- 1College of Economics and Management, Dongguan University of Technology, Dongguan, China
- 2China Research Center on Urban Resource-Based Transformation and Rural Revitalization, China University of Mining and Technology, Xuzhou, China
- 3College of Public Administration, Nanjing Agricultural University, Nanjing, China
Attaining agricultural sustainability and eliminating poverty are the key challenges of rural areas. Non-farm income diversification is a vital livelihood strategy that endorses sustainable agriculture and alleviates poverty. Considering the land degradation and poor economic situation of Pakistan's rain-fed areas, the current research examined the potential effects of non-farm income diversification on household poverty and adopting soil and water conservation (SWC) technologies. A survey of 441 farmers was conducted in rain-fed areas of Punjab, Pakistan, and for econometric analysis, the propensity score matching (PSM) technique was employed to explore the objectives. The results signified that diversified farmers were more likely to adopt SWC practices and were less vulnerable to poverty. The findings suggest that farmer-based organizations and agricultural extension activities must be strengthened as they support non-farm income diversification, thus facilitating investment in soil and water conservation technologies and reducing poverty.
1. Introduction
The rain-fed farming areas of Pakistan are recorded to have relatively high poverty levels due to overdependence on rain for farming activities and other livelihoods (Rashid and Rasul, 2011; Bakhsh and Kamran, 2019). Moreover, because of poor agricultural production, inefficient land use, and inadequate off-farm options, Punjab's northern regions, such as the Potohar region, confront significant challenges such as food security and poverty (Suleri and Iqbal, 2019).
Addressing pressing challenges, such as poverty and climate vulnerability, for a nation is the biggest obstacle to achieving sustainable development goals (Issahaku and Abdul-Rahaman, 2019). Poverty increases vulnerability, and susceptibility to climate uncertainty further exacerbates poverty (Eriksen and O'brien, 2007). In addition, soil degradation in rain-fed areas is primarily caused by primitive farming practices that physically, chemically, and biologically deteriorate the soil (Ali et al., 2020).
Intensive agriculture systems significantly negatively impact climate change, greenhouse gas emissions, and soil degradation and cause pollution. Adopting sustainable farming practices mitigates these effects and ensures a more sustainable future (Ali et al., 2019). Hence, farmers must replace traditional farming methods with more sustainable conservation practices (Nawab et al., 2021). Soil and water conservation (SWC) includes the set of technologies to cointegrate the management of soil, water, and further environmental resources to fulfill essential human needs by bringing long-term sustainability to biodiversity and livelihoods (Baig et al., 2013). SWC adoption is considered the entry point for increased productivity and income, thus, breaking the vicious circle of poverty (Manda et al., 2016). Despite demonstrating considerable enthusiasm and efforts initially, evidence of adopting SWC practices to achieve optimal results is weak (Qadir and Oster, 2004; Mazhar and Shirazi, 2023). SWC practices are capital-intensive. Therefore, smallholders are often cash-strapped due to crop failures, poor harvests, price instability, and imperfections in financial markets (Abidoye and Odusola, 2015). Hence, smallholders have acknowledged non-farm diversification as a sustainable strategy (Issahaku and Abdul-Rahaman, 2019).
Stifel (2010) described income diversification as increasing sources to stabilize household income. This study applied the concept of income diversification concept where a farmer is engaged in sources other than farming, such as self-employment, trading, paid work, and other occupations or enterprises. The major reasons behind income diversification are to decrease the low-income risk through diversification ex-ante, to achieve food security in the event of diminishing farm yield, and to avoid climate shocks through diversification ex-post due to failure of insurance coverage and lack of credit availability (Ellis, 2010). Additionally, it is a norm among households to diversify their income during the off-farm season to avoid low income (Ellis, 1998).
Existing literature suggests that income diversification provides a parallel source of household income (Pfeiffer et al., 2009; Owusu et al., 2011). Olugbire et al. (2011) suggested that the non-farm sector significantly donates to economic and rural development in a community. Income diversification facilitates on-farm investments and the adoption of the latest agricultural technologies, while on-farm income can be capitalized in commerce-related activities (Anang, 2019). In addition, income diversification is related to superior revenues and ensures consistent access to food (Babatunde and Qaim, 2010). Literature suggests two probable impacts of income diversification (Babatunde, 2015): the income effect, which increases farm-level investment, and the lost labor effect, the labor numbers probably are lowered owing to other occupations from farming operations. Multiple studies (Desbiez et al., 2004; Chang et al., 2008; Stampini and Davis, 2009; Anríquez and Daidone, 2010; Scharf and Rahut, 2014) acknowledged the significant effect of income diversification on farm production, labor hiring, procurement of farm inputs, and households' food security.
