- 1Leibniz Centre for Agricultural Landscape Research (ZALF), Program Area 2 “Land Use and Governance”, Working Group “Sustainable Land Use in Developing Countries”, Müncheberg, Germany
- 2Department of Human Nutrition and Consumer Sciences, Sokoine University of Agriculture, Morogoro, Tanzania
- 3Humboldt-Universität zu Berlin, Thaer-Institute, Division Urban Plant Ecophysiology, Berlin, Germany
Adopting processing technologies and innovative food preservation is crucial for improving the food security and nutritional status of rural populations in Tanzania and other countries in the Global South. However, low adoption rates among smallholders highlight the need for a better understanding of farmers’ decision-making processes. The aim of this study is to examine extrinsic and intrinsic factors influencing smallholders’ decision-making processes in the adoption of innovative food processing and preservation techniques (specifically, pigeon pea flour-based products, threshers, dehullers) in Mitumbati and Mibure in the Lindi Region in Tanzania. Primary data on 555 farm households were collected using a standardized survey. Extrinsic influential factors were analyzed using binary logistic regression analysis. The results on internal decision-making are based on an analysis of barriers and motivations identified by farmers in relation to the uptake of the different innovations. Training and awareness emerged as the most significant factors positively associated with the adoption of all innovative processing and preservation techniques. Moreover, the results show that the primary drivers for smallholders in the study region to adopt innovative technologies were the potential health benefits and time savings they offered. The main challenge they faced was a lack of knowledge about the innovations. The results indicate that disseminating knowledge is crucial for the successful adoption of innovative processing technology in the study region. Improving and expanding training programs to be more inclusive can help to create incentives and overcome barriers, leading to increased adoption.
1 Introduction
The adoption of new processes and technology can play a crucial role in supporting sustainable agriculture and promoting economic growth in African countries. By increasing yields and productivity, improving access to markets, and improving better risk management, the adoption of new technologies can bring many benefits to smallholder farmers and contribute to food security in the region. Agricultural productivity and related food and nutrition security are limited by poor harvest and processing practices, limited storage and food preservation techniques, as well as a lack of dietary knowledge (Okoedo-Okojie and Onemolease, 2009; Affognon et al., 2015; Adeyeye, 2017; Ambuko, 2017).
There are various factors influencing the adoption of agricultural innovations, which can be categorized into extrinsic and intrinsic factors. Extrinsic factors include personal, physical, institutional, and socio-economic characteristics of adopters and their environment (Feder et al., 1985; Sheikh et al., 2003). Farmers’ knowledge, perception and attitudes towards an agricultural innovation can be classified as intrinsic factors (Adesina, 1995; Meijer et al., 2015; Jha, 2021). The extent to which the different factors impact adoption is highly context-dependent, including, among others, on the type of innovation, on the cultural context, and on the geographic conditions (Feder and Umali, 1993; Ruzzante et al., 2021). Studies tend to focus on the influence of extrinsic factors, whereas the impacts of intrinsic factors are still understudied (Adesina, 1993, 1995; Meijer et al., 2015; Bisheko and Rejikumar, 2023). Existing literature on agricultural innovation adoption primarily examines practices and cultivation methods aiming to increase agricultural production (Awotide et al., 2012; Mottaleb, 2018; Zegeye et al., 2022). There is comparatively little existing research on the adoption variables of agricultural practices at the post-harvest level (Okoedo-Okojie and Onemolease, 2009; Arslan, 2020). The study of Bisheko and Rejikumar (2023) highlights a notable scarcity of research dedicated to the adoption of processing technology among smallholder farmers in sub-Saharan Africa and South Asia.
In Sub-Saharan Africa (SSA), post-harvest losses are estimated to range between 30 and 50%, exceeding the global average (Deloitte South Africa, 2017). The impact on small-scale farmers is particularly harsh, as their livelihoods are heavily dependent on food production (Sheahan and Barrett, 2017). While food losses can occur throughout the entire value chain, in smallholder farming systems these primarily originate from poor harvest and processing practices, alongside limited storage and food preservation techniques (Adeyeye, 2017; Ambuko, 2017). Enhancement of existing techniques through innovative processing and preservation methods bears the great potential of not just preserving agricultural goods and their nutritional quality but also further ensuring year around availability (Adeyeye, 2017). Further, farmers’ livelihoods and income levels can be significantly impacted through the uptake of post-harvest value addition technologies (Agor et al., 2020). However, despite these potentials, the actual adoption of innovative practices by smallholders is relatively slow (Meijer et al., 2015; Dhehibi et al., 2019). Understanding farmers’ decision-making processes alongside the determinants and barriers of agricultural innovation adoption can pave the way to more successful implementation (Dhehibi et al., 2019; Arslan, 2020).
Pigeon peas (Cajanus cajan L.) (PP) are legumes that are widely cultivated in semi-arid regions (Sharma et al., 2011; Sarkar et al., 2018). They are rich in fiber, vitamins (niacin and riboflavin), minerals (phosphorus, iron, and magnesium), and essential amino acids (lysine, methionine, and tryptophan) (Saxena et al., 2002; Karri and Nalluri, 2017). Due to their nutritional properties, they can contribute significantly to food and nutrition security. Tanzania (5.16 kg/year) holds the third highest per capita consumption rate of pigeon peas in SSA after Malawi (22.35 kg/year), and Kenya (6.72 kg/year) (Indexbox, 2018). In the agricultural season 2019 to 2020, the total harvested area of pigeon peas in Tanzania was 97,092 ha. After the Mtwara region, and the Manyara region, the Lindi region has the third largest production area for pigeon peas (16,540 ha, 17.2%) (URT, 2021). The Lindi region is a high production area accounting for almost half of the national pigeon pea production in Tanzania (Mponda et al., 2014). They are primarily cultivated for domestic consumption and as a source of revenue (Mergeai et al., 2001; Shiferaw et al., 2007). However, in Tanzania legumes, including pigeon peas, are still not widely consumed (Mfikwa and Kilima, 2014; Majili et al., 2020, 2022). Consumption is particularly low during the lean season due to high post-harvest losses and a lack of diversified and shelf-stable pigeon pea products (Majili et al., 2020, 2022). Due to the high nutritional value and the wide cultivation in the Lindi region, pigeon peas were selected as a core crop for processing technologies and products in the Vegi-Leg project, which seeks to improve the food and nutrition security situation of pigeon pea farmers in the Lindi region. This study is embedded in the final phase of the Vegi-Leg project after the identification, development, and implementation of pigeon pea processing technologies and products using co-design to assess the adoption of improved technologies and processing for reducing post-harvest loss and improving the nutritional value, thus enhancing food and nutrition security.
Pigeon pea farmers in the study region rely heavily on traditional processing and preservation methods, leading to time-consuming practices and significant post-harvest losses. To address this, threshers and dehullers were selected, developed, and implemented in a participatory approach, aiming to provide innovative techniques to meet the needs for improved processing options of target groups. Additionally, the introduction of pigeon pea flour-based products aimed to diversify diets and offer shelf-stable, nutritious options during the lean season. Threshers were petrol-driven and able to process up to 800 kgs of produce per hour, while dehullers were electric and could treat a maximal quantity of 300 kgs per hour. As part of the project, farmers were trained in machinery usage, with selected individuals forming “processing groups” in order to train others (train-the-trainer concept). Maintenance and coordination of machinery were assigned to specific individuals or groups in each village. A fee was charged based on grain weight to cover fuel expenses. The project’s selection process of pigeon pea flour-based products involved a participatory approach, incorporating input from the local population (Majili et al., 2022). The production process involves pre-treatment, milling, and sieving to obtain pigeon pea flour, which can then act as a substitute for, or added to, wheat flour. Farmers were familiarized with simple recipes to create a range of food items based on PP flour. Training sessions were conducted to facilitate the adoption and dissemination of the production process among farmers. Underlying this study’s main objective, there are two research questions. (i) What is the adoption rate of newly introduced post-harvest technologies and pigeon pea flour-based products by farmers in the Lindi region (Tanzania) and (ii) How do extrinsic and intrinsic factors impact farmers’ adoption? The study was conducted in the frame of a research project focusing on the development of processing technologies to improve the nutrient quality of products and the perennial availability of pigeon peas. Using co-design, two different pigeon pea processing technologies, namely pigeon pea threshers, and dehullers, alongside innovative pigeon pea flour-based products (instant porridge, noodles, Maandazi, Bhajia, Chapati, bread, and biscuits) were selected, developed, and implemented in the project setting.
