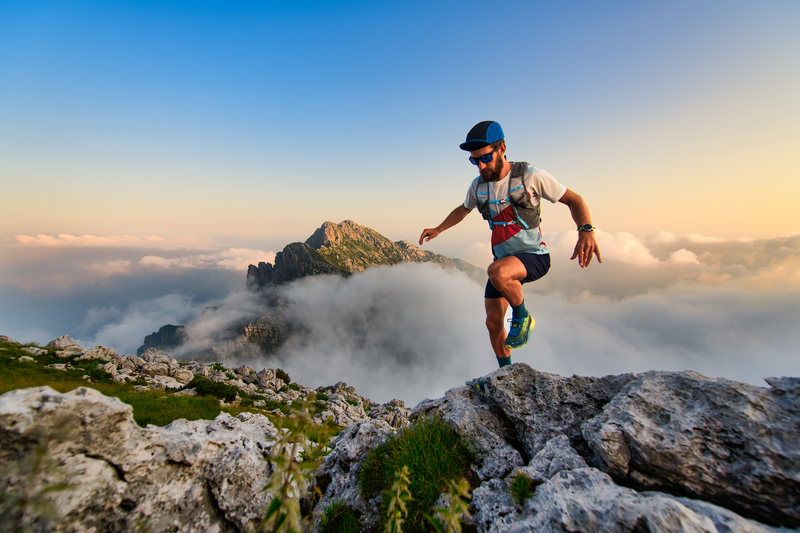
94% of researchers rate our articles as excellent or good
Learn more about the work of our research integrity team to safeguard the quality of each article we publish.
Find out more
ORIGINAL RESEARCH article
Front. Sustain. Food Syst. , 05 October 2023
Sec. Land, Livelihoods and Food Security
Volume 7 - 2023 | https://doi.org/10.3389/fsufs.2023.1250564
Research on livelihood resilience not only helps to understand the adverse impact of COVID-19 on farmers' livelihoods, but also helps to formulate concrete actions to enhance the resilience of farmers' livelihoods and support economic recovery. Taking rural residents in Jiangsu Province of China as an example, this paper uses one-way ANOVA, entropy method and obstacle degree models to quantitatively analyze the characteristics of livelihood resilience of different types of farmers and the main obstacle factors. The main findings are as follows: (1) Both laboring type and part-time type farmers together accounted for 75.98% of the survey sample, and the non-agricultural phenomenon of farming households in Jiangsu Province is obvious; There are significant differences in livelihood characteristics among different types of farmers, with relatively high livelihood capital for planting and breeding type farmers and relatively low livelihood capital for asset and subsidy type farmers. (2) The livelihood resilience of farmers in Jiangsu Province from high to low is breeding type farmers (0.501), planting type farmers (0.493), laboring type farmers (0.465), part-time type farmers (0.455), subsidy type farmers (0.400), asset type farmers (0.389). (3) In the diagnosis of barrier factors of livelihood resilience, buffer ability disorder is the most obvious, learning ability disorder is the second, and self-organization ability disorder is the last; Among the specific factors, the most important obstacle factors for planting, breeding and asset type farmers are the number of productive assets, the number of agricultural technical services for laboring type farmers, the number of channels for obtaining information for part-time type farmers, and the family labor income for subsidy type farmers. Therefore, it is recommended that policies be improved in the post-epidemic era in terms of raising farmers' livelihood capital, developing knowledge and skills for learning, and enhancing organizational awareness.
The COVID-19 pandemic has brought a great negative impact on China's rural areas, where the medical system is relatively weak, especially on the livelihood risk of farmers (Wang et al., 2021a,b,c). During the COVID-19 prevention and control period, measures such as road control directly affected the normal livelihood activities of farmers, home quarantine and business shutdown resulted in limited livelihood strategies of farmers, and increased costs of epidemic prevention expenditures reduced the quality of farmers' livelihoods (Cariappa et al., 2022; Hammond et al., 2022; Kuuwill et al., 2022). In the post-epidemic era, the level of livelihood resilience of farmers directly affects the livelihood security of farmers, and is related to the harmony and stability of local society and strong economic recovery. How to take targeted measures to improve livelihood resilience according to the characteristics of different types of farmers is an urgent requirement for China to enhance people's wellbeing and get rid of the impact of the epidemic (Zhao et al., 2023).
The sustainable livelihoods framework covers five components: vulnerability context, livelihood capital, livelihood strategies and livelihood outcomes, with the core component of livelihood capital consisting of five categories: human capital, natural capital, physical capital, social capital and financial capital. This framework can better analyze the resilience of farm households to environmental, market and other fluctuations, but it fails to adequately take into account the adaptation and adjustment behaviors of farm households under external shocks, as well as the intervention behaviors of relevant managers, such as community-based organizations (Lan et al., 2022; Zheng et al., 2023). Since the concept of resilience was used to study the stability of ecosystems in 1973, it has gradually been applied to a wide range of fields, including social-ecological systems, and has provided new perspectives on livelihoods research (Liang and Cao, 2021; Saja et al., 2021; Wang et al., 2022). Livelihood resilience is a measure of the ability of a farm household to maintain or even strengthen its livelihood capacity in the present and future, sustaining and improving wellbeing, based on the sustainable livelihoods framework. The combination of resilience thinking and livelihoods approaches can deepen our understanding of the dynamics of farmers' livelihoods, and better explain how farmers can effectively respond to external perturbations and strengthen their own livelihood capacity building (Zhao et al., 2021; Chen et al., 2023).
At present, natural disasters, climate change and food security are relatively common external disturbance factors in the study of farmers' livelihood resilience (Varis et al., 2010; Yang et al., 2021; Badewa and Dinbabo, 2023). Despite the complexity of external disturbances affecting the livelihood resilience of farmers, their evaluation indicator systems are mostly constructed from three dimensions: buffer capacity, self-organization capacity, and learning capacity (Sun et al., 2023). In addition, research on the influencing factors of farmers' livelihood resilience is an important link to analyze the influence mechanism of external disturbance factors on farmers' livelihood system, and also a key link to study and formulate countermeasures to improve farmers' livelihood resilience (Wu et al., 2023).
