- College of Economics, Sichuan Agricultural University, Chengdu, China
The objective of this study is to assess the effect of Internet use on the income disparity between rural households and to determine how Internet usage can be used to reduce this income gap. We use the Recentered Influence Function Regression (RIF) and data from the China Family Panel Studies (CFPS) conducted by the China Social Science Survey (CSSS) center at Peking University to make the results of regression estimation more reliable. The results reveal that Internet use can make rural households’ income gap shrink considerably, and that the degree of non-farm employment among rural families has a mediating effect between Internet use and the income disparity of farm households. In addition, the Eastern region experiences a stronger mitigating effect from Internet use, whereas ethnic minorities find out no such mitigating effect. This study expands the scope of income disparity theory, provides new ideas for the construction of digital villages, and identifies new empirical evidence and decision-making grounds for improving the livelihoods of rural households and narrowing the income gap between rural households.
1. Introduction
To achieve shared prosperity, reducing the income gap is one of the most essential concerns. In China, rural residents’ disposable income increased to 18,931 Yuan in the first quarter of 2022, the income gap continues to widen, increasing from 26,700 to 28,500 (MARA, 2018). In this aspect, Internet accessibility is effective for reducing the income gap. The principal sources of income in rural areas are farm revenue, business income, wage income, and transfer income; however, farm income is mostly dependent on subsidies (Tae, 2016; Zhou and Li, 2021). In China, company revenue and wage income increased by 4,662 Yuan between 2014 and 2020, accounting for 70.18 percent of disposable income (Garcia-Mora and Mora-Rivera, 2021). Therefore, raising business income and wage income is more effective for alleviating income disparities. On top of altering the way rural people obtain information, Internet connection has had a significant economic impact and increased rural earnings (Chandio et al., 2022). Internet development has similarly affected the income disparity between urban and rural areas. To alleviate the inequality in income distribution between urban and rural areas, the government should expedite Internet construction based on regional differences (Headey et al., 2016). Wernecke et al. (2021) revealed that rural areas with low income required to make better use of the Internet to access information, and that only 4.2% of the goal had been met. It is significantly under the South African average. This gap prevents an accurate reflection of local and national progress. In other words, the disparity between rural areas’ Internet penetration and the national average may hinder rural development.
Moreover, as the Internet’s prominence has grown, its other applications have become increasingly apparent. The Internet has increased the income of producers while promoting the dissemination of information and spawning new industries (Chandio et al., 2023). With the development of information technology, the importance of E-commerce in fostering economic growth continues to grow. In the modern era of rapid E-commerce growth, traditional money has migrated online, and digital financial initiatives have evolved. Yin and Choi (2022) revealed that E-commerce helped to bring down the income difference between urban and rural residents. According to China Rural E-commerce Development Report (2021–2022) (Affairs, M.O.R, 2018), rural e-commerce retail transaction volumes in 2021 were 2.05 trillion-yuan, accounting for 15.66% of the nation’s online retail sales and growing 11.3% annually. According to Mei et al. (2022) the Internet provides rural households with new opportunities to generate revenue and enhances their income.
Garcia-Mora and Mora-Rivera (2021) concluded that Internet information can reduce information asymmetry, and that labor migration to large cities is also dependent on information transfer. Off-farm employment in rural areas is also influenced by Internet use (Song et al., 2022). The study used a random effects model to examine how Internet use affects migrant workers’ off-farm income. The findings confirmed that migrant workers’ off-farm income increased significantly when they used the Internet (Chen H. et al., 2022). Internet’s role in disseminating information greatly facilitated rural families’ social networks and created more opportunities for them to engage in business operations and off-farm employment (Zou and Mishra, 2022). Similarly, smartphones, as one of the methods to access the Internet, have been proven to considerably boost the decision-making capacity of women by increasing their employment outside the home (Zheng et al., 2022). However, the role of the Internet in promoting non-farm employment varies by region, with rural women living in the East and Centre having more opportunities to benefit than that in Western China (Wang and Zhang, 2022).
Internet access can help to close the income gap by improving non-agricultural employment opportunities (Nguyen Phuc et al., 2020). In Sub-Saharan Africa, off-farm income accounts for a large portion of the household livelihood portfolio (Van den Broeck and Kilic, 2019). Considering the prevalence of poverty in rural regions, it is commonly believed that non-farm income helps farmers survive and reduces income disparities (Bouchakour and Saad, 2020). In China’s rural areas, non-farm employment has considerably broadened the income streams of rural residents. Off-farm employment in China has improved income and contributed to the reduction of poverty in the region, indicating that the Chinese government should create policies to expand their opportunities to participate in off-farm employment (Yu and Wang, 2021).
Wang et al. (2021) explored that non-farm employment in rural areas has considerably contributed to farmer income growth. Farmers’ income structure changed as a result of the off-farm employment process, with each unit increase in rural off-farm employment resulting in a 9.215-unit increase in farmers’ income. Meanwhile, the study investigated the educational returns to off-farm employment in various workplaces. There is heterogeneity in the returns to education across workplaces among rural laborers, with workers in large cities earning significantly higher returns than workers in smaller cities and counties (Duong et al., 2021; Springmann and Freund, 2022). Off-farm income has surpassed agricultural income in China since 2013, becoming the most important source of income for farmers. E-commerce on the Internet not only provides a new avenue for rural households to find work, but it also contributes to an increase in rural residents’ discretionary income (Statistics, N.B.O, 2021).
The rapid spread of the Internet and the development of entrepreneurial forms represented by E-commerce have also had an impact on rural entrepreneurship. Using the 2014–2018 Probit mode, Liu et al. (2022) discover that Internet use positively affects farmers’ entrepreneurship, and this result holds even after accounting for endogeneity. Ma (2022) revealed that rural residents have higher returns to Internet usage. However, the contribution of Internet use to reducing revenue disparity varies by education level and group, with the highly educated group and the younger generation having a more significant effect. Furthermore, at the micro level, the Internet protects the health status of people living in rural areas, as health status is an important factor affecting revenue inequality. Using the China Household Tracking Survey 2014 to 2018 as a research sample, Wang M. et al. (2020) explored that the Internet helped reduce health inequality because it reduced income inequality, promoted health awareness, and reduced depression. These results are of great value in promoting health equality.
Current study on the Internet and the income divide has produced exceptional outcomes. The preponderance of research supports the Internet’s effect on the income gap, its relationship with off-farm employment, and the relationship between off-farm employment and income inequality. However, the direction of the impact must still be determined. Under the background of China’s vigorous promotion of rural revitalization strategy, the disparity in household income contributes to the national income inequality. In order to accomplish rural revitalization and shared prosperity, it must also be addressed. The aforementioned data provide a sufficient theoretical basis for examining the Internet usage and income disparity in China.
