- 1Division of Genetics, ICAR-Indian Agricultural Research Institute, New Delhi, India
- 2Department of Seed Science and Technology, Acharya Narendra Deva University of Agriculture & Technology, Ayodhya, India
- 3Division of Plant Physiology, ICAR-Indian Agricultural Research Institute, New Delhi, India
- 4Rice Breeding and Genetics Research Centre, Aduthurai, Tamil Nadu, India
Introduction: Toxicity due to excess soil iron (Fe) is a significant concern for rice cultivation in lowland areas with acidic soils. Toxic levels of Fe adversely affect plant growth by disrupting the absorption of essential macronutrients, and by causing cellular damage. To understand the responses to excess Fe, particularly on seedling root system, this study evaluated rice genotypes under varying Fe levels.
Methods: Sixteen diverse rice genotypes were hydroponically screened under induced Fe levels, ranging from normal to excess. Morphological and root system characteristics were observed. The onset of leaf bronzing was monitored to identify the toxic response to the excess Fe. Additionally, agronomic and root characteristics were measured to classify genotypes into tolerant and sensitive categories by computing a response stability index.
Results: Our results revealed that 460 ppm of Fe in the nutrient solution served as a critical threshold for screening genotypes during the seedling stage. Fe toxicity significantly affected root system traits, emphasizing the consequential impact on aerial biomass and nutrient deprivation. To classify genotypes into tolerant and sensitive categories, leaf bronzing score was used as a major indicator of Fe stress. However, the response stability index provided a robust basis for classification for the growth performance. Apart from the established tolerant varieties, we could identify a previously unrecognized tolerant variety, ILS 12–5 in this study. Some of the popular mega varieties, including BPT 5204 and Pusa 44, were found to be highly sensitive.
Discussion: Our findings suggest that root system damage, particularly in root length, surface area, and root volume, is the key factor contributing to the sensitivity responses under Fe toxicity. Tolerant genotypes were found to retain more healthy roots than the sensitive ones. Fe exclusion, by reducing Fe2+ uptake, may be a major mechanism for tolerance among these genotypes. Further field evaluations are necessary to confirm the behavior of identified tolerant and sensitive lines under natural conditions. Insights from the study provide potential scope for enhancement of tolerance through breeding programs as well as throw light on the role root system in conferring tolerance.
1 Introduction
Rice serves as a fundamental dietary staple for approximately 3.5 billion people globally, contributing to 23% of the worldwide per capita energy and 16% of protein intake. To accommodate the rising food needs due to the swelling global population from 2000 to 2050, projections suggest that rice production should increase by 35% between 2000 and 2050 (Jaggard et al., 2010; Timmer et al., 2010; Singh et al., 2022). Despite continuous research in past years, there has been limited noticeable genetic improvement in rice yields, indicating a saturation of yield potential in certain favorable environments (Mahender et al., 2019).
Rice yields are susceptible to a range of biotic and abiotic stresses. These stresses may operate in isolation or synergistically, with their impacts fluctuating based on local environmental conditions and the specific rice variety cultivated (Zhang et al., 2013; Ma et al., 2015; Sikirou et al., 2015; Mongon et al., 2017; Kobayashi et al., 2018). Abiotic stresses including salinity, drought, temperature extremes, nutrient imbalances, soil acidity, and ion toxicity are recognized to markedly affect rice grain yields. In acidic soils, a surplus of iron (Fe) is commonly observed, exerting a detrimental effect on the assimilation of essential nutrients, notably phosphorus and potassium (Saleem et al., 2023). Given the anticipated increase in these stress factors due to climate shifts, there is a consensus on the imperative need for cultivating rice varieties, which is resilient to multiple stresses, especially considering unforeseen future challenges. Significant strides in research are made, leading to the development of rice genotypes tolerant to several biotic and abiotic stresses across various ecosystems. However, the challenge of addressing iron (Fe) toxicity in rice remains a critical area that demands intensified and concentrated research efforts (Kar and Panda, 2020).
Fe is a crucial microelement required by all plants for their proper growth and development. It is fundamental to the structure and function of vital biomolecules, such as iron–sulfur (FeS) proteins, ferredoxins, heme proteins, and numerous other cofactors. Its pivotal role encompasses processes such as chlorophyll production and maturation, photosynthesis, and mitochondrial functions. The redox characteristics of Fe render it indispensable, underscoring its irreplaceable nature in biological mechanisms (Marschner and Römheld, 1995; Robinson et al., 1999; Waters and Eide, 2002; Rout et al., 2014; Müller et al., 2015; Bashir et al., 2017; Connorton et al., 2017; Li and Lan, 2017).
Plants primarily absorb Fe from the soil in the form of ferrous (Fe2+) ions. The prevalent form of Fe in soil is ferric (Fe3+), notably in its oxide form known as ferric oxide or hematite. However, soil acidification can aid in the conversion of Fe3+ to the bioavailable Fe2+ form, facilitating optimal uptake by plants. In soils abundant in sesquioxides, intense rainfall and podsolization contribute to soil acidification and subsequent accumulation of Fe ions. Fe enrichment is a distinguishing feature of acid-sulfate soils and low-lying waterlogged terrains. The spectrum of Fe concentration in soil ranges from 10 to 1,000 ppm, and a concentration > 300 ppm is considered critical for toxicity in rice (Dobermann and Fairhurst, 2000). Depending on the intervening factors, Fe toxicity can occur even at lower concentrations, and therefore, contextual intervention may be required to alleviate toxic responses in various soils.
The toxic responses of Fe can exert a significant impact on rice cultivation, as observed particularly in Southeast Asian countries such as China, India, Philippines, Thailand, Malaysia, and Indonesia (Asch et al., 2005; Fageria et al., 2008; Matthus et al., 2015). In India, the major Fe-impacted regions are spread across North-eastern states such as Assam, Arunachal Pradesh, Meghalaya, Nagaland, Mizoram, and Sikkim, along with other states such as Kerala, Odisha, and West Bengal. High acidity and leaching are the common features of soils in the North-east India. Acidity forms due to the weathering of the acidic parental rocks that are rich in Al and Fe. High rainfall together with cultivation practices such as Jhum (slash and burn) encourages continuous soil erosion in these areas, accumulating large quantity of Fe in the lower altitudes and Al in the upper regions, rendering soils marginally fertile.
Rice exhibits a sensitive reaction to toxic levels of Fe in soil. Under toxicity, 18–30% grain yield reduction was observed and under severe conditions the damage can extend to complete crop failure (Audebert and Sahrawat, 2000; Chérif et al., 2009). Rice cultivars, exposed to excess of Fe in the soil, display different adaptive mechanisms, that result in toxicity tolerance (Vose, 1982). These mechanisms invoke sequestration responses such as taking up excess Fe and storing it in vacuoles and apoplasts, followed by a detoxification process that activates antioxidant enzymes to counteract the harmful effects of Fe-induced ROS. In certain instances, roots may undergo Fe exclusion, thereby preventing the excessive absorption of the ion. Overall, these mechanisms help plants to survive and grow under conditions of Fe toxicity. In regions characterized by persistent iron toxicity, effective management techniques include soil amendment and water management (Becker and Asch, 2005; Audebert and Fofana, 2009). However, the most sustainable and effective approach involves the identification and cultivation of rice varieties tolerant to Fe toxicity (Abifarin, 1989; Onaga et al., 2016; Pawar et al., 2017). When used alongside proper soil management practices, tolerant varieties can reduce the need for soil amendments and increase rice yields in lowland areas (Sahrawat et al., 1996; Mahender et al., 2019). Therefore, breeding for tolerance is imperative for Fe-prone areas and ecosystems.
The identification of cultivars resistant to Fe toxicity involves various methodologies, including field-based trials, pot experiments, and hydroponic studies (Hoan et al., 1992; Silveira et al., 2007; Vasconcelos and Grusak, 2014). Though field screening replicates the real time stress originating from the intricate chemical composition of flooded soil, it proves to be laborious, costly, and cumbersome due to the considerable variability in the soil. Conducting the pot experiments, on the other hand, necessitates a substantial quantity of pots and experimental space, leading to increased expenses. Furthermore, it involves the challenge of maintaining consistent stress levels uniformly across all pots (Elec et al., 2013). Among these methods, hydroponics has been widely used to screen for Fe toxicity tolerance as it is the most convenient in maintaining uniform stress levels for a large number of plants in various crops such as maize, chickpea including rice (Mahmoudi et al., 2007; Carvalhais et al., 2011; Dotaniya et al., 2013; Mamidi et al., 2014; Kabir et al., 2016). It has also been used in screening for parameters related to other essential nutrients, such as nitrogen and phosphorus use efficiency (Archana et al., 2021). Although hydroponic experiments may not simulate the actual field conditions, it is reasonably useful in identifying tolerant responses among the genotypes that can be used for further practical use.
Among the various response traits, leaf bronzing is considered one of the most important criteria for screening Fe toxicity tolerance. However, root attributes, such as total root length, root average diameter, root surface area, root projected area, root volume, and number of root tips play a significant role in differentiation between tolerant and resistant genotypes (Pathirana et al., 2002). Besides, the reports of critical Fe concentrations for identifying tolerant and sensitive genotypes were also found to vary across studies, making it difficult to choose a common threshold. Several of these studies lack confidence since they are made on a few genotypes or carried out in one season/location. Therefore, in this report, we present standardization of protocol for Fe toxicity screening along with the effect of Fe toxicity on different morphophysiological parameters leading to the identification and grouping of tolerant and susceptible genotypes. The objective of our investigation is to ascertain the threshold of Fe toxicity concentration, employing it as a criterion for screening and distinguishing between tolerant and susceptible rice genotypes. This selection process is crucial for subsequent integration into breeding programs and investigations at identifying specific genes/QTLs associated with this trait.
2 Materials and methods
2.1 Plant materials
The experiment materials comprised 16 rice genotypes collected from the Division of Genetics, ICAR-Indian Agricultural Research Institute, New Delhi (IARI), details of which are briefed in Table 1. Among these, Shahsarang was an established Fe toxicity tolerant variety (Debnath et al., 2021) while IR 64 was a known sensitive cultivar (Sikirou et al., 2016; Turhadi et al., 2019). The remaining genotypes represented popular cultivars in Basmati, non-basmati, aus and japonica type.
2.2 Hydroponic screening
The hydroponic system was set up at the National Phytotron Facility at IARI with 1X modified Hoagland mixture as the basic nutrient solution for providing optimal nutrients for rice seedlings (Hoagland and Arnon, 1950; Gamborg and Wetter, 1975). In total, 1 L of the nutrient solution consisted of 610.0 mg of KNO3, 120.0 mg of NH4H2PO4, 950.0 mg of Ca (NO3)2.4H2O, 490.0 mg of MgSO4.7H2O, 50.0 mg of FeSO4.7H2O, 2.0 mg of MnCl2.4H2O, 0.02 mg of H2MoO4, 3.0 mg of H3BO3, 0.25 mg of ZnSO4.7H2O, 0.1 mg of CuSO4.5H2O, and 33.0 mg of Na2EDTA. The pH of the nutrient solution was maintained between 5.0 and 5.5.