By assessing the adoption rate and drivers for adoption, the study results contribute to the improvement of implementation processes of innovative processing technologies and products to sustainably transform elements of the pigeon pea value chain, addressing food and nutrition insecurity on the ground.
2 Methods
2.1 Research framework
The empirical literature on agricultural technology uptake in the Global South identifies a variety of extrinsic factors impacting adoption, including the socio-economic characteristics of adopters (e.g., age, gender, education) (Doss, 2001b; Abdulai and Huffman, 2014; Jha, 2021), farm specific determinants (land size, labor availability) (Feder et al., 1985; Okoedo-Okojie and Onemolease, 2009; Raghu et al., 2014), and extension effects (e.g., training, familiarity) (Yaron et al., 1992; Imaita, 2013; Pignatti et al., 2015). There is relatively little research that emphasizes the significance of farmers’ internal attitudes and perceptions when it comes to adopting technology (Adesina, 1995; Meijer et al., 2015; Jha, 2021), but some find an impact of these factors in multiple settings (Kulshreshtha and Brown, 1993; Ammann et al., 2022). Additionally, a multitude of concepts strive to explain the interrelations of these variables within farmer decision making regarding the uptake of innovations [e.g., Rogers (2003), Fishbein and Ajzen (1975), Meijer et al. (2015)]. Rogers (2003) finds five distinct elements that impact the rate at which an idea is adopted: relative advantage, compatibility, complexity, trialability, and observability. The theory of reasoned action (TRA), by Fishbein and Ajzen (1975), highlights the importance of an individual’s attitude and subjective norms toward a behavior as being significant factors for decision-making. Yet, the importance of their influence depends on the agricultural innovation in consideration, geographical location, and other context specific aspects (Feder and Umali, 1993; Ruzzante et al., 2021).
In this study, to investigate the drivers for agricultural technology adoption, it is important to not only consider extrinsic factors, such as the farmers’ socioeconomic characteristics and farm environment but also intrinsic factors based on their perceptions of pigeon pea innovative produce and processing technology. Further additional factors for communication and extension should be incorporated. Therefore, to assess adoption behavior and those underlying drivers impacting its degree, a simplified version of the analytical framework proposed by Meijer et al. (2015) is applied. This framework fits well as it understands farmers’ decision-making as a complex nonlinear process and distinguishes between extrinsic and intrinsic influential factors. The intrinsic factors, namely farmers’ knowledge, perceptions, and attitudes, build the basis, directly influencing farmers’ adoption decisions, which are influenced by a range of extrinsic factors, such as farmers’ characteristics, environmental characteristics, as well as intervening factors communication, and extension. Thus, the chosen framework helps to analyze the complex adoption process in a systematic approach while structuring different impact factors. Meijer et al. (2015) include another group of influential extrinsic factors, referring to the characteristics of the agricultural technology, more precisely its costs and benefits. For the purpose of this study, the framework was simplified, omitting these characteristics due to the co-design approach of this research. The development of innovations was carried out in a participatory approach with all relevant stakeholders. As potential adopters were included in this process, costs and benefits were considered to be aligned with their needs.
2.2 Study area
This study was conducted in two villages of the Ruangwa (Mibure) and the Nachingwea (Mitumbati) districts of the Lindi Region in Tanzania (Figure 1). Target households are mainly engaged in subsistence farming or small-scale farming to create income and provide food for household consumption. The prevalence of malnutrition in the Lindi region is particularly severe with the prevalence of high stunting in children under the age of five (54%) (URT, 2016; Indili et al., 2018). The region is semi-arid and districts were purposefully selected due to market accessibility and for being the main pigeon pea producers in the region. The villages were chosen based on their high production volume of pigeon peas, which are cultivated during the rainy season (December–March) and intercropped with maize [compare Majili et al. (2020, 2022)].
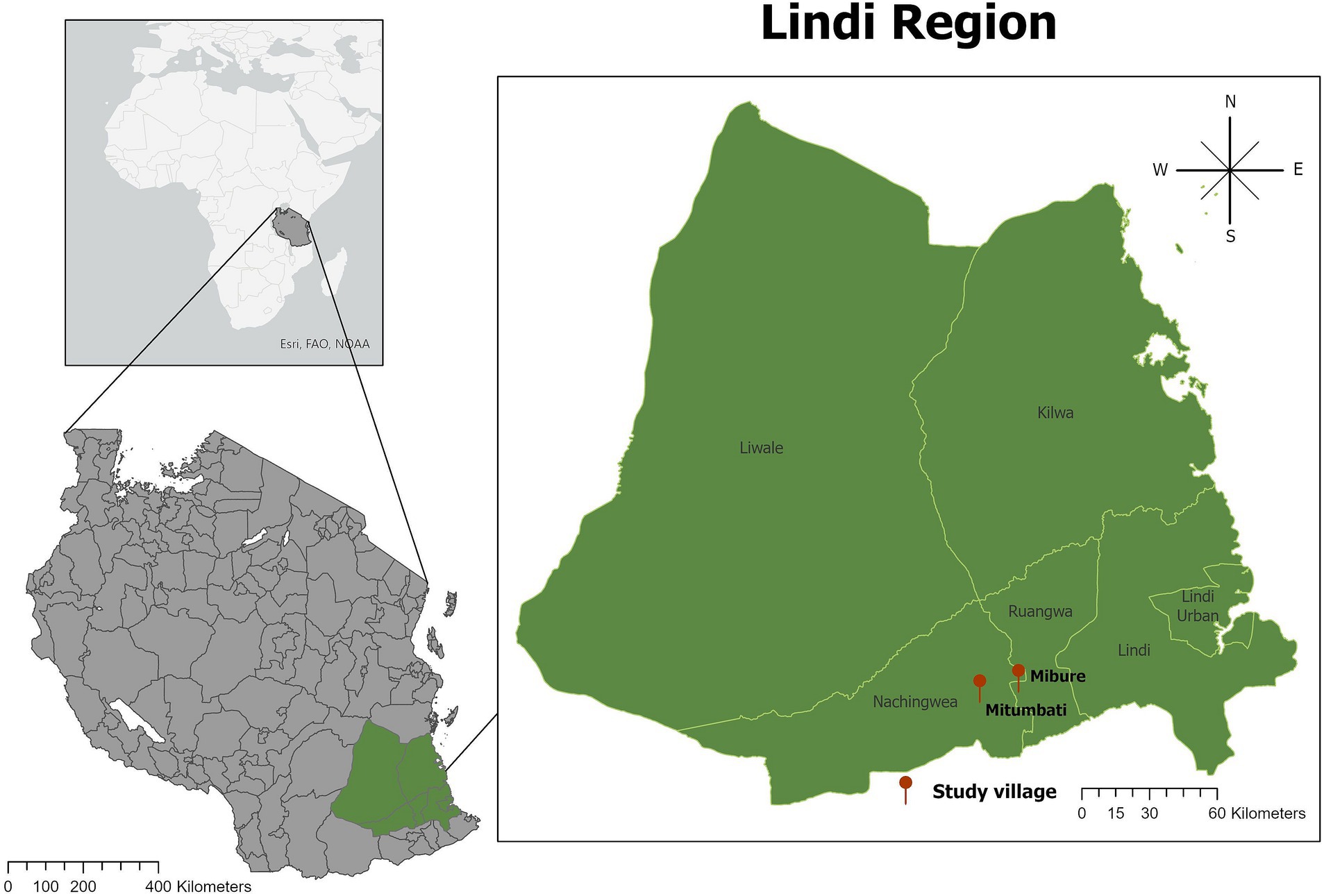
Figure 1. Geographic location of the study region Lindi Region in southeast Tanzania (green) and study villages (red) (Resource: Geo dataset: Humanitarian Data Exchange (2022)).