Livelihood resilience measurement involves issues such as comprehensive evaluation of multiple indicators, and there are two main types of evaluation methods. The first category is to analyze the level of livelihood resilience from a subjective perspective using methods such as fuzzy integrated evaluation, hierarchical analysis, and expert evaluation. Manlosa (2022) use a study of smallholder livelihoods and food security in southwestern Ethiopia as an example to construct a conceptual analytical framework for livelihood resilience that includes four institutional pillars: preconditions, processes, power, and possibilities. Nath et al. (2020) found that various variables related to conflict and rule-making would affect the level of livelihood resilience of residents in Bangladesh's paddock. Ssennoga et al. (2022) comprehensively evaluated the resilience of people with disabilities to climate-induced landslide hazards in vulnerable areas of Mount Elgon, Uganda. The second category analyzes the level of livelihood resilience of regional residents from an objective perspective using factor analysis, principal component analysis methods, and entropy weighting methods. Liu et al. (2019) used factor analysis to evaluate the livelihood resilience of relocated poor migrants in Ankang, southern Shaanxi. Ado et al. (2019) used principal component analysis to examine the factors influencing food security livelihood strategies and livelihood resilience of Aguié households in Niger and found that agriculturalists, and farmers had the highest adaptive capacity. Li et al. (2022a,b,c) used entropy TOPSIS to assess the livelihood resilience of pastoralists on the Tibetan Plateau and found that the overall level of livelihood resilience of pastoralists was weak. Based on the Bangladeshi river islands, Sarker et al. (2020) found that island residents could not resist the adverse impacts brought by nature. To visualize and analyze livelihood resilience and establish specific strategies to improve it, Quandt (2018) proposed the HLRA approach, drawing on the sustainable livelihoods approach, and inspired by this, Tran et al. (2022) used the HLRA approach to explore the changing characteristics of livelihood resilience of ethnic minorities in the mountainous areas of northwest Vietnam in the context of climate change; Stanford et al. (2017) took the livelihood resilience of fishermen as the research object, and pointed out that FLIRES method was more conducive to comparative analysis of the spatiotemporal characteristics of fishermen's livelihood resilience. In addition, some scholars have studied the sustainable utilization of natural resources from the perspective of farmers. Razzaq et al. (2022a,b) not only studied the factors influencing the extent of buying and selling in the groundwater market in Pakistan from both supply-side perspectives, but further analyzed the impact of farmers' participation in the groundwater market on the utilization of farmland, cropping practices, and income. Ehsan et al. (2022) explored the social acceptance of photovoltaic water pumps in rural areas of Pakistan, pointing out that subsidies and publicity can be used to effectively promote green energy technologies.
The above studies show that there are rich empirical studies and theoretical discussions on livelihood resilience, and the evaluation methods are mainly based on subjective and objective evaluation methods, which also lay a deep foundation for the research in this paper. With the characteristics of fast spread, strong infectivity and wide spread, COVID-19 is an important external disturbance factor affecting the livelihood of Chinese farmers shortly (Huang et al., 2017). How to avoid issues such as livelihood security for farmers due to the impact of the COVID-19 pandemic in China's modernization journey is of special importance for maintaining a stable economic environment and a harmonious social environment (Pan et al., 2022; Wang et al., 2022). Compared with other provinces in the country, Jiangsu Province has specific location advantages and agricultural production characteristics. Jiangsu Province is not only a major economic province in China, but also a major food and population province in China. With 3.2% of the country's arable land, Jiangsu Province produces 5.5% of the country's grain and feeds 6% of the country's population, thus playing a pivotal role in guaranteeing China's food security. Therefore, this research takes the rural residents of Jiangsu Province as the research object, uses the entropy value method to measure the level of livelihood resilience of different types of farming households in Jiangsu Province under the COVID-19 pandemic, and adopts the obstacle degree model to identify the main obstacle factors affecting the livelihood resilience of different types of farming households, with a view to providing references for the scientific formulation of specific strategies to enhance the livelihood resilience of different types of farming households in Jiangsu Province and even in the country as a whole.
Jiangsu Province is located in China's Yangtze River Delta, adjacent to Anhui, Shandong, Zhejiang, Shanghai, and other provinces (Ke et al., 2017) (see Figure 1). As a province with a high level of comprehensive development in China, Jiangsu is not only a strong industrial province, it is also a strong agricultural province (Cheng, 2020; Wang et al., 2022). In 2022, Jiangsu Province's GDP is 12.29 trillion yuan, ranking second in the country, and the output value of agriculture, forestry, animal husbandry, and fishery is 0.87 trillion yuan, ranking fifth in the country. The urbanization rate of Jiangsu's resident population at the end of the year reaches 74.4%, ranking fifth in the country; the per capita disposable income is 49,862 yuan, ranking fourth in the country, of which the per capita disposable income of rural residents is 28,486 yuan, ranking fifth in the country.
As of December 22, 2022, a total of 3327 cases were confirmed in Jiangsu Province (excluding overseas imports), distributed in more than 70% of the regions outside the provincial sink cities, in line with the basic characteristics of epidemiology. Based on the real-time reports of epidemic, cities and counties in Jiangsu province with relatively concentrated cases and relatively developed agricultural production are selected as the study areas of this paper, including Yancheng (184) and Huai'an (108) in northern Jiangsu, Yangzhou (596) and Taizhou (73) in central Jiangsu, and Nanjing (861) and Zhenjiang (40) in southern Jiangsu. These six prefecture-level cities accounted for 57.52% of the cumulative confirmed diagnosis and 47.11% of the agricultural GDP in Jiangsu Province, which is very representative.
The data in this paper are from the China Land Economy Survey Project jointly conducted by the Humanities and Social Sciences Division of Nanjing Agricultural University and the Jinshanbao Institute of Agricultural Modernization and Development in July and August 2022. The survey was conducted using the PPS sampling method, in which the interviewer asked the respondent to answer. The questionnaire included the basic situation of farm household livelihood, income and expenditure details, social relationship network, habitat environment, and rural retirement development, etc. Finally, 1,203 valid questionnaires were collected, which provided strong data support for the study of this paper.
The basic information of the respondents of the survey sample is shown in Table 1. From the composition of the respondents' household population in terms of gender, age, education level, and health status, there are more males than females, with males accounting for 73.32%; the respondents are mainly young adults and elderly people with good physical health; the education level is mainly primary and junior high school, accounting for 78.89%. From the perspective of respondents' household attributes, 366 households are members of the Communist Party of China, accounting for 30.42%; There are 75 ethnic minority households, accounting for 6.23%, and 125 poverty-stricken households and Five Guaranteed Households, accounting for 10.39%.
Farmers' livelihood resilience refers to the ability of farmers to maintain their original livelihood level or even further improve their livelihood level when they are impacted by external factors and disaster shocks (Bauer et al., 2022). In this paper, based on the existing research results, we combine the production characteristics of farm households in Jiangsu Province, China, and construct a farm household livelihood resilience evaluation index system from three dimensions: buffer capacity, self-organization capacity learning capacity (Ifejika Speranza et al., 2014; Chen et al., 2018). The specific indicators are shown in Table 2.
