- 1Department of Crop Production, Université Evangélique en Afrique (UEA), Bukavu, Democratic Republic of Congo
- 2Department of Agronomy, Université Officielle de Bukavu (UOB), Bukavu, Democratic Republic of Congo
- 3International Institute of Tropical Agriculture (IITA), Ibadan, Nigeria
Yam (Dioscorea spp.) is a key tuber crop in sub-Saharan Africa (SSA) with huge potential for poverty alleviation, food sovereignty, and nutrition security. Exploiting its full potential requires that factors holding it down are understood and mitigated. This study, conducted between May and July 2022 and 2023, assessed yam farming practices, varietal preferences, and land suitability in the Eastern Democratic Republic of Congo (DRC). We interviewed 765 smallholder farmers within four agro-ecological zones (AEZ) to assess the sociocultural, agronomic, varietal, and biophysical factors affecting yam production. Land suitability analyses were conducted using the Analytic Hierarchy Process (AHP) to identify areas suitable to extensive yam production and to cluster environments that could optimize yam varietal selection and testing. Results showed that yam is mainly cultivated by women in eastern DRC (70%). The seed delivery system is informal, relying primarily on farmer-saved seeds and farmer-seed exchanges (74.9%). Soil depletion (68.3%), limited access to high-quality seeds (54.5%), youth disengagement in yam value chain (50.3%), insect pests (17.9%), and short tuber shelf-life (65.8%) were, respectively, the main ecological, agronomic, sociocultural, biological, and tuber quality factors hindering yam production in eastern DRC. However, the perceived importance of most factors significantly varied with farmer gender and age categories. A multitude of varietal traits was used to assess yam varieties in eastern DRC, of which the tuber taste (59%) was the most valued trait regardless of gender and age categories, though it had highest scores among middle-aged adult women. Land suitability analyses discriminated five clusters; the most significant part of the region falling under suitable (27%), highly suitable (24%), and very highly suitable classes (37%). We further discussed how breeding initiatives for delivering yam varieties, suiting local producers’ and end-users’ needs, could unlock the crop’s potential for enhancing food security and wealth creation in eastern DRC. The land suitability map from this study is a valuable decision-making tool in defining priority areas for extensive yam production and varietal selection and testing. This study provided valuable insights on factors affecting yam production and suggested yam breeding as a backbone of a holistic approach seeking to address challenges faced in unlocking the potential for yam production in eastern DRC.
1 Introduction
Yam (Dioscorea spp.) is a key staple crop in tropical and subtropical regions of the globe. It produces starchy underground tubers or aerial bulbils, providing valuable sources of dietary carbohydrates, proteins, vitamins, and essential minerals (Agre et al., 2022a). Alongside other root and tuber crops, yam has been a major food security pillar in sub-Saharan Africa (SSA) owing to its resilience to climate hazards and pests when compared to cereals and legumes (Thiele et al., 2017). Despite its potential for food security and wealth creation, yam is still regarded as a subsistence crop, practiced mainly by women on small plots, in Democratic Republic of Congo (DRC) where it was listed among neglected and underutilized (orphan) crop species (Mondo et al., 2021; Adejumobi et al., 2022a, 2023).
This crop potential for food sovereignty and wealth creation is yet to be unlocked due to the absence of incentive policies from the government, changes in the nutritional habits among youths, rapid urbanization, and the lack of funds to support research institutions and universities on yam related topics. Consequently, yam has been gradually substituted for cassava, sweet potato, and maize due to a lack of genetic improvement to meet farmers’ and consumers’ preferred criteria (Adejumobi et al., 2022b). Based on the Food and Agriculture Organization (FAO) statistics for 2010–2020, yam yield in DRC has remained stagnant at ~4.3 t ha−1, a tenth of the crop potential in Africa (Agre et al., 2022a). On the other hand, an increase in production (20.3%) was recently observed and this is tightly associated with the expansion of cultivated lands (21.9%) rather than the yield increase per unit area (FAO, 2023). Such agricultural practices are not sustainable and climate-smart since they accelerate deforestation and greenhouse gas emissions (Kiba et al., 2020; Karume et al., 2022).
There is extensive yam production in the western forest regions of DRC and the highlands of Kivu in the eastern part (Adejumobi et al., 2023). In eastern DRC, mainly in the North-and South-Kivu provinces, yam is highly valued for its cultural and therapeutic virtues. For instance, yam is one of the staples with the highest market values in the local urban markets of Bukavu and Goma, though its price fluctuates significantly with the season. Rural populations in the vicinity of those cities cultivate yam as a cash crop, allocating a large share of harvest to market participation. Plant breeding could be instrumental to releasing this crop’s potential for food sovereignty and wealth creation by availing varieties that suit farmers and end-users needs and preferences (Mondo et al., 2020; Kalu et al., 2023). However, effective intervention strategies would imply that these farmers’ and end-users’ needs and preferences are assessed prior to any breeding and seed delivery initiatives, since no related contextual analysis exist in eastern DRC. This study fills that gap by documenting the ecological, agronomic, sociocultural, and food quality factors hindering yam production and recording farmers’ varietal preferences in eastern DRC.
In addition to understanding farmers’ perceptions towards factors hindering yam production, assessing land suitability is critical to recommending appropriate farming practices and developing varieties that suit local biophysical conditions (Alabi et al., 2019; Igbawua et al., 2022). Furthermore, for implementing a yam breeding program, clustering environments within agro-ecological zones (AEZ) will provide valuable insight for optimizing yam varietal selection and testing (Alabi et al., 2019). The use of geo-spatial tools, such as the Analytic Hierarchy Process (AHP), has proven effective in mapping suitable areas for the cultivation of various crops (Chuma et al., 2021; Mugiyo et al., 2021; Igbawua et al., 2022).
As in other SSA countries, DRC still faces unprecedented food insecurity and poverty challenges. In fact, DRC is the second largest hunger crisis in the world after Yemen and among the five poorest nations in the world (World Bank, 2023). As for the year 2022, nearly 62% of Congolese, around 60 million people, lived below the international poverty line ($2.15 a day), making that about one out of six people living in extreme poverty in SSA lives in DRC (World Bank, 2023). Despite the staggering levels of food insecurity in DRC, it has the highest agricultural potential in Africa owing to its favorable climates for agriculture and fertile soils (i.e., 80 million ha of arable lands of which only 10% is effectively exploited) with potential to feed over 2 billion people through suitable investments (OCHA, 2022). Root and tubers crops, including yams, are the backbone of food security across the humid tropics in SSA, providing food and income to more than 300 million people in developing countries (Thiele et al., 2017; Agre et al., 2022b). This importance is expected to increase under the present and future climate change scenarios as these crops are highly resilient as compared to cereals and legumes (Thiele et al., 2017; Karume et al., 2022). As for other root and tuber crops, yam is a food security crop in DRC for several reasons: (i) it is the third most consumed tuber crop after cassava and sweet potato, (ii) it fills the hunger gap during drought and lean periods; (iii) it provides dietary fibers, vitamin C, vitamin B6, proteins, potassium, manganese, and low saturated fat and sodium contents (Agre et al., 2022b); (iv) it is less sensitive to biotic and abiotic stresses and thus could substitute cassava in disease-prone areas; (iv) it is a source of income for households involved in its production and trade (Mondo et al., 2021); etc. We hypothesized that encouraging extensive yam production, as it is already the case in the African yam belt of West Africa (Asiedu and Sartie, 2010), could contribute to achieving food sovereignty in DRC as the Congolese climate is suitable to its cultivation and its starchy tubers are already rooted in local diets (Mondo et al., 2021). However, unlocking yam full potential for poverty alleviation and food sovereignty requires that improved varieties suiting farmers’ needs, practices, and environments are developed through breeding (Mondo et al., 2020).
