- 1Department of Agronomy, Kansas State University, Manhattan, KS, United States
- 2Department of Geographical Sciences, University of Maryland, Baltimore, MD, United States
- 3Department of Biosystems and Agricultural Engineering, Michigan State University, East Lansing, MI, United States
- 4Sustainable Intensification Innovation Lab, Kansas State University, Manhattan, KS, United States
Introduction: The lack of a holistic view of agriculture’s social and economic aspects emerges as a limiting factor in policy implementations of sustainable intensification. The aim of this study was to (i) synthesize data from the five domains of sustainable agriculture intensification framework focusing on Senegal as a case study, (ii) harmonize and scale data used to minimize spatial–temporal differences, and (iii) build a digital tool aiming researchers and policymakers to facilitate the data visualization and connection.
Methods: We propose and prototype an interactive digital decision-support tool as a potential solution to integrate environmental, social, economic, human and production domains in agriculture projects. To demonstrate the use of the tool, a case study using data from Senegal was developed to show the benefits of open science and connectivity among domains.
Results and discussion: The digital tool presented here allows users to explore an open repository from Senegal serving as a foundational approach to integrate diverse agricultural domains when developing sustainable intensification projects.
1. Introduction
Data availability in agriculture has expanded in recent decades as a result of the increased accessibility of remote sensing datasets, ubiquitous connectivity (Coble et al., 2018; Klerkx et al., 2019), production of scientific research (Bornmann and Mutz, 2015), and the open science data movement (Jetzek et al., 2014). The transition from data to actionable information for management, planning, and assessments presents significant challenges, especially for sustainable agriculture intensification (SAI) projects, where information from multiple domains is required (Musumba et al., 2017; Stewart et al., 2018; Klerkx et al., 2019). Challenges to developing usable data products include (i) the lack of multi-level perspective on transitions with social practice (Jakku et al., 2019), (ii) the lack of standard protocols to translate research outcomes to effective policies (Therond et al., 2009), (iii) mismatch between planned objectives and obtained benefits (Zuiderwijk et al., 2019), (iv) ethical issues such as privacy and data ownership (Rotz et al., 2019; Runck et al., 2022), (v) difficulties to harmonize data in different temporal and spatial scales (Zipkin et al., 2021), and (vi) lack of ground truthing data to validate regional outcomes (Saltelli et al., 2020). Furthermore, these challenges are exacerbated in Africa due to institutional, infrastructure, and social issues, restricting effective and long-term adoption of policies focusing on interventions (Wyche and Steinfield, 2016; Baumüller, 2018). The lack of long-term adoption highlights the overall fragility and the variable impacts of supporting vulnerable low-income settings on effective data use to implement agricultural innovations (Gurstein, 2011). In this context, developing digital tools that facilitate access, integration, visualization, and improved understanding of the available data can potentially support researchers and policymakers when designing SAI interventions, overcoming these challenges.
The absence of a holistic view of agriculture’s social and economic aspects is a main limitation of policy implementation for SAI (Birol et al., 2015). A recent study showed the narrow focus of agricultural research on productive outcomes, rarely engaging with social sciences (Brown et al., 2023). Despite the impressive advancements made in agricultural technologies, these are often derived from reductionist approaches (Siddique et al., 2012), lacking insights into the relevance of the techniques or technologies to livelihood improvements (Saltelli et al., 2020) and understanding how sustainable intensification is perceived by farmers, especially in low-income settings (Di Prima et al., 2022). A more robust alignment between agronomists and social science research communities is needed to create effective food system investments (Yaro, 2006). To address this problem, Musumba et al. (2017) and Stewart et al. (2018) proposed a framework for assessing agricultural innovations considering five domains (productivity, economic, environment, human condition, and social). Research needs to be interdisciplinary to develop innovations with a balance across domains, yet the lack of integration is conspicuous in agricultural research (Daraio et al., 2016). A major limitation beyond the lack of interlinkages among disciplines is the insufficient and slow knowledge transfer between scientists and end users (Rose et al., 2016), with the clear need for simple and relevant decision support tools that can facilitate adoption among relevant stakeholders.
Digital interactive decision support tools (hereafter “digital tools”) are a way to overcome the lack of integration across SAI domains in agricultural programs (Jones and Taylor, 2004) because of their capacity to integrate research with real applications (Shim et al., 2002; Rose et al., 2016). Several examples can be found in diverse fields, such as medicine, logistics, forecast, and agriculture (Ngo et al., 2020; Santos et al., 2020; Coelho et al., 2021; Laurent et al., 2021). Furthermore, digital tools can also integrate knowledge among different areas (González Rodríguez et al., 2020), and support the planning and assessment of interventions (Himesh et al., 2018). Focusing on these last points, Neufeldt et al. (2013) suggested that a systematic gathering and integration of data, overcoming spatial and temporal dimensions differences, is needed to plan agricultural interventions to support the food security agenda. More recently, and from the agriculture side, a few digital tools were generated in a relevant effort to demonstrate a potential path forward on moving from research outcomes to actionable decisions (e.g., Laurent et al., 2021; Correndo et al., 2022). Therefore, as demonstrated by recent agricultural developments, using digital tools reduces the effort and time needed to overcome the challenges to planning agricultural interventions (McCampbell et al., 2021) and potentially increases adoption.
Following the creation of the Data for Decisions to Expand Nutrition Transformation (DataDENT), several digital visualization tools aiming to display nutritional information were done (Manorat et al., 2019). Another example of digital tools in human nutrition is the Food Systems Dashboard, that compiles, transforms and displays data from various sources and drivers for national, regional, and subnational level (Fanzo et al., 2020). However, none of these tools are capable of integrating data from different domains described in the Sustainable Intensification Assessment Framework (SIAF) (Musumba et al., 2017; Stewart et al., 2018) and some of them do not display data at the sub-national level.
Following this rationale, this study presents the design of a digital tool to facilitate the access and integration of data from different domains as described in the Sustainable Intensification Assessment Framework (SIAF) (Musumba et al., 2017; Stewart et al., 2018). Understanding these needs, the aim of this study was to focus and use Senegal as a case study to (i) synthesize data from the five domains (productivity, economic, environment, human condition, and social), (ii) harmonize and scale data used to minimize spatial–temporal differences, (iii) build a digital tool aiming researchers and policymakers to facilitate or guide the data visualization and connection, and (iv) elaborate a study-case connecting the data from different domains from the repository to demonstrate the application of the tool.
2. Materials and methods
2.1. Data description and acquisition
Open and accessible data (Table 1) was employed to summarize indicators from the five domains (social, economic, environment, human, and production) described by Musumba et al. (2017) and Stewart et al. (2018). The data were selected based both on availability (be open and accessible) and relevance to the five domains presented in the Sustainable Intensification Assessment Framework (SIAF). Musumba et al. (2017) developed a full list of indicators in combination of exploration of the literature and interaction with scientists from the following organizations Africa RISING project and Sustainable Intensification Innovation Lab (SIIL), the Consortium of Improving Agriculture-based Livelihoods in Central Africa (CIALCA), among the most relevant institutions providing feedback.
Indicators of the environmental domain (soil moisture index, water demand index, vegetation index and precipitation, growing degree days) were in a gridded format, obtained from Banda et al. (2023). The Supplementary material provides the link for this repository. The variables were averaged, resulting in one value per district per year. Precipitation was presented as the total accumulation during the year and obtained from Climate Hazards Group InfraRed Precipitation with Station data (CHIRPS, Funk et al., 2015).
All indicators from the agricultural production domain were obtained from Direction de l’Analyse, de la Prévision et des Statistiques Agricoles—DAPSA (n.d.); https://www.dapsa.gouv.sn/. This information was originally provided at annual and district scales; therefore, no further processing was required.
Regarding the indicators from the economic domain, two sources were employed: DAPSA and the Demographic and Health Surveys Thee D. H. S., 2021; https://dhsprogram.com/. The economic data provided by DAPSA consisted of the prices registered at the local markets and the prices reported by the farmers for several crops. This information was obtained at the district spatial scale and monthly temporal scale. The methodology presented in Brown et al. (2017) was used to calculate the average trend over the year for representing prices on a yearly scale. The DHS data was obtained from the Senegal Continuous Survey Report; thus, one value per year was obtained from 2015 to 2019, and the spatial resolution was district or region. To downscale the data from region to district level, the reported value for each district in the region was considered.
