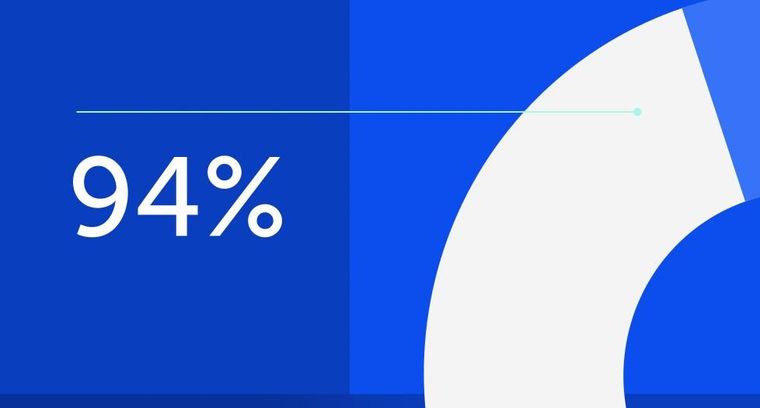
94% of researchers rate our articles as excellent or good
Learn more about the work of our research integrity team to safeguard the quality of each article we publish.
Find out more
REVIEW article
Front. Sustain. Food Syst., 05 June 2023
Sec. Crop Biology and Sustainability
Volume 7 - 2023 | https://doi.org/10.3389/fsufs.2023.1151367
This article is part of the Research TopicSoil Microbiome Metabolomics: A Way Forward to Sustainable IntensificationView all 8 articles
Plant-associated microorganisms play a key role in the future sustainability of terrestrial ecosystems. In nature, plants and their associated microbiome can form an assemblage of species, considered interacting metaorganisms or holobionts. Horticultural plants, including vegetables, fruit and berries, and ornamentals live in intimate association with complex and diverse microbial communities that have evolved a myriad of cooperative and competitive interaction mechanisms that shape the holobiont fitness. Nonetheless, our understanding of these interactions in shaping more complex microbial horticulture communities, along with their relevance for host growth, fitness, stress resilience, and health in a more natural context remains sparse. In this perspective, the holo-omic landscape that requires careful consideration of study design and integrates multi-omic data from both host and microbiota domains is a promising approach to unveil the interplay between the two to advance horticultural production. The analysis of horticulture (meta)-omics and phenotyping, along with mechanistic experiments in model systems, is revolutionizing research by enhancing our ability to understand the structure and core function of the plant-associated microbiome. These advancements are complemented by improvements in the throughput and accuracy of DNA sequencing, enabling us to delve deeper into the genomes of microbial communities. Yet, many challenges remain. We focus this review on the potential for holo-omics to generate a more holistic perspective of molecular networks, and we further discuss the implementation and current limitations of the holo-omic approach within microbial horticulture. We argue that holo-omics will pave the way to improve, from a horticultural perspective, food security and safety, sustainability, breeding practices, development of microbiota- and host-tailored horticultural crops’ health treatments and increasing production efficiency.
Horticultural crops are an essential part of agriculture for both food and nutritional security. In recent years, impressive progress has been made in improving the growth, productivity, and quality of horticultural crops. Given the distinct features of plant growth and development, research in the horticultural associated-microbes have increasingly been addressed. Many studies revealed an important diversity of the microbiome associated with horticultural crops affecting the quantity and quality of their products and their tolerance to biotic and abiotic stresses through close interactions with plant roots (Bulgari et al., 2019; Emmanuel and Babalola, 2020; Ma et al., 2020; Zulfiqar, 2021). Research has fundamentally altered our perception of plant-microorganism interactions to more understanding of their complex network biological processes. In their environment, plants coexist with a variety of microbes including bacteria, fungi, archaea, and protists constituting the plant microbiota (Lindow and Brandl, 2003; Buée et al., 2009; Berendsen et al., 2012; Vorholt, 2012; Turner et al., 2013). Several studies conducted on microbes originating from the phyllosphere, rhizosphere, and in endophytic plant compartments have reported a series of promoting effects on plant health and performance, including enhancement of nutrient acquisition (Anli et al., 2020; Lahbouki et al., 2022), boosting plant immune defense (Rolfe et al., 2019; Zheng et al., 2020), protection against pathogens (Ait Rahou et al., 2020; Liu H. et al., 2020), resistance to environmental stresses (Ait-El-Mokhtar et al., 2020; Ben-Laouane et al., 2020; Boutasknit et al., 2021), and promoting hormone production (Wang et al., 2017). Plants and their associated microorganisms interact in a bidirectional fashion. The microbes provide the necessary metabolites to boost plant health and fitness and the host plant supplies its microbial associates with metabolic capacities, resulting in the establishment of niche-specialized inhabitants providing positive (mutualistic), detrimental (pathogenic), or neutral (commensalistic) actions on plant performances (Thrall et al., 2007).
These interactions are based on complicated molecular connections including a mutual perception between the two partners and the management of resources and nutrients critical for their survival (Cotton et al., 2019; Finkel et al., 2019b; Huang et al., 2019; Campos-Soriano et al., 2020; Stassen et al., 2021). Furthermore, current discoveries suggest that the outcome for host performances may rely not only on host/microbe exchange of supplies but also on signaling and metabolic exchanges between microbiome members (Durán et al., 2018; Finkel et al., 2019a; Harbort et al., 2020). These investigations show that comprehending the plant microbiome will most likely need an analysis of these connections at different layers, such as activity and functional capacity and molecular interaction involving host and microorganisms. Although many advances were accomplished in the functional understanding of plant microbiome interactions especially through combining metabolomic and metatranscriptomic studies, this aspect is still lacking, in part because of the system’s complexity. One aspect of this complexity is that the phytomicrobiome is built from and lives in one of the most varied habitats on the planet: the soil (Compant et al., 2019; Fitzpatrick et al., 2020). Soils support a diverse microbial ecology interacting in complicated framed networks and shifting in abundance, composition, and activity which enhance the diversity of the whole system (Griffiths et al., 2011; Chemidlin et al., 2014; Terrat et al., 2017; Wei et al., 2019). Another level of complication is related to the quantity and composition of the metabolites exuded by plant roots that are in continuous changes (Jacoby et al., 2020), resulting in the differential recruitment of rhizospheric microbes. These aspects combine to form a dynamic and linked biological scheme that has tested our capacity to grasp the fundamental molecular mechanisms that build and sustain it. Still, our choice of assets may be a bigger reason for our delayed progress toward a functional understanding of plant microbiome interactions. Until recently, the majority of plant microbiome investigations have focused on descriptive studies of community structure with help of amplicon-based sequencing, including Internal Transcribed Spacer (ITS) sequencing for fungi and 16S rRNA sequencing for bacteria (Griffiths et al., 2011; Terrat et al., 2017).
While these data have provided a significant understanding of the broad dynamics that orchestrate the general structure of the plant microbiome and the relative intensity of their influence (Lundberg et al., 2012; Coleman-Derr et al., 2015), they frequently fail to provide mechanistic perception to interactions with the host plant. More recently, a growing number of research has started to investigate additional microbiome characteristics, such as functional capacity and activity, using shotgun metagenomics and metatranscriptomics (Galloway-Peña and Hanson, 2020; Jo et al., 2020; Gao et al., 2021; Singh et al., 2022). Even with this rise, there is still a scarcity of research relating plant microbiome information to plant physiology, metabolism, genetics, and other host aspects (Brunel et al., 2020), which might supply additional data from this unexplored aspect of plant-microbiome interactions. Emerging trends and research hotspots in this field—as co-cited references in Figure 1A—revealed a total of 16 clusters, where each one corresponds to a line of research. Except “#7 shoot tips” cluster, all the other clusters were closely related to the topic of the microbiome of horticultural crops. Among these clusters, “#0 disease resistance,” contains most of the nodes, has been widely reported followed by “#1 plant growth promoting rhizobacteria” and “#2 mycorrhizae” as the most active research areas. Studies from 2010 and 2017 were focused on cluster “#0 disease resistance,” but in the last 5 years, the hotspots and frontier trends highlight plant associated-beneficial microorganisms (“#1 plant growth-promoting rhizobacteria” and “#2 mycorrhizae”), apple replant disease, and internal transcribed spacer (ITS) (Figure 1B). To gain a more comprehensive understanding of plant microbiome function, we advocate for experimental datasets that combine host-centered omic approaches like transcriptomics, epigenomics, metabolomics, and proteomics with microbial-focused approaches like shotgun metagenomic, amplicon sequencing, metatranscriptomics, and exometabolomics. The term “holo-omics” characterize research that includes data from many-omic layers from both the host and microbial domains (Nyholm et al., 2020). We believe that holo-omic investigations have the potential to clarify the functionality of plant microbiome interactions by producing a picture of what is expressed, translated, and generated through plant-microbiome interactions (Griffiths et al., 2011). This multimodal view can assist narrow down results from various datasets to significant biological signals, as well as generate support for certain hypotheses using data gained via orthogonal methodologies. After the introduction of the holo-omic approach (Nyholm et al., 2020) and its adoption in plant microbiome interactions (Xu L. et al., 2021; Xu Q. et al., 2021), this review focuses on the potential of holo-omics to generate a more holistic understanding of molecular networks and discusses the implementation and current limitations of the holo-omic approach within the horticultural microbiome. More understanding of microbiome-horticultural crop interactions will harness the development of microbial tools for climate-proof horticulture production. We first highlight the horticultural-associated microbiome and discuss the different beneficial/pathogenic interactions between the host plant and the microbes, with a focus on experimental design considerations for plant holo-omic. Finally, we discuss the current challenges in appraising holo-omics datasets.
Figure 1. Co-cited references (A) and timeline visualization (B) of keyword co-occurrence clustering analysis in the study of microbiome associated with horticultural crops from 2018 to 2023. ITS, internal transcribed spacer; TYLCV, tomato yellow leaf curl virus; ROS, reactive oxygen species.
Multiple studies reported the diversity of bacterial and archaeal microbiota of horticultural plants and their functional traits influencing the host plant and the associated microbial community. Previous studies confirmed that there is no hazard in the establishment of the bacterial community on plants since this process is managed by an assemblage of specific rules (Dong et al., 2019; Yang H. et al., 2023). Many structuring factors of plant-associated bacterial communities such as soil type (Liu et al., 2020; Xu et al., 2020), plant compartment (López-Angulo et al., 2020; Bettenfeld et al., 2022), host genotype/species (Cheng Z. et al., 2020; Sousa et al., 2020; Shakir et al., 2021), plant immune system (Kohl, 2020; Knights et al., 2021), plant trait variation/developmental stage (Liu et al., 2020; Compant et al., 2021), and residence time/season (Chernov and Zhelezova, 2020; Wang et al., 2021; Yi et al., 2021). Various bacteria such as Alphaproteobacteria, Actinobacteria, Gammaproteobacteria, Firmicutes, Pseudomonas, and Paraburkholderia spp. were discovered in the rhizosphere of different horticultural crops (carrot, potato, neep, beetroot, onion, and topinambur) (Kõiv et al., 2019). Cheng F. et al. (2020) and Cheng Z. et al. (2020) reported that the rhizomicrobiome of 11 tomato cultivars is composed of a diversified community dominated by bacteria from the phyla Proteobacteria, Bacteroidetes, and Acidobacteria, mainly comprising Rhizobiales, Xanthomonadales, Burkholderiales, Nitrosomonadales, Myxococcales, Sphingobacteriales, Cytophagales, and Acidobacteria subgroups. Meanwhile, the pathogenic bacterial community has been found in a variety of horticultural crops; Pseudomonas syringae in olive, tomato, and green bean, Xylella fastidiosa and Ralstonia solanacearum in potato and banana, Escherichia coli strains in leafy greens and sprouts, Listeria monocytogenes in fresh-cut salad and melons, Salmonella spp. in tomato, spices seeds, and sprouts, Norovirus in berries, and Shigella in green onion (Mansfield et al., 2012; Leff and Fierer, 2013; Li et al., 2015; Deng et al., 2019; Shi et al., 2019). Although the important diversity of bacterial microbiota reported here and the myriad factors controlling the establishment of this microbiota, the bacterial community in both above- and belowground compartments of horticultural plants are dominated by Proteobacteria, Actinobacteria, and Bacteroidetes phyla, as is the case for most plants (Rai et al., 2021). Comparing root- and leaf-associated communities using an operational taxonomic unit (OTU) showed significant overlap in many crops, such as grapevine and tomato (Zarraonaindia et al., 2015; Dong et al., 2019). However, Bai et al. (2015) demonstrated through large-scale genome sequencing and re-colonization of germ-free plants that host-associated microorganisms are specific and adapted to their respective cognate plant organs. Despite representing ca. 36% of plant-associated microbiota (Müller et al., 2015), archaeal microbiota have not received the same level of attention as their bacterial counterparts, possibly because no archaeal pathogens have been reported. Therefore, more research should be conducted on archaeal members, particularly to clarify their interactions and significance for their host plants.
Community profiling studies showed a huge diversity of plant-associated fungi with Ascomycota and Basidiomycota as the main phyla (Sweeney et al., 2021; Zhou et al., 2021). A great number of phytobiome research identified many fungal microorganisms from the horticultural microbiome, including Trichoderma and mycorrhizal fungi, Penicillium sp., Cladosporium sp., Colletotrichum sp., Cryptococcus, Rhodotorula, Hanseniaspora, and Sphingomonas with beneficial effects on plant growth performances (Shi et al., 2019; Jo et al., 2020; Knapp et al., 2021; Liu and Howell, 2021; Darriaut et al., 2022; Fournier P. et al., 2022; Sangiorgio et al., 2022). Recent studies have identified several fruit trees-associated fungi (e.g., Fusarium, Cladosporium, Taphrina, Phoma, Septoria) as phytopathogenic fungi (Padraic et al., 2019; Nicoletti et al., 2020; Gusella et al., 2021; de Jager and Roets, 2023; Yang Y. et al., 2023). In the rhizosphere of sweet potato, Gao et al. (2019) revealed the presence of plant growth-promoting fungi, including Chaetomium and pathogenic fungi such as Fusarium, Verticillium, Guehomyces, and Colletotrichum. A phytobiome study on tomato identified several beneficial fungi, including Aspergillus, Hyphodiscus, Chrysosporium, Trichoderma, and Oidiodendron (Lee et al., 2019). Similar to bacteria, there are several structuring factors that govern the fungal phytobiome, including soil type, plant compartment, plant species, and seasons (Onufrak et al., 2020; Knapp et al., 2021; Liu and Howell, 2021; Runge et al., 2023). Fungal populations have high endemism and limited biogeographic dispersal due to their sensitivity to stochastic variations and distinct responses to environmental factors when compared to bacterial communities (Powell et al., 2015; Thomson et al., 2015; Vacher et al., 2016; Zhang et al., 2021; Wei et al., 2022). Studies examining plant-associated fungi and bacteria have shown that fungal microbiota is more influenced by biogeography than bacterial microbiota (Onufrak et al., 2020; Liu and Howell, 2021; Zhang et al., 2021; Wei et al., 2022; Runge et al., 2023). However, it remains unclear whether the taxonomic resolutions brought forth by the 16S rRNA and ITS marker loci impact this trend (Peay et al., 2016).