The main objective of this paper is to conduct a comprehensive empirical analysis of how Internet use affects the income gap of rural households and to examine the role of non-farm employment in it. Particularly, this paper’s marginal contributions are most apparent in the following three areas: (1) The existing literature on the examination of the income difference in farm households is rarely linked to off-farm employment, which is typically addressed separately, and the study contributes to the current literature on the margin. (2) Using RIF regression, off-farm employment is used as a mediating variable to examine an alternative channel of influence between Internet usage and income disparity of rural households, which is conducive to policy recommendations that are narrowly focused. (3) This study empirically investigates the relationship between the Internet, non-farm employment, and the income disparity of farm household. Also investigated is heterogeneity resulting from regional and ethnic variations.
The remaining sections of the paper are as follows. Section 2 addresses the theoretical framework, while Section 3 section addresses the methods and materials. In Section 4, summarizes empirical results and discussion are presented. Section 5 offers conclusions and policy recommendations.
2. Theoretical framework and research hypotheses
2.1. The universal usage of Internet is tended to reduce the income gap
The rapid expansion of the Internet has had a profound effect on both the areas of urban and rural. It drastically altered rural communities’ way of life. Internet usage generates additional income for rural households (Vatsa et al., 2022). As a source of new information, the Internet can broaden the horizons and knowledge of rural households (Hampton et al., 2021). As a result, they can improve their skills and participate in job training activities organized by the local government. Proper training equals a new educational experience for households, particularly the least educated (Khan et al., 2022). Therefore, the internet can cultivate learning ability to improve their employability (Ravizza et al., 2014), increase their income or create new revenue sources for rural households (Honkonen et al., 2018; Chen H. et al., 2022), and ultimately reduce the income disparity between the areas of rural and urban (Wang et al., 2021; Song et al., 2022).
With the rural spread of the Internet, the level of rural areas’ information technology has increased significantly, allowing rural low-income groups to access the Internet through cheaper and more portable mobile phones, thereby reducing information search costs and increasing the efficiency of agricultural markets, facilitating the integration of low-income groups into markets, fostering income growth, and reducing poverty levels. Since then, rural households have substantially more options than in the past (Logsdon et al., 2015). Through the Internet, rural households can learn more about employment opportunities, increasing their likelihood of non-farm employment and wage income. Internet use will also contribute to the development of rural production and new industries, thereby contributing to reducing the overall income disparity situation in the rural area.
H1: The internet usage of rural households can reduce the income gap.
2.2. Off-farm employment plays a mediating role in the impact of the internet on the income gap
As the principal streams of income for farm households and a major contributor to rural per capita income growth, non-farm work considerably contributes to the closing of the income disparity between the areas of rural and urban (Rajkhowa and Qaim, 2022). For industries and enterprises, expanding the Internet in rural areas affects not only households (Wang H. et al., 2020), but also the employment market in rural areas, the location of more industries and enterprises in rural areas, and the creation of additional jobs such as network e-commerce practitioners and travel industry practitioners (Garin-Munoz et al., 2019; Chen H.-C. et al., 2022; Sun and Luo, 2022). As a result, the demand for labor in rural areas increased. Consequently, a new avenue of income generation was created for households in rural areas. The disparity of income can be narrowed (Wang M. et al., 2020). Households can carry out business and become self-employed business people to run restaurants or homestays instead of being employed by enterprises (Zhao, 2020). Off-farm employment can improve income inequality among rural households by increasing their wage income while offsetting income shocks from natural and transactional risks in agricultural production. Increased levels of off-farm employment can also provide rural households with access to more sophisticated social capital, allowing rural households with low income to have more opportunities to broaden their income channels, thus alleviating the income disparity among rural households to some extent.
H2: The off-farm employment can reduce the rural households’ income gap and bridge the Internet and the income gap.
2.3. The impact of internet use on the rural households’ income gap differs by region or other factors
It is widely known that Internet usage provides numerous advantages, but there is also the likelihood that Internet usage tends to exacerbate the economic disparity (Liu et al., 2022). The dominant households are better educated and have more learning experience, their learning ability and receptivity are higher (Vaarmets et al., 2019). Hence, they have a greater opportunity to use the Internet, and this type of person may master Internet technology much better than others in rural areas. Higher educated people can obtain more technology, improve their employment abilities, and access more information on the Internet in order to earn a higher income (Wang H. et al., 2020). Less educated vulnerable households have more difficulty mastering the Internet, and it is more difficult for them to learn technology or obtain information efficiently from the Internet (Berner et al., 2015; Zhao, 2020). Therefore, the income of people with good learning ability increased significantly more than that of less educated households. The exceptional ability of the dominant farmers is more visible when compared to the weak farmers, which will widen the income gap among all farmers.
H3: There are heterogeneities in the impact of internet use on rural households’ income gap.
3. Data and methodology
3.1. Data
This paper examines the effect of Internet usage on income disparities in rural areas. We use data from China Family Panel Studies (CFPS), Which consists of 25 provinces, municipalities, and autonomous areas, and all members of sample families are surveyed. According to the needs of this paper’s research, the following screening procedures were conducted: first, retain the sample of farmers; second, exclude the sample whose core variable values are absent; and third, combine the data of people and households and delete missing values. Thus, 5,809 effective family samples were collected in total.
As shown in Figure 1, the rural households’ average revenue in China has increased with the growth of Internet penetration in rural areas between 2006 and 2018, and Internet use has significantly contributed to rural residents’ income. The comprehensive coverage of rural Internet infrastructure has considerably aided the steady enhancement of the rural areas’ Internet spread, and rural groups’ integration into the online society has accelerated. “Villages are connected to broadband” and “counties are connected to 5G,” according to China’s existing administrative villages. Rural residents have been rapidly integrating into the Internet society, and the number of Internet users in rural areas has steadily increased.
