- 1Institute for Scientific Interchange (ISI Foundation), Turin, Italy
- 2School of Politics and International Relations, University College Dublin, Dublin, Ireland
- 3World Food Programme, Research, Assessment and Monitoring Division (RAM), Rome, Italy
- 4Department of Network and Data Science, Central European University, Vienna, Austria
Food security is recognized as an inherent human right, enshrined within the principles of the Agenda 2030. The Global Report of Food Crises 2022 points out 193 million people facing severe food insecurity across 53 countries, posing challenges to decision-makers and institutions. Among the many causes of food crises, violent conflict, economic shocks, and environmental pressures are the most influential. In this work, we focus primarily on the conflict-related domain. Finding a stable relationship between conflict and food insecurity is complex for several reasons: first, the relationship is mutually reinforcing; second, the full impact of conflict on food insecurity may take time to have an effect; and third, conflict itself is a multidimensional phenomenon and can include multiple types of violent events. This research set out to comparatively assess the impact of different types of violence on self-reported food insecurity in three prominent food crisis contexts: Burkina Faso, Syria, and Yemen. A measure of food-related classifying events was developed using a rules-based approach. The analysis showed that this approach can effectively code and classify food-related conflict in diverse contexts. By refining the search string, it becomes possible to capture food-related conflict in various food systems. Our findings point out that the new-build measure of food-related conflict is more strongly correlated to subsequent self-reported insufficient food consumption than other forms of violence. The results demonstrate that this relationship is robust across a range of data collection windows and across discrete time periods of analysis. In summary, the research suggests that focusing on the use of food and food systems as tactics in conflict can be highly valuable for understanding and addressing food insecurity.
1. Introduction
The 2022 Global Report of Food Crises points out alarming levels of hunger and in 2021 they surpassed all previous records with close to 193 million people acutely food insecure and in need of urgent assistance across 53 countries (FSIN, 2022). Global food crises severely compromise human wellbeing and pose significant challenges to policymakers and civil society alike. Among the many causes of food crises, violent conflict, economic shocks, and environmental pressures are the most influential. The pursuit of both freedom from hunger and peace from violent conflict are central to achieving the UN Sustainable Development Goals (SDGs). Specifically, Sustainable Development Goal 2 aims to eliminate hunger by 2030, while Goal 16 focuses on building peaceful and inclusive societies, recognizing that armed violence and insecurity hinder a nation's development (United Nations, 2022). To better prepare countries to tackle future food and nutrition security crises, and to respond to the social change impacting all citizens, and food-related conflict, the implementation of preparedness plans plays a pivotal role. The World Bank, in partnership with various UN agencies and collaborators, actively engages in the development and execution of Food Security Crisis Preparedness Plans. These plans encompass collaborative monitoring initiatives that compile and analyze data from diverse sources to detect early indicators of food security crises, fostering coordination among humanitarian and development organizations. Moreover, these plans establish decision-making mechanisms, facilitating a unified and scaled-up response in times of crisis. These collective efforts aim to create a more comprehensive and effective response to food crises, benefiting people in various countries and reducing their vulnerability to future food security challenges, in alignment with the 2030 Agenda for Sustainable Development (The World Bank, 2023).
In this work, we focus primarily on the conflict-related domain. The Institute for Peace and Security Studies states that there is a complex link between peace, conflict, and food security because conflict not only causes loss of resources but also affects the ability to produce, hampers the regular farming and non-farming activities, and damages infrastructures (Africa Portal, 2021). The relationship between conflict and food insecurity is complex first because it is mutually reinforcing: conflict leads to food insecurity and food insecurity in turn aggravates conflict. Second, conflict's effects are also long-lasting: the full impact on food security may take time to become apparent and may last long after the violence ends. Third, finding a stable relationship between the two variables is complicated by the concurrent effects of the other drivers—including economic shocks and climate factors—that affect people's vulnerability. Also, conflict itself is a complex and multidimensional phenomenon: any single conflict may include multiple violence tactics such as violence against civilians and clashes between armed groups; conflict can involve prolonged periods of civil or interstate war which disrupts the entire economy, and/or violence specifically involving the food system.
The goal of this work is to build a comprehensive data-driven framework to study the role played by different classes of conflict on food insecurity through time. Efforts to explore conflict dynamics on the level of food insecurity are crucial to contribute to the growing body of research that demonstrates the value of analysis of micro-dynamics of conflict (Cederman and Gleditsch, 2009; Justino et al., 2013). In classifying conflict, we define two main classes of conflict: food-related conflict and non-food-related conflict, together with other subclasses of conflict based on the event type. In particular, this work addresses the following research questions: (1) Is the new sub-type of events (food-related conflict) more or less useful in understanding food security than the more conventional measures?; (2) more generally, is there a class of conflict that shows a predominant relationship with the level of food insecurity in all contexts and over time?; (3) if not, which classes of conflict influence food crises?; (4) How much the relationship changes over time? To achieve these aims, we refer to previous research conducted by Dowd (2022), in which she found that food-related conflict is more strongly correlated to subsequent food crisis than either general—or other sub-categories of—violent events across the sample, pointing to the distinct profile of this modality of violence.
Our analysis extends this study in several directions: (1) we expand to a wider range of countries (Syria and Yemen in addition to Burkina Faso) testing the methodological approach in a wider sample beyond sub-Saharan Africa alone; (2) we classify events in the two main classes (food-related events or non-food-related events) and we refine not only the search terms accordingly, but also the rule-based method employed in this work, that explore the words included in the event description to infer the category of the event; (3) we replace a count of the number of people estimated by IPC to be in food crisis with self-reported insufficient food consumption—a different measure of food insecurity; (4) we rely on a measure of food insecurity with higher time resolution (daily time series vs. semi-annual time series, the spatial resolution is the same: admin1 level). Further, we adapt the model in several ways: (5) we include different control variables such as the shocks in food prices and we apply the non-negative matrix factorization method to focus on the best subset of food items for each available admin; (6) we verify if the general hypothesis on a single case by examining separately each country; and (7) we explore whether the role played by different measures of conflict change over time by performing the analysis over time.
Since each driver of food insecurity reinforces the other creating complex situations that are not easily resolved (Sleet, 2020), we adopt a multidimensional approach and consider a multivariate model that controls several potentially confounding factors that explain food security levels. The bivariate model that considers only the conflict variable suffers from omitted variables bias: if some of the omitted variables are correlated with conflict as well, then the coefficient on the conflict variable will be biased. To take this into account, we include in the study information about economic shocks and environmental pressures. Food price volatility is closely connected to food availability, access to food, food utilization, and stability over time (FAO, 1996, 2015). Price shock-related food and nutrition insecurity may result in a less-than-adequate food supply and undermine the resilience of low-income countries. Climate change has led to droughts, floods, and many other unpredictable events, resulting in crops not having enough water and wilting and/or crops and infrastructure being destroyed (The Economist, 2023). The main purpose of this research is the focus on the role played by the different subclasses of conflict, but we account for these issues as cofactors.
The article is organized as follows. First, we introduce the case study and descriptions of the data. Next, we present the analytical strategy and the results. In the last section, we discuss the implications of the key findings, followed by concluding remarks.
2. Materials and methods
2.1. Study area and data
This analysis encompasses three countries: Syria, Yemen, and Burkina Faso. These cases were selected for a number of reasons. First, empirically, our research focuses on countries experiencing acute food insecurity driven by conflict, as well as the escalating impact of natural disasters, economic instability, and political turbulence. Yemen, Syria, and Burkina Faso are all ranked among the 2023 “hunger hotspots” of either the highest, or very high, concern by the World Food Programme and Food and Agriculture Organization (WFP and FAO, 2023). Second, geographically, our study builds upon a prior study by Dowd (2022), which exclusively focused on sub-Saharan African countries. Consequently, we opted to broaden the geographic scope of our research. We decided to expand the research to a wider range of countries including an example of an African country, and critical countries in the Middle-East that allows us to test the methodological approach in different regional contexts. Third, practically, the selection of Burkina Faso, Syria, and Yemen as a case study is also based on the availability of food security data, food prices data and environmental data spanning several years with spatial resolution at admin level.
Following more than 10 years of high-intensity conflict, an estimated 12.1 million people are in acute food insecurity in the Syrian Arab Republic (WFP and FAO, 2023). Despite improvements compared to earlier years of conflict, the security situation in the Syrian Arab Republic remained fragile. Conflict in Syria has severely impacted food security by damaging agricultural land, causing fires, and leading to deliberate arson attacks. Explosive weapons and attacks on farm workers further threaten food production. Water infrastructure destruction worsens water scarcity for crops. Additionally, the conflict disrupts food supply chains, leading to bakery closures, risks at markets, and challenges in providing humanitarian aid due to access issues and violent attacks. Finally, these effects are compounded by rapid economic deterioration and growing impacts of climate change (OCHA, 2022). Overall, it has devastating and long-lasting effects on food security (Insecurity Insights, 2023). In this complex humanitarian emergency, the civilian population has suffered enormously.
