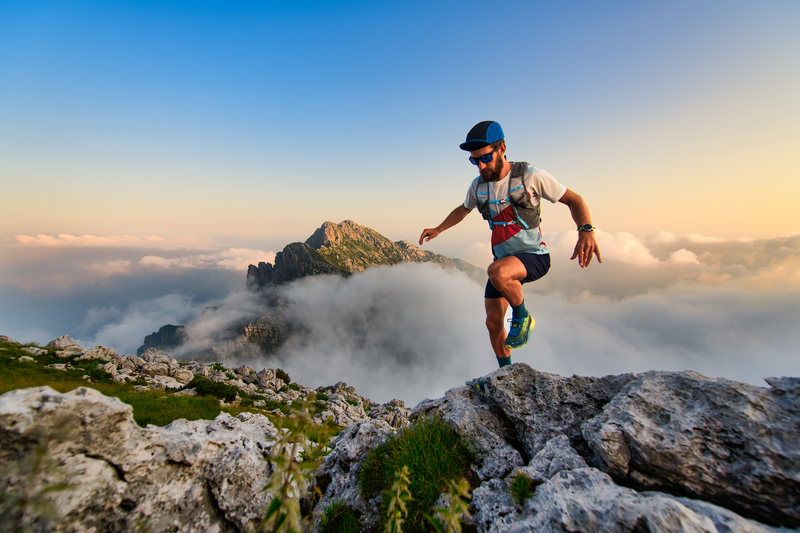
94% of researchers rate our articles as excellent or good
Learn more about the work of our research integrity team to safeguard the quality of each article we publish.
Find out more
ORIGINAL RESEARCH article
Front. Sustain. Food Syst. , 22 February 2024
Sec. Agricultural and Food Economics
Volume 8 - 2024 | https://doi.org/10.3389/fsufs.2024.1307978
Digital agricultural marketing platforms have emerged as one of the key tools for facilitating farmers’ access to markets. While previous studies have predominantly focused on examining factors influencing adoption of these platforms, there remains a gap in understanding factors that determine the proportion of a farmer’s produce marketed through the platforms. This knowledge is crucial for effective scaling up of digital marketing platforms. Consequently, this research aimed to evaluate the extent of commercialization of potato farming through Digital Agricultural Marketing Platforms (DAMPs), with a specific focus on the M-shamba platform in Kenya. To achieve this objective, data was collected in 2022 from a sample of 375 potato farmers from Nakuru County. The data was analyzed with Stata using probit and Tobit models through the conditional mixed process. The findings revealed that total farm income, price per kilogram of output, access to credit, size of the farm dedicated to potato cultivation, and age of the household head influenced the use of M-shamba platform. Further, the extent of commercialization through M-shamba was significantly determined by total livestock units, marketing decision-maker, having potato farming as primary enterprise, farm income, access to credit, membership to agricultural groups, and age of the household head. In light of these findings, the study recommends targeted support and training programs for older farmers to enhance their proficiency in utilizing DAMPs. Moreover, policies and initiatives aimed at increasing farm income should be implemented to assist farmers with limited incomes. Farmers in groups should be encouraged to participate in collective digital marketing efforts.
Agricultural commercialization has emerged as a pivotal approach in the Agricultural Sector Growth and Transformation Strategy (ASGTS) 2019–2029 (Gachuhi et al., 2021) to address food security and poverty alleviation. Nevertheless, small-scale farmers face challenges in participating in markets and boosting their economic prospects due to a lack of dependable market information and limited market access. Additionally, elevated transaction expenses like transportation costs have had adverse effects on farmers’ incomes, compelling them to rely on intermediaries or brokers. Consequently, these intermediaries have historically dictated the prices at which farmers can sell their produce.
However, the digital era has come to the rescue of the farmers by introducing digital platforms to curb various challenges faced by farmers. Digital platforms have emerged as a valuable solution, enabling interactions among multiple users and offering services such as advisory support, financial inclusion, and access to market information and linkages. According to Bolwig et al. (2021), digital platforms that facilitate market access have made the most significant impact within the horticulture sector. Furthermore, Digital Agricultural Marketing Platforms (DAMP) hold the potential to revolutionize small-scale marketing operations, as highlighted by Shakhovskoy and Saab (2021). These DAMP systems are designed with the goal of enhancing the exchange of information among farmers, traders, and consumers while reducing transaction costs, thereby promoting market participation by farmers.
In Kenya, 32 percent of the digital platforms dedicated to agriculture provide market information and linkages (Bolwig et al., 2021). Though there have been many start-up DAMPs, upscaling has been a challenge (Kieti et al., 2021). However, the successful platforms had to understand the local context and the dynamics and tailored the DAMPs to fit farmer’s needs while focusing on specific value chain. This is crucial in reducing the complexity of the system (Bolwig et al., 2021). An example of DAMP that has been successful in Kenya is M-shamba1 which has been operating in potato marketing. DAMPs are meant to enable farmers make their enterprises more profitable by providing the best prices and minimizing transactional costs.