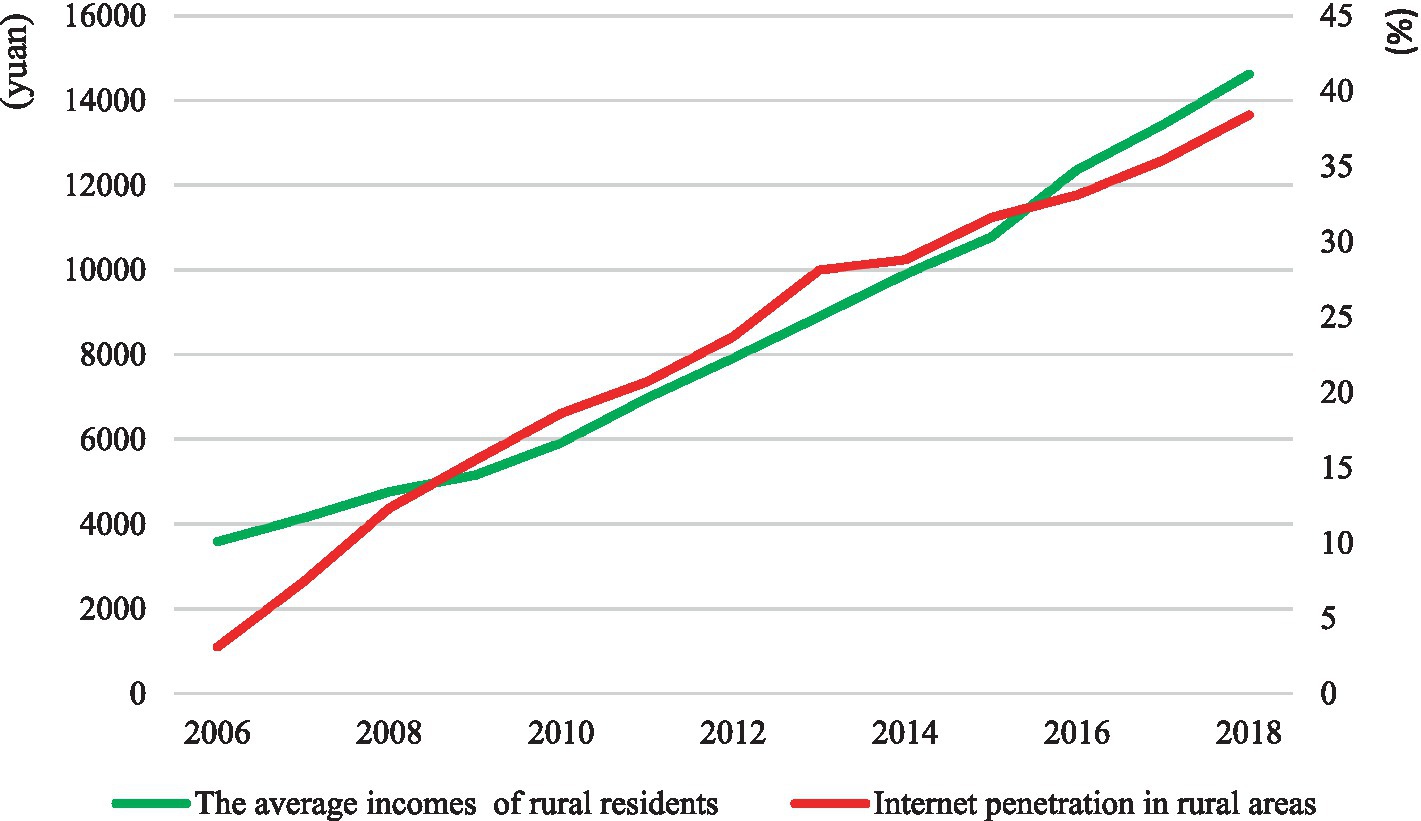
Figure 1. The development trend of rural Internet penetration rate and the rural households’ per capita income in China from 2006 to 2018.
With the advancement of Internet technology, the Internet and agriculture and rural areas have become inextricably linked, the level of agricultural production information is steadily improving, and agricultural transformation and upgrading have yielded remarkable results. The rural digital economy is rapidly developing, agricultural and rural e-commerce is expanding, and new business forms are flourishing. The rural areas’ Internet usage has significantly increased farm entrepreneurship as well as non-farm employment while also improving farmers’ access to market resources, social network delivery, and social fundraising. It increased farmers’ skill literacy, provided new opportunities and ways for farmers to start businesses, and enabled the majority of farmers to meet their income growth target.
Figure 2 depicts the significant spatial imbalance in Internet development in rural areas of China. The rural areas’ Internet penetration rate in eastern China is the highest, followed by that in central China, while it is the lowest in southwest Guizhou, Xizang, and other regions, and northeast China, such as Jilin. Internet development in China’s rural areas varies by province, with the East and South generally higher than the West and North.
Coastal areas such as Jiangsu, Zhejiang and Shanghai have excellent Internet infrastructure, a more vibrant Internet atmosphere and a higher level of information, so rural Internet penetration in these regions is relatively higher. The Central region has tremendous development potential for the Internet, and rural Internet penetration is also increasing. Additionally, rural areas have more ways and chances to generate returns from Internet resources. In places with low levels of informatization, however, the Internet development degree is low, the Internet atmosphere is thin, and the rural Internet penetration rate is relatively low. Increasing the popularity of regional rural Internet is hence beneficial to rural communities gaining greater returns from Internet resources.
3.2. Description of the variables
3.2.1. Dependent variable
The Gini coefficient of the logarithm of farm household income is used as a measure of farm household income inequality and as the dependent variable in this work, with reference to conventional research methodologies.
3.2.2. Core explanatory variable
Following the study of Honggen and Cheng-xiao (2020), this work employs the question “Do you have Internet access?” as a specific indication of farmers’ Internet usage. It is defined as using the Internet if “Yes” is selected, and as not using the Internet if “No” is selected.
Mediating variables: Off-farm employment is a family decision with the goal of maximizing the family’s financial well-being. From this vantage point, we refer to Jingna and Junfeng’s (2020) study and assign a value of 0 to household members who only engage in farming, 1 to those who combine farming and off-farm employment, and 2 to those who only engage in off-farm job.
Control variables: In this work, we refer to previous research and identify additional variables that influence the income gap of rural families at the person, household, and village levels, considering farm households as the micro-level basic unit. The individual level comprises health status (per capita) and level of education (per capita). The household level consists of household size, party member households, government subsidies, land transfer, the number of males in the household, the population dependence ratio, household financial assets, household debt, productive fixed assets, and land assets. At the village level, land topography and distance to the county are provided. Table 1 contains the descriptive statistics for the selected variables.
In terms of household income, the center of the Kernel density curve shifts to the right as household Internet usage increases, demonstrating that Internet usage can increase household income. The core density curve for Internet-using households exhibits a steeper slope trend, and the total income distribution is more concentrated (see Figure 3). It suggests that as the Internet becomes more prevalent in households, farmers will be able to get information and learn more quickly and easily to enhance their wealth and income, hence reducing the income gap across farmer groups.
Compared to non-agricultural families, the general income distribution of rural households has shifted to the right, indicating a tendency toward higher income groups, and the distribution is more concentrated (see Figure 4). It implies that the transition from non-farm to non-farm employment is also a migration toward groups with fewer internal income gaps. These modifications may reduce the economic disparity between agricultural households.