Little importance was given to protists as a component of the rhizosphere microbiome, although they are ubiquitous and play a key role in soil and plant functioning. The most known protists to interact with plants are belonging to the lineages of Oomycota (Stramenopiles) and Cercozoa (Rhizaria) which belong to the supergroup of Stramenopiles-Alveolata-Rhizaria (SAR) (Ruggiero et al., 2015). Phytophthora, Pythium, Albugo, and Peronospora genera, within Oomycota, contain some species closely associated with horticultural roots or leaves (Arcate et al., 2006; Coince et al., 2013; Sapkota and Nicolaisen, 2015; Agler et al., 2016). The main oomycete species are well known to induce deleterious effects on plants and thereby a significant decline in global crops production (Kamoun et al., 2015). In contrast, some oomycetes species that colonize roots can boost plant fitness and health performances (Benhamou et al., 1997, 2012; Van Buyten and Höfte, 2013), confirming the existence of beneficial protists with non-harmful interactions with plants. Protists profiling community studies have revealed unexpected diversity of soil protists (Dupont et al., 2016; Mahé et al., 2017). Oomycetal communities associated with horticultural crops showed very low diversity (Coince et al., 2013; Sapp et al., 2015), where they are mostly represented in plant tissue by species belonging to the Pythiaceae family (Sapp et al., 2015), while Cercozoan communities presented high diversity in plant leaves and roots (Sapp et al., 2015; Ploch et al., 2016). Protistan communities are controlled by many environmental factors (Geisen et al., 2015; Dupont et al., 2016) and they have been recently considered as determinant bioindicators of soil quality (Fournier B. et al., 2022). These studies highlight the importance of protists—especially Oomycota and Cercozoa—for holobiont functioning and sustainability.
The horticultural microbes provide many services through their effective bidirectional interactions with plants. These services (see Figure 2) are reviewed in the next section.
Figure 2. Microbiome services provided by beneficial/pathogenic horticulture–microbe interactions. SAR, Systemic acquired resistance; ISR, induced systemic resistance; MAMPs, microbe-associated molecular patterns; PGPM, plant growth-promoting microbes.
Previous studies have provided evidence of the promising role of plant interactions with beneficial soil microbes in agriculture sustainability (see reviews by Arif et al., 2020; Liu H. et al., 2020; Liu L. et al., 2020; Ali et al., 2022; Bai et al., 2022; Trivedi et al., 2022). This section does not intend to give a detailed account of horticulture–beneficial microbe interaction and the reader is directed to other publications to read about the beneficial interaction for plant growth promotion (Patel et al., 2018; Kumawat et al., 2019; Ullah et al., 2020), disease suppression (De Vrieze et al., 2018; Sharma et al., 2018; Ait Rahou et al., 2020), bioremediation (Ma et al., 2015; Bücker-Neto et al., 2017; Belimov et al., 2020), and abiotic stress tolerance, including heat stress (Mukhtar et al., 2020; Sangiorgio et al., 2020), salinity stress (Ait-El-Mokhtar et al., 2019; Toubali et al., 2020), drought stress (Silambarasan et al., 2019; Anli et al., 2020; Huang et al., 2020).
Signaling from horticultural plants to microbes through plant-secreted compounds has been demonstrated to promote various beneficial aspects for plants (Goddard et al., 2021; Lazazzara et al., 2021; Montesinos et al., 2021). This signaling process has been mainly investigated in close symbiotic associations, especially those involving mycorrhizal fungi and rhizobial bacteria (Emmanuel and Babalola, 2020; Sharma et al., 2020; Hanaka et al., 2021; Dabré et al., 2022). For instance, under nutrient-deficient conditions, the host plant enhances strigolactone secretion to stimulate the establishment of AMF and root colonization as a mechanism for nutrient acquisition (Li et al., 2023). Another group of signaling molecules involved in the communication between horticultural plants and microorganisms is flavonoids. These compounds have been shown to boost root infection by rhizobacteria that induce the formation of nodules allowing N fixation in legumes (Wang et al., 2022; Zhang et al., 2022). Plants emit flavonoids that serve as chemo-attractants and nodulation gene (Nod) inducers for symbiotic Rhizobia when N levels are low. Apigenin and luteolin, two of the Nod-inducing flavonoids, were both potent chemoattractants, whereas naringenin elicited a very weak response in Rhizobium leguminosarum biovar phaseoli (Dent and Cocking, 2017; Abedini et al., 2021), showing that various flavonoids play distinct roles. Besides these characterized signaling molecules, the plant-derived aromatic metabolite benzoxazinoids (BXs) can act as chemo-attractants for several beneficial microbes in addition to their role as toxins toward pathogens (Bhattarai et al., 2021). It has been shown that the presence of coumarins in root exudates alters the microbial makeup of the roots by promoting/restraining the growth of specific microorganisms. According to Stringlis et al. (2018), scopoletin, an antimicrobial coumarin, preferentially inhibits the growth of the pathogenic Fusarium oxysporum and Verticillium dahlia (both known to be associated with horticultural crops), while having no effect on the beneficial rhizobacteria Pseudomonas capeferrum and Pseudomonas simiae. Moreover, a wide range of amino acids is recognized by microbial chemoreceptors, which drive the bacteria to nutrient-rich niches around the plant roots (Dent and Cocking, 2017). For the development of symbiotic relationships, the capacity to utilize amino acids provided by the host plant for nourishment may be essential. For instance, Hida et al. (2020) demonstrated that Pseudomonas protegens CHA0, a rhizobacterium that promotes plant growth of various species including tomato, possesses four putative chemoreceptors for amino acids that may enhance chemotaxis toward amino acids. In the same vein, glycerol-3-phosphate (G3P) roots secretion is essential for strain-specific exclusion of non-desirable root-nodulating bacteria and the associated foliar pathogen immunity in soybean (Shine et al., 2019). Under stressful conditions, horticultural plants produce chemicals that specifically attract microbes that can increase plant resilience (Cheng F. et al., 2020; Xu Q. et al., 2021). Furthermore, plants interact with the microbiome through volatile compounds (VCs), such as aldehydes, terpenoids, phenylpropanoids, and common monoterpenes (i.e., limonene, −pinene, benzenoids, −caryophyllene) (Wolfgang et al., 2019; López et al., 2021; Jiuyun et al., 2022). Along with the low-molecular-weight organic acids, many other important bioactive agents (i.e., phenolics, flavonoids, cutin, monomers) and many other unidentified molecules are involved in plant-microbial chemical interactions (Gulati et al., 2020; López et al., 2021; Jiuyun et al., 2022).
Horticultural plants can detect signaling molecules produced by microorganisms that have an impact on the host gene expression, immune system, development, and stress responses (Shine et al., 2019; Fu et al., 2021; Oyserman et al., 2022). Microbe-associated molecular patterns (MAMPs), such as lipopolysaccharide, peptidoglycans, flagellin, and chitin are used by rhizosphere microbes to elicit plant responses (Fu et al., 2021; Oyserman et al., 2022). MAMPs cause induced systemic resistance (ISR) and they prime the rhizosphere-beneficial microorganisms. Systemic acquired resistance (SAR), primarily induced by pathogens, can also be triggered by MAMPs (Kim et al., 2020; Dye and Bostock, 2021). To initiate symbiosis with plants, several well-known chemicals are employed by microorganisms, including legumes rhizobium and mycorrhizal factors (Shine et al., 2019; Ge et al., 2022). In addition to plant signaling chemicals, metabolic interactions between microbes via quorum sensing (QS) are crucial in defining microbial communities. According to extensive research, the QS molecule N-acyl homoserine lactone (AHL), produced by Gram-negative bacteria, not only acts as a signaling molecule within and between bacterial species but also between bacteria and plants, influencing thereby gene expression and functions related to plant development, stress response, and immunity (Schuhegger et al., 2006; Ferluga et al., 2008; Babenko et al., 2022). QS compounds, such as cis-2-unsaturated fatty acids, pyrones, alkyl quinolones, and dialkyl resorcinols have recently been discovered. Beyond QS mechanisms, numerous microbial compounds such as VCs have been reported to act as signaling molecules, inducing directed movement of fungal-bacterial and plant-microbe associations. VCs from bacterial metabolic activity could be used by plants in stressful environments (Sangiorgio et al., 2020; Jiuyun et al., 2022; Nakayasu et al., 2023). Pseudomonas and Bacillus species have been found to produce VCs that either directly inhibit plant pathogens by changing the transcriptional expression levels of numerous genes involved in motility and pathogenicity or indirectly by increasing ISR in plants (Raza et al., 2016a,b). However, little is known about the processes through which microbial VCs promote growth and nutrient absorption. Besides, microorganisms regulate the nutritional and hormonal balance of plants and promote systemic tolerance to (a)biotic stimuli and plant growth. Several root-associated microbes produce auxins, cytokinins, gibberellins, salicylic acid, abscisic acid, and cytokinins (French et al., 2019). In a changing climate, the plant microbiome is also involved in host phenotypic plasticity, which can have an impact on plant phenology (Dastogeer et al., 2020). For instance, by altering the N cycle and converting the amino acid tryptophan in root exudates to the indoleacetic acid phytohormone, rhizosphere bacteria can control the flowering time (Lu et al., 2018).
Plants are equipped with multilayer defense strategies to cope with microbe attacks. A myriad of studies has highlighted the induced response during the horticultural plant-pathogen interaction at different levels. Advanced research in genomic profiling allows the sequencing of the whole genomes of horticultural pathogens. Table 1 shows a list of sequenced genomes of horticultural plant pathogens.
Transcriptomic studies have implicated the upregulation of several phenylpropanoid and terpenoid biosynthesis-related genes and the downregulation of photosynthesis-related genes at 48 h post-inoculation of lettuce with B. cinerea (De Cremer et al., 2013). Proteomics studies added a new layer to the understanding of plant-pathogen interaction. Margaria et al. (2013) investigated the proteome profile of grapevine infected by Flavescence doree (phytoplasma pathogen disease). In infected grapevine, 48 different proteins were de-regulated, including isocitrate dehydrogenase and glutathione S-transferase known by their antioxidant role. Another study (Parker et al., 2013) identified the tomato proteome during the infection by Pseudomonas syringae pv. tomato DC3000 (Pst). A total of 2,369 proteins were de-regulated in tomato leaves of which 477 Pst redox proteins such as glutathione S-transferase, thioredoxin, and superoxide dismutase as the most up-regulated proteins. Li et al. (2013) identified 38 proteins (i.e., antifungal proteins, PR proteins, and cell wall strengthening-related proteins) differentially expressed in Fusarium oxysporum f. sp. cubense infected-banana. Using LC–MS analysis, the metabolite profile changes were observed during the infection of grape with B. cinerea (Hong et al., 2012). The results provided evidence of carbohydrate and lipid metabolisms reprogramming toward the increased synthesis of secondary metabolites involved in plant defense, such as trans-resveratrol and gallic acid. The study provided metabolic biomarkers of infection (i.e., azelaic acid, a substance known to prime plant defense responses, arabitol, ribitol, 4-amino butanoic acid, 1-O-methyl- glucopyranoside, and several fatty acids) that alone or in combination can be used to monitor Botrytis early infection in the vineyard. In potato, The infection with Phytophthora infestans showed a resistance characterized by the presence of flavonoids, alkaloids, and phenylpropanoids (Yogendra et al., 2015). Soybean plants infected with Rhizoctonia solani yielded an altered metabolites profile with major antioxidant molecules (i.e., phytoalexins, flavonoids, coumarins, and hormones) likely to limit the invasion of the pathogen (Aliferis et al., 2014).
Characterizing the entire range of bioactive substances generated by plant pathogens is another crucial task for metabolomic studies in plant-microbes interactions. These compounds could be employed as plant-attacking non-protein effectors (Pusztahelyi et al., 2015). Pathogenic organisms of Aspergillus, Alternaria, Burkholderia, Fusarium, and Pseudomonas create a wide range of chemically varied toxins that include aflatoxin, alternariol, toxoflavin, fumonisin, or coronatine (Lee and Ryu, 2017; Adeniji et al., 2020). In addition, root pathogens must identify chemical cues in the rhizosphere to infect root tissues. For instance, the oomycete pathogen Phytophthora sojae recognizes trace amounts of the compounds daidzein and genistein released by soybean and uses them as chemotrophic cues to guide its growth (Hua et al., 2015). Similarly, cochliophilin A, belongs to the class of flavones, from spinach root exudates attracts Aphanomyces cochlioides causing root rot disease (Chen et al., 2021). Plant root exudates typically play a significant role in both pathogen attraction to the roots and their deterrence or activation of microbiome processes, as part of the action to defend against invading pathogens (Sasse et al., 2018; Jacoby et al., 2020). Most of the current studies on the interactions of microbial members with potential pathogenic behavior (pathobiota) with horticultural crops have employed basic simulation models containing a single host entity and a single pathogen entity. Yet, in their natural habitat, plants are in continuous interaction with a myriad of susceptible pathogens (Bartoli et al., 2018) and the establishment of these harmful microbes relies on whether the pathobiota cooperate with or compete with the plant microbiome. The next step is therefore to understand the way pathobiota and plant microbiomes interact in a long-term process in a realistic ecological context.
