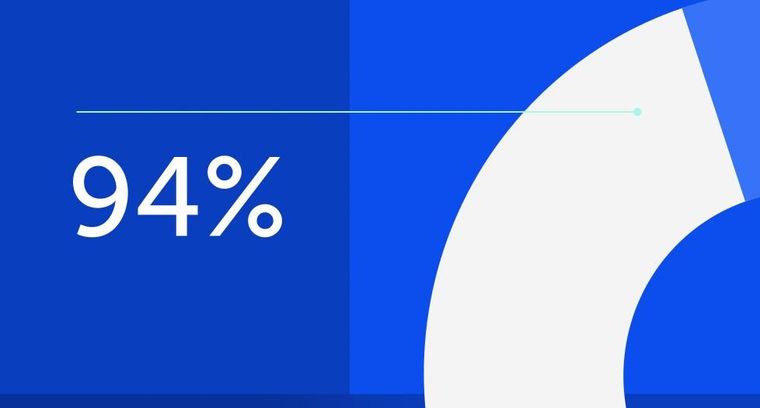
94% of researchers rate our articles as excellent or good
Learn more about the work of our research integrity team to safeguard the quality of each article we publish.
Find out more
ORIGINAL RESEARCH article
Front. Sustain. Food Syst., 26 September 2023
Sec. Sustainable Food Processing
Volume 7 - 2023 | https://doi.org/10.3389/fsufs.2023.1226583
Introduction: The implementation of artificial intelligence (AI) in postharvest agriculture has significantly improved in recent decades, thanks to extensive scientific research. The study aimed to identify research gaps and hotspots for future research based on keyword co-occurrence and clustering analyses, as well as to discuss the results and highlight the research trends.
Methods: This study analyses research trends in AI application in postharvest agriculture using novel scientometric tools such as the Bibliometrix R package, biblioshiny, and VosViewer. The research analysed 586 published papers on AI application in postharvest agriculture research between 1994 and September 2022, retrieved from the Scopus database.
Results and discussion: The results showed that publications on AI applications in postharvest agriculture research have been increasing for almost 30 years, with significant growth in the subject area in the last decade. China, the USA, and India were found to be the top three most productive countries, accounting for 52.4%, 22%, and 18.6% of the total selected publications, respectively. The analysis also revealed that topics such as the Internet of Things, cold chain logistics, big data, decision-making, and real-time monitoring have low development degrees in the knowledge domain. This study demonstrated increased research on AI applications in postharvest agriculture, aiming to reduce postharvest losses, enhance food nutrition and quality, and mitigate food insecurity. It also provides valuable scientific references on AI applications in postharvest agriculture research for researchers and scholars. By identifying research gaps and hotspots, this study can guide future research in AI applications in postharvest agriculture to further improve the industry.
Despite various efforts to combat global hunger, food insecurity, and the ability to provide adequate quality, quantity, and safe food for the world’s growing population remain enormous challenges in many countries (Pawlak and Kołodziejczak, 2020; Fadiji et al., 2021). With the world’s population projected to exceed 9 billion by 2050, food demand is expected to increase by nearly 100% (Fukase and Martin, 2020; Pawlak and Kołodziejczak, 2020). Consequently, to meet future demands, food production and agricultural productivity will have to rise by about 70%, which could double to meet the growing future demand (Cole et al., 2018; Sharma et al., 2020). Hence, an integrated and innovative approach to the global effort to ensure sustainable food production and consumption is required. The agricultural sector is critically important in alleviating food insecurity, improving nutrition, and reducing postharvest losses (Emami et al., 2018).
The postharvest stage is the final and most critical in agriculture and requires close attention because time and money have been used to cultivate food products. An ineffective postharvest stage or negligence may result in severe postharvest losses and consequent financial loss (Prusky, 2011). Postharvest loss includes food loss across the food supply chain from harvesting food crops until consumption (regarded as food waste at the consumption level; Kumar and Kalita, 2017), broadly categorized as weight loss due to spoilage, quality loss, nutritional loss, seed viability loss, and commercial loss (Kumar and Kalita, 2017). In severe cases, postharvest losses have been estimated to be up to 80% of the total production; in Africa, postharvest losses could be as high as 40%, which is significant given the low agricultural productivity in the region (Abass et al., 2014; Naziri et al., 2014; Kumar and Kalita, 2017; Minten et al., 2021). These losses can potentially reduce the economic value of food crops or make them unsuitable for human consumption, consequently impairing food security and nutrition (Prusky, 2011; Saima et al., 2014; Hailu and Derbew, 2015; Kumar and Kalita, 2017; Singh et al., 2022). A promising solution mentioned challenges is incorporating newer technology that increases food production while decreasing postharvest losses is critical to maintaining sustainable living standards and improving food security (Singh et al., 2022).
In postharvest agriculture, artificial intelligence (AI) has set an impeccable record (Kakani et al., 2020; Meshram et al., 2021). AI is a general term that includes machine learning (ML), deep learning (DL), and neural networks (NN). Machine learning is an approach to achieving artificial intelligence, while deep learning is a subfield of machine learning that includes convolutional and recurrent neural networks (Dokic et al., 2020). Given its outstanding performance combined with increased application in different sectors, AI has the potential to complement existing approaches and techniques to minimize the massive postharvest losses problem, and postharvest agriculture is one area ripe for disruption with AI implementation. In agriculture, postharvest handling is the stage of crop production immediately following harvest, including cooling, cleaning, sorting, and packing to help reduce the fast deterioration of crops (El-Ramady et al., 2015). In particular, the quality of horticultural products is critical in determining market acceptance and, as a result, directly affecting the storage and postharvest processing operations. There are pieces of evidence on the use of these techniques (AI, ML, and DL) on various agricultural products, especially in detecting defects, classification, sorting or grading, autonomous decision-making, predictive analytics, quality control, etc. For instance, Yang et al. (2022) developed an image recognition system based on AI to sort apple fruit efficiently. Takruri et al. (2020) employed machine learning algorithms to estimate the freshness and quality of apples in terms of age using polarization images. Amoriello et al. (2022) applied artificial neural networks (ANN) to predict the quality parameters of strawberry fruit. In another study, Makkar et al. (2017) analyzed the quality of fruits and vegetables based on color, shape, and size and successfully segmented defective fruit regions using neural networks.
Further, researchers have demonstrated the excellent performance of artificial intelligence in agricultural computer vision applications. A deep neural network binary classifier to detect defects in tomatoes was proposed by da Costa et al. (2020). In another study, Thinh et al. (2019) classified mango fruit in terms of color, volume, size, shape, and fruit density. The study combined computer vision, image processing, artificial intelligence, and artificial neural networks. Using computer vision and artificial intelligence, Chakraborty et al. (2021) proposed a model to prevent the propagation of rottenness in apple, banana, and orange fruits. The model was capable of classifying fresh and rotting fruits. Another study by Roy et al. (2021) performed a real-time segmentation of rotten apples, which led to the classification of fresh apples from rotten apples by utilizing deep learning architecture. Despite the extensive use of artificial intelligence in classification and defect identification, its application has cut across real-time monitoring of fruit quality, food fraud and authentication, and cold chain logistics (Tsang et al., 2018; Loisel et al., 2021).
It is evident, therefore, that research on the application of artificial intelligence in postharvest agriculture has grown significantly in the last decade. There are several excellent recent reviews on the application of artificial intelligence in agriculture, including machine learning applications to monitor food safety (Wang et al., 2021), digitalization and artificial intelligence for sustainable food systems (Marvin et al., 2022), improving food quality using artificial intelligence (Ben Ayed and Hanana, 2021; Sahni et al., 2021), Internet of Things, big data, and artificial intelligence in agriculture and food industry (Kamilaris and Prenafeta-Boldú, 2018; Liakos et al., 2018; Santos et al., 2019; Dokic et al., 2020; Misra et al., 2020; Ren et al., 2020; Bal and Kayaalp, 2021; Meshram et al., 2021), deep learning approaches in horticulture (Yang and Xu, 2021), fruit detection, recognition, and yield estimation (Koirala et al., 2019; Indira et al., 2021), food processing applications (Zhu et al., 2021), to mention a few. These reviews provided good insights into the applications, opportunities, and challenges of artificial intelligence in agriculture, but none evaluated a quantitative structured methodology such as bibliometrics in postharvest agriculture. Bibliometrics employs mathematical and statistical methods to assess a specific knowledge domain’s current state and future direction. Therefore, this study aimed to utilize bibliometric analysis to provide a comprehensive insight into artificial intelligence in postharvest agriculture research.
Bibliometrics has evolved into an independent discipline over the years. This study uses mathematics, statistics, and logic to organize and analyze aspects of literary works (Rons, 2018; Wang et al., 2021). In addition, critical decisions regarding expert and specialized issues are made using bibliometric analysis or methods, as it allows for monitoring scientific developments using various indicators, such as influential authors, journals, countries, academic affiliations, and research collaborations (Rons, 2018). Consequently, providing essential data for researchers to investigate current and future research trends (Benavides-Velasco et al., 2013; Albort-Morant and Ribeiro-Soriano, 2016; Rey-Martí et al., 2016; Rons, 2018; Cucino et al., 2021; de Castro et al., 2021). Ultimately, the bibliometric method ensures that research is presented transparently, objectively, and methodically (Donthu et al., 2021; Rejeb et al., 2021).
The present research data were collected and saved from the Scopus database, particularly on the 22nd of September 2022 at about 5:40 p.m., spanning 28 years from 1994 to 2022. Scopus is a world-renowned repository known for its comprehensive coverage and dependable content. It contains several publications published in journals from major and reputable publishers such as Elsevier, EmeraldInsight, Springer, Multidisciplinary Digital Publishing Institute (MDPI), and Taylor & Francis (Maflahi and Thelwall, 2016; Rejeb et al., 2021). The following search string with the “OR” and “AND” operators were used: [(“food quality” OR “food grad*” OR “food trac*” OR “food loss” OR “food waste” OR “food deteriorat*” OR “food discriminat*” OR postharvest OR post-harvest OR “cold chain” OR cold-chain OR coldchain OR “cold supply chain” OR agroprocessing OR agro-processing) AND (“machine learning” OR “artificial intelligence” OR “data mining” OR “data science” OR “deep learning” OR “Big data” OR “Real-time monitoring” OR “Transfer learning”)]. The search was performed in the title, abstract, and keyword fields. The titles and abstracts of these articles were screened, excluding all publications with missing bibliometric data (e.g., abstracts, keywords) and according to the subject area. We selected all languages to give an idea of the language distribution of published documents, resulting in 586 documents. The article selection process ensured that the chosen articles aligned with our research scope. This was achieved through manual exclusion, resulting in a focused and accurate representation of the literature that closely aligns with the search string used.
Figures 1A,B depict the proportion of documents by language and type, respectively. Among these publications, according to language category, English accounted for 95.90%, followed by Chinese (3.07%), Spanish (0.51%), French (0.17%), German (0.17%), and Japanese (0.17%; Figure 1A).
Figure 1. (A) The proportion of document distribution by language and (B) the proportion of document distribution by type.
The percentage proportions of the documents according to types are as follows: articles (57.68%), conference papers (30.20%), review (8.36%), book chapters (2.73%), editorial (0.34%), note (0.34%), book (0.17%), and letter (0.17%; Figure 1B). Figure 2 depicts a summary of the main information of the analyzed data.
Supplementary Table S1 shows the distribution of the patents obtained using the exact search string.