3.3. Empirical model
RIF research approach is employed in this work (Recentered Influence Function Regression). RIF regression is an extended unconditional regression method, initially proposed and developed by Firpo et al. (2009), which not only better reflects the marginal effects of small changes in the explanatory variable X on various distributional statistics (mean, quantile, variance, Gini coefficient, etc.) of the dependent variable Y, but also solves the endogeneity problem caused by omitted variables, etc., more effectively. To reduce endogeneity caused by “missing variables,” suitable proxy variables are substituted for variables that are difficult to observe but affect household revenue. The RIF regression estimates are more robust than the traditional OLS regressions. Previous researchers, such as Wei and Yingliang (2018) have utilized RIF regressions extensively to investigate income disparities in their research. Therefore, this research uses the Gini coefficient as a surrogate to characterize the income disparity of farm households and develops the RIF regression function using the Gini coefficient (Jiazhi and Wentao, 2016).
The Gini coefficient is defined by the following formula:
Equation (1) satisfies the follows:
In Formula (1), is the functional of income distribution function . In this paper, we use as the Gini coefficient to describe income distribution .
The Gini coefficient’s influence function is defined as follows:
Equation (3) satisfies the follows:
Based on Equations (1) and (3), the Redistribution Influence Function (RIF) of Gini coefficient is developed as follows:
Firpo et al. (2009) gave a detailed estimation method of Equation (5), so it will not be described here. Combined with the Equation (5), we take the Gini coefficient, which reflects the income distribution inside rural households, as the explained variable. And take Internet use and off-farm employment as the core explanatory variables, take other characteristics of households or households as control variables. The regression model for the impact of income gap redistribution based on the Gini coefficient is constructed as follows:
In Equation (6), RIF()is the influence function of household income based on Gini coefficient, , , , are constant term, Internet use (Net), off-farm employment (Ofemp) and the coefficient of control variable(), is the random disturbance term.
To further explore the mediating effect of off-farm employment on households’ income gap, following Baron and Kenny (1986), we take the off-farm employment level of households as the mediating variable. We examine the mediating role of off-farm employment in Internet use in influencing the revenue gap among farm households. We constructed the mediating effect model as follows:
We take M as the mediating variable, it is defined as off-farm employment (Ofemp). If the variable Net affects the variable Gini (Income) through the variable Ofemp, then Ofemp can be considered as the mediating variable. On the basis of the above model, the Sobel goodman test is conducted in this paper, and the test process is: in the first step, the significance of the coefficient is tested, and if it is not significant, it means that it is not relevant. The second step is to test whether at least one of the coefficients and is significant, if both are significant, it indicates that there is a mediating effect, and the third step; if at least one of the coefficients is not significant, the Sober test is conducted, and if it passes the significance test, the mediating effect is established.
4. Empirical results and discussion
4.1. Analysis of baseline regression results
This work employs the regression method of the recently modified impact function (RIF) presented by Firpo et al. (2009). The benchmark regression’s findings reveal the influence of Internet usage and non-farm work on farm households’ income disparity. Table 2 shows the results of the RIF estimates utilizing the Gini coefficient as a measure of income disparity among farm households.
As shown in Equation (1) in Table 2, the estimated coefficient of Internet use is −0.009 and is significant at the 1% level, indicating that Internet use significantly reduces the income gap of farm households. In models (3), (4), and (6), the direction of the estimated coefficients remains significantly negative without change when off-farm employment, control variables and both are added in turn. As can be seen from Equation (2) in Table 2, the estimated coefficient of non-farm employment is −0.018 and is significant at the 1% level. This indicates that non-farm employment can be effective in reducing the income disparity for farm households. In models (3), (5), and (6), the models’ the estimated coefficients do not change the direction of influence and remain significant when Internet use, control variables and both are added in turn, implying that the above results are more robust.
Among the control variables, per capita educational attainment (Edu), health status (Health), and household size (Size), population dependency ratio, household financial assets, and land assets can significantly affect the income disparity of rural families. At the individual level, the increase in education (Edu) and the improvement in health status (Health) both help to reduce the farm households’ income disparity, which is generally consistent with the findings of existing studies (Jiazhi and Wentao, 2016); at the household level, financial assets, land assets, and household size (Size) all significantly alleviate the intra-farm household income disparity. The financial assets of farm households have become a significant factor affecting the income disparity between rural households (Qinghua, 2002), and improving the rural financial market, encouraging farm households to make financial investments, and increasing the amount of farm household financial assets will help to reduce the farm households’ revenue disparity. In rural China, since the physical capital owned by low-income farmers is very poor, the marginal output of increasing capital inputs such as land assets for them is more significant. While continuing to promote urbanization and orderly transfer of rural labor, increasing land assets of farmers and establishing family farms of a certain scale will contribute to mitigate the income disparity of farmers. The increase in population dependency ratio will lead to a worsening of the income gap for farm households, probably because many laborers cannot leave home and devote themselves to work due to taking care of the elderly and young children, resulting in a lower level of household income, which in turn causes the income gap.
4.2. RIF regressions of income structure differences among farm households
As shown in Table 3, the income disparities of farm households were decomposed into five categories: wage income disparities, operating income disparities, property income disparities, transfer income disparities and other income disparities, to examine the effect of Internet use and non-farm employment on different types of the income disparities.
Specifically, Internet usage and non-farm employment will significantly reduce the disparity between farm household income from wages and property income. The reason may be that, on the one hand, the use of the Internet has facilitated communication and information transfer, giving rise to new industries such as e-commerce, thus widening employment channels and increasing the probability of employment. On the other hand, the popularity and usage of the Internet has broadened farmers’ perception of the world, supplemented the knowledge and skills required for employment of low-skilled workers, and increased employment opportunities to diversify farmers’ employment, thus increasing their sources of income, which has internally moderated the wage income gap. In addition, the Internet can enhance farmers’ awareness of investment and financial management and enrich their investment channels, so that they can use a variety of financial instruments and products to narrow the property income gap. In short, non-farm employment can increase farmers’ income and optimize the allocation of household economic structure (Xolunduo and Bing, 2017), and promote the rational allocation of various household idle assets and land, thus releasing the value of key household assets more fully. In addition, for farmers living in areas with relatively closed information and scarce production factors, the increase in off-farm employment can further increase their household economic welfare, thus contributing to reducing the overall wage and property income disparities among farmers.