Initially, the seeds of all the genotypes were surface sterilized using 3% sodium hypochlorite solution by shaking for 1 min, and subsequently, washing with ultrapure water three times. Sterilized seeds are then placed on a germination paper and kept in an incubator for germination at 25–30°C. After 6–7 days, seedlings presenting uniform shoot and root growth were transferred to 100 mm2 wells punched on an expanded polystyrene (EPS) float sheet of size 24 × 36 × 2 cm. Each sheet had 80 wells spaced at 10 × 20 mm.
The sheet was layered with a nylon net of 2 mm mesh size on the underside to prevent seedlings from drowning in the nutrient solution. Each well supported one seedling. The EPS floats were placed in a 10 L rectangular plastic trough of inner dimensions of 24 × 36 × 12 cm filled with 7 L nutrient solutions, with all the roots submerged. Each EPS float supported 16 genotypes with two genotypes accommodated in one row with five plants each. Two such trays per treatment were maintained, which represented two replications. The basic nutrient medium contained 10 ppm (0.2 mM) of Fe, and therefore, the treatment system included varying Fe levels, such as 10p pm (T0), 260 ppm (T1), 360 ppm (T2), and 460 ppm (T3) equivalent to 0.2, 4.7, 6.5, and 8.3 mM of Fe. For imposing treatments, for each replication, 36.5 g of FeSO4.7H2O was freshly dissolved in 400 mL of basic nutrient solution and made up to 500 mL. In addition, 55.0 g of citric acid monohydrate was dissolved separately in 500 mL of nutrient solution. For making T1 treatment, 119 mL of FeSO4 solution and 125 mL of citric acid solution were added to the trough, and the volume was made up to 7 L. Similarly, for T2 and T3 treatments, 167 mL and 214 mL of Fe solution were added respectively, together with 260 ppm of citric acid solution each. The T0 treatment received only 250 mL of citric acid solution. Citric acid was added to improve the effective availability of Fe in plants (Hoagland and Arnon, 1950).
The nutrient solution was changed every 7 days, and the pH of the nutrient solution was maintained between 5.0 and 5.5 using 0.1 M HCl or 0.1 M NaOH daily. Initially, all the trays were maintained uniformly for 7 days in the basic nutrient solution. On the 8th day, in different treatments, half the strength of the Fe concentrations was added to prevent the sudden shock of Fe flooding in the plants. On the 15th day, the strength was increased to full strength. Thereafter, the genotypes were critically observed for the appearance of the symptoms. The data on both root and shoot-related traits were recorded after 35 days of sowing (Figure 1).
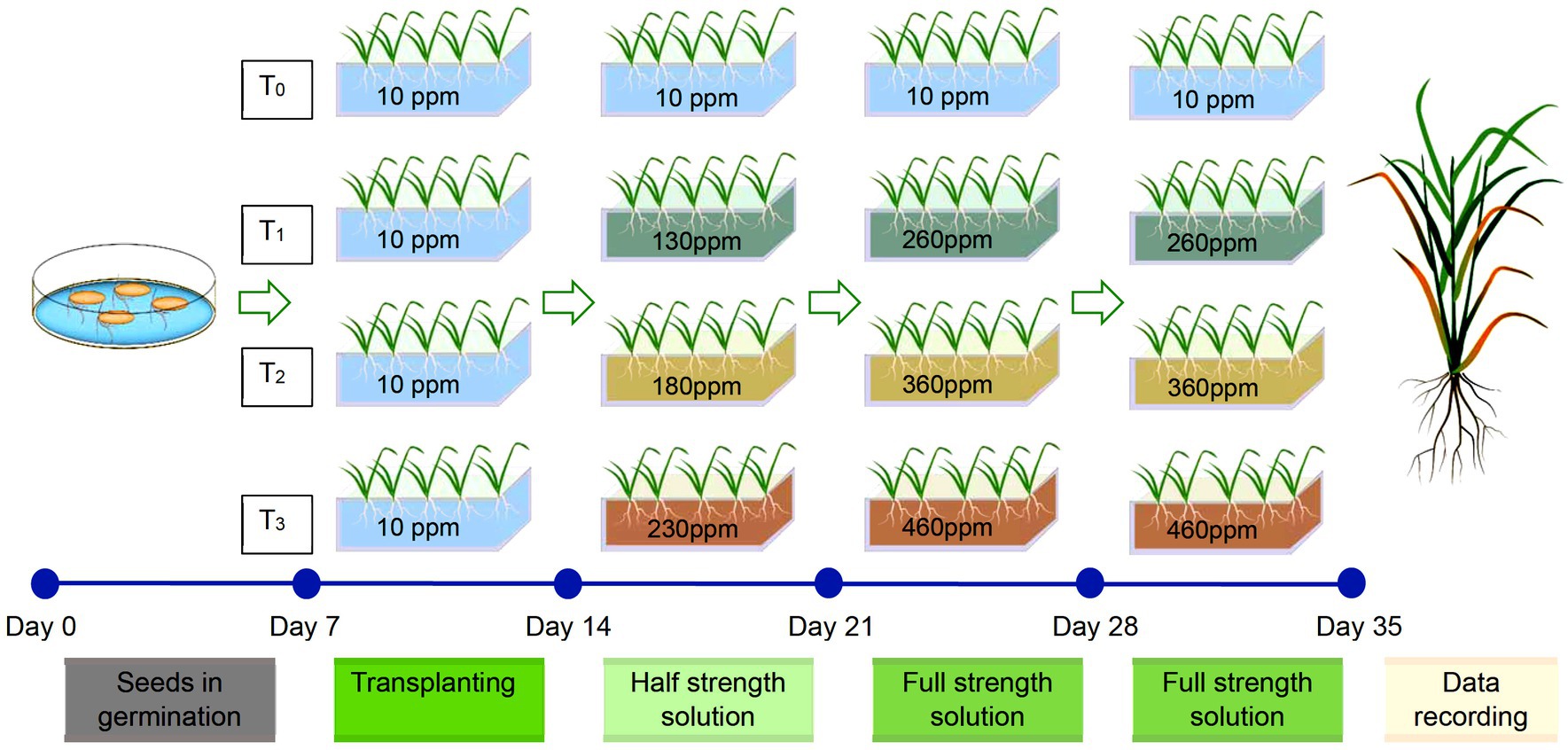
Figure 1. Schematic representation of hydroponic screening of genotypes at control (T0), 260 ppm (T1), 360 ppm (T2), and 460 ppm (T3).
2.3 Phenotyping
The plants were evaluated non-destructively for leaf bronzing score (LBS) after 10 days of the full-strength stress treatment using a modified score scale (Table 2 and Supplementary Figure S1), integrating the observations from the study by Shimizu et al. (2005) and Wissuwa et al. (2006). Thirty-five days after sowing, all five sample plants per genotype were individually picked from the wells, and measurements of maximum root length (RL) and shoot length (SL) were taken. Subsequently, the plants were dissected into root and shoot sections. After blotting dry with an absorbent paper, the fresh weight of the shoot was measured. The root section was immediately scanned with a WinRHIZO® optical scanner (model Expression 12000XL, Epson Inc.), to measure root system architectural traits (Arsenault et al., 1995), such as root volume (RV), total root length (TRL), average diameter (AD), and surface area (SA). Following measurement, the root samples were blotted dry with an absorbent paper, and their fresh weight was measured. Both root and shoot samples were then oven-dried at 60°C for 72 h, to determine the corresponding dry weight, and the biomass (BM) was calculated by summing up the shoot and root dry weight. Oven-dried shoot samples were subsequently used to estimate shoot Fe concentration (SFC). For this purpose, all five dry samples per genotype were pooled and ground in a porcelain mortar. A 250 mg portion of these powdered samples was then wet-digested in 4 mL of 65% HNO3 at 180°C for 8 h. The digest was subsequently diluted to 25 mL with distilled water and filtered. The filtrate was used to measure the Fe content using atomic absorption spectroscopy (AAS). Standard Fe solutions were prepared through serial dilutions ranging from 1 to 10 ppm.
2.4 Data analyses
The experiment was conducted in a completely randomized design with two factors, different levels of Fe and genotypes. The experiment was repeated twice, by setting the second experiment immediately after the first with the same setup, treatments and the management. The experimental data were subjected to analysis of variance using PBTools v.1.4.1 The degree of concordance (DoC) of the screening was calculated from the squared correlations between the experiment for every trait. The adjusted mean values were used for further analyses. The box plots were drawn from the average data, and the means were compared using Duncan’s multiple range test. Response stability of different traits relative to the unstressed treatment (T0) was calculated for the treatment with maximum stress (T3) by calculating the response stability index (RSI), which was similar to the yield stability index proposed by Bouslama and Schapaugh (1984). A correlogram of all the traits was visualized using the corrplot package in the R statistical environment. A principal component analysis (PCA) was performed to identify the most variable traits using princomp() function in the R stats package, and the biplots were drawn using the factoextra package. The contribution of different traits to principal components is graphically visualized using Circos Table Viewer v0.63–10 (Krzywinski et al., 2009). Principal component scores were utilized for the hierarchical clustering of genotypes, employing the Euclidean distances and the farthest neighbor clustering method. The clustering was performed using the pheatmap package under the R statistical environment.
3 Results
In both the screenings, a similar response to Fe concentration was observed among the genotypes. Combined analysis of variance indicated a significant variation of genotypes, Fe levels, and their interaction on all the root-related and shoot-related traits, except for root length in the screening 1. However, variation due to time of screening and respective interaction with genotypes was found non-significant except for LBS and RDW (Table 3 and Supplementary Table S1). The concordance of both experiments was high and significant as revealed by the degree of concordance, which was above 80% for all the traits except for RL.