The raw data from the Scopus search of 586 documents were saved in BibTeX and CSV formats for further analysis. The data were analyzed using bibliometric software, specifically the VosViewer and the “Bibliometrix” package software (Van Eck and Waltman, 2010; Aria and Cuccurullo, 2017; Sganzerla et al., 2021). The authors conducted a keyword co-occurrence network analysis to understand the current topic thoroughly. The VosViewer software generated maps based on the main keywords, authors, and their relationships. Furthermore, the Bibliometrix was used to illustrate the documents’ scientific trends and productivity, including the most productive authors and most significant articles published.
Figure 3 shows the annual distribution of the total (586) scientific documents depicting a growing trend since 1994, with a yearly growth rate of 17.79%. The figure is divided into two parts: the initial period and the rapid-growth period. In the initial period (1994–2009), the literature appears limited, where the maximum annual publication did not exceed two documents. Conversely, the rapid-growth period (2009–2022) showed an exponential increase in the yearly publication on artificial intelligence in postharvest agriculture, with 178 documents observed in 2021. At the time of this study, in 2022, the number of published papers was 54% of those published in 2021. This observation indicates rapid and significant growth in the subject area. Figure 4 illustrates the annual average of document citations. It was discovered that 2008 had the highest average number of document citations. This finding corroborates those made for 2009, the start of the rapid-growth era, in the publications by year depicted in Figure 3.
Figure 3. Yearly distribution of scientific publications on AI applications in postharvest agriculture research.
Figure 4. Yearly average citations of scientific publications on AI applications in postharvest agriculture research.
Sixty-nine countries (or regions) were represented in the data on artificial intelligence in postharvest agriculture research from 1994 to 2022. Figures 5A,B show the publication distribution according to country. Figure 5A depicts the distribution of scientific production in all 69 countries, with the deepest blue color indicating the country with the highest number of publications. As can be seen, a high concentration of publications occurs mainly in developed countries. Figure 5B shows the occurrence of the number of publications for the top 20 countries. Among these countries, China has the highest number of publications with 307 documents, which was followed by the USA (129), India (109), Spain (88), Brazil (65), UK (58), Italy (51), Iran (47), South Korea (41), and Australia (35). Similar observations were reported by Dokic et al. (2020), showing China as a dominating country in production in their study on applying machine learning, neural networks, and deep learning in agriculture. The composition of the top countries shows a mix of developing and developed countries publishing in the research area.
Figure 5. The distribution of the scientific publications on AI applications in postharvest agriculture research by country. (A) World map distribution on the scientific publications, with the deepest blue colour representing the higher number of publications; (B) Bar chart showing the top 20 scientific publications by country.
From the perspective of citations according to countries, the top 20 countries are shown in Figure 6. It was observed that China (1,040), Spain (877), USA (357), Iran (253), and South Korea (252) were the top five most cited countries. For both countries’ scientific production and most cited countries, China appeared in the leading position, indicating that China is the fastest-growing country in AI applications in postharvest agriculture research. Furthermore, results revealed that articles published in China were of high quantity.
Figure 6. The most cited countries of scientific publications on AI applications in postharvest agriculture research.
Figure 7 shows the top 20 countries in terms of publications based on the corresponding author. SCP represents the intra-country publication, while MCP represents the inter-county publication. Generally, for all the top 20 countries, when the nationality of the corresponding author was considered, SCP was higher than MCP. Additionally, China topped for combined SCP and MCP publications (102), followed by USA (32), India (29), Spain (29), and Brazil (21). The ratio of SCP to total publications in the top 20 countries was ~20%–90%, while MCP to total publications was ~10%–80% (Table 1). From Table 1, Brazil and Ireland have the highest percentage SCP and MCP ratios to total publications of 90% and 80%, respectively. This observation indicated that Ireland strongly preferred international cooperation or collaboration concerning research in AI applications in postharvest agriculture. Furthermore, some countries have similar % SCP and MCP ratios to total publications. For instance, the % SCP and MCP ratios to total publications for India and South were 76% and 24%; Italy, Japan, and Malaysia (56% and 44%), Hong Kong and Turkey (67% and 33%); Germany and Netherlands (60% and 40%), respectively. Interestingly, Greece has the same % SCP and MCP ratios to total publications of 50%. Notably, most countries showed more tendencies for intra-country collaborations than inter-country collaborations regarding research in AI applications in postharvest agriculture.
Figure 7. Corresponding author’s countries. SCP and MCP represent intra-country and inter-country collaborations or country publications and multiple-country publications, respectively.
Figure 8 illustrates the top 20 academic institutions contributing the most to AI applications in postharvest agriculture research. Zhejiang University, located in China, had the highest number of publications, with 19 documents, followed by China Agricultural University, with 18 publications, and the University of Kentucky (USA), with 14 publications. These results corroborated the observations on the scientific publications by country shown in Figure 5, where China was the top country regarding scientific publications, followed by the USA. Additionally, out of the top 20 academic affiliations, 20 and 15% of the institutions were from China and USA, respectively.
Figure 8. The most relevant affiliations of scientific publications on AI applications in postharvest agriculture research.
The top 10 relevant sources (journals) publishing articles on AI applications in postharvest agriculture are shown in Figure 9. Generally, it was observed that the top 10 journals published 110 documents, accounting for approximately 19% of the total sampled 586 papers. From Figure 9, Computers and Electronics in Agriculture rank first on the list, with 29 documents corresponding to about 26% of the documents published by the top 10 journals. This was followed closely by Food Control with 13 published documents and Postharvest Biology and Technology with 12 documents. The highest number of publications by Computers and Electronics in Agriculture may be attributed to the scope of the journal, which provides international coverage of advances in the development and application of computer hardware, software, electronic instrumentation, and control systems for solving problems in agriculture with specific emphasis on postharvest agriculture. Additionally, the journal covers relevant technology areas, including intelligence, sensors, machine vision, robotics, networking, and simulation modeling. Furthermore, given the distribution of the top 10 journals, the scope of the journals either falls within the technology application or the evaluation of food quality. Among the top 10 journals, the lowest number of published documents (n = 7) appeared in Food Chemistry, Food Analytical Methods, and ACM International Conference Proceedings Series.
Figure 9. The top 10 relevant sources of scientific publications on AI applications in postharvest agriculture research.
Figure 10 shows the impact of the relevant sources measured according to the H-index. Computers and Electronics in Agriculture and Food Control, the top 2 in the number of publications, top the list with an H-index of 13 and 8, respectively. Following closely, Meat Science and Sensors each has an H-index of 7, and Postharvest Biology and Technology with an H-index of 6. Although some journals appeared in the top 10 according to the source impact and were not in the top 10 in the most relevant sources, such as Computers and Electronics in Agriculture, Food Control, Postharvest Biology and Technology, Sensors, Sustainability, and Food Analytical Methods appeared in both.
Figure 10. The source impact of scientific publications on AI applications in postharvest agriculture research.
From 1994 to 2022, 2,160 participated in AI applications in postharvest agriculture research, with only 24 authors of single-authored documents, constituting 1.1%. The top 10 most prolific authors are shown in Figure 11. It can be noted that Zhang X and Zhang Z are the most productive authors, with an equal number of 9 publications. Zhang X’s 2016 study summarized current methods and technologies in stream data mining with applications in Internet of Things systems for supporting fruit cold chain logistics (Juric et al., 2016). Through real-time temperature monitoring using a Wireless Sensor Network (WSN) and correlation analysis of the various quality indicators, Zhang X’s study from 2017 identified the essential quality parameter(s) in the cold chain logistics of table grapes (Xiao et al., 2017). This was followed closely by Wang J and Wang X, with 8 publications each. Other productive authors include Li Y, Li Z, Wang Z, and Zhang C, each with 7 published documents, and the bottom two authors with 6 published papers were Cancilla JC and He Y.
Figure 11. The most relevant authors of scientific publications on AI applications in postharvest agriculture research.
Figure 12 shows a graph of the top 10 author’s productivity over time, and it calculates and plots the author’s production (in terms of the number of publications and impact) over time. The graph represents a measure of an author’s relevance over time based on productivity and impact in a subject area (Forliano et al., 2021). These metrics provide an overview of the top 10 most productive authors over the last 16 years (2006–2022). The number of articles published by an author in a given period was used to determine productivity. At the same time, the impact was assessed based on the number of citations received each year.
The intensity of the color in Figure 12 is proportional to the year of the citation, and the size of the bubbles represents the various authors’ annual output. For instance, Zhang X had approximately 2.73 total citations per year in 2012 (1 document published) and published 1, 3, and 4 articles in 2016, 2017, and 2019, respectively. Before the last decade, only two authors, Li Y and Zhang Z, with 1 article each published in AI applications in postharvest research in 2006 and 2010, respectively (Figure 12). The study by Li Y in 2006 applied data mining for early warning in food supply networks (Li et al., 2006). In 2010, the work by Zhang Z developed a novel system solution for global fresh food tracking services (Pang et al., 2010). As shown in Figure 12, most are emerging authors with more active publications observed from 2018 to date. Besides, most authors achieved a higher scientific production in 2021. From Figure 12, five authors had over 15 total citations per year. Wang J had 17 total citations per year in 2020 and 2021; Wang X had approximately 17 in 2021; Zhang C had 28.5 in 2019; Cancilla JC had 18 in 2020; He Y had 29 in 2019.
Supplementary Table S2 shows the 10 most relevant author impacts ordered by h_index. Three measures are provided concerning the local dataset and the top 10 most productive authors: the times cited (TC), the h-index (h_index), the g-index (g_index), and the m-index (m_index). The h-index measures quantity with quality by comparing publications to citations. It is a metric for assessing the overall impact of an author’s scholarly output and performance. The distribution of citations received by the publications of a particular researcher is used to calculate the g-index, which gives more weight to highly cited articles. The last impact measure, the m_index, is another variant of the h_index that displays the h-index per year since its first publication. The m-index is the h_index divided by the number of years a scientist has been active (Hirsch, 2007; Forliano et al., 2021). The most cited authors in the dataset are Blasco J (269 citations) and He Y (138 citations), closely followed by Alfian G and Rhee J (128 citations). Zhang X, who was top in the author productivity over time (Figure 12), has 96 citations, h_index, g_index, and m_index of 5, 6, and 0.455, respectively. It is worth mentioning that Cancilla JC and Torrecilla JS, with publication starting year in 2020, both have the highest h_index, g_index, and m_index of 5, 6, and 1.667.
Table 2 shows the top 20 most cited documents in AI applications in postharvest agriculture based on Scopus’s data analyzed, with total citations ranging from 52 to 146. Ruiz-Garcia et al. (2008), Tsugawa et al. (2011), Lorente et al. (2013), Jiménez-Carvelo et al. (2019), Zhou et al. (2019), and Zhai et al. (2020) have ranked top with total citations above 100. Ruiz-Garcia et al. (2008), Tsugawa et al. (2011), Zhou et al. (2019), and Zhai et al. (2020) received 146, 137, 134, and 126 citations, respectively. Both Jiménez-Carvelo et al. (2019) and Lorente et al. (2013) received 105 citations.