One of the aspects of the positive impact of the horticultural-associated microbiome on crop performance is the promotion of fruit quantity, quality, and/or functionality (Agnolucci et al., 2020; Emmanuel and Babalola, 2020). Bona et al. (2016) revealed that the inoculation of tomato with AMF (Glomus aggregatum, G. viscosum, Rhizophagus intraradices, Claroideoglomus claroideum, and C. etunicatum) and PGPB (Pseudomonas sp.) induced early flowering and fruiting and a significant increase in fruit length, diameter, and weight, providing thereby fruit with high commercial value. Mycorrhizae significantly improved sugar concentration in bigarade orange, eggplant, and grape berries (Antolín et al., 2020; Hadian-Deljou et al., 2020; Sabatino et al., 2020). Apple roots inoculated with Rhizophagus irregularis accumulated higher sugar concentration, especially under drought conditions. Similarly, inoculation of strawberry with PGPB strains increases yield, sugars, nutrients, and vitamins content in fruit (Esitken et al., 2010; Todeschini et al., 2018). It has long been noted that horticultural crops–beneficial microorganisms interactions are significantly influenced by the amounts of soluble sugar in the host plants (Agnolucci et al., 2020; Emmanuel and Babalola, 2020). Todeschini et al. (2018) showed an increase in fruit glucose and fructose levels in Septoglomus viscosum-inoculated strawberry, whereas Bona et al. (2015) revealed that the application of commercial inoculum Mybasol improved glucose and sucrose concentrations in strawberry fruits. Tomato plants inoculated with the same commercial inoculum yielded higher levels of glucose (Bona et al., 2016). The synthesis of fruits amino acids is favorably impacted by the beneficial microorganisms. Mycorrhizal cherry tomatoes showed an increase in valine, isoleucine, leucine, and lysine content in the fruits (Carillo et al., 2020). Todeschini et al. (2018) revealed that mycorrhizal inoculation can also differentially affect acid production in a strain-dependent manner. In fact, F. mosseae significantly affected total organic acid and citric acid content, while R. irregularis and S. viscosum altered the concentrations of ascorbic, malic, and fumaric acids. The dual inoculation of AMF and PGPB altered tomato fruit VCs production, indicating that the synergistic effect of both microorganisms is important in determining the sweet-fruity taste and flavor (Todeschini et al., 2018). Compared to the single inoculation, the dual inoculation significantly enhanced the total phenolic compounds and flavonoid levels in spinach (Khalid et al., 2017). In this vein, the production and quantity of anthocyanins in strawberry fruits were enhanced after the application of a mixture of Glomus sp. and Pseudomonas sp. strains (Lingua et al., 2013). Metabolic profiling revealed increased sugars, acids, and multiple secondary metabolites levels following AMF + PGPB inoculation. These combinations, however, did not induce the same effects, and fruit concentration of some elements and/or volatilome profile can be affected by the presence of specific beneficial soil microorganisms. For instance, Todeschini et al. (2018) revealed that fruits from each microbial strain were different and this can result in unique organoleptic and qualitative characteristics. In fact, the combined application of F. mosseae and P. fluorescens Pf4 produced sweeter fruit, while the dual application of R. irregularis and Pseudomonas sp. 5Vm1K induced low-acid fruits. The comparison studies above provide a demonstration of the differences between strains within the same species, indicating that the use of the right strain (or right combination) matters and could help boost plant growth and ultimately, is more likely to increase crop yield and/or quality in sustainable agriculture (Turrini et al., 2018; Plouznikoff et al., 2019).
A growing number of studies adopted “omics” and holo-omic approaches to study horticultural plant–microbes interaction (Table 2). The implementation of the holo-omics approach in horticulture required the use of a variety of methodological assets within different experimental setups with a need for diverse statistical and analytical approaches. Research depends on targeted approaches such as targeted RNA sequencing, amplicon sequencing, and western blot to link the host and the microbiota domains. Yet, untargeted approaches including shotgun DNA sequencing, shotgun proteomics, and shotgun metabolomics are increasingly completing and still used instead of targeted approaches since they provide a non-selective snapshot of the nucleotides, proteins, and metabolites present in the samples. Indeed, host/microbe-generated data from untargeted approaches seem to be more advantageous than those obtained from targeted approaches since the shotgun sequencing approach can generate metagenome-assembled genomes (MAGs) from metagenomic data and the construction of individual genomic profiles (Nyholm et al., 2020). In addition, Vieira-silva et al. (2021) confirm that steadily decreasing sequencing costs associated with improvements in computational methods are likely to promote the use of the shotgun sequencing approach.
The construction of high-dimensional data is the first step in implementing a complete holo-omics approach with horticultural host/microbe multiple-omics layers. According to the objectives of the research, the obtained data from each study sample could include genomic, transcriptomic, proteomic, and/or metabolomic measurements. For instance, millions of genes, thousands of gene orthologs, or hundreds of MAGs may be employed to characterize the microbiota. As a result, the huge amount of information and the multidimensionality of the generated data is challenging classic statistical methods including linear models and correlation-based methods. To reduce the complexity of the generated data, several authors suggest the reduction of dimensionality. For this purpose, Alberdi et al. (2022) proposed gathering MAGs following taxonomy or ecological guilds, and Ayesha et al. (2020) suggested clustering genes according to their functional properties. While this dimensionality reduction abridges analyses and diminishes computational complexity, it may induce pertinent data loss Ayesha et al. (2020).
The first studies in holo-genomics identified correlations among hosts and associated microbes using association analysis methods such as genome-wide association studies (GWASs). This approach associated precise loci in the host genome with the occurrence of beneficial/pathogenic microorganisms (Batstone et al., 2022; Demirjian et al., 2022). Similar methods were applied to study epigenomes (Zhang et al., 2023), proteomes (Zancarini et al., 2021), and metabolomes (Deng et al., 2021). Metagenome-wide association studies (MGWASs) were developed from GWASs to link specific loci in the metagenome to specific characters of the host phenotype (Ayesha et al., 2020). To date, the main applied methods to gather multi-omics data from host and microbiome domains were based on traditional statistical models including general linear and linear mixed methods in GWASs and MGWASs (Ayesha et al., 2020; Nyholm et al., 2020). The used models are frequently hindered by the complex dimensionality of the metagenomic data, which suggests the development of specific models to manage the complexity of holo-omics data (Xu L. et al., 2021).
To make progress in the holo-omics approach and overcome the association analyses issue, Limborg et al. (2018) developed a two steps methodological framework to expose the implemented mechanisms during host-microbiome interactions that modulate host phenotype variation. During the first step, an initial association is performed based on GWAS and MGWAS analysis to identify variants (SNPs) inside the genome and metagenome (e.g., amplicon sequence variants, MAGs, operational taxonomic units, or genes) related to specific host phenotypes. In the second step, the interactions between the associated GWAS variants and other-omic levels are investigated, resulting in the identification of the main molecular features orchestrating the pathway from host genotype to phenotype. Although the two-step method allows us to drill down into the interactions among the diverse-omic levels that impact host phenotype, the efficiency of the GWAS, carried out in the first step, in identifying causal variants still limits the number of interactions that should be cross-examined. The integrated inference by leveraging different-omics big data is challenging, and the development of computational methods in this field has been hindered by the inherent complexity of holo-omics and the biological process underlying them. So far, integrating various-omics datasets is based on different approaches including regularized regression-based methods (Pan, 2021), network-based approaches (Xiao et al., 2022), or other specialty tools (Abbott et al., 2021). None of these approaches, however, were developed to analyze metagenomic, metatranscriptomic, or metametabolomic data.
Many decisive considerations should be taken into account to obtain meaningful and precise data from holo-omics-related microbiome studies (Nyholm et al., 2020; Xu L. et al., 2021). The first consideration is the reflected choice of the type of study. Longitudinal studies (studies with sampling occurring throughout horticultural plant development, but not permanently from the same plant host) present substantial benefits for holo-omic research in comparison to the end-point studies. During microbiome end-point experiments, data are collected at a single defined time point in the experiment to explore the alterations of microbial communities under diverse treatments. Yet, choosing a suitable time point is challenging since there is frequently slight or no a priori information about when host and microbiome interactions will happen. In contrast, longitudinal studies aim to assess the evolution of the microbiome over time. These studies allow for a type of pseudo-replication as patterns recorded at several time points are most probably the consequence of actual biological processes. Further, longitudinal experiments improve the possibility of identifying modifications that occur exclusively during a certain period after treatment. As already stated in this review, samples gathered at a single randomly determined time point could miss the key treatment-dependent variations occurring outside the chosen temporal frame.
In addition, a longitudinal approach may help detect correlations across data types that are influenced by a delay of time (Chen et al., 2022). Signal transduction and decoding among host and microorganisms may be time-consuming (Pan, 2021; Xiao et al., 2022) as can the delay between transcriptional shifts and downstream changes affecting the biosynthesis of proteins and metabolites in the host (Jamil et al., 2020). Temporal delay is anticipated to have an even greater influence on microbiome composition and abundance, as well as the development of macroscopic host phenotypes. Finally, in orthogonal datasets, a longitudinal design may aid in the development of a clear hypothesis for the interconnection between associated characteristics. However, the longitudinal approach for holo-omics studies is constrained by the requirement of multiple rounds of sampling, effective scheduling of the sample collection timing to avoid the introduction of confusing variables (i.e., circadian variations) (Hubbard et al., 2018), abiotic factors, diurnal variations, and downstream statistical analyses. Indeed, several host-related data types, e.g., transcriptomics, are influenced by circadian variations and will need samples to be gathered in a restricted window at a set time of day during the experimental design when collecting huge numbers of samples, particularly in the field (Xu L. et al., 2021). While developing integrated holo-omics research, it is necessary to take into account the sample and sampling procedure restrictions. For instance, the compatibility of each gathered sample type for certain required data types should be considered, which may need changes to the sampling method in some circumstances. Phyllosphere microbiome samples would include more DNA, RNA, and proteins derived from the horticultural plant than from the microorganisms. Therefore, some microbial methods may be impossible to perform without removing horticultural plant-derived contamination. When an environment presents low microbial biomass, such as droughted sandy soils, a higher number of samples must be collected for the extraction of nucleic acids. Furthermore, collection methods or sampling time may differ following the horticultural plant compartments, which may have unintended consequences for sample viability and data results, particularly for -omics approaches owing to their high-sensitivity to time and temperature.
A thorough examination of what data type combinations are most achievable and appropriate for the system under study, the objectives of the study, and available resources for the project are required (Lucaciu et al., 2019). For instance, holo-omics could be more difficult in non-model plants (e.g., horticultural crops) than in other host species due to the presence of various organelles, integration, and interpretation of incomplete genomes, high metabolic diversity, and complicated interaction links with pathogens and symbionts at the same time (Jamil et al., 2020). It is worth mentioning that holo-omics designs for deciphering plant-microbiome interactions are quite expensive to implement owing to the integration of multi-omics approaches, specifically shotgun metagenomics and metatranscriptomics remain highly expensive, whereas others (i.e., amplicon analysis) can cost one to two orders of magnitude less per sample. Before conducting holo-omic research, focused pilot surveys, with less expensive techniques or limited sampling possibility, are highly recommended to determine whether the experimental factors in question have a significant impact on microbial community dynamics, warranting further holo-omic investigation. This will also allow preliminary system evaluations to be performed without requiring broad technological and biological knowledge.
Developing staging within holo-omic investigations in horticultural-microbes interaction, in which procedures require a larger investment, are deployed later and may reduce resource expenditure in longitudinal designs by selecting crucial periods to focus on less expensive early datasets. However, not all data types are equally suitable to this method; certain sample types require instant or quick analyses (RNA, metabolites), while others (DNA) may be retained for considerably longer periods for subsequent use.
The emergence of -omics technologies, such as genomics, transcriptomics, proteomics, metabolomics, lipidomics, hormonomics, and ionomics has permitted to identify components associated with plant-microbe interactions including molecular regulatory circuitries, how influential and important horticultural microbiomes play in plant fitness, plant disease, (a)-biotic stress susceptibility/tolerance, soil health, multi-organismal communication, and nutrient transfer and cycling, thus underpinning factors paving the way to harnessing the power of the microbiome to boost productivity and quality of horticultural crops.
With continual improvements, the integrated study of the genetic features of a horticultural host and its associated microbes is becoming more feasible, though still underdeveloped, approach to understanding horticultural holobionts. The combination of host and microbial genomic and transcriptomic information is critically important to improving our understanding of the plant holobiont (Figure 2). Yet, the microbial genetics contribution in holobionts is still difficult to assess owing to the vast majority of microbes lacking reference genomes. Across the complex landscape of studying horticultural–microbe interactions, transcriptomics only provides information on expressed gene levels and does not provide specifics on post-translational shifts, which are supplied by the proteomic method. Meanwhile, metabolites are the end products of biological activities supplied by the metabolomics platform. As a result, investigations are being conducted using a mix of -omics methodologies, which aids in the construction of bridges connecting all elements of cellular changes. This aids in the precise understanding of the complicated dynamics of cellular systems in both partners during plant-microbe interactions.
This section gives an overview of the application/integration of -omics techniques of what has been successfully achieved in microbial horticulture studies (Table 2). For instance, Bradyrhizobium japonicum bacteroid metabolism in soya bean root nodules was investigated using proteomics and transcriptomics datasets (Delmotte et al., 2014). A considerable number of proteins related to various forms of bacterial metabolism that were not previously thought to be present during symbiosis were revealed. Transcriptome and proteome data of potato during compatible and incompatible interactions with Phytophthora infestans (Ali et al., 2014) revealed the de-regulation of over 17,000 transcripts and 1,000 expressed proteins. Kunitz-like protease inhibitors, RCR3-like proteins, and several TFs were strictly activated during incompatible interactions. The change in transcript abundance corresponded to a change in half of the differentially abundant proteins. The protein and metabolite profiles of genetically near-resistant and susceptible tomato lines against tomato yellow leaf curl virus (TYLCV) infection were compared (Adi et al., 2012). In the susceptible line, antioxidant, pathogenesis-related, and wound-induced proteins were prominent, whereas protein and chemical chaperones preserved homeostasis. Carbon and nitrogen metabolisms were less influenced by resistant vs. susceptible ones. Recently, Chialva et al. (2020) sought to investigate whether host-targeted transcriptomics can identify the taxonomic and functional diversity of root microbiota by using an RNA-seq dataset generated for tomato plants growing on different native soils. The authors were able to reconstruct the microbial communities and grasp an overview of their functional diversity. By comparing the host transcriptome and the meta-transcriptome, the composition and metabolic activities of the microbiota seem to influence plant responses at the molecular level. Zolti et al. (2020) reported that microbial profiling can reveal stress signals that cannot be detected even when the host transcriptome is analyzed, providing a different method of assessing the in-situ conditions that host organisms encounter. The presence/absence and quantity of certain biological components may be precisely measured by applying and comparing transdisciplinary -omics techniques on horticultural plant-microbe interactions (Figure 3).
