- 1College of Economics and Management, Jilin Agricultural University, Changchun, China
- 2College of Economics and Management, Henan Agricultural University, Zhengzhou, China
- 3Jilin Business and Technology College, Changchun, China
Introduction: Contract farming is seen as a tool to create new market opportunities that can address market imperfections in many developing countries and thus increase smallholder income.
Methods: This study examines the impact of contract farming on farm household income using survey data from 610 rural households in China. The propensity score matching method addresses the sample selection bias of participation in contract farming.
Results: Contract farming can significantly increase farmers’ income, and both marketing contracts and production-management contracts can substantially increase farmers’ income levels, with production-management contracts having a greater degree of impact. Additional analysis reveals that breeding years, farm size, and training time can significantly affect how contract farming enhances farmers’ income. At the same time, contract farming can also considerably improve farmers’ technical efficiency in agricultural production. Participation in contract farming enhances the tendency to centralize the technical efficiency of agricultural production. Further analysis shows that the technical efficiency of agricultural production partially mediates the effects of contract farming on farm household income.
Discussion: Contract farming can be an effective institutional arrangement for improving the technical efficiency of farm household production and revenue. We also point out that farmers should strengthen their comprehensive ability levels and actively participate in training to acquire new knowledge and improve their cognitive ability. Simultaneously, according to the characteristics of farmers’ resource endowments, small farmers are encouraged to cooperate with companies in depth and develop contractual contracts in a targeted manner. Promote win-win cooperation and benefit-sharing among various business entities to promote the sustainable and high-quality development of China’s beef cattle industry.
1. Introduction
As an essential carrier for vertical collaboration in the agricultural value chain, contract farming can not only effectively help smallholders gain access to the market but also achieve higher production efficiency, which is conducive to improving the income level of farm households thus ensuring food security (Bi et al., 2022). Since the 1950s, contract farming has increased in many countries because of its significant advantages such as saving transaction costs and mitigating market risks (Gray and Boehlj, 2005; Bellemare et al., 2013). By the end of the 20th century, contract farming had become a fundamental characteristic of modern agriculture in developed countries. Compared with developed countries, contract farming in China began late, beginning in the mid-1980s in the southeast coastal region. It has been supported by the Chinese government There are two organizational models for agribusiness firms involved in contract farming: one is “firm + smallholder” and the other is “firm + intermediary + smallholder” (Luo and Gao, 2022). This study focuses on contract farming in the “firm + smallholder” organizational model. This type of contract farming, generally refers to a form of coordination between the agribusiness firm and farm households, involves the signing of a legally valid production and marketing contract under predetermined conditions, whereby the farm household produces a specific quantity and quality of agricultural products for the agribusiness firm, and complies with predetermined requirements such as transaction prices and production processes (Hou et al., 2018; Khan et al., 2019). Simultaneously, farmers and firms sign production and marketing contracts for agricultural products to achieve order-based agricultural production. This is an important way for farmers to reduce production costs, enhance farm income and resist market risks. Therefore, in the context of the active development of contract farming in China, it is of practical importance to explore the impact of contract farming on the income effect of farmers, in order for the government to formulate policies that support the synergistic and integrated development of enterprises and farmers.
China is the world’s largest livestock producer and the second-largest beef consumer. Both the rigid demand and quality demand of beef in China have incremental space, and the development of the beef cattle industry is of great significance (Li et al., 2018). At the same time, the development status of China’s beef cattle industry, with the shortage of beef supply, high prices, lack of feed-grain resources, and low level of standardization, has put the development of the beef cattle industry under tremendous pressure (Li et al., 2022). Meanwhile, the beef cattle industry is a pillar industry for China’s agricultural and rural economic development and a realistic path to promote increased production and income for farmers (Zan et al., 2015). However, the promotion of contract farming is more complex and arduous for China, which is in a period of profound transition (Wang et al., 2014). What are the income effects of Chinese farmers’ participation in contract farming, and can contract farming effectively achieve the goal of increasing farmers’ income? This fundamental question needs to be answered by China’s agricultural industrialization policy.
Regarding the impact of contract farming, the existing literature has found positive effects on food security (Bellemare and Novak, 2017), rural transformation (ArounA et al., 2021), production efficiency (Bidzakin et al., 2020), and green production transition (Tan et al., 2022), etc. Many studies have found that contract farming has a positive impact on the income of participating farm households. Ruml et al. (2022) empirically analyzed the relationship between contract farming and farm household income in the oil palm sector in Ghana, and the results showed that sales and resource provision contracts significantly increased farm household income. Dubbert (2019) found that contract farming had a substantial effect on raising the income levels of small farmers than on medium and large farms. Miyata et al. (2009) conducted a comparative study on contract farming and non-contract farming farmers of apples and green onions in Shandong Province, China. They found that contract farming can help increase the income of smallholders. However, others believe that marketing contracts do not promote the income of farm households and that production-management contracts only promote the income of farm households who have difficulty obtaining bank loans (Soullier and Moustier, 2018). Participation in contract farming is conducive to stimulating employment, guaranteeing farmers’ credit, adopting new production technologies, and improving agricultural production efficiencies. However, this does not improve the quality of farm household income completely (Ragasa et al., 2018; Meemken and Bellemare, 2020). When farmers invest in specific assets and rely excessively on their contracted agricultural products, it leaves farmers with a lack of decision-making power and power of commercial decision-making, forcing them to accept unfair contract terms (Warning and Key, 2002).
To date, studies have analyzed contract farming from multiple perspectives. Still, there is no consensus on the impact of contract farming on farmers’ production technology efficiency and income, which requires further verification of the welfare effect of contract farming. From the perspective of agricultural production technical efficiency, this study explores the mechanism of contract farming to improve farmers’ income, which can provide valuable insights for policymakers in formulating policy tools to support the integrated development and coordinated development of agribusiness firms and farmers. These policy tools can improve agricultural performance, promote sustainable rural development, and ensure food security.
The primary objective of this study is to estimate the impact of contract farming on farm household income, using data from 610 rural households engaged in beef cattle breeding in Heilongjiang, Jilin, Inner Mongolia, and Hebei in China. More specifically, we not only differentiated the differences in the impact of different types of contract farming on farmers’ income but also analyzed the heterogeneous effect of contract farming on farmers’ income in terms of cohort differences in the number of breeding years, farm size, and training time. We also further reveal the mediating effect of agricultural production technical efficiency, which is helpful to clarify the formation mechanism of contract farming on farmers’ income increase.
We attempted to make three contributions to the literature. First, the existing studies mainly focus on the comparative analysis of contract farming and non-participating contract farming and less clearly distinguish the differences in the effects of different types of contract farming on farmers’ income. Second, in addition to estimating the impacts of contract farming for the total sample, we also examined the disaggregated effects by breeding years, farm size, and training time, providing deeper insights to improve our understanding. Third, in addition to analyzing the direct income impact of contract farming on farm households, we estimate the indirect effects of contract farming on farm household income through the mediating path of agricultural production technical efficiency.
The rest of the paper is structured as follows. Section 2 presents the hypothesis. Section 3 describes the materials and methods. Section 4 presents and discusses the empirical results. Section 5 concludes and discusses the policy implications.