3.1 Phenotypic variation for Fe levels
Comparison based on the significant difference of treatment mean values for the traits indicated that, irrespective of the genotypes, there was a significant increase in LBS and SFC while there was a reduction in SL, SFW, SDW, RFW, RDW, TRL, and SA as the Fe concentration increased (Figure 2). The mean LBS substantially increased from 0.34 in T0 condition to 1.69 at T1, 5.28 at T2, and 6.62 at T3. The range of leaf bronzing was from 0.4 to 3.8 at T1, 3.0 to 7.0 at T2, and 3.0 to 9.0 at T3 of Fe, implying that the highest variability for LBS was observed at T3 of Fe treatment compared with T2 and T1 of Fe treatment (Table 4). A similar effect could also be observed for SFC among the treatments with the lowest mean 1.76 at T0, 6.41 ppm at T1, 11.01 ppm at T2, and 14.87 ppm at T3. Beyond T0, the treatment means did not vary significantly for SL, TRL, and SA. The SL was found reduced from 48.57 cm in T0 to an average of 27.2 cm among the rest of the Fe levels. Significant change in treatment mean could be observed beyond T2 for several traits, such as SFW, SDW, RL, RFW, RV, and AD (Table 4). The lowest values for most of the traits were encountered in T3, which was significantly lower than T2 for RV and RL. The relative differences over mean (RDM) among the treatments also showed distinct variations after T2 for almost all the traits, except SL and SA, for which no significant reduction could be observed. Among the treatments with high Fe levels, RDM values were higher in T3 for traits, such as RL, RDW, and RV, whereas this level showed effects at par with T2 for the remaining traits, except for SFW, SDW, and RFW. Coupled with the highest variance, T3 was the treatment that could reveal significant variation among the genotypes for the Fe toxicity response and was therefore considered as the critical concentration.
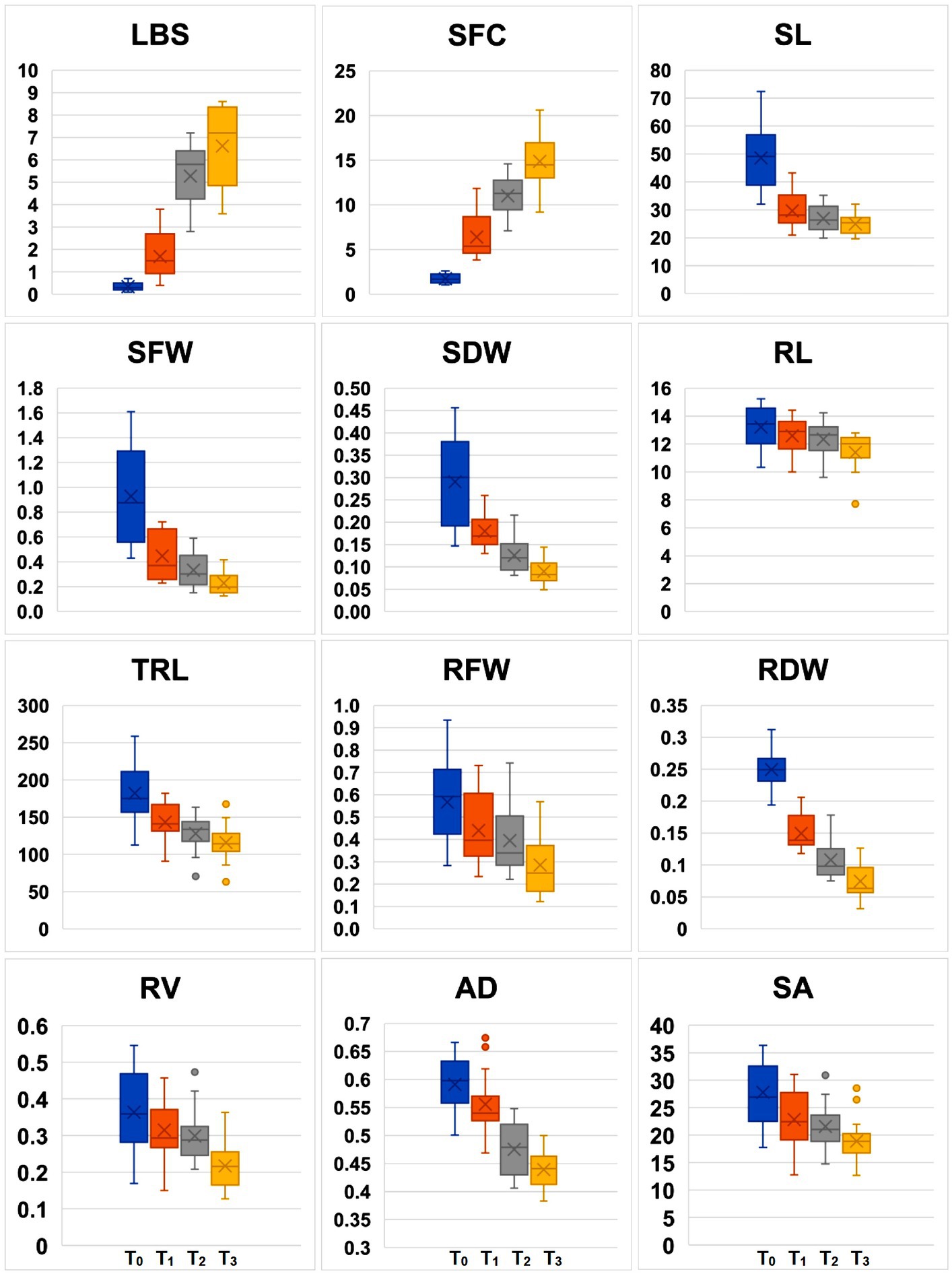
Figure 2. Box plots showing mean variability in root and shoot traits under four Fe concentrations. LBS, leaf bronzing score; SFC, shoot iron concentration in mg/kg; SL, shoot length in cm; RL, maximum root length in cm; SFW, shoot fresh weight in mg; SDW, shoot dry weight in mg; RFW, root fresh weight in mg; RDW, root dry weight in mg; TRL, total root length in cm; RV, root volume in mm3; AD, average diameter in μm; SA, surface area in cm2.
3.2 Response of genotypes
The response stability index of the genotypes under maximum stress exhibited varying degrees of response across the traits (Table 5). As mentioned previously, the most significant deviations were observed for LBS and SFC. In the case of LBS, the lowest index values were found in the already known tolerant cultivars such as Shahsarang (6.3) and Megha SA2 (7.2), while the high index values were observed in RTN 10B (62.0), Pusa 1,342, and IR 64 (43.0 each), as well as BPT 5204 (41.0). Similarly, for SFC, the lowest index values were recorded for NPT 34 (3.5), followed by ILS 12–5 (5.2). The remaining traits did not show significant differences among the index values. Consequently, the index sum was used to compare the overall response of the genotypes. Based on this analysis, Shahsarang (17.4), ILS 12–5 (20.4), and Megha SA2 (21.3) were identified as the genotypes, displaying a high level of tolerance to Fe toxicity. Among the sensitive genotypes, RTN 10B had the highest index sum (78.0), followed by IR 64 (57.3), BPT 5204 (55.6), and Pusa 1,342 (54.3).
At the maximum toxicity level, Shahsarang and Megha SA2 displayed LBS values of 4.0 and 3.4, respectively, whereas the susceptible variety IR 64 had an LBS value of 8.6 (Supplementary Table S2). The lowest SFC was observed in NPT 34 (9.34), while the highest was found in IR 64 (20.6). PB 1121 exhibited the highest SL at 32.3 cm, followed closely by Shahsarang at 32.1 cm, with BPT 5204 having the shortest SL with an average value of 19.6 cm. In terms of SFW among genotypes under the highest toxicity, it ranged from 0.5 mg (ILS 12–5) to 0.1 g for IRGC 127653. A similar pattern was observed for SDW. Among the root traits, RL ranged from 7.8 cm (NPT 34) to 14.0 cm (ILS 12–5) under high toxic conditions. As for RFW, ILS 13–5 had the highest value at 569.0 mg, followed by Megha SA2 and Shahsarang with an average dry weight of 506.0 mg. The same genotypes showed a similar response for RDW. Root biomass, as determined by RFW and RDW, was low among sensitive genotypes, such as RTN 10B, IR 64, BPT 5204, and others.
Root system architectural traits, such as SA, AD, and RV, under high toxicity levels, also highlighted the differences between tolerant and sensitive genotypes. SA was greater in Megha SA2 (31.0 cm2), ILS 12–5 (30.8 cm2), and Shahsarang (28.8 cm2), whereas Pusa 1,342 (13.3 cm2), BPT 5204 (14.3 cm2), IR 64 (18.5 cm2), and others had lower values for this trait. However, the AD of the roots showed relatively low variation among the genotypes under T3. RV was more robust in the tolerant genotypes, particularly in ILS 12–5 (363.0 mm3) and Megha SA2 (314.0 mm3), while the lowest RV was observed in NPT 24 (127.0 mm3).
3.3 Trait correlations and modelling the genotypic response for biomass
Correlation of component traits at maximum toxicity showed LBS having a negative but significant association with SFC, SFW, SDW, BM, PA, SA, AD, and RV. Trait pairs such as SFW:SDW and PA:SA:RV were autocorrelated. SFC exhibited high a positive association with SL, while SL indicated positive and significant associations with biomass traits, such as SFW, SDW, and BM and PA, SA, and RV. RL showed a positive association with TRL, SA, and RV. TRL also had a positive association with RFW, PA, SA, and RV. All the root system architecture traits indicated high association among themselves (Figure 3). Since several traits showed autocorrelations, further analyses were carried out with eight traits, viz., LBS, SFC, SL, BM, RL, TRL, AD, and RV.