In contrast, Pfeiffer et al. (2009) stated an inverse relation between non-farm participation, farm investment, and productivity. Kousar and Abdulai (2015) found an inverse relationship between non-farm income influx and fertilizer application in rural Punjab, Pakistan. Similarly, Huang et al. (2019) reported a negative association between non-farm diversification and adopting SWC practices among the farmers of the loess plateau in China. Non-farm participation restrains labor availability; hence, it does not necessarily support farm-level investment, contrary to the common assumption. The standard hypothesis suggests that the smallholders depending on agriculture are expected to invest the extra income in on-farm ventures. Conflicting empirical evidence makes it essential to investigate this further in the local context. Though some studies have explored income diversification in Pakistan, only scant literature discusses the role of non-farm income in adopting SWC and household poverty. The study thus contributes to Pakistan's empirical study by investigating the impact of non-farm income diversification on household poverty and the adoption of conservation technologies.
1.1. Farmer's decision to participate in non-farm income diversification
The study employed a sustainable livelihood framework (Figure 1) as the base for exploring the income diversification strategies being used by smallholders (Scoones, 1998). The framework comprises five core capitals: human capital, natural capital, financial capital, physical capital, and social capital. Context is the other major component of the framework, consisting of multiple sources of vulnerability, such as climate shocks, seasonality, and price variability of farming inputs and outputs. In this scenario, Solesbury (2003) argues that people have objectives (livelihood outcomes), and to achieve them, they undertake certain activities (adaptation strategies) using resources (livelihood assets) they can access. The marginal farmers depend heavily on crop production and seasonal wages from labor activities, whereas financially well farmers have sound access to productive assets (such as human and land capital) and use their capital base to engage in productive activities with higher returns. Farm households diversify their income portfolio by engaging in off-farm due to low farm income and excess family labor availability. For instance, Olale and Henson (2012) found a reduction of poverty in the fishing community by diversifying the income source and relieving the extra stress on fishing resources. Multiple researchers (Reardon, 1997; Abdulai and Delgado, 1999; Barrett et al., 2001; Woldenhanna and Oskam, 2001) have reported similar results in the past. Farm households diversify their income portfolio by engaging in off-farm due to low farm income and excess family labor availability. Hence, farmers allocate their part-time labor force to numerous non-farm activities such as sole proprietorship, petty trade, or participation in the migratory labor market. Income diversification enables farm households to generate substantial income, building resilience against climate change, reducing vulnerability, and escaping poverty. Smallholders from the rain-fed area, often called subsistence farmers, are considered susceptible to climate change and adapt their livelihood systems in the vulnerable context.
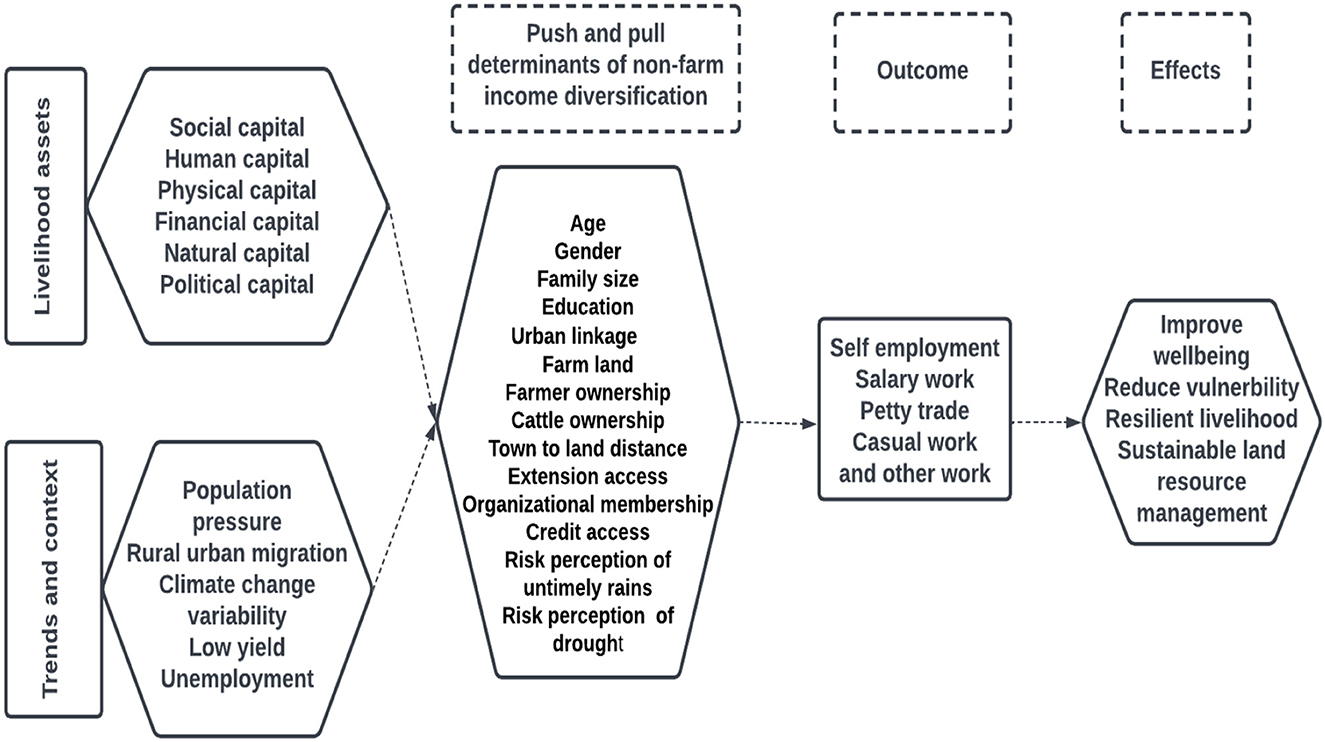
Figure 1. Conceptual framework [Source: Adapted from Scoones, 1998].
