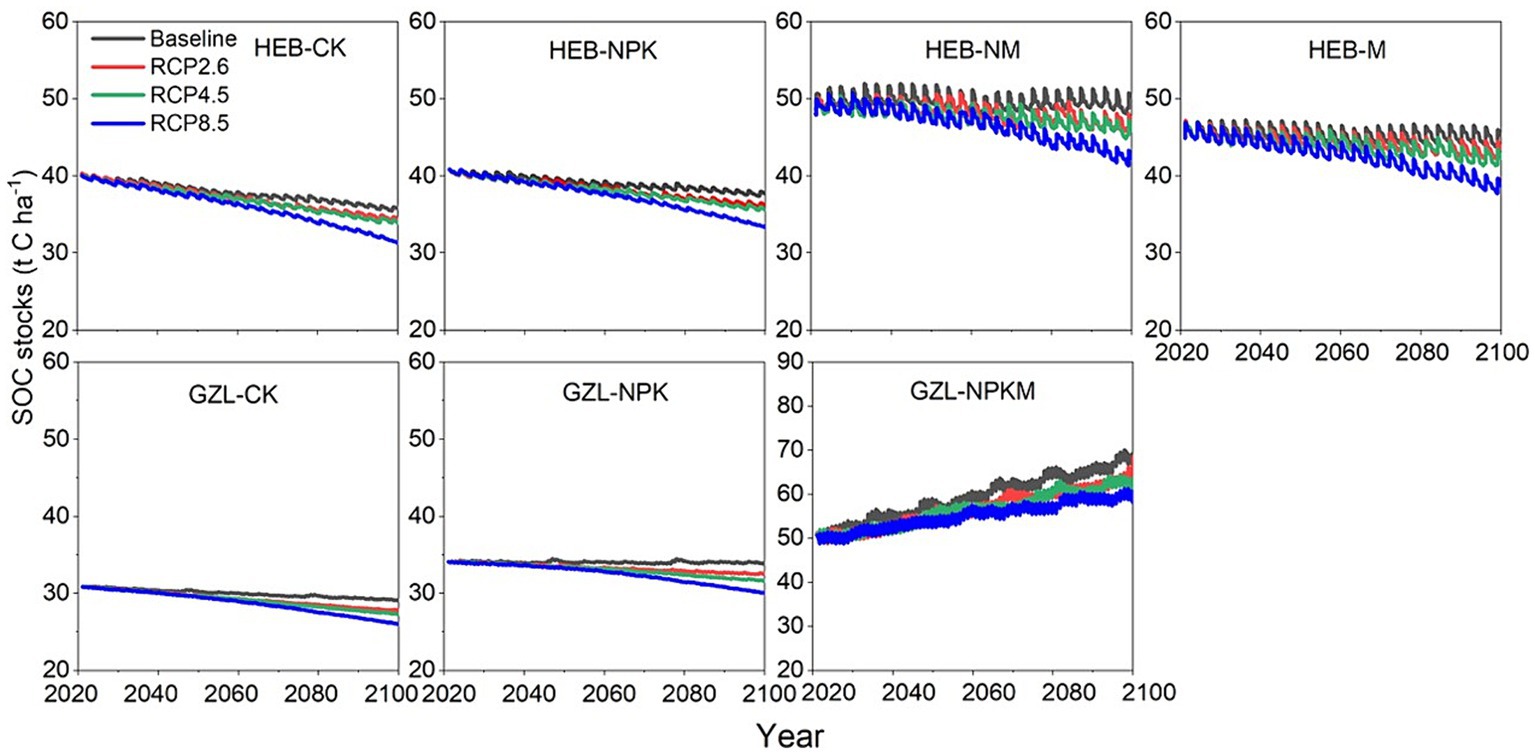
Figure 3. Simulated dynamics of soil organic carbon (SOC) stocks with different fertilization practices under future climate scenarios from 2021 and 2,100 in Harbin (HEB) and Gongzhuling (GZL).
3.3 Annual carbon input and SOC sequestration rate
The average annual C inputs from different sources under different fertilizer practices are presented in Figure 4. When considering the impacts of future climate change on C inputs, compared with the baseline, the C inputs from crops decreased by an average of 2.8% in Harbin and 9.5% in Gongzhuling under all RCP scenarios (Supplementary Table S6). For total C input, no significant difference was observed between the baseline and RCP 2.6 for all treatments, and the lowest C input was observed under RCP 8.5.
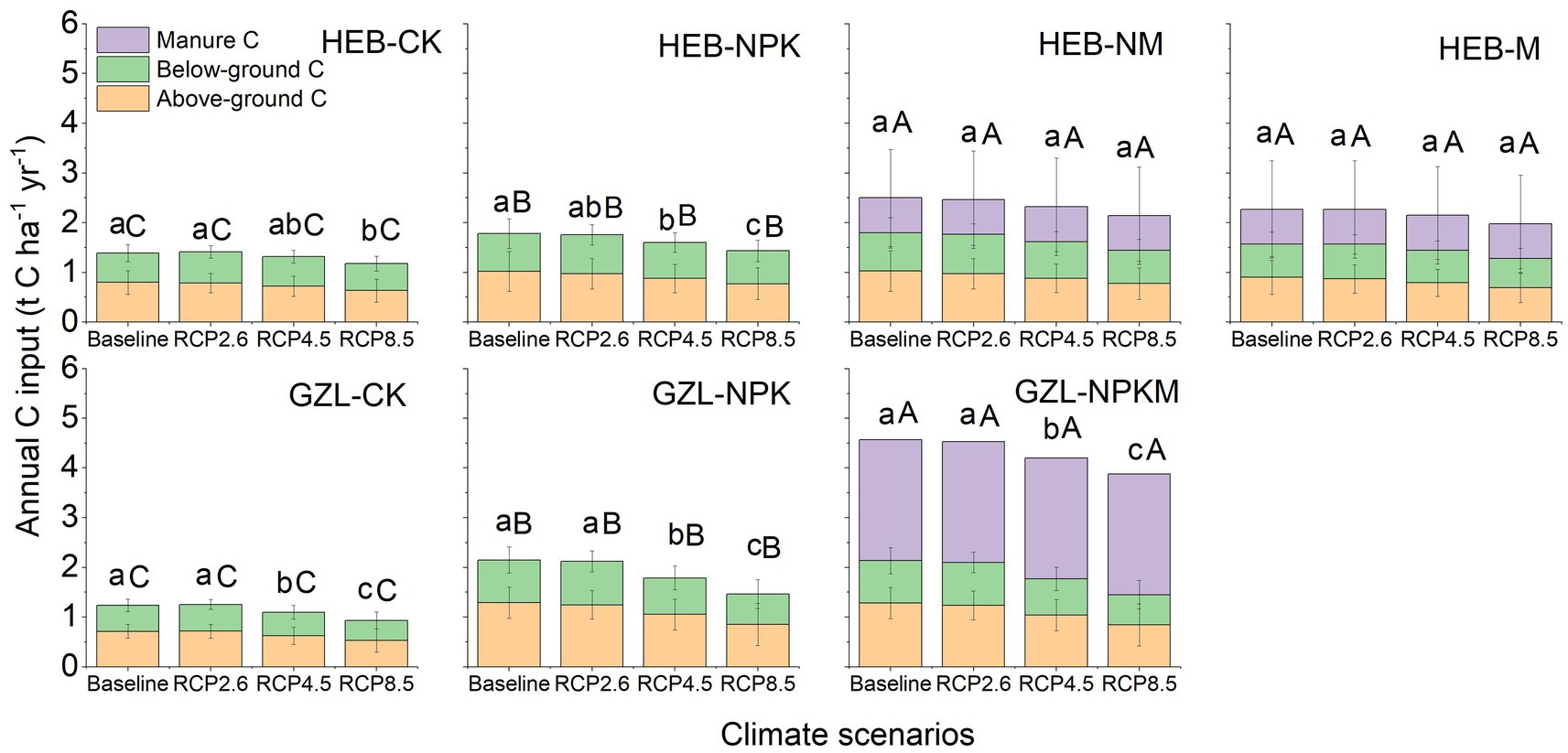
Figure 4. Average annual carbon (C) inputs from different sources with different fertilizer practices in Harbin (HEB) and Gonzghuling (GZL). Different lowercase letters over the columns indicate significant difference (p < 0.05) in total annual C input among different RCP scenarios under a specific treatment at a site, and different capital letters over the columns indicate significant difference (p < 0.05) in total annual C input among different fertilizer treatments under a specific climate scenario at a site.
