- 1School of Textile Science and Engineering, Zhejiang Sci-Tech University, Hangzhou, China
- 2School of Economics, Shandong University, Shandong, China
- 3School of Economics, Shandong University of Finance and Economics, Shandong, China
- 4School of Economics, Qufu Normal University, Shandong, China
Large scale agricultural business entities (hereinafter referred to as “the large scale entities”) are newly sprouted in the process of China’s agricultural transformation from traditional production to modern mode, while the improvement of agricultural total factor productivity is an important driving force to realize agricultural transformation in China. Therefore, it is of great significance to explore whether the former has a promoting effect on the latter. First of all, the effective improvement of agricultural total factor productivity by the large scale entity development is analyzed from the two aspects of scale effect and technology utilization effect, and the effect mechanism is discussed in depth in this paper. Secondly, empirical test was carried out by using the panel data of prefecture-level cities from 2015 to 2020. The benchmark regression results show that the development of large scale entities can significantly improve agricultural total factor productivity. The mechanism test results indicate that the development of large scale entities improves the agricultural total factor productivity by raising the agricultural mechanization level. The results of heterogeneity analysis show that the difference between large scale entities would affect the benchmarking results. Therefore, it is believed through the study as discussed in this paper that devoting greater effort to support the development of large scale entities is an important measure to improve agricultural total factor productivity and promote China’s agricultural transformation, but it is necessary to act according to local conditions based on the difference of entity types in the process.
1 Introduction
In the critical period of China’s modern agricultural transformation, exploring the important driving factors of improving agricultural total factor productivity is of great significance to the construction of modern agriculture and solving the problems of “agriculture, rural areas and farmers.” Agricultural total factor productivity refers to the development and utilization efficiency of factors related to agricultural production, including land, labor, capital and technology. From the perspective of connotation, the direct way to improve agricultural total factor productivity is to improve the utilization efficiency of production factors, and the key lies in the ability of agricultural production and management entities to make full use of production factors. Therefore, it is of great practical significance to explore whether the development of large scale agricultural business entities (hereinafter referred to as “the large scale entities”) at this stage is conducive to the improvement of agricultural total factor productivity.
The large scale entities refer to the professional farmers with culture, technical knowledge and management skills, and the agricultural management organizations managing on large scale with high degree of intensification and market competitiveness based on the improvement of the household contract responsibility system. From 2012 to 2022, the national government or central government of China had put forward guidance on accelerating the establishment of a large scale agribusiness system in its number 1 document each year, provided substantial support and policy environment for the sustainable development of the large scale entities, and has so far established a relatively complete policy system. Thus it can be seen that the government attaches great importance to the development of the large scale entities, and it is of great significance to explore its role in China’s agricultural transformation and whether it can improve agricultural total factor productivity.
On the basis of policy support, the large scale entities have risen and drawn much attention from the academic community, and many scholars have begun to explore its economic effects, mainly centering around the impact on land transfer, labor employment, and peasant life. In the first place, the large scale entities shall expand the business scale by renting a large amount of land to meet the demand for land elements (Jayne et al., 2016) and promote the transfer of agricultural land from farmers on a small scale to the large scale entities (Sipangule, 2017) from the perspective of land transfer. In the second palce, the large scale entities also need enough labor force to engage in agricultural production after having acquired land elements, so the large scale entities can recruit surplus rural labor (Nolte and Ostermeier, 2017). And besides their own needs, their driving effect on agricultural product processing, logistics, sales and other related industries creates more employment opportunities, thus attracting more rural surplus labor force to be hired (Burke et al., 2020). At the same time, land transfer by the large scale entities frees a large number of agricultural labor and promotes its transfer to non-agricultural sectors of higher pay rates (Deininger et al., 2011). Thirdly, the emergence of the large scale entities also has profound impact on farmers’ lives. For example, the business pattern of “agricultural cooperatives + farmers” can reduce the transport cost and expenses paid by farmers for collecting market information, and increase the net income of farmers (Bernard and Spielman, 2009; Sanjaya et al., 2021). In addition to reducing costs and increasing incomes, the connecting mechanism between the large scale entities and farmers helps to solve the problems of hidden unemployment and low income in agriculture (Ito et al., 2012), and thus has a certain effect of poverty alleviation (Herrmann, 2016; Lingjuan et al., 2021). However, the research perspective of the above literature is limited to discussing its development background and its impact on land transfer, labor employment, peasant life and other aspects. And little attention is paid to whether the development of the large scale entities can improve agricultural total factor productivity and whether it is more efficient than the traditional production by farmers on a small scale. In this view, this paper analyzes its advantages from the two aspects of scale effect and technology utilization effect, points out that the development of the large scale entities can improve agricultural total factor productivity; discusses its effect mechanism from the aspect of raising agricultural mechanization degree; and provides a large scale perspective for the relevant literatures which study the economic effects of the large scale entities.
Based on the above analysis, researchers have tested the economic effects of the large scale entities from multiple perspectives, but its efficiency promoting effect is rarely discussed in literatures. On the road of agricultural transformation in China, the improvement of agricultural total factor productivity is one of the key points that need to be concerned. Existing literature on agricultural total productivity mainly measures the agricultural total productivity of various provinces and analyzes its trend in China and various provinces based on econometric methods such as Stochastic Frontier Approach (SFA) and Data Envelopment Analysis (DEA) (Gong, 2018; Zhang et al., 2023); other studies also concern specific measures to improve agricultural total productivity (Sheng et al., 2019). However, ensuring the steady improvement of agricultural production efficiency and resource utilization efficiency while successfully completing the transformation from a traditional small-scale peasant economy to a large-scale modern agricultural economy remains the focus and a difficulty for current policymakers and academics. Therefore, ways are explored in this paper to realize both successful transformation and efficiency improvement of China’s agricultural economy by studying whether the large scale entities are capable of improving agricultural total factor productivity. To further enrich relevant research and provide a large scale theoretical perspective for improving agricultural total factor productivity.
2 Theoretical hypothesis
In terms of policy, China mainly cultivates the large scale entities by supplying fund, labor, land and other agricultural production factors, guiding the rational allocation of resources, and solving the problem of increasing the quantity and improving the quality of agricultural supply under the condition of limited agricultural factor resources to satisfy the exuberant demand of entities. Therefore, from the perspective of policy orientation, the development of the large scale entities in China will help to improve agricultural total factor productivity, and the specific principles include scale effect and technology utilization effect.