1. buffering capacity. buffer capacity represents the ability of farming households to withstand unexpected risks. farming households with strong buffer capacity can maintain the stability of the livelihood system with their livelihood capital in the face of external shocks this paper measures the buffer capacity of farm households in five dimensions: natural capital, human capital, physical capital, financial capital, and social capital. Specifically, in terms of natural capital, although the livelihoods of chinese farm households have diversified from a single to a diversified one with the rapid economic and social development, the land remains the last social security for farm households. therefore, the amount and structure of land is an essential indicator when measuring the buffer capacity of farm households (Li et al., 2022a,b,c); in terms of human capital, farm households with a larger labor force, healthier members and higher educational attainment have higher productivity and greater earning capacity (Zhuo et al., 2021); in terms of physical capital, housing is both a consumer good and an investment good for farm households, and it is the most basic material living condition for farm households; the ownership of productive and consumer assets can fully reflect the productive capacity and quality of life of farm households (Quan and Doluschitz, 2021); in terms of financial capital, household savings, income expenditure and dependency ratio profiles not only reflect the current economic status of farm households, but also determine, to some extent, the future welfare level of farm households (Zhu et al., 2021); in terms of social capital, household interest, dividend and bonus income can better measure the income of rural households through participating in social networks or joining group organizations (Yu and Nilsson, 2018).
2. Self-Organization capacity. Self-organization ability represents the ability of farming households to communicate and coordinate with the outside world. Farming households with strong self-organization ability can form a close social network with the outside world and actively participate in the construction of local economic and social development. This paper quantifies the self-organizational capacity of farm households in terms of 3 dimensions: social trust, social participation, and social networks. on the one hand, a close social network can help farming households identify potential livelihood risks and make rational management decisions (Wang and Zhao, 2023), and on the other hand, it can help farming households expand their risk management channels and improve risk prevention and control (Hou et al., 2022). For farm households, the higher the social trust, the more stable the social network; the higher the social participation, the broader the social network (Chen et al., 2021).
3. Learning capacity. Learning capacity represents the ability of farm households to acquire new knowledge and skills and to translate them into livelihood capital. This paper characterizes the learning capacity of farm households in four dimensions: information attention, information acquisition, skill training, and technology application. Good information identification and screening ability and more diversified information access can enable farming households to obtain more useful information, which enables them to integrate their factors in time to cope with risks when facing external shocks (Yue et al., 2023); skills training, on the other hand, reflects the ability of farming households to acquire knowledge and skills, and the more frequent the training, the greater the ability of farming households to acquire new knowledge and skills (Muktasam et al., 2019); the application of technology reflects the ability of rural households to transform knowledge and skills into livelihood capital. The more types of agricultural technology services used by rural households in production activities, not only help them improve production efficiency, but also effectively protect land resources (Wang and Dong, 2023).
Farmers' livelihood resilience includes three aspects: buffer capacity, self-organization capacity, and learning capacity (Li et al., 2022a,b,c). This paper uses the entropy method to evaluate the livelihood resilience of farmers in Jiangsu Province, China, which can not only avoid the interference of human factors to a large extent and ensure the objectivity of the results, but also judge the weight of each specific index of livelihood resilience and identify the key influencing factors, which has strong application (Chen et al., 2022; Li et al., 2022a,b,c). The measurement process of farmers' livelihood restoration is as follows:
In the first step, to eliminate the influence of dimensional differences and extreme data on the evaluation results, the raw data of the farmers' livelihood resilience evaluation indicators were standardized using the extreme value standardization method (Alary et al., 2022).
For positive indicators:
For negative indicators:
where Xij represents the original data of the jth indicator of the ith farmer, xmax is its maximum value, xmin is its minimum value, and is the value after normalization of the original data.
In the second step, the weight of the value of indicator j for the ith farm household is calculated:
In the third step, the information entropy ej and the redundancy dj of the jth indicator of the ith farmer are calculated:
Step 4: Calculate the weights of indicator j in each dimension:
Step 5: Calculate the composite score and livelihood resilience index for each dimension of farmer i:
In equation (7), riB denotes the farmer buffer capacity index, riS denotes the farmer self-organization capacity index, riL denotes the farmer learning capacity index, and Ri denotes the farmer livelihood resilience index.
Based on the scientific evaluation of the livelihood resilience of farmers in Jiangsu Province, China, the barrier model is used to identify the factors that constrain the livelihood resilience of farmers and to establish specific policy recommendations for improving the livelihood resilience of farmers in Jiangsu Province, China.
The analysis of the barrier degree of farmers' livelihood resilience mainly includes three indicators: factor contribution, indicator deviation, and barrier degree (Zhang et al., 2019; Zhou et al., 2021; Xiang et al., 2022). Among them, the factor contribution rate is used to analyze the degree of influence of individual indicators on the comprehensive evaluation results of farmers' livelihood resilience, the indicator deviation degree indicates the distance between individual indicators and the evaluation target of farmers' livelihood resilience, and the barrier degree can reflect the intensity of the negative effect of individual indicators on farmers' livelihood resilience. The specific calculation formula is:
In the equation: wij indicates the factor contribution, i.e., the weight of the jth indicator, uij indicates the weight of the dimensional layer to which the indicator belongs, indicates the standardized value of the extreme difference, Ej indicates the deviation of the indicator, and Oj indicates the barrier, the greater the barrier, the stronger the impact of that barrier factor on the level of livelihood resilience.
The income sources of farmers in Jiangsu Province show a diversified development trend. According to the characteristics of the income structure of farmers, farmers are divided into six livelihood types: planting type, breeding type, laboring type, part-time type, asset type and subsidy type. Among them, there are 418 laboring type farmers, 496 part-time type farmers and 168 asset type farmers, which account for 89.94% of the survey samples.
Under the sustainable livelihood framework, one-way ANOVA was applied to compare the livelihood characteristics of different types of farming households in Jiangsu Province along five dimensions, including human capital, physical capital, and natural capital (Table 3). The livelihood capacity of planting and farming type households is relatively strong, while that of asset and subsidy type farming households is relatively weak. In terms of human capital, the age, labor force size, and health status of households differed significantly among the six types of farming households. laboring type farm households had the youngest age, the largest labor force, the longest number of years in school, and the highest human capital, while asset type farm households has the oldest age, relatively small labor force, and the heaviest family support tasks. In terms of natural capital, the land area of the six types of farmers showed large differences, among which the land area of planting type farmers and part-time type farmers is much higher than that of the other four types of farmers, at 13.96 mu and 7.40 mu respectively, while the land area of breeding type, laboring, asset and subsidy type farmers do not differ significantly, at about 2.3 mu. From the perspective of household housing area and number of assets, breeding type farmers have the largest housing area (231.11 m2) and consumption assets (19.22). The living standard is relatively high, while subsidized farmers only have 112.92 mu of housing area, and consumption assets are less than other types of farmers. The number of productive assets owned by planting farmers is the largest, which is 2.71, while the number of productive assets owned by laboring type farmers is only 0.31, which indicates that the livelihood of farmers in Jiangsu Province is strongly differentiated. From the perspective of social interaction, breeding type farmers have the largest number of mobile phone contacts and more social interaction activities, while asset type farmers have relatively narrow activity circles. From the perspective of financial capital, there are obvious differences in absolute income gap and relative income gap between different types of farmers. The income of planting type, breeding type, part-time type, laboring type, asset type and subsidy type is ¥92,5014.38, ¥769,567.48, ¥132,518.87, ¥117,450.29, ¥326,667.37 and ¥10,648.17, respectively, decreasing successively. In terms of absolute income, the income of farmers of planting type, breeding type, part-time type, laboring type and asset type is ¥914,366.21, ¥758,919.31, ¥121,870.7, ¥106,802.12 and ¥22,019.2 higher than that of subsidized farmers. In terms of income multiple, planting type, breeding type, part-time type, laboring type and asset type are 86.87 times, 72.27 times, 12.44 times, 11.03 times and 3.07 times of the subsidy type of farmers respectively; In terms of expenditure, there is no significant difference among the six categories of farmers, with breeding type farmers ranking first with an expenditure of ¥53,372.30, while asset type farmers have the lowest expenditure of ¥37,452.85.