The framework offers a theoretical foundation for analyzing and comprehending the determinants that influence the selection of livelihood approaches and their interrelationships. There exists a correlation between the endowment of capital and contextual factors in the decision-making process of households in selecting livelihood activities that either enhance or maintain their means of subsistence. The sustainable dimension pertains to how households can leverage resources to mitigate susceptibility arising from health, climatic, and market-related perturbations.
2. Methodology
2.1. Study area and data collection
The study was conducted in the northern parts of Punjab province, Pakistan, between the Indus and Jhelum Rivers, often called the Potohar plateau shown in Figure 2. The area of the Potohar plateau is 13,000 square kilometers, with elevation from the sea level between 305 and 610 m. The region follows an erratic rain pattern and undulating topography (Amir et al., 2019).
Approximately 994 thousand hectares of the Potohar plateau are under cultivation, with only 4% of the cultivated land irrigated, and approximately 96% of the land depends on rainwater. Rain-fed agriculture has low efficiency because of soil dissolution, unanticipated and inadequate rainfall, relatively low matter substance, and undesirable ecological conditions such as dry air and high temperatures. As a result of these factors, the Potohar plateau is facing severe food shortages and poverty-related issues (Suleri and Iqbal, 2019). The study consists of districts such as Rawalpindi and Chakwal from the Potohar area. This study employs a simple random sampling technique for data collection. A survey was conducted through a well-trained interviewer, and the rural population of these areas was our unit of analysis. Punjab province was selected in the first data collection phase because of its agriculture and economic importance to the country. In the second stage of the study, three districts (Rawalpindi, Chakwal, Jhelum) were selected. Consequently, in the third stage two tehsils were chosen from each of the district. Furthermore, we selected four to five union councils from each of the tehsils, and at the next stage, two to three villages were randomly selected from each union council. Finally, nearly 5 to 7 farmers were randomly chosen from each village, and a combined 441 were chosen.
2.2. Variable specification
This study employed non-farm income diversification as the treatment variable, with 1 signifying participation in non-farm activities and 0 = otherwise. Poverty was measured via two indicators: food consumption per capita and vulnerability. Food consumption was the continuous variable suggesting per capita expenditure in rupees. The vulnerability to predicted poverty can be described as the likelihood of household consumption dropping beneath the poverty line. As described by Morduch (1994), stochastic poverty is a significant part of vulnerability and often results when people rely on agriculture that is highly susceptible to weather, has underdeveloped banking systems, and lacks adequate social support. Based on empirical evidence, this study operationalized the dummy variable as 1, signifying a farmer expected to suffer from a poverty incident, and 0 = otherwise. Based on a literature review (Lass et al., 1991; Beyene, 2008; Babatunde, 2015; Iqbal et al., 2015), the determinants of non-farm income diversification were characterized as farmers, farm level, and institutional and environmental characteristics (see Table 1 for definitions). Based on the literature review (Bhutto and Bazmi, 2007; Baig et al., 2013; Usman et al., 2016; Jabbar et al., 2020; Nawab et al., 2021) and local context, we chose three SWC technologies, namely bund making (BM), drip irrigation (DI), and improved varieties, being practiced in the study region. Drip irrigation is an agricultural water technology that uses a systematic network of pipes and tubes to give controlled water flow. It is an effective system supported by government and non-government channels to handle constrained water resources effectively (Usman et al., 2016). DI is taken as a dummy variable with 1 = drip irrigation adoption and 0 = otherwise. Bund making is used to conserve soil moisture and minimize soil erosion. This technique is quite useful in saving water and restoring soil productivity. Contour trenching, terracing, crib structures, stone check dams, etc. are the common forms of bund making (BM) (Pathak et al., 1989). BM is taken as the dummy variable with 1 = if the farmer applies bund making and 0 = otherwise. Improved varieties are considered resistant to heat and droughts and better suited to the warmer and drier climate, with the potential to counterbalance the yield losses linked to climate change (Jabbar et al., 2022).
2.3. PSM for the impact of non-farm income diversification on adopting SWC and poverty
This study employs a random utility framework conferring that farmers would diversify in case of utility gain is positive. Hence, farmers would likely diversify their income portfolio if = UDJ − UNDJ > 0, whereas UDJ and UNDJ are the utilities for non-farm diversification and non-diversification, correspondingly. Consider yi1 is the outcome for the non-farm participants, while yi0 is for non-participants. Likewise, Smith and Todd (2001), the effect of non-farm diversification can be expressed as follows:
Δ Y denotes the impact of non-farm diversification for the sampled farmers. The mean difference in equation 1 is only possible if the farmer simultaneously engages in treatment and control groups. Nonetheless, as the farmer can only be involved in one group, measuring the treatment effect on non-farm participants has severe limitations. This study applied the propensity score matching (PSM) as we are interested in calculating both marginal and average treatment on treated (ATT) effects to provide an accurate understanding. The study operationalized the propensity score matching (PSM) approach to compare the outcomes of non-farm income diversification (“treated”) and non-diversification (“controlled”) alike in observable characteristics, hence avoiding the bias which may arise when the groups are methodically diverse (Dehejia and Wahba, 2002). It encompasses two stages; in the first stage Eq. (2), we generate the propensity score for participating in non-farm diversification activities. In the second stage, the average treatment on treated is calculated as in Eq. (3).
where P1 = {0, 1} is an indicator of choosing to participate in off-farm work (j=1), while Z1 is the vector of pre-choice characteristics.