Therefore, this study aimed at assessing: (a) yam farming practices and varietal preferences in South-Kivu; (b) identifying the major factors limiting extensive yam production and utilization in South-Kivu; (c) identifying suitable lands for yam cultivation across AEZs in South-Kivu, eastern DRC. Outcomes from this study are valuable assets to enhancing the population food security in eastern DRC, since improved yam productivity in the region will increase physical and economic access to sufficient, safe, and nutritious food provided by yam starchy tubers to meet the population dietary needs and food preferences for an active healthy lifestyle (Karume et al., 2024).
2 Materials and methods
2.1 Study area
This study was conducted in South-Kivu province (1.58369–5.01032°S, 26.81065–29.43595°E, 465–3,400 m above sea level), eastern DRC (Figure 1). South-Kivu covers a surface area of ~65,070 km2, divided into eight territories of which six were included in surveys: Kabare (1,960 km2), Fizi (15,788 km2), Kalehe (5,126 km2), Idjwi (an island in Lake Kivu, 281 km2), Uvira (3,148 km2), and Walungu (1,800 km2). Specific characteristics of each of these territories are summarized in Supplementary Table S1. The South-Kivu Province is part of the Great Rift Valley and is bordered by Rwanda, Burundi, and Tanzania to the east; North-Kivu Province to the north; Tanganyika Province to the south, Maniema Province to the west. Its population is estimated at ~5,772,000 from at least 15 ethnic groups (Vlassenroot, 2002; OIM, 2022), living mainly of agriculture, livestock, fishing, mining, and trade as main economic activities, especially among rural populations (Maass et al., 2012). The province’s topography is an amalgamation of geographical features, comprising mountains, dense forests, cascading waterfalls, and fertile inland valleys. This diversity of climates and topographies allows a wide range of crops. Of these crops, banana, rice, cassava, maize, common bean, sweet potatoes, and yam are the most widely produced (Maass et al., 2012; Mugumaarhahama et al., 2021). South-Kivu also harbors a wide diversity of vegetables and industrial perennial crops such as tea, coffee, cinchona, etc. especially in its highlands of Kabare, Walungu, Kalehe, and Idjwi (Maass et al., 2012). The region’s rich natural resources, such as minerals and timber, have been a driving factor for recurrent conflicts since the mid-1990s (Vlassenroot, 2002).
2.2 Agro-ecological zones clustering
The province was first clustered into four agro-ecological zones (AEZ) based on 13 biophysical characteristics (Supplementary Table S2). To achieve this, we created points representing each surveyed farm. Then, using spatial analysis in ArcGIS 10.7 Esri-TM, we extracted information from images. Data included the ALOSPALSAR digital elevation model (DEM) with a spatial resolution of 12.5 m, as well as data from local weather stations. In areas without weather stations, we used data extracted from AfriClim and WorldClim v1.4 and CHIRPS v1.8 (Platts et al., 2015; Gebrechorkos et al., 2019).
Numerous statistical techniques exist for partitioning a population into different classes or sub-groups. Escofier and Pagès (1991) and Abdessemed and Escofier (1996) suggested the use of multivariate analyses such as multiple correspondence analysis (MCA), principal component analysis (PCA), factor analysis (FA), etc. In our case, we used PCA followed by ascending hierarchical classification (AHC). The aim was to ensure that farms grouped within the same class (intra-class homogeneity) are as similar as possible, while the typology obtained (represented in the factorial plan) with classes are as dissimilar as possible (inter-class heterogeneity). A new variable was thus created, “AEZ,” and grouped into “AEZ1, AEZ2, AEZ3, and AEZ4.” Comparative analyses of variables grouped by AEZ were then performed and presented in tabular form. Decision on how many principal components (PCs) to retain was based on three criteria: (1) the Kaiser criterion from which all principal components exceeding an eigenvalue of 1 were initially retained; (2) the Scree plot test and the minimum cumulative percentage of variance were chosen; (3) the interpretability criterion was used to assess the conceptual significance of components in terms of hypotheses being tested. Thus, a two-stage approach was followed: AHC using Ward’s method and the average maximum silhouette coefficient were used to define the number of groups or AEZs in the study area. Ward’s method provides a range of clustering solutions, where each observation starts out as its own group and is gradually joined by similar observations until only one group remains. This aggregation process is presented by a dendrogram, and the decision to cut the dendrogram was made based on the maximum mean silhouette coefficient width with different clusters (Supplementary Figure S1). Once the number of groups to be retained had been selected, the Kruskal-Wallis test was performed to determine significant differences between variables in different AEZs.
2.3 Farmers’ perceptions on farming practices, varietal preferences, and factors limiting yam intensification in south-Kivu
A pre-survey was conducted to determine the main yam growing areas in South-Kivu province and to map key stakeholders. For this purpose, secondary data was gathered from the Provincial Inspectorate of Agriculture (IPAGRI)’s annual reports. Besides, focus group discussions were organized with resource persons (extension agents, representatives of farmers’ associations, local administrative authorities, experienced yam producers, etc.). The questionnaire draft was also discussed with resource persons to assess the questions’ relevance to the survey’s target populations.
Sampling started by selecting territories based on the yam production importance, security, and accessibility. Within territories, a total of 17 local administrative zones (locally referred to as “Groupements”) were sampled based on the same criteria as for territories, and within the “Groupements,” 117 yam producing villages were randomly selected from those listed by resource persons (Supplementary Table S3). Since the exact number of yam farmers was unknown, a probabilistic saturation sampling technique was used to define the sample size in each selected village (Pires, 1997). In this sampling technique, the survey only ceased when interviews or observations of new participants no longer revealed further information. Our rationale in going for probabilistic sampling was rooted in the belief that additional data collection was unlikely to yield new insights. The snowball method was used to reach individual respondents (Etikan et al., 2016), as it proved effective in studying populations that are challenging to access through traditional sampling methods. In addition, this approach enabled us to leverage social networks for the identification and recruitment of participants. Though both methods are potentially subject to sampling biases, such as an underrepresentation of certain perspectives or regions (for the probabilistic saturation method) or a non-random selection of participants especially if the initial contacts share similar characteristics or opinions (for snowball method), these methods were both time-efficient and cost-effective in our study’s context. Besides, we were keen in selecting contrasting villages to ensure high representation of opinions and characteristics in the overall sample of a given territory in addition to associating the mixed-method approach combining the two methods with the triangulation to enhance the robustness of the study. In each village, an average of 5 farmers were interviewed using a semi-structured questionnaire addressed to the adult member of the household directly involved in yam production and/or trade. Prior informed consent of the respondents was taken and the study objectives were described to them. A total of 765 yam farmers were interviewed individually across the province. As highlighted above, the questionnaire was first discussed with resource persons to ensure chances of contradictory responses or divergent opinions among farmers were minimized prior the survey.
Collected data included socio-economic and demographic information on yam farmers (age, gender, education level, family size, farming experience, household income, main activity, and farm size), existing yam plant genetic resources (seed source, number of cultivars, and farmers’ preference criteria), farming practices (cropping system, planting season, crop cycle, harvesting frequency, tuber use, and post-harvest storage methods), and main factors hindering large-scale yam production and use in South-Kivu (agronomic, ecological, biological, sociocultural, and tuber quality factors). Farmers’ trait preferences based on agronomic, culinary, and therapeutic characteristics were documented using 14 traits, including tuber yield potential, tolerance to diseases and insect pests, seed accessibility, market demand, earliness, tuber flesh texture (boiled tuber texture, tuber taste, tuber flesh color, and storage capacity), nutritional value, presence or absence of tuber spines, shelf-life longevity, therapeutic virtues, and bitterness. Surveys were conducted at the beginning of 2022 and 2023 dry seasons (May to July), corresponding to the harvesting season in the region since the data collection was associated with the collection of planting materials for characterization. It is noteworthy that yam is a long cycle crop with a cycle spanning from 8 to 12 months, meaning that farmers can only grow yam once a year. In general, eastern DRC farmers plant yam at beginning of the long rainy season (September to early November) while harvest occurs from May to July the following year. Thus, since yam cycle spans to the entire year (including 3 months of seed dormancy), the seasonal effect could not be accounted for in this study. The questionnaire was coded in Kobo Collect tool.