Figure 3. Exploring the diverse approaches and relationships in holo-omics for microbial horticulture.
Different statistical methods can be used to analyze the metabarcoding data to determine which microbiome characteristics are most explanatory of the variation in the functional feature of interest. These explanatory features can be aggregated properties at the community, network layers (i.e., microbial network connectance or community alpha-diversity), presence or abundance of specific microbial taxa, or microbial functional groups (Barroso-Bergadà et al., 2021). According to Borokini et al. (2021), the PCNM eigenvectors method created for geographic distance matrices can be used to transform the compositional dissimilarity matrix between samples (community beta-diversity) into explanatory vectors. In the statistical model, the functional trait of interest will be considered as the dependent variable while the microbiome characteristics and environmental factors will be considered as explanatory variables (i.e., drought tolerance, Cambon et al., 2022; yield, Asad et al., 2021; an example of paired samples, Pérez-Valera et al., 2020). The differential abundance analysis methods (Nearing et al., 2022) or pTITAN2 approach (Figary et al., 2022) can be used to determine which microbial taxa are the determinant components when the microbiome significantly influences the functional trait of interest. Moreover, deep learning or machine learning approaches may also be used (Xu et al., 2022). For instance, the Random Forest algorithm has been applied to find combinations of horticultural yield indicative microbial taxa (Yergeau et al., 2020). Structural equation models (SEM) can test particular hypotheses regarding the direct and indirect impacts of the explanatory factors (e.g., microbiome) on the functional trait of interest in horticultural crops (Xiao et al., 2021).
The wealth of -omics data generated by high-throughput methods is used by computational methods to infer networks of ecological interactions within microbial taxa, reveal the trophic and metabolic relationships underlying them, and examine how these relationships affect microbiome dynamics at various time scales. Metabarcoding has been utilized for a long time by microbial ecologists to discover ecological connections between microbial taxa. Data showed that patterns in relative shifts in frequency may reveal information about the structure of the ecological interaction network since interactions between microbial taxa in the past or present may have an impact on their frequencies (Kerdraon et al., 2019; Cobo-Díaz et al., 2022). This procedure is not simple since metabarcoding data are always compositional and can be skewed by amplification and sequencing abnormalities (Arribas et al., 2022). SparCC (Chen et al., 2022) was one of the methods used for estimating interaction networks from relative sequence counts. SparCC avoided the compositional effect by obtaining correlation using log-transformed components. Correlation-based measurements were refined by other tools like CCLasso (Matchado et al., 2021) or SPIEC-EASI (Liu et al., 2023). While MPLasso (Chen et al., 2022) incorporates the application of prior microbiological knowledge by data-mining of external sources of information, PLN (Chiquet et al., 2019) improves inference accuracy by utilizing sample covariance. Recently, HMSC was used to estimate microbial networks and incorporates variables on samples and taxa (Abrego et al., 2020; Fort et al., 2021). These methods could be rapidly employed on even rather big datasets and are resistant to noise in experimental data. The statistical computing powerhouse R integrates the majority of these methods, which makes handling data from various sources easy (Dohlman and Shen, 2019; Tikhonov et al., 2020). These tools allow us to connect the identified positive and negative interactions and the ecological mechanisms. Explainable machine learning can link inferred interaction networks to ecological mechanisms (Tamaddoni-Nezhad et al., 2021). Several horticultural crops-microbiome interactions (i.e., mutualism, competition) affect the frequency of different species in different ways (Song et al., 2020; Venkataram et al., 2023). With minimal to no human involvement in the interpretation stage, explainable machine learning translates these requirements into logical rules that explicitly infer ecological interactions (Barroso-Bergadà et al., 2021). Through a process that simultaneously detects interactions and categorizes them as mutualism, competition, commensalism, or amensalism, hypotheses of ecological interactions among taxa pairs could be generated. This straightforward method is especially useful for reconstructing microbial networks in previously unexplored ecosystems, where human interpretation expertise may be deficient.
The combined collection of horticultural host and microbial data is a potential strategy for uncovering plant-microbiome interactions by creating mechanism-based questions and testable hypotheses. However, analyzing holo-omics has its own set of challenges; the high cost of the used techniques in holo-omic approaches such as shotgun sequencing. In some circumstances, targeted sequencing or DNA microarray techniques can be more cost-effective than shallow shotgun sequencing, which can recover better taxonomic resolution at the same cost, while also offering direct insight regarding functioning (Hillmann et al., 2018). Researchers may be able to show a link between the presence of certain microbes and genetic or phenotypic features of the host plant using targeted techniques, but shotgun sequencing will frequently be required to achieve whole-genome resolution. The implementation of holo-omics methods needs a wide range of experts. Indeed, plant and microbial biologists will need statisticians and computational biologists for the identification and implementation of the approaches with suitable statistical precision (Joyce and Palsson, 2006). Additionally, the lack of completely established analytical methods constitutes another key problem, and there is a considerable need for the development of adapted bioinformatic assets. For the existing assets, choosing which one to use can be challenging since some will be dependent on the specific problems under inquiry, while others will be generalizable to all experiments and data types (Franzosa et al., 2015). There is generally significantly more software suited for multi-omic analysis of either the host or microbiome in isolation (Xia et al., 2010; Rohart et al., 2017; Chong et al., 2018; Rahnavard et al., 2018; Jamil et al., 2020; Subramanian et al., 2020) than tools for integrating information from both at the same time (Gui et al., 2020; Su et al., 2020). For instance, gNOMO is a bioinformatic channel particularly intended to process and analyze non-model organism materials at up to three meta-omics layers including metagenomics, metatranscriptomics, and metaproteomics in an integrated way (Muñoz-Benavent et al., 2020), although analysis does not support the host information. The development and implementation of statistical approaches that directly combine orthogonal datasets into a single analytical framework remain challenging. Currently, the majority of plant microbiome studies employing the holo-omic approach focus on discrete -omic analyses first, then combine results from various levels afterward based on existing data and prior knowledge (Limborg et al., 2018; Liu et al., 2021). While this method is very simple to execute, it may overlook key correlations between various-omics levels (Chen et al., 2012; Sun and Hu, 2016). Another area of importance is the development of methods to integrate holo-omic with non-omic analyses. López de Maturana et al. (2019) suggested a joint modeling approach to increase our understanding of the extent of the impact of host-microbes interaction that will exceed host performances to theoretically broader environmental and evolutionary shifts.
Holo-omics figures as a useful asset that may be utilized to increase our understanding of the basis of horticultural plant-microbiome interactions. Implementation of this approach will need the boost of developing alternative sequencing-data integration techniques with potential applications outside of plant biology. Following this approach would inspire plant and microbial biologists, ecologists, statisticians, and computational scientists to collaborate in creating combined experimental frameworks that involve multiple scientific views. This process of technical and conceptual harmonization of approaches throughout the scientific community is, possibly, the most difficult obstacle to a comprehensive understanding of the natural environment. A better understanding of the plant-microbiome interactions through the holo-omics approach will provide more potentialities to predict, mitigate, and promote horticulture productivity under a changing climate. It is necessary to investigate how much we can alter the plant microbiome to boost sustainable agricultural productivity while preserving the environment. The influence of host genetics on the research of microbiome diversity and structure has the potential to aid in the selection of microbiomes with particular features. There is a need for the integration of a holo-omics method that might link the relationship between the microbial communities, horticultural plant, and environmental function to obtain advanced fundamental knowledge about the specific host–microbe interaction under changing global climate, taking into account the community context.
MB contributed to conceptualization, visualization, research design, and funding acquisition and revised and edited the review. MA-E-M drafted the first version of the manuscript with editorial input from MB. MB and MA-E-M designed the figures. All authors have read and agreed to the published version of the manuscript.
This work was supported as part of FOSC project (Sus-Agri-CC) from the European Union’s Horizon 2020 Research and Innovation Program (grant agreement no. 862555) and PRIMA project (SALAM-MED) from the European Union’s Horizon 2020 Research and Innovation Program (grant agreement no. 2131).
MB acknowledges the support of the Faculty of Sciences Semlalia (Marrakesh, Morocco). MB is grateful to Meddich Abdelillah and all other AGROBIOVAL team members for helpful comments. We apologize to colleagues whose work could not be referenced due to length constraints.
The authors declare that the research was conducted in the absence of any commercial or financial relationships that could be construed as a potential conflict of interest.
All claims expressed in this article are solely those of the authors and do not necessarily represent those of their affiliated organizations, or those of the publisher, the editors and the reviewers. Any product that may be evaluated in this article, or claim that may be made by its manufacturer, is not guaranteed or endorsed by the publisher.
Abbott, K. C., Eppinga, M. B., Umbanhowar, J., Baudena, M., and Bever, J. D. (2021). Microbiome influence on host community dynamics: conceptual integration of microbiome feedback with classical host–microbe theory. Ecol. Lett. 24, 2796–2811. doi: 10.1111/ele.13891
Abedini, D., Jaupitre, S., Bouwmeester, H., and Dong, L. (2021). Metabolic interactions in beneficial microbe recruitment by plants. Curr. Opin. Biotechnol. 70, 241–247. doi: 10.1016/j.copbio.2021.06.015
Abrego, N., Roslin, T., Huotari, T., Tack, A. J. M., Lindahl, B. D., Tikhonov, G., et al. (2020). Accounting for environmental variation in co-occurrence modelling reveals the importance of positive interactions in root-associated fungal communities. Mol. Ecol. 29, 2736–2746. doi: 10.1111/mec.15516
Adeniji, A. A., Babalola, O. O., and Loots, D. T. (2020). Metabolomic applications for understanding complex tripartite plant-microbes interactions: strategies and perspectives. Biotechnol. Rep. 25:e00425. doi: 10.1016/j.btre.2020.e00425
Adi, M., Jens, P., Brotman, Y., Mikhail, K., Iris, S., Henryk, C., et al. (2012). Stress responses to tomato yellow leaf curl virus (TYLCV) infection of resistant and susceptible tomato plants are different. J. Postgenomics Drug Biomark. Dev. s1, 1–13. doi: 10.4172/2153-0769.s1-006
Agler, M. T., Ruhe, J., Kroll, S., Morhenn, C., Kim, S. T., Weigel, D., et al. (2016). Microbial hub taxa link host and abiotic factors to plant microbiome variation. PLoS Biol. 14, 1–31. doi: 10.1371/journal.pbio.1002352
Agnolucci, M., Avio, L., Palla, M., Sbrana, C., Turrini, A., and Giovannetti, M. (2020). Health-promoting properties of plant products: the role of mycorrhizal fungi and associated bacteria. Agronomy 10, 1–20. doi: 10.3390/agronomy10121864