2. Theoretical framework and research hypothesis
Problems such as the high cost of beef cattle breeding in China, rising prices year by year, and beef quality and safety still exist. Contract farming is an essential bridge between decentralized “small production” and the uncertain “big market.” It helps farmers connect to the markets and often helps them with credit, technology, information, factor inputs (Ton et al., 2018). Contract farming increases the farmer’s operating income by reducing transaction costs. Contract farming is generally classified into three categories: marketing contract, production-management contract, and resource-providing contract (Mighell and Jones, 1963; ArounA et al., 2021). In the marketing contract, the agribusiness firm stipulates the transaction’s price, quantity, timing, and product attributes, and farmers independently decide on production and operation. By guaranteeing the transaction price, the impact of market systemic risks on farmers is reduced (Hu, 2013). In the production-management contract, the agribusiness firm specifies the production methods. The farmer produces agricultural products that comply with contract standards, reducing technical uncertainty by optimizing production (Bellemare and Lim, 2018). The agribusiness firm sells production chain necessities to farmers on credit in the resource-providing contract. It provides service support in terms of finance and technology to obtain a predetermined quantity and quality of agricultural products. By alleviating financial input constraints, it encourages farmers to adopt modern agricultural production technology and improve agriculture performance (Ruml and Qaim, 2021). In general, regardless of the type of contract farming involved, it provides a good market environment for farmers and helps to enhance their operating income (Kumar et al., 2018). Hence, we propose that: (H1). Farmers who participate in contract farming have higher net income from cattle raising than those who do not.
In agricultural production activities, the impact of participation in contract farming on farmers’ income can vary significantly depending on farmer heterogeneity (Li and Guo, 2017). When beef cattle farmers participate in contract farming, they are affected by differences in their endowments, such as breeding years, farm size, and training time, resulting in differences in how contract farming enhances their income levels. In terms of breeding years, as the breeding years grow, beef cattle farmers will have more experience in breeding, thus contributing to income growth (Ding and Xiao, 2019). Regarding farm size, the differences in the degree of specialization, marketization, and commercialization of farmers of different beef cattle farm sizes make the income increase brought by the scale effect different (Mishra et al., 2016). In terms of training time, attending technical training can help farmers reduce business risks, obtain information and knowledge related to beef cattle breeding, and update modern production and management concepts, thereby improving beef cattle production efficiency and realizing the improvement of farmers’ operating income (Gray and Boehlj, 2005). However, differences in the approach, quality, and training content may bring about a differential impact of contract farming on improving farmers’ operating income. Hence, we propose that: (H2). Contract farming had a significant heterogeneous effect on the net income from cattle rearing of different groups of farmers in terms of breeding years, farm size, and training time.
Contract farming, which is China’s leading model of industrial agriculture, can reduce transaction costs and resolve market imperfections by linking smallholder farmers to markets (Mishra et al., 2020). Contract farming helps increase crop productivity and yield growth in the agricultural sector by delivering better technology (Swain, 2016). Furthermore, it promotes agricultural development by changing traditional decentralized family production methods and improving the technical efficiency of farmers’ production. Mishra et al. (2019) argued that as factor inputs increase, the technical efficiency of production also increases. However, the increased use of labor in contract farming decreases technical efficiency. Higher use of land, capital, and other inputs increases technical efficiency. Harianto et al. (2019) argued that the formality and intensity of contractual arrangements in contract farming have different efficiency effects on production, with broiler farms under formal and detailed contract farming having higher technical efficiency. Min et al. (2021) argued that contract farming is an excellent tool for improving rice production efficiency. Contract farming has brought great benefits by improving the technical efficiency of food growers’ production, reducing transaction costs, and reducing production risks (Bellemare and Bloem, 2018; Mishra et al., 2019). In summary, contract farming provides viable solutions to the problems encountered by smallholders and family farms in terms of production inputs, credit, technology, information, and market access, thereby increasing productivity and income (Dong et al., 2020). Hence, we propose that: (H3). Contract farming affects farmers’ net income from cattle rearing by agricultural production technical efficiency, which plays an intermediary role between Contract farming and farmers’ net income from cattle rearing.
Based on the above theoretical analysis and research hypothesis, Figure 1 demonstrates the impact of contract farming on farmers’ income and the mediating role of agricultural production technical efficiency.
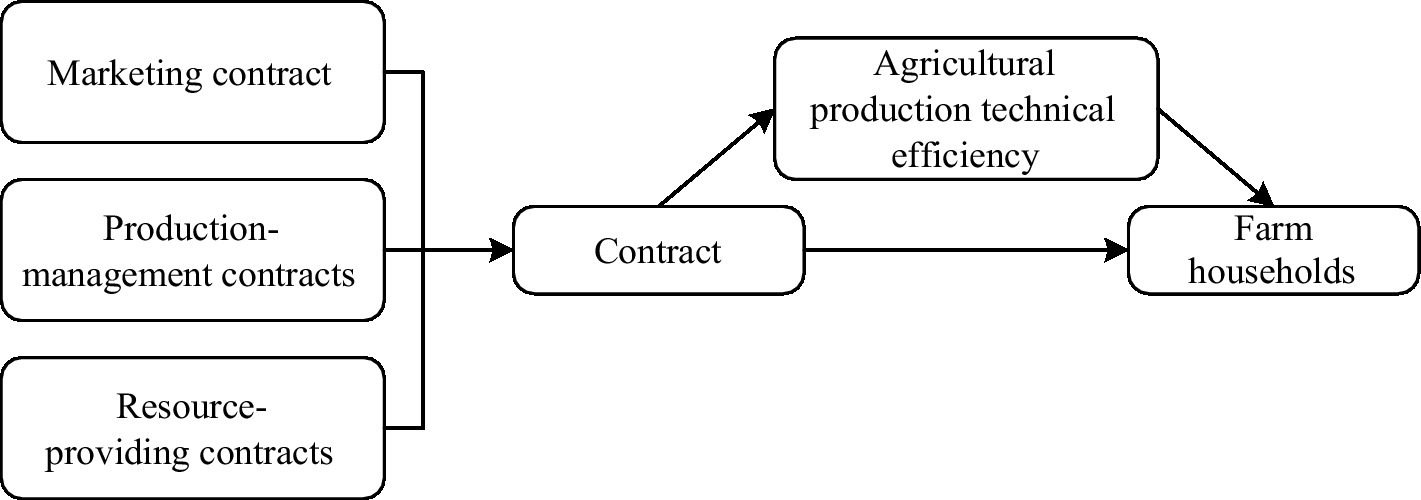
Figure 1. Mediating effects of agricultural production technical efficiency. Source: author collation.
3. Materials and methods
3.1. Data source
The data used in this study came from a household survey conducted from July 2020 to September 2021 in rural China. Due to budget constraints and other practical reasons, The data were collected through the questionnaire survey of the research group, from 4 provinces of Heilongjiang, Jilin, Inner Mongolia, and Hebei (As shown in Figure 2). The main reason for choosing this area is that these four provinces, respectively, include the dominant areas in the Northeast and Central Plains of China’s beef cattle production, and there are more farmers engaged in beef cattle production with a higher degree of organization. According to data from the China Rural Statistical Yearbook (2022), the proportion of beef cattle stock and beef production in 2021 was 15.17 and 25.63%, respectively, which are more representative and relevant. The content of the investigation includes the basic information of farmers, the operation of beef cattle breeding, and the participation of contract farming. The survey process adopts the methods of stratified sampling and random sampling. We randomly select three prefecture-level cities in Heilongjiang, Jilin, Inner Mongolia, and Hebei Province according to a certain proportion and scale from the two counties under the selected prefecture-level city, and then choose 1 to 2 townships, and then randomly select 1 to 2 administrative villages from each township. Finally, in each administrative village we randomly visited 6 to 8 farm households, conducted field visits and semi-structured interviews, and instructed them to fill in the questionnaire. A total of 641 questionnaires were obtained for this research, excluding those with missing data and logical errors, 610 valid questionnaires were received, with an efficiency rate of 95.16%. Regarding farmer participation in contract farming, 426 farmers participated in contract farming, 232 in marketing contracts, 115 in production-management contracts, and 79 in resource-providing contracts for empirical analysis.