Soil C sequestration rates for various fertilization treatments across the different periods are shown in Table 4. The rates for manure-amended treatments exceeded those without manure ranging from 62 to 154 kg C ha−1 yr.−1 in Harbin and 311 to 429 kg C ha−1 yr.−1 in Gongzhuling. Furthermore, the rates for NPK and NPKM/NM fertilizer practices in Gongzhuling were higher than those in Harbin. Overall, the rates under the RCP scenarios were lower than those under the baseline, with the lowest rates observed under RCP 8.5.
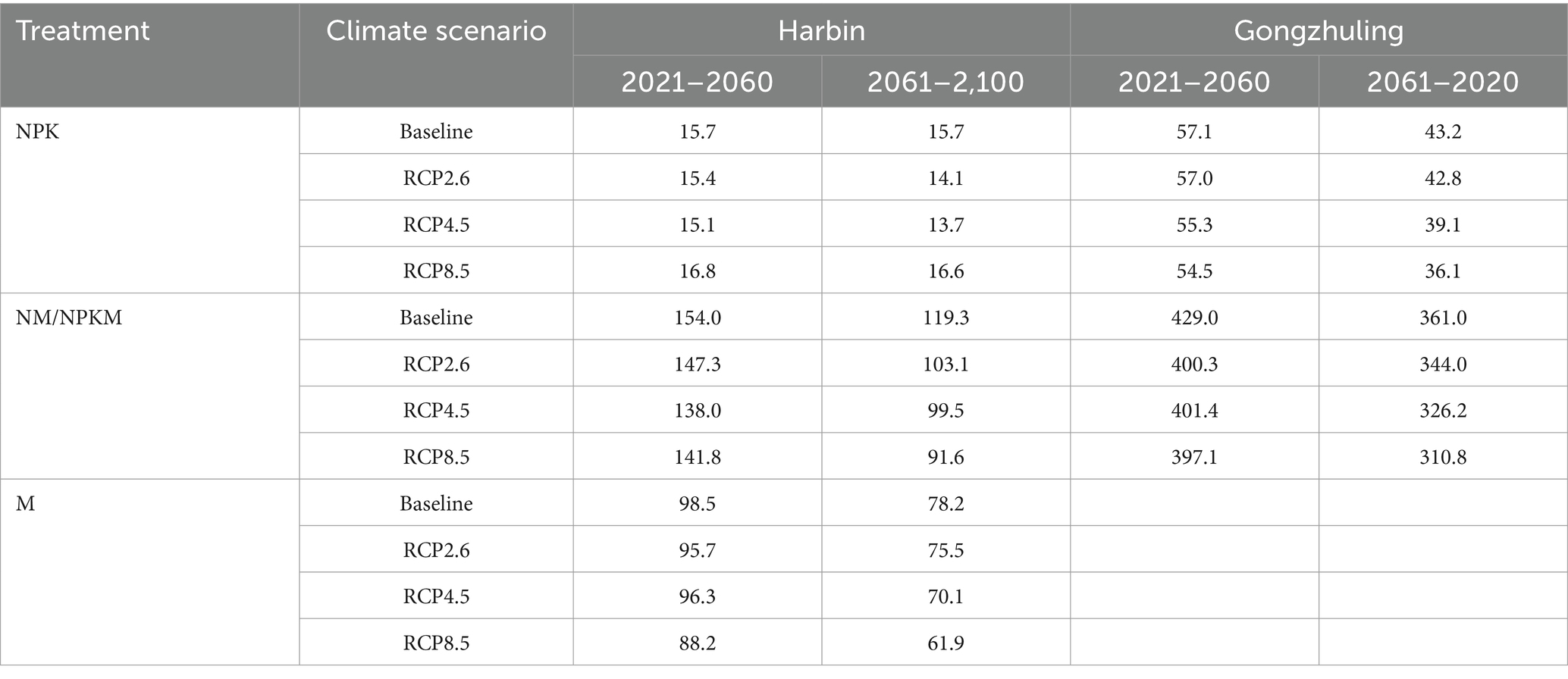
Table 4. Soil organic carbon sequestration rates (kg C ha−1 yr.−1) for various fertilization treatments across the different periods.
3.4 Carbon sequestration efficiency
As outlined in Table 3, the annual CSE averaged over the simulation period showed significantly reduction compared to the baseline across RCP scenarios at both sites (except those for NPK in Harbin). The decreased CSE due to climate change was 6.0% in Harbin (except for NPK) and 13.4% in Gongzhuling. In Harbin, excluding NPK, the CSE values were ranked as follows: baseline > RCP 2.6, RCP 4.5 ≥ RCP 8.5 (p < 0.05). Among the treatments, those with manure amendment (10.15–15.16%) were higher than those for NPK (3.99–6.68%).
The relationship between CSE and cumulative C input (Cinput) was accurately captured by an exponential decreasing function (CSE = b + a × e–k × Cinput, p < 0.001) for all the treatments (Figure 5; Table 5). The CSE asymptotic value, denoted by the parameter ‘b’ in the function, consistently exhibited higher values for M (Harbin: 7.62–9.78%) and NM/NPKM (Harbin: 9.80–11.29% and Gonghzuling: 8.93–10.91%) under all climate scenarios compared to NPK (Harbin: 3.54–4.54% and Gongzhuling: 5.29–6.36%). In addition, the asymptotic CSE values under all RCP scenarios (5.29–10.61%) generally were lower than those under the baseline (6.36–11.29%) for the majority of treatments in both Harbin (except for NPK) and Gongzhuling.