Ait Rahou, Y., Ait-El-Mokhtar, M., Anli, M., Boutasknit, A., Ben-Laouane, R., Douira, A., et al. (2020). Use of mycorrhizal fungi and compost for improving the growth and yield of tomato and its resistance to Verticillium dahliae. Arch. Phytopathol. Plant Prot. 54, 1–26. doi: 10.1080/03235408.2020.1854938
Ait-El-Mokhtar, M., Baslam, M., Ben-Laouane, R., Anli, M., Boutasknit, A., Mitsui, T., et al. (2020). Alleviation of detrimental effects of salt stress on date palm (Phoenix dactylifera L.) by the application of arbuscular mycorrhizal fungi and/or compost. Front. Sustain. Food Syst. 4:131. doi: 10.3389/fsufs.2020.00131
Ait-El-Mokhtar, M., Laouane, R. B., Anli, M., Boutasknit, A., Wahbi, S., and Meddich, A. (2019). Use of mycorrhizal fungi in improving tolerance of the date palm (Phoenix dactylifera L.) seedlings to salt stress. Sci. Hortic. 253, 429–438. doi: 10.1016/j.scienta.2019.04.066
Alberdi, A., Andersen, S. B., Limborg, M. T., Dunn, R. R., and Gilbert, M. T. P. (2022). Disentangling host–microbiota complexity through hologenomics. Nat. Rev. Genet. 23, 281–297. doi: 10.1038/s41576-021-00421-0
Ali, A., Alexandersson, E., Sandin, M., Resjö, S., Lenman, M., Hedley, P., et al. (2014). Quantitative proteomics and transcriptomics of potato in response to Phytophthora infestans in compatible and incompatible interactions. BMC Genomics 15, 1–18. doi: 10.1186/1471-2164-15-497
Ali, S., Tyagi, A., Park, S., Mir, R. A., Mushtaq, M., Bhat, B., et al. (2022). Deciphering the plant microbiome to improve drought tolerance: mechanisms and perspectives. Environ. Exp. Bot. 201:104933. doi: 10.1016/j.envexpbot.2022.104933
Aliferis, K. A., Faubert, D., and Jabaji, S. (2014). A metabolic profiling strategy for the dissection of plant defense against fungal pathogens. PLoS One 9:e111930. doi: 10.1371/journal.pone.0111930
Anli, M., Baslam, M., Tahiri, A.-I., Raklami, A., Boutasknit, A., Symanczik, S., et al. (2020). Biofertilizers as strategies to improve photosynthetic apparatus, growth, and drought stress tolerance in the date palm. Front. Plant Sci. 11:516818. doi: 10.3389/fpls.2020.516818
Antolín, M. C., Izurdiaga, D., Urmeneta, L., Pascual, I., Irigoyen, J. J., and Goicoechea, N. (2020). Dissimilar responses of ancient grapevines recovered in Navarra (Spain) to arbuscular mycorrhizal symbiosis in terms of berry quality. Agronomy 10:473. doi: 10.3390/agronomy10040473
Arcate, J. M., Karp, M. A., and Nelson, E. B. (2006). Diversity of Peronosporomycete (oomycete) communities associated with the rhizosphere of different plant species. Microb. Ecol. 51, 36–50. doi: 10.1007/s00248-005-0187-y
Arif, I., Batool, M., and Schenk, P. M. (2020). Plant microbiome engineering: expected benefits for improved crop growth and resilience. Trends Biotechnol. 38, 1385–1396. doi: 10.1016/j.tibtech.2020.04.015
Arribas, P., Andújar, C., Bohmann, K., de Waard, J. R., Economo, E. P., Elbrecht, V., et al. (2022). Toward global integration of biodiversity big data: a harmonized metabarcode data generation module for terrestrial arthropods. Gigascience 11:giac065. doi: 10.1093/gigascience/giac065
Asad, N. I., Tremblay, J., Dozois, J., Mukula, E., L’Espérance, E., Constant, P., et al. (2021). Predictive microbial-based modelling of wheat yields and grain baking quality across a 500 km transect in Québec. FEMS Microbiol. Ecol. 97, 1–12. doi: 10.1093/femsec/fiab160
Ayesha, S., Hanif, M. K., and Talib, R. (2020). Overview and comparative study of dimensionality reduction techniques for high dimensional data. Inf. Fusion 59, 44–58. doi: 10.1016/j.inffus.2020.01.005
Babenko, L. M., Kosakivska, I. V., and Romanenko, К. О. (2022). Molecular mechanisms of N-acyl homoserine lactone signals perception by plants. Cell Biol. Int. 46, 523–534. doi: 10.1002/cbin.11749
Bai, B., Liu, W., Qiu, X., Zhang, J., Zhang, J., and Bai, Y. (2022). The root microbiome: community assembly and its contributions to plant fitness. J. Integr. Plant Biol. 64, 230–243. doi: 10.1111/jipb.13226
Bai, Y., Müller, D. B., Srinivas, G., Garrido-Oter, R., Potthoff, E., Rott, M., et al. (2015). Functional overlap of the Arabidopsis leaf and root microbiota. Nature 528, 364–369. doi: 10.1038/nature16192
Barroso-Bergadà, D., Pauvert, C., Vallance, J., Delière, L., Bohan, D. A., Buée, M., et al. (2021). Microbial networks inferred from environmental DNA data for biomonitoring ecosystem change: strengths and pitfalls. Mol. Ecol. Resour. 21, 762–780. doi: 10.1111/1755-0998.13302
Bartoli, C., Frachon, L., Barret, M., Rigal, M., Huard-Chauveau, C., Mayjonade, B., et al. (2018). In situ relationships between microbiota and potential pathobiota in Arabidopsis thaliana. ISME J. 12, 2024–2038. doi: 10.1038/s41396-018-0152-7
Batstone, R. T., Lindgren, H., Allsup, C. M., Goralka, L. A., Riley, A. B., Grillo, M. A., et al. (2022). Genome-wide association studies across environmental and genetic contexts reveal complex genetic architecture of symbiotic extended phenotypes. MBio 13:e0182322. doi: 10.1128/mbio.01823-22
Belimov, A. A., Shaposhnikov, A. I., Azarova, T. S., Makarova, N. M., Safronova, V. I., Litvinskiy, V. A., et al. (2020). Microbial consortium of PGPR, rhizobia and arbuscular mycorrhizal fungus makes pea mutant SGECdt comparable with Indian mustard in cadmium tolerance and accumulation. Plan. Theory 9, 1–21. doi: 10.3390/plants9080975
Benhamou, N., le Floch, G., Vallance, J., Gerbore, J., Grizard, D., and Rey, P. (2012). Pythium oligandrum: an example of opportunistic success. Microbiology 158, 2679–2694. doi: 10.1099/mic.0.061457-0
Benhamou, N., Rey, P., Chérif, M., Hockenhull, J., and Tirilly, Y. (1997). Treatment with the mycoparasite Pythium oligandrum triggers induction of defense-related reactions in tomato roots when challenged with Fusarium oxysporum f. sp. radicis-lycopersici. Phytopathology 87, 108–122. doi: 10.1094/PHYTO.1997.87.1.108
Ben-Laouane, R., Baslam, M., Ait-El-Mokhtar, M., Anli, M., Boutasknit, A., Ait-Rahou, Y., et al. (2020). Potential of native arbuscular mycorrhizal fungi, rhizobia, and/or green compost as alfalfa (Medicago sativa) enhancers under salinity. Microorganisms 8:1695. doi: 10.3390/microorganisms8111695
Berendsen, R. L., Pieterse, C. M. J., and Bakker, P. A. H. M. (2012). The rhizosphere microbiome and plant health. Trends Plant Sci. 17, 478–486. doi: 10.1016/j.tplants.2012.04.001
Bettenfeld, P., Cadena i Canals, J., Jacquens, L., Fernandez, O., Fontaine, F., van Schaik, E., et al. (2022). The microbiota of the grapevine holobiont: a key component of plant health. J. Adv. Res. 40, 1–15. doi: 10.1016/j.jare.2021.12.008
Bhattarai, B., Steffensen, S. K., Gregersen, P. L., Kristensen, H. L., and Fomsgaard, I. S. (2021). Stepwise mass spectrometry-based approach for confirming the presence of benzoxazinoids in herbs and vegetables. Phytochem. Anal. 32, 283–297. doi: 10.1002/pca.2973
Bona, E., Cantamessa, S., Massa, N., Manassero, P., Marsano, F., Copetta, A., et al. (2016). Arbuscular mycorrhizal fungi and plant growth-promoting pseudomonads improve yield, quality and nutritional value of tomato: a field study. Mycorrhiza 27, 1–11. doi: 10.1007/s00572-016-0727-y
Bona, E., Lingua, G., Manassero, P., Cantamessa, S., Marsano, F., Todeschini, V., et al. (2015). AM fungi and PGP pseudomonads increase flowering, fruit production, and vitamin content in strawberry grown at low nitrogen and phosphorus levels. Mycorrhiza 25, 181–193. doi: 10.1007/s00572-014-0599-y
Borokini, I. T., Weisberg, P. J., and Peacock, M. M. (2021). Quantifying the relationship between soil seed bank and plant community assemblage in sites harboring the threatened Ivesia webberi in the western great basin desert. Appl. Veg. Sci. 24:e12547. doi: 10.1111/avsc.12547
Boutasknit, A., Baslam, M., Ait-El-Mokhtar, M., Anli, M., Ben-Laouane, R., Ait-Rahou, Y., et al. (2021). Assemblage of indigenous arbuscular mycorrhizal fungi and green waste compost enhance drought stress tolerance in carob (Ceratonia siliqua L.) trees. Sci. Rep. 11, 1–23. doi: 10.1038/s41598-021-02018-3
Brunel, C., Pouteau, R., Dawson, W., Pester, M., Ramirez, K. S., and van Kleunen, M. (2020). Towards unraveling macroecological patterns in rhizosphere microbiomes. Trends Plant Sci. 25, 1017–1029. doi: 10.1016/j.tplants.2020.04.015
Bücker-Neto, L., Paiva, A. L. S., Machado, R. D., Arenhart, R. A., and Margis-Pinheiro, M. (2017). Interactions between plant hormones and heavy metals responses. Genet. Mol. Biol. 40, 373–386. doi: 10.1590/1678-4685-gmb-2016-0087
Buée, M., de Boer, W., Martin, F., van Overbeek, L., and Jurkevitch, E. (2009). The rhizosphere zoo: an overview of plant-associated communities of microorganisms, including phages, bacteria, archaea, and fungi, and of some of their structuring factors. Plant Soil 321, 189–212. doi: 10.1007/s11104-009-9991-3
Bulgari, R., Franzoni, G., and Ferrante, A. (2019). Biostimulants application in horticultural crops under abiotic stress conditions. Agronomy 9:306. doi: 10.3390/agronomy9060306
Cambon, M. C., Cartry, D., Chancerel, E., Ziegler, C., Levionnois, S., Coste, S., et al. (2022). Drought tolerance traits in Neotropical trees correlate with the composition of phyllosphere fungal communities. Phytobiomes J. X, 1–15. doi: 10.1094/pbiomes-04-22-0023-r
Campos-Soriano, L., Bundó, M., Bach-Pages, M., Chiang, S. F., Chiou, T. J., and San Segundo, B. (2020). Phosphate excess increases susceptibility to pathogen infection in rice. Mol. Plant Pathol. 21, 555–570. doi: 10.1111/mpp.12916
Carillo, P., Kyratzis, A., Kyriacou, M. C., Dell’Aversana, E., Fusco, G. M., Corrado, G., et al. (2020). Biostimulatory action of arbuscular mycorrhizal fungi enhances productivity, functional and sensory quality in ‘Piennolo del vesuvio’ cherry tomato landraces. Agronomy 10:911. doi: 10.3390/agronomy10060911
Chemidlin, P.-B. N., Dequiedt, S., Thioulouse, J., Lelièvre, M., Saby, N. P. A., Jolivet, C., et al. (2014). Similar processes but different environmental filters for soil bacterial and fungal community composition turnover on a broad spatial scale. PLoS One 9, 1–11. doi: 10.1371/journal.pone.0111667
Chen, R., Mias, G. I., Li-Pook-Than, J., Jiang, L., Lam, H. Y. K., Chen, R., et al. (2012). Personal omics profiling reveals dynamic molecular and medical phenotypes. Cells 148, 1293–1307. doi: 10.1016/j.cell.2012.02.009
Chen, L., Schwier, M., Krumbach, J., Kopriva, S., and Jacoby, R. P. (2021). Metabolomics in plant-microbe interactions in the roots. Adv. Bot. Res. 98, 133–161. doi: 10.1016/bs.abr.2020.09.018
Chen, L., Wan, H., He, Q., He, S., and Deng, M. (2022). Statistical methods for microbiome compositional data network inference: a survey. J. Comput. Biol. 29, 704–723. doi: 10.1089/cmb.2021.0406
Cheng, F., Ali, M., Liu, C., Deng, R., and Cheng, Z. (2020). Garlic allelochemical diallyl disulfide alleviates autotoxicity in the root exudates caused by long-term continuous cropping of tomato. J. Agric. Food Chem. 68, 11684–11693. doi: 10.1021/acs.jafc.0c03894
Cheng, Z., Lei, S., Li, Y., Huang, W., Ma, R., Xiong, J., et al. (2020). Revealing the variation and stability of bacterial communities in tomato rhizosphere microbiota. Microorganisms 8, 1–15. doi: 10.3390/microorganisms8020170
Chernov, T. I., and Zhelezova, A. D. (2020). The dynamics of soil microbial communities on different timescales: a review. Eurasian Soil Sci. 53, 643–652. doi: 10.1134/S106422932005004X
Chialva, M., Ghignone, S., Novero, M., Hozzein, W. N., Lanfranco, L., and Bonfante, P. (2020). Tomato RNA-seq data mining reveals the taxonomic and functional diversity of root- associated microbiota. Microorganisms 8:38. doi: 10.3390/microorganisms8010038
Chiquet, J., Mariadassou, M., and Robin, S. (2019). “Variational inference of sparse network from count data.” in Proceedings of the 36th International Conference on Machine Learning; 2019 June 9-15. eds. K. Chaudhuri and R. Salakhutdinov (Long Beach, CA, USA), 97, 1162–1171.
Chong, J., Soufan, O., Li, C., Caraus, I., Li, S., Bourque, G., et al. (2018). MetaboAnalyst 4.0: towards more transparent and integrative metabolomics analysis. Nucleic Acids Res. 46, W486–W494. doi: 10.1093/nar/gky310
Cissé, O. H., Almeida, J. M. G. C. F., Fonseca, Á., Kumar, A. A., Salojärvi, J., Overmyer, K., et al. (2013). Genome sequencing of the plant pathogen Taphrina deformans, the causal agent of peach leaf curl. MBio 4, 1–8. doi: 10.1128/mBio.00055-13
Cobo-Díaz, J. F., Legrand, F., Le Floch, G., and Picot, A. (2022). Influence of maize residues in shaping soil microbiota and Fusarium spp. communities. Microb. Ecol. 83, 702–713. doi: 10.1007/s00248-021-01797-6
Coince, A., Caël, O., Bach, C., Lengellé, J., Cruaud, C., Gavory, F., et al. (2013). Below-ground fine-scale distribution and soil versus fine root detection of fungal and soil oomycete communities in a French beech forest. Fungal Ecol. 6, 223–235. doi: 10.1016/j.funeco.2013.01.002
Coleman-Derr, D., Desgarennes, D., Fonseca-Garcia, C., Gross, S., Clingenpeel, S., Woyke, T., et al. (2015). Biogeography and cultivation affect microbiome composition in the drought-adapted plant subgenus agave.