Yemen is one of the largest humanitarian crises in the world, with 21.6 million people in need of humanitarian assistance. As of May 2023, 10.1 million people (or 53% of the population) are estimated to be in acute food insecurity (WFP and FAO, 2023). Ongoing insecurity, high and rising food prices, damage to or destruction of productive assets and basic services, and severe humanitarian access restrictions have all contributed either directly or indirectly to a profound food crisis (USAID, 2019). The conflict has particularly severely impacted Yemen's agricultural sector with grain crops most affected in terms of production volumes (ACAPS, Food and Agriculture Organization of the United Nations, International Organization for Migration, UN Development Programme, 2023). Recent reporting highlights how “Yemeni farms have faced repeated airstrikes and shelling over a period of <3 years, intensifying the country's already dire food crisis [...] Fishing boats have been sunk, markets obliterated, flour mills razed, and farmers have been attacked through bombing” as reported by Norwegian Refugee Council (2020). This disruption of essential resources affects millions of people. Coupled with this, humanitarian access is particularly restricted in Yemen, with bureaucratic impediments, generalized insecurity, and continued to be challenging, with millions of people living in hard-to-reach districts, with bureaucracy and conflict the main impediments to meeting their humanitarian needs and the high number high levels of violence against humanitarian workers and assets, all affecting the ability to of populations to reach humanitarian assistance.
Burkina Faso has faced a challenging and volatile situation that has led to severe food insecurity on an unprecedented scale. As of May 2023, 3.4 million people are estimated to be in acute food insecurity (WFP and FAO, 2023). The crisis has been progressively worsening since 2018, marked by a consistent increase in the frequency and intensity of violence, exacerbating the issues of food insecurity. The growing crisis is closely tied to regionalized conflict encompassing multiple actors and forms of violence across the Sahel. Food security has been disrupted by large-scale displacement, abandonment of agricultural land, and deliberate attacks on water sources (Internal Displacement Monitoring Centre, 2023a). Climate shocks further compound vulnerabilities and undermine resilience in a country that has experienced rapid deforestation, desertification, soil erosion, and periods of drought followed by severe flooding (World Food Programme USA, 2023). Armed groups' increasing activity and expanding operational areas across the country have severely impeded movement of both civilians and humanitarian workers, while relief efforts have frequently been directly targeted, severely disrupting delivery of humanitarian assistance (Internal Displacement Monitoring Centre, 2023b). The three countries reflected the worsening acute food insecurity due to conflict-driven crises, with the growing severity of natural disasters and economic shocks as drivers. Starting from all these considerations, and considering the emergence based on the multidimensional phenomenon, we set out to understand how different forms of violence affect subsequent self-reported food insecurity in each of these three contexts. To do so, we combine data from two primary sources: the Word Food Programme (WFP) and The Armed Conflict Location and Event Data Project (ACLED, Raleigh et al., 2010). Thanks to these data sources we consider the three main domains often identified as the most important drivers of food insecurity: food insecurity data, environmental data, food prices data from the Word Food Programme, and information about conflict events from the ACLED dataset. The analysis is performed for each country at the admin 1 level, the administrative boundaries of the first sub-national level, and covers different time windows for each admin based on data availability. Table 1 details our analysis's space and time resolution. The main variables included in the final dataset are described below.
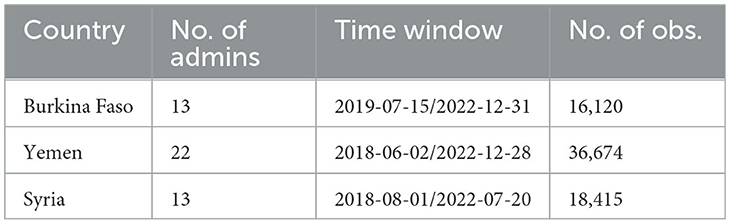
Table 1. Dataset characterization: the table reports space aspects with the number of admins considered in each case study, the available time window for each country, and the number of observations referring to each World Food Programme survey.
2.1.1. Prevalence of people with insufficient food consumption
The prevalence of people with insufficient food consumption is the target indicator of the analysis and is based on a household-level indicator: the Food Consumption Score (FCS). The FCS is computed starting from a survey of each available household. The WFP surveys record how often, during the last 7 days, people have consumed items from the different food groups: main staples, pulses, vegetables, fruit, meat and fish, milk, sugar, oil, and condiments (food group weights and thresholds are described in WFP, 2008a). Each consumption frequency is then weighted according to its relative nutritional importance to obtain the FCS = Σwixi where wi is the weight of food group i and xi the frequency of its consumption by the household, that is the number of days for which the food group was consumed during the past 7 days. Once the food consumption score is calculated, each household is then assigned a food consumption group (poor, borderline, or acceptable) based on standard thresholds, eventually adapted based on specific consumption behaviors in the country of interest (Martini et al., 2021). Finally, the prevalence of people with insufficient food consumption is obtained as the prevalence of households in the sample with poor or borderline food consumption (IPC, 2019).
2.1.2. Conflict
We rely on the Armed Conflict Location and Event Data Project (ACLED), an event-based dataset that collects information on the dates, actors, locations, fatalities, and types of all reported political violence and protest events. ACLED records six event types: battles, violence against civilians, protests, explosions/remote violence, strategic developments, and riots. We exclude from the analysis records of non-violent events, strategic developments, and non-violent protests because a broader range of non-violent actions is beyond the scope of analysis. Also, we exclude all events coded as riots because they are often associated with demonstrations concerning food policy, governance, and economic management rather than the target or setting of violence (Sneyd et al., 2013; Dowd, 2022).
ACLED reports for each event the latitude and the longitude and thanks to this information we match all events to the corresponding first-level administrative unit code, and the associated WFP dataset entry. Regarding the time dimension, we consider the count of recorded violent events per admin 1 that took place in the 90 days preceding the survey with the corresponding value of the percentage of people with insufficient food consumption. We select a period of 90 days to consider the impact of violent conflict on food security, which may take some time to have an effect. Since extended exposure to violence may undermine individual, household, and community resilience to future violence shocks, increasing the number of people experiencing food insecurity in subsequent cycles, we include a measure that quantifies the past general level of violence. The conflict-related variables extracted are listed and explained in Table 2 and are involved in the first phase of correlation analysis.
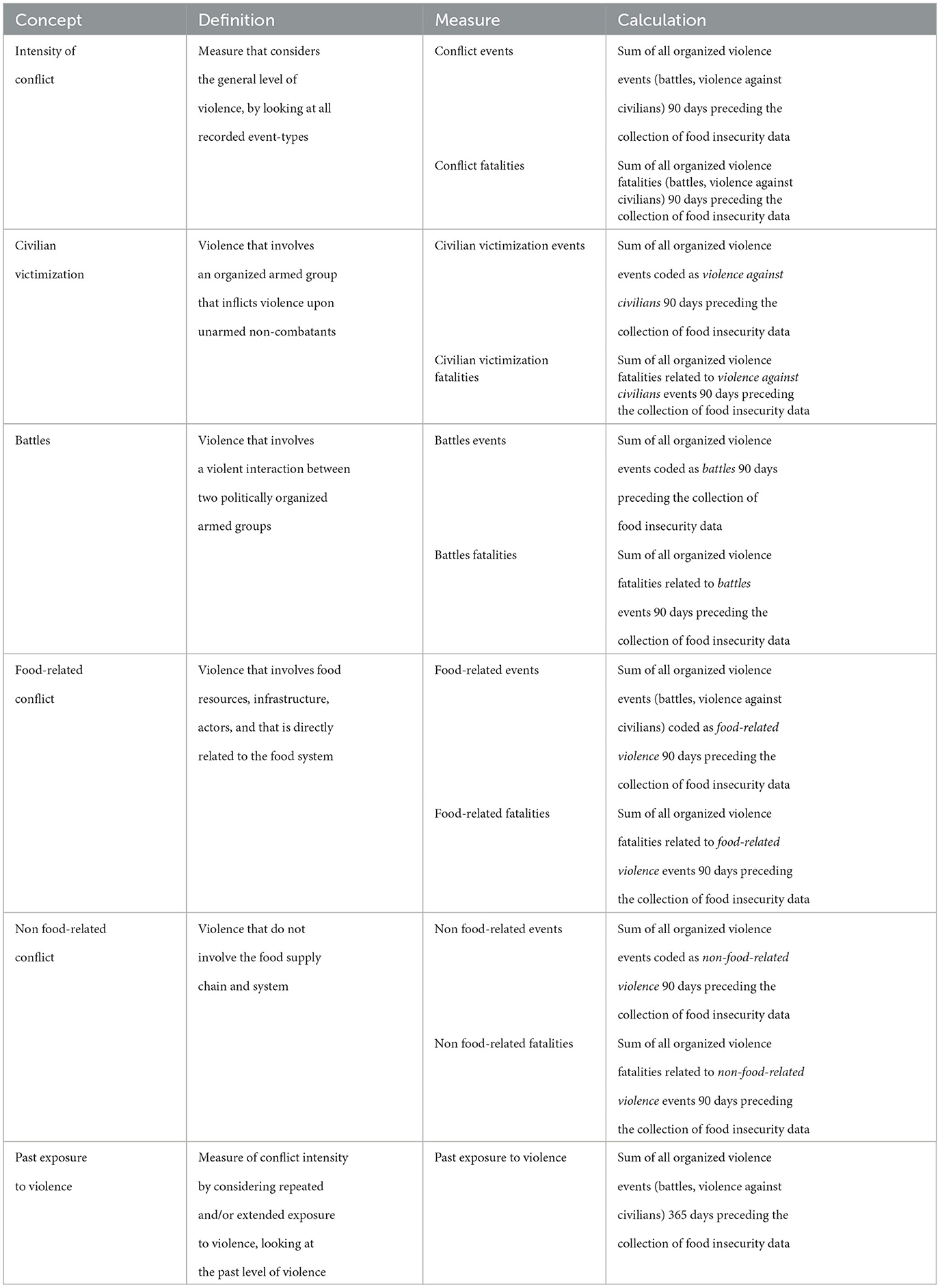
Table 2. Detailed description of conflict-related variables: concept, definition, measure, and calculation.