2.1 Scale effect
The main difference between the business models of large scale entities and individual farmers lies in that the former is on a larger scale and can generate strong scale effect no matter it is leading specialized household, family farm, specialized farmer cooperatives or agricultural leading enterprise. Scale effect refers to the situation where the output increases and the long-term average cost of a company decreases due to the expansion of its scale. From the perspective of economic history, scale effect originates with Adam Smith. Adam Smith believes that the division of labor can improve the skill and proficiency of each worker, save the time wasted by changing jobs, and facilitate the invention and application of machines. Since the division of labor is based on mass production at a certain scale, Adam Smith’s theory can be said to be a classical explanation of the effects of economies of scale on firms. The true scale effect originates in the United States. Alfred Marshall is one of the representative figures who believes that scale expansion is beneficial for enterprises to fully utilize resources and improve operational efficiency, but it cannot be uncontrolled expansion, otherwise it will lead to monopoly. On the basis of the above theory, many scholars have conducted research on the scale effect of enterprises. Schumpeter (1942), Beeson (1987) and Moomaw (1981) believe that compared to small enterprises, large enterprises have comparative advantages in economies of scale, risk sharing, and financing channels. Sveikauskas (1975) and Sleuwaegen and Goedhuys (2002) found that the larger a company is, the more capable it is to purchase advanced technological equipment, have sufficient funds to engage in high-risk technological innovation activities, and provide sufficient vocational training for personnel. This is beneficial for improving the productivity level of enterprises. The scale effect is more significant among large-scale agricultural operators. Qu et al. (2022) use data from Chinese farmers to study and find that the scale and mechanization of agricultural operations can improve the efficiency of rice harvesting outsourcing services. Xin and Li (2019) believe that the expansion of agricultural business scale will enhance China’s agricultural competitiveness. From existing research, it can be seen that the development of large-scale agricultural operators in China will improve agricultural production efficiency. While supporting the development of large scale entities, China emphasizes “appropriate scale,” which means not only the defects of small scale and low investment in traditional household business shall be overcome, but business scale shall not be pursued blindly. Instead, equal attention shall be paid to scale economy and earth give rate (Wang et al., 2021). Therefore, the large scale entities have larger scale, greater input–output ratio, more wage labor, and other features. According to the theory of scale economy, the integrity of the industrial chain and the improvement of resource allocation and regeneration efficiency will increase the marginal benefit of the business entities when they reach a certain scale, that is, the effect of 1 + 1 > 2 is generated due to the organic combination of various production factors. This scale effect improves the use efficiency of various production factors through effectively allocating resources, and thus improving the total factor productivity.
2.2 Technology utilization effect
According to the large scale economic growth theory, technological progress is an important driving force to promote economic growth and productivity. However, even if the technology progresses, it is also difficult to give play to the driving effect of technological progress without corresponding utilizer. Therefore, the development of technology utilizers is equally important. According to the Technology Acceptance Model (TAM) proposed by Davis (1989), if users find a technology useful and easy to use, they are more likely to adopt it, while if the technology is not easy to use or has complex operations, no one will hold a positive attitude toward it. This property is called perceived ease of use. However, there are subjective differences in perceived ease of use (Venkatesh et al., 2003; Mostafa, 2023). As for enterprises, compared to small-scale enterprises, large-scale enterprises have more sufficient capital, human resources, and production scale, so their perceived ease of use is lower. Therefore, large-scale enterprises are more likely to accept and utilize more complex technologies. This characteristic also applies to agricultural business entities. Due to the limited individual funds and the relatively dispersed farmland of farmers on a small scale, traditional farming techniques can basically meet the requirements of production, while the introduction of large scale technologies and frequent training may cause efficiency loss because of working time delay (Deininger and Fang, 2016). Therefore, it is more costly for farmers on a small scale to improve the total factor productivity by introducing modern agricultural technology. In addition, statistics show that the cultivated area of most farmers in China is less than 10 mu and relatively scattered (Li et al., 2022), which restricts the production efficiency and makes it difficult to realize large-scale agricultural mechanization. On the one hand, such large scale entities as leading specialized households and family farms have sufficient funds, and the scale effect and high prospective earnings create a better financing environment for them (Chamberlin and Jayne, 2019). Large-scale centralized training costs less and has higher returns. As a result, they have the ability and motivation to introduce large scale technologies; On the other hand, contiguous tracts of farmland are more favorable for large-scale agricultural machinery to carry out operations and improve production efficiency. For example, the introduction of large harvesters and sowers will greatly improve the efficiency of crop harvesting. Therefore, the large scale entities can make full use of advanced agricultural technology and improve agricultural total factor productivity.
Based on the above analysis, hypothesis H1 is proposed: Under the effect of scale effect, large scale entities have higher resource utilization efficiency, better division of labor models, and more effective business models, which can improve the efficiency of the use of various production factors and thus enhance total factor productivity. Under the effect of technological utilization, large scale entities can utilize their financial and continuous planting advantages to introduce agricultural technology and improve agricultural total factor productivity. Therefore, large scale entities can effectively improve agricultural total factor productivity under scale effect and technology utilization effect.
2.3 Analysis on the effect mechanism
The facilitating effect of agricultural mechanization. The large scale entities can improve agricultural total factor productivity by raising the level of agricultural mechanization. First of all, the large-scale production model of the large scale entities can raise the level of agricultural mechanization and improve machinery utilization efficiency. With the acceleration of industrialization and urbanization in China, agricultural labor force is decreasing. And under such a background, agricultural machinery has gradually become a “large scale labor force” replacing human labor. However, the land fragmentation caused by the popular individual small-scale farming in China and the situation of more people and less land has seriously hindered the development of agricultural mechanization. Not only the degree of mechanization is low, land fragmentation also leads to low machinery utilization efficiency. Moreover, the large scale entities can promote horizontally contiguous agricultural planting through land transfer or farmer cooperative, and remove the obstacles of land fragmentation to large-scale agricultural machinery (Abebaw and Haile, 2013; Ali et al., 2019). For example, leading specialized households and family farms can connect the fragmented land around into contiguously cultivated farmland through land transfer, which is conducive to operation by large-scale agricultural machinery; for another example, specialized farmer cooperatives can unite farmer owners of neighboring land for cooperating to introduce large agricultural machinery, thereby raising the level of mechanization. Therefore, the large scale entities have the role of raising the level of agricultural mechanization.