The work by Tsugawa et al. (2011) developed a data mining system to obtain metabolite information on Japanese green tea quickly and easily by using two mathematical methods: (1) Pearson’s product–moment correlation coefficient for identification based on retention time and weighted mass spectrum and (2) Soft Independent Modeling of Class Analogy (SIMCA), a supervised classification technique that is based on principal component analysis (PCA) for the annotation of unknown peaks. The developed data analysis tool could provide essential metabolite information accurately and rapidly and offer new insights into food quality evaluation and predictions. Zhai et al. (2020) presented a state-of-the-art review of decision support systems by exploring the upcoming challenges of their utilization in Agriculture 4.0. The authors systematically analyzed the decision support systems from the aspects of interoperability, scalability, accessibility, usability, uncertainty and dynamic factors, re-planning, expert knowledge, and analysis of historical information. Ruiz-Garcia et al. (2008) explored the potential of wireless sensor technology (ZigBee) for monitoring fruit storage and transport conditions. A comprehensive review of the application of deep learning in food was provided by Zhou et al. (2019), a first in the food domain. An in-depth discussion on deep learning and its ability as a data analysis tool to solve various problems and challenges encountered in the food domain was presented. These challenges include food recognition, calorie estimation, quality detection of fruits, vegetables, meat, and aquatic products, food supply chain, and food contamination. The authors concluded that deep learning had more outstanding performance capabilities than other methods, such as manual feature extractors, conventional machine learning algorithms, and serves as a promising tool in food quality and safety inspection.
The assurance of food authenticity is the primary concern of many consumers and manufacturers of high-quality products and official bodies and authorities in response to the need to protect consumers by detecting potential food fraud. Hence, Jiménez-Carvelo et al. (2019) reviewed alternative data mining/machine learning methods for evaluating food quality and authenticity (food analytics). Here, various methods such as principal component analysis (PCA), partial least squares-discriminant analysis (PLS-DA), soft independent modeling by class analogy (SIMCA), k-nearest neighbors (kNN), parallel factor analysis (PARAFAC), and multivariate curve resolution-alternating least squares (MCR-ALS) were identified as the most widely used techniques. Nonetheless, other methods, including support vector machine (SVM), classification and regression tree (CART), and random forest (RF), show a great potential than conventional ones. Automatic detection of fungal infections in fruits and vegetables, particularly during postharvest operations such as storage, handling, and transportation, is critical because only a few infected produce can spread the infection. The study by Lorente et al. (2013) proposed a methodology to select features in multi-class classification problems using the receiver operating characteristic (ROC) curve to detect rottenness in citrus fruits through hyperspectral images. The authors aimed to distinguish fruit with decay symptoms from sound fruit with minor defects.
In summary, It can be observed in Table 2 (top 20 most cited documents) that the studies focused on the following broad areas: real-time monitoring and decision support system for perishable products, food quality evaluation, and food classification (grading and sorting). These areas are broadly discussed in section 3.4.
The keyword co-occurrence network analysis helps researchers detect the literature’s core content and depict the knowledge’s structure. It is used to identify “keywords” that co-occur in at least two publications in a period (Rejeb et al., 2021; Zhong et al., 2021). This scientometric method helps to generate clusters that provide a broader view of different research foci in a specific knowledge domain (Rejeb et al., 2021). The keyword is a significant part of scholarly publications, which can play a vital role in information retrieval and research. In this study, using VosViewer to analyze all keywords (full counting method), we selected the minimum number of keywords occurrence as 5, of which 337 met the thresholds out of the 4,979 keywords. Figure 13 shows the co-occurrence network of all keywords in AI applications in postharvest agriculture research, while the occurrences and link strengths of all keywords are shown in Supplementary Table S3. Total link strength (TLS) represents the collaboration intensity of keywords. The link strength between the circles reflects the frequency of the keyword’s co-occurrence. The total link strength is the sum of the link strengths of the keyword over all the other keywords (Guo et al., 2019; Martynov et al., 2020). As shown in Figure 13, we identified five clusters of words with distinct colors. The nodes in the figure indicate a keyword, and the node size corresponds to the co-occurrence frequency of the keyword or the number of publications with the corresponding keywords. The distance between two keywords in the visualization is determined by density, and the higher this density, the closer the distance between the nodes (Rejeb et al., 2021).
The red cluster (cluster 1, with 123 items) has artificial intelligence as the highest occurring keyword. Cluster 2, represented by green, contained 86 items, with food quality as the highest occurring keyword. Clusters 3 and 4, represented by blue and yellow, have 67 and 60 items, with machine learning and deep learning as the highest occurring keywords, respectively. Finally, cluster 5, represented by purple color, has only one item (trees) as the only occurring keyword in the cluster.
Supplementary Table S3 shows the top 10 keywords in the co-occurrence all-keyword analysis. As can be seen, the highest occurring keywords in clusters 1 to 4 appeared in the top 10. Machine learning had the highest occurrence (178), which was followed by food quality (146), artificial intelligence (100), and deep learning (94). Other keywords in the top 10 include quality control (71), learning systems (67), food safety (64), fruits (64), learning algorithms (59), and data mining (59). Additionally, it was observed that the total link strength was independent of the keyword occurrences (Supplementary Table S3). Machine learning and data mining had the highest and lowest total link strength, respectively. The total link strength indicates the number of publications in which two keywords occur together.
Author keywords are those provided by the original authors. Here, we selected the minimum number of keyword occurrences as 5, of which 67 met the thresholds out of 1,653. Figure 14 shows the co-occurrence network of author keywords in AI applications in postharvest agriculture research. The co-occurrence network has 6 clusters represented with distinct colors. Specifically, as was shown in the red cluster (cluster 1, 16 items), keywords such as machine learning, deep learning, random forest, support vector machine, feature extraction, food processing, pattern recognition, etc., are evidently related to the topic of “AI algorithms in food processing.” In this cluster, machine learning was the highest occurring keyword. The green color represents cluster 2 (15 items) has artificial intelligence as the highest occurring keyword. Other keywords in this cluster are cold chain, cold chain logistics, real-time monitoring, wireless sensor network, etc. Cluster 3 was represented in blue and contained 11 items with keywords such as big data, food safety, food security, sustainability, traceability, industry 4.0, etc. The highest keyword in this cluster was food safety, which may be attributed to the topic of “food safety and sustainability.” Clusters 4 and 5, represented by yellow and purple colors, each have 10 items, respectively. Cluster 4 contained keywords such as food, feature selection, and food quality (highest occurring keyword). The keywords in cluster 5 include agriculture, precision agriculture, sensors, smart farming, food waste, etc. In this cluster, food waste was the highest occurring keyword. Finally, Cluster 6 has 5 items and contains keywords such as adulteration, food fraud, transfer learning, and convolution neural network (highest occurring keyword).
Supplementary Table S4 shows the top 10 keywords in the co-occurrence author-keyword analysis. Machine learning, artificial intelligence, deep learning, food quality, food safety, and convolution neural network were the highest occurring keywords in clusters 1, 2, 3, 4, 5, and 6, respectively, appearing in the top 10 author keywords occurrences. Like the all-keywords analysis, machine learning had the highest occurrence (116) in the author-keyword analysis. This was followed by deep learning (63), food quality (43), artificial intelligence (28), computer vision (26), classification (24), food safety (24), image processing (22), big data (19), and convolutional neural network (19). From Supplementary Table S4, Machine learning and big data had the highest and lowest total link strength, respectively. The total link strength indicates the number of publications in which two keywords occur together.
Scopus selects index keywords, standardized to vocabularies derived from thesauri owned or licensed by Elsevier. Index keywords, as opposed to Author keywords, consider synonyms, alternate spellings, and plurals (Golub et al., 2020). For the index keywords, we selected the minimum number of keywords occurrence as 5, of which 302 met the thresholds out of the 4,014 keywords. The co-occurrence network of index keywords in AI applications in postharvest agriculture research is shown in Figure 15. The co-occurrence network contains five clusters. Cluster 1, represented in red, contains 98 items with keywords such as artificial intelligence, data mining, Internet of Things, supply chain, cold chain logistics, food supply, and food waste. In this cluster, artificial intelligence was the highest occurring keyword and part of the top 10 index keywords occurrences (Supplementary Table S5). The green cluster represents the second cluster (72 items) and has deep learning as the highest occurring keyword. Other keywords in this cluster include learning systems, learning algorithms, neural networks, computer vision, image processing, support vector machines, decision trees, and fruits. Cluster 3, with 60 items in blue, has machine learning as the keyword with the highest occurrence. Other keywords in cluster 3 are quality control, artificial neural networks, and fruits. The yellow color represents cluster 4 (59 items) containing keywords such as food quality, metabolism, metabolomics, and algorithms. In this cluster, the occurrence of food quality was highest. The last cluster (cluster 5, 13 items), represented by purple, has forecasting as the highest occurring keyword. Other keywords in cluster 5 include vegetables and predictive analytics.
Supplementary Table S5 shows the top 10 keywords in the co-occurrence index-keyword analysis. As can be seen, the highest occurring keywords in clusters 1 to 4, except cluster 5, appeared in the top 10. Food quality had the highest occurrence (130), which was closely followed by machine learning (101), artificial intelligence (80.), and deep learning (72). Other keywords in the top 10 include quality control (70), learning systems (67), fruits (64), food safety (59), learning algorithms (58), and data mining (57). Here, food quality had the highest total link strength in contrast to the analyses of all keywords and author keywords, where machine learning had the highest total link strength (Supplementary Tables S3, S4). Furthermore, it was observed that the total link strength was independent of the keyword occurrences (Supplementary Table S5).
Figure 16 shows the thematic map of the keyword plus the dataset investigated in this study. The map is divided into four quadrants: Niche themes, Motor themes, Basic themes, and Emerging themes based on relevance degree (centrality) and development degree (density). In the analysis, the number of words was set as 1,000, the minimum cluster frequency (per thousand documents) was set as 5, the number of labels was set as 5, and the clustering algorithm used was the “Walktrap” algorithm. Based on Figure 16, more emphasis should be placed on the topic in the upper right quadrant based on its density and relevance. Here, it was observed that topics such as food quality, machine learning, deep learning, quality control, and learning systems have high density and relevance and should be examined and researched in-depth. The topics in the upper left quadrant have a high development degree and low centrality (i.e., relevance). The basic theme quadrant (lower right quadrant) contains topics such as artificial intelligence, food safety, data mining, food supply, and food waste; these topics have relatively high development and relevance degrees. Although the trend topics in the upper right quadrant are the most promising topics for future research, the topics in the lower right quadrant (basic themes) also have favorable research prospects and are worth investigating. Furthermore, the lower left quadrant contains topics such as the Internet of Things, cold chain logistics, big data, decision making, and real time monitoring. These topics have low development degree and relevance degree.
To show the historical facets of research shift to direct prospects, thematic evolution has become an effective technique in bibliometrix analysis (Moral-Munoz et al., 2018; Chansanam and Li, 2022). This technique highlights the most significant research themes and charts theme evolution through time, offering insight into the field’s future path (Chen et al., 2019; Chansanam and Li, 2022). Figure 17 depicts the thematic evolution of the keyword plus based on the dataset from 1994 to 2022. Two periods were chosen as cut-off points: 2008 and 2009. These cut-off points were based on the publication results by year (Figure 3), where we observed a significant increase in the number of publications in 2009, which has continued to increase. In the first period (1994–2008), the popular keywords were food processing and artificial intelligence, merged in the next period (2009–2022) as food quality, deep learning, and artificial intelligence. It was observed that the term artificial intelligence in the first period (1994–2008) is separated into two branches in the second period (2009–2022): food quality and artificial intelligence. In comparison, food processing in the first period is categorized into two in the second period: food quality and deep learning. This trend demonstrates that food quality is a core topic in the second period.