2.3 Data collection and sample
This study was embedded in the final phase of the Vegi-Leg project, which emphasized to select, develop and implement nutrition-sensitive post-harvest pigeon pea technologies and products for improved nutrition security using co-design. Within the entire project framing, a baseline and endpoint survey were conducted using a random sampling procedure to analyse the impact of innovations. Co-design was applied for the problem definition, selection, development, and implementation of post-harvest interventions by all relevant stakeholders such as pigeon pea farmers, local policymakers, extension services, and academia. To address the fostering and hindering factors for adoption, this study was enclosed in the endpoint survey of the Vegi-Leg project (random selection procedure).
The study population comprises female and male pigeon pea farmers (n = 555) who were randomly selected. Survey participants were part of a research project aiming at the development of processing technologies and preservation techniques to improve the nutrient quality of products and the perennial availability of pigeon peas. The key inclusion criterion for participation in the project is that the farmer had grown pigeon peas in the last 3 years prior to the project’s start. As part of the endline survey of the project in August 2021, a quantitative household survey was used to collect information on farmers’ adoption behavior and related extrinsic and intrinsic influential factors. The survey consisted of several sets of structured questionnaires, with data collected by 15 project enumerators. Interviews were approximately 90 min long and carried out in Swahili.
One part of the survey focused on the collection of extrinsic factors, such as information on household demographics, PP production, processing, consumption, extension, and experience with technologies. The variables examined within this study are listed in Table 1 below and were chosen based on extensive literature research, as they are already linked to adoption in a variety of studies [e.g., Feder et al. (1985), Okoedo-Okojie and Onemolease (2009), Raghu et al. (2014)]. Adoption was determined as a binary categorical variable referring to whether the farmer is using an innovative practice or not. Apart from the questionnaire on socio-economic information, intrinsic factors are assessed as farmers’ perceptions and attitudes toward adoption. Survey participants received an additional questionnaire asking for 1) drivers and 2) barriers related to the use of processing and preservation innovations. This section included predefined response options that are aligned with the conceptual and theoretical background of this study (Leite et al., 2014; Meijer et al., 2015; Antwi-Agyei et al., 2021). The entire survey was carried out according to the guidelines laid down in the ‘Declaration of Helsinki’ and approved by the National Institute for Medical Research, Dar es Salaam, and the Ministry of Health, Community Development, Gender, Elderly and Children in Dodoma, Tanzania (NIMR/HQ/R.8a/Vol.IX/3040). Written informed consent was obtained from all farmers.
2.3.1 Descriptive statistics
Descriptive statistics are used to describe the characteristics of the households and analyze the adoption rates. Continuous variables, like age, household size, and farm size, are analyzed using averages and standard deviations, whereas, for categorical variables (education, literacy, gender, off-farm income, and awareness of technology), frequencies and percentages are calculated. Likewise, to identify the key intrinsic factors driving technology adoption, the information from farmers’ interviews on motivation and barriers to innovation uptake was analyzed using frequency analysis.
2.3.2 Binary logistic regression
A logistic regression model was constructed for each innovation (threshers, dehullers, and PP flour-based products) to determine those key factors that influence farmer adoption in the region. The use of qualitative response models is necessary to model the connection between a farmer’s decision to adopt or not adopt innovative technology and the independent variables (Ntshangase et al., 2018). In this research, a logit model is used. Logit models are widely applied in adoption technology studies because they are well-suited for analyzing binary outcomes and due to their simplicity in application (Sheikh et al., 2003; Mlenga and Maseko, 2015; Ntshangase et al., 2018). The model allows researchers to estimate the probability of adoption from a set of independent variables, such as demographics, socioeconomic status, and attitudes toward the innovation. Logit models are also able to account for non-linear relationships between these factors and adoption, making them a useful tool for identifying the key drivers of technology adoption in different contexts.
From the input explanatory variables, the binary logistic regression model builds a logistic probability function for the dependent variable of innovation adoption. In line with Baltagi (2021), Gujarati and Porter (2009), and Laduber et al. (2016), the general logistic distribution equation for the adoption of agricultural technologies is expressed as follows in Equation 1:
Where, = is the dichotomous dependent variable that refers to whether the farmer is using a technology/product (innovation adoption = 1, and 0 = otherwise); P = Probability of adopting; 1-P = Probability farmer of not adopting; = Constant term (intercept); … = coefficients of the explanatory variables; X1…Xn = explanatory variables; and = error term of the model.
In this study, the extrinsic explanatory variables of importance are those that are assumed to influence the adoption of the respective innovative processing practices. Table 1 presents the explanatory variables and factors hypothesized to impact adoption.
The logistic regression model for the Adoption of the different innovative technologies is specified in Equation 2 as:
Within the scope of this study, three regression models were designed to calculate farmers’ likelihood of Adoption for each innovation. The explanatory variables were tested to ensure compliance with technical requirements for the data, set such as a lack of significantly influential outliers, the linearity of the logit for continuous variables, the absence of multicollinearity, and the independence of errors (Stoltzfus, 2011; Laduber et al., 2016; Fernandes et al., 2020). In line with Baltagi (2021), Laduber et al. (2016), and Okoedo-Okojie and Onemolease (2009), the models were assessed for their goodness of fit using the Omnibus test (likelihood-ratio χ2 test), the overall model correct prediction, and the Hosmer-Lemeshow test. The statistical software package SPSS version 27.0 was used for the econometric analysis.
3 Results
3.1 Socioeconomic characteristics of the respondents
Information on the socioeconomic characteristics of the respondents is listed in Table 2. The age of the household head ranged from 21 to 99 years, with an average of 51 years (±17.88 years SD). At 79.5%, most households are headed by men; the remaining fifth are led by women. The average respondent’s household consisted of three persons, with a maximum of twelve persons (±1.43 SD). More than 80% of the respondents had a primary school degree or at least received some primary education, whereas one-eight had no formal schooling. At 55.7%, the majority of respondents are able to read and write, another fourth at least to some extent, and one-fifth of the respondents are illiterate. Household farm size from 0.1 to a maximum of 18.41 ha with an average of 1.9 ha (±1,69 SD). For the majority of survey respondents (79.6%), farming is the only source of income for the household. The remaining 20.4% of respondents generated additional income through other activities. Two-thirds of the households had no prior experience in the use of machinery for farming or processing purposes. Results show that 33.9% were experienced in agricultural technology use prior to the implementation of processing machinery.
3.2 Adoption rates
Figure 2 presents the adoption rate of innovation technologies in both villages. According to the results, the highest adoption rate was recorded for PP dehullers at 78%. Further, it is shown that more than two-thirds of households adopted PP threshers. The lowest adoption rate is found for the PP flour-based products: only 47.4% of the respondents have adopted it. Moreover, as shown in Figure 2, the adoption rates for all technologies are generally higher in Mibure than in Mitumbati.
3.3 Extrinsic factors influencing farmers’ adoption of processing technology
In order to identify the key extrinsic factors driving technology adoption, explanatory variables were analyzed using binary logistic regression analysis. The results of all binary logistic regression models for the adoption of PP flour products and processing technologies are summarized in Table 3, presenting odds ratios of regression coefficients (Exp (B)) and variable significance. All models were statistically significant (PP flour-based products production, χ2 (15) =181.632, p < 0.001; PP threshers, χ2 (15) =143.069 p < 0.001 and PP dehullers, χ2 (15) =76.056 p < 0.001). The overall percentages of model correct predictions were 73.7% for PP threshers, 74.4% for PP flour-based products, and 79.1% for PP dehullers. Thus, for all regression models, more than two-thirds of the sample data are correctly classified into the respective groups of adopters and non-adopters.