Secondly, raising the level of agricultural mechanization can improve agricultural total factor productivity. For one thing, compared with traditional manual operations, operating by agricultural machinery is more consistent and stable since it will not affect the quality of agricultural production due to the differences in physical conditions, technical skills and other factors of the labor force, and has a positive effect on the improvement of agricultural total factor productivity. In the meantime, operating by agricultural machinery is also more efficient as crop yields would be reduced if the best sowing and harvesting times slip away due to the obvious seasonality and short harvest time. For another, using agricultural machinery for production is cheaper than manual production. The acceleration of urbanization and industrialization has increased non-agricultural employment opportunities, and the loss of a large number of agricultural labor forces has raised the relative price of labor (Baumgartner et al., 2015). Therefore, raising agricultural mechanization level not only makes up for the lost agricultural labor, but also helps to save production cost, and is more stable and efficient. Based on the above analysis, the large scale entities can improve the agricultural total factor productivity by raising the level of agricultural mechanization.
Based on the above analysis, hypothesis H2 is proposed: the large scale entities can improve the agricultural total factor productivity by raising the level of agricultural mechanization.
3 Study design
3.1 Variable measures and data sources
Panel data of prefecture-level cities in China from 2015 to 2020 were used in this empirical study and manually collated by the author from the database of registration information concerning industrial and commercial enterprises in China, China City Statistical Yearbook, provincial and prefecture-level city statistical yearbooks, provincial water resource bulletins, as well as agricultural and rural statistical yearbooks. Some prefecture-level cities were eliminated from this paper since too many variables were missing. Finally, 6 years of panel data of 213 prefecture-level cities were obtained. The main variables are explained and measured as follows:
3.1.1 The explained (dependent) variable
The logarithm of total factor productivity (lnTFP). Stochastic Frontier Approach (SFA) and Malmquist productivity index were adopted to measure and calculate the agricultural total factor productivities in prefecture-level cities. The model by SFA method is set as follow:
Wherein, Yit represents the agricultural output of prefecture-level city i in the year t. Zit stands for the agricultural production factor input of prefecture-level city i in the year t. δ is the parameter to be estimated. νit stands for the random error assuming a normal distribution, νit ~ N (0, σν2); φit is the technical non-efficiency term assuming a truncated normal distribution, φit ~ N+(φ, σφ2), and measured by the time-varying model; νit is independent of φit. f represents a specific functional form. In this paper, translogarithmic production function is used to build SFA model of panel fixed effect as an easily estimable and highly inclusive production function model with variable elasticity and ability to effectively study the interactions of input factors in production functions and the differences in technological progress upon various inputs. Furthermore, according to the research of Gong (2018), China’s agricultural production conforms to the assumption of constant returns to scale. In order to satisfy this assumption and keep consistent with the symmetry of translogarithmic function, the input and output variables in the model were standardized by using land input, namely, the input and output per unit of land. Referring to the research of Fu and Zhang (2022) and Chen et al. (2023), the added value of the primary industry is chosen as the output variable (calculated at the constant price in 2014). The input variables include labor force (employees in the primary industry of the society at large) and capital (total power of agricultural machinery). The total area under crops is chosen as land input to standardize the input and output variables. It should be noted that there is no such data on agricultural intermediate inputs as fertilizer, seed, feed, energy and service fees at the prefecture-level city level, so the added value is chosen as the output variable to eliminate these intermediate inputs and reduce measurement errors. The SFA translogarithmic production function model thus obtained is
Wherein, Yit is the added value of the primary industry on the unit land, Kit represents the employee of primary industry on the unit land, Lit stands for the total power of agricultural machinery per unit of land, and the rest variables have the same meanings as in Equation (1). After estimating Equation (2), the agricultural technical efficiency (EFF) of prefecture-level city i in the year t can be calculated by the following equation:
Then, the agricultural technical efficiency change (EFFCHit) and the agricultural technical progress change (TECHit) of prefecture-level city i in the year t can be obtained, respectively, by the following two equations:
Under the assumption of constant returns to scale, the agricultural total factor productivity (TFPit) can be calculated by the following equation according to the decomposition of Malmquist productivity index:
3.1.2 The explanatory variable
The number of large scale entities (lnzt). The number of large scale entities comes from the database of registration information concerning industrial and commercial enterprises in China. In the first step, enterprises of large scale entities are chosen according to the classification of industry information, which include leading specialized households, agricultural product processing enterprises, specialized farmer cooperatives, and family farms. Leading specialized households: According to the classification of national industries, specialized production enterprises engaged in agriculture, forestry, animal husbandry and fishing, and specialized agricultural enterprises in industries of auxiliary activities are incorporated as the leading specialized households. Agricultural product processing enterprises: Statistically, it is generally considered that the ventures in agroindustry include such 12 major categories of industries in China as agricultural and sideline food processing industry; food manufacturing industry; wine, beverage and refined tea manufacturing industry; cigarettes and tobacco manufacturing industry; textile industry; textile and clothing industry; leather, fur, down and its products and shoe industry; wood processing and bamboo, rattan and palm grass products industry; furniture manufacturing industry; paper and paper products industry; printing and recording media reproduction industry; rubber and plastic products industry upon the classification of national industries. On this basis, the industries that are not closely related to agricultural products are excluded from this paper, which include salt mining, plastic products industry, textile and clothing industry, printing and recording media reproduction industry, and the two industries that are closely related to agricultural products processing industry, namely, traditional Chinese medicine drinks and Chinese patent medicine production in the pharmaceutical industry, are included herein. Specialized farmer cooperatives: As it is stipulated in Article 6 of the Regulations on the Registration Management of Specialized Farmer Cooperatives, the words “specialized cooperatives” must be shown in the their registered names. Based on this, such enterprises are included as specialized farmer cooperatives. Family farms: Family farms are chosen by the clue that the enterprise name contains “family farm” and “family pasture” according to the registration standards of family farms in various provinces. In the second step, the large scale entities chosen are totaled up according to the prefecture-level city and year identifier to get the annual number of large scale entities in each prefecture-level city.