2.1.3. Economic shocks in food prices
Price data and Alert for Price Spikes (ALPS) indicator are publicly available on the WFP's Economic Explorer platform (WFP, 2022a). The Alert for Price Spikes (ALPS) is an indicator based on a trend analysis of monthly price data. In this study, we compare the long-term seasonal trend of a commodity's price series at each market with the last observed price in the same market. The ALPS provides a sense of the intensity of the price difference between the trend and the market price: the higher the difference, the more severe the alert (WFP, 2014). The ALPS can be applied to several markets and food commodities within a country and within each first subnational level (admin 1). For each commodity, we first average its ALPS value over all markets in which it is available, and then average over all commodities to attain the final value. We apply a time window of 3 months by averaging within this period to take into consideration that economic shocks take some time to have an effect. We then carry out the Non-Negative Matrix Factorization (NMF) on the ALPS data. The NMF method is an unsupervised algorithm that allows to the selection of the most suitable subgroups of food items to consider for each admin. We better clarify this analysis in Section 2.2.
2.1.4. Environmental variables
The WFP's Seasonal Explorer platform (WFP, 2022b) provides open data related to rainfall, rainfall anomalies, the normalized difference vegetation index (NDVI), and NDVI anomalies computed from CHIRPS (Funk et al., 2014) and MODIS data (MODIS Platform, 2000), at subnational level space resolution. The rainfall anomalies indicator is defined as the ratio between the amount of rainfall during the last 3 months and the historical average of the amount of rainfall in the same period of the year. The NDVI anomalies indicator is defined based on the last 10 days, and we average within a 3-month time window. We do the same for the two last indicators: rainfall and NDVI.
2.2. Methods
2.2.1. Food-related conflict classification
To construct a new measure of conflict that is directly related to the food systems, food actors, and food resources, we classify conflict into two classes: food-related conflict and non-food-related conflict. We define food-related conflict as the category of violent events directly connected to food system sites, food actors, components of the food system, and the food supply chain. In distinguishing between violent events and conflict more widely, “events” refer to individual targeted acts of violence—for example, an individual clash between parties to a conflict, or an attack on civilians. These are distinguished from broader violent conflict—often a long-running or geographically widespread series of connected events or larger category of events (ACLED, Raleigh et al., 2010).
Our classification methodology builds upon the prior research conducted by Dowd (2022). In her work, she analyzed textual descriptions of conflict events and applied a rule-based approach to classify violent events in 16 sub-Saharan countries. We have further refined this method to encompass a broader spectrum of countries, characterized by different geographical locations and contextual factors. This rule-based method is employed to categorize events as either food-related or non-food-related. It relies on the development of a set of rules that are derived from the expertise within the domain. These rules serve as the foundation for assigning labels to specific events. To facilitate this classification process, we utilize the descriptive event notes provided by the Armed Conflict Location and Event Data Project (ACLED), which offer narrative details describing each event. To distinguish between food-related and non-food-related events, we have established predetermined criteria primarily based on the wording contained within the event description notes and guide our decision-making process, following a series of “if... then...” rules.
The classification involves the process of searching for specific words included in the text description which may refer to food and food system. Specifically, this algorithm identifies events where food system sites (such as farms, factories, markets, etc.), actors (including farmers and pastoralists), or elements (such as livestock or specific crops) are mentioned in the descriptions of violence. When these references are present, the event is coded as food-related. In a small number of cases, events in which one or more key terms are present are nevertheless coded as non-food-related where potential ambiguities in certain words that must be excluded to prevent false positives. For example, the term “field” may be mistakenly categorized as food-related, even though it can refer to other contexts like an airfield or an oil field. Furthermore, specific locational terms like “north of the market” may lead to misclassifications, as they are mentioned solely as reference points. More generally, events referring broadly to living standards, living conditions, payments of wages, but with the absence of any reference to food prices, the cost of food, access to or availability of food are coded as non-food-related. However, if there is reference to any of the food-specific terms (food prices, quality, availability), then these would be food-related events. General insecurity such as shelling, bombings, protests etc. in which there is no reference to food, food systems or food system actors, or food system locations are coded as non-food-related, even if these may have an indirect effect on food security outcomes. This approach is taken because the study's primary objective is to focus on a narrower subset of events that align with the specific concept of food-related conflict.
We improve the already employed method by adding new rules during the classification process: (1) we create a list of exclusion terms divided into three categories for building different rules: unigrams, bigrams, and positional terms; (2) to avoid false positives and classify events as non-food-related when they do not directly occur in a place related to the food system, we add a rule that looks for the association of a positional term with a search term related to locations (e.g., near to the farm, near a field, on the north of a market); (3) to avoid false negatives we consider the likelihood to deal with the simultaneous presence of an ambiguous word and relevant term. We find that for the majority of the cases when an event includes at least one exclusion term, the event is not directly describing food-related violence. However, there are two highly relevant food-related terms that are definitely associated with food resources (food and foodstuff ), and for this reason, we add a rule that allows classifying the event as food-related even if there are one or more exclusion terms. To clarify the classification process we show some examples in Table 3.
Starting from a list of 31 search terms and six exclusion terms (Dowd, 2022), we extend it and build a new list of 63 search terms and 36 exclusion terms (without considering all the plurals of those words): the full list of food-related words and exclusion terms together with details about the applied method are reported in the Supplementary material. We test the results by manually labeling 858 event notes, extracted randomly from the original dataset, related to both classes. Firstly we make an inter-annotator test on 50 notes and we conclude a complete agreement on the manual annotations. Then, we test the automatic rule-based method on the subset of notes manually annotated. The results show high precision and recall in both classes: precision = 93% in Food-Related Class and precision = 99% in Non-Food-Related Class; recall = 99% in Food-Related Class and recall = 91% in Non-Food-Related Class.
Despite the development of extensive rules and resulting high level of precision and recall reported above, it is important to note that this approach has a number of limitations, discussed further below. Critically, ACLED data is not specifically collected with the purpose of recording food-related violence. As a result, it is possible that the descriptive notes—although detailed—do not capture the full universe of food-related violence that has taken place, as these details may be lost in reporting or subsequent coding. Nevertheless, in the absence of a dedicated dataset specifically designed to record and monitor food-related violence, we maintain that ACLED remains the most suitable source of data for analysis of this important phenomenon.
2.2.2. Non-negative matrix factorization method on shocks in food prices
In this research, we include as a control variable information about shocks in food prices. As we described in the Section 2.1.3, we use Alert for Price Spikes (ALPS) indicator data from WFP's Economic Explorer platform. The indicator is available at the admin 1 level and, depending on the admin, the ALPS data can be available for one or more markets. For each registered market, we have information for several food items that could be different among areas and countries. Also, following the WFP technical guidelines (WFP, 2008b), we consider different weights for commodities that belong to different categories (main staples, pulses, vegetables, fruit, meat and fish, milk, sugar, oil, condiments), based on the nutrient density of the food groups. In the early stage of the research, we considered all the available food commodities for each market and we weighted the average on markets to obtain an aggregated variable at the admin 1 level.
Then, following the methodology applied in Kalimeri et al. (2019), we propose an unsupervised framework able to extract for each admin the group of commodities that is in best correlation with the general trend of the shocks in food prices. The goals are to: (1) avoid biases caused by considering all available food items; (2) not select a priori a list of food items to consider in the study; (3) build an aggregated price-related variable specific for each admin; (4) explore whether the relationship between the variable obtained after applying the unsupervised method and the target variable improves. By using the monthly time series of ALPS data for each food commodity (considering a single admin) in the form of a matrix, we employ an approach based on Non-negative Matrix Factorization (NMF) to extract latent features of the matrix that correspond to linear combinations of groups of food items (Cichocki et al., 2006). We can select for each admin the latent component that better correlates with the general trend of shocks in food prices time series obtained in the first stage of the analysis. This component represents the combination of food items that more closely represent the general time series considering all available food commodities. We finally update the prices-related variable with the new one that comes out from the extracted linear combination. More details about the applied methodology are available in the Supplementary material. To evaluate whether the applied unsupervised method improves the relationship between the food prices variable and the percentage of people with insufficient food consumption, we compare the two Pearson correlation coefficients. By considering all available food items to derive shocks in the food prices variable we obtain a Pearson correlation coefficient ρ = 0.15 (p-value = 0.00); after applying the NMF method the Pearson correlation coefficient between the newly constructed variable and the target is ρ = 0.31 (p-value = 0.00).