Compant, S., Cambon, M. C., Vacher, C., Mitter, B., Samad, A., and Sessitsch, A. (2021). The plant endosphere world – bacterial life within plants. Environ. Microbiol. 23, 1812–1829. doi: 10.1111/1462-2920.15240
Compant, S., Samad, A., Faist, H., and Sessitsch, A. (2019). A review on the plant microbiome: ecology, functions, and emerging trends in microbial application. J. Adv. Res. 19, 29–37. doi: 10.1016/j.jare.2019.03.004
Cotton, T. E. A., Pétriacq, P., Cameron, D. D., Meselmani, M.Al, Schwarzenbacher, R., Rolfe, S. A., et al. (2019). Metabolic regulation of the maize rhizobiome by benzoxazinoids. ISME J. 13, 1647–1658. doi: 10.1038/s41396-019-0375-2
Crouch, J. A., Dawe, A., Aerts, A., Barry, K., Churchill, A. C. L., Grimwood, J., et al. (2020). Genome sequence of the chestnut blight fungus Cryphonectria parasitica EP155: a fundamental resource for an archetypical invasive plant pathogen. Phytopathology 110, 1180–1188. doi: 10.1094/PHYTO-12-19-0478-A
Dabré, É. E., Hijri, M., and Favret, C. (2022). Influence on soybean aphid by the tripartite interaction between soybean, a rhizobium bacterium, and an arbuscular mycorrhizal fungus. Microorganisms 10:1196. doi: 10.3390/microorganisms10061196
Darriaut, R., Lailheugue, V., Masneuf-Pomarède, I., Marguerit, E., Martins, G., Compant, S., et al. (2022). Grapevine rootstock and soil microbiome interactions: keys for a resilient viticulture. Hortic. Res. 9:uhac019. doi: 10.1093/hr/uhac019
Dastogeer, K. M. G., Tumpa, F. H., Sultana, A., Akter, M. A., and Chakraborty, A. (2020). Plant microbiome–an account of the factors that shape community composition and diversity. Curr. Plant Biol. 23:100161. doi: 10.1016/j.cpb.2020.100161
De Cremer, K., Mathys, J., Vos, C., Froenicke, L., Michelmore, R. W., Cammue, B. P. A., et al. (2013). RNAseq-based transcriptome analysis of Lactuca sativa infected by the fungal necrotroph Botrytis cinerea. Plant Cell Environ. 36, 1992–2007. doi: 10.1111/pce.12106
de Jager, M. M., and Roets, F. (2023). Pathogenicity of Fusarium euwallaceae, symbiont of the polyphagous shot hole borer beetle, to selected stone fruit trees in South Africa. J. Plant Pathol. 105, 5–13. doi: 10.1007/s42161-022-01258-0
De Vrieze, M., Germanier, F., Vuille, N., and Weisskopf, L. (2018). Combining different potato-associated Pseudomonas strains for improved biocontrol of Phytophthora infestans. Front. Microbiol. 9, 1–13. doi: 10.3389/fmicb.2018.02573
Delmotte, N., Mondy, S., Alunni, B., Fardoux, J., Chaintreuil, C., Vorholt, J. A., et al. (2014). A proteomic approach of Bradyrhizobium/Aeschynomene root and stem symbioses reveals the importance of the fixA locus for symbiosis. Int. J. Mol. Sci. 15, 3660–3670. doi: 10.3390/ijms15033660
Demirjian, C., Vailleau, F., Berthomé, R., and Roux, F. (2022). Genome-wide association studies in plant pathosystems: success or failure? Trends Plant Sci. 28, 471–485. doi: 10.1016/j.tplants.2022.11.006
Deng, S., Caddell, D. F., Xu, G., Dahlen, L., Washington, L., Yang, J., et al. (2021). Genome wide association study reveals plant loci controlling heritability of the rhizosphere microbiome. ISME J. 15, 3181–3194. doi: 10.1038/s41396-021-00993-z
Deng, S., Wipf, H. M. L., Pierroz, G., Raab, T. K., Khanna, R., and Coleman-Derr, D. (2019). A plant growth-promoting microbial soil amendment dynamically alters the strawberry root bacterial microbiome. Sci. Rep. 9, 1–15. doi: 10.1038/s41598-019-53623-2
Dent, D., and Cocking, E. (2017). Establishing symbiotic nitrogen fixation in cereals and other non-legume crops: the greener nitrogen revolution. Agric. Food Secur. 6:7. doi: 10.1186/s40066-016-0084-2
Dohlman, A. B., and Shen, X. (2019). Mapping the microbial interactome: statistical and experimental approaches for microbiome network inference. Exp. Biol. Med. 244, 445–458. doi: 10.1177/1535370219836771
Dong, C. J., Wang, L. L., Li, Q., and Shang, Q. M. (2019). Bacterial communities in the rhizosphere, phyllosphere and endosphere of tomato plants. PLoS One 14, 1–17. doi: 10.1371/journal.pone.0223847
Dupont, A. Ö. C., Griffiths, R. I., Bell, T., and Bass, D. (2016). Differences in soil micro-eukaryotic communities over soil pH gradients are strongly driven by parasites and saprotrophs. Environ. Microbiol. 18, 2010–2024. doi: 10.1111/1462-2920.13220
Durán, P., Thiergart, T., Garrido-Oter, R., Agler, M., Kemen, E., Schulze-Lefert, P., et al. (2018). Microbial Interkingdom interactions in roots promote Arabidopsis survival. Cells 175, 973–983.e14. doi: 10.1016/j.cell.2018.10.020
Dye, S. M., and Bostock, R. M. (2021). Eicosapolyenoic fatty acids induce defense responses and resistance to Phytophthora capsici in tomato and pepper. Physiol. Mol. Plant Pathol. 114:101642. doi: 10.1016/j.pmpp.2021.101642
Emmanuel, O. C., and Babalola, O. O. (2020). Productivity and quality of horticultural crops through co-inoculation of arbuscular mycorrhizal fungi and plant growth promoting bacteria. Microbiol. Res. 239:126569. doi: 10.1016/j.micres.2020.126569
Esitken, A., Yildiz, H. E., Ercisli, S., Figen Donmez, M., Turan, M., and Gunes, A. (2010). Effects of plant growth promoting bacteria (PGPB) on yield, growth and nutrient contents of organically grown strawberry. Sci. Hortic. 124, 62–66. doi: 10.1016/j.scienta.2009.12.012
Ferluga, S., Steindler, L., and Venturi, V. (2008). “N-acyl homoserine lactone quorum sensing in gram-negative rhizobacteria,” in Secondary Metabolites in Soil Ecology. ed. P. Karlovsky (Berlin, Heidelberg: Springer), 69–90.
Figary, S., DeWitt, P., and Detenbeck, N. (2022). pTITAN2: permutation of treatment labels and threshold indicator taxa ANalysis. F1000Research 11:267. doi: 10.12688/f1000research.83714.1
Finkel, O. M., Salas-González, I., Castrillo, G., Law, T. F., Conway, J. M., Teixeira, P. J. P. L., et al. (2019a). Root development is maintained by specific bacteria-bacteria interactions within a complex microbiome. bioRxiv :645655. doi: 10.1101/645655v2
Finkel, O. M., Salas-González, I., Castrillo, G., Spaepen, S., Law, T. F., Teixeira, P. J. P. L., et al. (2019b). The effects of soil phosphorus content on plant microbiota are driven by the plant phosphate starvation response. PLoS Biol. 17, 1–34. doi: 10.1371/journal.pbio.3000534
Fitzpatrick, C. R., Salas-González, I., Conway, J. M., Finkel, O. M., Gilbert, S., Russ, D., et al. (2020). The plant microbiome: from ecology to reductionism and beyond. Annu. Rev. Microbiol. 74, 81–100. doi: 10.1146/annurev-micro-022620-014327
Fort, T., Pauvert, C., Zanne, A. E., Ovaskainen, O., Caignard, T., Barret, M., et al. (2021). Maternal effects shape the seed mycobiome in Quercus petraea. New Phytol. 230, 1594–1608. doi: 10.1111/nph.17153
Fournier, P., Pellan, L., Barroso-Bergadà, D., Bohan, D. A., Candresse, T., Delmotte, F., et al. (2022). The functional microbiome of grapevine throughout plant evolutionary history and lifetime. Adv. Ecol. Res. 67, 27–99. doi: 10.1016/bs.aecr.2022.09.001
Fournier, B., Steiner, M., Brochet, X., Degrune, F., Mammeri, J., Carvalho, D. L., et al. (2022). Toward the use of protists as bioindicators of multiple stresses in agricultural soils: a case study in vineyard ecosystems. Ecol. Indic. 139:108955. doi: 10.1016/j.ecolind.2022.108955
Franzosa, E. A., Hsu, T., Sirota-Madi, A., Shafquat, A., Abu-Ali, G., Morgan, X. C., et al. (2015). Sequencing and beyond: integrating molecular “omics” for microbial community profiling. Nat. Rev. Microbiol. 13, 360–372. doi: 10.1038/nrmicro3451
French, E., Ghaste, M., Widhalm, J. R., and Iyer-Pascuzzi, A. S. (2019). Defense hormones modulate root microbiome diversity and composition in tomato. bioRxiv :656769. doi: 10.1101/656769
Fu, R., Feng, H., Dini-Andreote, F., Wang, Z., Bo, C., Cao, W., et al. (2021). Modulation of the tomato rhizosphere microbiome via changes in root exudation mediated by the ethylene receptor NR. Microorganisms 9:2456. doi: 10.3390/microorganisms9122456
Galloway-Peña, J., and Hanson, B. (2020). Tools for analysis of the microbiome. Dig. Dis. Sci. 65, 674–685. doi: 10.1007/s10620-020-06091-y
Gao, R., Cheng, Y., Wang, Y., Wang, Y., Guo, L., and Zhang, G. (2015). Genome sequence of Phytophthora fragariae var. fragariae, a quarantine plant-pathogenic fungus. Genome Announc. 3:e00034-15. doi: 10.1128/genomeA.00034-15
Gao, B., Chi, L., Zhu, Y., Shi, X., Tu, P., Li, B., et al. (2021). An introduction to next generation sequencing bioinformatic analysis in gut microbiome studies. Biomol. Ther. 11:530. doi: 10.3390/biom11040530
Gao, Z., Han, M., Hu, Y., Li, Z., Liu, C., Wang, X., et al. (2019). Effects of continuous cropping of sweet potato on the fungal community structure in rhizospheric soil. Front. Microbiol. 10:2269. doi: 10.3389/fmicb.2019.02269
Gao, L., Tu, Z. J., Millett, B. P., and Bradeen, J. M. (2013). Insights into organ-specific pathogen defense responses in plants: RNA-seq analysis of potato tuber-Phytophthora infestans interactions. BMC Genomics 14:340. doi: 10.1186/1471-2164-14-340
Gartemann, K. H., Abt, B., Bekel, T., Burger, A., Engemann, J., Flügel, M., et al. (2008). The genome sequence of the tomato-pathogenic actinomycete Chvibacter michiganensis subsp. michiganensis NCPPB382 reveals a large island involved in pathogenicity. J. Bacteriol. 190, 2138–2149. doi: 10.1128/JB.01595-07
Ge, S., He, L., Jin, L., Xia, X., Li, L., Ahammed, G. J., et al. (2022). Light-dependent activation of HY5 promotes mycorrhizal symbiosis in tomato by systemically regulating strigolactone biosynthesis. New Phytol. 233, 1900–1914. doi: 10.1111/nph.17883
Geisen, S., Bandow, C., Römbke, J., and Bonkowski, M. (2015). Erratum to “soil water availability strongly alters the community composition of soil protists” [Pedobiologia - J. soil Ecol, 57 (4-6) (2014) 205-213]. Pedobiologia 58:55. doi: 10.1016/j.pedobi.2015.01.005
Goddard, M.-L., Belval, L., Martin, I. R., Roth, L., Laloue, H., Deglène-Benbrahim, L., et al. (2021). Arbuscular mycorrhizal symbiosis triggers major changes in primary metabolism together with modification of defense responses and signaling in both roots and leaves of Vitis vinifera. Front. Plant Sci. 12:721614. doi: 10.3389/fpls.2021.721614
Griffiths, R. I., Thomson, B. C., James, P., Bell, T., Bailey, M., and Whiteley, A. S. (2011). The bacterial biogeography of British soils. Environ. Microbiol. 13, 1642–1654. doi: 10.1111/j.1462-2920.2011.02480.x
Gui, S., Yang, L., Li, J., Luo, J., Xu, X., Yuan, J., et al. (2020). ZEAMAP, a comprehensive database adapted to the maize multi-Omics era. iScience 23:101241. doi: 10.1016/j.isci.2020.101241
Gulati, S., Ballhausen, M.-B., Kulkarni, P., Grosch, R., and Garbeva, P. (2020). A non-invasive soil-based setup to study tomato root volatiles released by healthy and infected roots. Sci. Rep. 10:12704. doi: 10.1038/s41598-020-69468-z
Guo, L., Han, L., Yang, L., Zeng, H., Fan, D., Zhu, Y., et al. (2014). Genome and transcriptome analysis of the fungal pathogen Fusarium oxysporum f. Sp. cubense causing banana vascular wilt disease. PLoS One 9:e95543. doi: 10.1371/journal.pone.0095543
Gusella, G., Aiello, D., Michailides, T. J., and Polizzi, G. (2021). Update of pistachio leaf spot caused by Septoria pistaciarum in light of new taxonomic advances in Italy. Fungal Biol. 125, 962–970. doi: 10.1016/j.funbio.2021.08.006
Gyetvai, G., Sønderkær, M., Göbel, U., Basekow, R., Ballvora, A., Imhoff, M., et al. (2012). The transcriptome of compatible and incompatible interactions of potato (Solanum tuberosum) with Phytophthora infestans revealed by DeepSAGE analysis. PLoS One 7:e31526. doi: 10.1371/journal.pone.0031526
Haas, B. J., Kamoun, S., Zody, M. C., Jiang, R. H. Y., Handsaker, R. E., Cano, L. M., et al. (2009). Genome sequence and analysis of the Irish potato famine pathogen Phytophthora infestans. Nature 461, 393–398. doi: 10.1038/nature08358
Hadian-Deljou, M., Esna-Ashari, M., and Mirzaie-asl, A. (2020). Alleviation of salt stress and expression of stress-responsive gene through the symbiosis of arbuscular mycorrhizal fungi with sour orange seedlings. Sci. Hortic. 268:109373. doi: 10.1016/j.scienta.2020.109373
Hanaka, A., Ozimek, E., Reszczyńska, E., Jaroszuk-Ściseł, J., and Stolarz, M. (2021). Plant tolerance to drought stress in the presence of supporting Bacteria and Fungi: an efficient strategy in horticulture. Horticulturae 7:390. doi: 10.3390/horticulturae7100390
Harbort, C. J., Hashimoto, M., Inoue, H., Niu, Y., Guan, R., Rombolà, A. D., et al. (2020). Root-secreted Coumarins and the microbiota interact to improve iron nutrition in Arabidopsis. Cell Host Microbe 28, 825–837.e6. doi: 10.1016/j.chom.2020.09.006