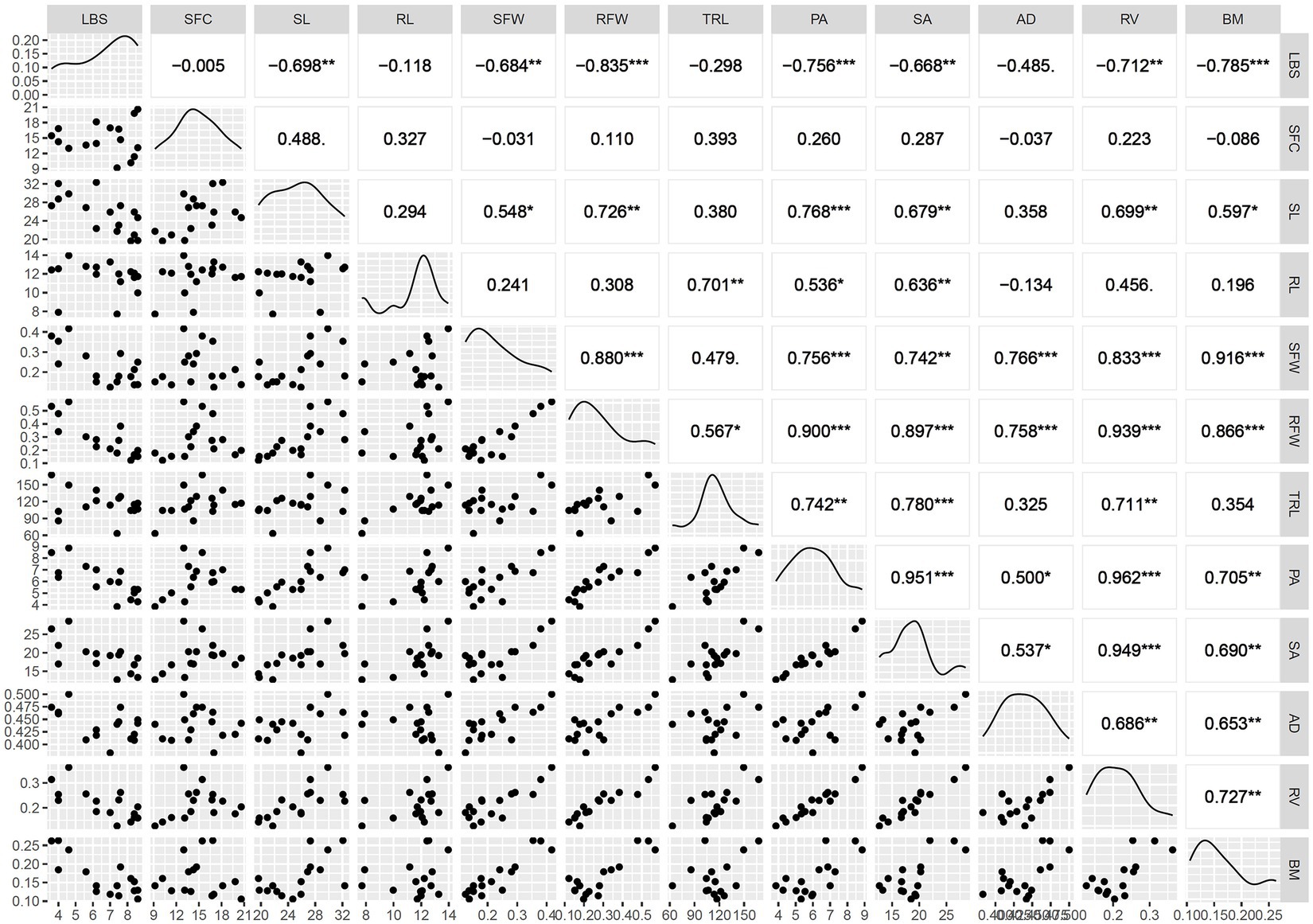
Figure 3. Correlogram showing Pearson correlation coefficient among the traits under the maximum Fe toxicity treatment. LBS, leaf bronzing score; SFC, shoot iron concentration in mg/kg; SL, shoot length in cm; RL, maximum root length in cm; SFW, shoot fresh weight in mg; RFW, root fresh weight in mg; TRL, total root length in cm; RV, root volume in mm3; AD, average diameter in μm; PA, projected area in cm2; SA, surface area in cm2; BM, Biomass in mg, ns, not significant at p ≤ 0.05; *p < 0.05; **p < 0.01, ***p < 0.001.
Considering the total dry matter (weight) as the important response variable under Fe toxicity among the rice seedlings, 25 regression models with best fit were identified using seven dependent variables (Supplementary Table S3). Among the models, the best-fit model with the highest R2 value, lowest Akaike information criterion (AIC), and lowest Mallow’s Cp was the model having two parameters, LBS and AD. This model explained 72% of the total variation in BM with the following coefficients. Additionally, the model also provided the lowest error variance of 850.0 among all the models. The Durbin– Watson (DW) statistic p-value shows no serial autocorrelation in the residuals at the 95.0% confidence level. The model coefficients are provided in following equation.
BM ~ 23.24–17.65*LBS + 0.59*AD
The model statistics are presented in Table 6. Although this mode accounted for the maximum variation in BM, the value of ps of AD remained at a non-significant level, signifying an inconspicuous contribution to this trait. Therefore, the model was modified as BM ~ 313.5–22.51*LBS, accounting for 62.5% of the total variation.
3.4 Multidimensional classification of genotypes
Taking into consideration of the variables that exhibited weak association among themselves, eight principal components (PCs) were extracted by PCA. The first two components were identified significant as they accounted for 74.3% of the total variation (Figure 4). The biplot of PC1 versus PC2 showed that the Fe toxicity tolerant genotypes Megha SA2, ILS 12–5, Shahsarang, and Sonasal located at the upper right quadrant. The upper left quadrant consisted of moderately sensitive genotypes such as NPT 34, Pusa 1,342, and BPT 5204. The lower right quadrant encompassed the moderately tolerant genotypes PB 1121 and PB 1, while sensitive genotypes IR 64, IRGC 127653, Nagina 22, and Pusa 44 fell in the lower left quadrant. Analyzing the influence of traits on PC1, greater contribution could be observed from traits like RV, BM, LBS, AD, and TRL, all demonstrating nearly equal levels of contributions. Conversely, on PC2, RL and FSC exhibited significant contributions.
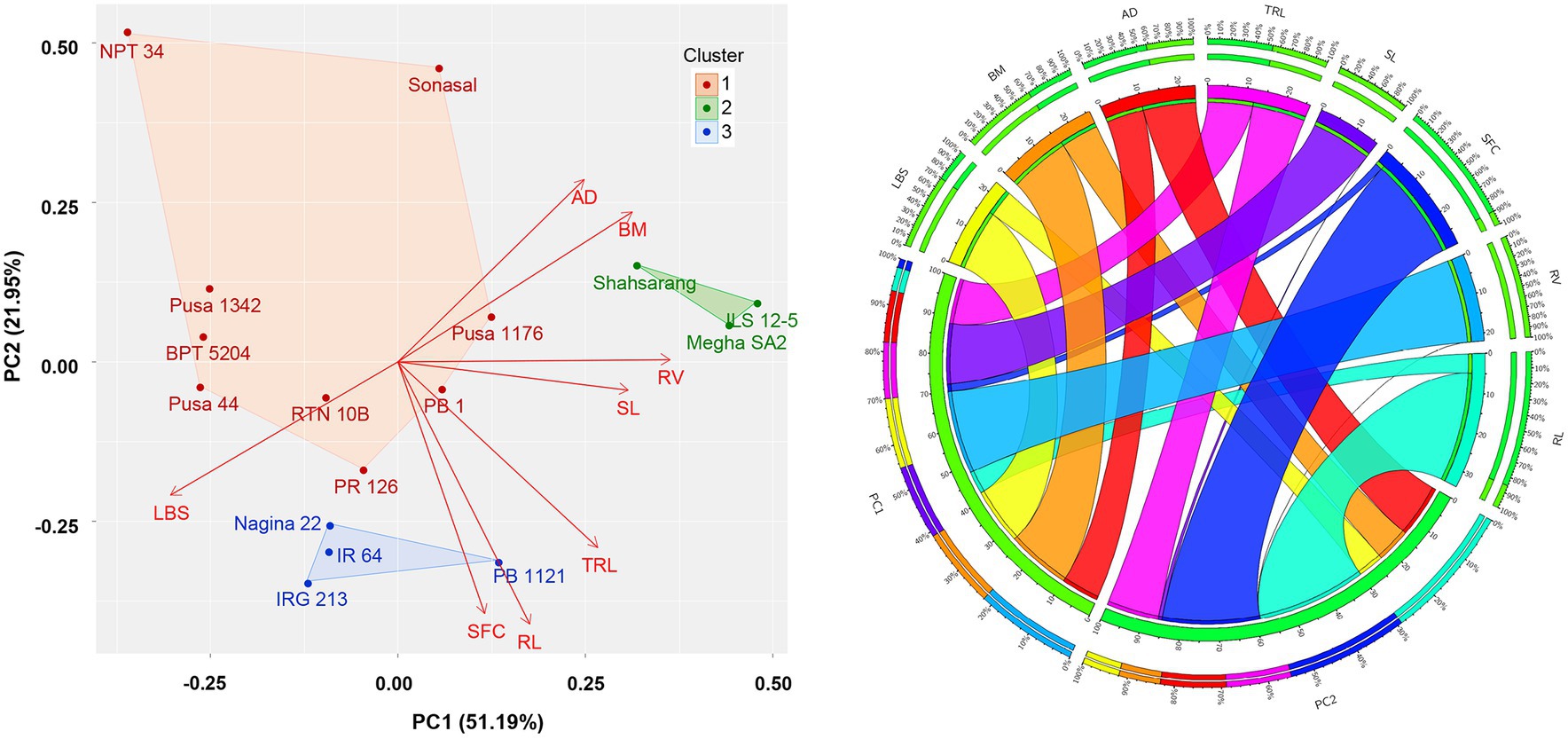
Figure 4. PCA-biplots depicting genotype clusters and trait contribution visualized via Circos-diagram for major principal components. LBS, leaf bronzing score; SFC, shoot iron concentration in mg/kg; SL, shoot length in cm; RL, maximum root length in cm; TRL, total root length in cm; RV, root volume in mm3; AD, average diameter in μm; BM, Biomass in mg.
PCA-based clustering generated three clusters of genotypes. Cluster 1 comprised of NPT 34, Pusa 1,342, Pusa 44, and BPT 5204, making it a group of sensitive genotypes. ILS 12–5, Megha SA2, and Shahsarang formed the Cluster 3, having tolerance to Fe toxicity. The Cluster 2 comprised of remaining genotypes that showed an intermediate response. Surprisingly, IR 64 was found included in this cluster, even though it is a well-known sensitive cultivar. Other members in the Cluster 2 were Pusa 1,176, PB 1, Sonasal, PB 1121, IRGC 127653, RTN 10B, PR 126, IR 64, and Nagina 22 (Figure 4).
4 Discussion
Fe toxicity is a nutritional stress that commonly affects lowland rice. In India, approximately 11.7 mha of rice area is affected by excess Fe in soil, potentially interfering with crop production. Identifying genotypes that can significantly withstand excess Fe stress is crucial for sustaining rice production in affected soils. Given that Fe affects the rice crop at all stages, an early-stage evaluation can help in identifying tolerant genotypes, justifying the usefulness of artificial screening. Although several studies have been reported, there seem to be many disagreements regarding the level of critical toxicity imposed (Elec et al., 2013; Rout et al., 2014; Reddy et al., 2015; Suma et al., 2022). Dobermann and Fairhurst (2000) identified 300–500 ppm to be a critical level of toxicity in soil for rice; however, the level changes with respect to soil properties and crop growth stage. Therefore, Fe toxicity screening requires standardization of screening conditions before setting up of experiments. Furthermore, conditions for soil-based screening needed to be customized depending on the soil type and properties, which warrants multiple testing, making it a cumbersome process. Soil-based or field-based screening (Gridley et al., 2006; Sikirou et al., 2016), pot culture (Sikirou et al., 2016), and hydroponics (Wan et al., 2003; Shimizu et al., 2005) are the major ways of studying genotype response. However, hydroponics is considered to be quick, efficient, and reliable (Suma et al., 2022). Among the various traits, LBS is considered as the most important parameter to study genotype response (Sikirou et al., 2016; Rumanti et al., 2017; Devi et al., 2018; Siriwardana et al., 2018). This is because the plants under excess Fe level produce a characteristic ‘bronzing’ symptom, due to the formation of numerous brown specks on the leaves (Wu et al., 2014). Bronzing occurs when the plants are deprived of oxygen supply from roots, and excess Fe accumulates in the leaves and shoots, leading to the Fenton reaction, cellular damage, and consequent yellowing and browning of tissues (Aung and Masuda, 2020). Plants that are adapted to Fe toxic ecologies show lesser bronzing than the un-adapted sensitive genotypes (Turhadi et al., 2019). Bronzing typically occurs on the lower leaves, starting to tip downward, and entire leaves can turn yellow or brown in severe cases. Bronzing is accompanied with growth retardation and plaque formation on the roots. Although many reports are available on various morphophysiological traits in different genotypes, not many deals with the effect of Fe toxicity on root system architecture (RSA) attributes. This study forms one such preliminary attempt to detail the effect of Fe toxicity stress on root system in rice.