2.2.3. Prelimary admin selection, correlation analysis, and feature selection
We first examine the descriptive statistics of all the variables included in the study. Considering the statistics of conflict-related variables, we notice that some admins show an average of zero events over the entire study period. Since the purpose of the paper is to characterize which groups of conflict are most correlated with the trend of food insufficiency, it is useful to focus on how the number of conflict fluctuates over time in regions that show the presence of conflict. For this reason, we find it functional to include a preliminary step with the aim to select only those admins characterized by a certain level of conflict. In order not to be too restrictive, we set a threshold th = 3, which means that only admins with at least one conflict per month in the preceding 3-month period are selected. More details and statistics of the admin selection step are reported in the Supplementary material.
Together with the admin selection analysis, we explore the correlation among the conflict-related variables, comparing separately the categories that measure the number of events and the ones that measure the number of fatalities. The two correlation matrices presented in Figure 1 and in Figure 2 show similar relationships among the subcategories. In particular, we notice a perfect positive correlation between the number of non-food-related violent events and the number of total violent events, most likely due to the fact that the number of non-food-related events is the most represented class, accounting for 90% of total conflict. We get the same result for the non-food-related fatalities and the total number of fatalities. Also, the number of battles (both events and fatalities) has a strong relationship with both non-food-related conflict and total conflict. The food-related category shows a moderate correlation with the total conflict, a weak correlation with the battles category and it shows a stronger correlation with violence against civilians events, which falls into a moderate correlation while considering the number of fatalities. In order to assess the relative value of the different conflict variables (which one is most useful for understanding the food crisis), and to avoid multicollinearity problems due to the noted relationships, we consider it valuable to run the models separately to allow a direct comparison of how they perform. Finally, we perform a correlation analysis for each country based on the Variance Inflation Factor (VIF) and the Person correlation coefficient, to detect highly collinear features among the initial set of control variables. The feature selection step removes the NDVI anomalies variable from all the country-specific datasets, the rainfall anomalies and the NDVI variable from Burkina Faso's dataset, the NDVI from Syria's dataset, and the rainfall anomalies from Yemen's dataset. Further details are reported in the Supplementary material.
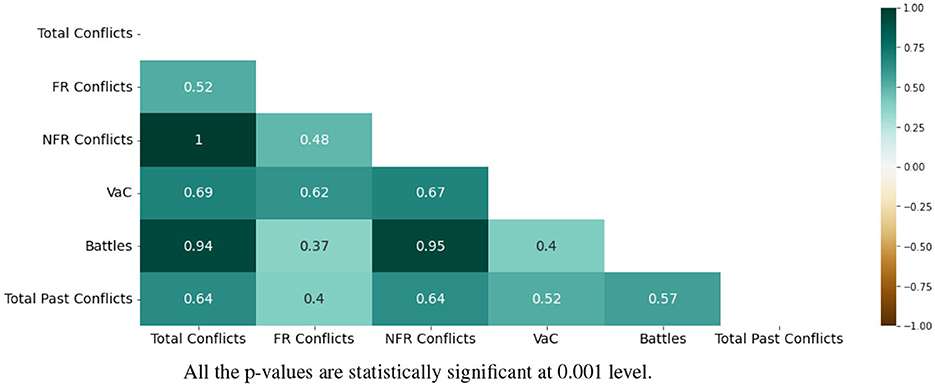
Figure 1. Correlation heatmap of conflict-related variables obtained by considering the number of events. All the p-values are statistically significant at 0.001 level.
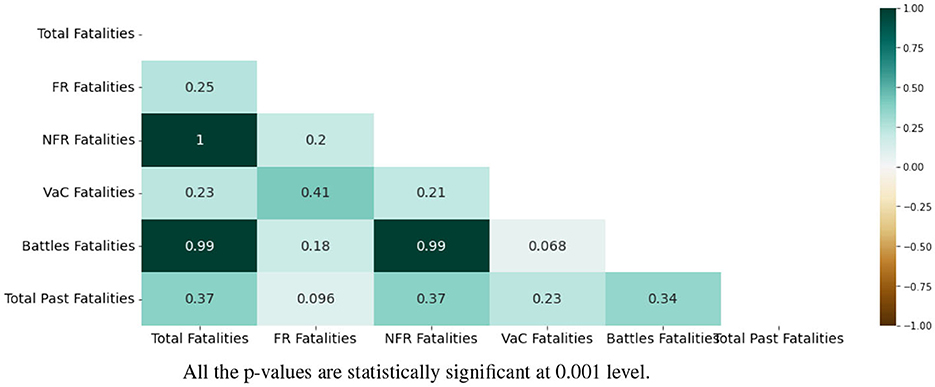
Figure 2. Correlation heatmap of conflict-related variables obtained by considering the number of fatalities. All the p-values are statistically significant at 0.001 level.
2.2.4. Panel regression models
The complete dataset is a panel dataset, a combination of cross-sectional and time-series data. We repeatedly observe admins over different periods. The panel dataset leads to a more accurate inference of model parameters and it has a greater capacity for capturing the complexity of human behavior than a single cross-section or time series data (Hsiao, 2007). To select the most suitable model for the data, we conducted the recommended tests to choose among: PooledOLS, Fixed-Effect Model, and Random-Effect Model. More details on the models are reported in the Supplementary material. Table 4 clarifies the model selection process. Since both the Wald test and the Breusch-Pagan LM test turn out to be significant and the Hausman test shows a p-value lower than 0.05, which leads to rejecting the null hypothesis, we select the Fixed-Effect model. Indeed, the individual characteristics have an effect on the regressors because countries', but also admins' conflict situations may be affected by their own internal characteristics such as cultural characteristics, type of government, political environment, etc. The entity and time-fixed effects regression model is represented by the following equation:
where Yit is the outcome variable (for entity i at time t); αi is the unknown intercept for each entity (nentity-specific intercepts); β is the coefficients that represents a common effect across entities controlling for individual and time heterogeneity; Xit is a vector of predictors (for entity i at time t); δt is the unknown coefficient for the time regressors (t), ui is the within-entity error term; eit is the overall error term.
3. Results
3.1. Time trends and spatial aspects of insufficient food consumption and conflict-related variables
We based our study on daily sub-national time series of the prevalence of people with insufficient food consumption in three countries considered to be affected by high levels of food insecurity and food crises (Burkina Faso, Syria, and Yemen). As mentioned in Section 2.1, we have available different study periods for each country, and for this reason, the time series length varies across countries. Also, we do not have data for all administrative units of a country. To better understand the structure and profile of the data on which we work, we report in Table 5 a general statement of the level of insufficient food consumption and the level of conflict through brief descriptive statistics at the country level. Figure 3 shows the time trends of insufficient food consumption and of the two new-build categories of conflict. For each country, we select an example, and in particular, we report the time series of the admin that shows the highest average of food-related conflict, after having normalized by the population. Through these plots, we can directly visualize the analyzed time series. More granular graphs included in the Supplementary material show the level of insufficient food consumption and conflict for all admins of a country. Exploring the situation subnationally is useful to highlight how dynamic the values are over time, and how they differ across different sub-national units. To better understand the spatial aspects of the phenomenon, we visualize on a map the level of insufficient food consumption and the intensity of food-related and non-food-related conflict (Figure 4). Specifically, we plot the distribution of the averaged values of percentage of people with insufficient food consumption in 2021, by the admin, and the distribution of total number of food-related and non-food-related conflict in 2021, normalized per 100,000 inhabitants of each admin. We use the year 2021 as an illustrative example, but the spatial plots for all the years are provided in the Supplementary material.
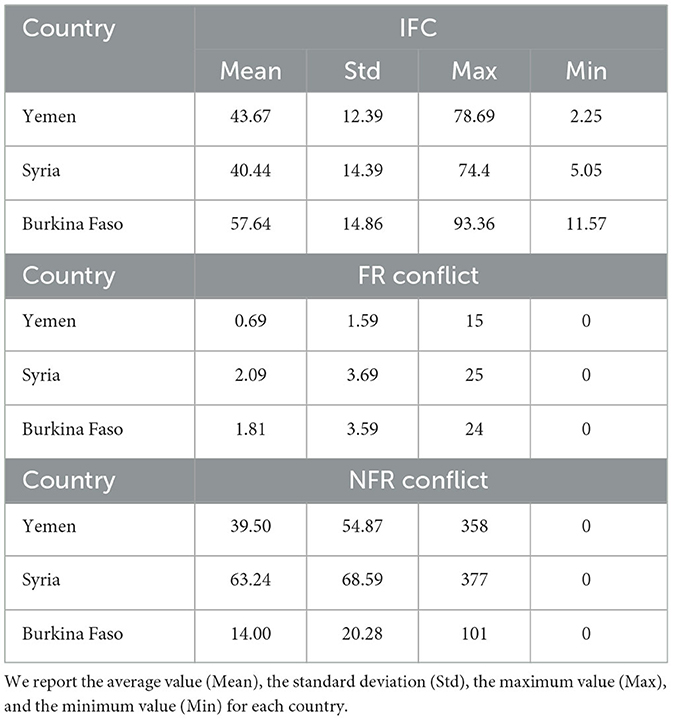
Table 5. Descriptive statistics at country level to understand the general level of insufficient food consumption (IFC, above), food-related conflict (FR Conflict, central), and non-food-related conflict (NFR Conflict, bottom).