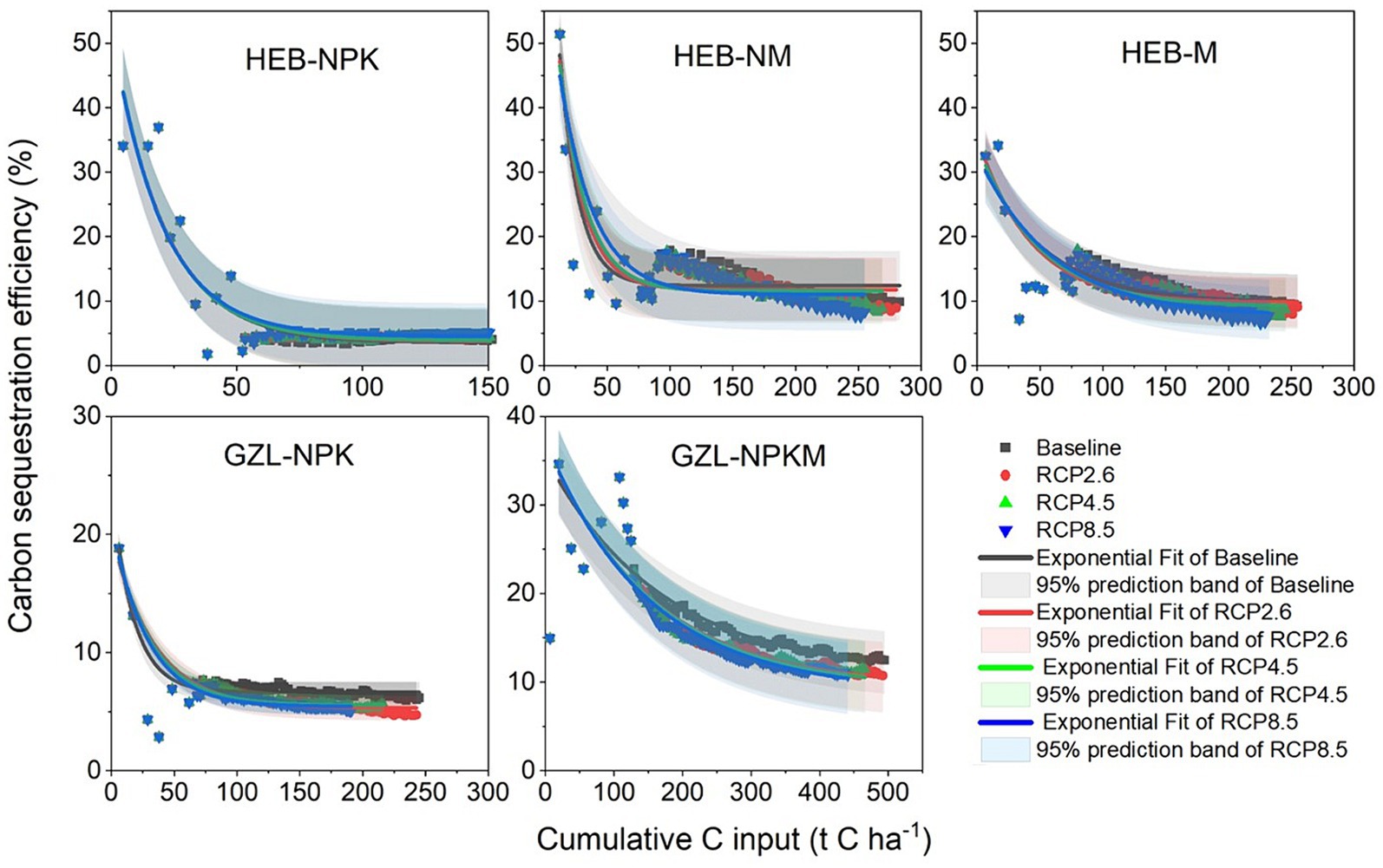
Figure 5. Dynamics of carbon sequestration efficiency (CSE) with carbon input cumulation for all fertilizer practices from the beginning to 2,100 under future climate scenarios in Harbin (HEB) and Gongzhuling (GZL).
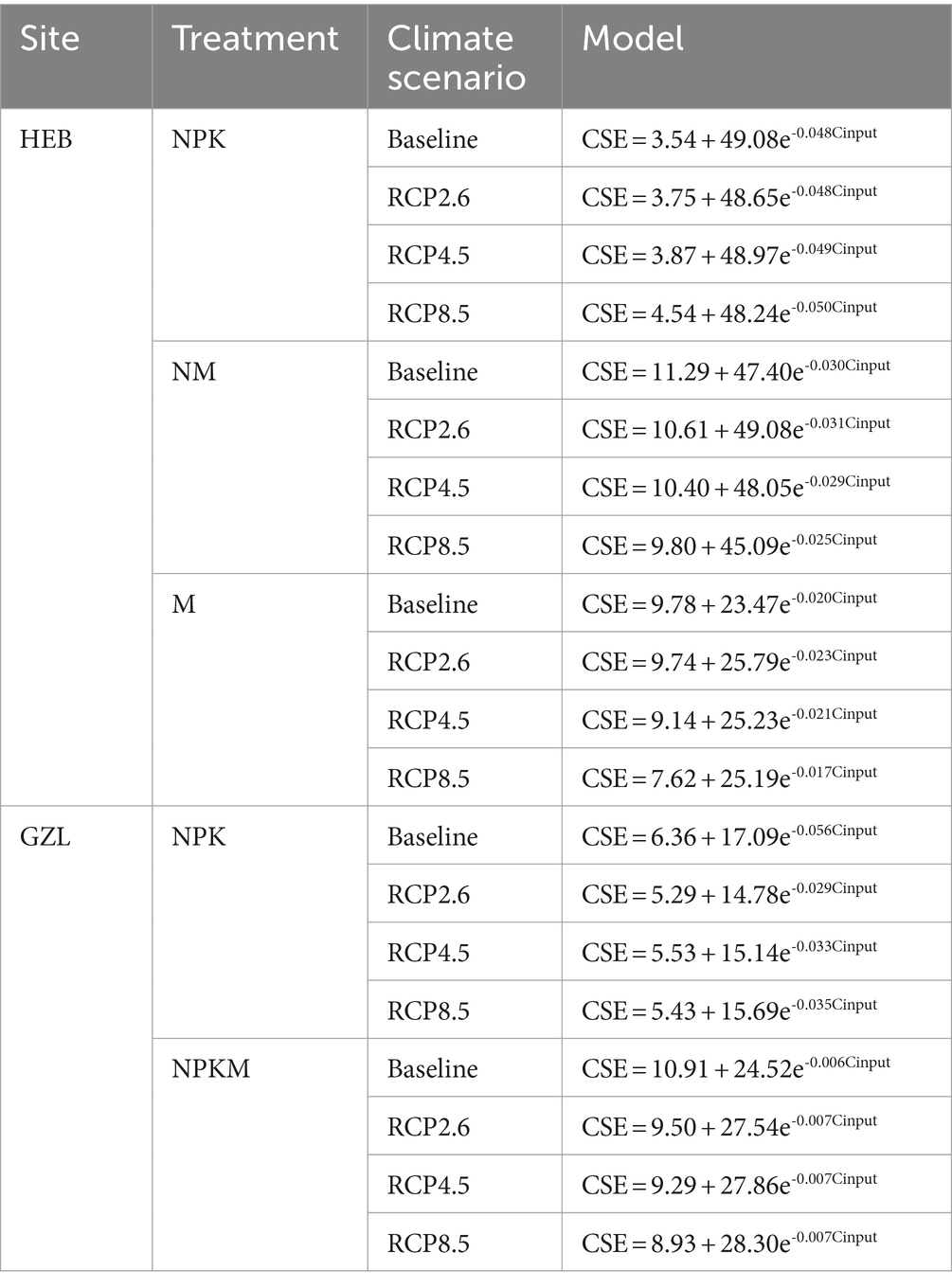
Table 5. Relationship between carbon sequestration efficiency (CSE) and cumulative carbon input to the plow soil layer over the simulation period in Harbin and Gongzhuling.