The social domain included the same sources as the economic domain: DAPSA and DHS. For the social domain, the DHS data was obtained from the 2015 to 2020 Senegal DHS Continuous Survey Dataset reports, obtaining one value per year. This data is reported in different scales, such as individuals and households. In this analysis, the household level data was utilized and aggregated to the district level. This was done by computing a weighted average of the samples within each district using the sample weighting factor provided by DHS. As detailed in the DHS Standard Recode Manual ICF (2018), all sample weights are normalized such that the weighted number of cases is identical to the unweighted number of households when using the full dataset with no selection.
Finally, all the indicators of the human domain were retrieved from the DHS, Senegal Continuous Survey Report, as explained above for the economic indicators.
2.2. Tool description and technical details
The tool (available at: https://ciampittilab.shinyapps.io/SIAF/)was developed using the R Shiny framework (Chang et al., 2023) and markdown format (Rmd) utilizing flexdashboard R package (Sievert et al., 2023). The tool is currently hosted on shinyapps.io platform, Shiny is a free build framework and allows users to manage interactions and stylization. Changes in Cascading Style Sheets (CSS) were made inside the Rmd file to develop the styles and build a user-friendly tool. The main packages included in the code of the tool are dplyr (Wickham et al., 2023), ggplot2 (Wickham, 2016), and plotly (Inc. P. T., 2015). The dplyr package (Wickham et al., 2023) is responsible for filtering the data according to users’ selections, and ggplot2 (Wickham, 2016) and plotly (Inc. P. T., 2015) packages are responsible for generating the charts from the filtered data.
Figure 1 illustrates the working flow of the development of the digital tool of this study. The orange dashed box indicates the steps manually made, such as the data collection and its summarization to district and year scales of different databases, as described in section 2.1. The blue dashed box indicates the processes occurring in the digital tool, which works by filtering the data from user selection based on desired parameters. After the desired parameters are selected, the chosen chart is plotted. The code and technical details used to develop this tool can be found in this open GitHub repository: Data Integration Dashboard1; also, the code is available in the Supplementary material.
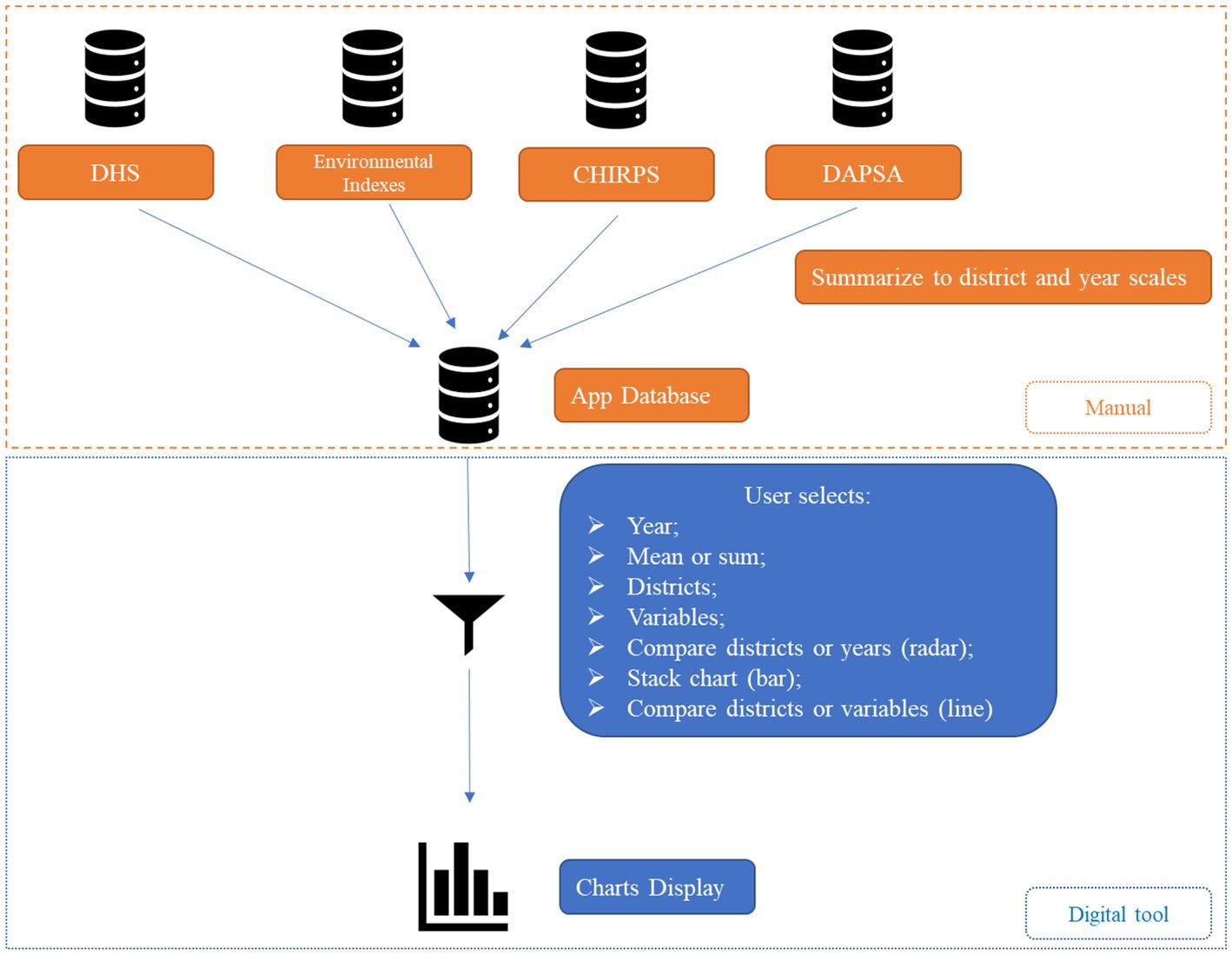
Figure 1. Development and digital tool working flow diagram. The orange dashed box indicates the manually made steps, where data from different databases were gathered and summarized on district and year scales, generating a database embeded in the digital tool. The blue dashed box indicates the processes occurring in the digital tool, where the database is filteried according to users’ selections and the output is displayed in charts.
The tool is divided into five navigation bars: Instructions, Visualization, Data, About and Data Source. The Instructions host the tutorial video on the utilization of the tool. After consulting with professor, students and staff during an informal process, we designed the data visualizations available in this digital tool, which are divided into six navigation tabs within the Visualizations navigation bar: map, Sustainable Intensification Assessment Framework (SIAF) plot, correlation matrix, line chart, bar chart and, box chart. The SIAF was proposed by Musumba et al. (2017) and improved web version by Stewart et al. (2018) and available at www.siltoolkit.com. Even with the existence of this web version of the SIAF, its usage can be complicated, requiring an interactive and visual tool to facilitate the interpretation of the data. This tool helpes to evaluate the holistic performance and look critically at trade-offs and synergies of innovations, analyzing the impacts through all five domains (social, production, economic, human, and environment). It can be used by researchers, policy makers, donors, and extension agents to identify the gaps and determine opportunities for interventions.
2.2.1. Visualization
The first navigation tab on the Visualization navigation bar is Map (Figure 1). It is a choropleth map of the different districts of Senegal made by a filtering process of the database after user selection of data, the interval between years, and the option to calculate the sum or mean of those selected year(s). The leaflet package for R programming (Cheng et al., 2022) was utilized to render the maps. The choropleth map allows the users to visualize the spatial distribution of a variable.
The SIAF navigation tab permits users to compare between districts or compare between years within one district by displaying a radar graph executed using the R package ggradar (Bion, 2023). In both cases, the variables are scaled using the scales package (Wickham and Seidel, 2022). This tab allows users to integrate data from different domains. In the correlation matrix navigation tab, users can generate a correlation matrix from ggcorplot package (Wei and Simko, 2021). Users can select a district, years, and variables, all filtered according to users’ choices. Using the correlation matrix, users can visualize the presence or absence of correlation between data of different domains. The line chart navigation tab (Figure 2) allows users a temporal visualization of variables in different districts or variables in one district. In the second case, the variables were presented using the scales package (Wickham and Seidel, 2022) since they were not in the same unit. The presence of a line chart in the tool allows users to visualize different variables over time.