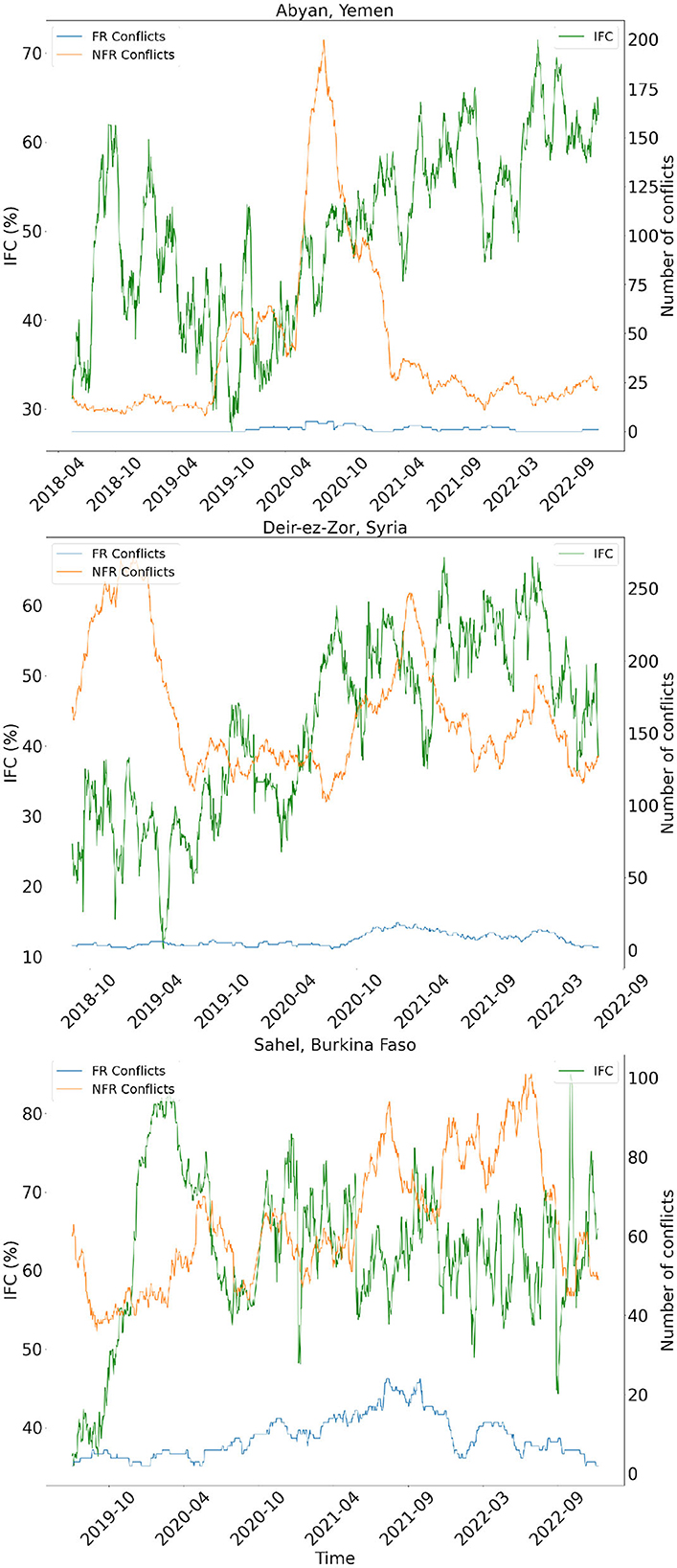
Figure 3. Time series trends. The plots show the time series of percentages of people with insufficient food consumption, food-related, and non-food-related conflict, for a specific admin in each country.
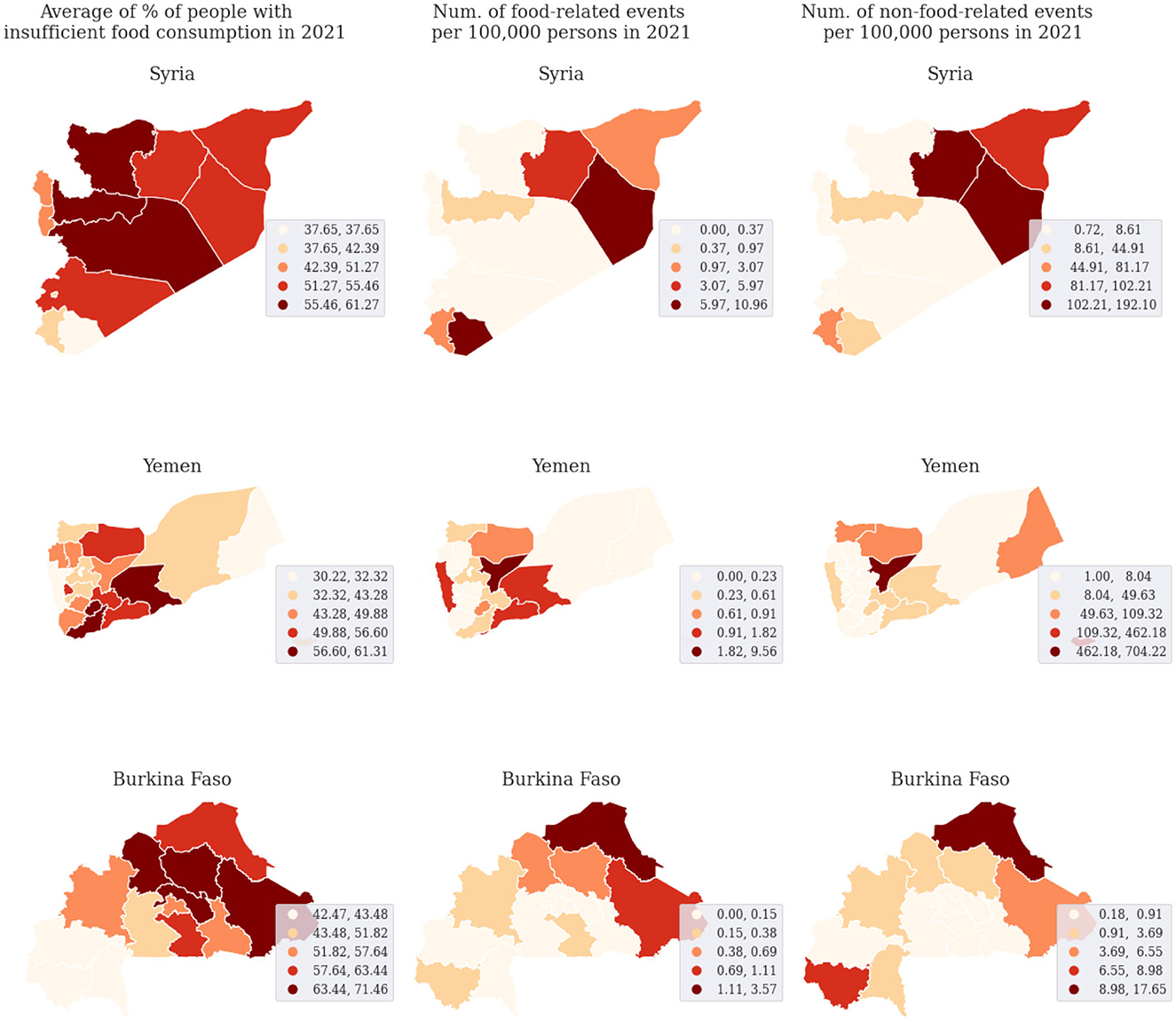
Figure 4. Spatial maps. The figure shows the level of food insecurity and conflict per each country: Syria (Top), Yemen (Middle), and Burkina Faso (Bottom). The maps report the averaged level of the percentage of people with insufficient food consumption in 2021 (left), the total number of food-related (center), and non-food-related conflict events (right) per 100,000 persons in 2021.
3.2. Regression results
We run the panel regression model for each country as described in Section 2.2.4, by including time-fixed effects considering all the different month-year time periods. We do not include in the model entity effects since the population variable already takes into account them. To evaluate the role played by different measures of conflict we run and compare several models; each model includes one conflict-related variable and all the controls. Tables 6–8 show the results related to the event-based conflict categories for each country-specific dataset. The results show that all categories of events have a statistically significant and positive relationship to the percentage of people with insufficient food consumption (except the sub-category of violence against civilians in Yemen), but food-related conflict has the strongest correlation. Food-related conflict has a strong relationship with subsequent food crisis and this relationship is stronger than considering total conflict or other sub-categories of conflict. In particular, its coefficient is between four times (Burkina Faso) and twenty times (Yemen and Syria) larger than both total events and non-food related events. These results further support the findings of Dowd (2022) on sub-Saharan African countries and expand on these by considering separate countries individually. We also test the hypothesis by comparing the number of fatalities as a dependent variable, and as a measure of conflict intensity, and the related results are reported in the Supplementary material. For Syria and Burkina Faso, the results are consistent with the principal test conducted using the number of events, while for Yemen the regression coefficient is nearly zero for all sub-categories.