Table 3. Comparative analysis of livelihood characteristics of different types of farming households in Jiangsu Province, China.
To better visualize the livelihood resilience index of different types of farmers, a stacked bar chart of the livelihood resilience index of farmers in Jiangsu Province, China is drawn (Figure 2). As can be seen from Figure 2, there are significant differences in the livelihood resilience indices of different types of farmers, with breeding type and planting type farmers ranking first and second, respectively, with 0.501 and 0.493 in that order. For breeding type farmers in Jiangsu Province, livestock and waterfowl products are the main breeds. During the epidemic prevention and control period, government departments actively guided leading agricultural enterprises, cooperatives, and large breeding and breeding enterprises to take the initiative to connect supply channels, alleviating the problem of unsalable livestock and poultry products, and guaranteeing the economic benefits of some breeding farmers. In addition, financial products such as live pig “insurance + futures” and aquaculture temperature index insurance enhance the ability of aquaculture farmers to withstand the risk of falling prices and hot weather; Planting type farmers are mostly new agricultural business entities, mainly planting food crops and vegetables and fruits products. During the prevention and control of COVID-19, the government actively guided planting type farmers to solve the problem of unsalable agricultural products through live streaming and online platform sales, and laid a smooth transportation system for agricultural products, which effectively alleviated the adverse impact of COVID-19 on planting type farmers. The livelihood resilience indexes of laboring and part-time type farmers are 0.465 and 0.455, ranking third and fourth, respectively. The COVID-19 pandemic has brought many shocks to the economy and business development, which has affected the wage income of laboring type farmers. Part-time type farmers are mainly concentrated in catering, construction, housekeeping, maintenance, and other high-contact industries, which are obviously affected by the COVID-19 epidemic. Compared with planting and breeding type farmers, Part-time type farmers do not have scale advantages in agricultural production and operation, and the ability to resist risks is relatively weak. The livelihood resilience indices of subsidy and asset type farmers ranked at the bottom with 0.400 and 0.389, respectively. For asset type farmers, the property income has decreased significantly due to the impact of COVID-19. Most of the subsidy type farmers are disabled elderly, and their main source of income is transfer income such as pension insurance. The COVID-19 pandemic not only directly threatens the physical health of the elderly, but also increases their health care costs.
Figure 2. Livelihood resilience index of different types of farm households in Jiangsu Province, China.
A violin plot of the livelihood resilience index of farmers in Jiangsu Province, China, is drawn using GraphPad Prism 8 software (Figure 3). From the graphical distribution, the upper quartile of the livelihood resilience index of planting type farmers is the largest, which is 0.592, and the distance between the upper quartile and the median is far, indicating that the deviation of the data at the upper boundary of the midpoint is greater than that at the lower boundary of the midpoint, that is, the livelihood resilience index of planting type farmers is generally high; The median of livelihood resilience index of breeding type farmers is the highest, which is 0.499, and its violin graph shows the characteristics of long width at both ends, indicating that the overall level of livelihood resilience of breeding type farmers is high, and the dispersion degree is low; laboring type farmers are the third group in terms of median livelihood resilience, at 0.455, and there are no extreme samples with too high or too low mean values, and the overall development of laboring type farmers is more balanced; The part-time type farmers is the group with the largest number of samples, and the violin plot shows a narrow top and wide bottom feature, indicating that there are more samples with livelihood resilience below the median for this group, with large differences among samples and more uneven group development; asset type farmers have the lowest median livelihood resilience at 0.364, and the median is close to the lower quartile, indicating that there is little data dispersion between the midpoint and lower bound of asset-based farmers' livelihood resilience, i.e., the overall asset type farmers' livelihood resilience index is low; The median and upper and lower quartile intervals of the livelihood resilience index of subsidy type farmers are not significantly different, and there are no extreme samples with too high or too low values for this type of farmers, indicating that the overall livelihood resilience index of subsidy type farmers is relatively similar.
Figure 3. Fiddle diagram of livelihood resilience index of different types of farm households in Jiangsu Province, China. Note: The upper and lower ends of the violin plot are the maximum and minimum values of the data, the widths of the left and right ends indicate the kernel density, i.e., the size of the frequency of data occurrence, the middle blue horizontal line indicates the median, and the upper and lower orange horizontal lines indicate the range of data in the upper 25% and lower 25% of the bound.
Farmers' livelihood resilience is the result of a combination of buffering capacity, self-organizing capacity, and learning capacity; therefore, the magnitude of each dimension index reflects the degree of its influence on farmers' livelihood resilience. The internal differences in livelihood resilience of different types of farmers in Jiangsu Province, China are shown in Table 4 and Figure 4. From a macroscopic point of view, regardless of the type of farmers, the buffer capacity index is the highest among the three dimensions, while the learning capacity index is the lowest among the three dimensions, and the values of each dimensional layer index vary widely, indicating that there is an uneven development of the livelihood resilience of farmers in Jiangsu Province, which is not conducive to the building of their sustainable livelihood capacity.
Table 4. Internal differences in livelihood resilience of different types of farm households in Jiangsu Province, China.
Figure 4. Index of different dimensions of different types of farm households in Jiangsu Province, China.