In terms of operating income, the coefficient of the effect of non-farm employment on rural households’ operating income is −0.031 and is significant at the 5% level. The possible reasons are that the increase in non-farm employment rates has led to a gradual shift from agricultural operations to part-time and sideline operations, and made the traditional agricultural operations change to more specialized and large-scale modern agriculture. The income gap between farming households is reduced.
For transfer income and other income aspects, Internet use can exacerbate the income gap for farming households. After the reform and opening up, the revenue streams and structure of farmers have been optimized to some extent, and the importance of wage income in farmers’ income is increasing, with its contribution rate reaching more than 40%, making it the most important factor affecting farmers’ income (Xiaohua, 2019). In contrast, the contribution rate of business income is 37%, and the contribution rate of property income is smaller at about 3%. Internet use and off-farm employment effectively narrow the farm households’ revenue disparity through their combined impact on various types of income.
4.3. Analysis of the mediating effect of off-farm employment
According to models (1) and (2) in Table 4, the coefficients of the effects of Internet use on the income gap of rural households and non-farm employment are −0.006 and 0.062, respectively. Furthermore, the results from model (3) show that after the inclusion of non-farm employment, the estimated coefficients of Internet use and non-farm employment on rural household income disparity are −0.005 and − 0.018 respectively, and both are significant at the 1% level. This series of results indicates that the mitigating effect of Internet use on the income disparity of farm households can be influenced through non-farm employment and that the mediating effect accounts for 19.3% of the process.
The development of the Internet has created many new types of flexible jobs, such as e-commerce and online taxi, which can attract more farmers into non-farm employment. Farmers can acquire new knowledge and improve their competency skills at a lower cost. Moreover, the more regularly the group uses the Internet, the more significant the positive marginal effect of non-farm employment being influenced by the Internet (Dong, 2016). At the same time, the rising level of non-farm employment breaks the original solidified social class, reduces income inequality, optimizes the absolute income profile, and thus achieves upward mobility in socioeconomic status, which in turn alleviates the overall income gap among farm households.
4.4. Unconditional quantile regression of farm household income levels
This study investigates the impact of Internet use on farm household income at different quartiles through unconditional quantile regression. Table 5 shows the estimation results of regressions with the logarithm of farm household income as the dependent variable at quartiles 0.1, 0.3, 0.5, 0.7 and 0.9. Columns (1) to (5) in Table 5 report the regression results at the 0.1, 0.3, 0.5, 0.7, and 0.9 quartiles, respectively. As seen in Table 5, the estimated coefficient for Internet use (Net) is not significant at the 0.1 quantile, while the coefficients are significantly positive at the 0.3, 0.5, 0.7 and 0.9 quantile, and the coefficient basically shows a decreasing trend.
As shown in Table 5, the intensity of the impact of Internet use on the income level of farm households shows a gradual weakening trend. This shows that the use of the Internet has a better impact on income enhancement for the low-income group than for the high-income group. The use of the Internet has a stronger effect on income security for the lower and middle-income farming groups, thus effectively alleviating the income disparity among farming households.
4.5. Heterogeneity analysis
4.5.1. Regional differences
As can be seen in Figure 5 that the use of the Internet has contributed to the revenue growth of rural households in the East, Central and West, but the positive effects of the Internet on revenues vary by region, and for farm households, the greatest impact is felt in the east, followed by the Central and West. Internet use in rural areas can affect farm household income, and the effect on reducing the income disparity varies from region to region (Hoang et al., 2014; Asongu and Odhiambo, 2019; Du et al., 2021; Do et al., 2022).
Regional differences are an important determinant of income disparity among farm households. China has a vast territory and uneven economic development among regions, coupled with the different resource endowments and economic policies that differ among regions, making regional differences in Internet penetration and usage, and the degree of informatization. By examining the index of China’s informatization development, Internet and cell phone penetration and other indicators Mingcheng et al. (2016) found that China’s informatization development shows obvious inter-regional disparities and spatial imbalances, with the highest level of informatization in the eastern coastal region and the southwest and northwest regions lag relatively behind (Chaodong and Jing, 2017).
To further explore regional differences in the impact of Internet use on income disparity among farm households, this section divides the sample into three regions: East, Central and West (Shehu and Sidique, 2014; Khanal and Mishra, 2016; Houngbonon and Liang, 2021; Mora-Rivera and Garcia-Mora, 2021; Hua and Zhang, 2022; Khan et al., 2022; Odhiambo, 2022). Table 6 demonstrates the RIF regression results of the farm households’ income disparity by region. The coefficient of Internet use on income disparity for rural households in the East is −0.009 and is significant at the 1% level, while the estimated coefficient for the Central and Western regions is −0.005 and is significant at the 5% level, indicating that there are regional differences in the impact of Internet use on income disparity among rural households. Possible explanations include the fact that the overall level of informatization in eastern regions is higher, the Internet infrastructure is better, and the Internet atmosphere is stronger. As a result, farmers in the eastern region have a deeper understanding of the Internet and have more opportunities to convert Internet resources into income returns, thereby more effectively narrowing the income gap of farmers’ families. Low levels of education in the Centre and West make it difficult for farmers to accept and utilize the Internet, and the relatively backward infrastructure construction, low level of information technology, and slow economic development in these regions make it difficult for Internet resources to provide sufficient employment and income-generating channels for farmers. Consequently, compared to the eastern regions, Internet usage’s impact on decreasing the income disparity among farming households in the central and western regions is weaker.
4.5.2. Ethnic differences
China has a vast territory, many ethnic groups and wide distribution, and Internet use is an individual micro-decision-making behavior, so the ethnic characteristics of farm households will have a certain degree of influence on Internet usage and changes in rural household income disparity. In this paper, farm households are divided into two groups, Han Chinese and ethnic minorities, according to their ethnic characteristics, and RIF regressions are conducted separately.
Equations (1) and (2) in Supplementary Table A1 report the results of the RIF estimates of the income gap for farm households by ethnic group. The results indicate that the use of the Internet can significantly reduce the income gap among Han Chinese rural households and has no impact on the rural ethnic minorities’ income disparity. The low level of education among farmers from ethnic minorities has had a massive effect on the efficiency of their Internet usage. Furthermore, the majority of China’s ethnic minority groups reside in economically underdeveloped Central and Western regions and border areas. Because of the regional imbalance in development and the influence of their own religious and cultural beliefs, ethnic minority groups are hampered in accessing Internet information resources, making it trouble for the Internet to perform at its best for employment and income generation. In contrast, Han Chinese farmers have a relatively high level of education and are better able to adapt to new technologies, allowing them to study and master the Internet more effectively. In addition to being more intelligent and motivated, Han farmers can actively utilize the Internet to acquire information, improve their professional capabilities, expand their employment channels and increase their streams of income. As a consequence, Han farming households can utilize the Internet more effectively than minority groups to reap the benefits, thereby reducing the income disparity among farming households.