As presented in Table 3, it was found that regardless of the practice implemented, training (p ≤ 0.1) and awareness (p < 0.01) of the technology tended to be the most important factors for their uptake. Households that attend training sessions are shown to be more likely to adopt the use of innovative processing technologies. As indicated through the odds ratios of coefficients, farmers who receive training in the production of PP flour-based products are 2.1 times more likely to adopt production than non-trained farmers. For the adoption of PP threshers (3.8 times) and dehullers (3.3 times), training impacts the likelihood of adoption even more. Awareness about an innovation further increased the likelihood of technology adoption by 6.7 times for PP flour-based products, 6.8 times for threshers, and 3.7 for dehullers.
Generally, the largest number of significant extrinsic influential factors were predicted by the logit model for PP flour products. It is found that the literacy level of respondents significantly influences (p < 0.05) their decision to produce PP flour-based products. Further, the likelihood of adopting the production of PP flour-based products increases by approximately two times (p < 0.01) if a respondent is literate compared to those who are only somewhat literate (Exp (B) = 0.494). An increase in farm size (p < 0.1) negatively affects farmers’ decision to produce PP flour-based products. The odds ratio of the coefficient (0.88) indicates that for every additional hectare of agricultural land, the probability of adoption is reduced by 12%. Households that generate off-farm income (p < 0.05) are about 1.9 times more likely to produce PP flour products than those that are exclusively occupied with agricultural production. By possessing prior knowledge of machinery, households increase their probability of adopting PP flour production by 1.6 times compared to households without technical knowledge.
Further extrinsic influential factors were estimated through the logit model for the adoption of PP threshers. The odds for the variable age (p < 0.1) are 0.978, indicating that with every additional year of the farmers’ age, the probability of adopting the use of threshers decreases by 1.3%. Additionally, female-headed households are 36.5% less likely to adopt PP threshers (Exp (B) = 0.635) than those led by male farmers.
3.4 Intrinsic factors influencing farmers’ adoption of processing technology
A variety of different intrinsic drivers (Figure 3) and barriers (Figure 4) to the adoption of the different PP processing technologies were identified, each contributing to adoption with varying degrees. As shown in Figure 3, more than one-fourth of the respondents stated that the health benefits were the most motivating factor to produce PP flour-based products. Another 21.1% of the respondents named the generation of additional income through selling the product as an incentive. The main motivating factor for the adoption of threshers and dehullers (66.8%) was the time savings they offer compared to traditional PP processing methods. Other households (16%) preferred the improved sensory attributes of machine processed PP. Further, with similar percentages for PP processing technologies (8.6%) and PP flour-based products (7.9%), the longer shelf life of processed goods motivated households’ adoption decision.
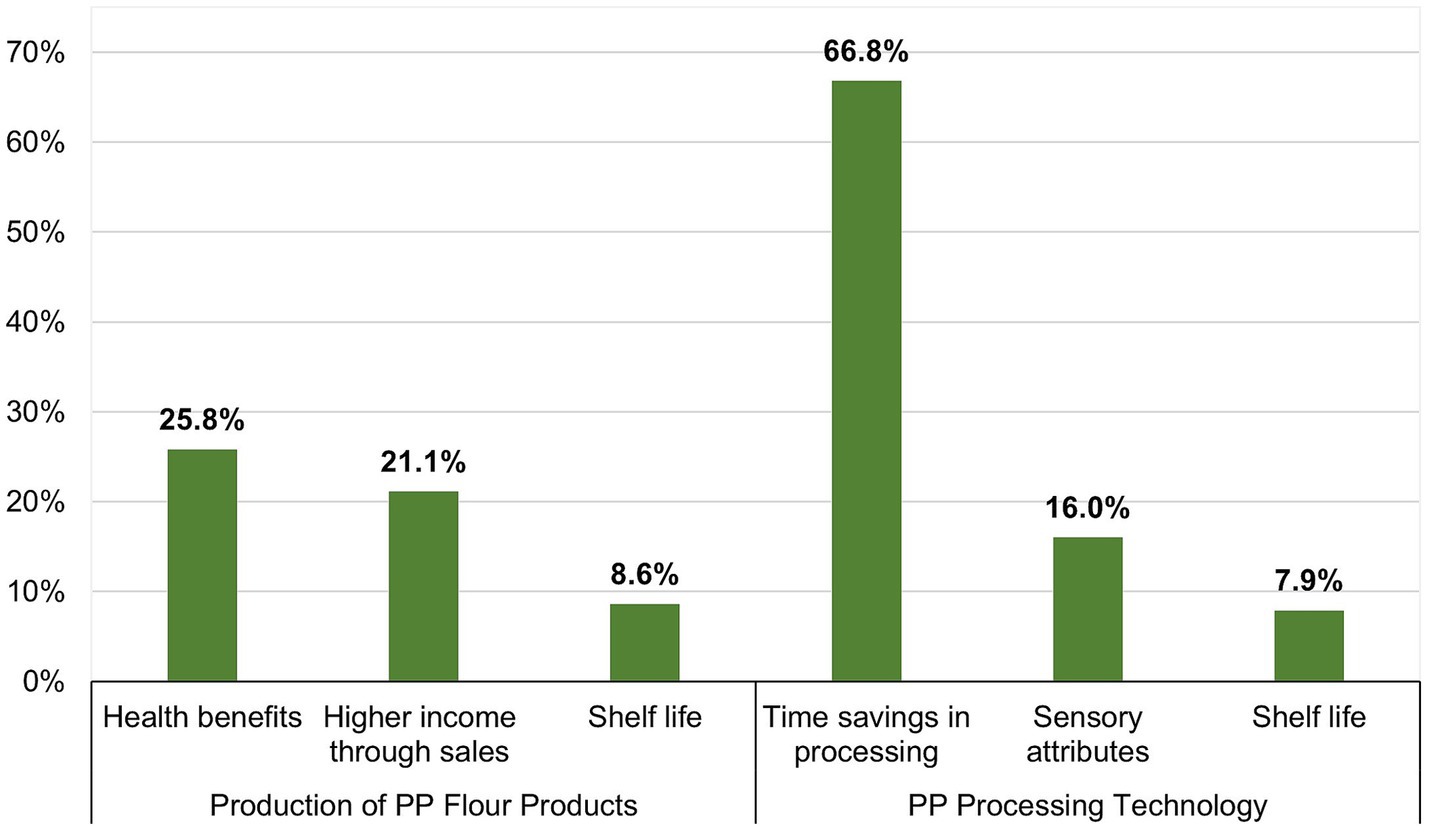
Figure 3. Motivating factors influencing the adoption of innovative pigeon pea processing technology and products.