4 Discussion
4.1 Model performance
Our results highlight that SPACSYS emerges as a competitive model for simulating maize yield in typical Mollisol regions of Northeast China achieving R2 values of 0.58–0.72 (Figure 1; Table 2). This performance compares favorably with the PRYM-Maize model (R2 = 0.57) and the CERES-Maize (R2 = 0.80) model (Jiang et al., 2021; Zhang et al., 2021). Similarly, SPACSYS demonstrated a competitive soybean yield simulation with an RMSE of 18–23%, comparable to the performance of the DSSAT model with an RMSE of 15–22% in Northeast China (Liu S. et al., 2013). It should be noted that SPACSYS has previously employed for simulating winter wheat yield (Liang et al., 2018; Liu et al., 2020), and our study further validates its effectiveness in simulating spring wheat, expanding the application scope of SPACSYS. Furthermore, SPACSYS adequately simulated SOC stocks in the study regions for all treatments (Figure 1; Supplementary Figures S3, S4; Table 2), despite underestimating SOC stocks by 20% during validation. Jiang et al. (2014) applied the Roth-C model to the same sites, our study demonstrated superior performance with R2 values of 0.79 for calibration and 0.71 for validation, while Roth-C achieved R2 values of 0.25 for Harbin and 0.77 for Gongzhuling. In terms of TN stock, SPACSYS also performed well with R2 values of 0.60–0.67 and RMSE of 7.5–8.6% (Table 2), surpassing the GWRK model (R2 = 0.50 and RMSE = 29%) when applied to data from the central Northeast China, including Gongzhuling (Li et al., 2020).
Nevertheless, the model still exhibited certain inevitable errors. For example, it consistently overestimated TN stock for all treatments after 2000 in Harbin. This discrepancy could be partially due to the relocation of the long-term experiment in Chi et al. (2016), despite the soil at a depth of 1.5 m being transferred under frozen soil conditions. Moreover, the model’s excessive reliance on stomatal resistance to soil water could lead to underestimations of maize grain and straw yields (Supplementary Figure S3) during the prolonged droughts from April to June in 2010, 2011 and 2012 in Gongzhuling. In addition, the extreme drought experienced after flowering in 2008, with the precipitation about 80–100 mm lower than those of adjacent years in Harbin, might have contributed to the overestimation of soybean yield by 2.5 times (Supplementary Figure S2). Improvements are needed in the model’s representation of how drought impacts crop growth and development in future iterations.
4.2 Climate and fertilizer impacts on crop yield
In our study, climate change was projected to decrease maize yield in the typical Mollisol regions of Northeast China. The increased temperature might be the primary factor contributing the decline in maize yield, inducing crop early development (i.e., anthesis precocity) and accelerating the reproductive stage compared to the cooler conditions under the baseline (Supplementary Figure S6). While a warming climate might alleviate the effects of chilling damage on maize growth in Harbin and Gongzhuling, our study supported the previous conclusion that the overall impact of future temperatures would be more negative than positive for maize growth (Lin et al., 2017). Furthermore, several studies have suggested that the maize yield increase resulting from the “CO2 fertilization effect” could not offset the reduction caused by the increased temperature in study regions of Northeast China (Wang et al., 2011; Lin et al., 2017; Jiang et al., 2021). Consequently, future climate was expected to significantly decrease maize yield in the Mollisol regions of Northeastern China, especially at the end of the simulation period (2090–2,100) under RCP 8.5 (57.6–69.2% for Harbin and 54.8–67.1% for Gongzhuling), coinciding with the largest increments in both CO2 concentration and temperature (Supplementary Table S7).
The decrease in soybean yield in Harbin was less pronounced (10.6%) compared to maize (14.5%). According to Jin et al. (2017), the impact of enhanced CO2 concentration on offsetting yield gaps caused by changes in temperature and precipitation is more substantial for soybean than for maize. On the other hand, earlier studies have indicated that soybean yield in Northeast China could potentially increase with rising precipitation and temperature, considering the effects of “CO2 fertilization” (Yin et al., 2015; Lin et al., 2017). However, our findings corroborate previous studies (Jin and Zhu, 2008; Guo et al., 2022) when temperatures reach high levels (e.g., a 4°C increase) as projected at our study sites. The maximum temperature enhancement under RCP 4.5 was generally above 4°C after 2080 and ranged from 4.0 to 8.5°C after 2060 under RCP 8.5 (Supplementary Figure S1). In such conditions, soybean yield would decline without cultivar improvement in response to temperature extremes, leading to a reduction in the number of grains by shortening the grain-filling period. Climate change did not significantly affect spring wheat yield in the study regions, being consistent with the earlier reports (Jin and Zhu, 2008; Yin et al., 2015). The anticipated warmer climate is expected to result in a shortened growing period, leading to a diminished accumulation of photosynthate (Supplementary Figure S6). For major C3 crops, the current atmosphere CO2 concentration constrains crop photosynthesis. Elevated CO2 concentration can increase crop net photosynthesis by increasing the availability of intercellular CO2 as a substrate and restraining competition with photorespiration for the Rubisco enzyme (Dermody et al., 2008).
4.3 Response of the carbon sequestration to climate change
Our simulations revealed a significant decrease in SOC stock under all future climate scenarios, consistent with previous studies conducted in Mollisol regions (Gao et al., 2008; Ramírez et al., 2019; Bao et al., 2023). The decline in C input for each fertilizer practice, due to the reduction of maize and soybean productions under the RCP scenarios, likely played a predominant role in the SOC decline. Consequently, SOC stock under RCP 8.5 was significantly lower than those under RCP 4.5 and RCP 2.6 (p < 0.05). Furthermore, the combined application of manure with chemical N treatments simultaneously showed the highest SOC stock and crop yield at both sites under each climate scenario (Figures 2, 3; Table 3). It has been reported that manure combined with chemical fertilizer treatment has the beneficial effects of both C inputs and readily available nutrients for plant growth, enhancing C inputs from crop residues and sequestrating a greater amount of C compared to other treatments (Triberti et al., 2016; Jiang et al., 2018; Gross and Glaser, 2021). Notably, the impact of fertilization on SOC stock was more pronounced with the manure-only treatment compared to NPK. The significantly higher annual C input (Figure 4) could be the primary reason for the observed difference, along with the very close amount of C inputs from crop residues for both treatments (Supplementary Table S6). However, a contrary conclusion was found when C inputs from manure into croplands were transferred from other ecosystems within the same region, rather than relying on consistent C inputs from fertilization (Schmidt et al., 2011).