Figure 18 shows the co-authorship-countries network. Here, we selected the maximum number of countries per document as 25 and the minimum number of documents of a country as 5, of which out of 79 countries, 38 met the threshold. The network contains seven clusters. Cluster 1, represented with the orange color, contains 10 items, with Iran having the highest occurrence. The second and third clusters (clusters 2 and 3) have 7 and 5 items, represented by the colors green and blue, respectively. In clusters 2 and 3, the countries with the highest occurrence were Italy and Spain, respectively. Clusters 4 and 5 (represented with pink and purple) contain 5 items, with the United States and China as the countries with the highest occurrences, respectively. In cluster 6 (light blue color), the United Kingdom occurred the highest, and the cluster contains 5 items. The last cluster (cluster 7), represented in yellow color contains 2 items, has South Korea occurring the highest, followed by Brazil.
In Figure 18, countries are indicated by a label and by a circle. The more critical a country, the larger its label and its circle. The size of each circle shows the number of papers written by authors from the country. Each link between two circles of different countries indicates a co-authorship between the organizations in those countries. Hence, more papers have been written by authors from China, closely followed by the United States, India, and Spain. This observation corroborates the results shown in Figure 5. It is with mentioning that the United States network links with all the clusters.
The postharvest stage is the final and most critical agricultural stage that needs extensive attention (Meshram et al., 2021). After completing all stages, from preharvesting to harvesting, negligence in postharvesting may spoil all the efforts and cause severe economic loss. Many studies have shown that, for instance, farmers lose as high as 40% of fruit and vegetables before they reach the final end-users (Kitinoja et al., 2011). Activities that can be considered in the postharvest stage are factors that affect the shelf life of the food and postharvest handling processes to maintain the food quality (Meshram et al., 2021). Hence, postharvest management is a collection of post-production practices that includes cleaning, washing, selection, grading, disinfection, drying, packing, and storage. These remove undesirable elements and improve product appearance while ensuring the product meets established quality standards for fresh and processed products (El-Ramady et al., 2015). Based on the analyzed data and the observed research evolution and trend, we categorized opportunities for future research into three broad categories discussed below.
The appearance of food, mainly fruits and vegetables, is an important property that influences its market value, affects consumer choices, and, to some extent, is an indicator of its internal quality (Naik and Patel, 2017). Human graders have typically performed quality inspections and classified agricultural and food products. Some features such as color, texture, size, shape, weight, and visual defects are generally examined to assess the outside quality of food (Naik and Patel, 2017). However, most manual inspections are time-consuming and labor-intensive (Brosnan and Sun, 2002; Naik and Patel, 2017). Moreover, the manual classification of food products exacerbates postharvest losses, but technology and emerging algorithms offer solutions to reduce such losses (Piedad et al., 2018). Hence, there is a need for a fast, efficient, and intelligent food grading system. Artificial Intelligence is rapidly becoming a part of agriculture’s technological evolution to increase food production and reduce losses. Artificial intelligence in food classification, grading, and sorting offers an excellent opportunity for reducing the manual work of classification and sorting to improve the quality of food grading. For instance, computer vision and numerous algorithms (such as machine learning and deep learning) help to achieve the required food classification and grading to produce accurate, rapid, consistent, and efficient outcomes over manual work, which can be done automatically, provided some standard grading criteria are made (Naik and Patel, 2017; Behera et al., 2020; Bhargava et al., 2022). The following are examples of research questions:
a. How can AI technologies aid the identification and classification of the ripening degree of different produce?
b. What are the possibilities of classifying various agricultural produce using higher-level features with more discriminative information with deep learning methods?
In food identification and classification, particularly in fresh produce, previous endeavors have commonly revolved around using various sensors in conjunction with machine learning or deep learning techniques. The primary objective of these efforts has been to decipher and identify the intrinsic features associated with different types of produce. For instance, attributes such as shape, color, texture, and size in fruit and vegetables have been harnessed to execute classification tasks (Hameed et al., 2018). However, classifying fruits and vegetables remains a formidable challenge, primarily due to several factors. These include the irregularities observed in their shapes, variations in size, the dynamic range of colors, and the profound variability inherent within a single category. The latter is particularly pronounced in cases where the maturity phase significantly influences visual characteristics, particularly in the context of fruits (Hameed et al., 2018; Hossain et al., 2018).
Addressing the intricate challenges stemming from their inherent diversity, the next generation of AI models is poised to take a transformative approach. These future models will be engineered to accommodate the multifaceted differences encompassing shape, size, color, and ripeness. Deep learning architectures, honed through extensive and diverse datasets, are set to fortify the system’s resilience across various environmental conditions. This resilience is a testament to the AI model’s capacity to withstand the complexities arising from variations in lighting, perspectives, and environmental factors, ensuring a steadfast and reliable performance (Shahi et al., 2022). Moreover, the augmentation of AI’s capabilities extends to the mastery of comprehensive datasets that encapsulate a diverse spectrum of fruit and vegetable characteristics. This embracement of a broad knowledge base enables AI systems to traverse beyond the confines of specific conditions and generalize their discernment. Such adaptability proves indispensable in securing accurate classifications, thus facilitating confident and precise identifications.
Furthermore, incorporating multispectral imaging techniques introduces an innovative dimension (Gaikwad and Tidke, 2022). By extending the scope of analysis beyond the constraints of the visible spectrum, AI-powered systems are endowed with the capability to capture information that transcends human visual perception. This broader perspective facilitates the identification of nuanced distinctions in elements such as shape attributes, color variations, surface textures, ripeness indicators, and even the freshness of the produce. As the amalgamation of AI technologies and multispectral imaging paves the way for a more comprehensive understanding of produce characteristics, the horizon of food classification and identification stands poised for a paradigm shift. These combined advancements address the complexities posed by variability and unlock novel avenues for improved accuracy, efficiency, and reliability, ultimately shaping the future landscape of food classification and identification.
Another aspect that has been identified is food quality. Although supplying similar products is critical, food quality is essential to the consumer’s delight (Hemamalini et al., 2022). Food quality assessment, including freshness assessment, is rapidly becoming a priority for both end-users (consumers) and food processing industries (Takruri et al., 2020). Although it is a complex concept that is frequently measured using objective indices related to the food’s nutritional, microbiological, or physicochemical characteristics or designated expert opinions (Cardello, 1995; Giménez et al., 2012), its role in decision-making regarding storage and processing requirements is critical (Takruri et al., 2020). Typically, conventional methods (chemical or biological analyses) are used to evaluate food quality. However, they are time-consuming, costly, require highly skilled or trained technicians (operators), and are often destructive, resulting in a high financial loss. Similarly, the use of nondestructive techniques for evaluating different food quality attributes has grown over the years and has proved to be rapid and accurate in estimating food quality. These include near-infrared spectroscopy, Fourier transform infrared spectroscopy, color and visual spectroscopy, electronic nose and tongue, computer vision (image analysis), ultrasound, X-ray, CT, and magnetic resonance imaging (Jha, 2010; Amoriello et al., 2019; Pu et al., 2019; Wang et al., 2019; Dold and Langowski, 2022; Malvandi et al., 2022). While nondestructive methods are beneficial for quality testing, their implementation necessitates expertise, skill, and experience; besides, data processing can be computationally expensive (Abasi et al., 2018).
Consumers place a high value on food quality, mainly fresh fruits and vegetables. Besides, it is critical to breeding activities’ success and the fruit industry’s competitiveness and profitability (Mezzetti et al., 2018; Amoriello et al., 2022). The entire food supply chain has recently demanded simple and quick quality evaluation systems. Artificial intelligence, machine learning, and deep learning have provided an avenue to effectively evaluate different food quality characteristics and play a crucial role in food safety and quality assurance (Dharmaraj and Vijayanand, 2018; Liu, 2020). For instance, the chemical attributes of food, mainly fruits and vegetables (such as total soluble solids, acidity, pH, firmness, etc.) and nutritional properties (such as total phenols, anthocyanins, antioxidant potential, etc.) can be determined efficiently using these techniques.
Furthermore, it is well established that the deterioration of most fruits and vegetables is linked to rapid metabolism, cellular damage, and softening that occurs due to external damage during postharvest handling (Li and Thomas, 2014; Fadiji et al., 2016a,b; Hussein et al., 2018; Opara and Fadiji, 2018; Choi et al., 2021). AI, ML, and DL can quickly detect small changes or defects that may occur in the produce subjected to mechanical damage during handling, distribution, and storage, allowing easy monitoring and sorting (Azizah et al., 2017). Also, these techniques are becoming valuable tools in conjunction with sensing devices and computer vision to detect pathological disorders in food associated with attacks by viruses, fungi, bacteria, or microbial pathogens (Ray et al., 2017; Cui et al., 2018; Gokulnath, 2021); physiological stresses, which can lead to disorders such as bitter pit, watercore, mealiness, sunburn, browning, superficial scald, granulation, and internal drying, among others (Magwaza et al., 2012; Zhang et al., 2020; Tang et al., 2022); morphological disorders that manifest themselves as deformations that make foods have an irregular or abnormal appearance (Moallem et al., 2017; Ireri et al., 2019); internal defects (Arendse et al., 2016, 2018a,b; Nturambirwe and Opara, 2020; Van De Looverbosch et al., 2020; Dubey et al., 2021; Çetin et al., 2022; Okere et al., 2022). The ability to detect or identify various food defects aids in reducing the risk of spoilage and decay. Moreover, identifying and sorting defective produce reduces contamination of healthy produce, mitigating food losses while maintaining high consumer satisfaction.
The following are examples of research questions:
a. How can AI technologies improve the shortcomings of physical field drying of fruits and vegetables?
b. How can AI technologies detect defects in produce such as fruit and vegetables effectively?
c. What is the feasibility of applying AI techniques (deep learning) in combination with other promising techniques, such as thermography and magnetic resonance, for the early detection of defects?
The application and development of Artificial Intelligence (AI) in the domain of food drying have garnered substantial attention. With advanced technologies such as big data and cloud computing, AI is dramatically reshaping the global landscape and transforming everyday life (Chen et al., 2020; Misra et al., 2020). AI emerges as a transformative solution as we strive to achieve high-quality end products, reduce operational and energy costs, enhance production rates, and optimize the design and operational parameters of industrial-scale dryers. Its inherent self-learning ability, adaptability, robust fault tolerance, and proficiency in mapping complex and dynamic phenomena make it a potent alternative strategy for various aspects of drying modeling, physicochemical property analysis, and quality optimization.
The utilization of AI in food drying has been particularly impactful in addressing critical challenges. Dry intelligence innovation, energy conservation, and intelligent process control are focal points that demand attention to ensure superior drying outcomes. AI’s capabilities have been harnessed to tackle these challenges, offering innovative approaches that lead to enhanced results, reduced costs, and optimized operational parameters.
AI’s contributions encompass a spectrum of applications, including the modeling, predicting, and optimizing of essential parameters such as heat and mass transfer, thermodynamic performance metrics, quality indicators, and physicochemical properties of dried products. These applications span artificial biomimetic technologies, such as electronic nose and computer vision, and various conventional drying methods. The implementation of AI tools, including Artificial Neural Networks (ANN), fuzzy logic, expert systems, and evolutionary algorithms, either individually or in combination, has proven exceptionally effective in addressing intricate problems within the drying process (Aghbashlo et al., 2015; Sun et al., 2019).
The potential of AI in defect detection holds significant promise, particularly in achieving the coveted characteristic of “fast detection.” While computer vision has made substantial strides in defect detection, its scope is often limited to surface-level defects. However, integrating hyperspectral and multispectral imaging with AI technologies presents a compelling avenue for rapid and comprehensive defect detection (Feng and Sun, 2012; Soni et al., 2022). Hyperspectral and multispectral imaging technologies are emerging as powerful contenders for rapidly detecting agricultural produce defects. AI, and specifically DL, plays a pivotal role in effectively implementing these “fast” detection systems (Wieme et al., 2022). The advancements enabled by DL in image processing and feature extraction have revolutionized the efficiency of direct defect detection. Despite the potential demonstrated by the application of DL in this domain, research in this area, particularly in the context of adulteration detection, remains relatively scarce. Consequently, there is a pressing need for further research to harness the full potential of AI and DL in realizing these goals.