3.3. Sensitivity analysis
We run a sensitivity analysis to test the robustness of the results. The goal of the test is to explore whether the higher relationship between the food-related conflict category and food insecurity is robust across both short and medium-term periods. To achieve this aim, we consider different time windows to construct the conflict-related variables. In other words, we replace the number of events that occurred in the preceding 90 days of each registered value of insufficient food consumption with shorter and longer windows. Aware that by comparing an increasing number of events the regression coefficients decrease merely because a longer time window is considered and much more events are captured, we focus on catching the relative comparison of the conflict-related variables across different ranges of data collection windows. The test holds consistent results for all the countries: over longer or shorter preceding periods, the food-related conflict class shows the strongest relationship with the outcome compared to the most-conventional conflict measures. Figure 5 shows the results. Also, we test and verify whether the models remain stable across different time windows, by including how the R-squared changes (detailed visualizations are available in the Supplementary material).
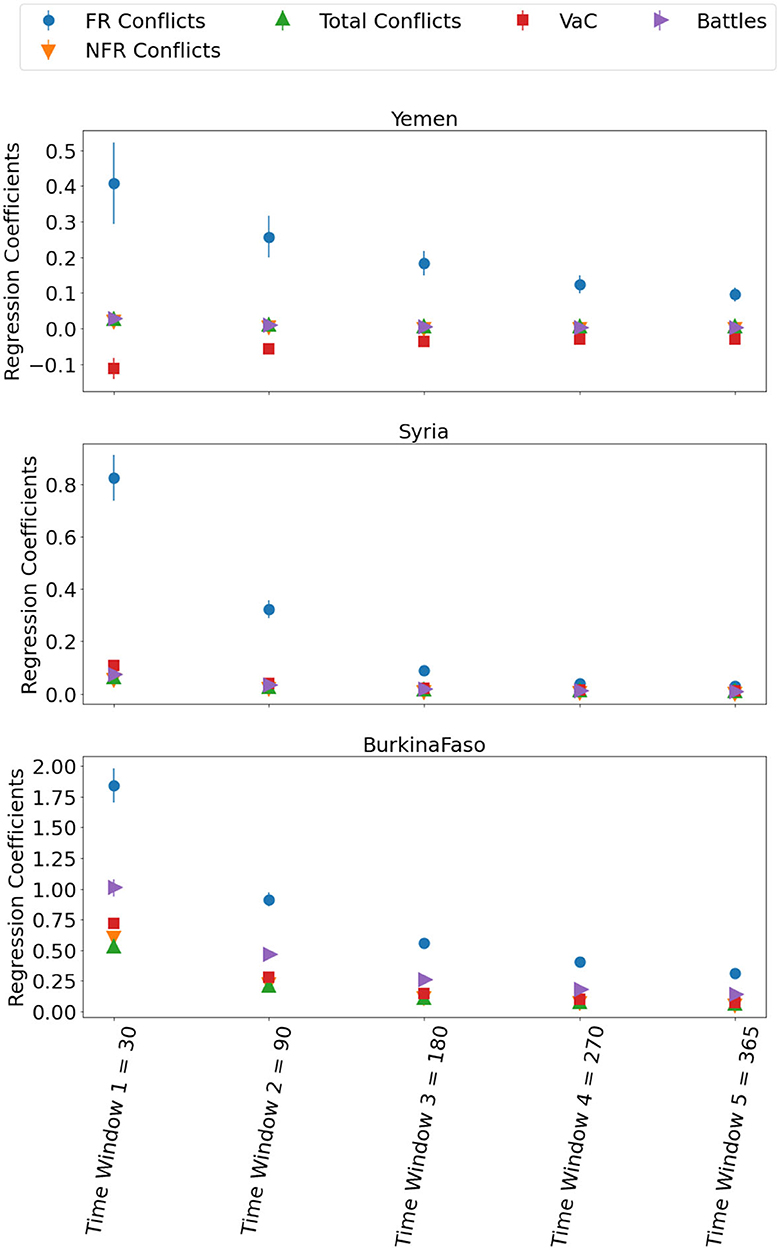
Figure 5. Sensitivity analysis. Each plot represents the results for a single country. More in detail, each plot shows the comparison among regression coefficients of different forms of conflict by considering different time windows to construct the conflict-related variables. In all three countries we can observe that over longer or shorter preceding periods, the food-related conflict class shows the strongest relationship with self-reported food insecurity.
3.4. Regression models through time
We run an additional analysis, focusing on the time dimension. The aim is to explore whether in different time windows and therefore phases of insufficient food consumption we have different coefficients (and/or different significance of regression coefficients) for some conflict variables than for others. To do this, we take 6-month-long time windows over which we run the panel regression with the different conflict variables we have built. By running the separately several regressions model over time we test the robustness of our analysis since we found that the regression coefficient related to food-related conflict, in general, has a strong relationship with the target also by considering different subsets of observations. Also, by focusing on narrower time windows we detect periods in which the relationship between food-related conflict and the percentage of people with insufficient food consumption particularly increases. We perform the analysis separately for the three countries, and since we have available different time periods for each of them, we consider 6-month time windows that start and end differently. Figure 6 reports the results separately per each country. The x-axis refers to the starting dates of the 6-month time windows, while the y-axis to the values of regression coefficients, and has a different range for each country. To discuss some of these points of interest, the second half of 2018 in Yemen points out an outsized impact of food-related conflict on the subsequent food crises, as well as the first half of 2020 in Syria and from July 2019 to July 2020 in Burkina Faso. Through this analysis we can also visualize periods in which the conflict-related variable is not significant in the model, suggesting periods in which probably other drivers play a higher role in the level of food insecurity.
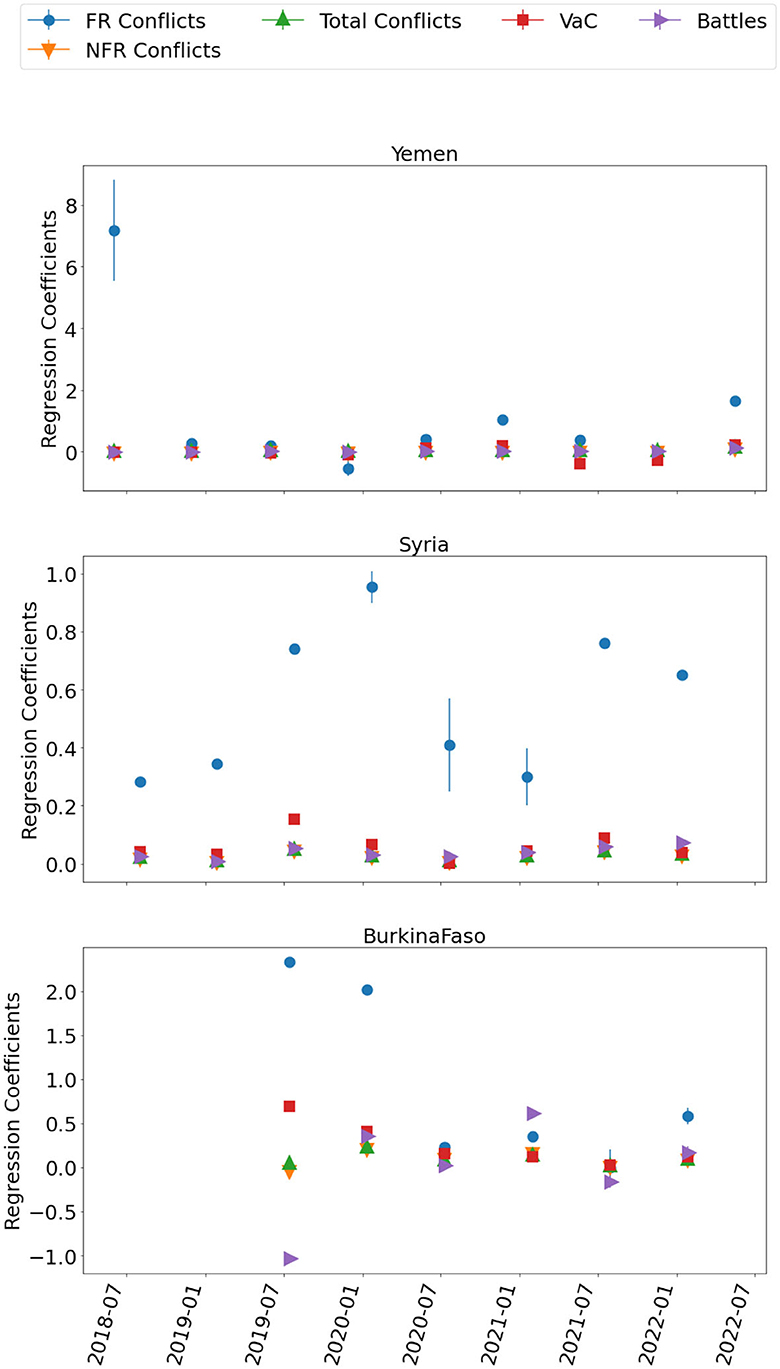
Figure 6. Regression model applied on multiple time windows. Each plot represents the results for a single country. More in detail, each plot shows the comparison among the regression coefficients for the conflict-related measures for each 6-month time window. For most of the time windows, in all three countries, we can generally observe a higher regression coefficient for food-related conflict than the other forms of conflict.
4. Discussion
Conflict's impacts on food insecurity are complex and multifaceted, not least because of the many forms and dynamics of violence that take place within any given conflict. This research set out to comparatively assess the impact of different types of conflict on self-reported food insecurity in three prominent food crisis contexts: Burkina Faso, Syria, and Yemen. The resulting analysis found that a specific form of conflict—food-related conflict—is more strongly correlated to subsequent self-reported insufficient food consumption than other forms of conflict. Specifically, the coefficient for food-related conflict is between four and 20 times larger than the coefficient for non-food-related conflict, meaning this sub-type of conflict has an outsized relationship to subsequent food insecurity.