At the micro level, planting and breeding type farmers have the strongest buffering and self-organization capacity; part-time type farmers have the strongest learning capacity; asset type farmers have the weakest buffering capacity and subsidy type farmers have the weakest learning capacity. For planting and breeding type farmers, the demand for quarantine and inspection of COVID-19 prevention and control has caused an increase in transportation time and labor costs, but objectively led to an increase in the prices of agricultural products and livestock and poultry products, increasing in the income of some planting and breeding type farmers. Take pig breeding as an example. Road closures and sealing up during the COVID-19 pandemic have led to a significant increase in pig breeding costs, coupled with a decline in China's pork imports due to trade frictions between China and the US, which have pushed up pork prices. The rise in the price of pork, a daily necessity, makes it difficult to recover from the previous price, making pig farming profitable; This group of part-time type farmers has a certain amount of human capital, and their children work outside the home to earn wage income, while the elderly are engaged in agricultural production at home to earn business income and have more diversified access to information. The COVID-19 pandemic has had a negative impact on laboring type farmers, reducing the income of some migrant workers; subsidy type farmers have more disabled elderly people, lack labor force, rely on state subsidies for their livelihoods, and are obviously at a disadvantage in learning ability compared with other types of farmers; Asset type farmers, whose income is mainly derived from rental income from non-farm assets, are most affected by the epidemic as the COVID-19 pandemic has resulted in no business income or significantly reduced business income for the lessees.
The results of the diagnosis of livelihood resilience barriers for different types of farm households in Jiangsu Province, China, are shown in Table 5. There are significant differences in the factors of livelihood resilience barriers among different types of farm households. For planting and breeding and asset type farmers, the largest obstacle factor is the number of productive assets, and the obstacle degree was 23.76 and 27.74%, respectively. For planting type farmers, although the COVID-19 pandemic has led to the failure of some agricultural products to be sold and unsalable products, the government has actively helped farmers to solve the problems, opened a green channel for the sales of agricultural products, issued production subsidies, encouraged planting and operating farmers to expand the production scale, and guaranteed the household food basket project; In terms of breeding type farmers, due to the continuous impact of African swine fever in 2018–2019, the stock of pigs in China fell rapidly in 2020, and the surviving farmers, who pay more attention to biosecurity prevention and control, are the best in terms of capital, technology and management measures, so just from the perspective of livestock and poultry breeding, the COVID-19 pandemic is conducive to higher prices of livestock products and stimulates some breeding type farmers to expand their production scale; The biggest obstacle factor for laboring type farmers is the type of agricultural technical services they receive, with an obstacle degree of 6.98%. Under the dual impact of COVID-19 and the pressure of urban survival, many laboring type farmers have chosen to return to their hometowns, but this group has worked in the city for many years, and their agricultural production skills and experience are lacking, so it is difficult to find suitable jobs after returning to their hometowns. If they are provided with technical guidance on agricultural production, it will help to improve their level of livelihood resilience; The most important barrier factor for part-time type farmers is access to information, with a barrier degree of 6.43%. During the COVID-19 control period, the business activities of labor units, entertainment places, the catering industry, and other social public activities business places are restricted. If they cannot obtain market information in time, not only it is difficult to sell agricultural products, but also the labor will be greatly affected. The most important obstacle factor for subsidy type farmers is labor income. For the rural elderly, COVID-19 has brought great risks to their lives and health, which not only directly increases the family's medical consumption expenditure, but also further reduces their ability to work. The government should improve the social security system, increase the proportion of medical insurance reimbursement for the elderly, and improve the quality of life of the elderly.
Table 5. Barrier factors and barriers to livelihood resilience of different types of farm households in Jiangsu Province, China.
According to the 24 obstacle factors of the six types of farmers, the obstacle factors in the dimension layer of buffer capacity account for more than 55%, learning ability accounts for about 25%, and self-organization ability accounts for about 20%, indicating that among the obstacle factors affecting the livelihood resilience of the six types of farmers, buffer capacity is the most important, followed by learning ability. The differences in the livelihood resilience indices of the six different types of farmers were also mainly in buffering capacity and learning capacity, among which the buffering capacity was the most obvious, and the self-organizing capacity was not prominent in the diagnosis of the barriers to livelihood resilience of the different types of farmers, i.e., there was no significant difference in the support of livelihood resilience by the resources owned by the different collectives of the farmers. The differences in the livelihood resilience indices of the six different types of farmers in Jiangsu Province, China, are also mainly in buffering capacity and learning capacity, with the most significant difference in buffering capacity, and self-organizing capacity was not prominent in the diagnosis of the barriers to livelihood resilience of different types of farmers, i.e., there was no significant difference in the support of livelihood resilience by the resources possessed by different collectives of farmers. Therefore, to improve the livelihood resilience of farmers and remedy the existing livelihood shortcomings, it is necessary to focus on improving learning capacity, i.e., the ability to learn new knowledge, acquire new skills, and transform them into livelihood capital, while maintaining the existing buffer capacity.
The COVID-19 pandemic has harmed on economic and social development in many ways and has had a direct impact on the production and livelihood of farmers. In the post-epidemic era, the government should, on the one hand, ensure the resumption of economic production and operation, and on the other hand, do a good job in the follow-up work of epidemic prevention and control. This study has the following implications for how to enhance the resilience of farmers' livelihoods, facilitate economic recovery, and cope with possible external shocks and disturbances.
(1) Buffer capacity is the basis for maintaining the livelihood resilience of different types of farmers. The government should improve microcredit, special agricultural credit, and special credit programs for non-agricultural production to strengthen the financial system's support for farmers' livelihood development and improve their buffering capacity. In addition, the government needs to improve the policy of subsidizing the purchase and application of agricultural machinery and explore subsidies linked to the amount of work to improve the material capital of farmers.
(2) Learning ability is the key to improving the livelihood resilience of different types of farmers. The government needs to build a special online information collection and distribution platform to help farmers quickly and effectively understand information related to agricultural markets, labor and employment, epidemic prevention and control, etc. In addition, the government should improve the system of adult education and production skills training for farmers, promote the transformation of knowledge households in the human capital structure of farmers, and improve the learning ability of farmers.
(3) All types of farmers should take the initiative to pay attention to national policy information, keep an eye on the changes in market conditions, and continuously enhance their ability to resist risks. Planting type farmers can expand the scale of planting through land transfer, expand the agricultural industry chain, and realize industrial integration development. Breeding type should actively develop standardized scale farming and explore “order breeding” business methods. Laboring type farmers should continue to improve their labor skills and quality, and seek more employment opportunities and financial support by strengthening their ties with community organizations. part-time type farmers are mostly “planting + labor” or “breeding + labor” and other livelihood combinations, and should actively realize livelihood reorganization by combining their own advantages, or trying large-scale operations if the family has more land area. The elderly population in asset type and subsidy type farm households who are still able to work can try to grow food crops that are more mechanized and socially serviced and use less labor.