2.4 Land suitability analysis for yam production
2.4.1 Satellite image sources and choice of variables used in land suitability analysis
Land suitability analyses were performed using data available from online platforms (Supplementary Table S4). These data included shapefiles of territories, villages, roads, and rivers in DRC. They were retrieved from the “Référentiel Géographique Commun” (RGC, http://www.rgc.cd/). Satellite images, also freely accessible, were added to this dataset. These satellite images included a digital elevation model (DEM) of the ALOSPALSAR type (with 12.5 m resolution). A ground cover for 2018 land cover image was also used. Soil and climate data were also included into analyses. All these files were first extracted according to the study area before launching the spatial analysis and building the model. ArcGIS 10.7 Esri-TM was used for spatial analysis. In this study, ten variables were used for land suitability analysis (Supplementary Table S5): land use and land cover (LULC), slope (%), elevation (m), climate type, pH, cation exchange capacity (CEC), Euclidean distances (ED) to roads, rivers, villages (in km). The selection of these variables was guided by several elements: (i) review of literature, (ii) consultation with local experts specialized in the thematic area, and (iii) consideration of local specificities, especially regarding accessibility and topography. An additional criterion was the availability and access of data. We also selected variables avoiding collinearity by ensuring they provide independent and non-redundant information while analyzing.
The major challenge was to determine the importance or relative weight of each factor/variables in the multi-criteria decision support process. In this study, weights were allocated to variables as in Supplementary Table S6 based on Saaty (1980)’s scale. It is noteworthy that ArcGIS 10.7 has a spatial tool called “weighted overlay” that applies the weight of each factor to produce the spatial result. Weighted overlay is a sub-type of many other overlay methods, though the one using weighted value is the mostly used. The consistency index (CI) was calculated as follows:
With the maximum eigenvalue of the matrix, and the number of criteria (here = 10). Derived CI/RI (Random Inconsistency Index) ratio, referred to as the consistency ratio (CR), was also calculated. To validate the matrix and the weights for each criterion, a threshold required by Saaty (1980) was adopted, i.e., the CR value had to be <0.1.
2.4.2 Analytic hierarchy process and determination of variable weights
Analytic Hierarchy Process (AHP) as proposed by Saaty (1990) was used. It has been proven effective in dealing with complex decision-making. Besides, AHP incorporates a useful technique for checking for consistency of decision-maker’s assessments, thus reducing bias in the decision-making process. AHP involved a pairwise comparison of all selected criteria. Criteria of equal importance were assigned the value 1, and those of extreme/absolute importance in the comparison matrix were assigned the value 9 (Supplementary Table S7). The matrix obtained after pairwise comparison was presented. Consistency of the 10th-order matrix was also assessed. Comparisons made using this method were subjective, and AHP tolerated inconsistency by the amount of redundancy in the approach. If this consistency index failed to reach a required threshold level, comparison responses were re-examined. In fact, the AHP analysis estimated the weights of factors (criteria) and involved a process of pairwise comparisons and mathematical calculations. The AHP method is widely used for decision-making and prioritization and consisted first of comparing each pair of factors to determine their relative importance, with respect to the criterion above them. This helped constructing a matrix based on the pairwise comparison (Supplementary Table S6). It is, however, noteworthy that AHP is subjective and relies on the judgments of decision-makers.
In this study, five FAO land suitability classes were used to quantify the extent of suitability for yam in South-Kivu (FAO, 1993; Supplementary Table S8). This framework classifies land into four suitability categories: land suitability orders, land suitability classes, land suitability subclasses, and land suitability units (Topuz and Deniz, 2023). In the FAO classification, orders indicate land suitable for cultivation (S) or unsuitable for cultivation (N), while classes indicate the degree of land suitability, such as (S1) highly suitable, (S2) moderately suitable, (S3) marginally unsuitable, (N1) currently unsuitable and (N2) definitely unsuitable, then subclasses explain limitations. The classification designates a single utilization index as the best for each land unit (Özkan et al., 2020). The land suitability index for yam was calculated using an additive linear combination model (Chuma et al., 2021). Yam soil and climate requirements were sourced from literature (Adifon et al., 2019a,b; Neina, 2021; Igbawua et al., 2022; Agre et al., 2022a) and summarized in Supplementary Table S9.
2.5 Data analyses
Though data were collected on the basis of territories, statistical analyses were performed based on AEZs, gender, and age categories. Descriptive statistics (frequency, means, standard deviation, etc.) was performed on survey data. Pearson’s χ2 test was used to compare the independence of AEZs for different qualitative variables. Analysis of variance (ANOVA) was performed on quantitative data to appreciate differences among AEZs while Tukey’s honestly significant difference (HSD) test facilitated mean separation at 5% p-value threshold. All analyses were performed using R-Studio 4.2 (R Core Team, 2020). More specifically, we used HH and likert R packages for graph creation and libraries ggplot2, questionr, and dplyr for data visualization and management.
Spatial analyses were conducted in ArcGIS 10.7 Esri-TM, starting with images reclassification and extraction of province-level information. Rasters obtained after these steps were also reclassified into different classes based on their suitability for yam production, using a five-level scale as suggested by Saaty (1980) and which was similar to the “Likert scale” (Lewis and Erdinç, 2017). These classes were referred to as: “Very high, high, moderate, low, and marginal.” Once all the criteria had been declassified, the “Weighted Overlay” tool in ArcGIS was used to generate suitability classes. For each criterion, a weighting factor as provided in Supplementary Table S8 was used at the end to generate final results. Finally, the resulting frame was reclassified into four suitability classes.
3 Results
3.1 Agro-ecological zones in south-Kivu province
Based on the Kaiser criterion and the minimum cumulative percentage of variance, we selected the first four components for interpretation, and which explained 88% of the total variance. Correlation circle of 13 variables used for AEZ clustering, correlation coefficients, and contribution of variables to the formation of component axes are presented in Supplementary Figure S2. Based on results from AHC performed after PCA, we found four AEZs in the South-Kivu province. Differences and designations among these AEZs are summarized in Table 1. AEZs differed primarily with altitudes, which in turn, regulated temperatures and rainfall patterns. AEZ1 was a low altitude agro-ecological zone with slightly rich soil, hot weather, with moderate access to land (farmers possessed very small plots). AEZ2 was characterized by medium altitude with moderate weather and depleted soils. AEZ3 was located at high altitudes and characterized by a humid tropical climate, bimodal rainfall patterns, long rainy season exceeding nine months, less farm accessibility, and larger farm plots. AEZ4 was as well located at high altitudes but experiencing tropical mountainous climate highly regulated by Lake Kivu, less marked dry season, highly accessible, and smaller farm plots (Table 1).
In terms of land coverage, AEZ1 made 46.4% of South-Kivu lands distributed across Fizi (97%), Uvira (25%), Kalehe (22%), and Kabare territories (12%). AEZ2 (44.9% of the provincial land) covered 80% of Walungu, 74% of Uvira, 64% of Kalehe, 51% of Kabare, and 3% of Fizi territories. AEZ3, representing 6.1% of available lands, extended to Kabare (24%), Kalehe (14%), Walungu (10%), Idjwi (6%), and Uvira territories (1%). AEZ4 (2.5% of South-Kivu lands) covered 94% of Idjwi, 13% of Kabare, and 10% of Walungu territories (Supplementary Table S10).
3.2 Yam farming practices, varietal preferences, and challenges across AEZs
3.2.1 Socioeconomic characteristics among south-Kivu yam farmers
Yam is mainly cultivated by women (>70%), aged between 30 and 49 years old (54.3%), with low education level (45.5% had no formal education and 35.78% had primary education level), and a yam farming experience of 10 years or more (58.2%). These farmers mainly rely on agriculture (including livestock and fishery) as their main economic activity (91.2%), though a minority had non-farm income-generating activities such as handicrafts, petty trade, and public services (Table 2). Their daily incomes were low (<1.6 US$ a day) to medium (1.6–4 US$ a day). Yam farmers possess very small plots (~0.6 ha), with significant disparities across AEZs. These producers had large households, more than 60% having 5–10 family members (Table 2).