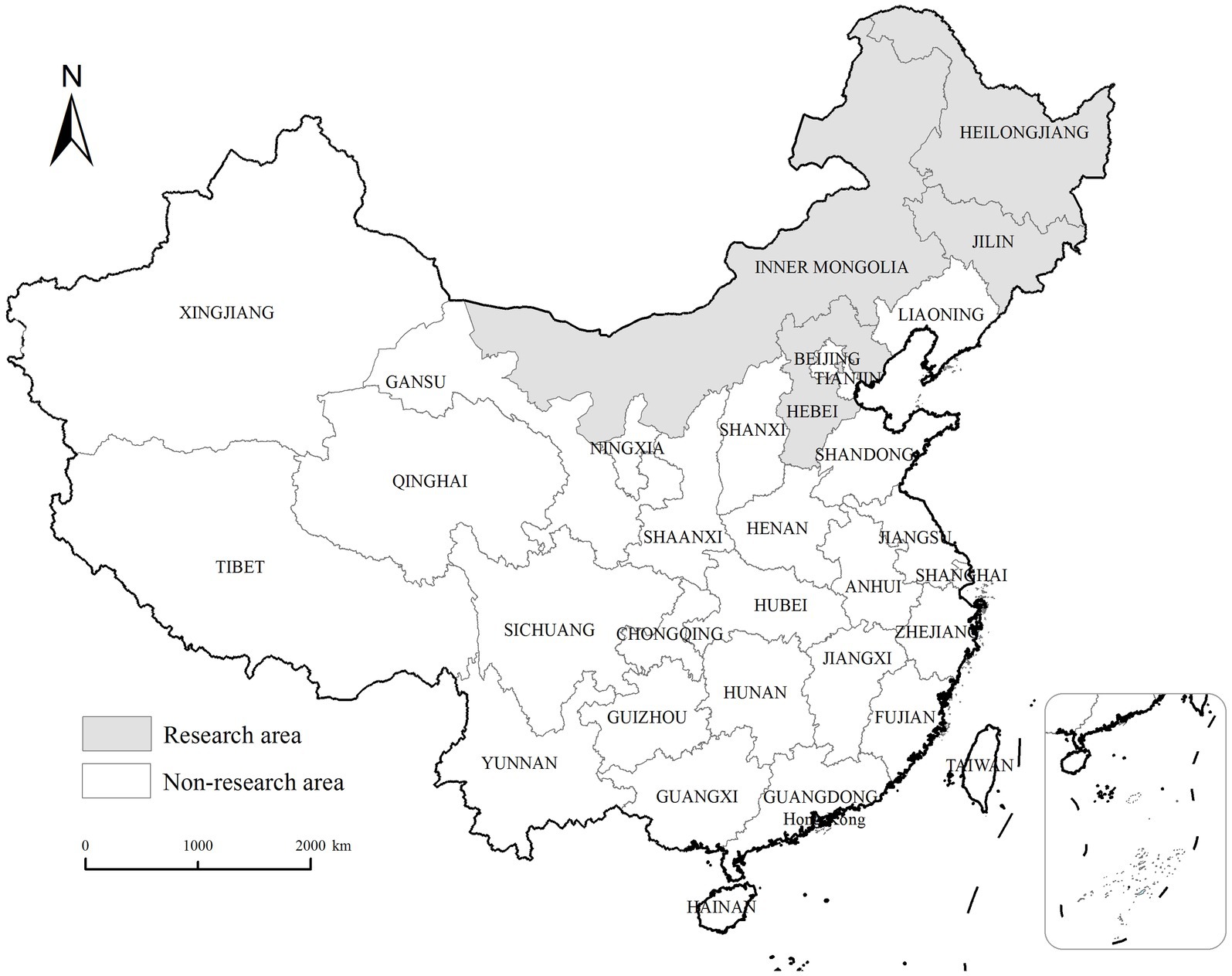
Figure 2. Spatial distribution of the study area. The base drawing is from the website of the ministry of natural resources (https://www.mnrgov.cn, (accessed on 12 October 2022)), the review drawing number is GS (2020) 462 L, and the base drawing has not been modified.
3.2. Variable definitions
3.2.1. Farm household income
As a large-scale business entity, the farming family usually aims to maximize profits. In this study, the annual net cattle income of farming households is selected as a measure, and the variable is continuous.
3.2.2. Contract farming
In this study, the counterfactual estimation method is chosen to analyze the treatment effects of contract farming on farmers’ income and the treatment effect of different types of contract farming on farmers’ income. Therefore, contract farming and three types of contract farming are dummy variables. A value of 1 is assigned if the sample farmer participates in contract farming, marketing contracts, production-management contracts, and resource-providing contracts; a value of 0 is assigned if the sample farmer does not participate in contract farming.
3.2.3. Agricultural production technical efficiency
In this study, technical efficiency of agricultural production was used as a mediating variable. Considering the reality of beef cattle production activities, this study used a stochastic frontier approach to measure the technical efficiency of beef cattle farmers. The output variable was the average weight of beef cattle slaughtered (kg/head), and the input variables included calf weight (kg/head), feed inputs (yuan/head), labor inputs (yuan/head), and other material inputs (yuan/head). Descriptive statistics for the input–output variables of beef cattle farming are shown in Table 1.
The technical efficiency of production of the sample farmers is estimated using the transcendental logarithmic stochastic frontier analysis (SFA). Due to the random phenomenon in beef cattle production activities, SFA considers the effect of random errors on individual efficiency and avoids the bias of nonparametric methods due to unexpected factors. Therefore, we choose the relatively flexible and variable elasticity of substitution translog production function to perform stochastic frontier analysis. According to the research of Cuesta et al. (2009) the specific functional form is as follows:
where refers to the beef cattle production of the farmer, , , and refers to the calf input, feed input, labor input and other material input of the farmer, respectively; β refers to the parameters to be estimated for the primary, interaction, and squared terms of the calf input, feed input, labor input and other material input.
The level of technical efficiency of each beef farmer is measured by comparing the observed output with the output of the stochastic frontier. According to the research of Bidzakin et al. (2020), the formula for measuring the technical efficiency of beef cattle farmers’ production is as follows:
where refers to the optimal output that may be achieved for a given level of inputs. takes the value between 0 and 1. The closer to 1 means the higher the technical efficiency of production of beef cattle farmers, and the closer to 0 means the lower the production technical efficiency of beef cattle farmers.
3.2.4. Control variables
To clarify the factors influencing farmers’ participation in contract farming, the effect of contract farming on farmers’ net income from cattle farming is further measured. In this study, individual characteristics, household endowment characteristics, and production and operation characteristics of beef cattle farmers are selected as control variables, considering the sample area and the characteristics of beef cattle production activities and drawing on existing research results. It mainly includes age, education, breeding years, breeding number, farm size, training time, formal loans, operating inputs, specific investments, and ease of selling beef cattle.