We have selected 16 genotypes, of which two were well-known Fe tolerant cultivars adapted to North-eastern ecology. These genotypes, Shahsarang and Megha SA2, are widely cultivated in the North-east states of India, such as Meghalaya, Mizoram, Manipur, and Nagaland. Shahsarang is a high-yielding hybrid derivative of Mirikrak/Rasi, with semi-dwarf and non-lodging habit. Among the several features of this variety, traits such as tolerance to Fe toxicity and adaptation to cold and low light stand out as typical. Megha SA 2 (Megha Semi-Aromatic 2) or RCPL 1–160 is a fine grain high-yielding semi-dwarf variety, with adaptation to lowlands of low-altitude ecology. Being established and tolerant to excess Fe, these varieties would help in comparative evaluation. In addition, IR 64 was included, a mega variety that was established as sensitive to excess Fe from various studies (Wu et al., 1997; Mackill and Khush, 2018; Turhadi et al., 2019). The remaining genotypes were selected at random but represented mega varieties, Basmati cultivars, stress-tolerant cultivars, and green super rice. The selection of an optimal set of genotypes provided an opportunity to evaluate the response to different Fe concentrations on various morphological traits and identify the critical iron concentration for screening. Critical iron concentration is a parameter influenced by tested genotypes, a form of Fe applied, and solution pH (De Dorlodot et al., 2005; Nozoe et al., 2008). Sharp contrast between tolerant and sensitive genotypes with respect to Fe toxicity symptoms and variation for traits was the main criterion to choose the optimum combination (Elec et al., 2013). Accordingly, 460 ppm of Fe was selected as critical, as it provided larger variance among the traits and could display remarkable contrasts between known tolerant and sensitive genotypes, and largest decrease in most of the morphologic traits from normal.
High contrast among various traits is desirable to differentiate genotypes from extreme responses under stress (Rinyu et al., 1995; Houle, 1998). Although significant variation could be observed on all the traits under normal Fe concentration, LBS and SFC increased with Fe level, indicating the progressive influence of toxicity. These observations are in concordance with several previous reports (Zhang et al., 2017; Onyango et al., 2018). A sharp increase in LBS among the genotypes with increased levels of Fe could imply an increased accumulation of toxic levels of Fe ions, driving Fenton reactions and cellular damage. One of the important features of excess Fe uptake in plants is the accumulation of Fe in the cellular component as revealed by the increase in SFC with increasing toxicity stress. In tolerant genotypes, accumulation of excess Fe2+ ions in vacuoles (plastids) forms a mode of tolerance (Asch et al., 2005; Wu et al., 2014; Santos et al., 2018). However, tolerant varieties, Shahsarang and Megha SA2, accumulated less Fe in the shoot as compared with IR 64, which indicated a different mechanism in operation in these genotypes. These varieties may have exclusion (type I) mechanism, wherein excess Fe is prevented from uptake by oxidation and precipitation on the root surface (Becker and Asch, 2005; Saikia and Baruah, 2012; Wu et al., 2014; Dufey et al., 2015; Sikirou et al., 2016; Turhadi et al., 2018; Bresolin et al., 2019; Aung and Masuda, 2020). As reported earlier, leaf bronzing remained the most important parameter and straightforward indicator of the intensity of Fe toxicity stress in this study (Wan et al., 2003; Asch et al., 2005; Dufey et al., 2009). As a result, increased bronzing with increasing levels of Fe indicated a strong positive correlation. Since the stress induced a reduction in all other traits, SFC, LBS showed a strong negative relationship with all those traits that showed a progressive reduction with increasing Fe levels. However, although the SFC exhibited a positive correlation with LBS, earlier studies indicate that the iron content in plant tissue may not always be associated with bronzing scores (Nugraha et al., 2016b; Turhadi et al., 2018; Sitaresmi et al., 2021).
Several earlier studies have reported a reduction in SL under Fe toxicity (Rout et al., 2014; Bresolin et al., 2019; Suma et al., 2022), we did not observe any significant difference in the height of seedlings at different Fe concentrations. However, reduction in RL was significant at critical Fe level, confirming root growth inhibition as one of the primary damages due to Fe toxicity. Toxic levels of Fe interfere with and inhibit cell division and elongation of the primary roots and, subsequently, the growth of lateral roots (Li et al., 2016a; Hamim et al., 2018; Suma et al., 2022). Since RL reduction appears after the primary damage to the root system, a concomitant reduction in nutrient uptake can be expected as a consequence. However, in the seedling stage evaluation, no apparent reduction in shoot growth was observed because of the short-term exposure to stress. Therefore, shoot traits cannot be considered as reliable parameters for screening genotypes for Fe toxicity response particularly in the seedling stage. Nevertheless, shoot and root biomass (fresh and dry weight) showed significant differences with increasing Fe stress, aligning with earlier studies (Dufey et al., 2009; Wu et al., 2014; Nugraha et al., 2016b; Reddy et al., 2019).
With the increasing reduction in biomass observed beyond 360 ppm, the relative deviation from the biomass under normal Fe level could clearly differentiate tolerant and sensitive genotypes at the critical level. As with the earlier reports, the reduction in biomass is reflective of the retardation in the growth of the plants as a result of stress due to Fe toxicity (Onaga et al., 2013; Nugraha et al., 2016b; Theerawitaya et al., 2022). Among the biomass traits, RDW, in particular, is reported to be influenced by the presence of Fe coating on the root surface, which comes as a result of the exclusion mechanism (De Dorlodot et al., 2005; Saikia and Baruah, 2012; Sikirou et al., 2016; Wu et al., 2017). In this study, higher relative root and shoot dry weight observed among the tolerant rice lines signifies better growth among these genotypes under stress, which aligns well with the previous reports by Wu et al. (2014) and Devi et al. (2018).
Notwithstanding the importance of aerial morphologic changes, the major focus of this study was on the root system architecture. Although much-needed attention was not provided to these traits earlier, the root system has recently recognized as an effective selection parameter to determine the ability of plants to manage various soil stresses (Mahender et al., 2019). However, the information on the effects of excess Fe on the root system architecture in rice is still scanty (Li et al., 2016a). Roots being the primary plant organ involved in Fe uptake from the soil solution, the first line of defense against excess Fe, must initiate at the rhizosphere (Onaga et al., 2016). Hence, Fe toxicity is considered to affect and alter the root system architectural traits (Mahender et al., 2019). The experiences from the other soil-based stresses, such as drought and phosphorus starvation, showed that highly dynamic adjustments in overall root system architecture (RSA) determine root plasticity and allow plants to efficiently adapt to environmental constraints. Irrespective of the sensitive or tolerant behavior of genotypes, we could observe a marked decrease in TRL, SA, AD, and TV, with maximum reduction occurring with the exposure to the highest toxicity level. Therefore, the reduction in RSA traits could occur due to the damage in the root system in sensitive genotypes, while it can also be a part of the root development adaptation strategy in the tolerant genotypes to restrict excess Fe absorption (Li et al., 2016b). Reduction in the TRL due to Fe toxicity was also reported by Ward et al. (2008) in Arabidopsis thaliana, where the primary root length was inhibited. Excess Fe inhibited the formation of 1st, 2nd, and 3rd order lateral roots confirmed by a decrease in the number of roots. Earlier studies have concluded that arrested cell division and elongation due to excess Fe could significantly distort the root system architecture (Reyt et al., 2015; Li et al., 2016b).
Among the various root traits, SA contributed >15% to the total genotypic variation both in control and stress conditions. A reduced root surface area can be explained as a strategy to restrict nutrient absorption (Kirk et al., 2022). Nurmalasari et al. (2016) observed a reduction in SA due to Fe toxicity in oil palm. The reduction in the SA may be considered as a potential mechanism for Fe exclusion, evidenced by a decrease in the number of root hairs. Reduction in SA could also be occurring due to reduced AD. Wu et al. (2014) reported a marked reduction in AD in the susceptible variety IR 29 as compared with the tolerant line Pokkali, which is similar to the observation in the tolerant varieties, Shahsarang and Megha SA2, as compared with IR 64. Higher AD in the tolerant genotypes facilitates the formation of aerenchyma in roots, enhancing air transport and the ability to oxidize Fe2+ in the rhizosphere, ultimately contributing to Fe exclusion. A decrease in RV among the sensitive genotypes could be interpreted as a reduction in RL, SA, and AD (Hussain et al., 2020).
Stress indices can serve as effective parameters to identify tolerant and sensitive genotypes (Kakar et al., 2019; Dwivedi et al., 2021). The response stability index used in this study was the ratio of response under stress to the un-stressed (Bouslama and Schapaugh, 1984), which is an indicator or genotype’s resilience to stress. When challenged with stress, if any genotype could respond similarly or better than the unstressed conditions, the index will tend toward one and above. Therefore, lower values of the index indicated tolerance, while higher values indicated sensitiveness. Accordingly, based on the index sum, we could identify genotypes such as Shahsarang, Megha SA2, and ILS 12–5 as the most resilient genotypes among all. Interestingly, most of the sensitive genotypes showed an index sum of >50, which included RTN 10B, IR 64, BPT 5204, Nagina 22, and Pusa 1,342. Genotypes such as PB 1121, Pusa 1,176, PR 126, NPT 34, Sonasal, and Pusa 44 showed moderate tolerance, while PB 1 and IRGC 127653 were moderately sensitive. Several stress evaluation studies reported the usefulness of stress indices for identifying tolerant genotypes and classifying them (Dhawan et al., 2021; Joshi et al., 2023). The grouping of 16 rice genotypes, based on their morphophysiological responses to Fe toxicity, also indicated conspicuous polarization of tolerant and sensitive genotypes. PCA-based clustering has been previously utilized in studies by Nugraha et al. (2016a,b), Pawar et al. (2017), Dziwornu et al. (2018), Theerawitaya et al. (2022), and Ahmed et al. (2023) for identification of sensitive and tolerant genotypes. Using the index sum and PCA the sensitive genotypes could be separated clearly from the tolerant genotypes with, the moderately responding genotypes falling in between. The results also indicated that the index sum was robust enough to differentiate between tolerant and sensitive genotypes. In previous studies, LBS served as the exclusive criterion for classifying tolerant and sensitive genotypes (Engel et al., 2012; Harahap et al., 2014; Nugraha et al., 2016b). However, in this study, we were able to show that changes in the root system can function as a significant criterion for classification.