3.1.3 Mediator variable
The agricultural mechanization level (lnjx). The total power of agricultural machinery is used to measure the total amount of machinery used in agricultural production. The greater the value, the more machinery is used, and the higher the mechanization level is.
3.1.4 Control variable
(1) The number of rural population (lnpop_xc) was used to control the influence of potential agricultural labor resources on the agricultural total factor productivity. (2) Gross regional product (lngdp) was used to control the influence of regional economic development level on the agricultural total factor productivity. (3) The primary industry’s production ratio (lnyczb) was used to control the primary industry dependence influence of regional development on the agricultural total factor productivity. (4) Regional fiscal expenditure (lnczzc) was used to control the effect of government support or fiscal subsidies on the agricultural total factor productivity. (5) The number of urban employees in the primary industry (lnyccz) was used to control the influence of the number of urban employees engaged in the primary industry on the agricultural total factor productivity. (6) The total water resources in the region (lnwaterz) was used to control the impact of available water resources on the agricultural total factor productivity.
3.2 Model design
In order to empirically test the theoretical logic and hypothesis of agricultural total factor productivity improvement by the above-mentioned large scale entities, the panel data of prefecture-level cities from 2015 to 2020 and fixed-effect model (FEM) are adopted for regression. And the specific model is set as follow:
Wherein, t is the year, and i represents the prefecture-level city (including the autonomous prefecture and league at the same level). lnTFPit means the agricultural total factor productivity of prefecture-level city i in the year t, and is the variable explained. lnztit stands for the number of large scale entities in prefecture-level city i in the year t, and is the core explanatory variable. lnXit represents a series of control variables of prefecture-level city i in the year t to control other factors that may affect the agricultural total factor productivity. β is the core coefficient concerned, and represents the direction and degree of the influence exerted by the development of the large scale entities on the agricultural total factor productivity. α is a constant, and γ is the coefficient of the control variable. λt represents the year fixed effect, μi is the individual fixed effect of the prefecture-level city, and εit means the error.
4 Model estimation and analysis
4.1 Descriptive statistics of the main variables
Before the model estimation, the article conducted descriptive statistics on each major variable. Descriptive statistics of the main variables are shown in Table 1.
4.2 Analysis on the benchmark regression results
In this paper, the effect of the development of large scale entities on agricultural total factor productivity was tested first, and the benchmark regression results are shown in Table 2. In Regression 1, the individual and year fixed effects in prefecture-level cities were controlled, and the results showed that the development of large scale entities significantly improved the agricultural total factor productivity by 5%. At this point, β equals 0.0015. In Regression 2, control was exerted on the individual and year fixed effects in prefecture-level cities and other variables that may have an impact on the agricultural total factor productivity, and the results showed that the development of large scale entities significantly improved the agricultural total factor productivity by 5%. At this point, β equals 0.0048. These results are consistent with the research conclusion drawn by Chari et al. (2022) using rural data in China, which suggests that the expansion of farmers’ production scale caused by rural land leasing activities can improve agricultural production efficiency. Meanwhile, these results are consistent with the research conclusion obtained by Chen (2017), which suggests that clear land ownership can promote land transactions between farmers, expand the scale of arable land, eliminate improper land allocation, and thereby improve agricultural productivity. Based on the above regression results, the development of large scale entities significantly improved agricultural total factor productivity, and the estimated values of core explanatory variable coefficients were not significantly different, indicating that the regression results were robust to a certain extent. According to the result of Regression 2, every 1% increase in the number of large scale entities will increase the agricultural total factor productivity by 0.0015%. And Hypothesis H1 is thus verified.
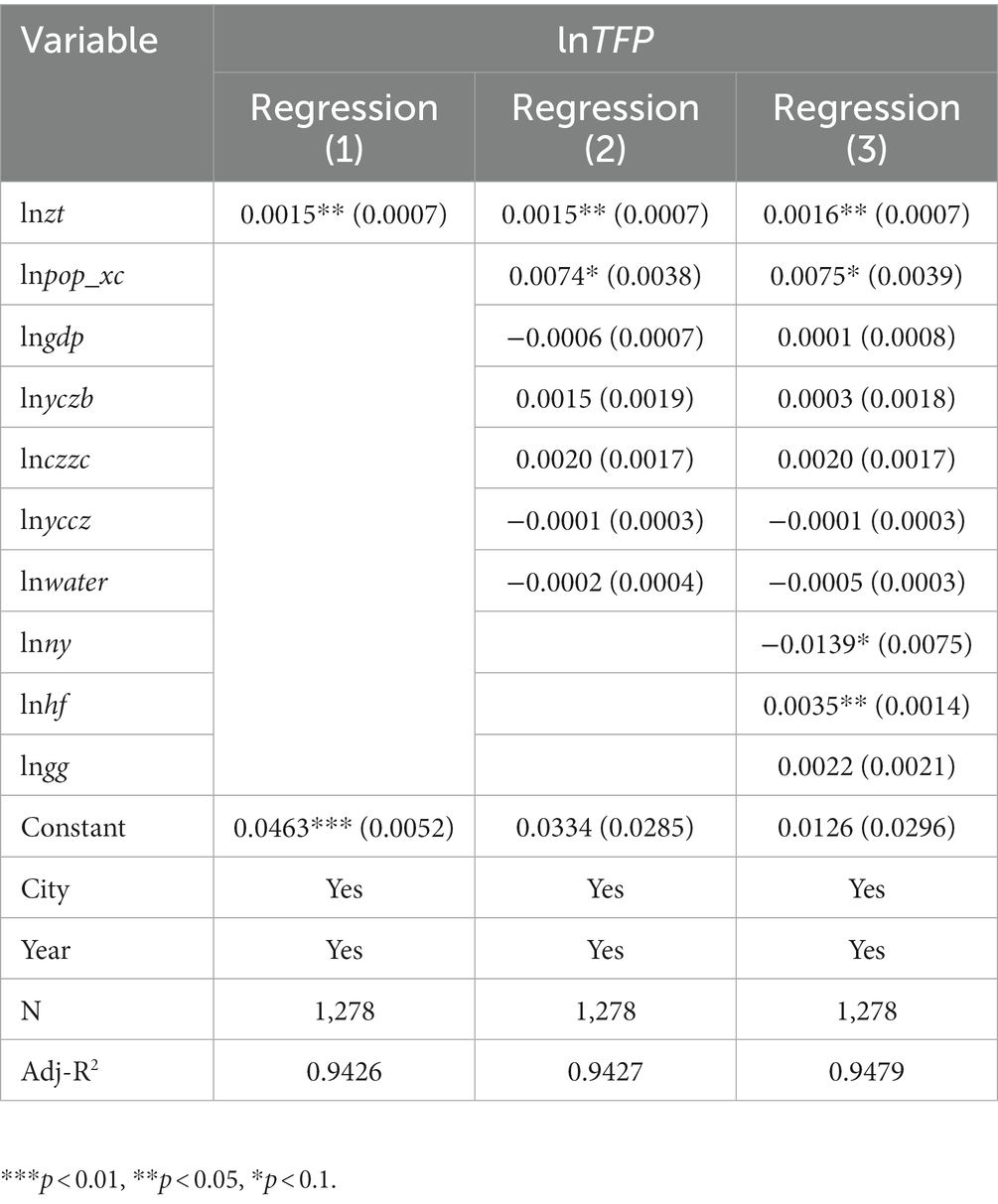
Table 2. The impact of large scale agricultural business entities on agricultural total factor productivity: benchmark regression results.