Though the benefits of marketing via the platforms are attractive, limited amounts of produce are marketed via the platforms. Therefore, in order to leverage digital platforms to solve the marketing access issues in the country, it is paramount to keep abreast with their interactions with the farmers and establish the cause for the low marketing trends via the digital platforms. While majority of the studies (Kiarie, 2020; Nwafor et al., 2020; Rabbi et al., 2020; Vasa and Trendov, 2020; Ayim et al., 2022; Zhu et al., 2022) have primarily focused on the determinants of adoption of Information and Communication Technologies (ICTs) in general, this current study narrows down and evaluates the determinants of use of digital marketing platforms. Moreover, the study goes a step further and assesses the socio-economic and institutional factors that influence the quantities farmers market via the platform from their total production.
As the study expands on the available empirical evidence, the findings provide valuable insights to platform developers as they work toward upscaling of digital platforms which according to Kieti et al. (2021) has been a challenge. Further, the findings are instrumental to the government and relevant stakeholders in planning and implementing of policies that facilitate the farmers to reap the benefits of digital platforms thus improving their livelihoods. The remainder of the paper is organized as follows. Section 2 outlines the study’s conceptual framework. Section 3 describes the study area, data, expected signs for the variables used and outlines the empirical strategy. Section 4 presents the results from the summary statistics and the empirical model. Section 5 concludes and provides recommendations.
Farmers’ extent of commercialization is defined by this study as how much a farmer sells out of their total production. This is similar to definitions of Ejeta et al. (2020), Kissoly et al. (2020), Gachuhi et al. (2021), and Thandeka et al. (2022). However, Kyaw et al. (2018), Kondo (2019), and Ayodele et al. (2021) referred to this as intensity of market participation. Intensity of market participation is a broader term that includes farmer’s market-related activities and can be easily confused with participation in different markets. Therefore, this study found extent of commercialization as the suitable term as it provides a clear indication of how much of the farmer’s produce is sold in the market. While Pingali and Rosegrant (1995) emphasized that commercialization encompasses more than just output marketing and includes households participating in the input market, this study focused on defining commercialization specifically as the marketing of output. The extent of commercialization was assessed through the commercialization index which was computed as the proportion of potatoes sold via the digital platform out of the farmers’ total potato production.
Farmers commercialize when the institutional environment is favorable and there are new market opportunities (Jaleta et al., 2009). The digital platforms provide farmers with new market opportunities and thus serve as an incentive for farmers to commercialize. Further commercialization is different based on different socio-economic and institutional environments. Therefore, multiple factors come into play when a farmer decides to market via DAMPs. The study thus conceptualized the various factors that determined the farmers’ use of the digital platforms as well as their extent of commercialization via the platform as shown in Figure 1.
Figure 1. Conceptual framework showing interrelationships between different factors, use of DAMPs and the extent of commercialization. Source: Author’s conceptualization.
Hypothesized socio-economic characteristics included age of household head, years of formal education of the household head, household’s head main occupation, having potato farming as main enterprise, marketing decision-maker, total livestock unit (TLU) and overall farm income. Further, farm characteristics included farm size under potato production and the total land owned while the external support factors were as group membership, access to extension and access to credit. Lastly, platform’s attributes considered was price offered by the platform per kilogram of potatoes. All these factors were jointly conceptualized to influence the use of DAMP and how much out of the total potato production was marketed via the platform.
The research was carried out in Nakuru County, one of the leading counties in potato production and advancement of digital agriculture in Kenya. The county is situated in the Rift Valley region of Kenya and spans a total area of 7,495.1 square kilometers, with 5,274 square kilometers designated as arable land. Approximately 70% of this land is utilized for agricultural activities, as reported by the National Potato Council of Kenya (2022). Majority of households in Nakuru County depend on agriculture as their primary source of income, with the Irish potato, being the most extensively cultivated crop in the county (National Potato Council of Kenya, 2022). In particular, the farmers in this study were primarily found to grow and market the shangi variety of potatoes.
Data collection occurred during the period from February to April 2022, using a multistage sampling approach. In the initial stage, Nakuru County was purposively selected based on predetermined criteria (Nakuru County is a leading advocate for digital agriculture in Kenya and is also among the leading counties in potato production). Subsequently, in the second stage, nine cooperatives (Nyota, MOKUNS, New Molo, Golden Valley, Starlight, Green Planet, Kitcher, Kamasis, and Mau Narok) located within the same agro-ecological zones were purposively chosen. In the third and final stage, farmers were systematically chosen from lists provided by these cooperatives. The sample size was determined using the Cochran formula (Cochran, 1977), resulting in a sample size of 384. To select participants, every 8th member was identified from the farmers’ list per cooperative. The number 8 was determined by dividing the total number of farmers across all nine cooperatives (850) by the sample size of 384. The number of farmers sampled per cooperative was in proportion to the total number of members in the cooperative.