Table 2 presents variable definitions and descriptive statistics. It shows that the average net income from cattle farming is higher among the surveyed farmers, and the income gap is more extensive. Meanwhile, 74.9% of the sample farmers participated in contract farming, of which 36.9, 22, and 16% participated in marketing contracts, production-management contracts, and resource-providing contracts, respectively.
3.3. Empirical model
3.3.1. Propensity score matching
In order to analyze the impact of participating in contract farming on farmers’ income, it is necessary to observe the differences between uniform subjects in two states (participants and non-participants). However, this cannot be directly observed because at any one time the farmers either participating or does not participate in contract farming to sell agricultural products. This means counterfactual outcomes are not observable. However, the research objective can be achieved by finding a control group of non-participating agricultural farmers whose characteristics are very similar to the participating farmers (Mojo et al., 2017; Michalek et al., 2018). Under the conditions of non-experimental intervention, farmers’ decision whether to participate in contract farming as a way of beef cattle production and management is not random, and selection bias should be considered (Liu et al., 2019). Therefore, we employ propensity score matching (PSM) to study the effect of contract farming on the income of beef cattle farmers. The specific analysis steps are as follows:
Step 1 is to calculate the propensity score. The logit model was used to calculate the probability of the farmer’s participation in contract farming, marketing contract, production-management contract, and resource-providing contract. The propensity score of sample i as indicated in the following equation:
where PS represents the propensity score which denotes the conditional probability of the farmer choosing the treatment group (contract farming, marketing contract, production-management contract, and resource-providing contract) given (covariate). represents dummy variables for farmer participation in contract farming. represents the participation of farmers i in contract farming, marketing contract, production-management contract, resource-providing contract. Where represents that farmers i are involved in contract farming (treatment group 1); represents that farmers i are involved in marketing contract (treatment group 2); represents that farmers i are involved in production-management contract (treatment group 3); represents that farmers i are involved in resource-providing contract (treatment group 4); represents that farmers i do not participate in contract farming (control group). represents observable individual characteristics, household endowment characteristics and production management characteristics of beef cattle farmers (Guo and Jolly, 2008; Ding and Xiao, 2019).
Step 2 is propensity score matching. The treatment group comprised farmers who participated in contract farming, marketing contract, production-management contract, and resource-providing contract, whereas those who did not participate in contract farming were assigned to the control group. The estimated propensity scores were utilized to match the treatment group to the control group with similar observed characteristics. First, the selection of matching method is carried out. Because each algorithm has drawbacks and strengths, it is instructive to use multiple algorithms to estimate treatment effects and to check the robustness of the results (Shumeta and D’Haese, 2016; Minah, 2022). In order to ensure the reliability of the matching results, we apply four matching methods, including nearest neighbor matching, caliper matching, nearest neighbor matching within the caliper, and kernel matching, which are selected in the study for matching, respectively. Then, the balancing test is applied. Once propensity score estimates are obtained, the quality of matching needs to be judged by comparing whether there is a significant difference between the treatment and control groups on the covariates .
Step 3 calculates the average treatment effect. Using PSM, one can estimate three types of treatment effects: the average treatment effects on the treated (ATT), the average treatment effects on the untreated (ATU), and the average treatment effects (ATE) (Khandker et al., 2009). However, Wang et al. (2017) pointed out that researchers and policymakers are more interested in explicitly assessing the effect of the interventions on those who actually received the intervention, i.e., they are interested in the estimation of ATT. We focus primarily on the contribution of farmers’ participation in contract farming to their net cattle income, and thus compare the three types of contract farming treatment groups. Therefore, only the estimated results of the average treatment effects on the treated (ATT) are explored. The specific calculation formula is as follows:
where represents the number of farmers in the treatment group j. represents the number of farmers involved in contract farming; represents the number of farmers involved in marketing contracts; represents the number of farmers involved in production-management contract; represents the number of farmers involved in resource-providing contract. represents summation over the treatment group j; represents the farm household cattle income of farmers in group j; represents the estimated farm household cattle income of farmers in the control group matched to the treatment group.
3.3.2. Mediating effect model
To explore the impact of contract farming and its three contractual arrangements on beef cattle farmers’ incomes, this paper tests whether the technical efficiency of production plays a mediating role between the two. The specific formula is as follows:
where Y is the net income from family cattle farming; X is contract farming, marketing contracts, production-management contracts, and resource-providing contracts; M is the technical efficiency of production; and Z is the control variable. c1 is the regression coefficient of contract farming, marketing contracts, production-management contracts, and resource-providing contracts on beef cattle farmers’ net household income from cattle farming. c2 is the regression coefficient of contract farming, marketing contracts, production-management contracts, and resource-providing contracts on the technical efficiency of production. c3 is the regression coefficient of contract farming, marketing contracts, production-management contracts, and resource-providing contracts on beef cattle farmers’ net household income from cattle farming after introducing the mediating variables. b is the regression coefficient of the technical efficiency of production on the net income of farmers’ households from cattle farming, α1, α2 and α3 denote the constant terms, and ε1, ε2 and ε3 denote the random error terms.
4. Empirical results
4.1. Comparative analysis of production technology efficiency
In this study, Froniter4.1 software was used to analyze the set stochastic frontier model, and Stata15.1 was used to measure the production technical efficiency of beef cattle farmers. The measurement results are listed in Table 3. The average technical efficiency of production reached 0.902 and 0.817 for those beef cattle farmers that participated and did not participate in contract farming, respectively. This finding indicates that participation in contract farming can improve the technical efficiency of beef cattle farmers’ agricultural production. Similarly, the average technical efficiency of beef cattle farmers participating in resource-providing contracts is the highest, followed by production-management contracts and marketing contracts. This indicates that resource-providing contracts effectively improve the technical efficiency of beef cattle farmers’ agricultural production by a large margin. The efficiency of the production technology distribution of participating contract farming beef cattle farmers is significantly more concentrated, with beef cattle farmers with a production technology efficiency greater than 0.9 cumulatively accounting for 59.86% of the sample beef cattle farmers. This suggests that participation in contract farming has increased the tendency for beef cattle farmers to centralize the technical efficiency of their agricultural production.
4.2. Determinants of participation in contract farming
To achieve sample matching, we analyzed the factors influencing farmer participation in contract farming based on a logit model. The estimation results in Table 4 show that training time and operational inputs significantly positively affect farmers’ participation in contract farming. In contrast, the effect of breeding years is significantly negative. Among them, training time and operational inputs have a significant positive effect on farmers’ participation in marketing contracts, while the effect of breeding years and formal loans is significantly negative. Training time, operational inputs, specific investments, and ease of selling beef cattle significantly positively affect farmers’ participation in production-management contracts. Age, education, training time, formal loans, operational inputs, and ease of selling beef cattle significantly positively affect farmers’ participation in resource-providing contracts, while breeding years are significantly negative.
4.3. Balancing test
Another critical assumption underlying the validity of PSM is the balance of the pre-existing variables between the treatment and the control groups after the matching. Since different matching methods produce different amounts of sample loss, to ensure the robustness of the matching results, we chose to use the following four matching methods, nearest neighbor matching, caliper matching, nearest neighbor matching within the caliper, and kernel matching. The results of the balancing test are given in Table 5. After sample matching by the four methods, the mean deviations of the matched covariates were significantly reduced compared with those before matching, and they were all within 20% below; Pseudo-R2 and LR statistics were reduced considerably compared to before matching. Thus, the propensity score matching method significantly reduced the differences in covariates between the four treatment and control groups and essentially eliminated the bias due to sample self-selection.