4.3 Robustness test
4.3.1 Control intermediate input variables
While measuring agricultural total factor productivities in prefecture-level cities, added value is adopted as the output variable, and this can effectively avoid measurement errors caused by missing intermediate input data. However, the use of agricultural intermediate inputs may still have an impact on the benchmark regression results, so intermediate input variables are best controlled in this paper to test the robustness of the benchmark regression results. The intermediate input variables include pesticide application amount (ny), fertilizer application amount (hf) and effective irrigation area (gg), and all of them are logarithms in regression. The data are collected from the statistical yearbooks of provincial and prefecture-level cities and rural statistical yearbooks of some provinces. Although some provinces or prefecture-level cities have statistical data of these three types of variables, they are not disclosed, and this part of data is collected by the author after inquiring local governments. The results are in Regression 3 in Table 2 and show that the development of large scale entities significantly improves the agricultural total factor productivity by 5%, with the β-value of 0.0016, and the estimated value of the coefficient does not change much, which verifies the robustness of the benchmark regression results.
4.3.2 Eliminate other policy impacts
Land and water are the two most basic and core ecological resource elements for agricultural production, and policy changes related to them may have an impact on the benchmark regression results, as a result, relevant impacts are eliminated by identifying such policies in this paper. First of all, collective construction land for commercial use entering the market, rural land expropriation, and pilot reform of the homestead system as land-related policies which would promote the transfer of agricultural land and improve the allocation of rural land factors, so there may be potential threats to the accuracy of the benchmark results. Therefore, the policy effect is identified to test whether the benchmark regression results are robust. Secondly, the national water-saving city appraisal policy is chosen as a policy related to water resources. The declaration and appraisal of national water-saving cities rise in response to the proper time and conditions for meeting the national requirements on water saving and promoting the high-quality and sustainable development of cities. Total water consumption is one of the important indicators of the appraisal criteria, and agricultural water consumption accounts for about 60% of the total water in the whole year. In order to meet the appraisal criteria, various cities may take measures to reduce agricultural water consumption and exert an impact on agricultural total factor productivity. To eliminate this policy effect, the dummy variable (jscs) of national water-saving cities was set. The appraised is set as 1, and others are 0, and model (7) was added for empirical test.
The results are shown from Regression 1 to 2 in Table 3. Regression 1 shows estimated results with the addition of dummy variable for such policies as collective construction land for commercial use entering the market. The results show that the coefficient of the number of large scale entities is significantly positive at the level of 5%, with the β-value of 0.0012, and the coefficient does not differ from the benchmark regression too much, which verifies the robustness of the benchmark regression results. Regression 2 shows estimated results with the addition of dummy variable for the policy of the national water-saving cities. The result shows that the coefficient of the policy dummy variable is significantly negative at the level of 10%. The reason may be that the policy makers will take measures to reduce agricultural water consumption in order to select the national water-saving cities, thus making it difficult for other resources such as cultivated land to obtain matching water, and bringing down the efficiency of resource utilization. However, the coefficient of the number of large scale entities is still significantly positive at the level of 5%, with the β-value of 0.0015, and the coefficient does not differ from the benchmark regression too much, indicating that the policy has little impact on the results of the benchmark regression and verifies the robustness of the benchmark regression results. Based on the above analysis, the results are still robust after having eliminated other policies that may affect the results of benchmark regression.