The survey involved the use of a semi-structured questionnaire to collect primary data with reference to the preceding potato production season (July–December 2021). Due to non-response and incomplete questionnaires, data analysis utilized responses from 375 farmers. Prior to conducting the interviews, informed consent was obtained from all participating farmers, and the principles of anonymity and voluntary participation were strictly adhered to. Data pertaining to household characteristics, potato production, and marketing activities were gathered.
Two steps were used for the analysis. In the first step, a Probit model was used to separate the marketing platform users from non-users. In the second step, a Tobit model was used since the dependent variable was bounded between 0 and 1. The extent of commercialization in terms of what the farmers sell via the platform out of what they produce ( ) was used as the proxy for the extent of commercialization. Conditional mixed process (cmp) in STATA was used to estimate the two models as they were deemed to have hierarchical random effects.
The probability of a household being a user of DAMP for the sale of potatoes was given by selection (Eq. 1).
Where ⁓ N [0,1] Dummy variable takes the value of 1 if a household is a user of DAMP and 0 otherwise; Vector parameter; Parameter to be estimated in the model; Error terms that are normally distributed with a mean of zero, unit variance and correlation coefficient ρ.
The Tobit model is as described by Eqs. 2a and 2b.
where, was a latent variable representing the proportion of potatoes sold via the DAMP out of the total production. This value of output was observed if and unobservable otherwise. captured the vector of independent variables affecting a household’s extent of commercialization via the digital platform. was a vector of parameters estimated and was the disturbance term assumed to be independently and normally distributed. Some variables are included and others excluded from the Probit and Tobit depending on their direct correlation with the dependent variables in the models.
The hypothesized signs on how various factors are likely to influence a farmer’s extent of commercialization via digital platforms are as shown in Table 1. Age is usually considered as a proxy for farming experience in various studies (Dessale, 2019; Marechera et al., 2019; Anum et al., 2022) and so was in this study. It was expected that age improves a farmer’s extent of commercialization due to marketing experience of a cash crop like potato. However, since the potato marketing involved the use of digital technology, age was also expected to have a negative influence on the extent of marketing via the platform as older farmers are less inclined toward the use of digital technology. Who decides to market was expected to positively influence the use of the marketing platform, considering that joint marketing decisions have been efficient in determining the ideal market (Gebre et al., 2021). This was a categorical variable and the household head was used as the base variable.
Various studies (Ariane, 2012; Mittal and Mehar, 2012) have shown years of formal education to have a positive influence on the use of new technologies in agriculture. Thus, it was expected that years of formal education of the household head positively influences their extent of commercialization. Household head having farming as their main occupation was hypothesized to have a positive influence how much potatoes they marketed via the digital platform. It was expected that a farmer who has potato farming as their main enterprise and those with a large area under potatoes would drive the farmer to sell more of their potatoes via digital platform. This would be attributed to platforms offering higher price so farmers aim to maximize their profits.
Larger farms were anticipated to embrace DAMP to a greater extent compared to smaller farms. This expectation arises from the perception that larger farms typically possess higher incomes, enabling them to invest in new technologies (Barnes et al., 2019). Additionally, these larger farms tend to produce more, leading to an increased volume of products available for sale through digital platforms. Further, it was hypothesized that total farm income would also positively influence farmers’ participation in digital platforms. A greater income from farming equips farmers with more capital (Biru et al., 2020), which can be allocated toward the acquisition of necessary tools such as phones and airtime, as well as seeking information about the advantages of utilizing marketing platforms.
According to Alene et al. (2008), higher commodity prices typically serve as incentives for farmers to increase their sales in the market. Consequently, in the context of this study, it was anticipated that the prices offered for potatoes through the platform would positively influence the extent of commercialization. TLU which was taken as a proxy for wealth in terms of tropical livestock unit (TLU) (Yao and Shanoyan, 2019; Biru et al., 2020) was hypothesized to have either positive or negative influence on extent of commercialization. A positive influence could be attributed to households with higher TLU being able to sell livestock and utilize the income for various purposes, including production, labor hiring, pesticide procurement, and leveraging manure for increased yield and marketable surplus (Alene et al., 2008; Zamasiya et al., 2014; Gani and Hossain, 2015). Conversely, a negative influence could be linked to resource division within households (Tura et al., 2016).