Although this study made a measure of livelihood resilience and a diagnosis of barriers to different types of farm households in Jiangsu Province, China, it did not pay enough attention to the formation mechanisms of livelihood resilience of different types of farm households. In addition, this study focuses on micro data of farm households, and pays insufficient attention to the influence of public capital such as region (community and township) on farm households' livelihood resilience. In our future research, we will, first, focus on the formation mechanism of livelihood resilience of farm households and the mechanism of its role in livelihood decision-making of farm households, second, explore the extent of the impact of public capital on livelihood resilience of farm households and the mechanism of its role, and third, explore the relationship between the development of rural industries and livelihood resilience of farm households.
This research constructs the livelihood resilience framework of farmers in Jiangsu Province from the three dimensions of buffer capacity, self-organizing capacity, and learning capacity, and uses the entropy method and obstacle degree model to quantitatively analyze the characteristics of livelihood resilience of different types of farmers and the main obstacle factors, which is helpful to understand the characteristics of livelihood resilience of different types of farmers under the impact of COVID-19 and formulate targeted policy suggestions. The main findings are as follows:
(1) The livelihood patterns of rural households in Jiangsu Province in China are mainly migrant workers and part-time workers, and the total number of laboring type and part-time type farmers accounts for 75.98% of the survey samples, and the phenomenon of non-agricultural farmers is obvious.
(2) The livelihood characteristics of different types of farming households in Jiangsu Province, China differ significantly, with planting type and breeding type farmers having relatively high livelihood capital, laboring type and part-time type farmers having relatively medium livelihood capital, and asset type and subsidy type farmers having relatively low livelihood capital.
(3) Under the impact of COVID-19, the livelihood resilience of farmers in Jiangsu Province from high to low is breeding type farmers (0.501), planting type farmers (0.493), working type farmers (0.465), part-time type farmers (0.455), subsidy type farmers (0.400), and asset type (0.389). From the perspective of each dimension, the buffer capacity and self-organization capacity of planting and breeding type farmers are the strongest; Part-time type farmers have the strongest learning ability; Asset type farmers have the weakest buffer capacity and subsidy type farmers have the weakest learning capacity.
(4) The greatest barrier to livelihood resilience for farmers in Jiangsu Province, China, is buffer capacity, followed by learning capacity, and finally, self-organization capacity. In the weight analysis, the buffering capacity dimension layer is the highest (0.543), followed by learning capacity (0.286) and finally self-organizing capacity (0.171); In the analysis of the barrier factors, the most important barrier factors for planting type farmers, breeding type farmers and asset type farmers are the number of productive assets owned, the number of agricultural technical services mastered by laboring type farmers, the number of access to information by part-time type farmers, and the household labor income by subsidy type farmers.
The original contributions presented in the study are included in the article/Supplementary material, further inquiries can be directed to the corresponding author.
Ethical review and approval was not required for the study on human participants in accordance with the local legislation and institutional requirements. Written informed consent from the [patients/ participants OR patients/participants legal guardian/next of kin] was not required to participate in this study in accordance with the national legislation and the institutional requirements.
CH: data curation, original draft, visualization, formal analysis, and writing—reviewing and editing. JD: supervision, conceptualization, writing, investigation, and funding acquisition. All authors have read and agreed to the published version of the manuscript.
This research is funded National Key Fund for Philosophy and Social Sciences, Grant Number 22AKS017.
The authors declare that the research was conducted in the absence of any commercial or financial relationships that could be construed as a potential conflict of interest.
All claims expressed in this article are solely those of the authors and do not necessarily represent those of their affiliated organizations, or those of the publisher, the editors and the reviewers. Any product that may be evaluated in this article, or claim that may be made by its manufacturer, is not guaranteed or endorsed by the publisher.
The Supplementary Material for this article can be found online at: https://www.frontiersin.org/articles/10.3389/fsufs.2023.1250564/full#supplementary-material
Ado, A. M., Savadogo, P., and Abdoul-Azize, H. T. (2019). Livelihood strategies and household resilience to food insecurity: insight from a farming community in Aguie district of Niger. Agricult. Hum. Values 36, 747–761. doi: 10.1007/s10460-019-09951-0
Alary, V., Caulfield, M. E., Amsidder, L., Juanes, X., Boujenane, I., Sraïri, T. M., et al. (2022). Heterogeneity of resilience of livelihood strategies in pastoral and agropastoral farming systems of rural semi-arid to arid areas in Morocco. Front. Sustain. Food Syst. 5, 994. doi: 10.3389/fsufs.2021.723994
Badewa, A. S., and Dinbabo, M. F. (2023). Multisectoral intervention on food security in complex emergencies: a discourse on regional resilience praxis in Northeast Nigeria. GeoJournal 88, 1231–1250. doi: 10.1007/s10708-022-10679-4
Bauer, T., de Jong, W., Ingram, V., Arts, B., and Pacheco, P. (2022). Thriving in turbulent times: Livelihood resilience and vulnerability assessment of Bolivian Indigenous forest households. Land Use Policy 119, 146. doi: 10.1016/j.landusepol.2022.106146
Cariappa, A. G. A., Acharya, K. K., Adhav, C. A., Sendhil, R., and Ramasundaram, P. (2022). COVID-19 induced lockdown effects on agricultural commodity prices and consumer behaviour in India—implications for food loss and waste management. Socio-Econ. Plann. Sci. 82, 1160. doi: 10.1016/j.seps.2021.101160
Chen, G., Tan, W., Zhang, S., and Yan, B. (2021). Influence of interpersonal and institutional trust on the participation willingness of farmers in e-commerce poverty alleviation. Front. Psychol. 12, 727644. doi: 10.3389/fpsyg.2021.727644