Additionally, AI’s successful application extends to the quantitative analysis of fruit deterioration, encompassing factors such as severity and degree of damage defects. In conjunction with objective non-destructive measuring instruments, learning methods have been employed to distinguish between different extents of deterioration. However, despite the advancements, this study area remains under-explored in leveraging sophisticated AI algorithms. The quantification of damage and disorders continues to pose challenges in the agriculture industry, warranting heightened attention to utilizing learning algorithms for enhanced accuracy and efficiency.
Due to the increased consumer demand for food, food industries are not keeping up with the demand–supply chain and also lacking in food safety. Similarly, until now, retailers lack visibility into the real-time status of foods in stock, resulting in massive food losses and waste. AI technology is a promising tool for monitoring food status in the supply chain (Wang et al., 2022). It is critical to monitor potential food safety hazards throughout the food supply chain to ensure the proper operation of food safety management systems and, consequently, the food products [International Organization for Standardization (ISO), 2013; Focker et al., 2018]. Besides, an AI-based system can manage food production and distribution processes more efficiently and effectively based on AI, ML, and DL algorithms (Kumar et al., 2021; Wang et al., 2022). For instance, the cold chain, in particular, plays an essential role in the supply chain’s quality control of perishable foods such as fresh fruits and vegetables (Lu and Wang, 2016; Loisel et al., 2021). Fluctuating temperatures or breaks could rapidly deteriorate the perishable products and significantly impact the food shelf life (Ndraha et al., 2018; Villa-Gonzalez et al., 2022). It is, therefore, critical to maintaining optimum environmental conditions to control the quality of perishable foods in real-time, detect cold chain breaks, and measure their impact on product quality. AI has proven to be more precise and efficient in performance compared to traditional or physic-based systems (models; Mercier and Uysal, 2018; Hoang et al., 2021; Loisel et al., 2022). In the cold chain, wireless temperature sensors and data transmission are expected to be widely used to provide a large amount of data, making real-time analyses and predictions possible (Loisel et al., 2021). Additionally, with the structured infrastructure of the sensor network in IoT (Internet of Things) and robust computations in AI, integrating IoT and AI is a promising way to establish risk monitoring systems in the cold chain for controlling product quality (Tsang et al., 2018).
Another aspect in which AI could be useful is in the area of food traceability. Food traceability involves tracking, tracing, and evaluating food quality along the supply chain and providing consumers with information to increase consumer experience and product confidence in the food supply chain (Aung and Chang, 2014; Islam and Cullen, 2021). AI has shown the potential to improve how food is traced, decrease losses and waste, and mitigate the vulnerability to food fraud (Hassoun et al., 2022).
This study conducted a bibliometric analysis utilizing techniques such as co-authorship, co-occurrence, co-citations, and visualization networks of AI applications in postharvest agriculture research. The study analyzed 586 documents obtained from Scopus and the most extended study period (1994–2022) to present the state of the art, future trends and identify research tendencies. The review results can be relevant to researchers actively applying AI in postharvest agriculture. Several significant observations were made in this study. First, the number of papers on AI applications in postharvest agriculture has substantially grown, particularly from 2009 to 2022. Country-wise productivity showed China and USA as the top countries in the research area. The distribution of papers published according to journals suggested that Computers and Electronics in Agriculture is the top or leading journal contributing to the knowledge on AI application in postharvest agriculture. Regarding author productivity, Zhang X and Zhang Z are the most prolific authors in the research area. In terms of contributions of academic institutions, results revealed Zhejiang University, located in China, as the most productive institution. The keywords analysis showed that the frequently used keywords include machine learning, food quality, artificial intelligence, deep learning, quality control, learning systems, food safety, fruit, learning algorithms, and data mining. According to the thematic map trend, food quality, machine learning, deep learning, quality control, and learning systems are well-developed themes fundamental for structuring the research field. Whereas Internet of Things, cold chain logistics, big data, decision making, and real time monitoring are weakly developed and marginal areas in the research domain.
The present study can help researchers and practitioners embarking on this topic who want a comprehensive overview of the scientific literature produced. Moreover, scholars can leverage the results of this study to address future studies better, considering the proposed avenues for future research. Additionally, this work can provide a valuable perspective for future research in the studied knowledge domain since it demonstrates the existence of an emerging area of study intended to enhance quality and reduce postharvest losses and waste in horticultural crops.
In the present study, only one database was used: Scopus. Scopus is a widely recognized multidisciplinary abstract and citation database offering comprehensive coverage of academic literature from various disciplines. Its user-friendly interface and powerful search capabilities make it efficient and familiar to researchers. Focusing on one database makes the study’s literature review process time and resource-efficient while ensuring access to peer-reviewed and high-quality content. Moreover, Scopus provides citation analysis and impact metrics to assess research significance. The consistency in data retrieval enhances data management and analysis throughout the study. The specificity of Scopus also aligns with the study’s focus, allowing for in-depth analysis and a comprehensive understanding of the research topic. Nevertheless, while Scopus is a valuable resource, future work could benefit from including additional databases such as the Web of Science (WOS) and Medline to strengthen the study’s robustness and broaden its scope. Furthermore, the keywords used in the search string may have excluded some important papers. Hence, for more enriched results, future studies should include more keywords. Another limitation is the lack of citations for newly published papers. New research takes time to accumulate citations, while older publications have more citations.
The raw data supporting the conclusions of this article will be made available by the authors, without undue reservation.
TF, TB, OF, and HT: conceptualization and writing—review and editing. TF and TB: methodology and writing—original draft preparation. OF and HT: funding acquisition. All authors contributed to the article and approved the submitted version.
This work was supported by the research grant from the University Research Committee (URC): Faculty Strategic Intervention at the University of Johannesburg for the Postharvest Applied Data Science Flagship and the Global Excellence Stature Fellowship 4.0 awarded to TF at the University of Johannesburg.
The authors declare that the research was conducted in the absence of any commercial or financial relationships that could be construed as a potential conflict of interest.
All claims expressed in this article are solely those of the authors and do not necessarily represent those of their affiliated organizations, or those of the publisher, the editors and the reviewers. Any product that may be evaluated in this article, or claim that may be made by its manufacturer, is not guaranteed or endorsed by the publisher.
The Supplementary material for this article can be found online at: https://www.frontiersin.org/articles/10.3389/fsufs.2023.1226583/full#supplementary-material
Abasi, S., Minaei, S., Jamshidi, B., and Fathi, D. (2018). Dedicated non-destructive devices for food quality measurement: a review. Trends Food Sci. Technol. 78, 197–205. doi: 10.1016/j.tifs.2018.05.009
Abass, A. B., Ndunguru, G., Mamiro, P., Alenkhe, B., Mlingi, N., and Bekunda, M. (2014). Post-harvest food losses in a maize-based farming system of semi-arid savannah area of Tanzania. J. Stored Prod. Res. 57, 49–57. doi: 10.1016/j.jspr.2013.12.004
Aghbashlo, M., Hosseinpour, S., and Mujumdar, A. S. (2015). Application of artificial neural networks (ANNs) in drying technology: a comprehensive review. Drying Technol. 33, 1397–1462. doi: 10.1080/07373937.2015.1036288
Albort-Morant, G., and Ribeiro-Soriano, D. (2016). A bibliometric analysis of international impact of business incubators. J. Bus. Res. 69, 1775–1779. doi: 10.1016/j.jbusres.2015.10.054
Alfian, G., Rhee, J., Ahn, H., Lee, J., Farooq, U., Ijaz, M. F., et al. (2017). Integration of RFID, wireless sensor networks, and data mining in an e-pedigree food traceability system. J. Food Eng. 212, 65–75. doi: 10.1016/j.jfoodeng.2017.05.008
Amoriello, T., Ciccoritti, R., and Carbone, K. (2019). Vibrational spectroscopy as a green technology for predicting nutraceutical properties and antiradical potential of early-to-late apricot genotypes. Postharvest Biol. Technol. 155, 156–166. doi: 10.1016/j.postharvbio.2019.03.013
Amoriello, T., Ciccoritti, R., and Ferrante, P. (2022). Prediction of Strawberries' quality parameters using artificial neural networks. Agronomy 12:963. doi: 10.3390/agronomy12040963
Arendse, E., Fawole, O. A., Magwaza, L. S., Nieuwoudt, H., and Opara, U. L. (2018b). Fourier transform near infrared diffuse reflectance spectroscopy and two spectral acquisition modes for evaluation of external and internal quality of intact pomegranate fruit. Postharvest Biol. Technol. 138, 91–98. doi: 10.1016/j.postharvbio.2018.01.001
Arendse, E., Fawole, O. A., Magwaza, L. S., and Opara, U. L. (2016). Non-destructive characterization and volume estimation of pomegranate fruit external and internal morphological fractions using X-ray computed tomography. J. Food Eng. 186, 42–49. doi: 10.1016/j.jfoodeng.2016.04.011
Arendse, E., Fawole, O. A., Magwaza, L. S., and Opara, U. L. (2018a). Non-destructive prediction of internal and external quality attributes of fruit with thick rind: a review. J. Food Eng. 217, 11–23. doi: 10.1016/j.jfoodeng.2017.08.009
Aria, M., and Cuccurullo, C. (2017). Bibliometrix: an R-tool for comprehensive science mapping analysis. J. Informet. 11, 959–975. doi: 10.1016/j.joi.2017.08.007
Aung, M. M., and Chang, Y. S. (2014). Traceability in a food supply chain: safety and quality perspectives. Food Control 39, 172–184. doi: 10.1016/j.foodcont.2013.11.007
Azizah, L. M. R., Umayah, S. F., Riyadi, S., Damarjati, C., and Utama, N. A. (2017) Deep learning implementation using convolutional neural network in mangosteen surface defect detection. In: 2017 7th IEEE international conference on control system, computing and engineering (ICCSCE) (pp. 242–246). IEEE.
Bal, F., and Kayaalp, F. (2021). Review of machine learning and deep learning models in agriculture. Int Adv Res Engineer J 5, 309–323. doi: 10.35860/iarej.848458
Behera, S. K., Rath, A. K., Mahapatra, A., and Sethy, P. K. (2020). Identification, classification & grading of fruits using machine learning & computer intelligence: a review. J. Ambient. Intell. Humaniz. Comput., 1–11. doi: 10.1007/s12652-020-01865-8
Ben Ayed, R., and Hanana, M. (2021). Artificial intelligence to improve the food and agriculture sector. J. Food Qual. 2021:5584754. doi: 10.1155/2021/5584754
Benavides-Velasco, C. A., Quintana-García, C., and Guzmán-Parra, V. F. (2013). Trends in family business research. Small Bus. Econ. 40, 41–57. doi: 10.1007/s11187-011-9362-3
Bhargava, A., Bansal, A., and Goyal, V. (2022). Machine learning–based detection and sorting of multiple vegetables and fruits. Food Anal. Methods 15, 228–242. doi: 10.1007/s12161-021-02086-1
Brosnan, T., and Sun, D. W. (2002). Inspection and grading of agricultural and food products by computer vision systems—a review. Comput. Electron. Agric. 36, 193–213. doi: 10.1016/S0168-1699(02)00101-1
Cardello, A. V. (1995). Food quality: relativity, context and consumer expectations. Food Qual. Prefer. 6, 163–170. doi: 10.1016/0950-3293(94)00039-X
Çetin, N., Karaman, K., Kavuncuoğlu, E., Yıldırım, B., and Jahanbakhshi, A. (2022). Using hyperspectral imaging technology and machine learning algorithms for assessing internal quality parameters of apple fruits. Chemom. Intel. Lab. Syst. 230:104650. doi: 10.1016/j.chemolab.2022.104650
Chakraborty, S., Shamrat, F. J. M., Billah, M. M., Al Jubair, M., Alauddin, M., and Ranjan, R. (2021). Implementation of deep learning methods to identify rotten fruits. In 2021 5th international conference on trends in electronics and informatics (ICOEI) (pp. 1207–1212). IEEE.