Farmers who have access to extension services tend to be more inclined to embrace new ICT tools, as they regularly receive information and training on emerging technologies (Diaz et al., 2021; Wu, 2022). As a result, this research posited that having access to extension services would likely have a favorable influence on the level of market involvement. Furthermore, it was anticipated that access to credit would be positively linked to the extent of a farmer’s market participation via the digital platform. Being a member of a group typically represents a form of social capital, and it is within these group meetings and seminars that farmers often become aware of and acquire knowledge about the latest technologies. Consequently, this study expected that group membership would positively affect the extent of commercialization via DAMP through reduction of transaction costs of dissemination of information and knowledge.
In this study, the DAMP users are classified as farmers that used M-shamba for marketing their potatoes while the DAMP non-users are those that did not use the digital platform for marketing. Summary statistics and statistical significance tests on equality of means for continuous variables and equality of proportions for binary variables for the DAMP users and non-users are as presented in Table 2. From the results, farmers that used the digital marketing platform received significantly higher prices per kilogram of the potatoes.
Further DAMP users had significantly higher farm income than the non-users. Perhaps this could be attributed to the better prices they received from the potato enterprise as part of their farm income. Moreover, farmers with higher incomes also have enough capital to purchase mobile phones to access the digital platforms. There was a significant difference in the age of the DAMP users and non-users. The results indicate that the users were significantly younger than the non-users. According to literature (S4YE, 2021; Masi et al., 2022; Tauzie et al., 2023), farmers who are young tend to adopt the use of digital platforms more easily.
The results from the two-step model are as shown in Table 3. The model’s chi-square was significant at 1% indicating the strong influence of the significant independent variables on the farmers’ extent of commercialization. Market participation is pegged on use of the platform, thus, the factors that influence the use were total farm income, output price per kg, access to credit, farm size under potatoes and the age of the household head. The significant determinants of extent of commercialization were, TLU, marketing decision-maker, having potato farming as main enterprise, total farm income, access to credit, group membership and age of the household head.
Total farm income had a positive significant influence on the use of M-shamba for potato marketing. As outlined by Zhu et al. (2022), farmers with higher incomes tend to be more predisposed to using new technologies, given their access to capital, enabling them to acquire information about the technologies and the technologies themselves. Digital marketing platforms are mostly developed to shield the farmers from exploitation by the middle-men by offering better prices [Alliance for a Green Revolution in Africa (AGRA), 2016]. The higher prices provided act as incentives for farmers (Alene et al., 2008) thus they use the platforms for marketing. This was evident in the results from this study that indicated that output price per kg had a positive significant influence on the use of DAMP.
Contrary to the expectation that access to credit would have a positive influence on the use of M-shamba for potato marketing, the study found that it had a significant negative influence on the use of the platform. Similar findings were reported by Mdoda and Mdiya (2022) in their study on factors influencing the use information and communication technologies. The farm size under potato was found to have a negative significant influence on use of the marketing platform. This could be attributed to farmers with larger farms under potato farming having already established markets for their potatoes. Age had a significant negative influence on the use of DAMP. Young farmers are more exuberant about new technologies and usually take risks to try the new innovation (Diaz et al., 2021).
TLU was found to have a negative significant influence on extent of commercialization through DAMP. This can be attributed to farmers having more concentration on livestock production rather than potato production thus having little to market via the platform. According to Rabbi et al. (2019), livestock ownership negatively affects participation in crop market. The findings indicate that in comparison to the marketing decision made by the household heads, the marketing decision made by spouse and joint increases the extent of commercialization via the marketing platforms. According to Mutimura et al. (2018) and Keenan et al. (2021), farmers make joint decisions when it comes to use of new technologies and marketing decisions. Further, females are known to get comfortable when using digital platforms and twice as many women as men prefer digital platforms for marketing (Fiocco et al., 2021).
Having potato farming as the main enterprise was found to positively influence extent of commercialization. This finding can be explained by the fact that farmers with potato as their main enterprise allocate inputs and labor adequately thus have higher production and consequently have more to market. Moreover, they are in search of the best prices to fetch higher incomes thus market through platforms where the prices are high. Similar findings were reported by Sekyi et al. (2021). Total farm income had a positive significant influence which can be attributed to the fact that incomes from other enterprises can provide capital to the potato enterprise to increase production and thus have more to market. Murathi (2018) reported similar findings on influence of farm income on extent of commercialization. Moreover, it can provide capital to access information on new technologies such as marketing platforms where the farmers can market their produce.