This study employed kernel-based matching (KBM), nearest-neighbor matching (NNM), and radius-based methods to estimate the treatment effects on the treated. To further corroborate the findings from the PSM estimations, the study conducts the covariate balancing test. A balancing test is conducted to check whether households with similar propensity scores share parallel characteristics independent of treatment assignment (non-farm diversification).
3. Results
3.1. Descriptive statistics
Table 1 shows the descriptive statistics with food consumption expenditure per capita (ln) was 9.764. Sole proprietorship (Figure 3) was the most employed non-farm activity in the study region, whereas few respondents were engaged in more than one activity. To sum it up, the study found that approximately 42% of households were involved in a non-farming activity.
The average age in this study was 44 years, the average household size was 5.7 people per house, and the average education years was 2.788, indicating that most could read and write. Nearly 32% of the farmers adopted DI and 52% practiced BM. The average farm size was 3.21 acres, and nearly 56% of the farmers had accessed credit in the past 12 months, while 46% received any agricultural advisory during the past year. The average distance from the village to the town was 3 kilometers.
Table 2 highlights a significant difference in means among diversified and non-diversified considering urban linkage, credit access, extension access, organizational membership, and risk perception about drought. The summary statistics suggest that the off-farm participants are younger, affluent, educated, and have better access to social networks than the non-participants.
3.2. Determinants of non-farm income diversification
This study explores the effects of non-farm income diversification on adopting SWC technologies into farming and household poverty. We employed propensity score matching (PSM) to fulfill the research objectives. In the first stage, the PSM model estimates the determinants of non-farm income diversification, and furthermore, the treatment effects determine the impact of non-farm income diversification on poverty. The test statistics in Table 3 show that the LR chi-squared values for non-farm income diversification are positive; moreover, the probability of chi-squared was reported at the 0.000 level. Likewise, the pseudo-R2 value was also acceptable and showed significant variation. We categorized determinants based on empirical evidence (Lass et al., 1991; Beyene, 2008; Babatunde, 2015; Iqbal et al., 2015), non-farm participation as a farmer, farm, and institutional and environmental characteristics. Since parameter coefficients do not explain regression estimate magnitudes, we used the marginal effect to describe the results. The results suggest that gender and urban linkage positively influence the decision to participate in non-farm activities. The findings revealed that the farmers with some relative or connection outside the district are 12.5% more likely to participate in diversification activities than others with no external link, whereas livestock ownership is inversely related to non-farm income diversification decisions. The coefficient of cattle ownership is negative, showing that farmers with livestock ownership are 2.5% less likely to participate in off-farm activities. Institutional factors such as extension access and organizational membership also significantly and positively determine non-farm income decisions, while climate change risk perception also influences farmers' decisions to engage in non-farm income diversification.
3.3. Impact of non-farm income diversification on SWC adoption and poverty
The mean analysis ignores other factors that may composite the impact of non-farm activities on the outcome. Hence, considering this drawback, we carefully employed comprehensive econometric models to categorize the causal effects of non-farm income diversification on SWC adoption and household poverty. Based on propensity score predictions for both diversified and non-diversified groups, the study conducted a diagnostic test to determine the validity of the matching procedure for deciding how income diversification influences the outcome. Figure 4 and Table 4 illustrate the covariate balancing test and the assumption of a common support condition, respectively. The distribution of the propensity scores is depicted in the PSM graph.
The propensity score is well spread, ranging from nearly zero (0.026) to one (0.955), with an overall mean and standard deviation of 0.414 and 0.244, respectively. Figure 4 illustrates that the propensity scores for participants and non-participants are identical, indicating that the common support condition is fulfilled. Furthermore, a balance test was also performed in Table 4 to ensure equality across the covariates showing mean factors do not statistically differ; hence, off-farm participants and non-participant farmers share the same characteristics.
Table 5 findings show that after matching, the standardized mean covariate variance dropped from 30.7 to 9.1% leading to a cumulative reduction in the bias of about 70.9%, and the standardized mean is not larger than 5% after matching, as suggested by Rosenbaum and Rubin (1983).
Using three different PSM algorithms, Table 6 displays the impact of non-farm income diversification on poverty and adopting SWC practices. The findings showed that non-farm diversification enhances household consumption per capita by 0.22, 0.19, and 0.18, respectively. Farmers with non-farm involvement were less vulnerable to poverty as the vulnerability was decreased by 13–18% due to non-farm work. Likewise, Martin and Lorenzen (2016) found that non-farm participation in rural areas increases wealth accumulation and improves the financial situation of farmers.