Chen, J., Yin, S., Gebhardt, H., and Yang, X. (2018). Farmers' livelihood adaptation to environmental change in an arid region: a case study of the Minqin Oasis, northwestern China. Ecol. Indicat. 93, 411–423. doi: 10.1016/j.ecolind.2018.05.017
Chen, S., Wu, J., Zhou, K., and Li, R. (2023). Livelihood resilience and livelihood construction path of China's rural reservoir resettled households in the energy transition. Front. Sustain. Food Syst. 6, 761. doi: 10.3389/fsufs.2022.1046761
Chen, Z., Yan, H., and Yang, C. (2022). A study on the impact of extreme weather on the poverty vulnerability of farming households—evidence from six counties in the hubei and yunnan provinces of china. Front. Environ. Sci. 10, 857. doi: 10.3389/fenvs.2022.942857
Cheng, M. (2020). Energy conservation potential analysis of Chinese manufacturing industry: the case of Jiangsu province. Environ. Sci. Pollut. Res. Int. 27, 16694–16706. doi: 10.1007/s11356-020-08084-w
Ehsan, E., Zainab, K., and Zhang, Z. X. (2022). Understanding farmers' intention and willingness to install renewable energy technology: a solution to reduce the environmental emissions of agriculture. Appl. Energy 309, 118459. doi: 10.1016/j.apenergy.2021.118459
Hammond, J., Siegal, K., Milner, D., Elimu, E., Vail, T., Cathala, P., et al. (2022). Perceived effects of COVID-19 restrictions on smallholder farmers: Evidence from seven lower- and middle-income countries. Agric. Syst. 198, 103367. doi: 10.1016/j.agsy.2022.103367
Hou, B., Liu, Q., Wang, Z., Hou, J., and Chen, S. (2022). The intermediary mechanism of social fairness perceptions between social capital and farmers' political participation: Empirical research based on masking and mediating effects. Front. Psychol. 13, 1021313. doi: 10.3389/fpsyg.2022.1021313
Huang, J., Lei, Y., Zhang, F., and Hu, Z. (2017). Spatio-temporal analysis of meteorological disasters affecting rice, using multi-indices, in Jiangsu province, Southeast China. Food Secur. 9, 661–672. doi: 10.1007/s12571-017-0689-8
Ifejika Speranza, C., Wiesmann, U., and Rist, S. (2014). An indicator framework for assessing livelihood resilience in the context of social–ecological dynamics. Global Environ. Change 28, 109–119. doi: 10.1016/j.gloenvcha.2014.06.005
Ke, W., Chen, W., and Yu, Z. (2017). Uncovering spatial structures of regional city networks from expressway traffic flow data: a case study from Jiangsu Province, China. Sustainability 9, 1541. doi: 10.3390/su9091541
Kuuwill, A., Kimengsi, J. N., and Campion, B. B. (2022). Pandemic-induced shocks and shifts in forest-based livelihood strategies: learning from COVID-19 in the Bia West District of Ghana. Environ. Res. Lett. 17, 70. doi: 10.1088/1748-9326/ac70ed
Lan, J., Song, B. Q., Li, Q. M., and Liu, Z. (2022). Farmers' livelihood strategies and sensitivity to climate change: evidence from southwest China. Indoor Built Environ. 10, 97065. doi: 10.1177/1420326X221097065
Li, E., Deng, Q., and Zhou, Y. (2022a). Livelihood resilience and the generative mechanism of rural households out of poverty: an empirical analysis from Lankao County, Henan Province, China. J. Rural Stud. 93, 210–222. doi: 10.1016/j.jrurstud.2019.01.005
Li, T., Cai, S., Singh, R. K., Cui, L., Fava, F., Tang, L., et al. (2022b). Livelihood resilience in pastoral communities: Methodological and field insights from Qinghai-Tibetan Plateau. Sci. Total Environ. 838, 155960. doi: 10.1016/j.scitotenv.2022.155960
Li, Z., Li, G., Zhang, K., and Zhu, J. (2022c). Do social pension and family support affect farmers' land transfer? Evid. China. Land 11, 4. doi: 10.3390/land11040497
Liang, F., and Cao, L. (2021). Linking employee resilience with organizational resilience: the roles of coping mechanism and managerial resilience. Psychol. Res. Behav. Manag. 14, 1063–1075. doi: 10.2147/PRBM.S318632
Liu, W., Li, J., and Xu, J. (2019). Evaluation of rural household's livelihood resilience of the relocation and settlement project in contiguous poor areas. Arid Area Geograph. 42, 673–680. doi: 10.12118/j.issn.1000-6060.2019.03.24
Manlosa, A. O. (2022). Operationalizing agency in livelihoods research: smallholder farming livelihoods in southwest Ethiopia. Ecol. Soc. 27, 1. doi: 10.5751/ES-12887-270111
Muktasam, A., Reid, R., Race, D., Wakka, A. K., Oktalina, S. N., Agusman, H.erawati, T., et al. (2019). Enhancing the knowledge and skills of smallholders to adopt market-oriented tree management practices: lessons from Master TreeGrower training courses in Indonesia. Austral. For. 82, 4–13. doi: 10.1080/00049158.2019.1605681
Nath, S., van Laerhoven, F., Driessen, P., and Nadiruzzaman, M. (2020). Capital, rules or conflict? factors affecting livelihood-strategies, infrastructure-resilience, and livelihood-vulnerability in the polders of bangladesh. Sustain. Sci. 15, 1169–1183. doi: 10.1007/s11625-020-00818-6
Pan, X., Li, J., Wei, J., Yue, Y., and Liu, L. (2022). Measuring green development level at a regional scale: framework, model, and application. Environ. Monit. Assess. 194, 343. doi: 10.1007/s10661-022-09953-2
Quan, X., and Doluschitz, R. (2021). Factors influencing the adoption of agricultural machinery by chinese maize farmers. Agriculture 11, 190. doi: 10.3390/agriculture11111090
Quandt, A. (2018). Measuring livelihood resilience: The Household Livelihood Resilience Approach (HLRA). World Develop. 107, 253–263. doi: 10.1016/j.worlddev.2018.02.024
Razzaq, A., Xiao, M., Zhou, Y, Liu, H., Abbas, A., and Liang, W. (2022b). Impact of participation in groundwater market on farmland, income, and water access: evidence from Pakistan. Water 14, 1832. doi: 10.3390/w14121832
Razzaq, A., Xiao, M., Zhou, Y., Anwar, M, Liu, H., and Luo, F. (2022a). Towards sustainable water use: factors influencing farmers' participation in the informal groundwater markets in Pakistan. Front. Environ. Sci. 10, 944156. doi: 10.3389/fenvs.2022.944156
Saja, A. M. A., Teo, M., Goonetilleke, A., and Ziyath, A. M. (2021). A critical review of social resilience properties and pathways in disaster management. Int. J. Disast. Risk Sci. 12, 790–804. doi: 10.1007/s13753-021-00378-y
Sarker, M. N. I., Wu, M., Alam, G. M. M., and Shouse, R. C. (2020). RETRACTED: Livelihood resilience of riverine island dwellers in the face of natural disasters: Empirical evidence from Bangladesh. Land Use Policy 95, 599. doi: 10.1016/j.landusepol.2020.104599
Ssennoga, M., Kisira, Y., Mugagga, F., and Nadhomi, D. (2022). Resilience of persons with disabilities to climate induced landslide hazards in the vulnerable areas of Mount Elgon, Uganda. Int. J. Disast. Risk Reduct. 80, 3212. doi: 10.1016/j.ijdrr.2022.103212