Our results showed that fields with manure application had greater efficiencies in C sequestration than those receiving chemical fertilizer alone. Applied manure creates a favorable growth environment for plants and microorganisms (Mandal et al., 2007). Our simulations demonstrated that manure amendments led to increased C inputs and microbial biomass. Those might be beneficial for retaining massive amounts of total C in the Mollisol regions of Northeast China (Yu et al., 2006; Ding et al., 2016; Wang et al., 2020). Consistently, compared to the practice using chemical fertilizer alone, manure application exhibited significantly higher SOC stocks and change rates (Figure 3; Table 4), supporting a higher value of CSE.
Our investigation revealed a pronounced negative exponential correlation between CSE and the cumulative C input. Specifically, CSE showed an initial rapid decline following the onset of C input, then a decelerated decrease, ultimately settling into a slow decay until reaching a stable asymptotic value with a substantial accumulation of C input. This dynamics pattern has been directly or indirectly supported by previous findings (Stewart et al., 2007, 2008; Yan et al., 2013; Maillard and Angers, 2014; Jiang et al., 2018). Stewart et al. (2007), in their synthesis of data from 14 long-term experiments in the USA and Canada, identified an asymptotic increase in the relationship between C inputs and SOC content, ascribable to the soil C saturation phenomena at high C inputs. Furthermore, Stewart et al. (2008) proposed that the stabilization efficiency of added C would decrease with increasing C input levels. These studies collectively supported our observation of a negative exponential relationship between CSE and C inputs. Warmer and wetter environmental conditions are likely to enhance the mineralization rate of added C (Fang et al., 2022; Bao et al., 2023), leading to a reduction in CSE. Our predictions indicated that the soil respiration rate under the RCP scenarios which soil temperature is a primary control factor increased 3.10–9.36% in Gongzhuling and 1.67–16.18% in Harbin compared to the baseline (Supplementary Table S8). Similar findings have been reported (Wang et al., 2022).
Notably, the average annual CSE for NPK under future climate scenarios in Harbin increased, contrasting with the absence of such a trend in Gongzhuling (Table 3). The incorporation of soybean into the crop rotation in Harbin likely contributed to the divergent responses observed in CSE. Biologically fixed N by soyabean can be available for subsequent crops, which can increase biomass accumulation in non-leguminous crops within the rotation (Stagnari et al., 2017). This results in an increase of C input to the soils. Additionally, root exudation and senescence of legume roots and nodules further contribute to the C input to the soils, offering benefits for SOC sequestration (Virk et al., 2022). The findings suggested that integrating leguminous crop in a rotation cropping system could contribute to improved C retention in soils, especially in situations where manure is not readily available.
4.4 Adaptation strategies for climate change
In our study, the average CSE for NPKM/NM/M treatments ranged from 9.54 to 15.16%, aligning closely with the reported global manure C conversion ratio of 12 ± 4%, derived from a meta-analysis of 130 observations spanning 4–82 years of manure applications (Maillard and Angers, 2014). Furthermore, predictions regarding the CSE of manure application in the soils of 20 long-term experiments in China, including the two Mollisols examined in our research, indicated that CSE approaching the stable stage ranged from 8.8 to 10.4% under baseline and RCP 4.5 conditions (Jiang et al., 2018). Our findings, ranging from 9.1 to 11.3%, closely resembled these results (Table 5). This suggested that our results can serve as a useful reference for investigating the characteristics of CSE under long-term fertilization conditions in Chinese Mollisols, especially with a substantial amount of C input. Our predictions indicated a worse situation for both food security and soil fertility for Mollisols in the future, in comparison to the current condition. In addition to spring wheat, the production of maize and soybean, which are the primary crops in the world’s Mollisol regions (Durán et al., 2011; Xu et al., 2020), would decline under future climate change. In addition, the goal of sequestrate more C in Mollisols to mitigate global warming cannot be achieved through the sole application of chemical fertilizers, as both the SOC sequestration rate and CSE decreased under all climatic scenarios. To address this, our predictions suggest the following interventions: (1) the development of new cultivars that better adapt to the anticipated warmer and wetter climates; (2) an earlier planting of crops to prevent the negative effects of heat and drought during the growing season; and (3) the optimization of nutrient application rates and the ratio of chemical fertilizers to manure.
5 Conclusion
Our study successfully validated the SPACSYS model for simulating yields of maize, spring wheat and soybean, and the SOC stock in typical Mollisol regions in China. SPACSYS predicted a decline in both yields and SOC stock under future climate scenarios compared with the baseline. The anticipated reduction in maize and soybean yields was primarily attributed to increased temperatures with maize being more significantly affected than soybean. Interestingly, the warmer and wetter future had no significant effects on spring wheat yield in this region. Future climate conditions led to a decrease in SOC stocks and CSE at the two typical Mollisol sites for fertilization treatments. Our prediction confirmed that CSE initially decreased rapidly following C inputs, exhibited a decelerated decline, and eventually decayed slowly until reaching a stable asymptotic value due to substantial C accumulation. Notably, the combined application of manure and chemical fertilizers demonstrated the highest potential to mitigate the negative impacts of climate change on crop yields and CSE. Our findings suggest that future research should prioritize improving crop cultivars, optimizing planting timings, and establishing effective fertilizer management strategies to mitigate climate change risks in Mollisol regions.
Data availability statement
The original contributions presented in the study are included in the article/Supplementary material, further inquiries can be directed to the corresponding authors.
Author contributions
SL: Conceptualization, Data curation, Formal analysis, Methodology, Software, Writing - original draft, Writing - review & editing. NS: Conceptualization, Funding acquisition, Supervision, Writing – review & editing. BL: Methodology, Supervision, Writing – review & editing. JM: Methodology, Supervision, Writing – review & editing. XM: Data curation and Writing – review & editing. HG: Data curation and Writing – review & editing. XZ: Methodology and Writing – review & editing. LQ: Funding acquisition and Writing – review & editing. GC: Methodology, Supervision, Writing – review & editing. MX: Conceptualization, Funding acquisition, Supervision, Writing – review & editing. LW: Conceptualization, Methodology, Writing – review & editing.
Funding
The author(s) declare that financial support was received for the research, authorship, and/or publication of this article. This study was supported by the National Key Research and Development Program of China (2021YFD1500205) and the National Natural Science Foundation of China (42177341). SL was supported by the China Scholarship Council (No. 202003250099).
Acknowledgments
We acknowledge the Chinese Academy of Agricultural Sciences - Gembloux Agro-Bio Tech joint PhD program and all the colleagues from the long-term fertilization experimental sites for their unremitting assistance.
Conflict of interest
The authors declare that the research was conducted in the absence of any commercial or financial relationships that could be construed as a potential conflict of interest.