Furthermore, the adoption of SWC practices was positively influenced by non-farm diversification. Accordingly, farmers with non-farm participation were 0.22 to 0.23 more likely to adopt DI, 0.23 to 31 more likely to adopt improved varieties, and 0.22 to 0.23 more likely to adopt BM.
4. Discussion
Considering the nation's culture and norms, the gender of the household head is significantly and directly related to participation in non-farm income diversification strategies. The findings seem validated, considering the traditional role of the household head in decision-making in such countries. Likewise, Amare and Belaineh (2013) supported the significant and positive role of gender in determining participation in non-farm income diversification strategies.
Among the farmer's characteristics, the findings revealed that the farmers with some relative or connection outside are more likely to participate in income diversification activities. The network outside the district facilitates their mobility and communication with other groups, enhancing their capacity to trade and employment opportunities better. Multiple studies support the influential role of networks in promoting trade and employment opportunities (Nagoda and Eriksen, 2014). The results are consistent with the study findings by Gautam and Andersen (2016), which also support external linkage's positive and significant role in off-farm decisions.
On the other hand, cattle ownership is negatively related to participation in non-farm income diversification activities. This is because managing livestock requires time and labor, leaving little space to work off-farm. Likewise, Kousar and Abdulai (2015) reported a negative relationship between livestock ownership and non-farm income diversification.
The farmer-based organizational membership (FBOs) significantly determined farmer engagement with non-farm income diversification. Membership in any organization will improve access to social capital and polish entrepreneurship skills. Organizational membership has been observed as an essential means to minimize the financial constraints among rural and urban communities (Yebisi, 2014). The farmer-based organizations provide a social platform where the stakeholders come together to solve their social and economic problems. Through these associations, individuals pool their financial and social resources, thus providing the resources required to fulfill their investment, production, and consumption needs. Likewise, Ritossa and Bulgacov (2009) supported the positive impact of organizational membership on non-farm income diversification decisions. The access to extension services significantly and positively determined the farmer's decision to diversify their income sources. Modern extension services help farmers expand their income sources, specifically in countries like Pakistan, where most farmers depend on the weather for the water demand of crops. Likewise, Danso-Abbeam et al. (2020) also found a significant and positive relationship between extension access and non-farm income diversification decisions.
The results indicate the existence of direct linkages between farmers' risk perception of drought and non-farm participation decisions. The increase in climatic uncertainties remains a significant factor in technology adoption decisions. Our results reflect that extreme climatic events may raise water scarcity and moisture loss issues, ultimately affecting farm output. Hence, non-farm income diversification is a risk mitigation strategy to offset the income losses from climate change. Likewise, Ullah and Shivakoti (2014) highlighted the mitigating risk potential of off-farm diversification against environmental hazards.
The findings highlighted the significance of non-farm income diversification in elevating the adoption of SWC practices. As explained earlier, soil and water conservation practices involve extensive labor and capital. In comparison, non-farm diversification generates an extra income stream that stabilizes the smallholders' economic situation. Hence, in such cases, the additional income can be used to adopt SWC practices or hire additional labor if required. Furthermore, our results indicate that the farmers with off-farm participation are likelier to adopt SWC practices. Likewise, the study by Issahaku and Abdul-Rahaman (2019) showed the positive role of non-farm income in adopting sustainable soil management practices in Ghana.
Smallholders with non-farm participation are food secure and less vulnerable to poverty. Reardon et al. (1992) indicate that the diversification into non-farm activities elevates calorie consumption among the farmers of Burkina Faso. Consequently, Ruben (2001) also reported similar results that showed a strong association between non-farm income and higher food consumption expenditures in Zimbabwe. Furthermore, Scharf and Rahut (2014) suggest that off-farm income effectively improves rural household income. Moreover, Chang et al. (2008) reported that household non-farm income diversification consumes more food than others. Likewise, Issahaku and Abdul-Rahaman (2019) confirmed that households with non-farm work participation are less vulnerable to poverty.
5. Conclusion
Recent climate uncertainties have endangered the livelihood of the farming community; hence, enhancing income and ensuring the food security of rural communities remain the foremost priority for most developing countries. This study considers the concern by exploring the role of off-farm participation in addressing climate-induced issues and suggests valuable policy insights in the South Asian context. The research employed propensity score matching (PSM) to explore the effects of non-farm diversification on SWC adoption and poverty (vulnerability, food consumption). The findings showed that gender, urban linkage, cattle ownership, extension access, organizational membership, and drought risk perception determine farmers' inclination toward non-farm diversification. The results indicate a positive impact of non-farm diversification on SWC adoption. Hence, it can be inferred that non-farm participation generates extra income, which can be used to buy farm inputs and hire labor, thus resolving both cash and labor constraints. These findings imply that farmers with non-farm participation were less vulnerable to poverty and consumed more food. The importance of non-farm participation will likely increase in upcoming years; hence policies and programs (extension access, farmer groups) that aim for environmental restoration must include it. Introducing a well-organized interest-free scheme for sole proprietorship and small-medium enterprise development seems attractive to mobilize and engage human resources. Furthermore, the scheme should prefer women entrepreneurs to eliminate gender biases and patriarchic issues. Female participation may improve the overall rural economy and the welfare of the farming community.