4.6. Robustness test
To further enhance the reliability of the research results, this paper conducted robustness tests, and the consequences are shown in Supplementary Table A2. The impact of internet usage on farm income inequality was re-tested using the variance of farm household income to measure farm income disparity for RIF regression. As can be seen from (1) in Supplementary Table A2 the regression results are essentially unchanged from the estimated baseline regression results, indicating that the use of the Internet significantly closes the farm-household income gap. The results in Supplementary Table A2 (2) also show Internet use significantly reduces the income disparity of farm households, again validating the robustness of the baseline regression results. Furthermore, to avoid the problem of data selectivity bias that may arise from using single-year data. This paper uses CFPS 2016 data for re-estimation, and a total of 8,783 household sample households are screened, Supplementary Table A2 (3) shows that the regression is robust. In addition, whether to use the Internet results from individual farmers’ voluntary choice, determined by their characteristics and comparative advantages. Therefore, to ensure the research conclusions’ reliability, the propensity score matching method (PSM) was used to correct potential sample selection bias to avoid endogeneity problems, and estimation results bias caused by selection bias.
According to Supplementary Table A3, it can be found that the average treatment effect (ATT) after matching remains between −0.0042 and − 0.0047, regardless of the matching method, and is significant at the 1% statistical level. The results show that after mitigating the endogeneity problem, the effect of Internet use on the income gap of farm households remains robust.
5. Conclusion and policy implications
Using the RIF regression and the China Household Tracking Survey 2018 as a research sample, this study examines the positive role of Internet use in alleviating the income gap among rural households. Off-farm employment is also considered an intermediate variable in this study. According to the data, the use of the Internet can massively decrease the economic disparity of rural households, with a mediating effect on non-farm employment, and this finding holds after robustness testing. In addition, a decomposition of rural household income reveals that Internet use and non-farm employment reduce the income gap among rural households mainly by improving wage income and property income. Furthermore, we discovered that there are heterogeneities in the effect of Internet use on rural household income disparity, meaning that Internet use is most effective in the East region due to its greater development. In terms of ethnic differences, Internet use can significantly reduce the income gap among Han Chinese rural households. Ethnic households had lower levels of education; therefore, the impact was not statistically significant.
This study’s findings indicate that the usage of the Internet is crucial to narrowing the disparity of income and expanding off-farm employment. It could provide useful evidence to the government in its efforts to encourage rural development while narrowing the income gap between households.
The empirical findings of our study support a number of recommendations. Firstly, there is a need to widely promote internet use in rural areas in order to eliminate household income gaps and establish long-term prosperity. Furthermore, rural households must be manually instructed. Consequently, rural families can receive more information quickly and correctly comprehend the job training and assistance policies.
Secondly, governments must increase the number of locally relevant online job training programs. It is imperative for those who never work outside of their native country to fully utilize the local features can also boost the number of employment. Online job training may help more people and cost less. In order to increase employability, it is crucial to actively promote internet usage.
Finally, there are still striking income differences between urban and rural populations. The Chinese government ought to think about expanding employment opportunities there. Recently, the main concern has been how to develop tourism in some rural areas. The main trend is to develop online e-commerce and sell items online. It can boost local industrial growth, stimulate tourism development, and generate economic gains and higher earnings.
Data availability statement
The raw data supporting the conclusions of this article will be made available by the authors, without undue reservation.
Ethics statement
Ethical review and approval was not required for the study on human participants in accordance with the local legislation and institutional requirements. Written informed consent from the participants was not required to participate in this study in accordance with the national legislation and the institutional requirements.
Author contributions
AAC and YL: conceptualization. AZ and TY: data curation. AZ: formal analysis. ZD: methodology. AAC: supervision. AZ, TY, and AAC: writing-original draft. YL and ZD: writing-review and editing. All authors contributed to the article and approved the submitted version.
Funding
This study was funded by the Major Program of the Development of Old Revolutionary Areas in Sichuan (SLQ2022SA-01).
Conflict of interest
The authors declare that the research was conducted in the absence of any commercial or financial relationships that could be construed as a potential conflict of interest.
Publisher’s note
All claims expressed in this article are solely those of the authors and do not necessarily represent those of their affiliated organizations, or those of the publisher, the editors and the reviewers. Any product that may be evaluated in this article, or claim that may be made by its manufacturer, is not guaranteed or endorsed by the publisher.
Supplementary material
The Supplementary material for this article can be found online at: https://www.frontiersin.org/articles/10.3389/fsufs.2023.1173158/full#supplementary-material
References
Asongu, S. A., and Odhiambo, N. M. (2019). How enhancing information and communication technology has affected inequality in Africa for sustainable development: an empirical investigation. Sustain. Dev. 27, 647–656. doi: 10.1002/sd.1929
Baron, R. M., and Kenny, D. A. (1986). The moderator-mediator variable distinction in social psychological research: conceptual, strategic, and statistical considerations. J. Pers. Soc. Psychol. 51, 1173–1182. doi: 10.1037/0022-3514.51.6.1173
Berner, J., Rennemark, M., Jogreus, C., Anderberg, P., Skoldunger, A., Wahlberg, M., et al. (2015). Factors influencing internet usage in older adults (65 years and above) living in rural and urban Sweden. Health Informatics J. 21, 237–249. doi: 10.1177/1460458214521226
Bouchakour, R., and Saad, M. (2020). Farm and farmer characteristics and off-farm work: evidence from Algeria. Aust. J. Agric. Resour. Econ. 64, 455–476. doi: 10.1111/1467-8489.12349
Chandio, A. A., Gokmenoglu, K. K., Sethi, N., Ozdemir, D., and Jiang, Y. (2023). Examining the impacts of technological advancement on cereal production in ASEAN countries: does information and communication technology matter? Eur. J. Agron. 144:126747. doi: 10.1016/j.eja.2023.126747
Chandio, A. A., Sethi, N., Dash, D. P., and Usman, M. (2022). Towards sustainable food production: what role ICT and technological development can play for cereal production in Asian–7 countries? Comput. Electron. Agric. 202:107368. doi: 10.1016/j.compag.2022.107368
Chaodong, Y., and Jing, M. (2017). Regional disparity and dynamic evolution of informationization development in China. Soft Sci. 31, 44–49. doi: 10.13956/j.ss.1001-8409.2017.07.10
Chen, H., Chen, C.-P., Li, Y., Qin, L., and Qin, M. (2022). How internet usage contributes to livelihood resilience of migrant peasant workers? Evidence from China. J. Rural. Stud. 96, 112–120. doi: 10.1016/j.jrurstud.2022.09.028
Chen, H.-C., Wang, C.-C., Hung, J. C., and Hsueh, C.-Y. (2022). Employing eye tracking to study visual attention to live streaming: a case study of Facebook live. Sustainability 14:7494. doi: 10.3390/su14127494
Do, M. H., Nguyen, T. T., Halkos, G., and Grote, U. (2022). Non-farm employment, natural resource extraction, and poverty: evidence from household data for rural Vietnam. Environ. Dev. Sustain. 24, 1–38. doi: 10.1007/s10668-022-02391-7
Dong, Z. (2016). Research on the effect of internet coverage driving rural employment. World Econ. Pap. 3, 76–90.