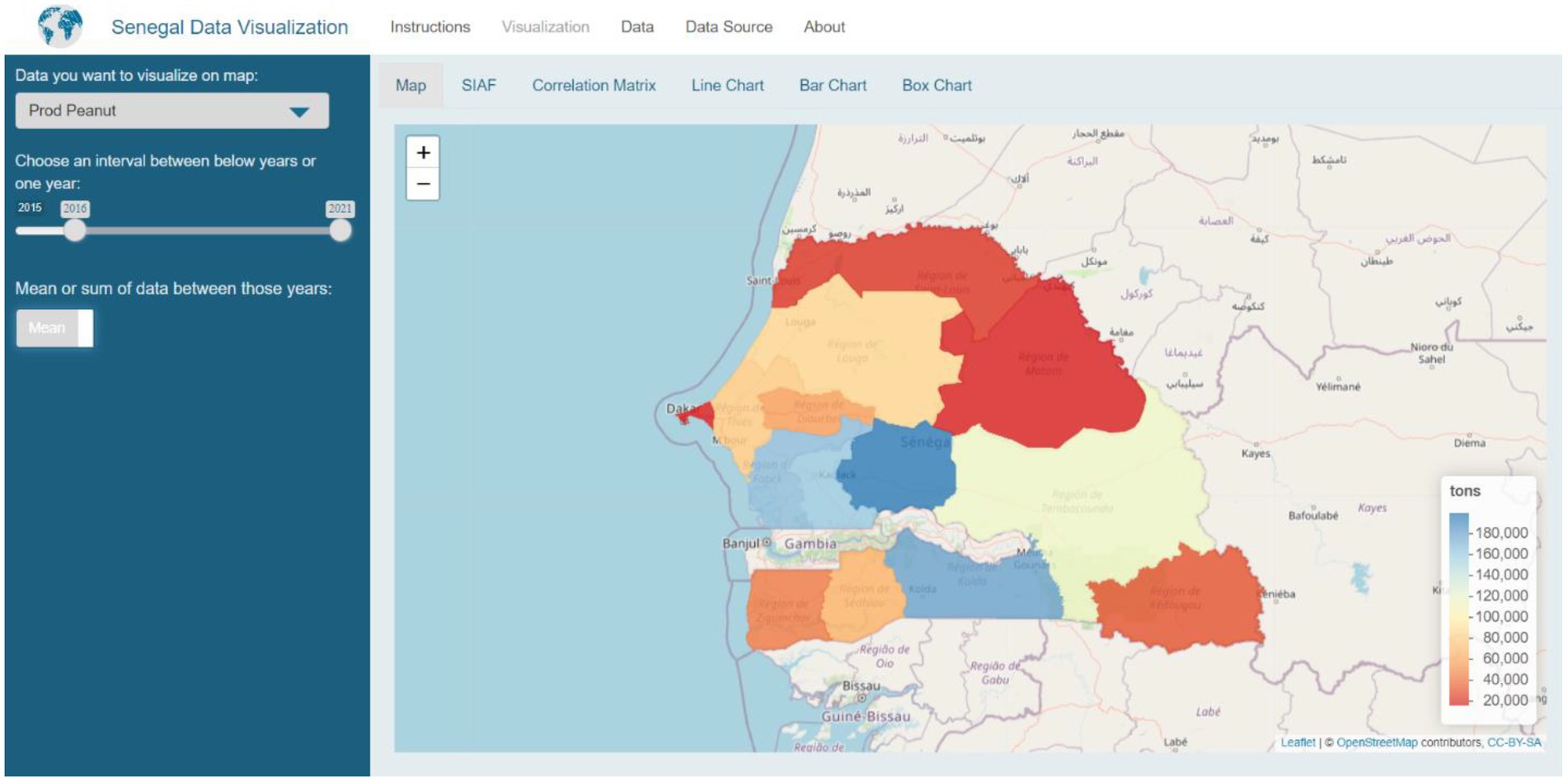
Figure 2. Navigation tab of map, displaying for this specific example a choropleth map of the mean of peanut production from 2016 to 2021 years at the district-level from Senegal. On the sidebar on the left, users can filter what will be displayed on the map. Above the map are the navigation tabs, providing users the possibility to access the different tool resources.
The bar chart (Figure 3) allows users to select places, year intervals, and the type of aggregation (mean or sum) to be displayed. Additionally, users can choose to stack the bars and select the variables to be shown. After data filtering, if the stacked option is selected, variable values are scaled using the scales package (Wickham and Seidel, 2022). The bar chart enables users to compare districts and the numerical values of variables between districts. The last option on the Visualization navigation bar is the box chart. Users select districts, years as repetitions, and variables. The boxplot in this tool allows users to see data distribution, outliers, and quartiles in selected years for variables selected previously (see Figure 4).
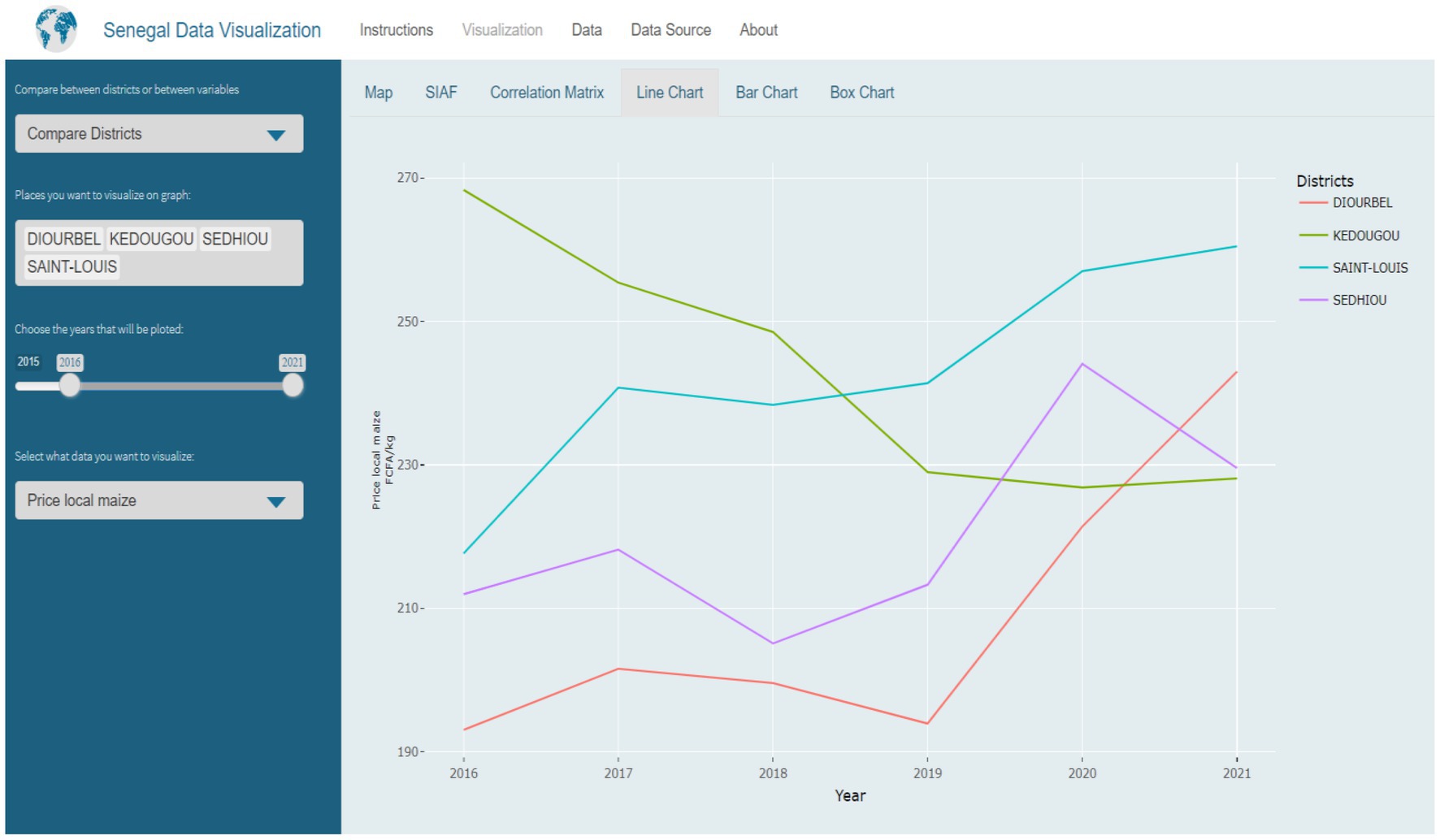
Figure 3. Line charts made in the tool display a comparison among Diourbel, Kedougou, Sedhiou, and Saint-Louis districts for local price of maize from 2016 to 2021. On the sidebar on the left, users can filter and select what will be displayed on the line chart, including the possibility to compare variables in a district or a variable between districts. Above the graph, are the navigation tabs, providing users the possibility to access the different tool resources.