Data availability statement
The original contributions presented in the study are included in the article/supplementary material, further inquiries can be directed to the corresponding authors.
Author contributions
AJ: conceptualization, data analysis, data description, explanation of results, and writing. LW and JL: data analysis and writing. YW and JP: conceptualization, methodology, explanation of results, reviewing, and editing. JZ and QW: conceptualization, explanation of results, reviewing, and editing. All authors contributed to the article and approved the submitted version.
Conflict of interest
The authors declare that the research was conducted in the absence of any commercial or financial relationships that could be construed as a potential conflict of interest.
Publisher's note
All claims expressed in this article are solely those of the authors and do not necessarily represent those of their affiliated organizations, or those of the publisher, the editors and the reviewers. Any product that may be evaluated in this article, or claim that may be made by its manufacturer, is not guaranteed or endorsed by the publisher.
References
Abdulai, A., and Delgado, C. L. (1999). Determinants of non-farm earnings of farm-based husbands and wives in northern Ghana. Am. J. Agric. Econ 81, 117–130. doi: 10.2307/1244455
Abidoye, B. O., and Odusola, A. F. (2015). Climate change and economic growth in Africa: an econometric analysis. J. Afr. Econ 24, 277–301. doi: 10.1093/jae/eju033
Ali, A., Ghani, M. I., Haiyan, D., Iqbal, M., Cheng, Z., Cai, Z., et al. (2020). Garlic substrate induces cucumber growth development and decreases Fusarium wilt through regulation of soil microbial community structure and diversity in replanted disturbed soil. Int. J. Mol. Sci 21, 6008. doi: 10.3390/ijms21176008
Ali, A., Ghani, M. I., Li, Y., Ding, H., Meng, H., Cheng, Z., et al. (2019). Hiseq base molecular characterization of soil microbial community, diversity structure, and predictive functional profiling in continuous cucumber planted soil affected by diverse cropping systems in an intensive greenhouse region of northern China. Int. J. Mol. Sci. 20, 2619. doi: 10.3390/ijms20112619
Amare, D., and Belaineh, L. (2013). Determinants of income diversification among rural households: The case of smallholder farmers in Fedis district, Eastern Hararghe zone, Ethiopia. J. Dev. Agric. Econ. 5, 120–128. doi: 10.5897/JDAE12.104
Amir, S., Saqib, Z., Khan, A., Khan, M. I., Khan, M. A., and Majid, A. (2019). Land cover mapping and crop phenology of Potohar region, Punjab, Pakistan. Pak. J. Agric. Sci. 56, 1–14.
Anang, B. T. (2019). Effect of off-farm work on agricultural productivity: empirical evidence from northern Ghana. Agric. Sci. Technolo. 11:49–58. doi: 10.15547/10.15547/ast.2019.01.008
Anríquez, G., and Daidone, S. (2010). Linkages between the farm and non-farm sectors at the household level in rural Ghana: a consistent stochastic distance function approach. Agric. Econ. 41, 51–66. doi: 10.1111/j.1574-0862.2009.00425.x
Babatunde, R. O. (2015). On-farm and Off-farm works: Complement or substitute? Evidence from Nigeria Maastricht School of Management Working Paper 2.
Babatunde, R. O., and Qaim, M. (2010). Impact of off-farm income on food security and nutrition in Nigeria. Food. Policy 35, 303–311. doi: 10.1016/j.foodpol.2010.01.006
Baig, M. B., Shahid, S. A., and Straquadine, G. S. (2013). Making rainfed agriculture sustainable through environmental friendly technologies in Pakistan: a review. Int. Soil. Water. Conserv. Res. 1, 36–52. doi: 10.1016/S2095-6339(15)30038-1
Bakhsh, K., and Kamran, M. A. (2019). Adaptation to climate change in rain-fed farming system in Punjab, Pakistan. Int. J. Commons 13, 887. doi: 10.5334/ijc.887
Barrett, C. B., Reardon, T., and Webb, P. (2001). Non-farm income diversification and household livelihood strategies in rural Africa: concepts, dynamics, and policy implications. Food Policy 26, 315–331. doi: 10.1016/S0306-9192(01)00014-8
Beyene, A. D. (2008). Determinants of off-farm participation decision of farm households in Ethiopia. Agrekon 47, 140–161. doi: 10.1080/03031853.2008.9523794
Bhutto, A. A., and Bazmi, A. W. (2007). Sustainable agriculture and eradication of rural poverty in Pakistan. Nat. Resour. Forum 31, 253–262. doi: 10.1111/j.1477-8947.2007.00162.x
Chang, H.-H., and Mishra, A. (2008). Impact of off-farm labor supply on food expenditures of the farm household. Food. Policy 33, 657–664. doi: 10.1016/j.foodpol.2008.02.002
Danso-Abbeam, G., Dagunga, G., and Ehiakpor, D. S. (2020). Rural non-farm income diversification: implications on smallholder farmers' welfare and agricultural technology adoption in Ghana. Heliyon 6, e05393. doi: 10.1016/j.heliyon.2020.e05393
Dehejia, R. H., and Wahba, S. (2002). Propensity score-matching methods for nonexperimental causal studies. Rev. Econ. Stat. 84, 151–161. doi: 10.1162/003465302317331982
Desbiez, A., Matthews, R., Tripathi, B., and Ellis-Jones, J. (2004). Perceptions and assessment of soil fertility by farmers in the mid-hills of Nepal. Agric. Ecosyst. Environ 103, 191–206. doi: 10.1016/j.agee.2003.10.003
Ellis, F. (1998). Household strategies and rural livelihood diversification. J. Dev. Stud 35, 1–38. doi: 10.1080/00220389808422553
Ellis, F. (2010). “Strategic dimensions of rural poverty reduction in sub-Saharan Africa,” in The Comparative Political Economy of Development: Africa and South Asia, 47–63.