3.2.2 Yam farming practices in south-Kivu
There were significant disparities among AEZs for most farming practices (p < 0.05). In general, yam is grown as a subsistence crop (either for food or medicine) in eastern DRC and is practiced on house gardens and small farmlands (~0.15 ha), using traditional farming methods and local landraces. Varietal diversity was low with almost all farmers (98%) relying on one or two cultivars (Table 3). The seed delivery system was mainly informal; ~75% farmers acquired seed through farmer-saved seed or farmer-seed exchanges, less than 10% farmers paid for seed at local market while forest environments via ennoblement efforts provided seed to ~15% farmers in South-Kivu. Planting and harvesting seasons depended on AEZs, the planting season generally spanned from September to mid-November across AEZs while the harvests occurred from June to September (Table 3). It is noteworthy that in some equatorial forest zones with almost no dry season, planting yam occurred at any time. Yam was mainly grown associated with other staple crops (88%), using mainly family labor (62%). The most common staple crops associated with yam were cassava (32.7%), banana (13.7%), and sweet potato (7.6%, Supplementary Table S11). Field management practices included three to four weeding (depending on AEZs and the cultivar cycle), ridging, staking, and rarely, gap filling (Supplementary Datasheet 1). At harvest, mainly decided upon plant senescence (drying), tubers were stored ex-situ using traditional techniques such as in-soil storage (especially for seed), on ground, in polythene bags and baskets or dried (Table 3). Such storage techniques allowed extending tuber shelf-life for one to four months until the next planting season.
3.2.3 Knowledge of diversity and use of yam cultivars in south-Kivu
Farmers had limited information on yam varieties and species they grew; vernacular yam names were used instead of the cultivar or species names. Ethnic diversity in the region made it difficult to confirm whether cultivars grown were different or were only designated by different names across ethnic groups. It is noteworthy that South-Kivu farmers mainly differentiated cultivars based on tuber flesh color and bitterness. Consequently, they only used vernacular name followed by flesh color or bitterness to designate a particular variety (Supplementary Table S12, Supplementary Datasheet 1). Based on farmers’ characterization, a total of 35 yam cultivars were identified in surveyed AEZs. Their use, however, varied from one ethnic group to another. Further information on yam varieties collected in South-Kivu are provided in Supplementary Datasheet 1. The existing genotypes could provide with an opportunity for cross-breeding since 18 of them flowered and possessed contrasting attributes for cropping cycle, tuber quality, and adaptation traits (Supplementary Table S12). These cultivars had either purple, white, or yellow flesh color after cooking. It is noteworthy that among consumed yams in eastern DRC, four were wild or semi-domesticated (Mange, Shekera, Birongo, and Kanyaburholi). These are popular among Batwa autochthonous people of Kabare and Idjwi.
3.2.4 Factors hindering yam production and its use in south-Kivu
Though all AEZs faced similar constraints, their magnitudes were different across AEZs (Table 4). Among ecological constraints, declining soil fertility (68.24%), recurrent droughts (16.08%), and soil erosion (12.0) were the most pressing factors hindering yam production across AEZs. Low access to quality seed (54.51%), low yield potential of existing cultivars (19.22%), and poor farming practices were listed as leading agronomic constraints. Farmers acknowledged youth lack of interest in the crop (50.33%), introduction of more profitable exotic crops (38.43%), and loss of cultural values owing to rapid urbanization (11.24%) as critical sociocultural factors hindering yam production and use across South-Kivu AEZs. Farmers were not much aware of biotic factors but raised insect pests (17.91%), rodents (7.58%), and weeds (4.58%) as the most pressing biological factors. On the other hand, low tuber shelf-life (65.75%), tuber bitterness (12.03%), and tuber oxidative browning (10.72%) were the leading food quality constraints (Table 4).
3.2.5 Influence of farmers’ gender and age on varietal trait preferences in south-Kivu
Trait preferences varied significantly with gender (χ2 = 44.14; p < 0.001) and age (χ2 = 45.99; p = 0.009) across AEZs (Table 5). A multitude of varietal traits were used to appreciate yam in South-Kivu, of which the tuber taste (59%) was the most valued regardless of gender and age, though it had highest scores among middle-aged adult women. Another trait with high scores was therapeutic virtues but more relevant to men than women (12.3 vs. 4.1%) and to old-aged than youth (10.2 vs. 7.3%). Other women traits were reduced tuber bitterness, high yield, tuber shelf-life, and crop rusticity. On the other hand, men were inclined toward easy seed access, market demand, early maturity, high profitability, and nutritional values. When considering age categories, most traits relevant to men were also attractive to youth: easy seed access, high market demand, high profitability, and nutritional values (Table 5).
3.3 Land suitability mapping for yam production in south-Kivu
The pair-wise comparison matrix of criteria and/or variables used in yam suitability in South-Kivu is provided in Supplementary Table S6. The CI was compared to a random matrix RI (RI for = 10 was 1.51). In our case, CI was estimated at 0.10 and the CR at 0.07 which was below 0.1, a threshold required by Saaty (1980). Thus, good consistency and coherence indices were observed for the selected criteria.
All climate types in South-Kivu were suitable to yam cultivation (Supplementary Table S13). The dominant climate was the Aw type, a tropical savannah climate with an average monthly temperature exceeding 18°C throughout the year and an annual rainfall >1,500 mm. This climate type was highly suitable for yam and was experienced in 41% of the province. Other climate types across the province included Am (22.7%), Cwb (15.1%), Af (12.2%), Cfb (5.2%), and Csa (3.8%). The most predominant soils were Humic Cambisols (31.64%), Haplic Acrisols (37.92%), Humic Ferralsols (14.20%), and Humic Nitisol (14.72%), all being suitable to yam. South-Kivu soils were mainly acidic (89.3% of the area having a pH of 4–5). Such acidic soils had low to moderate suitability for yam production. About 90% of the area had marginal to low suitability when considering CEC (<12.5 Cmol/kg). The altitude was suitable for yam in most areas (<1,500 masl) as well as slopes (<15%). Land use and land cover was favorable to yam since ~87.6% of farmland were closer to forests, and thus making it easy to access stakes or to adopt agroforestry. About 40% of farmlands had access to roads (<1 km) and thus very highly suitable since farm inputs could be supplied easily and the produce easily conveyed to market. The same trend is observed in terms of distance from settlements (for easy access to labor) and rivers (for convenient irrigation). Spatial distributions of these land suitability variables are presented in Supplementary Figure S3.
When combining these suitability criteria (Figure 2), we found that most of the lands in South-Kivu were favorable to extensive yam production. Of the total land, 37% were very highly suitable, 24% were highly suitable, and 27% were moderately suitable while the remaining areas were characterized by marginal to low suitability levels. Figure 3 provides the spatial distributions of lands according to their suitability to yam production in South-Kivu.