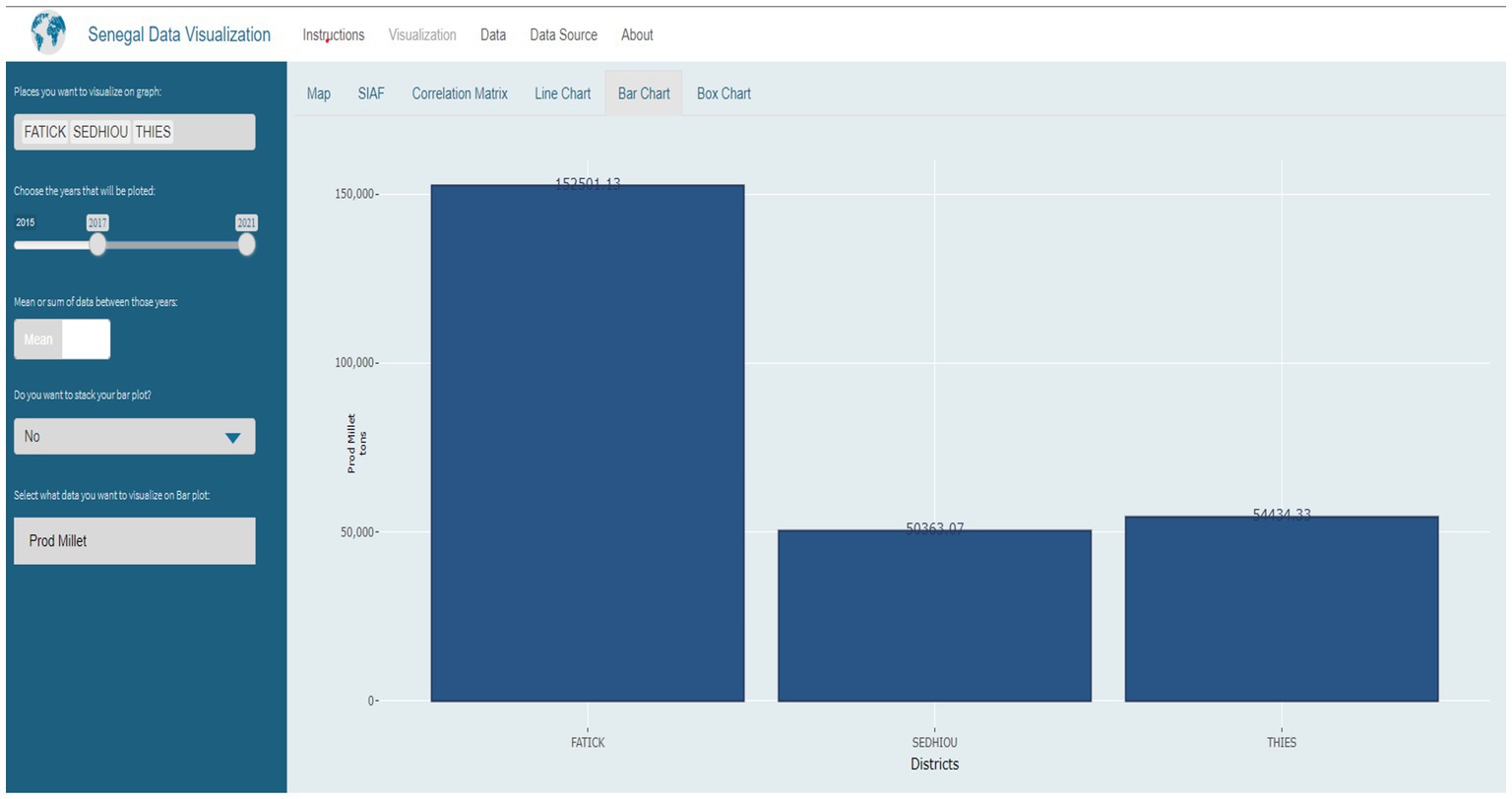
Figure 4. Bar chart made using the tool demonstrating the comparison of the mean values of millet produced at Thies, Fathick and Sedhiou from 2017 to 2021 with bars not stacked. On the sidebar on the left, users can filter and select what will be displayed on the bar chart, including the options to display the mean or sum of selected years and to stack the chart. Above the graph, are the navigation tabs, providing users the possibility to access the different tool resources.
2.2.2. Instructions, data, about and data source
The Instructions section is the home page of the tool. A tutorial video provides guidance to users on how to utilize the tool described in this study. All usage of the navigation bars and options was recorded, and subtitles in English were included to increase the tool’s accessibility to a wider range of users. The video is hosted on YouTube2 and embedded in the tool. In the data section, all data gathered and used in the tool is displayed using the DT package (Xie et al., 2023). This package enables users to search for specific values and names in the table, select entries, and identify data gaps for districts, years, and variables. The last navigation bar is the Data Source, where using the DT package (Xie et al., 2023). Here users can visualize a table containing the domains, metric, abbreviation, source, units, the methodology used to get data for each variable, the original temporal and spatial scales and the year range of collection. Both sections have a button at the top, beneath the title, which allows users to download the table with a single click.
The utilization of this interactive web tool by researchers and policymakers will allow them to guide new research, develop more focused hypotheses, and set direction for future studies. For example, questions such as if the inclusion of legume crops can enhance productivity, resiliency, profitability, environmental sustainability and produce economic/social/human impacts can be analyzed and interpreted first by understanding the current data available on this topic and potential indicators included in the different domains. If data is available, the study of the behavior of a similar indicator under different environments can present new insights on the major factor behind this trend and direct new efforts on studying this. In overall, many different scenarios could be evaluated to more effectively guide new interventions, refine research questions, and implement new studies on the ground to obtain key responses to issues faced by the community. The policymakers can evaluate the potential actions addressed by researchers to assess the effectiveness of the interventions from the perspective of the sustainable intensification agricultural process.
3. Case-study
To display this digital interactive tool in action, a case study is demonstrated focusing on two districts for the peanut-millet basin region, Kaolack and Kaffrine. Our main focus is to demonstrate the integration of different domains of the SIAF (selecting some variables considered more relevant from production, human and social) and the potential of this visualization tool to provide new insights into the current data.
As a first step, the user can go to the Map section of the tool and select the two most productive districts (in tons) of both millet and peanut (Figure 5A). As a second step, focusing on the Kaolack and Kaffrine districts as the largest producers of these crops, we can explore the differences in mortality of children between one and five years old from 2015 to 2019 using the line chart for the two districts. The analysis of juvenile mortality employing a line chart (Figure 5B) shows a decreasing rate for both districts during this period. Employing the Box Chart tab shows that despite having similar average production, Kaffrine presented more variation in the percentage of households with lower incomes (Figure 5C) and had less rainfall relative to Kaolack.