Access to credit negatively affected extent of potato commercialization through the digital platforms. Kahenge et al. (2019) reported that access to credit had negative effect on level of soya bean commercialization. Murathi (2018) found a negative influence of access to credit on smallholder farmers’ commercialization which he attributed to limited supply of credit facilities. The negative effect on how much the farmers sell through DAMP could be attributed to the need for quick income offered by middlemen at farm gate so as to clear the credit debt. The study found further that group membership had a negative significant influence on the fraction sold via DAMP contrary to most studies such as Ayuya et al. (2021). This finding can be attributed to the fact that most smallholder farmers in groups aggregate their produce to sell to specific markets thus do not market via DAMP.
Similar to some studies (Kissoly et al., 2020; Ayuya et al., 2021) age was found to significantly affect extent of commercialization through the digital platforms negatively. Older farmers are known to have established trust to various marketing channel thus they shy away from emerging marketing channels such as digital platforms. Moreover, older farmers have a high risk aversion hence are more reserved when it comes to the quantity they market through DAMP. Further, digitalization requires digital literacy, skills and knowledge (Abdulai, 2022), which majority of the older farmers lack.
This study aimed to elucidate the potential factors influencing the extent of commercialization through the digital marketing platform, M-shamba. The findings from the probit model, which was employed to explore why M-shamba is used, revealed that the output per kilogram and the total farm income are key factors that drive usage. Conversely, farm size allocated to potato cultivation, access to credit, and the age of the household head were associated with a negative influence on DAMP use. The results for the assessment of the extent of commercialization revealed that the marketing decision-maker, prioritizing potato farming as the primary enterprise, and the total farm income positively contributed to the extent of commercialization through DAMP. Contrariwise, total livestock units (TLU), access to credit, group membership and the age of the household head had negative influence on the extent of potato commercialization through DAMP.
The insights from this study serve as a valuable resource for policymakers, agricultural stakeholders, and farmers looking to optimize their engagement with DAMPs, ultimately enhancing their market participation. Based on the findings, it is evident that higher incomes empower farmers with the financial capacity to invest in technology and engage more actively with digital marketing platforms. Consequently, the study recommends that the government and relevant stakeholders such as, NGOs, and private investors, implement income support programs aimed at increasing farm incomes such as subsidies, grants and incentives. Although no one guarantees that the farmers will use the income to invest in technology, it is assumed that the farmers are demand driven and will use the income to acquire the new technologies.
Further, it recommends that stakeholders such as the cooperatives and developers of DAMPs direct their support toward older farmers, equipping them with digital literacy training to proficiently grasp DAMPs and effectively utilize these marketing platforms. Additionally, it is crucial for farmers in groups to be encouraged on collective marketing via the digital platforms considering the platforms offer better prices and thus will have higher incomes. Considering the positive influence of prioritizing potato farming as the main enterprise on the extent of commercialization through DAMPs, the study recommends that the government [through organizations such as Kenya Agricultural and Livestock Research Organization (KARLO) and National Potato Council of Kenya] and relevant stakeholders [such as the International Potato Center (CIP)] implement programs and incentives such as access to quality seeds and fertilizers, and technical support to encourage farmers to specialize in specific high-value crops like potatoes.
This study contributes to the academic discourse by offering empirical evidence on the factors influencing the use and extent of commercialization through digital agricultural marketing platforms. The findings provide a foundation for future research exploring the intersection of technology and agriculture in respect to marketing. To advance the understanding of digital marketing platforms in agriculture, future research should explore the dynamics of farmers behavior on digital marketing platforms, exploring the reasons behind the observed influences and identifying strategies to mitigate barriers to adoption. Additionally, investigating the role of social networks and community engagement in shaping farmers’ decisions to commercialize through DAMPs could provide a richer understanding of the social aspects influencing digital agricultural marketing.
Although the study has addressed an important gap in the literature by providing empirical evidence of the determinants of extent of commercialization via digital agriculture marketing platforms, the results should be interpreted with the following considerations in mind. First, the potato value chain may not represent other agricultural value chains, limiting the generalization of results to include other value chains. Second, the study focused on one platform (M-shamba), and including more than one digital marketing platform could provide a more accurate assessment of the determinants of access and utilization of digital agriculture marketing platforms. Moreover, the study did not consider the gender aspect concerning the extent of commercialization. Therefore, the study suggests that academics conduct further research to integrate the gender perspective.
The raw data supporting the conclusions of this article will be made available by the authors, without undue reservation.
Ethical review and approval was not required for the study on human participants in accordance with the local legislation and institutional requirements. Written informed consent from the participants or participants legal guardian was not required to participate in this study in accordance with the national legislation and the institutional requirements.
FM: Conceptualization, Formal analysis, Investigation, Methodology, Writing – original draft, Writing – review & editing, Data curation. JM: Conceptualization, Supervision, Writing – review & editing. HN: Conceptualization, Supervision, Writing – review & editing.