Hida, A., Oku, S., Miura, M., Matsuda, H., Tajima, T., and Kato, J. (2020). Characterization of methyl-accepting chemotaxis proteins (MCPs) for amino acids in plant-growth-promoting rhizobacterium Pseudomonas protegens CHA0 and enhancement of amino acid chemotaxis by MCP genes overexpression. Biosci. Biotechnol. Biochem. 84, 1948–1957. doi: 10.1080/09168451.2020.1780112
Hillmann, B., Al-ghalith, G. A., Shields-cutler, R. R., Zhu, Q., Gohl, D. M., Beckman, K. B., et al. (2018). Evaluating the information content of shallow shotgun metagenomics. mSystems 3, 1–12. doi: 10.1128/mSystems.00069-18
Hong, Y.-S., Martinez, A., Liger-Belair, G., Jeandet, P., Nuzillard, J.-M., and Cosenza, C. (2012). Metabolomics reveals simultaneous influences of plant methylation and chromatin patterning defence system and fungal growth in Botrytis cinerea- infected Vitis vinifera cv. chardonnay berries. J. Exp. Bot. 63, 695–709. doi: 10.1093/jxb/err313
Hua, C., Yang, X., and Wang, Y. (2015). Phytophthora sojae and soybean isoflavones, a model to study zoospore chemotaxis. Physiol. Mol. Plant Pathol. 92, 161–165. doi: 10.1016/j.pmpp.2015.05.003
Huang, A. C., Jiang, T., Liu, Y. X., Bai, Y. C., Reed, J., Qu, B., et al. (2019). A specialized metabolic network selectively modulates Arabidopsis root microbiota. Science 364:eaau6389. doi: 10.1126/science.aau6389
Huang, D., Ma, M., Wang, Q., Zhang, M., Jing, G., Li, C., et al. (2020). Arbuscular mycorrhizal fungi enhanced drought resistance in apple by regulating genes in the MAPK pathway. Plant Physiol. Biochem. 149, 245–255. doi: 10.1016/j.plaphy.2020.02.020
Hubbard, C. J., Brock, M. T., Van Diepen, L. T., Maignien, L., Ewers, B. E., and Weinig, C. (2018). The plant circadian clock influences rhizosphere community structure and function. ISME J. 12, 400–410. doi: 10.1038/ismej.2017.172
Ichihashi, Y., Ichihashi, Y., Date, Y., Date, Y., Shino, A., Shimizu, T., et al. (2020). Multi-omics analysis on an agroecosystem reveals the significant role of organic nitrogen to increase agricultural crop yield. Proc. Natl. Acad. Sci. U. S. A. 117, 14552–14560. doi: 10.1073/pnas.1917259117
Jacoby, R. P., Chen, L., Schwier, M., Koprivova, A., and Kopriva, S. (2020). Recent advances in the role of plant metabolites in shaping the root microbiome. F1000Research 9, 1–7. doi: 10.12688/f1000research.21796.1
Jamil, I. N., Remali, J., Azizan, K. A., Nor Muhammad, N. A., Arita, M., Goh, H.-H., et al. (2020). Systematic multi-omics integration (MOI) approach in plant systems biology. Front. Plant Sci. 11:944. doi: 10.3389/fpls.2020.00944
Jiuyun, S., Shuhua, L., Chunyang, F., Kangjia, C., Hongxiao, T., Liping, Q., et al. (2022). N-Acetylglucosamine promotes tomato plant growth by shaping the community structure and metabolism of the rhizosphere microbiome. Microbiol. Spectr. 10, e00358–e00322. doi: 10.1128/spectrum.00358-22
Jo, Y., Back, C.-G., Choi, H., and Cho, W. K. (2020). Comparative microbiome study of mummified peach fruits by metagenomics and metatranscriptomics. Plan. Theory 9:1052. doi: 10.3390/plants9081052
Joardar, V., Lindeberg, M., Jackson, R. W., Selengut, J., Dodson, R., Brinkac, L. M., et al. (2005). Whole-genome sequence analysis of Pseudomonas syringae pv. phaseolicola 1448A reveals divergence among pathovars in genes involved in virulence and transposition. J. Bacteriol. 187, 6488–6498. doi: 10.1128/JB.187.18.6488-6498.2005
Joyce, A. R., and Palsson, B. Ø. (2006). The model organism as a system: integrating “omics” data sets. Nat. Rev. Mol. Cell Biol. 7, 198–210. doi: 10.1038/nrm1857
Jupe, F., Witek, K., Verweij, W., Śliwka, J., Pritchard, L., Etherington, G. J., et al. (2013). Resistance gene enrichment sequencing (RenSeq) enables reannotation of the NB-LRR gene family from sequenced plant genomes and rapid mapping of resistance loci in segregating populations. Plant J. 76, 530–544. doi: 10.1111/tpj.12307
Kamoun, S., Furzer, O., Jones, J. D. G., Judelson, H. S., Ali, G. S., Dalio, R. J. D., et al. (2015). The top 10 oomycete pathogens in molecular plant pathology. Mol. Plant Pathol. 16, 413–434. doi: 10.1111/mpp.12190
Kerdraon, L., Barret, M., Laval, V., and Suffert, F. (2019). Differential dynamics of microbial community networks help identify microorganisms interacting with residue-borne pathogens: the case of Zymoseptoria tritici in wheat. Microbiome 7, 1–17. doi: 10.1186/s40168-019-0736-0
Khalid, M., Hassani, D., Bilal, M., Asad, F., and Huang, D. (2017). Influence of bio-fertilizer containing beneficial fungi and rhizospheric bacteria on health promoting compounds and antioxidant activity of Spinacia oleracea L. Bot. Stud. 58:35. doi: 10.1186/s40529-017-0189-3
Khayi, S., Mondy, S., Beury-Cirou, A., Moumni, M., Hélias, V., and Faure, D. (2014). Genome sequence of the emerging plant pathogen Dickeya solani strain RNS 08.23.3.1A. Genome Announc. 2, 8–9. doi: 10.1128/genomeA.e01270-13
Kim, W., Prokchorchik, M., Tian, Y., Kim, S., Jeon, H., and Segonzac, C. (2020). Perception of unrelated microbe-associated molecular patterns triggers conserved yet variable physiological and transcriptional changes in Brassica rapa ssp. pekinensis. Hortic. Res. 7:186. doi: 10.1038/s41438-020-00410-0
Knapp, D. G., Lázár, A., Molnár, A., Vajna, B., Karácsony, Z., Váczy, K. Z., et al. (2021). Above-ground parts of white grapevine Vitis vinifera cv. furmint share core members of the fungal microbiome. Environ. Microbiol. Rep. 13, 509–520. doi: 10.1111/1758-2229.12950
Knights, H. E., Jorrin, B., Haskett, T. L., and Poole, P. S. (2021). Deciphering bacterial mechanisms of root colonization. Environ. Microbiol. Rep. 13, 428–444. doi: 10.1111/1758-2229.12934
Kohl, K. D. (2020). Ecological and evolutionary mechanisms underlying patterns of phylosymbiosis in host-associated microbial communities. Philos. Trans. R. Soc. B Biol. Sci. 375:20190251. doi: 10.1098/rstb.2019.0251
Kõiv, V., Arbo, K., Maiväli, Ü., Kisand, V., Roosaare, M., Remm, M., et al. (2019). Endophytic bacterial communities in peels and pulp of five root vegetables. PLoS One 14, 1–17. doi: 10.1371/journal.pone.0210542
Kumawat, K. C., Sharma, P., Sirari, A., Singh, I., Gill, B. S., Singh, U., et al. (2019). Synergism of Pseudomonas aeruginosa (LSE-2) nodule endophyte with Bradyrhizobium sp. (LSBR-3) for improving plant growth, nutrient acquisition and soil health in soybean. World J. Microbiol. Biotechnol. 35:47. doi: 10.1007/s11274-019-2622-0
Kundu, S., Chakraborty, D., Kundu, A., and Pal, A. (2013). Proteomics approach combined with biochemical attributes to elucidate compatible and incompatible plant-virus interactions between Vigna mungo and Mungbean Yellow Mosaic India virus. Proteome Sci. 11, 1–14. doi: 10.1186/1477-5956-11-15
Lahbouki, S., Ben-Laouane, R., Anli, M., Boutasknit, A., Ait-Rahou, Y., Ait-El-Mokhtar, M., et al. (2022). Arbuscular mycorrhizal fungi and/or organic amendment enhance the tolerance of prickly pear (Opuntia ficus-indica) under drought stress. J. Arid Environ. 199:104703. doi: 10.1016/j.jaridenv.2021.104703
Landi, L., De Miccolis Angelini, R. M., Pollastro, S., Abate, D., Faretra, F., and Romanazzi, G. (2018). Genome sequence of the brown rot fungal pathogen Monilinia fructigena 06 biological sciences 0604 genetics 06 biological sciences 0607 plant biology. BMC. Res. Notes 11, 10–12. doi: 10.1186/s13104-018-3854-z
Lazazzara, V., Vicelli, B., Bueschl, C., Parich, A., Pertot, I., Schuhmacher, R., et al. (2021). Trichoderma spp. volatile organic compounds protect grapevine plants by activating defense-related processes against downy mildew. Physiol. Plant. 172, 1950–1965. doi: 10.1111/ppl.13406
Lee, S. A., Kim, Y., Kim, J. M., Chu, B., Joa, J. H., Sang, M. K., et al. (2019). A preliminary examination of bacterial, archaeal, and fungal communities inhabiting different rhizocompartments of tomato plants under real-world environments. Sci. Rep. 9, 1–15. doi: 10.1038/s41598-019-45660-8
Lee, H. J., and Ryu, D. (2017). Worldwide occurrence of mycotoxins in cereals and cereal-derived food products: public health perspectives of their co-occurrence. J. Agric. Food Chem. 65, 7034–7051. doi: 10.1021/acs.jafc.6b04847
Leff, J. W., and Fierer, N. (2013). Bacterial communities associated with the surfaces of fresh fruits and vegetables. PLoS One 8, 1–9. doi: 10.1371/journal.pone.0059310
Li, X., Bai, T., Li, Y., Ruan, X., and Li, H. (2013). Proteomic analysis of Fusarium oxysporum f. sp. cubense tropical race 4-inoculated response to Fusarium wilts in the banana root cells. Proteome Sci. 11, 1–14. doi: 10.1186/1477-5956-11-41
Li, Y., Gu, Y., Li, J., Xu, M., Wei, Q., and Wang, Y. (2015). Biocontrol agent Bacillus amyloliquefaciens LJ02 induces systemic resistance against cucurbits powdery mildew. Front. Microbiol. 6, 1–15. doi: 10.3389/fmicb.2015.00883
Li, X., Jousset, A., de Boer, W., Carrión, V. J., Zhang, T., Wang, X., et al. (2019). Legacy of land use history determines reprogramming of plant physiology by soil microbiome. ISME J. 13, 738–751. doi: 10.1038/s41396-018-0300-0
Li, L., Liu, Q., Ge, S., Tang, M., He, L., Zou, Y., et al. (2023). SlIAA23-SlARF6 module controls arbuscular mycorrhizal symbiosis by regulating strigolactone biosynthesis in tomato. Plant Cell Environ. 46, 1921–1934. doi: 10.1111/pce.14580
Limborg, M. T., Alberdi, A., Kodama, M., Roggenbuck, M., Kristiansen, K., and Gilbert, M. T. P. (2018). Applied hologenomics: feasibility and potential in aquaculture. Trends Biotechnol. 36, 252–264. doi: 10.1016/j.tibtech.2017.12.006
Lindow, S. E., and Brandl, M. T. (2003). Microbiology of the phyllosphere. Appl. Environ. Microbiol. 69, 1875–1883. doi: 10.1128/AEM.69.4.1875-1883.2003
Lingua, G., Bona, E., Manassero, P., Marsano, F., Todeschini, V., Cantamessa, S., et al. (2013). Arbuscular mycorrhizal fungi and plant growth-promoting pseudomonads increases anthocyanin concentration in strawberry fruits (Fragaria x ananassa var. Selva) in conditions of reduced fertilization. Int. J. Mol. Sci. 14, 16207–16225. doi: 10.3390/ijms140816207
Liu, H., Brettell, L. E., Qiu, Z., and Singh, B. K. (2020). Microbiome-mediated stress resistance in plants. Trends Plant Sci. 25, 733–743. doi: 10.1016/j.tplants.2020.03.014
Liu, D., and Howell, K. (2021). Community succession of the grapevine fungal microbiome in the annual growth cycle. Environ. Microbiol. 23, 1842–1857. doi: 10.1111/1462-2920.15172
Liu, L., Huang, X., Zhang, J., Cai, Z., Jiang, K., and Chang, Y. (2020). Deciphering the relative importance of soil and plant traits on the development of rhizosphere microbial communities. Soil Biol. Biochem. 148:107909. doi: 10.1016/j.soilbio.2020.107909
Liu, Z., Ma, A., Mathé, E., Merling, M., Ma, Q., and Liu, B. (2021). Network analyses in microbiome based on high-throughput multi-omics data. Brief. Bioinform. 22, 1639–1655. doi: 10.1093/bib/bbaa005
Liu, X., Shi, Y., Yang, T., Gao, G. F., and Chu, H. (2023). QCMI: a method for quantifying putative biotic associations of microbes at the community level. iMeta 2:e92. doi: 10.1002/imt2.92
López de Maturana, E., Alonso, L., Alarcón, P., Martín-Antoniano, I. A., Pineda, S., Piorno, L., et al. (2019). Challenges in the integration of Omics and non-Omics data. Genes (Basel) 10:238. doi: 10.3390/genes10030238
López, S. M. Y., Pastorino, G. N., and Balatti, P. A. (2021). Volatile organic compounds profile synthesized and released by endophytes of tomato (Solanum lycopersici L.) and their antagonistic role. Arch. Microbiol. 203, 1383–1397. doi: 10.1007/s00203-020-02136-y
López-Angulo, J., de la Cruz, M., Chacón-Labella, J., Illuminati, A., Matesanz, S., Pescador, D. S., et al. (2020). The role of root community attributes in predicting soil fungal and bacterial community patterns. New Phytol. 228, 1070–1082. doi: 10.1111/nph.16754
Lowe, R. G. T., Cassin, A., Grandaubert, J., Clark, B. L., Van De Wouw, A. P., Rouxel, T., et al. (2014). Genomes and transcriptomes of partners in plant-fungal- interactions between canola (Brassica napus) and two Leptosphaeria species. PLoS One 9:e103098. doi: 10.1371/journal.pone.0103098
Lu, T., Ke, M., Lavoie, M., Jin, Y., Fan, X., Zhang, Z., et al. (2018). Rhizosphere microorganisms can influence the timing of plant flowering. Microbiome 6, 1–12. doi: 10.1186/s40168-018-0615-0
Lucaciu, R., Pelikan, C., Gerner, S. M., Zioutis, C., Köstlbacher, S., Marx, H., et al. (2019). A bioinformatics guide to plant microbiome analysis. Front. Plant Sci. 10:1313. doi: 10.3389/fpls.2019.01313