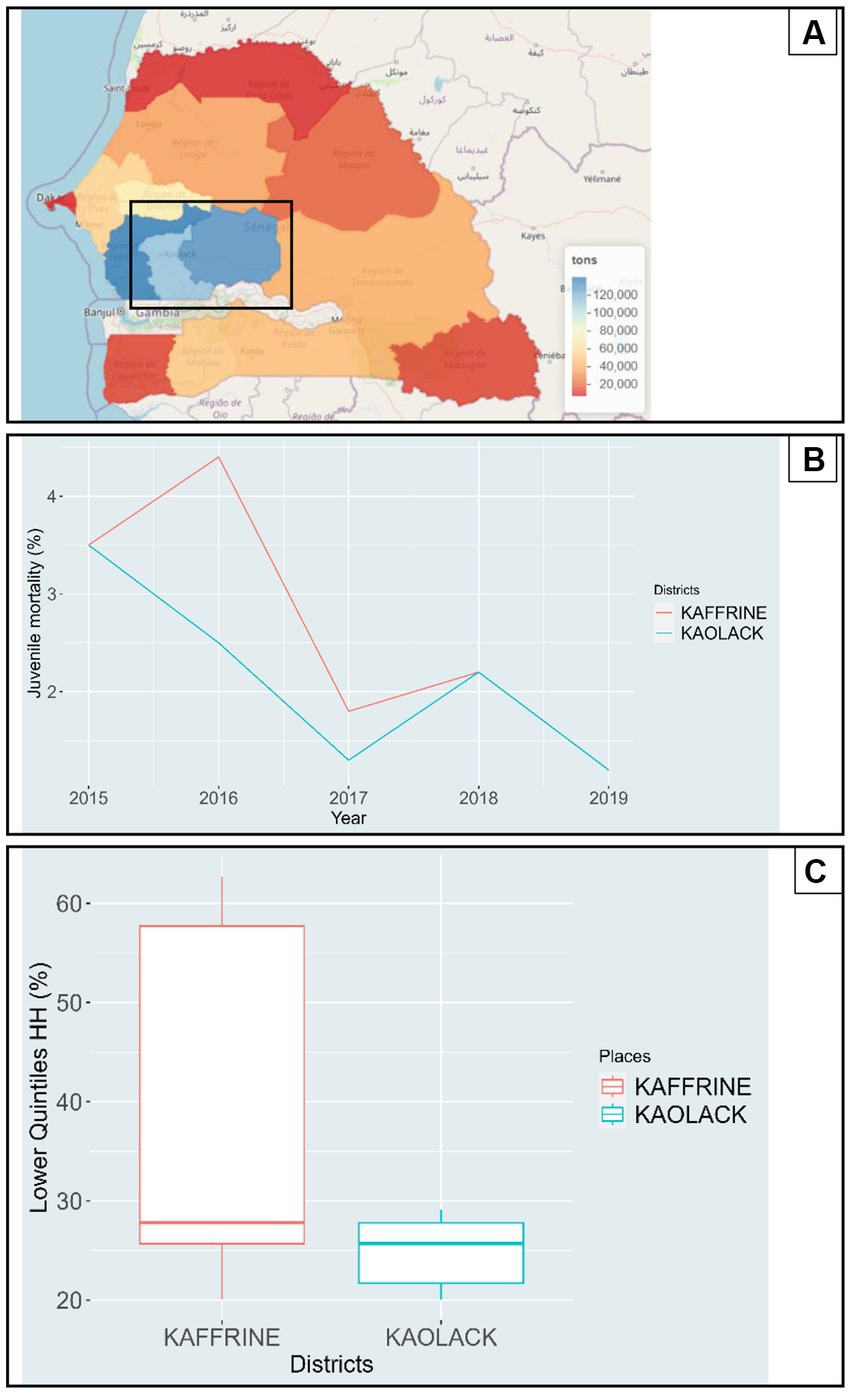
Figure 5. (A) Choropleth map of average millet production in Senegal from 2015 to 2021. Inside the black rectangle are the districts of Kaolack and Kaffrine (left and right respectively). (B) Line chart of juvenile mortality of Kaffrine and Kaolack districts from 2015 to 2019, showing a decrease of this index during those years in the selected district. (C) Box chart of percentage of households from the lower quintile of Kaolack and Kaffrine districts from 2015 to 2021, presenting that Kaffrine district had a higher variation of the index.
A correlation matrix was employed to visualize the correlations of annual variations from different domains at the district level for both Kaolack and Kaffrine (Figures 6A,B). This analysis showed a strong correlation between the annual variability of millet production, wealth factor, juvenile mortality, and stunting less than minus 2 standard deviation in the district of Kaffrine (Figure 6A). Meanwhile, in the Kaolack district (Figure 6B), the annual variability of millet production presented a strong correlation with the percentage of households in the lower quintile, child-juvenile, and juvenile mortality. Furthermore, the juvenile mortality for the Kaolack district showed a stronger correlation with the local millet price than with the millet production itself, with mortality increasing as prices rose. The variables with strong correlations were included on a radar chart to easily compare across domains, following the framework proposed by Musumba et al. (2017) and Stewart et al. (2018). On the radar chart (Figure 6C), it is possible to see that the Kaffrine district has higher juvenile mortality, higher levels of poverty, and stunting prevalence. In contrast, Kaolack has higher millet production and electricity availability, indicating higher levels of development (Ezeoha et al., 2020).
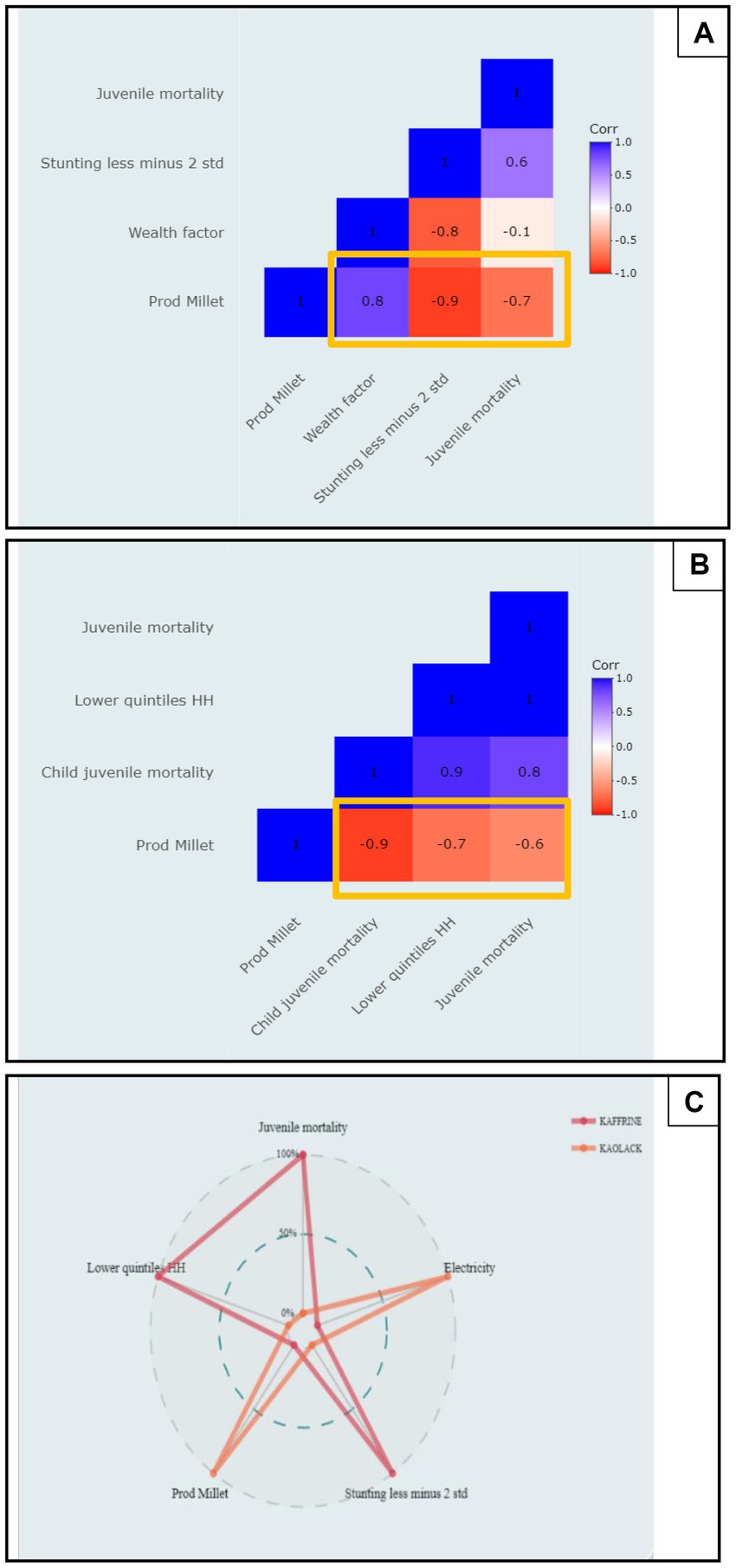
Figure 6. (A) Correlation matrix of Kaolack district from 2016 to 2019. The yellow box highlights the strong correlations between millet production and child juvenile mortality, households in the lower quintile and juvenile mortality. (B) Correlation matrix of Kaolack district from 2016 to 2019. The yellow box highlights the strong correlations between millet production and wealth factor, stunting less −2 standard deviation and juvenile mortality. (C) Radar chart of Kaolack and Kaffrine districts from 2016 to 2019 of juvenile mortality, millet production, households of the lower quintile, electricity and stunting less than −2. In the comparation, Kaolack presented higher values of millet production and electricity and lower values of juvenile mortality, households of the lower quintile and stunting less than −2.
In summary, this case study demonstrated how the tool allows for rapid visualization of multiple drivers that cause greater variability for food production at Kaffrine relative to Kaolack and the overall difference in wealth and resource availability. A diagram summarizing the case study is presented in Figure 7. Furthermore, interventions that focus on improving the monitoring of crop prices and providing social safety nets may have a greater impact on food accessibility and child mortality at the smallholder community level compared to interventions aimed solely at providing agricultural inputs. If the focus is on productivity, these two districts are similar. However, potential challenges linked to crop prices and juvenile mortality were evident when the social and human domains were considered.