4.4. Income effect of contract farming on farmers
From the estimation results in Table 6, it is clear that after controlling for differences in a range of observable variables using PSM, the four estimates were consistent, and ATT passes the test at least at the 5% significance level. Regarding averages, farmers who do not participate in contract farming have a net income from cattle farming of 4.154; however, their net income from cattle farming increased to 4.789 with a growth rate of 15.32% due to participation in contract farming. This shows that participation in contract farming significantly contributes to farmers’ net income from cattle raising, thus verifying hypothesis 1. In addition, we further focused on the extent of the impact of the three contract farming types on the growth of farmers’ net cattle income. Regarding averages, participation in production-management contracts significantly increased the net income of farmers raising cattle, growing to 4.803 with a growth rate of 13.82%. Participation in marketing contracts also significantly increased the net income of farmers raising cattle, growing to 4.702 with a growth rate of 14.91%. However, participation in resource provision contracts failed to significantly increase the level of net income from cattle farming for farmers. It can be seen that among the three contract farming types, both marketing contracts and production-management contracts can significantly increase the level of net income of cattle farmers, with production-management contracts having a greater degree of impact. However, resource-providing contracts cannot significantly increase farmers’ income levels.
The results have some similarities with the conclusions of other contract farming studies, such as those of Qaim et al. (2020) and Barrett et al. (2012). Their research emphasizes that possible reasons are that participation in marketing contracts helps mitigate the impact of market price risk on farmers. For farmers involved in production-management contracts, the firm provides technical training, market information, financing, and loans, which help them complete their order contracts and significantly enhance their operational capabilities, resulting in high value-added income. Although resource-providing contracts cannot significantly increase farmers’ income levels. This conclusion is supported by Vamuloh et al. (2020) and Briones (2015). They emphasize farmers involved in resource-providing contracts have established a closer benefit linkage mechanism with the firm, they are still in a vulnerable position regarding the firm’s monopolistic exploitation, unfair agricultural trade, and demand for high-quality agricultural products, and their profit level is highly susceptible to the company’s opportunistic behavior.
4.5. Sensitivity analysis
Observable variables were controlled for by the propensity score matching (PSM) method to mitigate the selectivity bias problem. Still, the presence of unobservable variables may have led to hidden bias. To address this issue, we verify the robustness of the results above using the bounds sensitivity analysis proposed by Rosenbaum (2002). The Gamma coefficient indicated the effect of unobservable variables on the net income of cattle farmers. The main observation was that the PSM estimation results were more reliable. The findings become insignificant only when the Gamma coefficient had a significant value (usually close to 2). According to the estimation results shown in Table 7, when the Gamma coefficient increased to 2.5, the upper bound of the Wilcoxon signed rank test significance (sig +) remained significant at the 1% level. None of the Hodges-Lehmann point estimates (t - hat + and t - hat -) at the 95% confidence interval (CI + and CI -) contained a value of 0. This suggests that the propensity score matching estimates were less sensitive to these omitted factors and that the conclusion that participation in contract farming is beneficial in enhancing farmers’ net income from cattle farming is robust and reliable.
4.6. Heterogeneous effects of participation in contract farming
The propensity score matching (PSM) method was used to examine the effect of contract farming on farmers’ net cattle income. Still, this estimate only reflects the mean increase in net cattle income from farmers’ participation in contract farming. Therefore, we grouped farmers according to breeding years, farm size, and training time to analyze the differences in the impact of farmers’ participation in contract farming on net income from cattle farming for different endowment characteristics. To ensure the effectiveness of the analysis, the mean values of the grouping variables were first calculated. Then the two groups of sample farmers with “greater than the mean” and “less than the mean” were compared and analyzed. The results of ATT estimation based on the nearest neighbor matching (n = 4) to estimate the comparison of group differences in the income effects of contract farming on farmers are shown in Table 8.
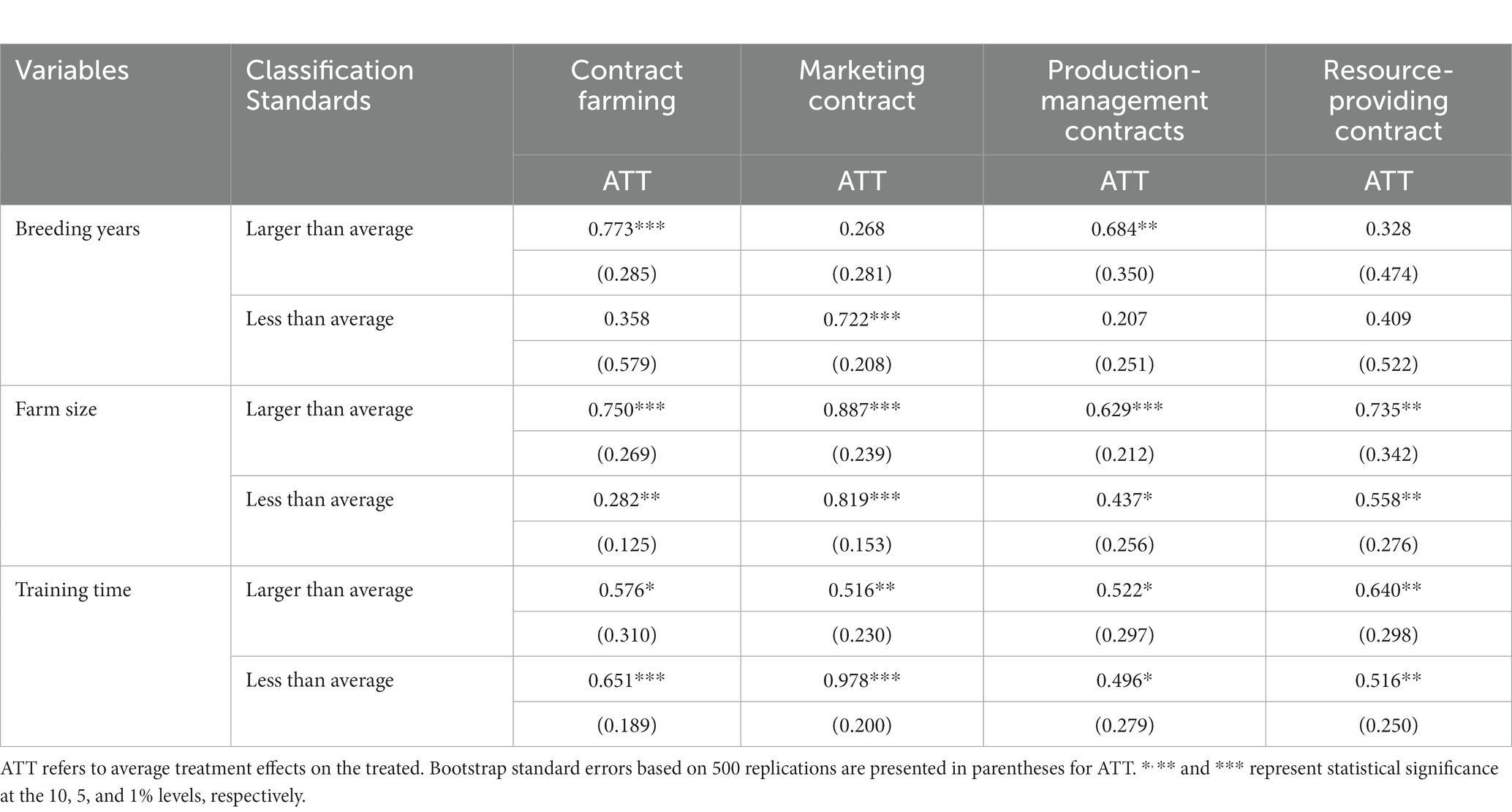
Table 8. Heterogeneity analysis of income effects of different groups participating in contract farming.