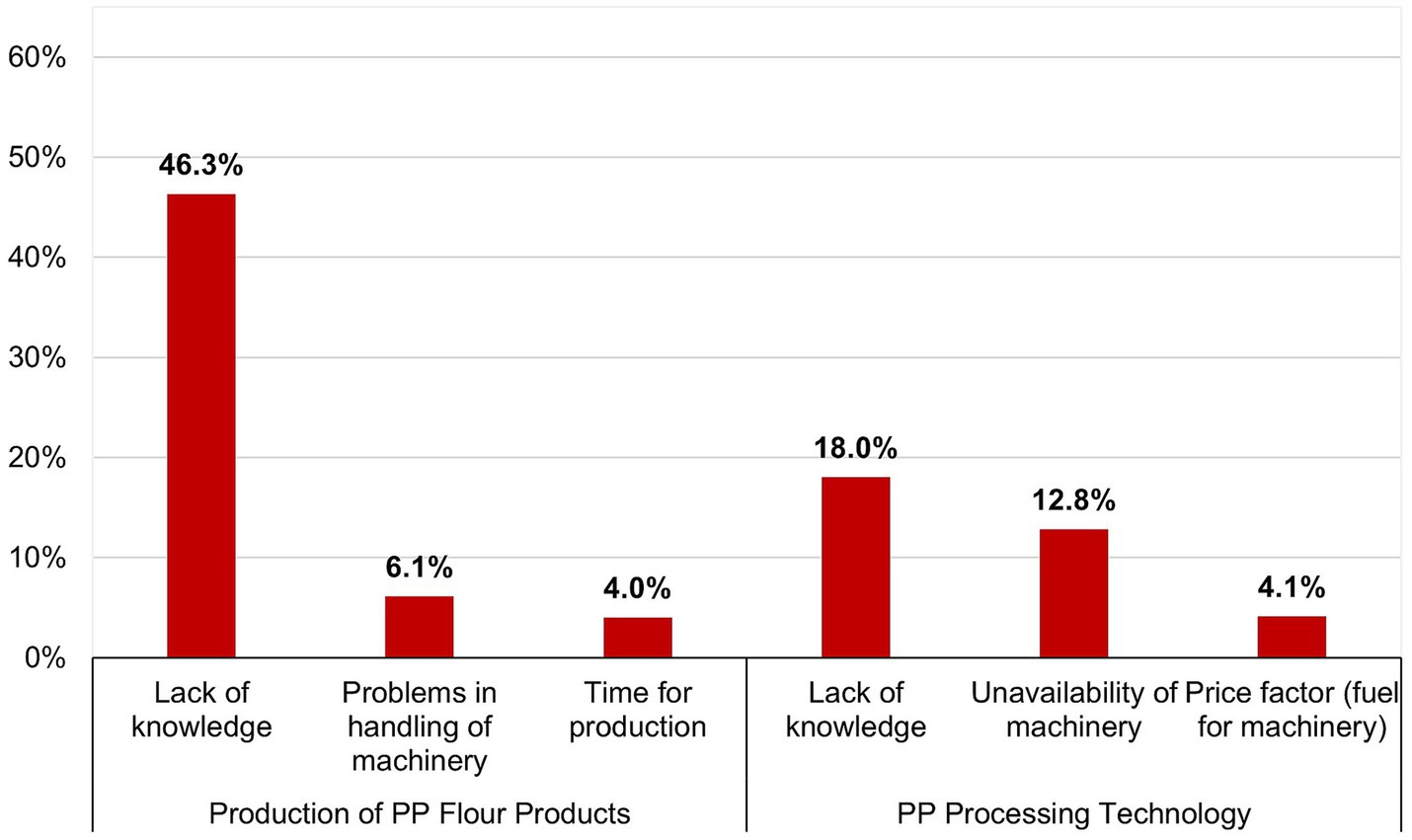
Figure 4. Hindering factors influencing the adoption of innovative pigeon pea processing technology and products.
The largest factor that prevented the households from producing PP flour-based products (46.3%) and PP processing technology (18.0%) was a lack of knowledge. Further, households faced problems with the handling of machinery for PP flour products production (6.1%) or perceived the production of these products as too time-consuming. Other barriers to the use of processing machinery were their unavailability (12.8%) and input prices for fuel (4.1%).
4 Discussion
This study aims, first, at assessing the adoption rates of innovative PP flour-based products and processing technologies in two villages (Mitumbati and Mibure) in the Lindi Region of Tanzania and, secondly, understanding the extrinsic and intrinsic drivers of their adoption. The examined processing technologies – PP threshers and dehullers – were well received by the study population, whereas the adoption of innovative PP processing, like flour-based products, was significantly lower. Overall, the most significant extrinsic factors influencing the adoption of innovative processing technologies and products were training and awareness of the technology, irrespective of the agricultural post-harvest innovation examined. The use of processing machinery was influenced by fewer external factors than the uptake of the new production processes for PP products. A deeper insight into farmers’ perceptions, knowledge, and attitudes shows that technology adoption is mainly driven by health benefits assigned to the products and time savings of machinery use; simultaneously it is hindered by a lack of knowledge about the innovations as well as their unavailability. Farmers considered these factors as more important in relation to their adoption decision than financial incentives and barriers.
It is found that extension measures, like training and awareness building, are critical measures for adoption. Despite an implemented training program for the target households, it is found that the most important factor hindering the adoption of technologies is a lack of knowledge about the innovations. These findings are in line with the results of Singh and Yadav (2014) and Kragt et al. (2017), both finding that a knowledge gap impedes adoption, especially in relation to risks and uncertainties in farmers’ production outcomes. Rogers (2003) states that most people refrain from innovation adoption due to a fear of unknown future risks. This further implies that farmers in the study region may perceive that they require more knowledge and skills for technology adoption, for instance in order to reduce risks related to the input of goods or capital (Lalani et al., 2016). The knowledge gap also leads to other assumptions that farmers expressed as barriers to the use of technology, such as problems in handling the machinery for PP flour products production. Another misconception is that farmers perceived the production of PP flour products as too time consuming, although the production time of PP flour products is almost equal compared to traditionally used practices. Thus, misinformation and insufficient knowledge underlie farmers’ subjective interpretation of reality, negatively affecting farmers’ adoption decisions. This is consistent with a broad range of evidence; in particular, suggesting farmers’ attitudes and their negative perceptions are major constraints to technology adoption (Adesina, 1993; Kulshreshtha and Brown, 1993; Mottaleb, 2018). In line with this finding are the results of this study, which identified training and awareness as most significant extrinsic factors, directly influencing farmers’ intrinsic perceptions, knowledge, and attitudes towards innovation. These findings are in line with the results of Parwada et al. (2010), who find that the adoption of agroforestry technology is significantly higher for trained farmers. Okoedo-Okojie and Onemolease (2009) find that contact with extension agents increases the adoption of Yam storage technologies by 79%. Training in the use of agricultural innovation plays an important role in capacity building and strengthens the farmer’s knowledge (Maguire, 2012). As it can be seen as a prerequisite for awareness that farmers are exposed to the use of an innovation through training, users, or another source of information, the variable integrates the impact of informal information dissemination on adoption (Halloran et al., 2021). The project implemented one thresher and one dehuller per village. Therefore, these machines were shared at the village level and individual use was limited in time. Due to high demand of farmers, threshers and dehullers were often occupied and thus not equally available to every individual farmer (unavailability).
In line with the findings of Voh (1982) and Weir and Knight (2004), this study finds that the likelihood of adopting the production of PP flour products is higher for literate farmers than for only somewhat or illiterate farmers. Since recipes involve multiple steps, it is important to remember them accurately. Literate farmers are at an advantage, as they have the possibility to write down recipes and new production processes, such as making PP flour noodles, where it might be important to keep a record of the process until it is internalized. Moreover, this study finds that farmers generating additional income besides farming are more likely to adopt the production of PP flour-based products, which is consistent with the findings of Fernandez-Cornejo et al. (2007) who find that the adoption of improved soybean varieties is positively impacted by the generation of off-farm income. Feder et al. (1985) argue that off-farm income may help finance initial fixed costs. As for value added products, like Maandazi, Bhajia, Chapati, or biscuits, farmers must purchase additional ingredients (oil, wheat, sugar), all of which must be financed by their own resources. Farm size negatively affects the adoption of PP flour-based products. Although a variety of studies find that farm size positively affects technology adoption (Okoedo-Okojie and Onemolease, 2009; Raghu et al., 2014; Hu et al., 2022), the literature review of Feder et al. (1985) arrives at mixed results: size can act in both directions. Ntshangase et al. (2018) argue that larger farms also have higher labor requirements, which may discourage farmers from adopting no-till conservation agriculture as it takes additional time and labor resources. Farmers’ technology skills positively affect the production of PP flour-based products. Several studies find that the farming experience of farmers positively impacts technology adoption (Adetunji, 2009; Okoedo-Okojie and Onemolease, 2009; Ajibesin et al., 2019). Pignatti et al. (2015) report that an individual’s skillset is a crucial factor for technology adoption. Agor et al. (2020) find that the probability of uptake for value adding sweet potato processing technology is higher for individuals with prior experience in processing. As PP flour production requires the use of a miller, farmers who are already familiar with technical equipment may be more likely to have the confidence to use the machine. The results of this study indicate that older age negatively affected farmers’ probability of adopting the use of threshers. Therefore, older farmers at the study sites are less likely to use threshers than younger farmers. Based on our field observations, the reasons for this phenomenon may be attributed to heightened risk aversion among elderly farmers in the study region, possibly influenced by concerns about potential failures or losses associated with the adoption of threshers. Moreover, they might prefer the traditional farming methods they have used throughout their long farming careers. Additionally, a lack of training and education in modern farming techniques might hinder their ability to use these tools effectively. Kinyangi (2012) argues that older farmers may be hesitant to try a completely new practice since they have invested several years in perfecting the traditional method. These findings are consistent with the results of Okoedo-Okojie and Onemolease (2009), which find that older farmers tend to be less willing to take risks than younger farmers. Another reason might be that old age is often connected to poor health conditions. Abdulai and Huffman (2014) state that households may lose valuable labor due to illness and, therefore, poor health acts as a barrier, leading to low adoption rates. However, as the use of threshing machines in this study is less time and labor intensive than the traditional processing method, a lack of knowledge of elderly farmers might also have contributed to low adoption rates. For the use of threshers, the results show that households that have a male household head are 1.6 times more likely to use the threshers for PP processing than those led by female farmers. These findings are in line with studies from Doss (2001a) and Kumar (1994). Doss (2001b) report that adoption decisions are not solely connected to gender, but can rather be related to other gender-linked factors, like restrained access to resources (e.g., extension contacts, education, land size, labor force).