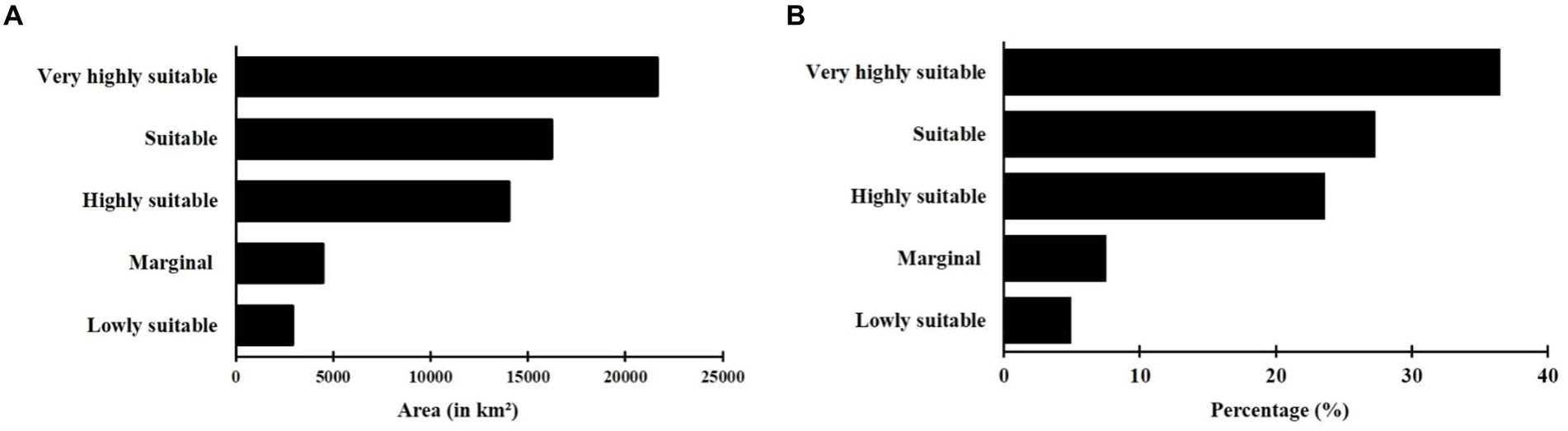
Figure 2. Suitability classes (A) in surface area (km2) and (B) in percentage (%) in South-Kivu, eastern DRC.
At the territorial level, we found that yam could be grown in all eight territories of South-Kivu, though Walungu (61%), Kabare (49%), and Shabunda (38%) had the highest proportions of lands with very high suitability to yam production (Supplementary Figure S4). Uvira had the largest proportion of marginal lands (41%) while Shabunda had the least (2.7%).
4 Discussion
4.1 Yam farming practices are still rudimentary and highly dependent on informal seed system in eastern DRC
Regardless of the location, yam was mostly grown by women in South-Kivu, and management practices for this crop were still rudimentary and related to those in other African regions where yam is grown for subsistence. In eastern DRC, yam was generally grown as a secondary crop in association with other root and tuber crops (cassava, sweet potato, or taro), legumes (mainly common bean), and cereals (maize or sorghum). In this way, yam farming is key for food security as it diversifies food and income of rural households in eastern DRC. These results agree with those of Adejumobi et al. (2022b, 2023) in northwestern DRC that showed that yam is, in surface and volume, a secondary crop and is mainly practiced on house gardens and small farmlands (<0.25 ha), using traditional farming methods and local landraces. Previous reports listed yam among neglected and underutilized (orphan) crop species in DRC (Mondo et al., 2021; Adejumobi et al., 2023).
Farmers preferred a limited number of cultivars that best met their expectations. These varieties were mainly landraces acquired through an informal seed system. Seed access depended largely on seed exchanges among farmers or purchased from informal markets. Since yam diversity is high in DRC (Magwé-Tindo et al., 2018), farmers were also acquiring seeds from forest environments, a practice referred to as ennoblement elsewhere (Scarcelli et al., 2006; Chikwendu and Okezie, 2014; Agre et al., 2021; Adejumobi et al., 2023). In addition to poor farming practices and informal seed system, farmers lacked sufficient knowledge of tuber storage techniques and value addition, leading to high post-harvest losses. Aboudou and Auriole (2006) showed that poor post-harvest storage led to 30–40% loss in Benin. Therefore, efforts to promote yam production should encourage investment in seed production and institutional support to yam farmer organizations as it has been the case in Burkina Faso, Cameroon, and Côte d’Ivoire (Ngassam et al., 2009; Cornet, 2015; Kiba et al., 2020).
Based on the above-listed constraints, it can be concluded that yam sector greatly lack institutional supports (local and international investments) as compared to other root and tuber crops in DRC. The farmer-support structures are, however, instrumental in introducing better agronomic practices and technologies (inputs), establishing producer-market connections, training of farmers, raising awareness on health benefits of the crop, introducing value-addition and processing options that can attract farmers and other stakeholders, etc. (Kiba et al., 2020). These actions are necessary to release yam potential for food security and income generation in eastern DRC. From a discussion with stakeholders, this lack of sufficient institutional support has widened the gap between the advancement of yam research vis-à-vis other root and tuber crops such cassava which has become more attractive since awareness has been raised on more profitable options and potentials of the crop.
4.2 Improving yam production and use in eastern DRC would require a holistic approach
Yam has been a central crop to food sovereignty and poverty alleviation in Africa (Asiedu and Sartie, 2010). However, its full potential is yet to be achieved in DRC owing to several factors holding it down. This study found that yam production is mainly constrained by lack of quality seed, declining soil fertility, youth’s lack of interest in the crop value chain, loss of cultural values due to rapid urbanization, insect pests, and short tuber shelf-life. This implies that yam promotion would only yield high impact if agronomic packages are introduced along with improved varieties, and market opportunities are increased by linking farmers with potential markets, increased awareness on nutritional and health benefits, and encouraging value-addition to meet the demand of the elites and increasingly urbanized populations. In such a holistic approach, breeding should be the backbone since low attractiveness of yam, as compared to other crops, is attributed to lack of varieties with farmer-preferred traits (Adejumobi et al., 2022b). Fortunately, more than 50% of yam cultivars grown in eastern DRC flowered, and thus, it opens an avenue for cross-breeding to generate variable offspring for selection (Mondo et al., 2023). Some of the traits lacking in yam in DRC are mainly yield-related traits, earliness, disease and pest resistance, and tuber quality attributes (long post-harvest shelf life, low tuber flesh oxidation, sweet taste, tuber softness, short cooking time, high tuber dry matter, ease of peeling, reduced bitterness, tuber size and shape) of local landraces (Adejumobi et al., 2022b, 2023). Consequently, yam has been gradually replaced by other staples like cassava, maize, sweet potato, etc. for which improved varieties exist and are easily accessible in the farmer vicinity. Lack of interest in yam production has also resulted in high prices at local markets to the extent that yam has become a rare, luxurious food, and unaffordable commodity by resource-poor families, especially in urban areas where yam is prized by people with chronic disease conditions such as diabetes and heart diseases.
It is noteworthy that yam farmers can only capture the benefit of the genetic gains from breeding programs when seed systems are well-functioning (Mausch et al., 2021; Stuart et al., 2021; Kalu et al., 2023). Stakeholders, especially seed multipliers, should be trained on how to rapidly multiply seeds. Different technologies of generating adequate and high quality seed yam (minisett, microsetts/micro tubers, tissue culture, vine cuttings, aeroponics, bioreactor systems, and the semi-autotrophic hydroponics (SAH)) as already implemented in West Africa should be proposed to Congolese farmers and seed companies (Adewumi et al., 2021; Adejumobi et al., 2023). Some of those technologies, such as the SAH, have already been introduced by the International Institute of Tropical Agriculture (IITA) for other root and tuber crops such cassava and sweet potato in eastern DRC.
This study provided entry points that could address challenges facing yam production sector in eastern DRC to unlock its potential for food sovereignty and wealth creation in the region. Such entry points once implemented would improve food security among local populations, since yam is a potentially high yielding crop (40 to 50 t ha−1) as compared to most cereals and legumes that rarely exceed 2 and 1 t ha−1, respectively, in eastern DRC (Kazige et al., 2022; Agre et al., 2022a). Like in West Africa where yam is already playing key roles in food security (Asiedu and Sartie, 2010), promoting yam in DRC could alleviate hunger, food insecurity, malnutrition, and poverty. Current initiatives seeking to develop breeding programs and a functional seed delivery system are instrumental to ensure varieties suiting farmers within clustered AEZs are developed and delivered to farmers. Consequently, these farmers would experience productivity increase, and thus meeting food security which is a state where all people, always have physical and economic access to sufficient, safe, and nutritious food to meet their dietary needs and food preferences for an active healthy lifestyle (Giller, 2020; Karume et al., 2024). In addition to increasing productivity through improved varieties and farming practices, further efforts should be oriented towards structuring the value chain to ensure farmers are translating yield increase into wealth creation.