Chansanam, W., and Li, C. (2022). Scientometrics of poverty research for sustainability development: trend analysis of the 1964–2022 data through Scopus. Sustainability 14:5339. doi: 10.3390/su14095339
Chen, X., Lun, Y., Yan, J., Hao, T., and Weng, H. (2019). Discovering thematic change and evolution of utilizing social media for healthcare research. BMC Med. Inform. Decis. Mak. 19, 39–53. doi: 10.1186/s12911-019-0757-4
Chen, J., Zhang, M., Xu, B., Sun, J., and Mujumdar, A. S. (2020). Artificial intelligence assisted technologies for controlling the drying of fruits and vegetables using physical fields: a review. Trends Food Sci. Technol. 105, 251–260. doi: 10.1016/j.tifs.2020.08.015
Choi, J. Y., Seo, K., Cho, J. S., and Moon, K. D. (2021). Applying convolutional neural networks to assess the external quality of strawberries. J. Food Compos. Anal. 102:104071. doi: 10.1016/j.jfca.2021.104071
Cole, M. B., Augustin, M. A., Robertson, M. J., and Manners, J. M. (2018). The science of food security. NPJ Sci Food 2, 1–8. doi: 10.1038/s41538-018-0021-9
Cucino, V., Passarelli, M., Bongiorno, G., Piccaluga, A., and Cariola, A. (2021). Student entrepreneurship: a bibliometric analysis. Piccola impresa/small. Business 3, 142–162. doi: 10.14596/pisb.2851
Cui, S., Ling, P., Zhu, H., and Keener, H. M. (2018). Plant pest detection using an artificial nose system: a review. Sensors 18:378. doi: 10.3390/s18020378
da Costa, A. Z., Figueroa, H. E., and Fracarolli, J. A. (2020). Computer vision based detection of external defects on tomatoes using deep learning. Biosyst. Eng. 190, 131–144. doi: 10.1016/j.biosystemseng.2019.12.003
de Castro, G. A., Hidalgo, E. R., Bueno, J. C. C., and Jainaga, T. I. (2021). Family business research in the last decade. A bibliometric review. European J Fam Bus 11, 33–44. doi: 10.24310/ejfbejfb.v11i1.12503
Dharmaraj, V., and Vijayanand, C. (2018). Artificial intelligence (AI) in agriculture. Int. J. Curr. Microbiol. App. Sci. 7, 2122–2128. doi: 10.20546/ijcmas.2018.712.241
Ding, Y., Chang, J., Ma, Q., Chen, L., Liu, S., Jin, S., et al. (2015). Network analysis of postharvest senescence process in citrus fruits revealed by transcriptomic and metabolomic profiling. Plant Physiol. 168, 357–376. doi: 10.1104/pp.114.255711
Dokic, K., Blaskovic, L., and Mandusic, D. (2020). From machine learning to deep learning in agriculture–the quantitative review of trends. In IOP conference series: Earth and environmental science (Vol. 614, p. 012138). IOP Publishing.
Dold, J., and Langowski, H. C. (2022). Optical measurement systems in the food packaging sector and research for the non-destructive evaluation of product quality. Food Packag. Shelf Life 31:100814. doi: 10.1016/j.fpsl.2022.100814
Donthu, N., Kumar, S., Mukherjee, D., Pandey, N., and Lim, W. M. (2021). How to conduct a bibliometric analysis: an overview and guidelines. J. Bus. Res. 133, 285–296. doi: 10.1016/j.jbusres.2021.04.070
Dubey, A. K., Arora, R. R., and Mathur, A. (2021). Fruit defect prediction model (fdpm) based on three-level validation. J. Nondestruct. Eval. 40, 1–12. doi: 10.1007/s10921-021-00778-6
El-Ramady, H. R., Domokos-Szabolcsy, É., Abdalla, N. A., Taha, H. S., and Fári, M. (2015). Postharvest management of fruits and vegetables storage. Sustain Agricult Rev 15, 65–152. doi: 10.1007/978-3-319-09132-7_2
Emami, M., Almassi, M., Bakhoda, H., and kalantari, I. (2018). Agricultural mechanization, a key to food security in developing countries: strategy formulating for Iran. Agric Food Secur 7, 1–12. doi: 10.1186/s40066-018-0176-2
Fabris, A., Biasioli, F., Granitto, P. M., Aprea, E., Cappellin, L., Schuhfried, E., et al. (2010). PTR-TOF-MS and data-mining methods for rapid characterization of agro-industrial samples: influence of milk storage conditions on the volatile compounds profile of Trentingrana cheese. J. Mass Spectrom. 45, 1065–1074. doi: 10.1002/jms.1797
Fadiji, T., Ashtiani, S. H. M., Onwude, D. I., Li, Z., and Opara, U. L. (2021). Finite element method for freezing and thawing industrial food processes. Foods 10, 869.
Fadiji, T., Coetzee, C., Chen, L., Chukwu, O., and Opara, U. L. (2016b). Susceptibility of apples to bruising inside ventilated corrugated paperboard packages during simulated transport damage. Postharvest Biol. Technol. 118, 111–119. doi: 10.1016/j.postharvbio.2016.04.001
Fadiji, T., Coetzee, C., Pathare, P., and Opara, U. L. (2016a). Susceptibility to impact damage of apples inside ventilated corrugated paperboard packages: effects of package design. Postharvest Biol. Technol. 111, 286–296. doi: 10.1016/j.postharvbio.2015.09.023
Feng, Y. Z., and Sun, D. W. (2012). Application of hyperspectral imaging in food safety inspection and control: a review. Crit. Rev. Food Sci. Nutr. 52, 1039–1058. doi: 10.1080/10408398.2011.651542
Focker, M., van Der Fels-Klerx, H. J., and Oude Lansink, A. G. J. M. (2018). Systematic review of methods to determine the cost-effectiveness of monitoring plans for chemical and biological hazards in the life sciences. Compr. Rev. Food Sci. Food Saf. 17, 633–645. doi: 10.1111/1541-4337.12340
Forliano, C., De Bernardi, P., and Yahiaoui, D. (2021). Entrepreneurial universities: a bibliometric analysis within the business and management domains. Technol. Forecast. Soc. Chang. 165:120522. doi: 10.1016/j.techfore.2020.120522
Fukase, E., and Martin, W. (2020). Economic growth, convergence, and world food demand and supply. World Dev. 132:104954. doi: 10.1016/j.worlddev.2020.104954
Gaikwad, S., and Tidke, S. (2022). Multi-spectral imaging for fruits and vegetables. Int. J. Adv. Comput. Sci. Appl. 13, 743–760. doi: 10.14569/IJACSA.2022.0130287
Giménez, A., Ares, F., and Ares, G. (2012). Sensory shelf-life estimation: a review of current methodological approaches. Food Res. Int. 49, 311–325. doi: 10.1016/j.foodres.2012.07.008
Gokulnath, B. V. (2021). Identifying and classifying plant disease using resilient LF-CNN. Eco. Inform. 63:101283. doi: 10.1016/j.ecoinf.2021.101283
Golub, K., Tyrkkö, J., Hansson, J., and Ahlström, I. (2020). Subject indexing in humanities: a comparison between a local university repository and an international bibliographic service. J. Doc. 76, 1193–1214. doi: 10.1108/JD-12-2019-0231
Gunasekaran, S., and Ding, K. (1994). Using computer vision for food quality evaluation: applications of immunobiosensors and bioelectronics in food sciences and quality control. Food Technol. 48, 151–154.
Guo, Y. M., Huang, Z. L., Guo, J., Li, H., Guo, X. R., and Nkeli, M. J. (2019). Bibliometric analysis on smart cities research. Sustainability 11:3606. doi: 10.3390/su11133606
Hailu, G., and Derbew, B. (2015). Extent, causes and reduction strategies of postharvest losses of fresh fruits and vegetables–a review. J Biol Agric Healthcare 5, 49–64.
Hameed, K., Chai, D., and Rassau, A. (2018). A comprehensive review of fruit and vegetable classification techniques. Image Vis. Comput. 80, 24–44. doi: 10.1016/j.imavis.2018.09.016
Hassoun, A., Alhaj Abdullah, N., Aït-Kaddour, A., Ghellam, M., Beşir, A., Zannou, O., et al. (2022). Food traceability 4.0 as part of the fourth industrial revolution: key enabling technologies. Crit. Rev. Food Sci. Nutr., 1–17. doi: 10.1080/10408398.2022.2110033
Hemamalini, V., Rajarajeswari, S., Nachiyappan, S., Sambath, M., Devi, T., Singh, B. K., et al. (2022). Food quality inspection and grading using efficient image segmentation and machine learning-based system. J. Food Qual. 2022, 1–6. doi: 10.1155/2022/5262294
Hirsch, J. E. (2007). Does the h index have predictive power?. Proceedings of the National Academy of Sciences, 104, 19193–19198.
Hoang, H. M., Akerma, M., Mellouli, N., Le Montagner, A., Leducq, D., and Delahaye, A. (2021). Development of deep learning artificial neural networks models to predict temperature and power demand variation for demand response application in cold storage. Int. J. Refrig. 131, 857–873. doi: 10.1016/j.ijrefrig.2021.07.029
Hossain, M. S., Al-Hammadi, M., and Muhammad, G. (2018). Automatic fruit classification using deep learning for industrial applications. IEEE Trans. Industr. Inform. 15, 1027–1034. doi: 10.1109/TII.2018.2875149
Humston, E. M., Knowles, J. D., McShea, A., and Synovec, R. E. (2010). Quantitative assessment of moisture damage for cacao bean quality using two-dimensional gas chromatography combined with time-of-flight mass spectrometry and chemometrics. J. Chromatogr. A 1217, 1963–1970. doi: 10.1016/j.chroma.2010.01.069
Hussein, Z., Fawole, O. A., and Opara, U. L. (2018). Preharvest factors influencing bruise damage of fresh fruits–a review. Sci. Hortic. 229, 45–58. doi: 10.1016/j.scienta.2017.10.028
Indira, D. N. V. S. L. S., Goddu, J., Indraja, B., Challa, V. M. L., and Manasa, B. (2021). A review on fruit recognition and feature evaluation using CNN. Materials Today: Proceedings.
International Organization for Standardization (ISO). (2013). ISO 22003 food safety management systems. Available at: https://www.iso.org/standard/60605.html
Ireri, D., Belal, E., Okinda, C., Makange, N., and Ji, C. (2019). A computer vision system for defect discrimination and grading in tomatoes using machine learning and image processing. Artificial Intell Agric 2, 28–37. doi: 10.1016/j.aiia.2019.06.001
Islam, S., and Cullen, J. M. (2021). Food traceability: a generic theoretical framework. Food Control 123:107848. doi: 10.1016/j.foodcont.2020.107848
Jha, S. N. (2010). Nondestructive evaluation of food quality: Theory and practice. Heidelberg: Springer Science & Business Media.