Eriksen, S. H., and O'brien, K. (2007). Vulnerability, poverty and the need for sustainable adaptation measures. Climate Policy 7, 337–352. doi: 10.1080/14693062.2007.9685660
Gautam, Y., and Andersen, P. (2016). (2016). Rural livelihood diversification and household well-being: insights from Humla, Nepal. J Rural Stud. 44:239–249. doi: 10.1016/j.jrurstud.2016.02.001
Huang, X., Lu, Q., Wang, L., et al. (2019). Does aging and off-farm employment hinder farmers' adoption behavior of soil and water conservation technology in the Loess Plateau? Int J Clim Chang Strateg Manag. 12, 92–107. doi: 10.1108/IJCCSM-04-2019-0021
Iqbal, M. A., Ping, Q., Ahmed, U. I., and Nazir, A. (2015). Determinants of off-farm activity participation among Cotton farmers in Punjab, Pakistan. Int. J. Manage. Accounting Econ. 2, 707–718.
Issahaku, G., and Abdul-Rahaman, A. (2019). Sustainable land management practices, off-farm work participation and vulnerability among farmers in Ghana: is there a nexus? Int. Soil Water Conserv. Res 7, 18–26. doi: 10.1016/j.iswcr.2018.10.002
Jabbar, A., Liu, W., Wang, Y., Zhang, J., Wu, Q., and Peng, J. (2022). Exploring the impact of farmer field schools on the adoption of sustainable agricultural practices and farm production: a case of pakistani citrus growers. Agronomy 12, 2054. doi: 10.3390/agronomy12092054
Jabbar, A., Wu, Q., Peng, J., Zhang, J., Imran, A., and Yao, L. (2020). Synergies and Determinants of Sustainable Intensification Practices in Pakistani Agriculture. Land 9, 110. doi: 10.3390/land9040110
Kousar, R., and Abdulai, A. (2015). Off-farm work, land tenancy contracts and investment in soil conservation measures in rural. J. Res. Econ. 60, 307–325. doi: 10.1111/1467-8489.12125
Lass, D. A., Findeis, J. L., and Hallberg, M. C. (1991). Factors affecting the supply of off-farm labor: a review of empirical evidence. Farm Families 255, 239–262.
Manda, J., Alene, A. D., Gardebroek, C., et al. (2016). Adoption and impacts of sustainable agricultural practices on maize yields and incomes: evidence from rural Zambia. J. Agric. Econ 67, 130–153. doi: 10.1111/1477-9552.12127
Martin, S. M., and Lorenzen, K. A. I. (2016). Livelihood diversification in rural Laos. World. Dev 83, 231–243. doi: 10.1016/j.worlddev.2016.01.018
Mazhar, N., and Shirazi, S. A. (2023). Community perceptions of the impacts of desertification as related to adaptive capacity in drylands of South Punjab, Pakistan. Asia-Pac. J. Reg. Sci. 7, 549–568.
Nagoda, S., and Eriksen, S. (2014). The Role Of Local Power Relations in Household Vulnerability to Climate Change in Humla, Nepal. New York, NY: Routledge, 200–218.
Nawab, J., Din, Z. U., Faisal, S., Khan, S., Ali, A., and Rahman, Z. (2021). Farmlands degradation with conventional agricultural practices and human health risk assessment: a case-study of Punjab Province, Pakistan. Land. Degrad. Dev 32, 4546–4561. doi: 10.1002/ldr.4051
Olale, E., and Henson, S. (2012). Determinants of income diversification among fishing communities in Western Kenya. Fish. Res. 125, 235–242. doi: 10.1016/j.fishres.2012.02.029
Olugbire, O. O., Falusi, A. O., Adeoti, A. I., Oyekale, A. S., and Adeniran, O. A. (2011). Non-farm income diversification and poverty reduction in Nigeria: A propensity-score matching analysis. Cont. J. Agric. Sci. 5, 21–28.
Owusu, V., Abdulai, A., and Abdul-Rahman, S. (2011). Non-farm work and food security among farm households in Northern Ghana. Food Policy. 36, 108–118.