4.3.3 Endogeneity processing
While exploring the impact of the development of large scale entities on agricultural total factor productivity, endogeneity is an unavoidable problem. There may be a problem of self-selection between the development of large scale entities and agricultural total factor productivity, that is, regions with higher agricultural total factor productivity usually have higher levels of agricultural mechanization and informatization, and there are sufficient conditions to support the development of large scale entities, so the large scale entities in these regions develop fast. However, the regions with low agricultural total factor productivity may lack the corresponding conditions to support the development of large scale entities, which leads to the slow development of large scale entities. Therefore, there may be a problem of self-selection between the two. In this paper, the instrumental variable method is adopted to solve the problem above. Instrumental variables need to be exogenous and correlative, that is, they need to meet the two conditions of being related to the development of large scale entities and not affecting agricultural total factor productivity through other ways. However, it is not easy to find instrumental variables that meet the above two conditions at the same time, because the factors that affect the development of large scale entities usually affect agricultural production too, and thus affect agricultural total factor productivity. Based on this, Bartik IV (Shift-Share IV) instrumental variable method is adopted to construct instrumental variables. The principle of this method is to multiply an exogenous variable that changes only with the entity and another exogenous variable that changes only with time to obtain an instrumental variable that not only changes with the entity and with time, but also has correlation. Therefore, the reciprocal of the spherical distance between the prefecture-level city and Hangzhou is chosen as a variable that changes only with the entity, and the proportion of rural householders with junior high school degree of education or above at the national level as a variable that changes only with time. The reasons are as follows: The first is the reciprocal of the spherical distance from each prefecture-level city to Hangzhou. The development of large scale entities is greatly affected by the financing environment (The synthesis of various factors that affect the financing activities of enterprises. The better the financing environment, the easier it is for enterprises to raise funds.). Poor financing environment leads to a relative lack of capital sources, and it will be difficult for large scale entities to expand their production scale. The variables used to measure regional financing environment have correlation but are not exogenous. And the direct use of regional financing environment variables will also affect agricultural total factor productivity. Therefore, it is necessary to search for variables that can maintain correlation and enhance exogeneity. The reciprocal of the spherical distance from each prefecture-level city to Hangzhou is a good variable. Since the headquarters of Ant Financial is located in Hangzhou, the prefecture-level city closer to Hangzhou has a higher degree of digital finance and a better financing environment, while the variable of distance does not have a direct impact on the agricultural total factor productivity, so this variable not only has the correlation but also enhances the exogeneity, meeting the requirements on instrumental variables. The closer the distance to Hangzhou, the better the financing environment; and the smaller the variable value, the better the financing environment. In this paper, reciprocal processing of this variable is carried out to make the two have a proportional relationship, that is, the greater the reciprocal of the spherical distance between each prefecture level city and Hangzhou, the better the financing environment. The second is the proportion of rural householders with junior high school degree of education or above at the national level. The operation of the large scale entity requires the operator to have a certain degree of education, and the higher the degree of education a householder has, the better the large scale entity will develop. However, the degree of education of the householders at the national level does not have direct relationship with the effect of the agricultural total factor productivity in a certain region, so this variable also has correlation and enhances the exogeneity, meeting the requirements on instrumental variables. Based on the above two reasons, the product of the reciprocal of the spherical distance from the prefecture-level city to Hangzhou and the proportion of rural householders with junior high school degree of education or above at the national level (diswh) is chosen as an instrumental variable, which should be in the same direction as the development of large scale entities. And logarithm processing is carried out in empirical regression.
Regression results in the first stage show that the instrumental variables significantly promote the development of large scale entities at the 1% level. For instance, Kleibergen-Paap rk LM test results are significant at the 1% level, indicating that there is no insufficient identification of instrumental variables. The regression results of Kleib by instrumental variable method are shown in Table 4. Ergen-Paap rk Wald F-value is 18.0280, and greater than the critical value 16.3800 at the 10% level given by Stock and Yogo (2005), indicating that there is no weak instrumental variable. Therefore, the results of the first stage regression prove that the instrumental variables chosen satisfy the basic conditions. The results of the second stage regression show that the large scale entity development significantly promotes the agricultural total factor productivity at the 5% level. In general, after solving the problem of endogeneity by instrumental variable method, the development of large scale entities can still significantly improve agricultural total factor productivity, which further proves the robustness of the regression results.
4.4 Mechanism analysis
According to Hypothesis H2, the effect mechanism of large scale entities on agricultural total factor productivity is reflected in its improvement of agricultural mechanization. In order to verify the above research hypothesis, the mediating effect model is adopted for testing. The following mediating effect model is constructed:
Wherein, Formula (8) is a model to test the relationship between explanatory variables and mediating variables. Formula (9) is a model to test the relationship between the mediating variable and the explained variable. lnjxit is the mediating variable, agricultural mechanization (lnjx), and other symbols have the same meaning as formula (7). As expected, the large scale entities can improve agricultural mechanization, and then promote the agricultural productivity. Both β2 andβ3 should be greater than 0.
The resulting estimates are shown in Table 5, wherein, the coefficient of the number of large scale entities in Regression 1 is significantly positive at the level of 10%, with the β2-value of 0.0291, indicating that the development of large scale entities has improved the degree of agricultural mechanization. This result is consistent with the findings of Chen (2020) and Foster and Rosenzweig (2022), which suggest that expanding the scale of farmers’ operations or land use can improve the degree of agricultural mechanization. The coefficient of agricultural mechanization in Regression 2 is significantly positive at the level of 1%, with the β3-value of 0.0243, indicating that the improvement of agricultural mechanization can effectively improve agricultural total factor productivity. Combined with the results of Regression 1 and 2 in Table 5, both β2 and β3 are greater than 0, and Hypothesis H3 is thus verified.
4.5 Heterogeneity analysis based on the difference of the large scale entities
The large scale entities fall into four types: leading specialized household, family farm, specialized farmer cooperatives, and agricultural product processing enterprise. The four types of large scale entities have different main businesses and business scopes, thus different types of large scale entities may have different effects on agricultural total factor productivity. In this paper, the large scale entities are divided into leading specialized household, family farm, specialized farmer cooperatives and agricultural product processing enterprise, and are, respectively, used as explanatory variables, and model (7) is adopted for regression.
The results are in Regression 1 to 4 in Table 6. The results of Regression 1 show that the coefficient is not significant, with the β-value of 0.0001, when the number of leading specialized households is used as the explanatory variable, indicating that leading specialized households do not have obvious effect on the improvement of agricultural total factor productivity. The results of Regression 2 show that the coefficient is significantly positive at the level of 10%, with the β-value of 0.0006, when the number of family farms is used as the explanatory variable, indicating that family farms have a significant effect on the improvement of agricultural total factor productivity. The results of Regression 3 show that the coefficient is significantly positive at the level of 10%, with the β-value of 0.0008, when the number of specialized farmer cooperatives is used as the explanatory variable, indicating that specialized farmer cooperatives have an obvious effect on the improvement of agricultural total factor productivity. The results of Regression 4 show that the coefficient is insignificant, with the β-value of 0.0004, when the number of agricultural product processing enterprises is used as the explanatory variable, indicating that agricultural product processing enterprises do not have an obvious effect on the improvement of agricultural total factor productivity. The above heterogeneity analysis is thus verified.