The author(s) declared that they were an editorial board member of Frontiers, at the time of submission. This had no impact on the peer review process and the final decision.
Publisher’s note
All claims expressed in this article are solely those of the authors and do not necessarily represent those of their affiliated organizations, or those of the publisher, the editors and the reviewers. Any product that may be evaluated in this article, or claim that may be made by its manufacturer, is not guaranteed or endorsed by the publisher.
Supplementary material
The Supplementary material for this article can be found online at: https://www.frontiersin.org/articles/10.3389/fsufs.2024.1332483/full#supplementary-material
Footnotes
References
Bao, Y., Yao, F., Meng, X., Fan, J., Zhang, J., Liu, H., et al. (2023). Dynamic modeling of topsoil organic carbon and its scenarios forecast in global Mollisols regions. J. Clean. Prod. 421:138544. doi: 10.1016/j.jclepro.2023.138544
Bingham, I. J., and Wu, L. (2011). Simulation of wheat growth using the 3D root architecture model SPACSYS: validation and sensitivity analysis. Eur. J. Agron. 34, 181–189. doi: 10.1016/j.eja.2011.01.003
Cheng, S., Fang, H., Zhu, T., Zheng, J., Yang, X., Zhang, X., et al. (2010). Effects of soil erosion and deposition on soil organic carbon dynamics at a sloping field in Black soil region, Northeast China. Soil Sci. Plant Nutr. 56, 521–529. doi: 10.1111/j.1747-0765.2010.00492.x
Chu, Z., Guo, J., and Zhao, J. (2017). Impacts of future climate change on agroclimatic resources in Northeast China. J. Geogr. Sci. 27, 1044–1058. doi: 10.1007/s11442-017-1420-6
Chi, F., Liu, J., Kuang, E., Zhang, J., and Su, Q. (2016). Effects of the relocation of undisturbed black soil after long-term test on soil fungal community diversity. China Soil Fert. 6, 141–150. (in Chinese). doi: 10.11838/sfsc.20160607
Collins, W. J., Bellouin, N., Gedney, N., and Halloran, P. (2011). Development and evaluation of an earth-system model – HadGEM2. Geosci. Model Dev. Discuss. 4:997. doi: 10.5194/gmdd-4-997-2011
Dermody, O., Long, S. P., McConnaughay, K., and DeLucia, E. H. (2008). How do elevated CO2 and O3 affect the interception and utilization of radiation by a soybean canopy? Glob. Chang. Biol. 14, 556–564. doi: 10.1111/j.1365-2486.2007.01502.x
Ding, J., Jiang, X., Ma, M., Zhou, B., Guan, D., Zhao, B., et al. (2016). Effect of 35 years inorganic fertilizer and manure amendment on structure of bacterial and archaeal communities in black soil of Northeast China. Appl. Soil Ecol. 105, 187–195. doi: 10.1016/j.apsoil.2016.04.010
Durán, A., Morrás, H., Studdert, G., and Liu, X. (2011). Distribution, properties, land use and management of Mollisols in South America. Chinese Geogr. Sci. 21, 511–530. doi: 10.1007/s11769-011-0491-z
Fang, R., Li, Y., Yu, Z., Xie, Z., Wang, G., Liu, X., et al. (2022). Warming offsets the beneficial effect of elevated CO2 on maize plant-carbon accumulation in particulate organic carbon pools in a Mollisol. Catena 213:106219. doi: 10.1016/j.catena.2022.106219
Gao, C. S., Wang, J. G., Zhang, X. Y., and Sui, Y. Y. (2008). The evolution of organic carbon in Chinese Mollisol under different farming systems: validation and prediction by using century model. Agric. Sci. China 7, 1490–1496. doi: 10.1016/S1671-2927(08)60407-1
Gollany, H. T., Rickman, R. W., Liang, Y., Albrecht, S. L., Machado, S., and Kang, S. (2011). Predicting agricultural management influence on long-term soil organic carbon dynamics: implications for biofuel production. Agron. J. 103, 234–246. doi: 10.2134/agronj2010.0203s
Gross, A., and Glaser, B. (2021). Meta-analysis on how manure application changes soil organic carbon storage. Sci. Rep. 11, 5516–5513. doi: 10.1038/s41598-021-82739-7
Guo, S., Guo, E., Zhang, Z., Dong, M., Wang, X., Fu, Z., et al. (2022). Impacts of mean climate and extreme climate indices on soybean yield and yield components in Northeast China. Sci. Total Environ. 838:156284. doi: 10.1016/j.scitotenv.2022.156284
Hassink, J., and Whitmore, A. P. (1997). A model of the physical protection of organic matter in soils. Soil Sci. Soc. Am. J. 61, 131–139. doi: 10.2136/sssaj1997.03615995006100010020x
IPCC (2021). “Climate change 2021: the physical science basis” in Contribution of working group I to the sixth assessment report of the intergovernmental panel on climate change. eds. V. Masson-Delmotte, P. Zhai, A. Pirani, S. L. Connors, C. Péan, and S. Berger, et al. Cambridge: Cambridge University Press.