In this study, health benefits were one of the major enabling intrinsic factors for adoption. Improving someone’s health turns out to be a motivating factor to produce PP flour products. The same applies to the factor of a longer shelf life, which is also mentioned as a motivating factor for all innovations. These findings are consistent with the results of Mellon-Bedi et al. (2020), who find that improvements in household dietary diversity are an enabler for the adoption of sustainable intensification practices in Ghana. Further, Greiner and Gregg (2011) report that farmers’ decisions are motivated by the active pursuit of personal and family well-being. This underlines that knowledge building through training measures and /or information dissemination surrounding the use of processing innovations is a crucial element for adoption. As nutrition education is an important part of the training curriculum, a large proportion of the farmers had knowledge about the health benefits processed goods provide. Sensory attributes of processed pigeon pea products are motivating factors for the adoption of processing machinery. This finding supports Mellon-Bedi et al. (2020), who find that fulfillment of farmers’ personal satisfaction is an enabler for adoption. As farmers were able to taste the products produced with innovative processing technologies, they were directly rewarded with their benefits in terms of sensory attributes. This is in line with Rogers (2003), who states that the degree to which an innovation provides tangible results is an important factor in its adoption. Moreover, as perceptions about taste can be different for every farmer, these findings highlight the importance of subjective opinions within the adoption process. For PP processing machinery, farmers considered time savings through reduced labor as a main motivating factor for adoption. This is in line with the concept of the “relative advantage” of innovation according to Rogers (2003), which the author determines as one of the elements impacting the rate of adoption. Thus, farmers are more likely to adopt new agricultural technology if they perceive that it offers more advantages than the current method while also saving them time and effort.
The results indicate that knowledge about the general use, the benefits, the risks, and related uncertainties are among the most important intrinsic factors shaping adoption decisions. This implies that well-adapted training interventions that sufficiently educate farmers have the potential to increase adoption rates. Farmers in the study region were also mainly motivated, among other factors, by the health benefits the processed goods provide. As nutrition education about the health benefits of PP products was part of the training curriculum, scaling up nutrition education could provide further incentives to adopt the improved technologies. To overcome the knowledge gap, it remains important to promote knowledge transfer on agricultural innovations and to explicitly communicate their benefits through training sessions. Based on the findings of this study, it is important to strengthen inclusivity within the training measures, especially regarding the integration of illiterate farmers, but also in terms of measures that explicitly target women or older farmers. Moreover, further incorporating other actors throughout the value chain, such as supermarkets and local media outlets, will increase accessibility to information, thus raising knowledge and awareness levels; this bears great potential to improve adoption on the ground. Further research on extrinsic and intrinsic adoption relevant factors can help to identify opportunities and challenges along the adoption process, thus providing an important basis to tackle existing problems and design future implementation processes for agricultural innovations in a sustainable and context-specific manner to address food and nutrition security.
Data availability statement
The raw data supporting the conclusions of this article will be made available by the authors, without undue reservation.
Ethics statement
The studies involving humans were approved by the National Institute for Medical Research, Dar es Salaam, and the Ministry of Health, Community Development, Gender, Elderly and Children in Dodoma, Tanzania (NIMR/HQ/R.8a/Vol.IX/3040) and carried out according to the guidelines laid down in the ‘Declaration of Helsinki’. The studies were conducted in accordance with the local legislation and institutional requirements. The participants provided their written informed consent to participate in this study.
Author contributions
MS and CR contributed to the conception and design of the study. CR raised the funds. ZM, HM, and JK collected the data. MS, NK, and CM analysed the data. MS wrote the first draft of the manuscript and CR, NK, CM, and KL critically reviewed and edited the manuscript. All authors contributed to the manuscript revision, read and approved the submitted version.
Funding
The authors acknowledge financial support from the Vegi Leg project, funded by the Federal Ministry of Food and Agriculture (BMEL) based on a decision of the Parliament of the Federal Republic of Germany via the Federal Office for Agriculture and Food (BLE) (grant number Vegi-Leg/2816PROC09). The publication of this article was funded by the Open Access Fund of the Leibniz Association.
Acknowledgments
We are grateful to the Leibniz Centre for Agricultural Landscape Research (ZALF) and the Sokoine University of Agriculture for the project Implementing innovative processing technologies for nutrient-dense plant foods (African indigenous vegetables and legumes) to safeguard perennial nutrition security (Vegi-Leg). In particular, we thank the farmers in Mitumbati and Mibure who took part in the survey and made this research possible. We extend our sincere thanks to the Open Access Fund of the Leibniz Association for their invaluable support in covering the publication fund.
Conflict of interest
The authors declare that the research was conducted in the absence of any commercial or financial relationships that could be construed as a potential conflict of interest.
Publisher’s note
All claims expressed in this article are solely those of the authors and do not necessarily represent those of their affiliated organizations, or those of the publisher, the editors and the reviewers. Any product that may be evaluated in this article, or claim that may be made by its manufacturer, is not guaranteed or endorsed by the publisher.
References
Abdulai, A., and Huffman, W. (2014). The adoption and impact of soil and water conservation technology: an endogenous switching regression application. Land Econ. 90, 26–43. doi: 10.3368/le.90.1.26
Adesina, A. (1993). Technology characteristics, farmers' perceptions and adoption decisions: a Tobit model application in Sierra Leone. Agric. Econ. 9, 297–311. doi: 10.1016/0169-5150(93)90019-9
Adesina, A. (1995). Farmers' perceptions and adoption of new agricultural technology: evidence from analysis in Burkina Faso and Guinea, West Africa. Agric. Econ. 13, 1–9. doi: 10.1016/0169-5150(95)01142-8
Adetunji, M. O. (2009). Determinants of the use of maize storage techniques by farmers in Kwara state, Nigeria. J. New Seeds 10, 31–40. doi: 10.1080/15228860802664798
Adeyeye, S. A. O. (2017). The role of food processing and appropriate storage technologies in ensuring food security and food availability in Africa. NFS 47, 122–139. doi: 10.1108/NFS-03-2016-0037
Affognon, H., Mutungi, C., Sanginga, P., and Borgemeister, C. (2015). Unpacking postharvest losses in sub-Saharan Africa: a Meta-analysis. World Dev. 66, 49–68. doi: 10.1016/j.worlddev.2014.08.002
Agor, E., Blessing, U., and Flora, N. N. (2020). Gender analysis in the adoption of Sweetpotato value addition technologies by rural farmers in Imo state, Nigeria. J. Community Commun. Res. 5, 1–8.