The findings build on and advance previous work on this topic (Dowd, 2022) in several ways. First, the analysis more rigorously tests temporal variations and finds the results robust to several alternative specifications. In sensitivity analyses, we alter the time window of recorded violence preceding the collection of food security data. In doing so, we find that while the precise time window with the highest coefficient varies by country, the overall pattern holds: food-related conflict remains consistently more strongly correlated to subsequent self-reported food insecurity, than other forms of conflict. Thereafter, we run the regression on distinct 6-month time windows in each country and the results remain almost entirely unchanged: with the exception of a small number of time periods in Yemen, the coefficient for food-related conflict remains the most strongly correlated with subsequent food insecurity.
Together, these results offer further support for the finding that food-related conflict is a significant driver of food insecurity and crisis. They demonstrate that this relationship is robust across a range of data collection windows and across discrete time periods of analysis. This is particularly significant in that both violent conflict, and food insecurity, are dynamic and can vary significantly over time in any given context. In other words, the results demonstrate that the relationship between food-related conflict and subsequent food insecurity is unlikely to be driven by any particular, isolated window of time in which either (or both) variables are particularly elevated (or depressed). That the observed relationship between food-related conflict and subsequent food insecurity persists across these variations attests to the enduring importance of this form of violence in driving food insecurity.
The research also advances previous analysis by substituting an expert-derived estimate of the food crisis for a self-reported measure of insufficient food consumption. This is important for a number of reasons. While expert-derived estimates of food crises remain the most widely used figures for assessing food security needs, recent food security crises have shone a spotlight on challenges in the consensus-based process leading to the unavailability of figures (Buchanan-Smith and Sharp, 2021). Furthermore, as food crisis estimates are regularly leveraged to mobilize policy and financial resources in humanitarian response, estimates may be susceptible to potential biases and politicization (Maxwell and Hailey, 2020). Lastly, food crisis data are most widely collected and available for contexts in which food insecurity is already elevated. This inhibits the ability to understand the relationship between conflict and food insecurity at lower levels of both phenomena. This may skew analysis (and subsequent response) toward higher-insecurity contexts, when early warning and early action at lower levels of insecurity may be preferable. While this research has focused on high-insecurity contexts in its analysis, in leveraging self-reported food insecurity data, it demonstrates the potential for monitoring, analyzing, and responding to signals in this relationship at lower levels of both violent conflict and food insecurity.
In addition, the research tests the relationship between food-related conflict and subsequent food insecurity in a wider range of geographic contexts than in past work. In expanding analysis beyond sub-Saharan Africa to both Yemen and Syria, the research makes two key contributions.
The first, is methodological, in that the analysis demonstrates that the rules-based approach to coding and classifying food-related violence can be applied in diverse contexts. The search string can be revised and refined to capture food-related violence in diverse food systems. This points to its potential generalizability as a method for capturing and coding food-related violence in diverse food crises and indeed, globally. Scholars may find this valuable in analyzing and comparing patterns of food-related conflict in diverse conflict and food system contexts, while policymakers and response agencies concerned with security and food crises in a large number of regions may require an approach to identifying food-related violence that is widely applicable.
However, it is important to acknowledge that the rule-based method we employ does have certain limitations. As noted above, the underlying source for event data is not specifically designed for the purpose of capturing and monitoring food-related conflict, and as such, may not capture a comprehensive account of all food-related violence that has taken place in a given conflict. In the absence of a dedicated data collection effort specifically concerned with food-related conflict, we maintain that the ACLED dataset presents the most suitable and valuable source for analysis of this important phenomenon, but advise readers that the results may under-report the true extent of food-related conflict. Beyond this, our approach does not distinguish or weigh events based on their duration, and it does not consider the impact of other forms of violence related to food in the vicinity of administrative areas. The introduction of these additional layers of complexity to the process could potentially enhance our capacity to identify and classify this subclass of events, ultimately refining the precision of monitoring systems.
The second contribution is analytical, in that in analyzing a wider range of contexts, the research finds support for the observed relationship between food-related conflict and food insecurity beyond a small number of countries alone. In other words, the research lends support for the significance of this relationship more widely, in contrast to food-related conflict being a function of particular contexts, food systems, or conflict.
Empirically, it is important to note that food-related conflict as a sub-type of conflict is relatively rare in our dataset, constituting ~10% of all violence recorded in our three country cases. However, it is likely that the methodology for coding this form of conflict underestimates the true universe of violence involving and targeting food and food sources (see Dowd, 2022 for further discussion on this). Moreover, even if relatively rare, each instance of this specific modality of violence is correlated to a greater increase in the share of the population subsequently reporting insufficient food consumption than other forms of conflict. Consequently, although broader categories of violence such as battles or violence against civilians are more frequent, food-related conflict has an outsized relationship with food insecurity.
Taken together, the findings suggest that greater scholarly and policy attention to contexts in which these tactics—specifically, the targeting and leveraging of food and food systems in conflict—are employed, could prove particularly effective in understanding and responding to food insecurity. The conflict-hunger nexus has been the focus of extensive research (Shemyakina, 2022), and specific tactics such as the use of food as a weapon of war have been highlighted in recent policy, humanitarian response, and legal discourses (Global Right Compliance, 2019; Beltrami, 2020; Celis, 2022). However, to date, most data-driven analysis of conflict-driven hunger has neglected to study specific attacks on food and food systems. In leveraging an existing, large-scale, and open-access dataset to identify and analyze this phenomenon, we highlight the potential for data science to play an important role in tackling this global challenge.
The study highlights the impacts of food-related conflict on food security and underscores the need for collaborative efforts to address the underlying causes and effects. The available disaggregated data provides a unique opportunity to unpack the temporal and spatial footprints of these overlapping challenges. The presence of data at the local level empowers us to conduct extensive analyses by integrating information from different sources. This data availability assumes a crucial role in supporting the need for multi-sectoral approaches to address the pressing challenges posed by these crises. Data availability could be also enhanced using the latest developments in large language models (LLMs), which can be used to detect food-related violence events in textual reports with a greater flexibility and nuance than the rule-based approach used in this study. These can be utilized by early warning mechanisms for food-insecurity such as the HungerMap LIVE (WFP, 2020), which have advanced over the past decades toward a better model for managing food crises, by leveraging the power of big data to track and predict food-insecurity in near real-time (Kemmerling et al., 2023). The in-depth analysis conducted here focused on specific conflict subtypes could be taken into account to enhance the evaluation of risks associated with food insecurity. The results could be integrated into an early-warning system that utilizes a machine learning model. This system would focus on the specific subclass of food-related conflict as the primary driver of food insecurity, rather than encompassing all types of conflict events without prior selection. This, in turn, could enhance existing systems for predicting food insecurity and facilitate more proactive humanitarian efforts in fragile and conflict-affected areas.
Data availability statement
The data analyzed in this study is subject to the following licenses/restrictions: We have been granted an unlimited access key to download the ACLED dataset, which typically provides data for only the past 3 years from the date of download. Consequently, we have shared the final aggregated CSV file that encompasses the available data (at link https://doi.org/10.5281/zenodo.7995105). Requests to access these datasets should be directed to access@acleddata.com.
Author contributions
CD, GM, YM, EO, DP, and MT contributed to the interpretation of the results. SF and CD took the lead in writing the manuscript. All authors conceived and planned the experiments. SF carried out the experiments and the data analysis. All authors provided critical feedback and helped shape the research, analysis, and manuscript. All authors contributed to the article and approved the submitted version.
Funding
The authors acknowledge support from the Lagrange Project of the Institute for Scientific Interchange Foundation (ISI Foundation) funded by Fondazione Cassa di Risparmio di Torino (Fondazione CRT). CD's work was supported in part by an award from Dublin City University's Research Initiative Fund.
Acknowledgments
We are very grateful to Ruth Santini and Rossano Schifanella for fruitful discussions and collaborations. We would like to express our gratitude to the World Food Programme (WFP) for sharing the data.
Conflict of interest
The authors declare that the research was conducted in the absence of any commercial or financial relationships that could be construed as a potential conflict of interest.
Publisher's note
All claims expressed in this article are solely those of the authors and do not necessarily represent those of their affiliated organizations, or those of the publisher, the editors and the reviewers. Any product that may be evaluated in this article, or claim that may be made by its manufacturer, is not guaranteed or endorsed by the publisher.