4.3 Eastern DRC biophysical factors are suitable to extensive yam production
Eastern DRC is suitable for yam production since prevailing climates, topography, and altitude meet the crop’s requirements. Besides, the most prevalent soil types (Humic Cambisols, Haplic Acrisols, Humic Ferralsols, and Humic Nitisol) are favorable though the region’s CEC and soil acidity would require interventions for better productivity. Another promising aspect is that the region contains several water and evacuation points. This could facilitate irrigation and trade. Similar modeling techniques have proven effective in land suitability mapping, including yam (Akinci et al., 2013; Ayehu and Besufekad, 2015; Mugiyo et al., 2021). The land suitability map generated by the study provides initial information on areas suitable for yam in the event of extensive yam production in South-Kivu. However, the use of satellite images, often with high resolution could lead to slight imprecision in decision-making. Thus, areas identified need inspections at field level prior to yam intensification in South-Kivu. Further studies are needed to elaborate an agricultural calendar for clustered AEZs of eastern DRC to facilitate farming activities’ planning in eastern DRC.
Though used methodology is suitable for such a study, some of the tools employed are subject to criticism. For instance, this study used low resolution satellite images (~5 km for soil and 100 m for climate data) that may not result in accurate mapping. Further improvements are necessary using alternative land suitability analysis approaches based on more powerful statistical methods that could potentially offer a more refined assessment compared to that provided by the AHP (Mokarram et al., 2015; Taghizadeh-Mehrjardi et al., 2020). Although the AHP limitation is widely acknowledged, it continues to be widely utilized due to its ease in delivering convincing results with readily accessible and user-friendly free-to-use images. The AHP-GIS is also valued for its simplicity, avoiding the integration of complex and cumbersome steps found in other approaches (García et al., 2014).
5 Conclusion
This study serves as a baseline information for understanding practices, varietal preferences, and challenges faced by local farmers in yam production areas of the eastern DRC. It also offers an overview of the suitability of South-Kivu’s biophysical parameters for yam production and discusses how yam breeding could strengthen long-term food sovereignty in the region. To arrive at the following conclusions, the study employed a combination of fieldwork and remote sensing:
a. Yam farming practices are still rudimentary and typical of subsistence farming. The seed system is informal, relying mainly on seed exchanges among farmers.
b. Factors limiting yam production and use are mainly the lack of quality seeds, declining soil fertility, youth’s lack of interest in the crop value chain, and above all, inadequate post-harvest storage practices and value addition options. These challenges significantly hinder yam crop as a means of poverty alleviation and food sovereignty in eastern DRC.
c. Effective promotion efforts, seeking to strengthen yam potential for long-term food sovereignty, will only yield high impact if agronomic packages are incorporated along with improved varieties, and market opportunities are increased by linking farmers with potential markets, increased awareness on nutritional and health benefits, and encouraging value-addition to meet the demands of both elites and the increasingly urbanized populations.
d. Most biophysical factors in eastern DRC are suitable for yam, opening an avenue for extensive yam production that is necessary for poverty alleviation and food sovereignty. The generated land suitability map will serve as a decision-support tool to guide yam intensification efforts in South-Kivu. Furthermore, environmental clusters identified in this study will provide valuable insights to optimize yam varietal selection and testing, for implementing an effective yam breeding program.
Findings from this research that promotes extensive yam production could contribute to achieving food sovereignty in DRC as the Congolese climate is suitable to its cultivation and its starchy tubers are already rooted in local diets. This study highlights that unlocking yam full potential for poverty alleviation and food sovereignty requires that improved varieties suiting farmers’ needs, practices, and environments are developed through breeding and complemented by agronomic packages, and institutional supports at all levels.
Data availability statement
The original contributions presented in the study are included in the article/Supplementary material, further inquiries can be directed to the corresponding author.
Author contributions
JM: Conceptualization, Formal analysis, Investigation, Writing – original draft, Writing – review & editing. GC: Data curation, Formal analysis, Methodology, Writing – original draft, Writing – review & editing. HM: Data curation, Investigation, Methodology, Writing – original draft, Writing – review & editing. AB: Investigation, Supervision, Writing – review & editing. JK: Investigation, Methodology, Writing – review & editing. RA: Investigation, Supervision, Writing – review & editing. PAg: Formal analysis, Methodology, Writing – review & editing. VB: Writing – review & editing, Methodology, Validation. PAd: Conceptualization, Funding acquisition, Project administration, Supervision, Writing – review & editing. AA: Conceptualization, Funding acquisition, Methodology, Project administration, Supervision, Validation, Writing – original draft, Writing – review & editing.
Funding
The author(s) declare that financial support was received for the research, authorship, and/or publication of this article. Bill and Melinda Gates Foundation.
Acknowledgments
The funding support from the Bill and Melinda Gates Foundation (BMGF) through the AfricaYam project of the International Institute of Tropical Agriculture (IITA) (INV-003446) is acknowledged. This research benefited also from financial support from the Université Evangélique en Afrique (UEA)’s project on “Improvement of Research and Teaching Quality” (Project A-COD-2023-0035) funded by Pain Pour le Monde (PPM). We are grateful to Fabrice Iragi, Elie Magendo, Pascal Kitungano, Lucien Makiro, and Grace Kwigomba for active participation in surveys.
Conflict of interest
The authors declare that the research was conducted in the absence of any commercial or financial relationships that could be construed as a potential conflict of interest.
Publisher’s note
All claims expressed in this article are solely those of the authors and do not necessarily represent those of their affiliated organizations, or those of the publisher, the editors and the reviewers. Any product that may be evaluated in this article, or claim that may be made by its manufacturer, is not guaranteed or endorsed by the publisher.
Supplementary material
The Supplementary Material for this article can be found online at: https://www.frontiersin.org/articles/10.3389/fsufs.2024.1324646/full#supplementary-material
References
Abdessemed, L., and Escofier, B. (1996). Analyse factorielle multiple de tableaux de fréquences; comparaison avec l'analyse canonique des correspondances. J. Soc. Stat. Paris 137, 3–18.
Aboudou, R., and Auriole, L. (2006). Impact de la croissance urbaine sur les filières agricoles en Afrique de l’Ouest: cas de l’igname à Parakou au Bénin. Ifeas, Lares, document de travail Ecocité 13, 1–51.
Adejumobi, I. I., Agre, P. A., Adeyinka, A. S., Cipriano, I. M., Adheka, J. G., and Onautshu, D. O. (2023). Status of yam (Dioscorea spp.) in the Democratic Republic of Congo. Plant Breed. 13, 1–10. doi: 10.1111/pbr.13123
Adejumobi, I. I., Agre, P. A., Onautshu, D. O., Adheka, J. G., Bambanota, M. G., Monzenga, J. C. L., et al. (2022b). Diversity, trait preferences, management and utilization of yams landraces (Dioscorea species): an orphan crop in DR Congo. Sci. Rep. 12:2252. doi: 10.1038/s41598-022-06265-w
Adejumobi, I. I., Agre, P. A., Onautshu, D. O., Adheka, J. G., Cipriano, I. M., Monzenga, J. C. L., et al. (2022a). Assessment of the yam landraces (Dioscorea spp.) of DR Congo for reactions to pathological diseases, yield potential, and tuber quality characteristics. Agriculture 12:599. doi: 10.3390/agriculture12050599
Adewumi, A. S., Asare, P. A., Adu, M. O., Taah, K. J., Akaba, S., Mondo, J. M., et al. (2021). Farmers’ perceptions on varietal diversity, trait preferences and diversity management of bush yam (Dioscorea praehensilis Benth.) in Ghana. Sci. Afr. 12:e00808. doi: 10.1016/j.sciaf.2021.e00808
Adifon, F., Yabi, I., Balogoun, I., Dossou, J., and Saïdou, A. (2019b). Caractérisation socio-économique des systèmes de culture à base d’igname dans trois zones agro-écologiques pour une gestion durable des terres au Bénin. Eur. Sci. J. 15, 211–232. doi: 10.19044/esj.2019.v15n12p211
Adifon, F. H., Yabi, I., Vissoh, P., Balogoun, I., Dossou, J., and Saïdou, A. (2019a). Écologie, systèmes de culture et utilisations alimentaires des ignames en Afrique tropicale: synthèse bibliographique. Cah. Agric. 28:22. doi: 10.1051/cagri/2019022
Agre, P. A., Dassou, A. G., Loko, L. E., Idossou, R., Dadonougbo, E., Gbaguidi, A., et al. (2021). Diversity of white Guinea yam (Dioscorea rotundata Poir.) cultivars from Benin as revealed by agro-morphological traits and SNP markers. Plant Genet. Resour. 19, 437–446. doi: 10.1017/S1479262121000526
Agre, P. A., Mondo, J. M., Edemodu, A., Matsumoto, R., Asiedu, R., Akoroda, M., et al. (2022a). “Breeding for abiotic stress resistance in yam (Dioscorea spp.) using biotechnology approaches: present practices and prospects” in Genomic designing for abiotic stress resistant technical crops (Cham: Springer International Publishing), 429–457.