Jiménez-Carvelo, A. M., González-Casado, A., Bagur-González, M. G., and Cuadros-Rodríguez, L. (2019). Alternative data mining/machine learning methods for the analytical evaluation of food quality and authenticity–a review. Food Res. Int. 122, 25–39. doi: 10.1016/j.foodres.2019.03.063
Juric, P., Brkic Bakaric, M., Wang, X., Zhang, X., and Matetic, M. (2016). Mining data streams for the analysis of parameter fluctuations in IoT-aided fruit cold-chain. In: Annals of DAAAM & Proceedings, 0756.
Kakani, V., Nguyen, V. H., Kumar, B. P., Kim, H., and Pasupuleti, V. R. (2020). A critical review on computer vision and artificial intelligence in food industry. J Agricult Food Res 2:100033. doi: 10.1016/j.jafr.2020.100033
Kamilaris, A., and Prenafeta-Boldú, F. X. (2018). Deep learning in agriculture: a survey. Comput. Electron. Agric. 147, 70–90. doi: 10.1016/j.compag.2018.02.016
Kitinoja, L., Saran, S., Roy, S. K., and Kader, A. A. (2011). Postharvest technology for developing countries: challenges and opportunities in research, outreach and advocacy. J. Sci. Food Agric. 91, 597–603. doi: 10.1002/jsfa.4295
Koirala, A., Walsh, K. B., Wang, Z., and McCarthy, C. (2019). Deep learning–method overview and review of use for fruit detection and yield estimation. Comput. Electron. Agric. 162, 219–234. doi: 10.1016/j.compag.2019.04.017
Kumar, D., and Kalita, P. (2017). Reducing postharvest losses during storage of grain crops to strengthen food security in developing countries. Foods 6:8. doi: 10.3390/foods6010008
Kumar, I., Rawat, J., Mohd, N., and Husain, S. (2021). Opportunities of artificial intelligence and machine learning in the food industry. J. Food Qual. 2021:4535567. doi: 10.1155/2021/4535567
Li, Y., Kramer, M. R., Beulens, A. J. M., and VD Vorst, J. G. A. J. (2006). Applying data Mining for Early Warning in food supply networks. In: 18th Belgium-Netherlands Conference on Artificial Intelligence, 2006. Namur, Belgium.
Li, B., Lecourt, J., and Bishop, G. (2018). Advances in non-destructive early assessment of fruit ripeness towards defining optimal time of harvest and yield prediction—a review. Plan. Theory 7:3. doi: 10.3390/plants7010003
Li, Z., and Thomas, C. (2014). Quantitative evaluation of mechanical damage to fresh fruits. Trends Food Sci. Technol. 35, 138–150. doi: 10.1016/j.tifs.2013.12.001
Liakos, K., Busato, P., Moshou, D., Pearson, S., and Bochtis, D. (2018). Machine learning in agriculture: a review. Sensors 18:2674. doi: 10.3390/s18082674
Liu, S. Y. (2020). Artificial intelligence (AI) in agriculture. IT Profess 22, 14–15. doi: 10.1109/MITP.2020.2986121
Liu, D., Zeng, X. A., and Sun, D. W. (2015). Recent developments and applications of hyperspectral imaging for quality evaluation of agricultural products: a review. Crit. Rev. Food Sci. Nutr. 55, 1744–1757. doi: 10.1080/10408398.2013.777020
Loisel, J., Cornuéjols, A., Laguerre, O., Tardet, M., Cagnon, D., Duchesne de Lamotte, O., et al. (2022). Machine learning for temperature prediction in food pallet along a cold chain: comparison between synthetic and experimental training dataset. J. Food Eng. 335:111156. doi: 10.1016/j.jfoodeng.2022.111156
Loisel, J., Duret, S., Cornuéjols, A., Cagnon, D., Tardet, M., Derens-Bertheau, E., et al. (2021). Cold chain break detection and analysis: can machine learning help? Trends Food Sci. Technol. 112, 391–399. doi: 10.1016/j.tifs.2021.03.052
Lorente, D., Aleixos, N., Gómez-Sanchis, J., Cubero, S., and Blasco, J. (2013). Selection of optimal wavelength features for decay detection in citrus fruit using the ROC curve and neural networks. Food Bioproc. Tech. 6, 530–541. doi: 10.1007/s11947-011-0737-x
Lu, S., and Wang, X. (2016). Toward an intelligent solution for perishable food cold chain management. In 2016 7th IEEE international conference on software engineering and service science (ICSESS) (pp. 852–856). IEEE.
Maflahi, N., and Thelwall, M. (2016). When are readership counts as useful as citation counts? Scopus versus M endeley for LIS journals. J. Assoc. Inf. Sci. Technol. 67, 191–199. doi: 10.1002/asi.23369
Magwaza, L. S., Opara, U. L., Terry, L. A., Landahl, S., Cronje, P. J., Nieuwoudt, H., et al. (2012). Prediction of 'Nules Clementine'mandarin susceptibility to rind breakdown disorder using Vis/NIR spectroscopy. Postharvest Biol. Technol. 74, 1–10. doi: 10.1016/j.postharvbio.2012.06.007
Makkar, T., Verma, S., and Dubey, A. K. (2017). Analysis and detection of fruit defect using neural network. In International conference on recent developments in science, engineering and technology (pp. 554–567). Springer, Singapore.
Malvandi, A., Feng, H., and Kamruzzaman, M. (2022). Application of NIR spectroscopy and multivariate analysis for non-destructive evaluation of apple moisture content during ultrasonic drying. Spectrochim. Acta A Mol. Biomol. Spectrosc. 269:120733. doi: 10.1016/j.saa.2021.120733
Martynov, I., Klima-Frysch, J., and Schoenberger, J. (2020). A scientometric analysis of neuroblastoma research. BMC Cancer 20, 1–10. doi: 10.1186/s12885-020-06974-3
Marvin, H. J., Bouzembrak, Y., van der Fels-Klerx, H. J., Kempenaar, C., Veerkamp, R., Chauhan, A., et al. (2022). Digitalization and artificial intelligence for sustainable food systems. Trends Food Sci. Technol. 120, 344–348. doi: 10.1016/j.tifs.2022.01.020
Mercier, S., and Uysal, I. (2018). Neural network models for predicting perishable food temperatures along the supply chain. Biosyst. Eng. 171, 91–100. doi: 10.1016/j.biosystemseng.2018.04.016
Meshram, V., Patil, K., Meshram, V., Hanchate, D., and Ramkteke, S. D. (2021). Machine learning in agriculture domain: a state-of-art survey. Artificial Intell Life Sci 1:100010. doi: 10.1016/j.ailsci.2021.100010
Mezzetti, B., Giampieri, F., Zhang, Y. T., and Zhong, C. F. (2018). Status of strawberry breeding programs and cultivation systems in Europe and the rest of the world. J Berry Res 8, 205–221. doi: 10.3233/JBR-180314
Minten, B., Tamru, S., and Reardon, T. (2021). Post-harvest losses in rural-urban value chains: evidence from Ethiopia. Food Policy 98:101860. doi: 10.1016/j.foodpol.2020.101860
Misra, N. N., Dixit, Y., Al-Mallahi, A., Bhullar, M. S., Upadhyay, R., and Martynenko, A. (2020). IoT, big data and artificial intelligence in agriculture and food industry. IEEE Internet Things J. 9, 6305–6324. doi: 10.1109/JIOT.2020.2998584
Moallem, P., Serajoddin, A., and Pourghassem, H. (2017). Computer vision-based apple grading for golden delicious apples based on surface features. Inform Process Agric 4, 33–40. doi: 10.1016/j.inpa.2016.10.003
Moral-Munoz, J. A., Arroyo-Morales, M., Herrera-Viedma, E., and Cobo, M. J. (2018). An overview of thematic evolution of physical therapy research area from 1951 to 2013. Front Res Met Analyt 3:13. doi: 10.3389/frma.2018.00013
Naik, S., and Patel, B. (2017). Machine vision based fruit classification and grading-a review. Int J Comput Appl 170, 22–34. doi: 10.5120/ijca2017914937
Naziri, D., Quaye, W., Siwoku, B., Wanlapatit, S., Viet Phu, T., and Bennett, C. (2014). The diversity of postharvest losses in cassava value chains in selected developing countries. J. Agric. Rural. Dev. Trop. Subtrop. 115, 111–123.
Ndraha, N., Hsiao, H. I., Vlajic, J., Yang, M. F., and Lin, H. T. V. (2018). Time-temperature abuse in the food cold chain: review of issues, challenges, and recommendations. Food Control 89, 12–21. doi: 10.1016/j.foodcont.2018.01.027
Nturambirwe, J. F. I., and Opara, U. L. (2020). Machine learning applications to non-destructive defect detection in horticultural products. Biosyst. Eng. 189, 60–83. doi: 10.1016/j.biosystemseng.2019.11.011
Okere, E. E., Arendse, E., Nieuwoudt, H., Perold, W. J., and Opara, U. L. (2022). Non-destructive evaluation of the quality characteristics of pomegranate kernel oil by Fourier transform near-infrared and mid-infrared spectroscopy. Front. Plant Sci. 13:867555. doi: 10.3389/fpls.2022.867555
Opara, U. L., and Fadiji, T. (2018). Compression damage susceptibility of apple fruit packed inside ventilated corrugated paperboard package. Sci. Hortic. 227, 154–161. doi: 10.1016/j.scienta.2017.09.043
Pang, Z., Chen, J., Zhang, Z., Chen, Q., and Zheng, L. (2010). Global fresh food tracking service enabled by wide area wireless sensor network. In 2010 IEEE sensors applications symposium (SAS) (pp. 6–9). IEEE.
Pawlak, K., and Kołodziejczak, M. (2020). The role of agriculture in ensuring food security in developing countries: considerations in the context of the problem of sustainable food production. Sustainability 12:5488. doi: 10.3390/su12135488
Piedad, E. J., Larada, J. I., Pojas, G. J., and Ferrer, L. V. V. (2018). Postharvest classification of banana (Musa acuminata) using tier-based machine learning. Postharvest Biol. Technol. 145, 93–100. doi: 10.1016/j.postharvbio.2018.06.004
Prusky, D. (2011). Reduction of the incidence of postharvest quality losses, and future prospects. Food Secur 3, 463–474. doi: 10.1007/s12571-011-0147-y
Pu, H., Lin, L., and Sun, D. W. (2019). Principles of hyperspectral microscope imaging techniques and their applications in food quality and safety detection: a review. Compr. Rev. Food Sci. Food Saf. 18, 853–866. doi: 10.1111/1541-4337.12432
Ray, M., Ray, A., Dash, S., Mishra, A., Achary, K. G., Nayak, S., et al. (2017). Fungal disease detection in plants: traditional assays, novel diagnostic techniques and biosensors. Biosens. Bioelectron. 87, 708–723. doi: 10.1016/j.bios.2016.09.032
Rejeb, A., Rejeb, K., Abdollahi, A., Zailani, S., Iranmanesh, M., and Ghobakhloo, M. (2021). Digitalization in food supply chains: a bibliometric review and key-route main path analysis. Sustainability 14:83. doi: 10.3390/su14010083
Ren, C., Kim, D. K., and Jeong, D. (2020). A survey of deep learning in agriculture: techniques and their applications. J Information Process Syst 16, 1015–1033. doi: 10.3745/JIPS.04.0187
Rey-Martí, A., Ribeiro-Soriano, D., and Palacios-Marqués, D. (2016). A bibliometric analysis of social entrepreneurship. J. Bus. Res. 69, 1651–1655. doi: 10.1016/j.jbusres.2015.10.033
Rons, N. (2018). Bibliometric approximation of a scientific specialty by combining key sources, title words, authors and references. J. Informet. 12, 113–132. doi: 10.1016/j.joi.2017.12.003
Ropodi, A. I., Panagou, E. Z., and Nychas, G. J. (2016). Data mining derived from food analyses using non-invasive/non-destructive analytical techniques; determination of food authenticity, quality & safety in tandem with computer science disciplines. Trends Food Sci. Technol. 50, 11–25. doi: 10.1016/j.tifs.2016.01.011
Roy, K., Chaudhuri, S. S., and Pramanik, S. (2021). Deep learning based real-time industrial framework for rotten and fresh fruit detection using semantic segmentation. Microsyst. Technol. 27, 3365–3375. doi: 10.1007/s00542-020-05123-x
Ruiz-Garcia, L., Barreiro, P., and Robla, J. I. (2008). Performance of ZigBee-based wireless sensor nodes for real-time monitoring of fruit logistics. J. Food Eng. 87, 405–415. doi: 10.1016/j.jfoodeng.2007.12.033
Sahni, V., Srivastava, S., and Khan, R. (2021). Modelling techniques to improve the quality of food using artificial intelligence. J. Food Qual. 2021:2140010. doi: 10.1155/2021/2140010
Saima, P., Bushra, I., Humaira, K., Shazia, S., and Ali, A. M. (2014). Value addition: a tool to minimize the post-harvest losses in horticultural crops. Greener J Agric Sci 4, 195–198. doi: 10.15580/GJAS.2014.5.042914208
Santos, L., Santos, F. N., Oliveira, P. M., and Shinde, P. (2019). Deep learning applications in agriculture: a short review. In Iberian Robotics Conference (pp. 139–151). Springer, Cham.