Supplementary material
The Supplementary Material for this article can be found online at: https://www.frontiersin.org/articles/10.3389/fsufs.2023.1239992/full#supplementary-material
References
ACAPS Food Agriculture Organization of the United Nations International Organization for Migration, UN Development Programme. (2023). Food Affordability in Conflict-Torn Yemen in Light of the Ukraine War 2023. Available online at: https://reliefweb.int/report/yemen/food-affordability-conflict-torn-yemen-light-ukraine-war-2023
Africa Portal (2021). Food Insecurity in the Horn of Africa and Its Impact on Peace in the Region. Available online at: https://www.africaportal.org/publications/food-insecurity-horn-africa-and-its-impact-peace-region/
Beltrami, S. (2020). No End to World Hunger Without an End to Conflict-WFP Warns. World Food Programme. Available online at: https://www.wfp.org/stories/no-end-world-hunger-without-end-conflict-wfp-warns
Buchanan-Smith Margie, C. J., and Sharp, S. (2021). Independent Review of the IPC South Sudan. ODI Humanitarian Policy Group. Available online at: https://cdn.odi.org/media/documents/ODI_HPG_IPC_South_Sudan_report_final_BXOKlof.pdf
Cederman, L.-E., and Gleditsch, K. S. (2009). Introduction to special issue on “disaggregating civil war”. J. Conflict Resol. 53, 487–495. doi: 10.1177/0022002709336454
Celis, B. (2022). The Day the UN Barred Using Hunger and Starvation as Weapons of War. World Food Programme. Available online at: https://www.wfp.org/stories/un-barred-using-hunger-and-starvation-weapons-war
Cichocki, A., Zdunek, R., and Amari, S.-i. (2006). “New algorithms for non-negative matrix factorization in applications to blind source separation,” in 2006 IEEE International Conference on Acoustics Speech and Signal Processing Proceedings, Vol. 5(Toulouse).
Dowd, C. (2022). Food-related violence, hunger and humanitarian crises. J. Peace Res. doi: 10.1177/00223433221099309
FAO (1996). Rome Declaration on World Food Security. Available online at: https://www.fao.org/3/w3613e/w3613e00.htm
FAO (2015). Hunger and Food Insecurity. Available online at: https://www.fao.org/hunger/en/
FSIN (2022). Global Report on Food Crisis. Available online at: https://www.fsinplatform.org/sites/default/files/resources/files/GRFC%202022%20Final%20Report.pdf
Funk, C. C., Peterson, P. J., Landsfeld, M. F., Pedreros, D. H., Verdin, J. P., Rowland, J. D., et al. (2014). A Quasi-Global Precipitation Time Series for Drought Monitoring. Technical report, U.S. Geological Survey, Reston, VA. doi: 10.3133/ds832
Global Right Compliance (2019). The Crime of Starvation and Methods of Prosecution and Accountability. Global Rights Compliance and World Peace Foundation. Available online at: https://reliefweb.int/report/world/crime-starvation-and-methods-prosecution-and-accountability
Insecurity Insights (2023). The Links Between Conflict and Hunger in Syria. Available online at: https://insecurityinsight.org/wp-content/uploads/2023/04/Syria-Conflict-and-Hunger-March-2023.pdf
Internal Displacement Monitoring Centre (2023a). Burkina Faso: People Caught in a Perfect Storm of Conflict, Displacement and Food Insecurity. Available online at: https://story.internal-displacement.org/burkina-faso-People-caught-in-a-perfect-storm-of-conflict-displacement-and-food-insecurity/
Internal Displacement Monitoring Centre (2023b). Surviving in Crisis: Unpacking the Link Between Conflict, Displacement and Food Security in Burkina Faso. Available online at: https://reliefweb.int/report/burkina-faso/surviving-crisis-unpacking-link-between-conflict-displacement-and-food-security-burkina-faso
IPC (2019). Technical Manual Version 3.0 Evidence and Standards for Better Food Security and Nutrition Decisions. Available online at: https://www.ipcinfo.org/fileadmin/user_upload/ipcinfo/docs/IPC_Technical_Manual_3_Final.pdf
Justino, P., Brück, T., and Verwimp, P. (2013). A Micro-Level Perspective on the Dynamics of Conflict, Violence, and Development. Oxford: Oxford University Press.
Kalimeri, K., Delfino, M., Cattuto, C., Perrotta, D., Colizza, V., Guerrisi, C., et al. (2019). Unsupervised extraction of epidemic syndromes from participatory influenza surveillance self-reported symptoms. PLoS Comput. Biol. 15:e1006173. doi: 10.1371/journal.pcbi.1006173
Kemmerling, B., Schetter, C., and Wirkus, L. (2023). Addressing Food Crises in Violent Conflicts. Cham: Springer International Publishing. doi: 10.1007/978-3-031-15703-5_12
Martini, G., Bracci, A., Jaiswal, S., Corea, M., Riches, L., Rivers, J., et al. (2021). Nowcasting food insecurity on a global scale. medRxiv doi: 10.1101/2021.06.23.21259419
Maxwell, D., and Hailey, P. (2020). The Politics of Information and Analysis in Famines and Extreme Emergencies: Synthesis of Findings From Six Case Studies. Feinstein International Center.
MODIS Platform (2000). Modis Vegetation Index Products (NDVI and EVI). Available online at: https://modis.gsfc.nasa.gov/data/dataprod/mod13.php
Norwegian Refugee Council (2020). Yemen: 900 Airstrikes and Shells Hit Farms in Less Than Three Years. Available online at: https://reliefweb.int/report/yemen/yemen-900-airstrikes-and-shells-hit-farms-less-three-years
OCHA (2022). About OCHA Syria. Available online at: https://www.unocha.org/syrian-arab-republic/about-ocha-syria
Raleigh, C., Linke, A., Hegre, H., and Karlsen, J. (2010). Introducing ACLED: an armed conflict location and event dataset. J. Peace Res. 47, 651–660. doi: 10.1177/0022343310378914
Shemyakina, O. (2022). War, conflict, and food insecurity. Annu. Rev. Resour. Econ. 14, 313–332. doi: 10.1146/annurev-resource-111920-021918
Sleet, P. (2020). Food Security Declines in East Africa Following Economic, Climate and Conflict Shocks. Research Analyst. Global Food and Water Crises Research Programme.
Sneyd, L. Q., Legwegoh, A., and Fraser, E. D. G. (2013). Food riots: media perspectives on the causes of food protest in Africa. Food Secur. 5, 485–497. doi: 10.1007/s12571-013-0272-x
The Economist (2023). The Global Food Crisis. Available online at: https://impact.economist.com/sustainability/social-sustainability/topic-1-or-the-global-food-crisis-teef
The World Bank (2023). Breaking the Cycle of Food Crises in Yemen. Available online at: https://www.worldbank.org/en/news/feature/2023/04/27/breaking-the-cycle-of-food-crises-in-yemen
United Nations (2022). Goal 16: Promote Just, Peaceful and Inclusive Societies. Available online at: https://www.un.org/sustainabledevelopment/peace-justice/
USAID (2019). Food Assistance Fact Sheet-Yemen. Available online at: https://www.usaid.gov/food-assistance/yemen
WFP (2008a). Food Consumption Analysis Calculation and Use of the Food Consumption Score in Food Security Analysis. Available online at: https://documents.wfp.org/stellent/groups/public/documents/manual_guide_proced/wfp197216.pdf
WFP (2008b). Food Consumption Analysis. Calculation and Use of the Food Consumption Score in Food Security Analysis. Available online at: https://documents.wfp.org/stellent/groups/public/documents/manual_guide_proced/wfp197216.pdf
WFP (2014). Calculation and Use of the Alert for Price Spikes (ALPS) Indicator, Technical Guidance Note, Analysis and Nutrition Service. Available online at: https://documents.wfp.org/stellent/groups/public/documents/manual_guide_proced/wfp264186.pdf
WFP (2020). Hungermap Live. Available online at: https://innovation.wfp.org/project/hungermap-live
WFP (2022a). WFP Economic Explorer. Available online at: https://dataviz.vam.wfp.org/economic_explorer/macro-economics/exchange_rate
WFP (2022b). WFP Seasonal Explorer. Available online at: https://dataviz.vam.wfp.org/seasonal_explorer/rainfall_vegetation/visualizations
WFP FAO (2023). Hunger Hotspots. FAO-WFP Early Warnings on Acute Food Insecurity, June 2023 to November 2023 Outlook. Available online at: https://docs.wfp.org/api/documents/WFP-0000149627/download/
World Food Programme USA (2023). Burkina Faso Hunger From Conflict and Climate. Available online at: https://www.wfpusa.org/countries/burkina-faso/
Keywords: data science, social impact, sustainable development goals, food insecurity, conflict, food-related conflict
Citation: Fiandrino S, Dowd C, Martini G, Mejova Y, Omodei E, Paolotti D and Tizzani M (2023) Impact of food-related conflicts on self-reported food insecurity. Front. Sustain. Food Syst. 7:1239992. doi: 10.3389/fsufs.2023.1239992
Received: 15 June 2023; Accepted: 26 September 2023;
Published: 12 October 2023.
Edited by:
Antonio Santoro, University of Florence, ItalyReviewed by:
Roberto Interdonato, UMR9000 Territoires, Environnement, Télédétection et Information Spatiale (TETIS), FranceHendra Manurung, Universitas Prof. Dr. Moestopo, Indonesia
Copyright © 2023 Fiandrino, Dowd, Martini, Mejova, Omodei, Paolotti and Tizzani. This is an open-access article distributed under the terms of the Creative Commons Attribution License (CC BY). The use, distribution or reproduction in other forums is permitted, provided the original author(s) and the copyright owner(s) are credited and that the original publication in this journal is cited, in accordance with accepted academic practice. No use, distribution or reproduction is permitted which does not comply with these terms.
*Correspondence: Stefania Fiandrino, stefania.fiandrino@isi.it
†ORCID: Stefania Fiandrino orcid.org/0000-0002-4513-3988