Agre, P. A., Mondo, J. M., Edemodu, A., Matsumoto, R., Kolade, O., Kumar, L. P., et al. (2022b). Biotechnology approaches in breeding for biotic stress resistance in yam (Dioscorea spp.). Genomic Design Biotic Stress Resistant Technical Crops 11, 583–616. doi: 10.1007/978-3-031-09293-0_11
Akinci, H., Özalp, A. Y., and Turgut, B. (2013). Agricultural land use suitability analysis using GIS and AHP technique. Comput. Electron. Agric. 97, 71–82. doi: 10.1016/j.compag.2013.07.006
Alabi, T. R., Adebola, P. O., Asfaw, A., De Koeyer, D., Lopez-Montes, A., Asiedu, R., et al. (2019). Spatial multivariate cluster analysis for defining target population of environments in West Africa for yam breeding. International Journal of Applied Geospatial Research (IJAGR), 10, 1–30. doi: 10.4018/IJAGR.2019070104
Asiedu, R., and Sartie, A. (2010). Crops that feed the world 1. Yams: yams for income and food security. Food Secur. 2, 305–315. doi: 10.1007/s12571-010-0085-0
Ayehu, G. T., and Besufekad, S. A. (2015). Land suitability analysis for Rice production: a GIS based multi-criteria decision approach. Amer. J. Geogr. Inf. Syst. 5, 95–104. doi: 10.5923/j.ajgis.20150403.02
Chikwendu, V.E., and Okezie, C.E.A. (2014). Factors responsible for the ennoblement of African yams: inferences from experiments in yam domestication. Foraging and farming (pp. 344–357). Milton Park, Abingdon, Oxfordshire, USA: Routledge
Chuma, G. B., Cirezi, N. C., Mondo, J. M., Mugumaarhahama, Y., Ganza, D. M., Katcho, K., et al. (2021). Suitability for agroforestry implementation around Itombwe natural reserve (RNI), eastern DR Congo: application of the analytical hierarchy process (AHP) approach in geographic information system tool. Trees Forests People 6:100125. doi: 10.1016/j.tfp.2021.100125
Cornet, D. (2015). Influence des premiers stades de croissance sur la variabilité du rendement parcellaire de deux espèces d'igname (Dioscorea spp.) cultivées en Afrique de l'Ouest, PhD Thesis. AgroParisTech, France. Available at: https://agritrop.cirad.fr/575177/
Etikan, I., Alkassim, R., and Abubakar, S. (2016). Comparison of snowball sampling and sequential sampling technique. Biometrics and Biostatistics International Journal, 3, 55. doi: 10.15406/bbij.2015.03.00055
Escofier, B., and Pagès, J. (1991). Presentation of correspondence analysis and multiple correspondence analysis with the help of examples. Applied multivariate analysis in SAR and environmental studies (pp. 1–32). Dordrecht: Springer Netherlands
FAO (1993). Guidelines for land-use planning. FAO development series 1, food and agriculture Organization of the United Nations : Rome, Italy: Soil Resources, Management, and Conservation Service.
FAO . (2023). Food and agriculture Organization of the United Nations. FAOSTAT statistical database. [Rome]: FAO, 2023. Accessed August 2022
García, J. L., Alvarado, A., Blanco, J., Jiménez, E., Maldonado, A. A., and Cortés, G. (2014). Multi-attribute evaluation and selection of sites for agricultural product warehouses based on an analytic hierarchy process. Comput. Electron. Agric. 100, 60–69. doi: 10.1016/j.compag.2013.10.009
Gebrechorkos, S. H., Hülsmann, S., and Bernhofer, C. (2019). Long-term trends in rainfall and temperature using high-resolution climate datasets in East Africa. Sci. Rep. 9:11376. doi: 10.1038/s41598-019-47933-8
Giller, K. E. (2020). The food security conundrum of sub-Saharan Africa. Glob. Food Secur. 26:100431. doi: 10.1016/j.gfs.2020.100431
Igbawua, T., Gbanger, M. H., and Ujoh, F. (2022). Suitability analysis for yam production in Nigeria using satellite and observation data. J. Niger. Soc. Phys. Sci. 4:883. doi: 10.46481/jnsps.2022.883
International Organization for Migration (IOM) . (2022). DTM RDC — Sud Kivu: Tableau de bord - Suivi de mouvements de populations (Avril 2022). IOM, Democratic Republic of the Congo. Available at: https://dtm.iom.int/reports/rdc-sud-kivu-tableau-de-bord-suivi-de-mouvements-de-populations-avril-2022
Kalu, C., Nnabue, I., Edemodu, A., Agre, P. A., Adebola, P., Asfaw, A., et al. (2023). Farmers’ perspective toward a demand led yam breeding in Nigeria. Front. Sustain. Food Syst. 7:1227920. doi: 10.3389/fsufs.2023.1227920
Karume, K., Mondo, J. M., Chuma, G. B., Ibanda, A., Bagula, E. M., Aleke, A. L., et al. (2022). Current practices and prospects of climate-smart agriculture in Democratic Republic of Congo: a review. Land 11:1850. doi: 10.3390/land11101850
Karume, K., Mondo, J.M., and Kiyala, J.C.K. (2024). Drought, the war in Europe and its impacts on food insecurity in sub-Saharan Africa, East Africa. J. C. K. Kiyala and N. Chivasa (eds.), Climate change and socio-political violence in sub-Saharan Africa in the Anthropocene, the Anthropocene: Politik – Economics – Society – Sciences, 37, Springer Nature Switzerland AG
Kazige, O. K., Chuma, G. B., Lusambya, A. S., Mondo, J. M., Balezi, A. Z., Mapatano, S., et al. (2022). Valorizing staple crop residues through mushroom production to improve food security in eastern Democratic Republic of Congo. J. Agric. Food Res. 8:100285. doi: 10.1016/j.jafr.2022.100285
Kiba, D. I., Hgaza, V. K., Aighewi, B., Aké, S., Barjolle, D., Bernet, T., et al. (2020). A transdisciplinary approach for the development of sustainable yam (Dioscorea sp.) production in West Africa. Sustain. For. 12:4016. doi: 10.3390/su12104016
Lewis, J. R., and Erdinç, O. (2017). User experience rating scales with 7, 11, or 101 points: does it matter? J. Usability Stud. 12, 73–91. doi: 10.5555/3122114.3122117
Maass, B. L., Katunga Musale, D., Chiuri, W. L., Gassner, A., and Peters, M. (2012). Challenges and opportunities for smallholder livestock production in post-conflict south Kivu, eastern DR Congo. Trop. Anim. Health Prod. 44, 1221–1232. doi: 10.1007/s11250-011-0061-5
Magwé-Tindo, J., Wieringa, J. J., Sonké, B., Zapfack, L., Vigouroux, Y., Couvreur, T. L., et al. (2018). Guinea yam (Dioscorea spp., Dioscoreaceae) wild relatives identified using whole plastome phylogenetic analyses. Taxon 67, 905–915. doi: 10.12705/675.4
Mausch, K., Almekinders, C. J., Hambloch, C., and McEwan, M. A. (2021). Putting diverse farming households’ preferences and needs at the Centre of seed system development. Outlook Agric. 50, 356–365. doi: 10.1177/00307270211054111
Mokarram, M., Hamzeh, S., Aminzadeh, F., and Zarei, A. R. (2015). Using machine learning for land suitability classification. West Afric. J. Appl. Ecol. 23, 63–73.