By analyzing the heterogeneity of farmers’ participation in contract farming on their income under different endowment characteristics. It showed that the effect of contract farming on farmers’ net income from cattle farming was significantly different among the cohorts with different breeding years, farm sizes, and training time. This finding had similarity with the studies of Gray and Boehlj (2005), Hou et al. (2018), Ding and Xiao (2019). Farmers with longer breeding years experience a significant increase in net income from cattle farming after participating in contract farming. Among them, farmers involved in marketing contracts with shorter breeding years and those involved in production-management contracts with longer breeding years were able to increase their net income from cattle farming significantly. Participation in contract farming has a more significant effect on larger farmers’ net income from cattle farming. Similarly, farmers who participated in contract farming training relatively less often had a more substantial effect on their net income from cattle farming. This indicates that training time should be pursued more than just in quantity but also in training methods, content, and quality. It is only in high-quality beef cattle breeding training that the net income of cattle farmers can be effectively improved. Thus, hypothesis 2 was verified.
4.7. Results of mediating effect analysis
Previous empirical literature has studied the effect of contract farming on the technical efficiency of farmers’ production and on farmers’ income. Accordingly, we used a mediating effects model to test the transmission path of the technical efficiency of agricultural production on the effect of contract farming on farm household income. The estimation results are presented in Table 9. First, as shown in columns (2), (5), (8), and (11), contract farming and all three contractual arrangements positively affect the technical efficiency of farmers’ production and are statistically significant at the 1% level. Compared to the planting industry, animal husbandry requires more inputs of production factors; however, the beef cattle production process is characterized by a long cycle, high cost, consumption, pollution, etc., and is subject to more resource environmental and technological constraints. Contract farming can provide farmers with credit, technology, information, and factor inputs. This helps reduce production risks, improve yields, and promote specialization, thereby increasing farmers’ incomes and profits and ensuring food security. This indicates that participation in contract farming can effectively improve the technical efficiency of farmers’ production. This finding is consistent with Dubbert (2019), Harianto et al. (2019), and Mishra et al. (2019) regarding impact of contract farming on the production efficiency of farm households.
The mediating effect of agricultural technical efficiency on farmers’ net income from cattle farming in different contract farming types was also verified. Contract farming partially mediated farmers’ income, with significant production technical efficiency as a mediating variable, accounting for 14.7% of the total effect. Similarly, there was a partial mediating effect with significant production technical efficiency as a mediating variable in the marketing contract, production-management contract, and production-management contract affecting farmers’ income, and the proportion of this mediating effect to the total effect was 22.5, 47.7, and 53.3%, respectively. Thus, hypothesis H3 was verified. The above findings suggest that contract farming not only directly affects farmers’ income but also indirectly affects farmers’ income through production technical efficiency. This finding is in line with findings by Guo and Wu (2022) on farmer participation in contract farming in the Chinese plantation sector. This means that improvements in production technical efficiency are a critical factor for farmers to benefit from contract farming, and the benefits of participating in resource-providing contracts are more significant for farmers.
5. Conclusions and policy implications
5.1. Conclusion
Contract farming is an essential tool to help farmers share market risk, help reduce risk and transaction costs for buyers and sellers, and link markets through inputs and credits. It mitigats problems such as capital constraints, loan defaults, and other credit market failures. This paper contributes to the limited evidence on the causal impact of contract farming on the income of beef cattle farmers, using data collected from Heilongjiang, Jilin, Inner Mongolia, and Hebei provinces in China. In terms of substance, we go beyond analyzing the effect of contract farming on farmers’ income by examining the impact of different types of contract farming on farmers’ income. We further analyzed the mediating effects of agricultural production technical efficiency in the influence of contract farming on farmers’ income. We employed the PSM model as an empirical strategy to address the selection bias caused by observed and unobserved factors.
The study results show that contract farming plays a significant role in enhancing farmers’ income. Specifically, farmers’ participation in marketing contracts and production-management contract increased their income by 13.82 and 14.91%, respectively, and resource-providing contracts did not significantly enhance farmers’ income levels. Disaggregated analysis reveals that the extent to which participation in contract farming enhances farmers’ income can be influenced by factors such as breeding years, farm size, and training time. In contrast, farmers with longer breeding years, larger farm sizes, and less training time had a higher degree of income enhancement from contract farming. Finally, contract farming can effectively improve farmers’ technical efficiency, and the technical efficiency concentration trend is enhanced by participation in contract farming. Meanwhile, the analysis of mediating effects showed that contract farming indirectly affects farmers’ income through the mediating path of agricultural production technical efficiency, with a mediating effect of 14.7%. Specifically, agricultural production technical efficiency significantly raised farmers’ income through marketing contracts, production-management contracts, and resource-providing contracts, with the mediating effects accounting for 22.5, 47.7, and 53.3%, respectively.
5.2. Policy implications
Based on the study results, we propose some policy implications for the sustainable development of contract farming. The critical role of contract farming in increasing farm households’ income and the technical efficiency of agricultural production suggests that policies to promote smallholders’ participation in contract farming and increase organization are sensible. This is particularly important for China and several production constraints and food security challenges. The government should actively create favorable conditions to encourage and guide the development of “firm + smallholder” contract farming and should continue to provide policy support for contract farming. It should be clear that smallholders are disadvantaged in market competition. In addition to traditional policy support, such as new technology training and financial aid, the focus should be on increasing the degree of organization of farmers. In addition, the government needs to encourage and support smallholders to engage in deeper cooperation with companies. According to the characteristics of farmers’ resource endowment, the contract is formulated in a targeted manner. The government should gradually improve the mechanism for distributing benefits between companies and farmers, expand the industrial, value, and benefit chains, and promote win-win cooperation and benefit-sharing among various business entities while fully tapping and improving the efficiency of agricultural operations. Policy directives to promote contract production will be the right direction, as it will help farmers maximize their agricultural production. Improving production efficiency can increase agriculture yield and improve farmers’ income and gross margin. Contract farming is a management strategy or tool worth practicing. Beef cattle farmers should strengthen their own comprehensive ability. On the one hand, beef cattle farmers should actively participate in training and learning new knowledge, enhance their own cognitive capabilities, and rationally identify industrial organization policies and market information, master social resources information, and improve labor productivity. On the other hand, according to the resource characteristics of different beef cattle farmers, guide beef cattle farmers to organize,contractualization and institutionalization, improve the operating capabilities of farmers, there by reducing transaction costs, improve farmers’ evaluation of contract farming and promote the stability of agricultural contracts. Most importantly, beef cattle farmers should minimize marginal costs and increase their advantages by improving technical efficiency to break the technological gap, thereby capturing more market share and maximizing profit.