Semary, N. A., Tharwat, A., Elhariri, E., and Hassanien, A. E. (2015). “Fruit-based tomato grading system using features fusion and support vector machine” in Intelligent Systems' 2014. Ed. D. Filev (Cham: Springer), 401–410.
Sganzerla, W. G., Ampese, L. C., Mussatto, S. I., and Forster-Carneiro, T. (2021). A bibliometric analysis on potential uses of brewer's spent grains in a biorefinery for the circular economy transition of the beer industry. Biofuels Bioprod. Biorefin. 15, 1965–1988. doi: 10.1002/bbb.2290
Shahi, T. B., Sitaula, C., Neupane, A., and Guo, W. (2022). Fruit classification using attention-based MobileNetV2 for industrial applications. PloS One 17:e0264586. doi: 10.1371/journal.pone.0264586
Sharma, R., Kamble, S. S., Gunasekaran, A., Kumar, V., and Kumar, A. (2020). A systematic literature review on machine learning applications for sustainable agriculture supply chain performance. Comput. Oper. Res. 119:104926. doi: 10.1016/j.cor.2020.104926
Singh, A., Vaidya, G., Jagota, V., Darko, D. A., Agarwal, R. K., Debnath, S., et al. (2022). Recent advancement in postharvest loss mitigation and quality Management of Fruits and Vegetables Using Machine Learning Frameworks. J. Food Qual. 2022:6447282. doi: 10.1155/2022/6447282
Soni, A., Dixit, Y., Reis, M. M., and Brightwell, G. (2022). Hyperspectral imaging and machine learning in food microbiology: developments and challenges in detection of bacterial, fungal, and viral contaminants. Compr. Rev. Food Sci. Food Saf. 21, 3717–3745. doi: 10.1111/1541-4337.12983
Sun, Q., Zhang, M., and Mujumdar, A. S. (2019). Recent developments of artificial intelligence in drying of fresh food: a review. Crit. Rev. Food Sci. Nutr. 59, 2258–2275. doi: 10.1080/10408398.2018.1446900
Takruri, M., Abubakar, A., Alnaqbi, N., Al Shehhi, H., Jallad, A. H. M., and Bermak, A. (2020). DoFP-ML: a machine learning approach to food quality monitoring using a DoFP polarization image sensor. IEEE Access 8, 150282–150290. doi: 10.1109/ACCESS.2020.3016904
Tang, Y., Bai, H., Sun, L., Wang, Y., Hou, J., Huo, Y., et al. (2022). Multi-band-image based detection of apple surface defect using machine vision and deep learning. Horticulturae 8:666. doi: 10.3390/horticulturae8070666
Thinh, N. T., Thong, N. D., Cong, H. T., and Phong, N. T. T. (2019). Mango classification system based on machine vision and artificial intelligence. In 2019 7th international conference on control, mechatronics and automation (ICCMA) (pp. 475–482). IEEE.
Toffali, K., Zamboni, A., Anesi, A., Stocchero, M., Pezzotti, M., Levi, M., et al. (2011). Novel aspects of grape berry ripening and post-harvest withering revealed by untargeted LC-ESI-MS metabolomics analysis. Metabolomics 7, 424–436. doi: 10.1007/s11306-010-0259-y
Tsang, Y. P., Choy, K. L., Wu, C. H., Ho, G. T., Lam, C. H., and Koo, P. S. (2018). An internet of things (IoT)-based risk monitoring system for managing cold supply chain risks. Ind. Manag. Data Syst. 118, 1432–1462. doi: 10.1108/IMDS-09-2017-0384
Tsugawa, H., Tsujimoto, Y., Arita, M., Bamba, T., and Fukusaki, E. (2011). GC/MS based metabolomics: development of a data mining system for metabolite identification by using soft independent modeling of class analogy (SIMCA). BMC Bioinformatics 12, 1–13. doi: 10.1186/1471-2105-12-131
van de Looverbosch, T., Rahman Bhuiyan, M. H., Verboven, P., Dierick, M., van Loo, D., de Beenbouwer, J., et al. (2020). Nondestructive internal quality inspection of pear fruit by X-ray CT using machine learning. Food Control 113:107170. doi: 10.1016/j.foodcont.2020.107170
Van Eck, N., and Waltman, L. (2010). Software survey: VOSviewer, a computer program for bibliometric mapping. Scientometrics 84, 523–538. doi: 10.1007/s11192-009-0146-3
Vélez Rivera, N., Gómez-Sanchis, J., Chanona-Pérez, J., Carrasco, J. J., Millán-Giraldo, M., Lorente, D., et al. (2014). Early detection of mechanical damage in mango using NIR hyperspectral images and machine learning. Biosyst. Eng. 122, 91–98. doi: 10.1016/j.biosystemseng.2014.03.009
Villa-Gonzalez, F., Bhattacharyya, R., Athauda, T., Sarma, S., and Karmakar, N. (2022). Detecting breaks in cold chain integrity using Chipless RFID time-temperature sensors. IEEE Sensors J. 22, 17808–17818. doi: 10.1109/JSEN.2022.3194249
Wang, X., Bouzembrak, Y., Lansink, A. O., and van der Fels-Klerx, H. J. (2022). Application of machine learning to the monitoring and prediction of food safety: a review. Compr. Rev. Food Sci. Food Saf. 21, 416–434. doi: 10.1111/1541-4337.12868
Wang, H., He, H., Liu, L., Ma, H., Liu, X., Mo, H., et al. (2019). Recent progress in hyperspectral imaging for nondestructive evaluation of fish quality. Shipin Kexue/Food Sci 40, 329–338. doi: 10.7506/spkx1002-6630-20180129-392
Wang, R. L., Hsu, T. F., and Hu, C. Z. (2021). A bibliometric study of research topics and sustainability of packaging in the greater China region. Sustainability 13:5384. doi: 10.3390/su13105384
Wang, L., Kwok, S. K., and Ip, W. H. (2010). A radio frequency identification and sensor-based system for the transportation of food. J. Food Eng. 101, 120–129. doi: 10.1016/j.jfoodeng.2010.06.020
Wieme, J., Mollazade, K., Malounas, I., Zude-Sasse, M., Zhao, M., Gowen, A., et al. (2022). Application of hyperspectral imaging systems and artificial intelligence for quality assessment of fruit, vegetables and mushrooms: a review. Biosyst. Eng. 222, 156–176. doi: 10.1016/j.biosystemseng.2022.07.013
Xiao, X., He, Q., Li, Z., Antoce, A. O., and Zhang, X. (2017). Improving traceability and transparency of table grapes cold chain logistics by integrating WSN and correlation analysis. Food Control 73, 1556–1563. doi: 10.1016/j.foodcont.2016.11.019
Yang, M., Kumar, P., Bhola, J., and Shabaz, M. (2022). Development of image recognition software based on artificial intelligence algorithm for the efficient sorting of apple fruit. Int J Syst Assur Engineer Manage 13, 322–330. doi: 10.1007/s13198-021-01415-1
Yang, B., and Xu, Y. (2021). Applications of deep-learning approaches in horticultural research: a review. Horticult Res 8:123. doi: 10.1038/s41438-021-00560-9
Yu, X., Lu, H., and Wu, D. (2018). Development of deep learning method for predicting firmness and soluble solid content of postharvest Korla fragrant pear using Vis/NIR hyperspectral reflectance imaging. Postharvest Biol. Technol. 141, 39–49. doi: 10.1016/j.postharvbio.2018.02.013
Zhai, Z., Martínez, J. F., Beltran, V., and Martínez, N. L. (2020). Decision support systems for agriculture 4.0: survey and challenges. Comput. Electron. Agric. 170:105256. doi: 10.1016/j.compag.2020.105256
Zhang, W., Hu, J., Zhou, G., and He, M. (2020). Detection of apple defects based on the FCM-NPGA and a multivariate image analysis. IEEE Access 8, 38833–38845. doi: 10.1109/ACCESS.2020.2974262
Zhong, Q., Wang, L., and Cui, S. (2021). Urban food systems: a bibliometric review from 1991 to 2020. Foods 10:662. doi: 10.3390/foods10030662
Zhou, L., Zhang, C., Liu, F., Qiu, Z., and He, Y. (2019). Application of deep learning in food: a review. Compr. Rev. Food Sci. Food Saf. 18, 1793–1811. doi: 10.1111/1541-4337.12492
Keywords: artificial intelligence, postharvest technology, machine learning, deep learning, food quality
Citation: Fadiji T, Bokaba T, Fawole OA and Twinomurinzi H (2023) Artificial intelligence in postharvest agriculture: mapping a research agenda. Front. Sustain. Food Syst. 7:1226583. doi: 10.3389/fsufs.2023.1226583
Received: 21 May 2023; Accepted: 14 August 2023;
Published: 26 September 2023.
Edited by:
Samuel Ayofemi Olalekan Adeyeye, Hindustan University, IndiaReviewed by:
Tomasz Jakubowski, University of Agriculture in Krakow, PolandCopyright © 2023 Fadiji, Bokaba, Fawole and Twinomurinzi. This is an open-access article distributed under the terms of the Creative Commons Attribution License (CC BY). The use, distribution or reproduction in other forums is permitted, provided the original author(s) and the copyright owner(s) are credited and that the original publication in this journal is cited, in accordance with accepted academic practice. No use, distribution or reproduction is permitted which does not comply with these terms.
*Correspondence: Tobi Fadiji, dG9iaWZAdWouYWMuemE=; dGZhZGlqaUB1ai5hYy56YQ==
Disclaimer: All claims expressed in this article are solely those of the authors and do not necessarily represent those of their affiliated organizations, or those of the publisher, the editors and the reviewers. Any product that may be evaluated in this article or claim that may be made by its manufacturer is not guaranteed or endorsed by the publisher.
Research integrity at Frontiers
Learn more about the work of our research integrity team to safeguard the quality of each article we publish.