Mondo, J. M., Agre, P. A., Chuma, G. B., Asiedu, R., Akoroda, M. O., and Asfaw, A. (2023). Agronomic and hormonal approaches for enhancing flowering intensity in white Guinea yam (Dioscorea rotundata Poir.). Front. Plant Sci. 14:1250771. doi: 10.3389/fpls.2023.1250771
Mondo, J. M., Agre, P. A., Edemodu, A., Adebola, P., Asiedu, R., Akoroda, M. O., et al. (2020). Floral biology and pollination efficiency in yam (Dioscorea spp.). Agriculture 10:560. doi: 10.3390/agriculture10110560
Mondo, J. M., Chuma, B., Kwalya, P. B., Balagizi, A., Ndjadi, S. S., Mugumaarhahama, Y., et al. (2021). Neglected and underutilized crop species in Kabare and Walungu territories, eastern D.R. Congo: identification, uses and socio-economic importance. Agric. Food Res. 6:100234. doi: 10.1016/j.jafr.2021.100234
Mugiyo, H., Chimonyo, V. G. P., Sibanda, M., Kunz, R., Masemola, C. R., Modi, A. T., et al. (2021). Evaluation of land suitability methods with reference to neglected and underutilised crop species: a scoping review. Land 10, 1–24. doi: 10.3390/land10020125
Mugumaarhahama, Y., Mondo, J. M., Cokola, M. C., Ndjadi, S. S., Mutwedu, V. B., Kazamwali, L. M., et al. (2021). Socio-economic drivers of improved sweet potato varieties adoption among smallholder farmers in south-Kivu Province. DR Congo. Sci. Afr. 12:e00818. doi: 10.1016/j.sciaf.2021.e00818
Neina, D. (2021). Ecological and edaphic drivers of yam production in West Africa. Appl. Environ. Soil Sci. 2021, 1–13. doi: 10.1155/2021/5019481
Ngassam, S. B., Malaa, D. K., Ngome, T. P., and Aighewi, A. B. (2009). Analyse diagnostique des circuits de commercialisation de l’igname au Cameroun: atouts et contraintes. Securing Livelihoods through Yams 79–94.
OCHA (2022). Achieving long-term food security in DRC is all about building resilience. Available at: https://reliefweb.int/report/democratic-republic-congo/achieving-long-term-food-security-drc-all-about-building-resilience (Accessed on 8th February 2024)
OIM (2022). is a spelling error. Put IOM to match what is in the Reference list: International Organization for Migration (IOM). (2022). DTM RDC — Sud Kivu: Tableau de bord - Suivi de mouvements de populations (Avril 2022). IOM, Democratic Republic of the Congo. Available at: https://dtm.iom.int/reports/rdc-sud-kivu-tableaude-bord-suivi-de-mouvements-de-populations-avril-2022
Özkan, B., Dengiz, O., and Turan, İ. D. (2020). Site suitability analysis for potential agricultural land with spatial fuzzy multi-criteria decision analysis in regional scale under semi-arid terrestrial ecosystem. Sci. Rep. 10, 22074–22018. doi: 10.1038/s41598-020-79105-4
Pires, A. (1997). Échantillonnage et recherche qualitative: essai théorique et méthodologique. In La recherche qualitative. Enjeux épistémologiques et méthodologiques, Gaëtan Morin, Montréal, Canada, 169, 113. Available at: http://classiques.uqac.ca/
Platts, P. J., Omeny, P., and Marchant, R. (2015). AFRICLIM: high-resolution climate projections for ecological applications in Africa. Afr. J. Ecol. 53, 103–108. doi: 10.1111/aje.12180
R Core Team (2020). R Core team R: A language and environment for statistical computing. Foundation for Statistical Computing. Vienna, Austria. Available at: https://www.r-project.org/
Saaty, T.L. (1980). The analytic hierarchy process: Planning, priority setting, Resource Allocation. McGraw Hill International, New York, NY, USA
Saaty, T. L. (1990). How to make a decision: the analytic hierarchy process. Eur. J. Oper. Res. 48, 9–26. doi: 10.1016/0377-2217(90)90057-I
Saaty, T.L., and Vargas, L.G. (2013). The analytic network process. Decision making with the analytic network process. International series in Operations Research & Management Science, 195. Springer, Boston, MA
Scarcelli, N., Tostain, S., Vigouroux, Y., Agbangla, C., Daïnou, O., and Pham, J. L. (2006). Farmers’ use of wild relative and sexual reproduction in a vegetatively propagated crop. The case of yam in Benin. Molec. Ecol. 15, 2421–2431. doi: 10.1111/j.1365-294X.2006.02958.x
Stuart, E., Asfaw, A., Adebola, P., Maroya, N., Edemodu, A., Adeosun, T., et al. (2021). Yam seed system characteristics in Nigeria: local practices, preferences, and the implications for seed system interventions. Outlook Agric. 50, 455–467. doi: 10.1177/00307270211058209
Taghizadeh-Mehrjardi, R., Nabiollahi, K., Rasoli, L., Kerry, R., and Scholten, T. (2020). Land suitability assessment and agricultural production sustainability using machine-learning models. Agronomy 10:573. doi: 10.3390/agronomy10040573
Thiele, G., Khan, A., Heider, B., Kroschel, J., Harahagazwe, D., Andrade, M., et al. (2017). Roots, tubers and bananas: planning and research for climate resilience. Open Agric. 2, 350–361. doi: 10.1515/opag-2017-0039
Topuz, M., and Deniz, M. (2023). Application of GIS and AHP for land use suitability analysis: case of Demirci district (Turkey). Humanit. Soc. Sci. Commun. 10, 1–15. doi: 10.1057/s41599-023-01609-x
Vlassenroot, K. (2002). Citizenship, identity formation & conflict in south Kivu: the case of the Banyamulenge. Rev. Afr. Polit. Econ. 29, 499–516. doi: 10.1080/03056240208704635
World Bank (2023). Democratic Republic of Congo: overview. Available at: https://www.worldbank.org/en/country/drc/overview (Accessed on 8th February 2024)
Keywords: production factors, land suitability, analytic hierarchy process (AHP), breeding, seed delivery system, food sovereignty
Citation: Mondo JM, Chuma GB, Matiti HM, Balezi AZ, Kihye JB, Ayagirwe RB, Agre PA, Banda VB, Adebola P and Asfaw A (2024) Farming practices, varietal preferences, and land suitability analyses for yam production in Eastern D.R. Congo: implications for breeding initiatives and food sovereignty. Front. Sustain. Food Syst. 8:1324646. doi: 10.3389/fsufs.2024.1324646
Edited by:
Horman Chitonge, University of Cape Town, South AfricaReviewed by:
Adrino Mazenda, University of Pretoria, South AfricaTertsea Igbawua, Federal University of Agriculture Makurdi (FUAM), Nigeria
Copyright © 2024 Mondo, Chuma, Matiti, Balezi, Kihye, Ayagirwe, Agre, Banda, Adebola and Asfaw. This is an open-access article distributed under the terms of the Creative Commons Attribution License (CC BY). The use, distribution or reproduction in other forums is permitted, provided the original author(s) and the copyright owner(s) are credited and that the original publication in this journal is cited, in accordance with accepted academic practice. No use, distribution or reproduction is permitted which does not comply with these terms.
*Correspondence: Jean M. Mondo, bW9uZG8ubXViYWxhbWFAeWFob28uZnI=