Stanford, R. J., Wiryawan, B., Bengen, D. G., Febriamansyah, R., and Haluan, J. (2017). The fisheries livelihoods resilience check (FLIRES check): a tool for evaluating resilience in fisher communities. Fish Fisher. 18, 1011–1025. doi: 10.1111/faf.12220
Sun, Y., Wang, Y., Huang, C., Tan, R., and Cai, J. (2023). Measuring farmers' sustainable livelihood resilience in the context of poverty alleviation: a case study from Fugong County, China. Humanit. Soc. Sci. Commun. 10, 75. doi: 10.1057/s41599-023-01575-4
Tran, V. T., An-Vo, D.-A., Mushtaq, S., and Cockfield, G. (2022). Nuanced assessment of livelihood resilience through the intersectional lens of gender and ethnicity: evidence from small-scale farming communities in the upland regions of Vietnam. J. Rural Stud. 92, 68–78. doi: 10.1016/j.jrurstud.2022.03.011
Varis, O., Keskinen, M., and Nuorteva, P. (2010). Water, livelihoods and climate change adaptation in the Tonle Sap Lake area, Cambodia: learning from the past to understand the future. J. Water Clim. Change 1, 87–101. doi: 10.2166/wcc.2010.010
Wang, B., and Dong, H. (2023). Research on the farmers' agricultural digital service use behavior under the rural revitalization strategy—based on the extended technology acceptance model. Front. Environ. Sci. 11, 80072. doi: 10.3389/fenvs.2023.1180072
Wang, H., Dill, S. E., Zhou, H., Ma, Y., Xue, H., Sylvia, S., et al. (2021a). Health, economic, and social implications of COVID-19 for China's rural population. Agric Econ 52, 495–504. doi: 10.1111/agec.12630
Wang, L., and Zhao, Y. (2023). Will social network relationship significantly enhance farmers' participation in the supply of small water-saving irrigation and water conservancy Facilities in China? Agriculture 13, 1. doi: 10.3390/agriculture13010216
Wang, P., Li, Y., and Zhang, Y. (2021b). An urban system perspective on urban flood resilience using SEM: evidence from Nanjing city, China. Nat. Hazards 109, 2575–2599. doi: 10.1007/s11069-021-04933-0
Wang, Q., Dong, W., Yang, K., Ren, Z., Huang, D., Zhang, P., et al. (2021c). Temporal and spatial analysis of COVID-19 transmission in China and its influencing factors. Int. J. Infect. Dis. 105, 675–685. doi: 10.1016/j.ijid.2021.03.014
Wang, Z., Huang, L., Yin, L., Wang, Z., and Zheng, D. (2022). Evaluation of sustainable and analysis of influencing factors for agriculture sector: evidence From Jiangsu Province, China. Front. Environ. Sci. 10. doi: 10.3389/fenvs.2022.836002
Wu, J., Zuo, J., Li, L., and Liu, S. (2023). Evaluation and influencing factors of farmers' sustainable livelihood response to ecocultural tourism in minority areas of China. Front. Environ. Sci. 10, 277. doi: 10.3389/fenvs.2022.1080277
Xiang, K., Zheng, N., Chen, J., and Gao, W. (2022). Evaluation and obstacle degree analysis of low-carbon development level in Fujian province–based on entropy weight TOPSIS method. Front. Energy Res. 10, 293. doi: 10.3389/fenrg.2022.948893
Yang, X., Guo, S., Deng, X., Wang, W., and Xu, D. (2021). Study on livelihood vulnerability and adaptation strategies of farmers in areas threatened by different disaster types under climate change. Agriculture 11, 11. doi: 10.3390/agriculture11111088
Yu, L., and Nilsson, J. (2018). Social capital and the financing performance of farmer cooperatives in Fujian Province, China. Agribusiness 34, 847–864. doi: 10.1002/agr.21560
Yue, S., Xue, Y., Lyu, J., and Wang, K. (2023). The effect of information acquisition ability on farmers' agricultural productive service behavior: an empirical analysis of corn farmers in Northeast China. Agriculture 13, 3. doi: 10.3390/agriculture13030573
Zhang, X., Zhang, Q., and Sun, T. (2019). Performance evaluation and obstacle factors analysis of urban public transport priority. Transport. Plann. Technol. 42, 696–713. doi: 10.1080/03081060.2019.1650433
Zhao, X., Chen, H., Zhao, H., and Xue, B. (2021). Farmer households' livelihood resilience in ecological-function areas: case of the Yellow River water source area of China. Environ. Develop. Sustainab. 24, 9665–9686. doi: 10.1007/s10668-021-01827-w
Zhao, X., Xiang, H., and Zhao, F. (2023). Measurement and Spatial Differentiation of Farmers' Livelihood Resilience Under the COVID-19 Epidemic Outbreak in Rural China. Soc. Indic Res. 166, 239–267. doi: 10.1007/s11205-022-03057-7
Zheng, Y., Jacobsen, J. B., Thorsen, B. J., and Liu, Z. (2023). How do rural households prefer to adapt livelihoods to economic effects of climate and policy changes?. The Singapore Econ. Rev. 68, 265–284. doi: 10.1142/S0217590819500061
Zhou, J., Wu, Y., Liu, F., Tao, Y., and Gao, J. (2021). Prospects and obstacles analysis of applying blockchain technology to power trading using a deeply improved model based on the DEMATEL approach. Sustain. Cities Soc. 70, 910. doi: 10.1016/j.scs.2021.102910
Zhu, C., Zhou, Z., Ma, G., and Yin, L. (2021). Spatial differentiation of the impact of transport accessibility on the multidimensional poverty of rural households in karst mountain areas. Environ. Develop. Sustain. 24, 3863–3883. doi: 10.1007/s10668-021-01591-x
Keywords: livelihood resilience, COVID-19 pandemic, entropy method, obstacle factor, Jiangsu Province, China
Citation: Hu C and Dong J (2023) Measuring livelihood resilience of farmers and diagnosing obstacle factors under the impact of COVID-19 in Jiangsu Province, China. Front. Sustain. Food Syst. 7:1250564. doi: 10.3389/fsufs.2023.1250564
Received: 30 June 2023; Accepted: 18 September 2023;
Published: 05 October 2023.
Edited by:
Apurbo Sarkar, The University of Queensland, AustraliaReviewed by:
Lu Qian, Northwest A&F University, ChinaCopyright © 2023 Hu and Dong. This is an open-access article distributed under the terms of the Creative Commons Attribution License (CC BY). The use, distribution or reproduction in other forums is permitted, provided the original author(s) and the copyright owner(s) are credited and that the original publication in this journal is cited, in accordance with accepted academic practice. No use, distribution or reproduction is permitted which does not comply with these terms.
*Correspondence: Jiayun Dong, ZGp5MjAyMEBuamZ1LmVkdS5jbg==
Disclaimer: All claims expressed in this article are solely those of the authors and do not necessarily represent those of their affiliated organizations, or those of the publisher, the editors and the reviewers. Any product that may be evaluated in this article or claim that may be made by its manufacturer is not guaranteed or endorsed by the publisher.
Research integrity at Frontiers
Learn more about the work of our research integrity team to safeguard the quality of each article we publish.