The author(s) declare financial support was received for the field research. This research was supported by the Kenya Climate Smart Agricultural Project (KCSAP), funded jointly by the Government of Kenya and the World Bank.
The authors acknowledge the financial support of KSCAP and the support, and participation of the Nakuru Potato Union officials, the M-shamba platform developer, the potato cooperatives and the enumerators in this study.
The authors declare that the research was conducted in the absence of any commercial or financial relationships that could be construed as a potential conflict of interest.
All claims expressed in this article are solely those of the authors and do not necessarily represent those of their affiliated organizations, or those of the publisher, the editors and the reviewers. Any product that may be evaluated in this article, or claim that may be made by its manufacturer, is not guaranteed or endorsed by the publisher.
1. ^M-shamba was used as a representative of the successful DAMPs in Kenya in this current study.
Abdulai, A. R. (2022). Toward digitalization futures in smallholder farming systems in sub-Sahara Africa: a social practice proposal. Front. Sustain. Food Syst. 6, 58–64. doi: 10.3389/fsufs.2022.866331
Alene, A. D., Manyong, V. M., Omanya, G., Mignouna, H. D., Bokanga, M., and Odhiambo, G. (2008). Smallholder market participation under transactions costs: maize supply and fertilizer demand in Kenya. Food Policy 33, 318–328. doi: 10.1016/j.foodpol.2007.12.001
Alliance for a Green Revolution in Africa (AGRA) (2016). Digital harvest (issue October). Available at: https://agra.org/wp-content/uploads/2020/10/Digital-Harvest-Final-Report.pdf
Anum, R., Ankrah, D. A., and Anaglo, J. N. (2022). Influence of demographic characteristics and social network on peri-urban smallholder farmers adaptation strategies – evidence from southern Ghana. Cogent Food Agric. 8, 15–16. doi: 10.1080/23311932.2022.2130969
Ariane, Z. (2012). Assessment of adoption and impact of rainwater harvesting technologies on rural FARM household income; the CASE of rainwater harvesting ponds in RWANDA.
Ayim, C., Kassahun, A., Addison, C., and Tekinerdogan, B. (2022). Adoption of ICT innovations in the agriculture sector in Africa: a review of the literature. Agric. Food Secur. 11, 1–16. doi: 10.1186/s40066-022-00364-7
Ayodele, O. O., Aboaba, K. O., Oladeji, S. O., and Tolorunju, E. T. (2021). Factors affecting productivity and intensity of market participation of leafy vegetable growers. Int. J. Veg. Sci. 27, 96–101. doi: 10.1080/19315260.2020.1718820
Ayuya, O. I., Adongo, S., Wanjohi, L., and Rajendran, S. (2021). Role of digital platforms in maintaining the value chains of vegetable, root and tuber crops functional in the face of Covid-19.
Barnes, A., De Soto, I., Eory, V., Beck, B., Balafoutis, A., Sánchez, B., et al. (2019). Influencing factors and incentives on the intention to adopt precision agricultural technologies within arable farming systems. Environ. Sci. Policy 93, 66–74. doi: 10.1016/j.envsci.2018.12.014
Biru, W. D., Zeller, M., and Loos, T. K. (2020). The impact of agricultural technologies on poverty and vulnerability of smallholders in Ethiopia: a panel data analysis. Soc. Indic. Res. 147, 517–544. doi: 10.1007/s11205-019-02166-0
Bolwig, S., Haselip, J., Strange, L., Hornum, S. T., and Pedersen, M. B. (2021). Digital solutions for agricultural value chains in Kenya: the role of private-sector actors. Available at: https://unepdtu.org/project/strengthening-value-chains-and-capacities-for-expanding-clean-energy-markets-in-kenya-and-uganda/
Dessale, M. (2019). Analysis of technical efficiency of small holder wheat-growing farmers of Jamma district, Ethiopia. Agric. Food Secur. 8, 1–8. doi: 10.1186/s40066-018-0250-9
Diaz, A. C., Sasaki, N., Tsusaka, T. W., and Szabo, S. (2021). Factors affecting farmers’ willingness to adopt a mobile app in the marketing of bamboo products. Resour. Conserv. Recycl. Adv. 11:200056. doi: 10.1016/j.rcradv.2021.200056
Ejeta, B., Ermias, D., and Ashetu, G. (2020). Determinants of smallholder commercialization of tomato crop in Siltie zone, Southern Ethiopia. Agric. For. Fish. 9, 163–173. doi: 10.11648/j.aff.20200906.12
Fiocco, D., Ganesan, V., Harrison, L., and Pawlowski, J. (2021). Farmers value digital engagement, but want suppliers to step up their game (issue April).