5.3. Limitations
Finally, this study only examines the increase in income at one point in time in the relationship between contract farming and farm household income without considering the bias caused by temporal dynamics. Therefore, the evolution of the relationship between contract farming, agricultural production technical efficiency, and farm household income over time needs to be considered in future studies. In addition, this study focuses on household-level data; we do not have plot-level data. Thus, when the required plot-level data are available, future studies may investigate how farmers’ plot-level participation in contract farming affects the economic performance of farm households and rural household welfare.
Data availability statement
The original contributions presented in the study are included in the article/supplementary material, further inquiries can be directed to the corresponding author.
Author contributions
YL and WB conceived, designed the study, and completed the original manuscript. YL and YZ reviewed and revised the original manuscript and provided funding and resources.
Funding
This research was funded by National Beef-cattle Industrial Technology System and Industrial Economy Research Project under the Ministry of Agriculture in the People’s Republic of China (PRC) (Grant no CARS-37), Jilin Provincial Agricultural Economics Association special project (Grant no 2021ZX02), Ministry of Education of Humanities and Social Science Research Project of China (Grant no: 16YJA790057), Jilin Province Science and Technology Development Project (Grant no 20210601039FG).
Acknowledgments
The authors wish to acknowledge Jilin Agricultural University and Henan Agricultural University for Research, Innovation, and Discovery for making the publication of this article in an open access journal possible. We also thank the reviewers for their helpful and extensive comments that contributed to a greatly improved manuscript.
Conflict of interest
The authors declare that the research was conducted in the absence of any commercial or financial relationships that could be construed as a potential conflict of interest.
Publisher’s note
All claims expressed in this article are solely those of the authors and do not necessarily represent those of their affiliated organizations, or those of the publisher, the editors and the reviewers. Any product that may be evaluated in this article, or claim that may be made by its manufacturer, is not guaranteed or endorsed by the publisher.
References
ArounA, A., Michler, J. D., and LokossoU, J. C. (2021). Contract farming and rural transformation: evidence from a field experiment in Benin. J. Dev. Econ. 151:102626. doi: 10.1016/j.jdeveco.2021.102626
Barrett, C. B., Bachke, M. E., Bellemare, M. F., Michelson, H. C., Narayanan, S., and Walker, T. F. (2012). Smallholder participation in contract farming: comparative evidence from five countries. World Dev. 40, 715–730. doi: 10.1016/j.worlddev.2011.09.006
Bellemare, M. F., Barrett, C. B., and Just, D. R. (2013). The welfare impacts of commodity price volatility: evidence from rural Ethiopia. Am. J. Agric. Econ. 98, 676–678. doi: 10.1093/ajae/aav070
Bellemare, M. F., and Bloem, J. R. (2018). Does contract farming improve welfare? A review. World Dev. 112, 259–271. doi: 10.1016/j.worlddev.2018.08.018
Bellemare, M. F., and Lim, S. (2018). In all shapes and colors: varieties of contract farming. Appl. Econ. Perspect. Policy 40, 379–401. doi: 10.1093/aepp/ppy019
Bellemare, M. F., and Novak, L. (2017). Contract farming and food security. Am. J. Agric. Econ. 99, 357–378. doi: 10.1093/ajae/aaw053
Bi, W., Song, Y., Liu, Y., Li, Z., and Zhang, Y. (2022). Food consumption structure and food security—through mediating effect analysis of Agricultural R&D and agricultural investment. Int. J. Environ. Res. Public Health 19:12524. doi: 10.3390/ijerph191912524
Bidzakin, J. K., Fialor, S. C., Awunyo-Vitor, D., and Yahaya, I. (2020). Contract farming and rice production efficiency in Ghana. Journal of Agribusiness in Developing and Emerging Economies. 10, 269–284. doi: 10.1108/JADEE-11-2018-0160
Briones, R. M. (2015). Small farmers in high-value chains: binding or relaxing constraints to inclusive growth? World Dev. 72, 43–52. doi: 10.1016/j.worlddev.2015.01.005
Cuesta, R. A., Lovell, C. A. K., and Zofío, J. L. (2009). Environmental efficiency measurement with translog distance functions: a parametric approach. Ecol. Econ. 68, 2232–2242. doi: 10.1016/j.ecolecon.2009.02.001
Ding, C. Z., and Xiao, H. (2019). Transaction Characteristics,Farmers’ industrial organization model selection and income growth: an analysis based on multivariate logit model and MTE model. J. Nanjing Agric. Univ. 5, 130–142. doi: 10.19714/j.cnki.1671-7465.2019.0078
Dong, D. D., Moritaka, M., Liu, R., and Fukuda, S. (2020). Restructuring toward a modernized agro-food value chain through vertical integration and contract farming: the swine-to-pork industry in Vietnam. J. Agribusiness Dev. Emerging Eco. 10, 493–510. doi: 10.1108/jadee-07-2019-0097
Dubbert, C. (2019). Participation in contract farming and farm performance: insights from cashew farmers in Ghana. Agric. Econ. 50, 749–763. doi: 10.1111/agec.12522
Gray, A. W., and Boehlj, M. D. (2005). Risk sharing and transactions costs in producer-processor supply chains. Choices 20, 281–286.
Guo, H., and Jolly, R. W. (2008). Contractual arrangements and enforcement in transition agriculture: theory and evidence from China. Food Policy 33, 570–575. doi: 10.1016/j.foodpol.2008.04.003
Guo, X. B., and Wu, F. (2022). Can contract farming effectively improve the technical efficiencyand income of family farms?: propensity score matching analysis based on questionnairedata from 812 farming family farms. J. Agrotech. Eco. 12, 53–62. doi: 10.13246/j.cnki.jae.20220111.001
Harianto, H., Kusnadi, N., and Paramita, D. A. (2019). The impact of vertical integration intensity on broiler farms technical efficiency: the case of contract farming in west Sumatera. Trop. Ani. Sci. J. 2, 167–174. doi: 10.5398/tasj.201942.2.167
Hou, J., Ying, R., and Zhou, L. (2018). Does contract farming effectively improve farmers 'Income?An empirical research based on quantile regression. J. Nanjing Agric. Univ. 3, 122–132. doi: 10.1671/j.cnki.1671-7465/f.2018.03.0122
Hu, W. Y. (2013). Effect of contract farming on the US crop farmers’ average return. Agric. Econ. 59, 195–201. doi: 10.17221/100/2012-agricecon
Khan, M. F., Nakano, Y., and Kurosaki, T. (2019). Impact of contract farming on land productivity and income of maize and potato growers in Pakistan. Food Policy 85, 28–39. doi: 10.1016/j.foodpol.2019.04.004
Khandker, S. R., Koolwal, G. B., and Samad, H. A. (2009). Handbook on impact evaluation: Quantitative methods and practices. World Bank Publications. Washington, D.C
Kumar, A., Roy, D., Tripathi, G., Joshi, P. K., and Adhikari, R. P. (2018). Does contract farming improve profits and food safety? Evidence from tomato cultivation in Nepal. J. Agribusiness Dev. Emerging Eco. 8, 603–624. doi: 10.1108/JADEE-09-2017-0095
Li, L., and Guo, H. (2017). The impact of industrial organization models on farmers’ production income: the case of vegetable farmers in Hebei and Zhejiang provinces. Chinese Rural Eco. 9, 62–79. doi: 10.13246/j.cnki.jae.20170928.005
Li, X., Ma, X., Wang, M., and Zhang, H. (2022). International evaluation of China’s beef cattle industry development level and lagging points. Agriculture 12:1597. doi: 10.3390/agriculture12101597
Li, X. Z., Yan, C. G., and Zan, L. S. (2018). Current situation and future prospects for beef production in China-a review. Asian Australas. J. Anim. Sci. 31, 984–991. doi: 10.5713/ajas.18.0212
Liu, Y., Ma, W., Renwick, A., and Fu, X. (2019). The role of agricultural cooperatives in serving as a marketing channel: evidence from low-income regions of Sichuan province in China. Int. Food Agribusiness Manag. Rev. 22, 265–282. doi: 10.22434/ifamr2018.0058
Luo, C., and Gao, Q. (2022). The operation mode of new order agriculture and its high-quality development path under the background of rural revitalization strategy. Macroeconomics. 5, 94–103. doi: 10.16304/j.cnki.11-3952/f.2022.05.014
Meemken, E. M., and Bellemare, M. F. (2020). Smallholder farmers and contract farming in developing countries. Proc. Natl. Acad. Sci. 117, 259–264. doi: 10.1073/pnas.1909501116
Michalek, J., Ciaian, P., and Pokrivcak, J. (2018). The impact of producer organizations on farm performance: the case study of large farms from Slovakia. Food Policy 75, 80–92. doi: 10.1016/j.foodpol.2017.12.009
Mighell, R. L., and Jones, L. A. (1963). Vertical coordination in agriculture. Farm Economics Division, Economic Research Service, US Department of Agriculture, Washington, D.C.