Jiang, R., He, W., He, L., Yang, J. Y., Qian, B., Zhou, W., et al. (2021). Modelling adaptation strategies to reduce adverse impacts of climate change on maize cropping system in Northeast China. Sci. Rep. 11, 810–813. doi: 10.1038/s41598-020-79988-3
Jiang, G., Xu, M., He, X., Zhang, W., Huang, S., Yang, X., et al. (2014). Soil organic carbon sequestration in upland soils of northern China under variable fertilizer management and climate change scenarios. Global Biogeochem. Cycles 28, 319–333. doi: 10.1002/2013GB004746.Received
Jiang, G., Zhang, W., Xu, M., Kuzyakov, Y., Zhang, X., Wang, J., et al. (2018). Manure and mineral fertilizer effects on crop yield and soil carbon sequestration: a Meta-analysis and modeling across China. Global Biogeochem. Cycles 32, 1659–1672. doi: 10.1029/2018GB005960
Jin, Z. Q., and Zhu, D. W. (2008). Impacts of changes in climate and its variability on food production in Northeast China. Acta Agron. Sin. 34, 1588–1597. doi: 10.1016/S1875-2780(09)60005-5
Jin, Z., Zhuang, Q., Wang, J., Archontoulis, S. V., Zobel, Z., and Kotamarthi, V. R. (2017). The combined and separate impacts of climate extremes on the current and future US rainfed maize and soybean production under elevated CO2. Glob. Chang. Biol. 23, 2687–2704. doi: 10.1111/gcb.13617
Jones, C. D., Hughes, J. K., Bellouin, N., Hardiman, S. C., Jones, G. S., Knight, J., et al. (2011). The HadGEM2-ES implementation of CMIP5 centennial simulations. Geosci. Model Dev. Discuss. 4, 689–763. doi: 10.5194/gmdd-4-689-2011
Lal, R., Follett, R. F., Stewart, B. A., and Kimble, J. M. (2007). Soil carbon sequestration to mitigate climate change and advance food security. Soil Sci. 172, 943–956. doi: 10.1097/ss.0b013e31815cc498
Leuning, R., Kelliher, F. M., De Pury, D. G. G., and Schulze, E.-D. (1995). Leaf nitrogen, photosynthesis, conductance and transpiration: scaling from leaves to canopies. Plant Cell Environ. 18, 1183–1200. doi: 10.1111/j.1365-3040.1995.tb00628.x
Li, Y., Liang, S., Zhao, Y., Li, W., and Wang, Y. (2017). Machine learning for the prediction of L. chinensis carbon, nitrogen and phosphorus contents and understanding of mechanisms underlying grassland degradation. J. Environ. Manag. 192, 116–123. doi: 10.1016/j.jenvman.2017.01.047
Li, H., Peil, J. B., Wang, J. K., Li, S. Y., and Gao, G. W. (2013). Organic carbon density and storage of the major black soil regions in Northeast China. J. Soil Sci. Plant Nutr. 13, 883–893. doi: 10.4067/S0718-95162013005000070
Li, X., Shang, B., Wang, D., Wang, Z., Wen, X., and Kang, Y. (2020). Mapping soil organic carbon and total nitrogen in croplands of the Corn Belt of Northeast China based on geographically weighted regression kriging model. Comput. Geosci. 135:104392. doi: 10.1016/j.cageo.2019.104392
Liang, S., Li, Y., Zhang, X., Sun, Z., Sun, N., Duan, Y., et al. (2018). Response of crop yield and nitrogen use efficiency for wheat-maize cropping system to future climate change in northern China. Agric. For. Meteorol. 262, 310–321. doi: 10.1016/j.agrformet.2018.07.019
Liang, S., Sun, N., Meersmans, J., Longdoz, B., Colinet, G., Xu, M., et al. (2024). Impacts of climate change on crop production and soil carbon stock in a continuous wheat cropping system in Southeast England. Agric. Ecosyst. Environ. 365:108909. doi: 10.1016/j.agee.2024.108909
Liang, S., Sun, N., Wang, S., Colinet, G., Longdoz, B., Meersmans, J., et al. (2023). Manure amendment acts as a recommended fertilization for improving carbon sequestration efficiency in soils of typical drylands of China. Front. Environ. Sci. 11:1173509. doi: 10.3389/fenvs.2023.1173509
Liang, A. Z., Zhang, X. P., Yang, X. M., McLaughlin, N. B., Shen, Y., and Li, W. F. (2009). Estimation of total erosion in cultivated Black soils in Northeast China from vertical profiles of soil organic carbon. Eur. J. Soil Sci. 60, 223–229. doi: 10.1111/j.1365-2389.2008.01100.x
Lin, Y., Feng, Z., Wu, W., Yang, Y., Zhou, Y., and Xu, C. (2017). Potential impacts of climate change and adaptation on maize in Northeast China. Agron. J. 109, 1476–1490. doi: 10.2134/agronj2016.05.0275
Liu, X., Lee Burras, C., Kravchenko, Y. S., Duran, A., Huffman, T., Morras, H., et al. (2012). Overview of Mollisols in the world: distribution, land use and management. Can. J. Soil Sci. 92, 383–402. doi: 10.4141/CJSS2010-058
Liu, C., Wang, L., Cocq, K.Le, Chang, C., Li, Z., Chen, F., et al. (2020). Climate change and environmental impacts on and adaptation strategies for production in wheat-rice rotations in southern China. Agric. For. Meteorol. 292,:108136. doi: 10.1016/j.agrformet.2020.108136
Liu, S., Yang, J. Y., Zhang, X. Y., Drury, C. F., Reynolds, W. D., and Hoogenboom, G. (2013). Modelling crop yield, soil water content and soil temperature for a soybean-maize rotation under conventional and conservation tillage systems in Northeast China. Agric. Water Manag. 123, 32–44. doi: 10.1016/j.agwat.2013.03.001
Liu, H., Zhang, T., Liu, B., Liu, G., and Wilson, G. V. (2013). Effects of gully erosion and gully filling on soil depth and crop production in the black soil region, Northeast China. Environ. Earth Sci. 68, 1723–1732. doi: 10.1007/s12665-012-1863-0
Maillard, É., and Angers, D. A. (2014). Animal manure application and soil organic carbon stocks: a meta-analysis. Glob. Chang. Biol. 20, 666–679. doi: 10.1111/gcb.12438
Mandal, A., Patra, A. K., Singh, D., Swarup, A., and Ebhin Masto, R. (2007). Effect of long-term application of manure and fertilizer on biological and biochemical activities in soil during crop development stages. Bioresour. Technol. 98, 3585–3592. doi: 10.1016/j.biortech.2006.11.027
Perego, A., Wu, L., Gerosa, G., Finco, A., Chiazzese, M., and Amaducci, S. (2016). Field evaluation combined with modelling analysis to study fertilizer and tillage as factors affecting N2O emissions: a case study in the Po valley (northern Italy). Agric. Ecosyst. Environ. 225, 72–85. doi: 10.1016/j.agee.2016.04.003
Posner, J. L., Baldock, J. O., and Hedtcke, J. L. (2008). Organic and conventional production systems in the Wisconsin integrated cropping systems trials: I. Productivity 1990-2002. Agron. J. 100, 253–260. doi: 10.2134/agronj2007.0058
Ramírez, P. B., Calderón, F. J., Fonte, S. J., and Bonilla, C. A. (2019). Environmental controls and long-term changes on carbon stocks under agricultural lands. Soil Tillage Res. 186, 310–321. doi: 10.1016/j.still.2018.10.018
Riahi, K., Rao, S., Krey, V., Cho, C., Chirkov, V., Fischer, G., et al. (2011). RCP 8.5-a scenario of comparatively high greenhouse gas emissions. Clim. Chang. 109, 33–57. doi: 10.1007/s10584-011-0149-y
Sanford, G. R., Posner, J. L., Jackson, R. D., Kucharik, C. J., Hedtcke, J. L., and Lin, T. L. (2012). Soil carbon lost from Mollisols of the north Central U.S.a. with 20 years of agricultural best management practices. Agric. Ecosyst. Environ. 162, 68–76. doi: 10.1016/j.agee.2012.08.011