Gachuhi, M. W., Owour, G., and Gathungu, E. (2021). Determinants of intensity of soybean commercialization among smallholder farmers in Butere, Kenya. J. Agric. Appl. Econ. 24, 101–111. doi: 10.15414/raae.2021.24.02.101-111
Gani, O., and Hossain, E. (2015). Market participation decision of smallholder farmers and its determinants in Bangladesh. Ekonomika Poljoprivrede 62, 163–179. doi: 10.5937/ekopolj1501163g
Gebre, G. G., Isoda, H., Amekawa, Y., Rahut, D. B., Nomura, H., and Watanabe, T. (2021). Marketing efficiency among gender-based decision-making farm households in southern Ethiopia. J. Int. Food Agribusiness Mark. 34, 538–563. doi: 10.1080/08974438.2021.1911906
Jaleta, M., Gebremedhi, B., and Hoekstra, D. (2009). Smallholder commercialization: processes, determinants and impact. In Improving productivity and market success (IPMS) of Ethiopian farmers project (no. 18; Vol. 147, issue 16).
Kahenge, Z., Muendo, K., and Nhamo, N. (2019). Factors influencing crop commercialization among soybeans. Jomo Kenyatta Univ. Agric. Technol. 19, 13–31.
Keenan, M., Karanja, S., Pamuk, H., and Ruben, R. (2021). Smallholder Farming Households’ Make-or-Buy Decisions: Linking Market Access, Production Risks, and Production Diversity to Dietary Diversity Smallholder Farming Households’ Make-or-Buy Decisions: Linking Market Access, Production Risks, and Prod (Issue 360).
Kiarie, H. (2020). Determinants of digital technologies adoption among small scale farmers in Kenya-a case of Embu and Kirinyaga counties. Available at: http://hdl.handle.net/11071/10422
Kieti, J., Waema, T. M., Ndemo, E. B., Omwansa, T. K., and Baumüller, H. (2021). Sources of value creation in aggregator platforms for digital services in agriculture – insights from likely users in Kenya. Digital Bus. 1:100007. doi: 10.1016/j.digbus.2021.100007
Kissoly, L., Fasse, A., and Ulrike, G. (2020). Intensity of commercialization and the dimensions of food security: the case of smallholder farmers in rural Tanzania. J. Agribusiness Dev. Emerg. Econ. 10, 731–750. doi: 10.1108/JADEE-06-2019-0088
Kondo, E. (2019). Market participation intensity effect on productivity of smallholder cowpea farmers: evidence from the northern region of Ghana. Rev. Agric. Appl. Econ. 22, 14–23. doi: 10.15414/raae.2019.22.01.14-23
Kyaw, N. N., Ahn, S., and Lee, S. H. (2018). Analysis of the factors influencing market participation among smallholder rice farmers in Magway Region, central dry zone of Myanmar. Sustainability (Switzerland) 10, 7–9. doi: 10.3390/su10124441
Marechera, G., Macharia, I., Muinga, G., Mugo, S., Rotich, R., Oniang, K., et al. (2019). Duration analysis of drought TEGO hybrid maize adoption in Kenya. Agric. Sci. Res. J. 9, 48–60. Available at: https://www.aatf-africa.org/wp-content/uploads/2019/08/George-Marechera-et-al-July-2019.pdf
Masi, M., De Rosa, M., Vecchio, Y., Bartoli, L., and Adinolfi, F. (2022). The long way to innovation adoption: insights from precision agriculture. Agric. Food Econ. 10, 1–17. doi: 10.1186/s40100-022-00236-5
Mdoda, L., and Mdiya, L. (2022). Factors affecting the using information and communication technologies (ICTs) by livestock farmers in the eastern cape province. Cogent Soc. Sci. 8, 1–13. doi: 10.1080/23311886.2022.2026017
Mittal, S., and Mehar, M. (2012). How mobile phones contribute to growth of small farmers? Evidence from India. Q. J. Int. Agri. 51, 227–244.
Murathi, K. N. (2018). Determinants and welfare effect of smallholder farmers’ commercialization in Kenya.
Mutimura, M., Guthiga, P., Haug, R., Dechassa, N., Ketema, M., Hundessa, F., et al. (2018). Socio-economic status affecting smallholder farming and food security: A study from six case countries in Africa (issue 727201).