5 Conclusion and policy implications
In the critical transition period of China’s agriculture from traditional production by farmers on a small scale to modern production, it is of great significance to explore whether and how the development of large scale entities can improve agricultural total factor productivity. In this paper, the panel data of prefecture-level cities from 2015 to 2020 are used, and the empirical research shows that the development of large scale entities can significantly improve agricultural total factor productivity, and the effect mechanism is the improvement of agricultural mechanization. The results of heterogeneity analysis based on large scale entity difference show that family farms and specialized farmer cooperatives have a more obvious effect on the improvement of agricultural total factor productivity, while leading specialized households and agricultural product processing enterprises have a less obvious effect on the improvement of agricultural total factor productivity. Based on the above research conclusions, the following policy implications are proposed:
First of all, greater efforts shall be devoted to support the development of large scale entities. According to the benchmark regression results of this paper, the development of large scale entities can significantly improve agricultural total factor productivity. Therefore, devoting greater efforts to support the development of large scale entities is one of the important ways to ensure that agricultural total productivity does not decline or would get improved during China’s agricultural transformation. For instance, the entry standards and conditions of large-scale business entities shall be standardized, preferential tax policies for large scale entities shall be innovated, and their tax burdens shall be eased; the rural financial system shall be standardized, mutual aid organizations for rural funds shall be developed, rural credit guarantee methods shall be innovated, the financing environment of large scale entities shall be optimized, and the availability of funds shall be ensured.
Secondly, the development of large scale entities should be guided by classification according to their differences. The results of the heterogeneity analysis based on the difference of large scale entities show that different types of large scale entities have different effects on the improvement of agricultural total factor productivity. It is necessary to regulate the development of leading specialized households, constantly expand their scale, improve their mechanization and intensification, standardize the division standards, and curb their extensive operation; Then, to strengthen the organic connection between agricultural product processing enterprises and small farmers, it is necessary to strengthen the benefit affiliating mechanism between them and farmers, improve the policy mechanism of government support for agricultural product processing enterprises, innovate the business model of “enterprise + farmer” and “enterprise + intermediary organization + farmer,” and alleviate the problems of information asymmetry, incomplete contract and high transaction cost between enterprises and farmers so as to improve the stability of agricultural industry chain.
Finally, there are still some shortcomings although the effect of the development of large scale entities on agricultural total factor productivity is analyzed both theoretically and empirically in this paper. Specifically, the number of large scale entities is used as an indicator to measure the development of large scale entities. Although this indicator can reflect the development speed of large scale entities in each region, it cannot replace the main business income, output value and other data that can represent the development scale of large scale entities. And the lack of data leads to the inadequacy of this study.
Data availability statement
The original contributions presented in the study are included in the article/supplementary material, further inquiries can be directed to the corresponding author.
Author contributions
XJ: Writing – original draft, Writing – review & editing, Conceptualization, Data curation, Formal Analysis, Investigation, Methodology, Software, Supervision. HL: Writing – original draft, Writing – review & editing, Conceptualization, Data curation, Investigation, Methodology, Software, Formal Analysis, Supervision. JL: Formal Analysis, Project administration, Supervision, Writing – review & editing, Conceptualization, Validation. PY: Formal Analysis, Methodology, Project administration, Supervision, Writing – review & editing.
Funding
The author(s) declare that no financial support was received for the research, authorship, and/or publication of this article.
Conflict of interest
The authors declare that the research was conducted in the absence of any commercial or financial relationships that could be construed as a potential conflict of interest.
Publisher’s note
All claims expressed in this article are solely those of the authors and do not necessarily represent those of their affiliated organizations, or those of the publisher, the editors and the reviewers. Any product that may be evaluated in this article, or claim that may be made by its manufacturer, is not guaranteed or endorsed by the publisher.
References
Abebaw, D., and Haile, G. M. (2013). The impact of cooperatives on agricultural technology adoption: empirical evidence from Ethiopia. Food Policy 38, 82–91. doi: 10.1016/j.foodpol.2012.10.003
Ali, D., Deininger, K., and Harris, A. (2019). Does large farm establishment create benefits for neighboring smallholders? Evidence from Ethiopia. Land. Econ. 95, 71–90. doi: 10.3368/le.95.1.71
Baumgartner, P., Braun, V. J., Abebaw, D., and Müller, M. (2015). Impacts of large-scale land investments on income, prices, and employment: empirical analyses in Ethiopia. World Dev. 72, 175–190. doi: 10.1016/j.worlddev.2015.02.017
Beeson, P. (1987). Total factor productivity growth and agglomeration economies in manufacturing: 1959-1973. J. Regional. Sci. 27, 183–199. doi: 10.1111/j.1467-9787.1987.tb01154.x
Bernard, T., and Spielman, D. J. (2009). Reaching the rural poor through rural producer organizations? A study of agricultural marketing cooperatives in Ethiopia. Food Policy 34, 60–69. doi: 10.1016/j.foodpol.2008.08.001
Burke, W. J., Jayne, T. S., and Sitko, N. J. (2020). Do medium-scale farms improve market access conditions for Zambian smallholders? J. Agr. Econ. 71, 517–533. doi: 10.1111/1477-9552.12360
Chamberlin, J., and Jayne, T. (2019). Does farm structure affect rural household incomes? Evidence from Tanzania. Food Policy 90:101805. doi: 10.1016/j.foodpol.2019.101805
Chari, A., Liu, E. M., Wang, S. Y., and Wang, Y. X. (2022). Property rights, land misallocation, and agricultural efficiency in China. Rev. Econ. Stud. 88, 1831–1862. doi: 10.1093/RESTUD/RDAA072
Chen, C. (2017). Untitled land, occupational choice, and agricultural productivity. Am. Econ. J. Macroecon. 9, 91–121. doi: 10.1257/mac.20140171
Chen, C. (2020). Technology adoption, capital deepening, and international productivity differences. J. Dev. Econ. 143:102388. doi: 10.1016/j.jdeveco.2019.102388
Chen, S., Wang, X. Y., and Yao, S. B. (2023). National water-saving city and its impact on agricultural total factor productivity: a case study of nine provinces along the Yellow River, China. J. Clean. Prod. 417:138019. doi: 10.1016/J.JCLEPRO.2023.138019
Davis, F. D. (1989). Perceived usefulness, perceived ease of use, and user acceptance of information technology. Mis. Q. 13, 319–340. doi: 10.2307/249008
Deininger, K., Ali, D. A., and Alemu, T. (2011). Impacts of land certification on tenure security, investment, and land market participation: evidence from Ethiopia. Land Econ. 87, 312–334.