Du, H. F., Zhou, N., Cao, H. J., Zhang, J. T., Chen, A. L., and King, R. B. (2021). Economic inequality is associated with lower internet use: a nationally representative study. Soc. Indic. Res. 155, 789–803. doi: 10.1007/s11205-021-02632-8
Duong, P. B., Thanh, P. T., and Ancev, T. (2021). Impacts of off-farm employment on welfare, food security and poverty: evidence from rural Vietnam. Int. J. Soc. Welf. 30, 84–96. doi: 10.1111/ijsw.12424
Firpo, S., Fortin, N. M., and Lemieux, T. (2009). Unconditional Quantile Regressions. Econometrica 77, 953–973. doi: 10.3982/ECTA6822
Garcia-Mora, F., and Mora-Rivera, J. (2021). Exploring the impacts of internet access on poverty: A regional analysis of rural Mexico. New Media Soc. 25, 26–49. doi: 10.1177/14614448211000650
Garin-Munoz, T., Lopez, R., Perez-Amaral, T., Herguera, I., and Valarezo, A. (2019). Models for individual adoption of eCommerce, eBanking and eGovernment in Spain. Telecommun. Policy 43, 100–111. doi: 10.1016/j.telpol.2018.01.002
Hampton, K. N., Robertson, C. T., Fernandez, L., Shin, I., and Bauer, J. M. (2021). How variation in internet access, digital skills, and media use are related to rural student outcomes: GPA, SAT, and educational aspirations. Telematics Inform. 63:101666. doi: 10.1016/j.tele.2021.101666
Headey, D. H., Derek, D., and Martin, W. J. (2016). The impact of food prices on poverty and food security. Ann. Rev. Resour. Econ. 8, 329–351. doi: 10.1146/annurev-resource-100815-095303
Hoang, T. X., Pham, C. S., and Ulubasoglu, M. A. (2014). Non-farm activity, household expenditure, and poverty reduction in rural Vietnam: 2002-2008. World Dev. 64, 554–568. doi: 10.1016/j.worlddev.2014.06.027
Honggen, Z., and Cheng-Xiao, S. (2020). The effect of internet use on labor resource allocation in family farms. J. Agrotech. Econ. 8, 40–53. doi: 10.13246/j.cnki.jae.2020.08.004
Honkonen, N., Liira, J., Lamminpaa, A., and Liira, H. (2018). Work ability meetings-a survey of Finnish occupational physicians. Occup. Med. 68, 551–554. doi: 10.1093/occmed/kqy115
Houngbonon, G. V., and Liang, J. (2021). Broadband internet and income inequality. Rev. Netw. Econ. 20, 55–99. doi: 10.1515/rne-2020-0042
Hua, Y., and Zhang, H. Y. (2022). Internet penetration and income inequality: evidence from the Chinese young labor market. Appl. Econ. 55, 1–15. doi: 10.1080/00036846.2022.2156471
Jiazhi, X., and Wentao, W. (2016). Social structure change, social capital transformation and peasant household income gap. China Soft Sci. 10, 20–36.
Jingna, Z., and Junfeng, Z. (2020). Internet use and the degree of rural labor transfer: also on the influence of household division of labor pattern. Finan. Econ. 1, 93–105.
Khan, N., Ray, R. L., Zhang, S. M., Osabuohien, E., and Ihtisham, M. (2022). Influence of mobile phone and internet technology on income of rural farmers: Evidence from Khyber Pakhtunkhwa Province, Pakistan. Technol. Soc. 68:101866. doi: 10.1016/j.techsoc.2022.101866
Khanal, A. R., and Mishra, A. K. (2016). Financial performance of small farm business households: the role of internet. China Agric. Econ. Rev. 8, 553–571. doi: 10.1108/CAER-12-2014-0147
Liu, Z., Ren, Y., and Mei, Y. (2022). How does internet use promote farmer entrepreneurship: evidence from rural China. Sustainability 14:16915. doi: 10.3390/su142416915
Logsdon, M. C., Mittelberg, M., and Myers, J. (2015). Use of social media and internet to obtain health information by rural adolescent mothers. Appl. Nurs. Res. 28, 55–56. doi: 10.1016/j.apnr.2014.04.006
Ma, X. (2022). Internet use and income gaps between rural and urban residents in China. J. Asia Pac. Econ. 27, 1–21. doi: 10.1080/13547860.2022.2054133
MARA (2018). Ministry of Agriculture and Rural Affairs, Available at: http://www.gov.cn/xinwen/2023-01/18/content_5737816.htm (Accessed November 5, 2022).