Schmidt, M. W. I., Torn, M. S., Abiven, S., Dittmar, T., Guggenberger, G., Janssens, I. A., et al. (2011). Persistence of soil organic matter as an ecosystem property. Nature 478, 49–56. doi: 10.1038/nature10386
Six, J., Conant, R. T., Paul, E. A., and Paustian, K. (2002). Stabilization mechanisms of SOM implications for C saturation of soils. Plant Soil 241, 155–176. doi: 10.1023/A:1016125726789
Smith, J., Smith, P., Wattenbach, M., Zaehle, S., Hiederer, R., Jones, R. J. A., et al. (2005). Projected changes in mineral soil carbon of European croplands and grasslands, 1990-2080. Glob. Chang. Biol. 11, 2141–2152. doi: 10.1111/j.1365-2486.2005.001075.x
Stagnari, F., Maggio, A., Galieni, A., and Pisante, M. (2017). Multiple benefits of legumes for agriculture sustainability: an overview. Chem. Biol. Technol. Agric. 4, 1–13. doi: 10.1186/s40538-016-0085-1
Stewart, C. E., Paustian, K., Conant, R. T., Plante, A. F., and Six, J. (2007). Soil carbon saturation: concept, evidence and evaluation. Biogeochemistry 86, 19–31. doi: 10.1007/s10533-007-9140-0
Stewart, C. E., Paustian, K., Conant, R. T., Plante, A. F., and Six, J. (2008). Soil carbon saturation: evaluation and corroboration by long-term incubations. Soil Biol. Biochem. 40, 1741–1750. doi: 10.1016/j.soilbio.2008.02.014
Triberti, L., Nastri, A., and Baldoni, G. (2016). Long-term effects of crop rotation, manure and mineral fertilisation on carbon sequestration and soil fertility. Eur. J. Agron. 74, 47–55. doi: 10.1016/j.eja.2015.11.024
Tuzet, A., Perrier, A., and Leuning, R. (2003). A coupled model of stomatal conductance, photosynthesis and transpiration. Plant Cell Environ. 26, 1097–1116. doi: 10.1046/j.1365-3040.2003.01035.x
van Vuuren, D. P., Stehfest, E., den Elzen, M. G. J., Kram, T., van Vliet, J., Deetman, S., et al. (2011). RCP 2.6: exploring the possibility to keep global mean temperature increase below 2°C. Clim. Chang. 109, 95–116. doi: 10.1007/s10584-011-0152-3
Virk, A. L., Lin, B. J., Kan, Z. R., Qi, J. Y., Dang, Y. P., Lal, R., et al. (2022). Simultaneous effects of legume cultivation on carbon and nitrogen accumulation in soil. Adv. Agron. 171, 75–110. doi: 10.1016/bs.agron.2021.08.002
Vrugt, J. A., Gupta, H. V., Bastidas, L. A., Bouten, W., and Sorooshian, S. (2003). Effective and efficient algorithm for multiobjective optimization of hydrologic models. Water Resour. Res. 39, 1–19. doi: 10.1029/2002WR001746
Walkley, A., and Black, I. A. (1934). An examination of the degtjareff method for determining soil organic matter, and a proposed modification of the chromic acid titration method. Soil Sci. 37, 29–38. doi: 10.1097/00010694-193401000-00003
Wang, M., Li, Y., Ye, W., Bornman, J. F., and Yan, X. (2011). Effects of climate change on maize production, and potential adaptation measures: a case study in Jilin province, China. Clim. Res. 46, 223–242. doi: 10.3354/cr00986
Wang, Y., Tao, F., Chen, Y., and Yin, L. (2022). Interactive impacts of climate change and agricultural management on soil organic carbon sequestration potential of cropland in China over the coming decades. Sci. Total Environ. 817:153018. doi: 10.1016/j.scitotenv.2022.153018
Wang, S., Zhao, Y., Wang, J., Gao, J., Zhu, P., Cui, X., et al. (2020). Estimation of soil organic carbon losses and counter approaches from organic materials in black soils of northeastern China. J. Soils Sediments 20, 1241–1252. doi: 10.1007/s11368-019-02520-2
Wu, L., McGechan, M. B., McRoberts, N., Baddeley, J. A., and Watson, C. A. (2007). SPACSYS: integration of a 3D root architecture component to carbon, nitrogen and water cycling-model description. Ecol. Model. 200, 343–359. doi: 10.1016/j.ecolmodel.2006.08.010
Wu, L., Rees, R. M., Tarsitano, D., Zhang, X., Jones, S. K., and Whitmore, A. P. (2015). Simulation of nitrous oxide emissions at field scale using the SPACSYS model. Sci. Total Environ. 530-531, 76–86. doi: 10.1016/j.scitotenv.2015.05.064
Xu, X., Pei, J., Xu, Y., and Wang, J. (2020). Soil organic carbon depletion in global Mollisols regions and restoration by management practices: a review. J. Soils Sediments 20, 1173–1181. doi: 10.1007/s11368-019-02557-3
Xu, H., Shen, H. P., Zhou, S. W., Wang, X. L., and Sun, N. (2016). Determination of soil organic matter content by heating method with an aluminum module digesting device. China Soil Fert. 3, 140–144. (in Chinese). doi: 10.11838/sfsc.20160323
Yan, X., Zhou, H., Zhu, Q. H., Wang, X. F., Zhang, Y. Z., Yu, X. C., et al. (2013). Carbon sequestration efficiency in paddy soil and upland soil under long-term fertilization in southern China. Soil Tillage Res. 130, 42–51. doi: 10.1016/j.still.2013.01.013
Yin, X., and Struik, P. C. (2009). C3 and C4 photosynthesis models: an overview from the perspective of crop modelling. NJAS Wageningen J. Life Sci. 57, 27–38. doi: 10.1016/j.njas.2009.07.001
Yin, Y., Tang, Q., and Liu, X. (2015). A multi-model analysis of change in potential yield of major crops in China under climate change. Earth Syst. Dynam. 6, 45–59. doi: 10.5194/esd-6-45-2015
Yu, G., Fang, H., Gao, L., and Zhang, W. (2006). Soil organic carbon budget and fertility variation of black soils in Northeast China. Ecol. Res. 21, 855–867. doi: 10.1007/s11284-006-0033-9
Zhang, S., Bai, Y., Zhang, J. H., and Ali, S. (2021). Developing a process-based and remote sensing driven crop yield model for maize (PRYM–maize) and its validation over the Northeast China plain. J. Integr. Agric. 20, 408–423. doi: 10.1016/S2095-3119(20)63293-2
Keywords: yield, SOC stock, carbon sequestration efficiency, Mollisols, SPACSYS model, long-term fertilization, climate change
Citation: Liang S, Sun N, Longdoz B, Meersmans J, Ma X, Gao H, Zhang X, Qiao L, Colinet G, Xu M and Wu L (2024) Both yields of maize and soybean and soil carbon sequestration in typical Mollisols cropland decrease under future climate change: SPACSYS simulation. Front. Sustain. Food Syst. 8:1332483. doi: 10.3389/fsufs.2024.1332483
Edited by:
Ashim Datta, Central Soil Salinity Research Institute (ICAR), IndiaReviewed by:
Dibakar Roy, National Bureau of Soil Survey and Land Use Planning (ICAR), IndiaSamrat Ghosh, Emergent Ventures India, India
Copyright © 2024 Liang, Sun, Longdoz, Meersmans, Ma, Gao, Zhang, Qiao, Colinet, Xu and Wu. This is an open-access article distributed under the terms of the Creative Commons Attribution License (CC BY). The use, distribution or reproduction in other forums is permitted, provided the original author(s) and the copyright owner(s) are credited and that the original publication in this journal is cited, in accordance with accepted academic practice. No use, distribution or reproduction is permitted which does not comply with these terms.
*Correspondence: Minggang Xu, eHVtaW5nZ2FuZ0BjYWFzLmNu; Nan Sun, c3VubmFuQGNhYXMuY24=