Another flow would be required for a policymaker to use the digital tool. For example, policymakers interested in the Kaolack district can explore different indicators based on their interest. In a hypothetical scenario, employing the line chart tool to explore different human health indicators, the policymaker might notice that child mortality increased in the last five years. Then, the next step would be exploring additional variables using the correlation matrix. In this case study, child mortality might have been related to a decline in millet or peanut production. Thus, policymakers will be able to analyze, integrate, and compare variables of different domains in a district at a specific time. Musumba et al. (2017) provided full details in a case study of application of this framework in Malawi with the main goal of increasing the use of legumes compared to continuous maize and all the implications from a sustainability standpoint, enhancing diversification, including high nutritional value crops for improving human conditions, and promoting conservations of natural resources via extension of ground cover and addition of nitrogen via fixation. This is only one of the potential uses of this interactive for assessing and planning future interventions.
4. Discussion
This digital interactive decision tool is one of the first attempts to summarize, integrate, and provide an opportunity for fostering research and integration across multiple domains in sustainable agricultural research. Open science accelerates new knowledge by making current research datasets more accessible (easily discoverable), reliable, and adaptive to changes (Burgelman et al., 2019). Relevant data is either not available or does not exist due to policies, privacy, and security issues, which reduces the transmission of knowledge regarding sustainable agriculture intensification (Runck et al., 2022). The relevance of the present study is encompassed by the data gathered for current open sources integrated into one visualization tool, with all parameters presented in comparable scales for easy assessment and in an interface that facilitates visualization. Furthermore, the methodology followed to establish this digital tool is provided in this document, favoring the transparency of the process (García-Peñalvo et al., 2010). This digital tool provides a foundational framework that can be easily deployed to other countries/regions around the globe.
The development of relevant digital tools may help policymakers and researchers to overcome food security in low and middle-income countries, as it allows the access, process, and visualization of available data (Cotter et al., 2020). Digital tools are becoming common for diverse purposes, such as decision support, data visualization, and manipulation (Turner et al., 2015; Avazpour et al., 2019; Ramalho and Segundo, 2020). Previous efforts developed tools to facilitate data visualization. For example, the National Aeronautics and Space Administration (NASA) generated several tools to access environmental information [Land, Atmosphere Near real-time Capability for EOS (LANCE) Earth Science Data Systems, 2021] and satellite images for vegetation indexes calculations (Turner et al., 2015; The Early Warning Explorer (EWX), (n.d.)). This digital tool provides relevant data for the environment and a proxy of production, leaving behind human, economic, and social features. Furthermore, these sources are deployed in different temporal and spatial scales, complexifying their integration (Avazpour et al., 2019).
Different solutions have been provided to address the problem of data in different scales (Kharel et al., 2020). Within these options, Harmonizer+ is a framework focused on generating a harmonization and visual display of integrated data (Avazpour et al., 2019). Frameworks such as Harmonizer+, provide the methods, but do not have data embedded in them, requiring the user to provide the data in a less user-friendly interface. However, to our knowledge, no tools integrating data from different domains, overcoming scale challenges, and acting as an open repository, are available.
The use of digital tools to integrate and visualize open datasets can facilitate the discovery of potential links between agricultural productivity, economics, and the environment with demographic and human problems (Brown et al., 2023). The tool presented in this study can display data and provide the outcomes in an open repository with comparable spatial and temporal scales from different domains. Consequently, outcomes from this digital tool can provide new insights to researchers and policymakers to have a holistic and transdisciplinary view from diverse domains when assessing interventions and planning future studies (Neufeldt et al., 2013). Furthermore, open data sources support policies and scientific advances, promote transparency and guarantee the accountability of scientific results (Harrison and Sayogo, 2014). Furthermore, open data accelerates scientific progress as it reduces the time needed to gather the necessary information (Lowndes et al., 2017). Finally, these features should be encompassed within an easy-to-work interface to have a perceptible impact during the tool usage (Hartkamp et al., 1999). Another example is the Food Systems Dashboard (The Food Systems Dashboard, 2023), which contains indicators covering several domains, and is divided into drivers, food supply chains, food environments, individual factors, and outcomes at national level worldwide. It also includes a section on Policies and Actions, indicating impacts derived from actions implemented by policymakers. However, one limitation of this tool is the spatial scale, as it does not cover subnational levels. Therefore, the tool presented in this study solves two key challenges for agricultural systems production presented by Antle et al. (2017): moving from a research-only data into visualization and developing a user-friendly tool.
The main limitations encountered while working on the development of this digital tool include (i) the lack of standard protocols for reporting comparable data variables, (ii) the lack of open data, and the data that is available is in multiple and unconnected web repositories, (iii) the lack of universally accepted harmonization protocols, (iv) several bureaucratic barriers for data accessibility, (v) data that focus only on a few domains (Brown et al., 2023), (vi) the lack of data for analyzing outcomes, and (vii) lack of open and accessible input datasets to obtain more indicators (e.g., related to animal husbandry), and (viii) completeness of existent datasets even when focused on a few domains, several critical indicators are usually missing to provide a more holistic view of the sustainability of agricultural systems. A major element of the solution for these issues is to encourage open science and the development and release of data that allows for analysis across social, economic, political, and biophysical domains so that the solutions identified are put into context.
Crüwell et al. (2019) listed crucial steps to move open science forward, which include open access, open repositories with data and codes for reproducible analyses, and training our next generation of scientists on the importance of this topic. Furthermore, efforts are being made to facilitate the management of repositories for usage in digital tools (Wickham and Müller, 2022). These efforts should be translated into other areas to support policymakers and researchers in planning agricultural interventions that consider a holistic view of the implementation context (Pandey et al., 2016). Beyond these limitations, the data visualization tool presented here can be further enhanced. The next steps are to integrate new datasets, incorporate new research, and provide scenarios such as future weather extremes and population increase rates, and for alternative interventions like farming strategies adoption or application of environmental policies, based on the data visualization.
5. Conclusion
The digital tool presented here allows users to explore an open repository of SAI domains from Senegal, allowing easy visualization and connection in harmonized data. All methods and protocols to collect, synthesize, and process data are open to users. Therefore, the digital interactive tool can serve as a foundational approach to developing next generation decision support tools to integrate diverse agricultural domains for sustainable agriculture intensification and provide visualization of large complex datasets.
Data availability statement
The datasets presented in this study can be found in online repositories. The names of the repository/repositories and accession number(s) can be found at: Link for the environment dataset used in this study: https://rb.gy/jczv1. Link for the complete table of variables description and code: https://rb.gy/lh0p3.
Author contributions
GNS: Software, Writing - original draft, Visualization. AJPC: Conceptualization, Methodology, Writing, Supervision. MEB: Writing - review & editing, Conceptualization. APN: Data curation, Writing - review & editing. PVVP: Funding acquisition, Writing - review & editing. IAC: Conceptualization, Methodology, Validation, Resources, Writing, Supervision, Project administration, Funding acquisition.
Funding
This study was supported by the Feed the Future Innovation Lab for Collaborative Research on Sustainable Intensification (SIIL) at Kansas State University through funding United States Agency for International Development (USAID) under the Cooperative Agreement (Grant number AIDOAA-L-14-00006). This is contribution no. 24-056-J from the Kansas Agricultural Experiment Station.
Conflict of interest
The authors declare that the research was conducted in the absence of any commercial or financial relationships that could be construed as a potential conflict of interest.
Publisher’s note
All claims expressed in this article are solely those of the authors and do not necessarily represent those of their affiliated organizations, or those of the publisher, the editors and the reviewers. Any product that may be evaluated in this article, or claim that may be made by its manufacturer, is not guaranteed or endorsed by the publisher.
Footnotes
References
Antle, J. M., Jones, J. W., and Rosenzweig, C. E. (2017). Next generation agricultural system data, models, and knowledge products: introduction. Agric. Syst. 155, 186–190. doi: 10.1016/j.agsy.2016.09.003
Avazpour, I., Grundy, J., and Zhu, L. (2019). Engineering complex data integration, harmonization, and visualization systems. J. Ind. Inf. Integr. 16:100103. doi: 10.1016/j.jii.2019.08.001
Banda, E., Kpodo, J., Rafiei, V., Singh, G., Das, N. N., Nejadhashemi, A. P., et al. (2023). Regional water-stress identification and crop yield estimation in Senegal based on a novel water demand index. Climate risk management. In preparation.