Min, S. H., Paudel, K. P., and Chen, F. B. (2021). Mechanization and efficiency in rice production in China. J. Integr. Agric. 20, 1996–2008. doi: 10.1016/S2095-3119(20)63439-6
Minah, M. (2022). What is the influence of government programs on farmer organizations and their impacts? Evidence from Zambia. Annals Public Cooperative Eco. 93, 29–53. doi: 10.1111/apce.12316
Mishra, A. K., Kumar, A., Joshi, P. K., and D'souza, A. (2016). Impact of contracts in high yielding varieties seed production on profits and yield: the case of Nepal. Food Policy 62, 110–121. doi: 10.1016/j.foodpol.2016.05.005
Mishra, A. K., Rezitis, A. N., and Tsionas, M. G. (2019). Estimating technical efficiency and production risk under contract farming: a Bayesian estimation and stochastic dominance methodology. J. Agric. Econ. 70, 353–371. doi: 10.1111/1477-9552.12291
Mishra, A. K., Rezitis, A. N., and Tsionas, M. G. (2020). Production under input endogeneity and farm-specific risk aversion: evidence from contract farming and Bayesian method. Eur. Rev. Agric. Econ. 47, 591–618. doi: 10.1093/erae/jbz027
Miyata, S., Minot, N., and Hu, D. (2009). Impact of contract farming on income: linking small farmers, packers, and supermarkets in China. World Dev. 11, 1781–1790. doi: 10.1016/j.worlddev.2008.08.25
Mojo, D., Fischer, C., and Degefa, T. (2017). The determinants and economic impacts of membership in coffee farmer cooperatives: recent evidence from rural Ethiopia. J. Rural. Stud. 50, 84–94. doi: 10.1016/j.jrurstud.2016.12.010
Qaim, M., Sibhatu, K. T., Siregar, H., and Grass, I. (2020). Environmental, economic, and social consequences of the oil palm boom. Ann. Rev. Resour. Econ. 12, 321–344. doi: 10.1146/annurev-resource-110119-024922
Ragasa, C., Lambrecht, I., and Kufoalor, D. S. (2018). Limitations of contract farming as a pro-poor strategy: the case of maize outgrower schemes in upper West Ghana. World Dev. 102, 30–56. doi: 10.1016/j.worlddev.2017.09.008
Ruml, A., and Qaim, M. (2021). New evidence regarding the effects of contract farming on agricultural labor use. Agric. Econ. 52, 51–66. doi: 10.1111/agec.12606
Ruml, A., Ragasa, C., and Qaim, M. (2022). Contract farming, contract design and smallholder livelihoods. Aust. J. Agric. Resour. Econ. 66, 24–43. doi: 10.1111/1467-8489.12462
Shumeta, Z., and D’Haese, M. (2016). Do coffee cooperatives benefit farmers? An exploration of heterogeneous impact of coffee cooperative membership in Southwest Ethiopia. Int. Food Agribusiness Manag. Rev. 19, 37–52. doi: 10.22434/ifamr2015.0110
Soullier, G., and Moustier, P. (2018). Impacts of contract farming in domestic grain chains on farmer income and food insecurity. Contrasted evidence from Senegal. Food Policy 79, 179–198. doi: 10.1016/j.foodpol.2018.07.004
Swain, B. B. (2016). Does technological linkage in contract farming increase farm productivity and efficiency? The case of hybrid paddy seed cultivation in undivided Andhra Pradesh. Agric. Econ. Res. Rev. 29, 211–224. doi: 10.5958/0974-0279.2016.00049.5
Tan, Y. F., Lu, Q., and Zhang, S. (2022). Can contract farming promote farmers’ green production transition? J. Agrotechnical Eco. 7, 16–33. doi: 10.13246/j.cnki.jae.20220428.001
Ton, G., Vellema, W., Desiere, S., Weituschat, S., and D'Haese, M. (2018). Contract farming for improving smallholder incomes: what can we learn from effectiveness studies? World Dev. 104, 46–64. doi: 10.1016/j.worlddev.2017.11.015
Vamuloh, V. V., Kozak, R. A., and Panwar, R. (2020). Voices unheard: barriers to and opportunities for small farmers’ participation in oil palm contract farming. J. Clean. Prod. 275:121955. doi: 10.1016/j.jclepro.2020.121955
Wang, A., Nianogo, R. A., and Arah, O. A. (2017). G-computation of average treatment effects on the treated and the untreated. BMC Med. Res. Methodol. 17, 1–5. doi: 10.1186/s12874-016-0282-4
Wang, H. H., Wang, Y., and Delgado, M. S. (2014). The transition to modern agriculture: contract farming in developing economies. Am. J. Agric. Econ. 96, 1257–1271. doi: 10.1093/ajae/aau036
Warning, M., and Key, N. (2002). The social performance and distributional consequences of contract farming: an equilibrium analysis of the Arachide de bouche program in Senegal. World Dev. 30, 255–263. doi: 10.1016/S0305-750X(01)00104-8
Keywords: contract farming, farm household income, production-management contracts, propensity score matching, beef cattle farmers
Citation: Liang Y, Bi W and Zhang Y (2023) Can contract farming improve farmers’ technical efficiency and income? Evidence from beef cattle farmers in China. Front. Sustain. Food Syst. 7:1179423. doi: 10.3389/fsufs.2023.1179423
Edited by:
Francesco Caracciolo, University of Naples Federico II, ItalyReviewed by:
Simone Russo, University of Bari Aldo Moro, ItalyPan Jingtao, Hubei University of Technology, China
Copyright © 2023 Liang, Bi and Zhang. This is an open-access article distributed under the terms of the Creative Commons Attribution License (CC BY). The use, distribution or reproduction in other forums is permitted, provided the original author(s) and the copyright owner(s) are credited and that the original publication in this journal is cited, in accordance with accepted academic practice. No use, distribution or reproduction is permitted which does not comply with these terms.
*Correspondence: Yuejie Zhang, emhhbmcteWpAamxhdS5lZHUuY24=
†These authors have contributed equally to this work