5 Conclusion
The present study concluded that 460 ppm of Fe in the nutrient solution was critical for screening genotypes for their response to Fe toxicity. Based on the significant effect of excess Fe on morphological and root system characteristics in rice genotypes, these genotypes could be classified into tolerant and sensitive ones. Leaf bronzing was the major response to Fe stress in plants, indicating a high level of contrast between tolerant and sensitive genotypes. The response stability index across traits provided ample opportunity for classification. In addition to the known tolerant genotypes, ILS 12–5 was identified as a tolerant, qualifying it to be tested under Fe-rich field conditions. Some of the most sensitive genotypes included mega varieties such as BPT 5204 and Pusa 44, making them suitable candidates for Fe toxicity tolerance improvement. This would enable these mega-varieties to be recommended for Fe-toxic regions of Northeastern India. Fe toxicity had a severe effect on RSA traits, such as RL, SA, and RV. This report provides insight into the influence of toxic levels of Fe on the root system. There is a reasonable indication that the responses in aerial biomass are consequential to root system damage, resulting from the nutrient deprivation. Furthermore, Fe exclusion could be a major mechanism for conditioning toxicity tolerance in tolerant genotypes, enabling them to reduce Fe2+ uptake. These valuable inferences will be useful for managing Fe toxicity and improving tolerance in rice through selection strategies in breeding programs. Field evaluation of identified tolerant/sensitive lines is still required to confirm their behavior under field conditions and use them in breeding programs. The effect of Fe toxicity on RSA traits can be further investigated for a better understanding of intrinsic mechanisms of root system variations, differentiating tolerant and sensitive genotypes.
Data availability statement
The original contributions presented in the study are included in the article/Supplementary material, further inquiries can be directed to the corresponding author.
Author contributions
So: Data curation, Formal analysis, Investigation, Methodology, Validation, Writing – original draft. SN: Formal analysis, Validation. VS: Data curation, Investigation, Writing – review & editing. RP: Methodology, Investigation. SG: Supervision, Resources. PB: Methodology, Resources, Validation. RE: Formal analysis. HB: Validation. BH: Investigation. SY: Investigation. RB: Data curation. MN: Resources. AS: Project administration. KV: Conceptualization, Data curation, Investigation, Methodology, Resources, Supervision, Visualisation, Writing – review & editing.
Funding
The author(s) declare financial support was received for the research, authorship, and/or publication of this article. The author(s) declare financial support received in the form of a senior research fellowship received by the senior author from ICAR-IARI. The author(s) declare publication support of this article from the Crop Research Platform - Hybrid Technology (CRP-HT) project code 12-142A from the Indian Council of Agricultural Research (ICAR).
Acknowledgments
The authors greatly acknowledge the facilities provided by the National Phytotron Facility and the Divisions of Genetics and Crop Physiology in conducting this study.
Conflict of interest
The authors declare that the research was conducted in the absence of any commercial or financial relationships that could be construed as a potential conflict of interest.
Publisher’s note
All claims expressed in this article are solely those of the authors and do not necessarily represent those of their affiliated organizations, or those of the publisher, the editors and the reviewers. Any product that may be evaluated in this article, or claim that may be made by its manufacturer, is not guaranteed or endorsed by the publisher.
Supplementary material
The Supplementary material for this article can be found online at: https://www.frontiersin.org/articles/10.3389/fsufs.2023.1334487/full#supplementary-material
References
Abifarin, A. O. (1989). Progress in breeding rice for tolerance to iron toxicity. WARDA Ann. Rep., 34–39.
Ahmed, S. F., Ullah, H., Aung, M. Z., Tisarum, R., Cha-Um, S., and Datta, A. (2023). Iron toxicity tolerance of rice genotypes in relation to growth, yield and physiochemical characters. Rice Sci. 30, 321–334. doi: 10.1016/j.rsci.2023.02.002
Archana, R., Bollinedi, H., Nagarajan, M., Gangadhara, K. N., Ellur, R. K., Singh, V. J., et al. (2021). Stay green behaviour of a novel mutant, PSG16 shows complex inheritance and functional relations with grain yield in rice. Indian J. Genet Plant Breed. 81, 495–504. doi: 10.31742/IJGPB.81.4.1
Arsenault, J. L., Poulcur, S., Messier, C., and Guay, R. (1995). WinRHlZO™, a root-measuring system with a unique overlap correction method. Hortic. Sci. 30:906D. doi: 10.21273/HORTSCI.30.4.906D
Asch, F., Becker, M., and Kpongor, D. S. (2005). A quick and efficient screen for resistance to iron toxicity in lowland rice. J. Plant. Nutr. Soil Sci. 168, 764–773. doi: 10.1002/jpln.200520540
Audebert, A., and Fofana, M. (2009). Rice yield gap due to iron toxicity in West Africa. J. Agron. Crop. Sci. 195, 66–76. doi: 10.1111/j.1439-037X.2008.00339.x
Audebert, A., and Sahrawat, K. L. (2000). Mechanisms for iron toxicity tolerance in lowland rice. J. Plant Nutr. 23, 1877–1885. doi: 10.1080/01904160009382150
Aung, M. S., and Masuda, H. (2020). How does rice defend against excess iron? Physiological and molecular mechanisms. Front. Plant Sci. 11:1102. doi: 10.3389/fpls.2020.01102
Bashir, K., Nozoye, T., Nagasaka, S., Rasheed, S., Miyauchi, N., Seki, M., et al. (2017). Paralogs and mutants show that one DMA synthase functions in iron homeostasis in rice. J. Exp. Bot. 68, 1785–1795. doi: 10.1093/jxb/erx065
Becker, M., and Asch, F. (2005). Iron toxicity in rice—conditions and management concepts. J. Plant. Nutr. Soil Sci. 168, 558–573. doi: 10.1002/jpln.200520504
Bouslama, M., and Schapaugh, W. T. (1984). Stress tolerance in soybeans. I. Evaluation of three screening techniques for heat and drought tolerance 1. Crop Sci. 24, 933–937. doi: 10.2135/cropsci1984.0011183X002400050026x
Bresolin, A. P. S., Dos Santos, R. S., Wolter, R. C. D., de Sousa, R. O., da Maia, L. C., and Costa de Oliveira, A. (2019). Iron tolerance in rice: an efficient method for performing quick early genotype screening. BMC Res. Note. 12, 361–366. doi: 10.1186/s13104-019-4362-5
Carvalhais, L. C., Dennis, P. G., Fedoseyenko, D., Hajirezaei, M. R., Borriss, R., and von Wirén, N. (2011). Root exudation of sugars, amino acids, and organic acids by maize as affected by nitrogen, phosphorus, potassium, and iron deficiency. J. Plant. Nutr. Soil Sci. 174, 3–11. doi: 10.1002/jpln.201000085
Chérif, M., Audebert, A., Fofana, M., and Zouzou, M. (2009). Evaluation of iron toxicity on lowland irrigated rice in West Africa. Tropicultra 27, 88–92.
Connorton, J. M., Balk, J., and Rodríguez-Celma, J. (2017). Iron homeostasis in plants – a brief overview. Metallomics 9, 813–823. doi: 10.1039/C7MT00136C
De Dorlodot, S., Lutts, S., and Bertin, P. (2005). Effects of ferrous iron toxicity on the growth and mineral composition of an interspecific rice. J. Plant Nutr. 28, 1–20. doi: 10.1081/PLN-200042144
Debnath, A., Rai, M., and Tyagi, W. (2021). Identification of Swarna x O. Nivara (RPBio4918) advanced backcross lines performing well under acidic soil conditions. J. Environ. Biol. 42, 240–246. doi: 10.22438/jeb/42/2/MRN-1481
Devi, A. G., Rangappa, K., Yadav, G. S., Devi, H. L., Barman, K. K., Kandpal, B. K., et al. (2018). Physiological tolerance mechanism of selected rice germplasm of Northeast India to iron toxicity. Indian J. Hill Farm. 31, 75–81.
Dhawan, G., Kumar, A., Dwivedi, P., Gopala Krishnan, S., Pal, M., Vinod, K. K., et al. (2021). Introgression of qDTY1. 1. Agronomy 11:202. doi: 10.3390/agronomy11020202
Dobermann, A., and Fairhurst, T. (2000). Rice: Nutrient disorders and nutrient management, International Rice Research Institute: Los Baños.
Dotaniya, M. L., Meena, H. M., Lata, M., and Kumar, K. (2013). Role of phyto-siderophores in iron uptake by plants. Agric. Sci. Dig. 33, 73–76.
Dufey, I., Draye, X., Lutts, S., Lorieux, M., Martinez, C., and Bertin, P. (2015). Novel QTLs in an interspecific backcross Oryza sativa× Oryza glaberrima for resistance to iron toxicity in rice. Euphytica 204, 609–625. doi: 10.1007/s10681-014-1342-7
Dufey, I., Hakizimana, P., Draye, X., Lutts, S., and Bertin, P. (2009). QTL mapping for biomass and physiological parameters linked to resistance mechanisms to ferrous iron toxicity in rice. Euphytica 167, 143–160. doi: 10.1007/s10681-008-9870-7
Dwivedi, P., Ramawat, N., Dhawan, G., Gopala Krishnan, S., Vinod, K. K., Singh, M. P., et al. (2021). Drought tolerant near isogenic lines (NILs) of Pusa 44 developed through marker assisted introgression of qDTY2.1 and qDTY3.1 enhances yield under reproductive stage drought stress. Agriculture 11:64. doi: 10.3390/agriculture11010064
Dziwornu, A. K., Shrestha, A., Matthus, E., Ali, B., Wu, L. B., and Frei, M. (2018). Responses of contrasting rice genotypes to excess manganese and their implications for lignin synthesis. Plant Physiology and Biochemistry 123, 252–259. doi: 10.1016/j.plaphy.2017.12.018
Elec, V., Quimio, C. A., Mendoza, R., Sajise, A. G. C., Beebout, S. E., Gregorio, G. B., et al. (2013). Maintaining elevated Fe 2+ concentration in solution culture for the development of a rapid and repeatable screening technique for iron toxicity tolerance in rice (Oryza sativa L.). Plant Soil. 372, 253–264. doi: 10.1007/s11104-013-1739-4
Engel, K., Asch, F., and Becker, M. (2012). Classification of rice genotypes based on their mechanisms of adaptation to iron toxicity. J. Plant. Nutr. Soil Sci. 175, 871–881. doi: 10.1002/jpln.201100421
Fageria, N. K., Santos, A. B., Barbosa Filho, M. P., and Guimarães, C. M. (2008). Iron toxicity in lowland rice. J. Plant Nutr. 31, 1676–1697. doi: 10.1080/01904160802244902
Gamborg, O. L., and Wetter, L. R. (1975). Plant tissue culture methods. Saskatoon: National research council of Canada. Prairie regional laboratory.