Deininger, K., and Fang, X. (2016). Quantifying spillover effects from large land-based investment: the case of Mozambique. World Dev. 87, 227–241. doi: 10.1016/j.worlddev.2016.06.016
Foster, A. D., and Rosenzweig, M. R. (2022). Are there too many farms in the world? Labor market transaction costs, machine capacities, and optimal farm size. J. Polit. Econ. 130, 636–680. doi: 10.1086/717890
Fu, W. Q., and Zhang, R. W. (2022). Can digitalization levels affect agricultural Total factor productivity? Evidence from China. Front. Sustain. Food. Syst. 6:860780. doi: 10.3389/fsufs.2022.860780
Gong, B. (2018). Agricultural reforms and production in China: changes in provincial production function and productivity in 1978–2015. J. Dev. Econ. 132, 18–31. doi: 10.1016/j.jdeveco.2017.12.005
Herrmann, R. T. (2016). Large-scale agricultural investments and smallholder welfare: a comparison of wage labor and Outgrower channels in Tanzania. World Dev. 90, 294–310. doi: 10.1016/j.worlddev.2016.10.007
Ito, J., Bao, Z., and Su, Q. (2012). Distributional effects of agricultural cooperatives in China: exclusion of smallholders and potential gains on participation. Food Policy 37, 700–709. doi: 10.1016/j.foodpol.2012.07.009
Jayne, T. S., Chamberlin, J., Traub, L., Sitko, N., Muyanga, M., Yeboah, F. K., et al. (2016). Africa's changing farm size distribution patterns: the rise of medium-scale farms. Agric. Econ. 47, 197–214. doi: 10.1111/agec.12308
Li, Y. F., Zhu, Z. H., and Xu, P. (2022). Research on the green production motivation of new agricultural business entities: benefit perception and environmental regulation. J. Environ. Public Health 2022, 1–10. doi: 10.1155/2022/9182725
Lingjuan, C., Wei, Z., and Kaifeng, D. (2021). The influence of new agricultural business entities on the economic welfare of Farmer’s families. Agriculture 11:880. doi: 10.3390/AGRICULTURE11090880
Moomaw, R. L. (1981). Productivity and City size: a critique of the evidence. Q. J. Econ. 96, 675–688. doi: 10.2307/1880747
Mostafa, A. E. (2023). Beyond technology acceptance: development and evaluation of technology-environmental, economic, and social sustainability theory. Technol. Soc. 75:102383. doi: 10.1016/j.techsoc.2023.102383
Nolte, K., and Ostermeier, M. (2017). Labour market effects of large-scale agricultural investment: conceptual considerations and estimated employment effects. World Dev. 98, 430–446. doi: 10.1016/j.worlddev.2017.05.012
Qu, X., Kojima, D., Wu, L. P., and Mitsuyoshi, A. (2022). Do farming scale and mechanization affect moral Hazard in Rice harvest outsourcing Service in China? Agriculture 12:1205. doi: 10.3390/AGRICULTURE12081205
Sanjaya, F., Elena, G., Thiagarajah, R., and Nicola, S. (2021). Organisational attributes of cooperatives and farmer companies. J. Co-oper. Organ. Manag. 9:100132. doi: 10.1016/J.JCOM.2021.100132
Sheng, Y., Tian, X., Qiao, W., and Peng, C. (2019). Measuring agricultural Total factor productivity in China: pattern and drivers over the period of 1978-2016. Aust. J. Agricult. Resour. Econ. 64, 82–103. doi: 10.1111/1467-8489.12327
Sipangule, K. (2017). Agribusinesses, smallholder tenure security and plot-level investments: evidence from rural Tanzania. Afr. Dev. Rev. 29, 179–197. doi: 10.1111/1467-8268.12271
Sleuwaegen, L., and Goedhuys, M. (2002). Growth of firms in developing countries, evidence from Côte d'Ivoire. J. Dev. Econ. 68, 117–135. doi: 10.1016/S0304-3878(02)00008-1
Stock, J., and Yogo, M. (2005). “Testing for weak instruments in linear IV regression” in Identification and inference for econometric models (New York: Cambridge University Press), 80–108.
Venkatesh, V., Morris, M. G., Davis, G. B., and Davis, F. D. (2003). User acceptance of information technology: toward a unified view. Mis. Q. 27, 425–478. doi: 10.2307/30036540
Wang, Z., Liu, J. H., Li, T. S., Chao, J., and Gao, X. P. (2021). Factors affecting new agricultural business Entities' adoption of sustainable intensification practices in China: evidence from the Main apple-producing areas in the loess plateau. Agronomy 11:2435. doi: 10.3390/agronomy11122435
Xin, L. G., and Li, X. B. (2019). Rental rates of grain land for consolidated plots and their determinants in present-day China. Land Use Policy 86, 421–426. doi: 10.1016/j.landusepol.2019.05.037
Keywords: agricultural transformation in China, large scale agricultural business entities, agricultural total factor productivity, scale effect, technology utilization effect
Citation: Ju X, Li H, Liu J and Yao P (2023) Can development of large scale agricultural business entities improve agricultural total factor productivity in China?: an empirical analysis. Front. Sustain. Food Syst. 7:1281328. doi: 10.3389/fsufs.2023.1281328
Edited by:
Marian Rizov, University of Lincoln, United KingdomReviewed by:
Justice Gameli Djokoto, Dominion University College, GhanaViswanathan Pozhamkandath Karthiayani, Amrita Vishwa Vidyapeetham (Amritapuri Campus), India
Copyright © 2023 Ju, Li, Liu and Yao. This is an open-access article distributed under the terms of the Creative Commons Attribution License (CC BY). The use, distribution or reproduction in other forums is permitted, provided the original author(s) and the copyright owner(s) are credited and that the original publication in this journal is cited, in accordance with accepted academic practice. No use, distribution or reproduction is permitted which does not comply with these terms.
*Correspondence: Huizhao Li, lihuizhao2020@163.com
†These authors have contributed equally to this work and share first authorship