National Potato Council of Kenya (2022). Nakuru County. Available at: https://npck.org/nakuru-4/
Nwafor, C. U., Ogundeji, A. A., and van der Westhuizen, C. (2020). Adoption of ICT-based information sources and market participation among smallholder livestock farmers in South Africa. Agriculture (Switzerland) 10, 2–9. doi: 10.3390/agriculture10020044
Pingali, P. L., and Rosegrant, M. W. (1995). Agricultural commercialization and diversification: processes and policies. Food Policy 20, 171–185. doi: 10.1016/0306-9192(95)00012-4
Rabbi, F., Ahamad, R., Ali, S., Ali, A., Ahmad, W., and Ilyas, A. (2019). Determinants of commercialization and its impact on the welfare of smallholder rice farmers by using Heckman’ s two-stage approach. J. Saudi Soc. Agric. Sci. 18, 224–233. doi: 10.1016/j.jssas.2017.06.001
Rabbi, F., Idrees, M., Ali, S., Zamin, M., and Bilal, H. (2020). Farmers’ perceptions and adoption of information and communication technologies (ICTs) in peach (Prunus persica L.) production and marketing. Sarhad J. Agric. 36, 882–893. doi: 10.17582/journal.sja/2020/36.3.882.893
S4YE (2021). Digital Solutions for Youth Agripreneurs. World Bank Group, April. Available at: https://www.consilium.europa.eu/media/23551/2017-g20-rural-youth-employment-en.pdf
Sekyi, S., Quaidoo, C., and Wiafe, E. A. (2021). Does crop specialization improve agricultural productivity and commercialization? Insight from the northern Savannah ecological zone of Ghana. J. Agribusiness Dev. Emerg. Econ. 13, 16–35. doi: 10.1108/JADEE-01-2021-0021
Shakhovskoy, M., and Saab, W. (2021). Agricultural “Platforms” in a digital era: defining the landscape. Available at: https://isfadvisors.org/wp-content/uploads/2021/03/ISF_RAFLL_Agricultural_Platforms_Report.pdf
Tauzie, M., Hermans, T. D. G., and Whitfield, S. (2023). The new achikumbe elite: food systems transformation in the context of digital platforms use in agriculture in Malawi. Agric. Hum. Values. 2–13. doi: 10.1007/s10460-023-10494-8
Thandeka, N., Zondi, B., Simon, M., and Ngidi, C. (2022). Factors influencing the extent of the commercialization of indigenous crops among smallholder farmers in the Limpopo and Mpumalanga provinces of South Africa. Front. Sustain. Food Syst. 5, 1–11. doi: 10.3389/fsufs.2021.777790
Tura, E. G., Goshu, D., Demisie, T., and Kenea, T. (2016). Determinants of market participation and intensity of marketed surplus of teff producers in Bacho and Dawo districts of Oromia state, Ethiopia. J. Agric. Econ. Dev. 5, 20–32. Available at: http://academeresearchjournals.org/journal/jaed
Vasa, L., and Trendov, N. (2020). Farmers’ experience in adoption and usage of ICT solutions for agriculture in the republic of Macedonia. Appl. Stud. Agribusiness Commer. 14, 25–30. doi: 10.19041/apstract/2020/2-3/3
Wu, F. (2022). Adoption and income effects of new agricultural technology on family farms in China. PLoS ONE 17, 1–19. doi: 10.1371/journal.pone.0267101
Yao, B., and Shanoyan, A. (2019). The impact of Mobile money on smallholder producer resilience: evidence from Kenya. Agricultural & Applied Economics Association Annual Meeting.
Zamasiya, B., Mango, N., Nyikahadzoi, K., and Siziba, S. (2014). Determinants of soybean market participation by smallholder farmers in Zimbabwe. J. Dev. Agric. Econ. 6, 49–58. doi: 10.5897/jdae2013.0446
Keywords: commercialization, platforms, digitalization, marketing, extent
Citation: Maina F, Mburu J and Nyang’anga H (2024) Drivers of extent of commercialization of potato farming through digital marketing platforms in Nakuru County, Kenya. Front. Sustain. Food Syst. 8:1307978. doi: 10.3389/fsufs.2024.1307978
Received: 05 October 2023; Accepted: 12 February 2024;
Published: 22 February 2024.
Edited by:
Josef Abrham, Czech University of Life Sciences Prague, CzechiaReviewed by:
Siphe Zantsi, Agricultural Research Council of South Africa (ARC-SA), South AfricaCopyright © 2024 Maina, Mburu and Nyang’anga. This is an open-access article distributed under the terms of the Creative Commons Attribution License (CC BY). The use, distribution or reproduction in other forums is permitted, provided the original author(s) and the copyright owner(s) are credited and that the original publication in this journal is cited, in accordance with accepted academic practice. No use, distribution or reproduction is permitted which does not comply with these terms.
*Correspondence: Florence Maina, ZmxvZmxvbWFpbmFAZ21haWwuY29t
Disclaimer: All claims expressed in this article are solely those of the authors and do not necessarily represent those of their affiliated organizations, or those of the publisher, the editors and the reviewers. Any product that may be evaluated in this article or claim that may be made by its manufacturer is not guaranteed or endorsed by the publisher.
Research integrity at Frontiers
Learn more about the work of our research integrity team to safeguard the quality of each article we publish.