Mei, B., Khan, A. A., Khan, S. U., Ali, M. A. S., and Luo, J. (2022). Complementarity or substitution: a study of the impacts of internet finance and rural financial development on agricultural economic growth. Agric. Basel. 12:1786. doi: 10.3390/agriculture12111786
Mingcheng, C., Yanhong, J., Eun, G. K., and Qinghua, S. (2016). Household income inequality and its determinants in China: regression decomposition based on micro household data. Economics (Quarterly) 15, 1253–1274. doi: 10.13821/j.cnki.ceq.2016.02.17
Mora-Rivera, J., and Garcia-Mora, F. (2021). Internet access and poverty reduction: evidence from rural and urban Mexico. Telecommun. Policy. 45:102076. doi: 10.1016/j.telpol.2020.102076
Nguyen Phuc, C., Schinckus, C., Su Dinh, T., and Ling, F. C. H. (2020). Effects of the internet, mobile, and land phones on income inequality and the Kuznets curve: cross country analysis. Telecommun. Policy. 44:102041. doi: 10.1016/j.telpol.2020.102041
Odhiambo, N. M. (2022). Information technology, income inequality and economic growth in sub-Saharan African countries. Telecommun. Policy. 46:102309. doi: 10.1016/j.telpol.2022.102309
Qinghua, S. (2002). Research on household saving and borrowing behavior and its evolution trend. China Econ. Stud. 6, 66–78. doi: 10.19365/j.issn1000-4181.2002.06.008
Rajkhowa, P., and Qaim, M. (2022). Mobile phones, off-farm employment and household income in rural India. J. Agric. Econ. 73, 789–805. doi: 10.1111/1477-9552.12480
Ravizza, S. M., Hambrick, D. Z., and Fenn, K. M. (2014). Non-academic internet use in the classroom is negatively related to classroom learning regardless of intellectual ability. Comput. Educ. 78, 109–114. doi: 10.1016/j.compedu.2014.05.007
Shehu, A., and Sidique, S. F. (2014). A propensity score matching analysis of the impact of participation in non-farm enterprise activities on household wellbeing in rural Nigeria. UMK Proc. 1, 26–32. doi: 10.1016/j.umkpro.2014.07.004
Song, Y., Tan, L., Wang, Y., and Li, L. (2022). Data-driven modeling of the impact of internet inclusive finance on the urban-rural income gap in the E-commerce era. J. Internet Technol. 23, 1377–1388. doi: 10.53106/160792642022112306020
Springmann, M., and Freund, F. (2022). Options for reforming agricultural subsidies from health, climate, and economic perspectives. Nat. Commun. 13:82. doi: 10.1038/s41467-021-27645-2
Statistics, N.B.O. (2021). China statistical Yearbook Available at: http://www.stats.gov.cn/tjsj/ndsj/ (Accessed November 5, 2022).
Sun, J., and Luo, N. (2022). Research on innovative development of Crossborder e-commerce under the background of wireless communication and internet of things. Wireless Commun. Mob. Comput. 2022:1803276. doi: 10.1155/2022/1803276
Tae, J. S. (2016). A study on China’s farmers’ income structure and interregional income inequality. Korean-Chin. Soc. Sci. Stud. 14, 129–151.
Vaarmets, T., Liivamagi, K., and Talpsepp, T. (2019). How does learning and education help to overcome the disposition effect? Rev. Finan. 23, 801–830. doi: 10.1093/rof/rfy006
Van Den Broeck, G., and Kilic, T. (2019). Dynamics of off-farm employment in sub-Saharan Africa: a gender perspective. World Dev. 119, 81–99. doi: 10.1016/j.worlddev.2019.03.008
Vatsa, P., Li, J., Phong Quoc, L., and Botero-R, J. C. (2022). Internet use and consumption diversity: evidence from rural China. Rev. Dev. Econ. doi: 10.1111/rode.12935
Wang, H., Ding, L., Guan, R., and Xia, Y. (2020). Effects of advancing internet technology on Chinese employment: a spatial study of inter-industry spillovers. Technol. Forecast. Soc. Chang. 161:120259. doi: 10.1016/j.techfore.2020.120259
Wang, M., Kang, W., and Zhang, R. (2020). The gap between urban and rural development levels narrowed. Complexity 2020, 1–10. doi: 10.1155/2020/4615760
Wang, M., Yin, Z., Pang, S., and Li, Z. (2021). Does internet development affect urban-rural income gap in China? An empirical investigation at provincial level. Inf. Dev. 39, 107–122. doi: 10.1177/02666669211035484
Wang, W., and Zhang, S. (2022). The impact of internet use on rural Women’s off-farm work participation: empirical evidence from China. Sustainability 14:16972. doi: 10.3390/su142416972
Wei, L., and Yingliang, Z. (2018). Non-farm employment and household income gap: based on the heterogeneity analysis of “leaving land” and “leaving home”. J. Huazhong Agric. 3, 56–64+155. doi: 10.13300/j.cnki.hnwkxb.2018.03.007
Wernecke, B., Mathee, A., Kunene, Z., Balakrishna, Y., Kapwata, T., Mogotsi, M., et al. (2021). Tracking Progress towards the sustainable development goats in four rural villages in Limpopo, South Africa. Ann. Glob. Health. 87:16. doi: 10.5334/aogh.3139
Xiaohua, W. (2019). The evolutionary logic of Chinese farmers’ income structure and its effect measurement. J. Southwest Univ. 45, 67–77. doi: 10.13718/j.cnki.xdsk.2019.05.008
Xolunduo,, and Bing, Z. (2017). Financial availability, non-farm employment and farmer income: an empirical study based on CHFS data. Econ. Sci. 2, 74–87. doi: 10.19523/j.jjkx.2017.02.006
Yin, Z. H., and Choi, C. H. (2022). Does e-commerce narrow the urban-rural income gap? Evidence from Chinese provinces. Internet Res. 32, 1427–1452. doi: 10.1108/INTR-04-2021-0227
Yu, N., and Wang, Y. (2021). Can digital inclusive finance narrow the Chinese urban-rural income gap? The Perspective of the Regional Urban-Rural Income Structure. Sustainability 13:6427. doi: 10.3390/su13116427
Zhao, J. (2020). Internet usage and rural self-employment in China. Asian Perspect. 44, 77–101. doi: 10.1353/apr.2020.0003
Zheng, H., Zhou, Y., and Rahut, D. B. (2022). Smartphone use, off-farm employment, and women’s decision-making power: evidence from rural China. Rev. Dev. Econ. doi: 10.1111/rode.12966
Zhou, Q., and Li, Z. (2021). The impact of industrial structure upgrades on the urban-rural income gap: an empirical study based on China’s provincial panel data. Growth Chang. 52, 1761–1782. doi: 10.1111/grow.12502
Keywords: Internet use, income, RIF method, CFPS, China
Citation: Zhang A, Chandio AA, Yang T, Ding Z and Liu Y (2023) Examining how internet use and non-farm employment affect rural households’ income gap? Evidence from China. Front. Sustain. Food Syst. 7:1173158. doi: 10.3389/fsufs.2023.1173158
Edited by:
Shah Fahad, Leshan Normal University, ChinaReviewed by:
Devi Prasad Dash, Indian Institute of Technology Jodhpur, IndiaHuasheng Zeng, Yangzhou University, China
Asad Amin, Nanjing University of Aeronautics and Astronautics, China
Copyright © 2023 Zhang, Chandio, Yang, Ding and Liu. This is an open-access article distributed under the terms of the Creative Commons Attribution License (CC BY). The use, distribution or reproduction in other forums is permitted, provided the original author(s) and the copyright owner(s) are credited and that the original publication in this journal is cited, in accordance with accepted academic practice. No use, distribution or reproduction is permitted which does not comply with these terms.
*Correspondence: Abbas Ali Chandio, YWxpY2hhbmRpb0BzaWNhdS5lZHUuY24=; Yan Liu, MTM4MjlAc2ljYXUuZWR1LmNu