Gridley, HE, Efisue, A, Tolou, B, and Bakayako, T (2006) Breeding for tolerance to iron toxicity at WARDA. Iron toxicity in rice-based systems in West Africa, Africa Rice Center (WARDA): Cotonou 96–111.
Hamim, H., Miftahudin, M., and Setyaningsih, L. (2018). “Cellular and ultrastructure alteration of plant roots in response to metal stress” in Plant growth and regulation-alterations to sustain unfavorable conditions, eds. D. Ratnadewi and Hamim (London: IntechOpen), 21–41.
Harahap, S. M., Ghulamahdi, M., Aziz, S. A., and Sutandi, A. (2014). Relationship of ethylene production and aerenchyme formation on oxidation ability and root surfaced-iron (Fe+2) accumulation under different iron concentrations and rice genotypes. Int. J. Appl. Sci. Technol. 4, 186–194.
Hoagland, D. R., and Arnon, D. I. (1950). The water-culture method for growing plants without soil. Circular. Calif. Agric. Exp. Stn. 347:32.
Hoan, N. T., Prasada Rao, U., and Siddiq, E. A. (1992). Genetics of tolerance to iron chlorosis in rice. Plant Soil. 146, 233–239. doi: 10.1007/BF00012017
Houle, D. (1998). How should we explain variation in the genetic variance of traits? Genetica 102, 241–253. doi: 10.1023/A:1017034925212
Hussain, H. A., Men, S., Hussain, S., Zhang, Q., Ashraf, U., Anjum, S. A., et al. (2020). Maize tolerance against drought and chilling stresses varied with root morphology and antioxidative defense system. Plants 9:720. doi: 10.3390/plants9060720
Jaggard, K. W., Qi, A., and Ober, E. S. (2010). Possible changes to arable crop yields by 2050. Phil. Trans. R. Soc. B. 365, 2835–2851. doi: 10.1098/rstb.2010.0153
Joshi, N., Pappula Reddy, S. P., Kumar, N., Bharadwaj, C., Tapan, K., Patil, B. S., et al. (2023). Siphoning novel sources of seedling salinity tolerance from the diverse chickpea landraces. Crop Pasture Sci. 74, 1080–1093. doi: 10.1071/CP22319
Kabir, A. H., Begum, M. C., Haque, A., Amin, R., Swaraz, A. M., Haider, S. A., et al. (2016). Genetic variation in Fe toxicity tolerance is associated with the regulation of translocation and chelation of iron along with antioxidant defence in shoots of rice. Funct. Plant Biol. 43, 1070–1081. doi: 10.1071/FP16068
Kakar, N., Jumaa, S. H., Redoña, E. D., Warburton, M. L., and Reddy, K. R. (2019). Evaluating rice for salinity using pot-culture provides a systematic tolerance assessment at the seedling stage. Rice 12, 57–14. doi: 10.1186/s12284-019-0317-7
Kar, S., and Panda, S. K. (2020). Iron homeostasis in rice: deficit and excess. Proc. Natl. Acad. Sci. India Sect. B Biol. Sci. 90, 227–235. doi: 10.1007/s40011-018-1052-3
Kirk, G. J., Manwaring, H. R., Ueda, Y., Semwal, V. K., and Wissuwa, M. (2022). Below-ground plant–soil interactions affecting adaptations of rice to iron toxicity. Plant Cell Environ. 45, 705–718. doi: 10.1111/pce.14199
Kobayashi, N. I., Ogura, T., Takagi, K., Sugita, R., Suzuki, H., Iwata, R., et al. (2018). Magnesium deficiency damages the youngest mature leaf in rice through tissue-specific iron toxicity. Plant Soil. 428, 137–152. doi: 10.1007/s11104-018-3658-x
Krzywinski, M., Schein, J., Birol, I., Connors, J., Gascoyne, R., Horsman, D., et al. (2009). Circos: an information aesthetic for comparative genomics. Genome Res. 19, 1639–1645. doi: 10.1101/gr.092759.109
Li, G., Kronzucker, H. J., and Shi, W. (2016a). Root developmental adaptation to Fe toxicity: mechanisms and management. Plant Signal. Behav. 11:e1117722. doi: 10.1080/15592324.2015.1117722
Li, G., Kronzucker, H. J., and Shi, W. (2016b). The response of the root apex in plant adaptation to iron heterogeneity in soil. Front. Plant Sci. 7:344. doi: 10.3389/fpls.2016.00344
Li, W., and Lan, P. (2017). The understanding of the plant iron deficiency responses in strategy I plants and the role of ethylene in this process by omic approaches. Front. Plant Sci. 8:40. doi: 10.3389/fpls.2017.00040
Ma, C., Burd, S., and Lers, A. (2015). Mi R 408 is involved in abiotic stress responses in Arabidopsis. Plant J. 84, 169–187. doi: 10.1111/tpj.12999
Mackill, D. J., and Khush, G. S. (2018). IR64: a high-quality and high-yielding mega variety. Rice 11, 1–11. doi: 10.1186/s12284-018-0208-3
Mahender, A., Swamy, B. M., Anandan, A., and Ali, J. (2019). Tolerance of iron-deficient and-toxic soil conditions in rice. Plants 8:31. doi: 10.3390/plants8020031
Mahmoudi, H., Labidi, N., Ksouri, R., Gharsalli, M., and Abdelly, C. (2007). Differential tolerance to iron deficiency of chickpea varieties and Fe resupply effects. C. R. Biol. 330, 237–246. doi: 10.1016/j.crvi.2007.02.007
Mamidi, S., Lee, R. K., Goos, J. R., and McClean, P. E. (2014). Genome-wide association studies identifies seven major regions responsible for iron deficiency chlorosis in soybean (Glycine max). PLoS One 9:e107469. doi: 10.1371/journal.pone.0107469
Marschner, H., and Römheld, V. (1995). “Strategies of plants for acquisition of iron” in Iron nutrition in soils and plants. Developments in plant and soil sciences. ed. J. Abadía, vol. 59 (Dordrecht: Springer)
Matthus, E., Wu, L. B., Ueda, Y., Höller, S., Becker, M., and Frei, M. (2015). Loci, genes, and mechanisms associated with tolerance to ferrous iron toxicity in rice (Oryza sativa L.). Theor. Appl. Genet. 128, 2085–2098. doi: 10.1007/s00122-015-2569-y
Mongon, J., Chaiwong, N., Bouain, N., Prom-U-Thai, C., Secco, D., and Rouached, H. (2017). Phosphorus and iron deficiencies influences rice shoot growth in an oxygen dependent manner: insight from upland and lowland rice. Int. J. Mol. Sci. 18:607. doi: 10.3390/ijms18030607
Müller, J., Toev, T., Heisters, M., Teller, J., Moore, K. L., Hause, G., et al. (2015). Iron-dependent callose deposition adjusts root meristem maintenance to phosphate availability. Dev. Cell 33, 216–230. doi: 10.1016/j.devcel.2015.02.007
Nozoe, T., Agbisit, R., Fukuta, Y., Rodriguez, R., and Yanagihara, S. (2008). Characteristics of iron tolerant rice lines developed at IRRI under field conditions. JARQ 42, 187–192. doi: 10.6090/jarq.42.187
Nugraha, Y., Ardie, S. W., Ghulammahdi, M., Suwarno,, and Aswidinnoor, H. (2016a). Nutrient culture media with agar is effective for early and rapid screening of iron toxicity tolerance in rice. J. Crop Sci. Biotechnol. 19, 61–70. doi: 10.1007/s12892-015-0075-z
Nugraha, Y., Utami, D. W., Rosdianti, I. D. A., Ardie, S. W., Ghulammahdi, M., Suwarno, S., et al. (2016b). Markers-traits association for iron toxicity tolerance in selected Indonesian rice varieties. Biodiversitas 17, 753–763. doi: 10.13057/biodiv/d170251
Nurmalasari, A. I., Putra, E. T. S., and Yudono, P. (2016). Root morphology of eight hybrid oil palms under iron (Fe) toxicity. Ilmu Pertanian 1, 013–018. doi: 10.22146/ipas.11254
Onaga, G., Dramé, K. N., and Ismail, A. M. (2016). Understanding the regulation of iron nutrition: can it contribute to improving iron toxicity tolerance in rice? Funct. Plant Biol. 43, 709–726. doi: 10.1071/FP15305
Onaga, G., Edema, R., and Asea, G. (2013). Tolerance of rice germplasm to iron toxicity stress and the relationship between tolerance, Fe2+, P and K content in the leaves and roots. Arch. Agron. Soil Sci. 59, 213–229. doi: 10.1080/03650340.2011.622751
Onyango, D. A., Entila, F., Dida, M. M., Ismail, A. M., and Drame, K. N. (2018). Mechanistic understanding of iron toxicity tolerance in contrasting rice varieties from Africa: 1. Morpho-physiological and biochemical responses. Funct. Plant Biol. 46, 93–105. doi: 10.1071/FP18129
Pathirana, R., Wijithawarna, W. A., Jagoda, K., and Ranawaka, A. L. (2002). Selection of rice for iron toxicity tolerance through irradiated caryopsis culture. Plant Cell Tissue Organ Cult. 70, 83–90. doi: 10.1023/A:1016025728740
Pawar, S., Pandit, E., Arjun, P., Wagh, M., Bal, D., Panda, S., et al. (2017). Genetic variation and association of molecular markers for iron toxicity tolerance in rice. ORYZA-An International Journal on Rice 54, 356–366. doi: 10.5958/2249-5266.2017.00066.2
Reddy, M. A., Francies, R. M., Abida, P. S., and Kumar, P. S. (2019). Correlation among morphological, biochemical and physiological responses under iron toxic conditions in rice. Int. J. Curr. Microbiol. App. Sci. 8, 37–44. doi: 10.20546/ijcmas.2019.801.005
Reddy, M. A., Francies, R. M., Elsy, C. R., Jiji, J., Kumar, P. S., and Abida, P. S. (2015). Screening of rice genotypes for iron toxicity tolerance through hydroponics. Trends Biosci. 8, 3087–3090.