Ajibesin, D. T., Oluwasola, O., and Ajayi, D. (2019). Socio-economic factors determining the adoption of post-harvest technologies among maize farmers in Kwara state, Nigeria. IJAES 6, 8–17. doi: 10.14445/23942568/IJAES-V6I5P103
Ambuko, J. (2017). Why reducing post-harvest losses is a priority for Africa. Available at: https://theconversation.com/why-reducing-post-harvest-losses-is-a-priority-for-africa-87312 (Accessed February 17, 2023).
Ammann, J., Walter, A., and El Benni, N. (2022). Adoption and perception of farm management information systems by future Swiss farm managers – an online study. J. Rural. Stud. 89, 298–305. doi: 10.1016/j.jrurstud.2021.12.008
Antwi-Agyei, P., Abalo, E. M., Dougill, A. J., and Baffour-Ata, F. (2021). Motivations, enablers and barriers to the adoption of climate-smart agricultural practices by smallholder farmers: evidence from the transitional and savannah agroecological zones of Ghana. Reg. Sustain. 2, 375–386. doi: 10.1016/j.regsus.2022.01.005
Arslan, A. (2020). The adoption of improved agricultural technologies - a Meta-analysis for Africa. SSRN J. IFAD Research Series. 63. doi: 10.2139/ssrn.3681375
Awotide, B., Diagne, A., Wiredu, A. N., and Ojehomon, V. E. (2012). Wealth Status And Agricultural Technology Adoption Among Smallholder Rice Farmers In Nigeria. OIDA Int. J. Sustain. Dev. 2, 97–114.
Bisheko, M. J., and Rejikumar, G. (2023). Major barriers to adoption of improved postharvest technologies among smallholder farmers in sub-Saharan Africa and South Asia: a systematic literature review. World Dev. Sustain. 2:100070. doi: 10.1016/j.wds.2023.100070
Dhehibi, B., Ruediger, U., and Dhraief, M. Z. (2019). Factors influencing farmers’ decisions to adopt improved Technologies in Semi-Arid Farming Systems: A case study of the barley variety Kounouz and feed blocks Technology in Tunisia: Working paper. Cairo, Egypt: International Center for Agricultural Research in the Dry Areas (ICARDA).
Doss, C. R. (2001a). Designing agricultural Technology for African Women Farmers: lessons from 25 years of experience. World Dev. 29, 2075–2092. doi: 10.1016/S0305-750X(01)00088-2
Doss, C. R. (2001b). How does gender affect the adoption of agricultural innovations? The case of improved maize technology in Ghana. Agric. Econ. 25, 27–39. doi: 10.1016/S0169-5150(00)00096-7
Feder, G., Just, R. E., and Zilberman, D. (1985). Adoption of agricultural innovations in developing countries: a survey. Econ. Dev. Cult. Chang. 33, 255–298. doi: 10.1086/451461
Feder, G., and Umali, D. L. (1993). The adoption of agricultural innovations. Technol. Forecast. Soc. Chang. 43, 215–239. doi: 10.1016/0040-1625(93)90053-A
Fernandes, A. A. T., Figueiredo Filho, D. B., Da Rocha, E. C., and Da Nascimento, W. S. (2020). Read this paper if you want to learn logistic regression. Rev. Sociol. Polit. 28:6. doi: 10.1590/1678-987320287406en
Fernandez-Cornejo, J., Mishra, A. K., Nehring, R. F., Hendricks, C., Southern, M., and Gregory, A. (2007). Off-FARM income, technology adoption, and FARM economic performance. Unknown.
Fishbein, M., and Ajzen, I. (1975). Belief, attitude, intention and behavior: An introduction to theory and research. Reading (Mass): Addison-Wesley.
Greiner, R., and Gregg, D. (2011). Farmers’ intrinsic motivations, barriers to the adoption of conservation practices and effectiveness of policy instruments: empirical evidence from northern Australia. Land Use Policy 28, 257–265. doi: 10.1016/j.landusepol.2010.06.006
Gujarati, D. N., and Porter, D. C. (2009). Basic econometrics. Boston, Burr Ridge, IL, Dubuque, IA, New York, San Francisco, St. Louis, Bangkok, Bogotá, Caracas, Kuala Lumpur: McGraw-Hill Irwin.
Halloran, A., Ayieko, M., Oloo, J., Konyole, S. O., Alemu, M. H., and Roos, N. (2021). What determines farmers’ awareness and interest in adopting cricket farming? A pilot study from Kenya. Int. J. Trop. Insect. Sci. 41, 2149–2164. doi: 10.1007/s42690-020-00333-2
Hu, Y., Li, B., Zhang, Z., and Wang, J. (2022). Farm size and agricultural technology progress: evidence from China. J. Rural. Stud. 93, 417–429. doi: 10.1016/j.jrurstud.2019.01.009
Imaita, I. G. (2013). Training as a factor influencing adoption of innovations along mango value chains in Meru County, Kenya. IJMS 5:74. doi: 10.5539/ijms.v5n2p74
Indexbox (2018). World: Pigeopn peas-market report: Analysis and forecast to 2025. Palo Alto, CA, USA.
Indili, B., Zaeem, U. H., Ayella, S., Thawar, S. G., and Seleman, M. (2018). Factors influencing implementation of integrated management of childhood illness in Lindi region, Southern Tanzania. Tanzan. J. Health Res. 20:7. doi: 10.4314/thrb.v20i1.7
Jha, S. (2021). Adoption and scaling-up of climate resilient sustainable agricultural (CRSA) practices for climate change adaptation and food security: Assessing the scaling up potential of selected agricultural innovations in Tanzania. Dissertation Berlin: Humboldt Universitaet zu Berlin.
Karri, V. R., and Nalluri, N. (2017). Pigeon pea (Cajanus cajan L.) by-products as potent natural resource to produce protein rich edible food products. Int. J. Curr. Agric. Sci. 7:229.
Kinyangi, A. A. (2012). Factors influencing the adoption of agricultural technology among smallolder farmers in Kakamega north Sub-County, Kenya. Master Thesis Nairobi, Kenya: University of Nairobi, Schol of Mathematics.
Kragt, M. E., Dumbrell, N. P., and Blackmore, L. (2017). Motivations and barriers for Western Australian broad-acre farmers to adopt carbon farming. Environ. Sci. Pol. 73, 115–123. doi: 10.1016/j.envsci.2017.04.009
Kulshreshtha, S. N., and Brown, W. J. (1993). Role of farmers' attitudes in adoption of irrigation in Saskatchewan. Irrig. Drain. Syst. 7, 85–98. doi: 10.1007/BF00880869
Kumar, S. K. (1994). Adoption of hybrid maize in Zambia: Effects on gender roles, food consumption, and nutrition. Washington, D.C. International Food Policy Research Institute.
Laduber, W., Dessalegn, M., and Daniel, T. (2016). Logit analysis of factors affecting adoption of improved bread wheat (Triticum aestivum L.) variety: the case of Yilmana Densa District, west Gojam, Ethiopia. J. Agric. Ext. Rural Dev. 8, 258–268. doi: 10.5897/JAERD2016.0768
Lalani, B., Dorward, P., Holloway, G., and Wauters, E. (2016). Smallholder farmers' motivations for using conservation agriculture and the roles of yield, labour and soil fertility in decision making. Agric. Syst. 146, 80–90. doi: 10.1016/j.agsy.2016.04.002
Leite, A. E., Castro, R.De, Jabbour, C. J. C., Batalha, M. O., and Govindan, K. (2014). Agricultural production and sustainable development in a Brazilian region (southwest, São Paulo state): motivations and barriers to adopting sustainable and ecologically friendly practices. Int. J. Sust. Dev. World Ecol. 21, 422–429. doi: 10.1080/13504509.2014.956677
Maguire, C. (2012). “Agricultural education and training to support agricultural innovation systems” in The World Bank eBooks, 107–177.