Pathak, P., Laryea, K. B., and Singh, S. (1989). A modified contour bunding system for Alfisols of the semi-arid tropics. Agric. Water. Manag 16, 187–199. doi: 10.1016/0378-3774(89)90002-4
Pfeiffer, L., López-Feldman, A., and Taylor, J. E. (2009). Is off-farm income reforming the farm? Evidence from Mexico. Agric. Econ. 40, 125–138. doi: 10.1111/j.1574-0862.2009.00365.x
Qadir, M., and Oster, J. D. (2004). Crop and irrigation management strategies for saline-sodic soils and waters aimed at environmentally sustainable agriculture. Sci. Total Environ. 323, 1–19.
Rashid, K., and Rasul, G. (2011). Rainfall variability and maize production over the Potohar Plateau of Pakistan. Pakistan J. Meteorology 8, 63–74.
Reardon, T. (1997). Using evidence of household income diversification to inform study of the rural non-farm labor market in Africa. World. Dev 25, 735–747. doi: 10.1016/S0305-750X(96)00137-4
Reardon, T., Delgado, C., and Matlon, P. (1992). Determinants and effects of income diversification amongst farm households in Burkina Faso. J. Dev. Stud 28, 264–296. doi: 10.1080/00220389208422232
Ritossa, C. M., and Bulgacov, S. (2009). Internationalization and diversification strategies of agricultural cooperatives: a quantitative study of the agricultural cooperatives in the state of Parana. BAR-Braz. Admin. Rev. 6, 187–212. doi: 10.1590/S1807-76922009000300003
Rosenbaum, P. R., and Rubin, D. B. (1983). The central role of the propensity score in observational studies for causal effects. Biometrika 70, 41–55. doi: 10.1093/biomet/70.1.41
Ruben, R. (2001). Non-farm employment and poverty alleviation of rural farm households in Honduras. World. Dev 29, 549–560. doi: 10.1016/S0305-750X(00)00107-8
Scharf, M. M., and Rahut, D. B. (2014). Non-farm employment and rural welfare: Evidence from the Himalayas. Am. J. Agric. Econ 96, 1183–1197. doi: 10.1093/ajae/aau040
Smith, J. A., and Todd, P. E. (2001). Reconciling conflicting evidence on the performance of propensity-score matching methods. Am. Econ. Rev. 91, 112–118. doi: 10.1257/aer.91.2.112
Solesbury, W. (2003). Sustainable livelihoods: A case study of the evolution of DFID policy (Vol. 217). London: Overseas Development Institute.
Stampini, M., and Davis, B. (2009). Does nonagricultural labor relax farmers' credit constraints? Evidence from longitudinal data for Vietnam. Agric. Econ. 40, 177–188. doi: 10.1111/j.1574-0862.2009.00368.x
Stifel, D. (2010). The rural non-farm economy, livelihood strategies and household welfare. Afr. J. Agric. Resour. Econ. 4, 82–109.
Suleri, A. Q., and Iqbal, M. (2019). National food security challenges and strategies in Pakistan. Reg. Coop. Sust. Food. Secur. 211, 1–21. doi: 10.4324/9780429346507-9
Ullah, R., and Shivakoti, G. P. (2014). Adoption of on-farm and off-farm diversification to manage agricultural risks: are these decisions correlated? Outlook. Agric. 43, 265–271. doi: 10.5367/oa.2014.0188
Usman, K. M., Muhammad, T., Majid, M., Ali, S. M., Shilan, R., Alireza, M., et al. (2016). Drip irrigation in Pakistan: status, challenges and future prospects. Russ. J. Agric. Socioecon. Sci 56, 1–4.
Woldenhanna, T., and Oskam, A. (2001). Income diversification and entry barriers: evidence from the Tigray region of northern Ethiopia. Food. Policy 26, 351–365. doi: 10.1016/S0306-9192(01)00009-4
Keywords: non-farm income diversification, soil and water conservation practices, poverty, rain-fed areas, propensity score matching
Citation: Jabbar A, Wei L, Zhang J, Liu J, Wang Y, Wu Q and Peng J (2023) Exploring the contributions of non-farm income diversification for improving soil and water conservation practices and reducing rural poverty in rain-fed areas of Punjab, Pakistan. Front. Sustain. Food Syst. 7:1179919. doi: 10.3389/fsufs.2023.1179919
Received: 05 March 2023; Accepted: 21 June 2023;
Published: 26 July 2023.
Edited by:
Matebu Tadesse, Addis Ababa University, EthiopiaReviewed by:
Nugun P. Jellason, Teesside University, United KingdomAhmad Ali, Jiangsu University, China
Copyright © 2023 Jabbar, Wei, Zhang, Liu, Wang, Wu and Peng. This is an open-access article distributed under the terms of the Creative Commons Attribution License (CC BY). The use, distribution or reproduction in other forums is permitted, provided the original author(s) and the copyright owner(s) are credited and that the original publication in this journal is cited, in accordance with accepted academic practice. No use, distribution or reproduction is permitted which does not comply with these terms.
*Correspondence: Liu Wei, liuw@dgut.edu.cn; Jiyun Liu, 493468065@qq.com