Baumüller, H. (2018). The little we know: an exploratory literature review on the utility of Mobile phone-enabled Services for Smallholder Farmers: the little we know. J. Int. Dev. 30, 134–154. doi: 10.1002/jid.3314
Bion, R. (2023). Ggradar [R]. Available at: https://github.com/ricardo-bion/ggradar (Original work published 2016)
Birol, E., Meenakshi, J. V., Oparinde, A., Perez, S., and Tomlins, K. (2015). Developing country consumers’ acceptance of biofortified foods: a synthesis. Food Security 7, 555–568. doi: 10.1007/s12571-015-0464-7
Bornmann, L., and Mutz, R. (2015). Growth rates of modern science: a bibliometric analysis based on the number of publications and cited references: growth rates of modern science: a bibliometric analysis based on the number of publications and cited references. J. Assoc. Inf. Sci. Technol. 66, 2215–2222. doi: 10.1002/asi.23329
Brown, M. E., Carcedo, A. J. P., Eggen, M., Grace, K. L., Neff, J., and Ciampitti, I. A. (2023). Integrated modeling framework for sustainable agricultural intensification. Front. Sustainable Food Sys. 6:1039962. doi: 10.3389/fsufs.2022.1039962
Brown, M. E., Carr, E. R., Grace, K. L., Wiebe, K., Funk, C. C., Attavanich, W., et al. (2017). Do markets and trade help or hurt the global food system adapt to climate change? Food Policy 68, 154–159. doi: 10.1016/j.foodpol.2017.02.004
Burgelman, J.-C., Pascu, C., Szkuta, K., Von Schomberg, R., Karalopoulos, A., Repanas, K., et al. (2019). Open Science, open data, and open scholarship: European policies to make science fit for the twenty-first century. Front. Big Data 2:43. doi: 10.3389/fdata.2019.00043
Chang, W., Cheng, J., Allaire, J., Sievert, C., Schloerke, B., Xie, Y., et al. (2023). Shiny: web application framework for R. R package version 1:4.9002. Available at: https://shiny.rstudio.com/
Cheng, J., Karambelkar, B., Xie, Y., Wickham, H., Russell, K., Johnson, K., et al. (2022). Leaflet: Create interactive web maps with the JavaScript “leaflet” library (2.1.1). Available at: https://CRAN.R-project.org/package=leaflet
Coble, K. H., Mishra, A. K., Ferrell, S., and Griffin, T. (2018). Big data in agriculture: a challenge for the future. Appl. Econ. Perspect. Policy 40, 79–96. doi: 10.1093/aepp/ppx056
Coelho, F., Relvas, S., and Barbosa-Póvoa, A. P. (2021). Simulation-based decision support tool for in-house logistics: the basis for a digital twin. Comput. Ind. Eng. 153:107094. doi: 10.1016/j.cie.2020.107094
Correndo, A., McArtor, B., Prestholt, A., Hernandez, C., Kyveryga, P. M., and Ciampitti, I. A. (2022). Interactive soybean variable-rate seeding simulator for farmers. Agron. J. 114, 3554–3565. doi: 10.1002/agj2.21181
Cotter, M., Asch, F., Abera, B. B., Andre Chuma, B., Senthilkumar, K., Rajaona, A., et al. (2020). Creating the data basis to adapt agricultural decision support tools to new environments, land management and climate change—a case study of the RiceAdvice app. J. Agron. Crop Sci. 206, 423–432. doi: 10.1111/jac.12421
Crüwell, S., van Doorn, J., Etz, A., Makel, M. C., Moshontz, H., Niebaum, J. C., et al. (2019). Seven easy steps to Open Science: an annotated Reading list. Z. Psychol. 227, 237–248. doi: 10.1027/2151-2604/a000387
Daraio, C., Lenzerini, M., Leporelli, C., Moed, H. F., Naggar, P., Bonaccorsi, A., et al. (2016). Data integration for research and innovation policy: an ontology-based data management approach. Scientometrics 106, 857–871. doi: 10.1007/s11192-015-1814-0
DHS Standard Recode Manual ICF. (2018). Demographic and health surveys standard recode manual for DHS7. The demographic and health surveys program. Rockville, Maryland, U.S.A.: ICF
Di Prima, S., Wright, E. P., Sharma, I. K., Syurina, E., and Broerse, J. E. W. (2022). Implementation and scale-up of nutrition-sensitive agriculture in low- and middle-income countries: a systematic review of what works, what doesn’t work and why. Glob. Food Sec. 32:100595. doi: 10.1016/j.gfs.2021.100595
Direction de l’Analyse, de la Prévision et des Statistiques Agricoles—DAPSA. (n.d.). Retrieved February 8, 2023, Available at: https://www.dapsa.gouv.sn/
Earth Science Data Systems (2021). LANCE: NASA near real-time data and imagery [basic page]. Earthdata; Earth Science Data Systems, NASA. Available at: https://www.earthdata.nasa.gov/learn/find-data/near-real-time
Ezeoha, A. E., Obi, A., Igwe, A., and Ezeruigbo, C. (2020). The mobile phone revolution, the internet and rural electricity: what are the implications for food security in Africa? Inf. Dev. 36, 603–622. doi: 10.1177/0266666919884991
Fanzo, J., Haddad, L., McLaren, R., Marshall, Q., Davis, C., Herforth, A., et al. (2020). The food systems dashboard is a new tool to inform better food policy. Nat Food 1, 243–246. doi: 10.1038/s43016-020-0077-y
Funk, C., Peterson, P., Landsfeld, M., Pedreros, D., Verdin, J., Shukla, S., et al. (2015). The climate hazards infrared precipitation with stations—a new environmental record for monitoring extremes. Scientific Data 2:150066. doi: 10.1038/sdata.2015.66
García-Peñalvo, F. J., García de Figuerola, C., and Merlo, J. A. (2010). Open knowledge: challenges and facts. Online Inf. Rev. 34, 520–539. doi: 10.1108/14684521011072963
González Rodríguez, G., Gonzalez-Cava, J. M., and Méndez Pérez, J. A. (2020). An intelligent decision support system for production planning based on machine learning. J. Intell. Manuf. 31, 1257–1273. doi: 10.1007/s10845-019-01510-y
Gurstein, M. B. (2011). Open data: empowering the empowered or effective data use for everyone? First Monday. doi: 10.5210/fm.v16i2.3316
Harrison, T. M., and Sayogo, D. S. (2014). Transparency, participation, and accountability practices in open government: a comparative study. Gov. Inf. Q. 31, 513–525. doi: 10.1016/j.giq.2014.08.002
Hartkamp, A. D., White, J. W., and Hoogenboom, G. (1999). Interfacing geographic information systems with agronomic Modeling: a review. Agron. J. 91, 761–772. doi: 10.2134/agronj1999.915761x
Himesh, S., Rao, E. V. S. P., Gouda, K. C., Ramesh, K. V., Rakesh, V., Mohapatra, G. N., et al. (2018). Digital revolution and big data: a new revolution in agriculture. CABI Reviews 2018, 1–7. doi: 10.1079/PAVSNNR201813021
Inc. P. T. (2015). Collaborative data science. Montreal, QC: Plotly Technologies Inc. Available at: https://plot.ly
Jakku, E., Taylor, B., Fleming, A., Mason, C., Fielke, S., Sounness, C., et al. (2019). “If they don’t tell us what they do with it, why would we trust them?” trust, transparency, and benefit-sharing in smart farming. NJAS: Wageningen journal of. Life Sci. 90–91, 1–13. doi: 10.1016/j.njas.2018.11.002
Jetzek, T., Avital, M., and Bjorn-Andersen, N. (2014). Data-driven innovation through open government data. J. Theor. Appl. Electron. Commer. Res. 9, 15–16. doi: 10.4067/S0718-18762014000200008
Jones, M., and Taylor, G. (2004). Data integration issues for a farm decision support system. Trans. GIS 8, 459–477. doi: 10.1111/j.1467-9671.2004.00196.x
Kharel, T. P., Ashworth, A. J., Owens, P. R., and Buser, M. (2020). Spatially and temporally disparate data in systems agriculture: issues and prospective solutions. Agron. J. 112, 4498–4510. doi: 10.1002/agj2.20285
Klerkx, L., Jakku, E., and Labarthe, P. (2019). A review of social science on digital agriculture, smart farming, and agriculture 4.0: new contributions and a future research agenda. NJAS: Wageningen journal of. Life Sci. 90–91, 1–16. doi: 10.1016/j.njas.2019.100315
Laurent, A., Lyu, X., Kyveryga, P., Makowski, D., Hofmann, H., and Miguez, F. (2021). Interactive web-based data visualization and analysis tool for synthetizing on-farm research networks data. Res. Synth. Methods 12, 62–73. doi: 10.1002/jrsm.1440
Lowndes, J. S. S., Best, B. D., Scarborough, C., Afflerbach, J. C., Frazier, M. R., O’Hara, C. C., et al. (2017). Our path to better science in less time using open data science tools. Nature Ecology & Evolution 1:0160. doi: 10.1038/s41559-017-0160
Manorat, R., Becker, L., and Flory, A. (2019). Global data visualization tools to empower decision-making in nutrition. Sight and Life 33, 108–114.