Majili, Z. S., Nyaruhucha, C., Kulwa, K., Mutabazi, K., Rybak, C., and Sieber, S. (2020). Preferences and consumption of pigeon peas among rural households as determinants for developing diversified products for sustainable health. Sustainability 12:6130. doi: 10.3390/su12156130
Majili, Z., Nyaruhucha, C. N., Kulwa, K., Mutabazi, K., Rybak, C., and Sieber, S. (2022). Identification and prioritization of pigeon pea-based products tailored to consumer preference perspective: a mixed method assessment approach. Legum. Sci. 4:3. doi: 10.1002/leg3.137
Meijer, S. S., Catacutan, D., Ajayi, O. C., Sileshi, G. W., and Nieuwenhuis, M. (2015). The role of knowledge, attitudes and perceptions in the uptake of agricultural and agroforestry innovations among smallholder farmers in sub-Saharan Africa. Int. J. Agric. Sustain. 13, 40–54. doi: 10.1080/14735903.2014.912493
Mellon-Bedi, S., Descheemaeker, K., Hundie-Kotu, B., Frimpong, S., and Groot, J. (2020). Motivational factors influencing farming practices in northern Ghana. NJAS Wagening. J. Life Sci. 92, 1–13. doi: 10.1016/j.njas.2020.100326
Mergeai, G., Kimani, P., Mwang’ombe, A., Olubayo, F., Smith, C., Audi, P., et al. (2001). Survey of pigeon pea production systems, utilization and marketing in semi-arid lands of Kenya. Biotechnol. Agron. Soc. Environ. 5:145.
Mfikwa, A. E., and Kilima, F. T. (2014). Factors influencing consumption of pulses in rural and urban areas of Tanzania. Tanzan. J. Agric. Sci. 13:59.
Mlenga, D., and Maseko, S. (2015). Factors Influencing Adoption of Conservation Agriculture: A Case for Increasing Resilience to Climate Change and Variability in Swaziland. J. Earth Environ. Sci. 5, 11–15.
Mottaleb, K. A. (2018). Perception and adoption of a new agricultural technology: evidence from a developing country. Technol. Soc. 55, 126–135. doi: 10.1016/j.techsoc.2018.07.007
Mponda, O., Kidunda, B., Bennett, B., Orr, A., and Mausch, K. (2014). A value chain analysis for pigeon pea in the southern regions of Tanzania, Socioeconomics discussion paper series Number 17.
Ntshangase, N., Muroyiwa, B., and Sibanda, M. (2018). Farmers’ perceptions and factors influencing the adoption of no-till conservation agriculture by small-scale farmers in Zashuke, Kwa Zulu-Natal Province. Sustainability 10:555. doi: 10.3390/su10020555
Okoedo-Okojie, D. U., and Onemolease, E. A. (2009). Factors affecting the adoption of yam storage Technologies in the Northern Ecological Zone of Edo state, Nigeria. J. Hum. Ecol. 27, 155–160. doi: 10.1080/09709274.2009.11906205
Parwada, C., Gadzirayi, C., Muriritirwa, W., and Mwenye, D. (2010). Adoption of agro-forestry technologies among small-holder farmers: a case of Zimbabwe. J. Dev. Agric. Econ. 2, 351–358.
Pignatti, E., Carli, G., and Canavari, M. (2015). What really matters? A qualitative analysis on the adoption of innovations in agriculture. JAI 6, 73–84. doi: 10.17700/jai.2015.6.4.212
Raghu, P. T., Manaloor, V., and Nambi, V. A. (2014). Factors influencing adoption of farm management practices in three agrobiodiversity hotspots in India: an analysis using the count data model. J. Nat. Res. Dev. 4, 46–53. doi: 10.5027/jnrd.v4i0.07
Ruzzante, S., Labarta, R., and Bilton, A. (2021). Adoption of agricultural technology in the developing world: a meta-analysis of the empirical literature. World Dev. 146:105599. doi: 10.1016/j.worlddev.2021.105599
Sarkar, S., Panda, S., Yadav, K. K., and Kandasamy, P. (2018). Pigeon pea (Cajanus cajan) an important food legume in Indian scenario. A review. Legum. Res. 43, 601–610. doi: 10.18805/LR-4021
Saxena, K. B., Kumar, R. V., and Rao, P. V. (2002). Pigeonpea nutrition and its improvement. J. Crop. Prod. 5, 227–260. doi: 10.1300/J144v05n01_10
Sharma, S., Agarwal, N., and Verma, P. (2011). Pigeon pea (Cajanus cajan L.): a hidden treasure of regime nutrition. J. Func. Env. Bot. 1:91. doi: 10.5958/j.2231-1742.1.2.010
Sheahan, M., and Barrett, C. B. (2017). Review: food loss and waste in sub-Saharan Africa. Food Policy 70, 1–12. doi: 10.1016/j.foodpol.2017.03.012
Sheikh, A. D., Rehman, T., and Yates, C. M. (2003). Logit models for identifying the factors that influence the uptake of new ‘no-tillage’ technologies by farmers in the rice–wheat and the cotton–wheat farming systems of Pakistan's Punjab. Agric. Syst. 75, 79–95. doi: 10.1016/S0308-521X(02)00014-8
Shiferaw, B., Silim, S., Muricho, G., Audi, P., Mligo, J., Lyimo, S., et al. (2007). Assessment of the adoption and impact of improved pigeonpea varieties in Tanzania. J. SAT Agric. Res. 3:1.
Singh, D. P., and Yadav, S. K. (2014). Knowledge and adoption gap of tribal farmers of Bastar towards rice production technology. Am. Int. J. Res. Humanities Arts Soc. Sci. 5, 54–56.
Stoltzfus, J. C. (2011). Logistic regression: a brief primer. Acad. Emerg. Med. 18, 1099–1104. doi: 10.1111/j.1553-2712.2011.01185.x
URT (2016). Lindi region: Basic demographic and socio-economic profile, vol. 2012. Dar es Salaam and Zanzibar, Tanzania: Population and housing census.
URT (2021). National Sample Census of agriculture 2019/20- National Report for crop and livestock sectors and fish farming. Dar es Salaam, Tanzania: The United Republic of Tanzania (URT).
Voh, J. P. (1982). A study of factors associated with the adoption of recommended farm practices in a Nigerian village. Agric. Adm. 9, 17–27. doi: 10.1016/0309-586X(82)90093-0
Weir, S., and Knight, J. (2004). Externality effects of education: dynamics of the adoption and diffusion of an innovation in rural Ethiopia. Econ. Dev. Cult. Chang. 53, 93–113. doi: 10.1086/423254
Yaron, D., Voet, H., and Dinar, A. (1992). Innovations on family farms: the Nazareth region in Israel. Am. J. Agric. Econ. 74, 361–370. doi: 10.2307/1242490
Keywords: adoption, smallholder farmers, Tanzania, processing technology, pigeon peas
Citation: Sarr M, Majili Z, Khalili N, Matavel CE, Mbwana HA, Kaingo J, Löhr K and Rybak C (2024) Adoption of processing technologies and innovative food preservation techniques: findings from smallholders in the Lindi Region in Tanzania. Front. Sustain. Food Syst. 7:1169578. doi: 10.3389/fsufs.2023.1169578
Edited by:
Walter Odongo, Gulu University, UgandaReviewed by:
Bettina König, University of Kassel, GermanyFunso Raphael Kutu, University of Mpumalanga, South Africa
Copyright © 2024 Sarr, Majili, Khalili, Matavel, Mbwana, Kaingo, Löhr and Rybak. This is an open-access article distributed under the terms of the Creative Commons Attribution License (CC BY). The use, distribution or reproduction in other forums is permitted, provided the original author(s) and the copyright owner(s) are credited and that the original publication in this journal is cited, in accordance with accepted academic practice. No use, distribution or reproduction is permitted which does not comply with these terms.
*Correspondence: Niloofar Khalili, Tmlsb29mYXIuS2hhbGlsaUB6YWxmLmRl