Reyt, G., Boudouf, S., Boucherez, J., Gaymard, F., and Briat, J.-F. (2015). Iron- and ferritin-dependent reactive oxygen species distribution: impact on Arabidopsis root system architecture. Mol. Plant 8, 439–453. doi: 10.1016/j.molp.2014.11.014
Rinyu, E., Varga, J., and Ferenczy, L. (1995). Phenotypic and genotypic analysis of variability in Aspergillus fumigatus. J. Clin. Microbiol. 33, 2567–2575. doi: 10.1128/jcm.33.10.2567-2575.1995
Robinson, N. J., Procter, C. M., Connolly, E. L., and Guerinot, M. L. (1999). A ferric-chelate reductase for iron uptake from soils. Nature 397, 694–697. doi: 10.1038/17800
Rout, G. R., Sunita, S., Das, A. B., and Das, S. R. (2014). Screening of iron toxicity in rice genotypes on the basis of morphological, physiological and biochemical analysis. J. Exp. Biol. Agric. 2, 567–582.
Rumanti, I., Wening, R. H., and Sitaresmi, T. (2017). Leaf bronzing symptom score as related to grain yield of rice under iron toxicity of acid-sulfate soil area. Proceedings of PERIPI-2017 International Seminar, October 2nd 2017, Bogor, Indonesia. 154–161.
Sahrawat, K. L., Mulbah, C. K., Diatta, S., Delaune, R. D., Patrick, W. H., Singh, B. N., et al. (1996). The role of tolerant genotypes and plant nutrients in the management of iron toxicity in lowland rice. J. Agric. Sci. 126, 143–149. doi: 10.1017/S002185960007307X
Saikia, T., and Baruah, K. K. (2012). Iron toxicity tolerance in rice (Oryza sativa) and its association with anti-oxidative enzyme activity. J. Crop Sci. 3:90.
Saleem, A., Zulfiqar, A., Saleem, M. Z., Ali, B., Saleem, M. H., Ali, S., et al. (2023). Alkaline and acidic soil constraints on iron accumulation by Rice cultivars in relation to several physio-biochemical parameters. BMC Plant Biol. 23:397. doi: 10.1186/s12870-023-04400-x
Santos, F. I. D. C. D., Marini, N., Santos, R. S. D., Hoffman, B. S. F., Alves-Ferreira, M., and de Oliveira, A. C. (2018). Selection and testing of reference genes for accurate RT-qPCR in rice seedlings under iron toxicity. PLoS One 13:e0193418. doi: 10.1371/journal.pone.0193418
Shimizu, A., Guerta, C. Q., Gregorio, G. B., Kawasaki, S., and Ikehashi, H. (2005). QTLs for nutritional contents of rice seedlings (Oryza sativa L.) in solution cultures and its implication to tolerance to iron-toxicity. Plant Soil 275, 57–66. doi: 10.1007/s11104-004-4683-5
Sikirou, M., Saito, K., Achigan-Dako, E. G., Drame, K. N., Ahanchede, A., and Venuprasad, R. (2015). Genetic improvement of iron toxicity tolerance in rice-progress, challenges and prospects in West Africa. Plant Prod. Sci. 18, 423–434. doi: 10.1626/pps.18.423
Sikirou, M., Saito, K., Dramé, K. N., Saidou, A., Dieng, I., Ahanchédé, A., et al. (2016). Soil-based screening for iron toxicity tolerance in rice using pots. Plant Prod. Sci. 19, 489–496. doi: 10.1080/1343943X.2016.1186496
Silveira, V. C. D., Oliveira, A. P. D., Sperotto, R. A., Espindola, L. S., Amaral, L., Dias, J. F., et al. (2007). Influence of iron on mineral status of two rice (Oryza sativa L.) cultivars. Braz. J. Plant Physiol. 19, 127–139. doi: 10.1590/S1677-04202007000200005
Singh, V. J., Bhowmick, P. K., Vinod, K. K., Krishnan, S. G., Nandakumar, S., Kumar, A., et al. (2022). Population structure of a worldwide collection of tropical japonica rice indicates limited geographic differentiation and shows promising genetic variability associated with new plant type. Genes 13:484. doi: 10.3390/genes13030484
Siriwardana, K. G. D. I., Weerasinghe, W. D. P., Priyantha, G. D. A., Chandrasekara, K. K. D., Rupasinghe, M. D. N., Wickramasinghe, W. R. K. D. W. K. V., et al. (2018). Screening of selected rice varieties and advanced breeding lines against iron toxicity under field conditions in the low country wet zone of Sri Lanka. Trop. Agric. Res. 30, 33–46. doi: 10.4038/tar.v30i2.8307
Sitaresmi, T., Dewi, K., Rumanti, I. A., Herlinda, S., and Nugraha, Y. (2021). Response of Indonesian rice varieties to iron toxicity under field and green house condition. In E3S Web of Conferences 306, 01012. E3S Web Conf. 306, 01012
Suma, A., Francies, R. M., Radhakrishnan, V. V., Sureshkumar, P., and Varghese, E. (2022). Elucidating the response of rice genotypes to iron toxicity through hydroponics. Ind. J. Plant Genet. Res. 35, 66–72. doi: 10.5958/0976-1926.2022.00010.9
Theerawitaya, C., Wanchana, S., Ruanjaichon, V., Tisaram, R., Samphumphuang, T., Sotesaritkul, T., et al. (2022). Determination of traits responding to iron toxicity stress at different stages and genome-wide association analysis for iron toxicity tolerance in rice (Oryza sativa L.). Front. Plant Sci. 13:994560. doi: 10.3389/fpls.2022.994560
Timmer, C. P., Block, S., and Dawe, D. (2010). “Long-run dynamics of rice consumption, 1960-2050” in Rice in the global economy: Strategic research and policy issues for food security. eds. S. Pandey, D. Byerlee, D. Dawe, A. Dobermann, S. Mohanty, and S. Rozelle, et al. (Los Baños, Philippines: International Rice Research Institute), 139–173.
Turhadi, T., Hamim, H., Ghulamahdi, M., and Miftahudin, M. (2018). Morpho-physiological responses of rice genotypes and its clustering under hydroponic iron toxicity conditions. Asian J. Agric. Biol. 6, 495–505.
Turhadi, T., Hamim, H., Ghulamahdi, M., and Miftahudin, M. (2019). Iron toxicity-induced physiological and metabolite profile variations among tolerant and sensitive rice varieties. Plant Signal. Behav. 14:1682829. doi: 10.1080/15592324.2019.1682829
Vasconcelos, M. W., and Grusak, M. A. (2014). Morpho-physiological parameters affecting iron deficiency chlorosis in soybean (Glycine max L.). Plant Soil 374, 161–172. doi: 10.1007/s11104-013-1842-6
Vose, P. B. (1982). Iron nutrition in plants: a world overview. J. Plant. Nutr. 5, 233–249. doi: 10.1080/01904168209362954
Wan, J. L., Zhai, H. Q., Wan, J. M., and Ikehashi, H. (2003). Detection and analysis of QTLs for ferrous iron toxicity tolerance in rice, Oryza sativa L. Euphytica 131, 201–206. doi: 10.1023/A:1023915710103
Ward, J. T., Lahner, B., Yakubova, E., Salt, D. E., and Raghothama, K. G. (2008). The effect of iron on the primary root elongation of Arabidopsis during phosphate deficiency. Plant Physiol. 147, 1181–1191. doi: 10.1104/pp.108.118562
Waters, B. M., and Eide, D. J. (2002). Combinatorial control of yeast FET4 gene expression by iron, zinc, and oxygen. J. Biol. Chem. 277, 33749–33757. doi: 10.1074/jbc.M206214200
Wissuwa, M., Ismail, A. M., and Yanagihara, S. (2006). Effects of zinc deficiency on rice growth and genetic factors contributing to tolerance. Plant Physiol. 142, 731–741. doi: 10.1104/pp.106.085225
Wu, P., Luo, A., Zhu, J., Yang, J., Huang, N., and Senadhira, D. (1997). Molecular markers linked to genes underlying seedling tolerance for ferrous iron toxicity. Plant Soil 196, 317–320. doi: 10.1023/A:1004288427140
Wu, L. B., Shhadi, M. Y., Gregorio, G., Matthus, E., Becker, M., and Frei, M. (2014). Genetic and physiological analysis of tolerance to acute iron toxicity in rice. Rice 7, 8–12. doi: 10.1186/s12284-014-0008-3
Wu, L. B., Ueda, Y., Lai, S. K., and Frei, M. (2017). Shoot tolerance mechanisms to iron toxicity in rice (Oryza sativa L.). Plant Cell Environ. 40, 570–584. doi: 10.1111/pce.12733
Zhang, J., Chen, K., Pang, Y., Naveed, S. A., Zhao, X., Wang, X., et al. (2017). QTL mapping and candidate gene analysis of ferrous iron and zinc toxicity tolerance at seedling stage in rice by genome-wide association study. BMC Genom. 18, 828–815. doi: 10.1186/s12864-017-4221-5
Keywords: iron toxicity, root system response, tolerance, hydroponics, seedling stage
Citation: Nandakumar S, Singh VJ, Pandey R, Gopala Krishnan S, Bhowmick PK, Ellur RK, Bollinedi H, Harshitha BS, Yadav S, Beniwal R, Nagarajan M, Singh AK and Vinod KK (2024) Implications of tolerance to iron toxicity on root system architecture changes in rice (Oryza sativa L.). Front. Sustain. Food Syst. 7:1334487. doi: 10.3389/fsufs.2023.1334487
Edited by:
Md Khairul Alam, Bangladesh Agricultural Research Institute, BangladeshReviewed by:
Heena Rani, Agricultural Research Service (USDA), United StatesJoong Hyoun Chin, Sejong University, Republic of Korea
Naveen Puppala, New Mexico State University, United States
Santosh Kumar Gupta, National Institute of Plant Genome Research (NIPGR), India
Copyright © 2024 Sonu, Nandakumar, Singh, Pandey, Gopala Krishnan, Bhowmick, Ellur, Bollinedi, Harshitha, Yadav, Beniwal, Nagarajan, Singh and Vinod. This is an open-access article distributed under the terms of the Creative Commons Attribution License (CC BY). The use, distribution or reproduction in other forums is permitted, provided the original author(s) and the copyright owner(s) are credited and that the original publication in this journal is cited, in accordance with accepted academic practice. No use, distribution or reproduction is permitted which does not comply with these terms.
*Correspondence: Kunnummal Kurungara Vinod, a2t2aW5vZGhAZ21haWwuY29t