McCampbell, M., Adewopo, J., Klerkx, L., and Leeuwis, C. (2021). Are farmers ready to use phone-based digital tools for agronomic advice? Ex-ante user readiness assessment using the case of Rwandan banana farmers. J. Agric. Educ. Ext. 29, 1–23. doi: 10.1080/1389224X.2021.1984955
Musumba, M., Grabowski, P., Palm, C., and Snapp, S. (2017). Guide for the sustainable intensification assessment framework. SSRN Electron. J. doi: 10.2139/ssrn.3906994
Neufeldt, H., Jahn, M., Campbell, B. M., Beddington, J. R., DeClerck, F., De Pinto, A., et al. (2013). Beyond climate-smart agriculture: toward safe operating spaces for global food systems. Agric Food Security 2:12. doi: 10.1186/2048-7010-2-12
Ngo, E., Truong, M. B.-T., and Nordeng, H. (2020). Use of decision support tools to empower pregnant women: systematic review. J. Med. Internet Res. 22:e19436. doi: 10.2196/19436
Pandey, V. L., Mahendra Dev, S., and Jayachandran, U. (2016). Impact of agricultural interventions on the nutritional status in South Asia: a review. Food Policy 62, 28–40. doi: 10.1016/j.foodpol.2016.05.002
Ramalho, L. D. F., and Segundo, W. R. D. C. (2020). R-shiny as an Interface for data visualization and data analysis on the Brazilian digital library of theses and dissertations (BDTD). Publica 8:24. doi: 10.3390/publications8020024
Rose, D. C., Sutherland, W. J., Parker, C., Lobley, M., Winter, M., Morris, C., et al. (2016). Decision support tools for agriculture: towards effective design and delivery. Agric. Syst. 149, 165–174. doi: 10.1016/j.agsy.2016.09.009
Rotz, S., Duncan, E., Small, M., Botschner, J., Dara, R., Mosby, I., et al. (2019). The politics of digital agricultural technologies: a preliminary review. Sociol. Rural. 59, 203–229. doi: 10.1111/soru.12233
Runck, B. C., Joglekar, A., Silverstein, K. A. T., Chan-Kang, C., Pardey, P. G., and Wilgenbusch, J. C. (2022). Digital agriculture platforms: driving data-enabled agricultural innovation in a world fraught with privacy and security concerns. Agron. J. 114, 2635–2643. doi: 10.1002/agj2.20873
Saltelli, A., Bammer, G., Bruno, I., Charters, E., Di Fiore, M., Didier, E., et al. (2020). Five ways to ensure that models serve society: a manifesto. Nature 582, 482–484. doi: 10.1038/d41586-020-01812-9
Santos, C. H. D., Lima, R. D. C., Leal, F., de Queiroz, J. A., Balestrassi, P. P., and Montevechi, J. A. B. (2020). A decision support tool for operational planning: a digital twin using simulation and forecasting methods. Production 30:e20200018. doi: 10.1590/0103-6513.20200018
Shim, J. P., Warkentin, M., Courtney, J. F., Power, D. J., Sharda, R., and Carlsson, C. (2002). Past, present, and future of decision support technology. Decis. Support. Syst. 33, 111–126. doi: 10.1016/S0167-9236(01)00139-7
Siddique, K. H. M., Johansen, C., Turner, N. C., Jeuffroy, M.-H., Hashem, A., Sakar, D., et al. (2012). Innovations in agronomy for food legumes. A review. Agronomy Sustainable Development 32, 45–64. doi: 10.1007/s13593-011-0021-5
Sievert, C., Iannone, R., Allaire, J., and Borges, B., (2023). Flexdashboard: R markdown format for flexible dashboards. Available at: https://pkgs.rstudio.com/flexdashboard/, https://github.com/rstudio/flexdashboard/.
Stewart, Z. P., Middendorf, B. J., Musumba, M., Grabowski, P., Palfzm, C., Snapp, S., et al. (2018). Feed the future innovation lab for collaborative research on sustainable intensification, Kansas State University, Manhattan, KS, United States.
Thee, D. H. S. (2021). Program—Quality information to plan, monitor and improve population, health, and nutrition programs. Retrieved February 8, 2023, from Available at: https://dhsprogram.com/
The Early Warning Explorer (EWX). (n.d.). A user-friendly Interface to view maps and time series of geospatial data | climate hazards Center—UC Santa Barbara. Retrieved February 16, 2023, from Available at: https://www.chc.ucsb.edu/tools/ewx
Therond, O., Belhouchette, H., Janssen, S., Louhichi, K., Ewert, F., Bergez, J.-E., et al. (2009). Methodology to translate policy assessment problems into scenarios: the example of the SEAMLESS integrated framework. Environ. Sci. Pol. 12, 619–630. doi: 10.1016/j.envsci.2009.01.013
Turner, W., Rondinini, C., Pettorelli, N., Mora, B., Leidner, A. K., Szantoi, Z., et al. (2015). Free and open-access satellite data are key to biodiversity conservation. Biol. Conserv. 182, 173–176. doi: 10.1016/j.biocon.2014.11.048
Wei, T., and Simko, V., (2021). R package 'corrplot': Visualization of a correlation matrix. (Version 0.92), Available at: https://github.com/taiyun/corrplot.
Wickham, H., (2016). ggplot2: Elegant graphics for data analysis. Springer-Verlag New York. Available at: https://ggplot2.tidyverse.org
Wickham, D., and Müller, K., (2022). “DBI: R Database Interface.” Available at: https://cran.rproject.org/web/packages/DBI/index.html
Wickham, H., François, R., Henry, L., Müller, K., and Vaughan, D. (2023). Dplyr: a grammar of data manipulation. Available at: https://dplyr.tidyverse.org, https://github.com/tidyverse/dplyr.
Wickham, H., and Seidel, D., RStudio. (2022). Scales: Scale functions for visualization (1.2.1). Available at: https://CRAN.R-project.org/package=scales
Wyche, S., and Steinfield, C. (2016). Why Don’t farmers use cell phones to access market prices? Technology affordances and barriers to market information services adoption in rural Kenya. Inf. Technol. Dev. 22, 320–333. doi: 10.1080/02681102.2015.1048184
Xie, Y., Cheng, J., Tan, X., Allaire, J. J., Girlich, M., Ellis, G. F., et al. (2023). DT: A wrapper of the JavaScript library “DataTables” (0.27). Available at: https://CRAN.R-project.org/package=DT
Yaro, J. A. (2006). Is Deagrarianisation real? A study of livelihood activities in rural northern Ghana. J. Mod. Afr. Stud. 44, 125–156.
Zipkin, E. F., Zylstra, E. R., Wright, A. D., Saunders, S. P., Finley, A. O., Dietze, M. C., et al. (2021). Addressing data integration challenges to link ecological processes across scales. Front. Ecol. Environ. 19, 30–38. doi: 10.1002/fee.2290
Keywords: shiny, open repository, sustainable intensification domains, open science, digital tool
Citation: Santiago GN, Carcedo AJP, Brown ME, Nejadhashemi AP, Prasad PVV and Ciampitti IA (2023) Data integration dashboard for assessing and planning sustainable intensification agricultural interventions: a case study in Senegal. Front. Sustain. Food Syst. 7:1208286. doi: 10.3389/fsufs.2023.1208286
Edited by:
Manjeet Singh Nain, Indian Agricultural Research Institute (ICAR), IndiaReviewed by:
Willingthon Pavan, International Fertilizer Development Center, United StatesRebecca McLaren, Global Alliance for Improved Nutrition (GAIN), Switzerland
Copyright © 2023 Santiago, Carcedo, Brown, Nejadhashemi, Prasad and Ciampitti. This is an open-access article distributed under the terms of the Creative Commons Attribution License (CC BY). The use, distribution or reproduction in other forums is permitted, provided the original author(s) and the copyright owner(s) are credited and that the original publication in this journal is cited, in accordance with accepted academic practice. No use, distribution or reproduction is permitted which does not comply with these terms.
*Correspondence: Gustavo N. Santiago, gsantiago@ksu.edu; Ignacio A. Ciampitti, ciampitti@ksu.edu