Lundberg, D. S., Lebeis, S. L., Paredes, S. H., Yourstone, S., Gehring, J., Malfatti, S., et al. (2012). Defining the core Arabidopsis thaliana root microbiome. Nature 488, 86–90. doi: 10.1038/nature11237
Ma, H., Pineda, A., Hannula, S. E., Kielak, A. M., Setyarini, S. N., and Bezemer, T. M. (2020). Steering root microbiomes of a commercial horticultural crop with plant-soil feedbacks. Appl. Soil Ecol. 150:103468. doi: 10.1016/j.apsoil.2019.103468
Ma, Y., Oliveira, R. S., Nai, F., Rajkumar, M., Luo, Y., Rocha, I., et al. (2015). The hyperaccumulator Sedum plumbizincicola harbors metal-resistant endophytic bacteria that improve its phytoextraction capacity in multi-metal contaminated soil. J. Environ. Manag. 156, 62–69. doi: 10.1016/j.jenvman.2015.03.024
Mahé, F., De Vargas, C., Bass, D., Czech, L., Stamatakis, A., Lara, E., et al. (2017). Parasites dominate hyperdiverse soil protist communities in neotropical rainforests. Nat. Ecol. Evol. 1, 1–8. doi: 10.1038/s41559-017-0091
Mansfield, J., Genin, S., Magori, S., Citovsky, V., Sriariyanum, M., Ronald, P., et al. (2012). Top 10 plant pathogenic bacteria in molecular plant pathology. Mol. Plant Pathol. 13, 614–629. doi: 10.1111/j.1364-3703.2012.00804.x
Margaria, P., Abbà, S., and Palmano, S. (2013). Novel aspects of grapevine response to phytoplasma infection investigated by a proteomic and phospho-proteomic approach with data integration into functional networks. BMC Genomics 14:38. doi: 10.1186/1471-2164-14-38
Markus Wilken, P., Steenkamp, E. T., Wingfield, M. J., Wilhelm de Beer, Z., and Wingfield, B. D. (2013). Draft nuclear genome sequence for the plant pathogen, Ceratocystis fimbriata. IMA Fungus 4, 357–358. doi: 10.5598/imafungus.2013.04.02.14
Matchado, M. S., Lauber, M., Reitmeier, S., Kacprowski, T., Baumbach, J., Haller, D., et al. (2021). Network analysis methods for studying microbial communities: a mini review. Comput. Struct. Biotechnol. J. 19, 2687–2698. doi: 10.1016/j.csbj.2021.05.001
Montesinos, L., Gascón, B., Ruz, L., Badosa, E., Planas, M., Feliu, L., et al. (2021). A bifunctional synthetic peptide with antimicrobial and plant elicitation properties that protect tomato plants from bacterial and fungal infections. Front. Plant Sci. 12:756357. doi: 10.3389/fpls.2021.756357
Mukhtar, T., Ur Rehman, S., Smith, D., Sultan, T., Seleiman, M. F., Alsadon, A. A., et al. (2020). Mitigation of heat stress in Solanum lycopersicum L. by ACC-deaminase and exopolysaccharide producing Bacillus cereus: effects on biochemical profiling. Sustainability 12:2159. doi: 10.3390/su12062159
Müller, H., Berg, C., Landa, B. B., Auerbach, A., Moissl-Eichinger, C., and Berg, G. (2015). Plant genotype-specific archaeal and bacterial endophytes but similar Bacillus antagonists colonize Mediterranean olive trees. Front. Microbiol. 6, 1–9. doi: 10.3389/fmicb.2015.00138
Muñoz-Benavent, M., Hartkopf, F., Van Den Bossche, T., Piro, V. C., García-Ferris, C., Latorre, A., et al. (2020). gNOMO: a multi-omics pipeline for integrated host and microbiome analysis of non-model organisms. NAR Genom. Bioinform. 2:14482. doi: 10.1093/nargab/lqaa083
Nakayasu, M., Takamatsu, K., Yazaki, K., and Sugiyama, A. (2023). Plant specialized metabolites in the rhizosphere of tomatoes: secretion and effects on microorganisms. Biosci. Biotechnol. Biochem. 87, 13–20. doi: 10.1093/bbb/zbac181
Nearing, J. T., Douglas, G. M., Hayes, M. G., MacDonald, J., Desai, D. K., Allward, N., et al. (2022). Microbiome differential abundance methods produce different results across 38 datasets. Nat. Commun. 13, 1–16. doi: 10.1038/s41467-022-28034-z
Nicoletti, R., Di Vaio, C., and Cirillo, C. (2020). Endophytic fungi of olive tree. Microorganisms 8:1321. doi: 10.3390/microorganisms8091321
Niemi, O., Laine, P., Koskinen, P., Pasanen, M., Pennanen, V., Harjunpää, H., et al. (2017). Genome sequence of the model plant pathogen Pectobacterium carotovorum SCC1. Stand. Genomic Sci. 12, 1–8. doi: 10.1186/s40793-017-0301-z
Nyholm, L., Koziol, A., Marcos, S., Botnen, A. B., Aizpurua, O., Gopalakrishnan, S., et al. (2020). Holo-omics: integrated host-microbiota multi-omics for Basic and Applied Biological Research. iScience 23:101414. doi: 10.1016/j.isci.2020.101414
Ofek-Lalzar, M., Sela, N., Goldman-Voronov, M., Green, S. J., Hadar, Y., and Minz, D. (2014). Niche and host-associated functional signatures of the root surface microbiome. Nat. Commun. 5, 1–9. doi: 10.1038/ncomms5950
Onufrak, A. J., Williams, G. M., Klingeman, W. E., Cregger, M. A., Klingeman, D. M., DeBruyn, J. M., et al. (2020). Regional differences in the structure of juglans nigra phytobiome reflect geographical differences in thousand cankers disease severity. Phytobiomes J. 4, 388–404. doi: 10.1094/PBIOMES-05-20-0044-R
Oyserman, B. O., Flores, S. S., Griffioen, T., Pan, X., van der Wijk, E., Pronk, L., et al. (2022). Disentangling the genetic basis of rhizosphere microbiome assembly in tomato. Nat. Commun. 13:3228. doi: 10.1038/s41467-022-30849-9
Padraic, H. P. R. A., Darragh, N., Claudine, D., Paurush, K., Peadar, O., et al. (2019). Draft genome sequence of the birch tree fungal pathogen Taphrina betulina UCD315. Microbiol. Resour. Announc. 8, e01255–e01219. doi: 10.1128/MRA.01255-19
Pan, A. Y. (2021). Statistical analysis of microbiome data: the challenge of sparsity. Curr. Opin. Endocr. Metab. Res. 19, 35–40. doi: 10.1016/j.coemr.2021.05.005
Parker, J., Koh, J., Yoo, M. J., Zhu, N., Feole, M., Yi, S., et al. (2013). Quantitative proteomics of tomato defense against Pseudomonas syringae infection. Proteomics 13, 1934–1946. doi: 10.1002/pmic.201200402
Passos, M. A. N., de Cruz, V. O., Emediato, F. L., de Teixeira, C. C., Azevedo, V. C. R., Brasileiro, A. C. M., et al. (2013). Analysis of the leaf transcriptome of Musa acuminata during interaction with Mycosphaerella musicola: gene assembly, annotation and marker development. BMC Genomics 14:78. doi: 10.1186/1471-2164-14-78
Patel, P., Trivedi, G., and Saraf, M. (2018). Iron biofortification in mungbean using siderophore producing plant growth promoting bacteria. Environ. Sustain. 1, 357–365. doi: 10.1007/s42398-018-00031-3
Peay, K. G., Kennedy, P. G., and Talbot, J. M. (2016). Dimensions of biodiversity in the earth mycobiome. Nat. Rev. Microbiol. 14, 434–447. doi: 10.1038/nrmicro.2016.59
Pérez-Valera, E., Verdú, M., Navarro-Cano, J. A., and Goberna, M. (2020). Soil microbiome drives the recovery of ecosystem functions after fire. Soil Biol. Biochem. 149:107948. doi: 10.1016/j.soilbio.2020.107948
Petriccione, M., Di Cecco, I., Arena, S., Scaloni, A., and Scortichini, M. (2013). Proteomic changes in Actinidia chinensis shoot during systemic infection with a pandemic Pseudomonas syringae pv. actinidiae strain. J. Proteome 78, 461–476. doi: 10.1016/j.jprot.2012.10.014
Ploch, S., Rose, L. E., Bass, D., and Bonkowski, M. (2016). High diversity revealed in leaf-associated protists (Rhizaria: Cercozoa) of brassicaceae. J. Eukaryot. Microbiol. 63, 635–641. doi: 10.1111/jeu.12314
Plouznikoff, K., Asins, M. J., de Boulois, H. D., Carbonell, E. A., and Declerck, S. (2019). Genetic analysis of tomato root colonization by arbuscular mycorrhizal fungi. Ann. Bot. 124, 933–946. doi: 10.1093/aob/mcy240
Powell, J. R., Karunaratne, S., Campbell, C. D., Yao, H., Robinson, L., and Singh, B. K. (2015). Deterministic processes vary during community assembly for ecologically dissimilar taxa. Nat. Commun. 6, 1–10. doi: 10.1038/ncomms9444
Pusztahelyi, T., Holb, I. J., and Pócsi, I. (2015). Secondary metabolites in fungus-plant interactions. Front. Plant Sci. 6, 1–23. doi: 10.3389/fpls.2015.00573
Rahnavard, A., Hitchcock, D., Pacheco, J. A., Deik, A., Dennis, C., Jeanfavre, S., et al. (2018). Netome: a computational framework for metabolite profiling and omics network analysis. bioRxiv :443903. doi: 10.1101/443903
Rai, S., Solanki, A. C., and Anal, A. K. D. (2021). “Modern biotechnological tools: an opportunity to discover complex phytobiomes of horticulture crops,” in Microbiomes and Plant Health. ed. M. K. Solanki, P. L. Kashyap, R. A. Ansari, and B. Kumari (Academic Press), 85–124.
Raza, W., Ling, N., Yang, L., Huang, Q., and Shen, Q. (2016a). Response of tomato wilt pathogen Ralstonia solanacearum to the volatile organic compounds produced by a biocontrol strain Bacillus amyloliquefaciens SQR-9. Sci. Rep. 6:24856. doi: 10.1038/srep24856
Raza, W., Wang, J., Wu, Y., Ling, N., Wei, Z., Huang, Q., et al. (2016b). Effects of volatile organic compounds produced by Bacillus amyloliquefaciens on the growth and virulence traits of tomato bacterial wilt pathogen Ralstonia solanacearum. Appl. Microbiol. Biotechnol. 100, 7639–7650. doi: 10.1007/s00253-016-7584-7
Rohart, F., Gautier, B., Singh, A., and Lê Cao, K. A. (2017). mixOmics: an R package for ‘omics feature selection and multiple data integration. PLoS Comput. Biol. 13, 1–19. doi: 10.1371/journal.pcbi.1005752
Rolfe, S. A., Griffiths, J., and Ton, J. (2019). Crying out for help with root exudates: adaptive mechanisms by which stressed plants assemble health-promoting soil microbiomes. Curr. Opin. Microbiol. 49, 73–82. doi: 10.1016/j.mib.2019.10.003
Ruggiero, M. A., Gordon, D. P., Orrell, T. M., Bailly, N., Bourgoin, T., Brusca, R. C., et al. (2015). A higher level classification of all living organisms. PLoS One 10, 1–60. doi: 10.1371/journal.pone.0119248
Runge, P., Ventura, F., Kemen, E., and Stam, R. (2023). Distinct phyllosphere microbiome of wild tomato species in central peru upon dysbiosis. Microb. Ecol. 85, 168–183. doi: 10.1007/s00248-021-01947-w
Sabatino, L., Iapichino, G., Consentino, B. B., D’Anna, F., and Rouphael, Y. (2020). Rootstock and arbuscular mycorrhiza combinatorial effects on eggplant crop performance and fruit quality under greenhouse conditions. Agronomy 10, 1–15. doi: 10.3390/agronomy10050693
Salanoubat, M., Genin, S., Artiguenave, F., Gouzy, J., Mangenot, S., Arlat, M., et al. (2002). Genome sequence of the plant pathogen Ralstonia solanacearum. Nature 415, 497–502. doi: 10.1038/415497a
Sangiorgio, D., Cellini, A., Donati, I., Ferrari, E., Tanunchai, B., Wahdan, F. M., et al. (2022). Taxonomical and functional composition of strawberry microbiome is genotype-dependent. J. Adv. Res. 42, 189–204. doi: 10.1016/j.jare.2022.02.009
Sangiorgio, D., Cellini, A., Donati, I., Pastore, C., Onofrietti, C., and Spinelli, F. (2020). Facing climate change: application of microbial biostimulants to mitigate stress in horticultural crops. Agronomy 10:794. doi: 10.3390/agronomy10060794
Sapkota, R., and Nicolaisen, M. (2015). An improved high throughput sequencing method for studying oomycete communities. J. Microbiol. Methods 110, 33–39. doi: 10.1016/j.mimet.2015.01.013
Sapp, M., Ploch, S., Fiore-Donno, A. M., Bonkowski, M., and Rose, L. E. (2015). Protists are an integral part of the Arabidopsis thaliana microbiome. Environ. Microbiol. 20, 30–43. doi: 10.1111/1462-2920.13941
Sasse, J., Martinoia, E., and Northen, T. (2018). Feed your friends: do plant exudates shape the root microbiome? Trends Plant Sci. 23, 25–41. doi: 10.1016/j.tplants.2017.09.003
Scandiani, M. M., Luque, A. G., Razori, M. V., Casalini, L. C., Aoki, T., O’Donnell, K., et al. (2015). Metabolic profiles of soybean roots during early stages of Fusarium tucumaniae infection. J. Exp. Bot. 66, 391–402. doi: 10.1093/jxb/eru432
Schuhegger, R., Ihring, A., Gantner, S., Bahnweg, G., Knappe, C., Vogg, G., et al. (2006). Induction of systemic resistance in tomato by N-acyl-L-homoserine lactone-producing rhizosphere bacteria. Plant Cell Environ. 29, 909–918. doi: 10.1111/j.1365-3040.2005.01471.x
Sebaihia, M., Bocsanczy, A. M., Biehl, B. S., Quail, M. A., Perna, N. T., Glasner, J. D., et al. (2010). Complete genome sequence of the plant pathogen Erwinia amylovora strain ATCC 49946. J. Bacteriol. 192, 2020–2021. doi: 10.1128/JB.00022-10
Shakir, S., Zaidi, S. S. E. A., De Vries, F. T., and Mansoor, S. (2021). Plant genetic networks shaping phyllosphere microbial community. Trends Genet. 37, 306–316. doi: 10.1016/j.tig.2020.09.010
Sharma, M. P., Grover, M., Chourasiya, D., Bharti, A., Agnihotri, R., Maheshwari, H. S., et al. (2020). Deciphering the role of trehalose in tripartite symbiosis among rhizobia, arbuscular mycorrhizal fungi, and legumes for enhancing abiotic stress tolerance in crop plants. Front. Microbiol. 11:509919. doi: 10.3389/fmicb.2020.509919
Sharma, C. K., Vishnoi, V. K., Dubey, R. C., and Maheshwari, D. K. (2018). A twin rhizospheric bacterial consortium induces systemic resistance to a phytopathogen Macrophomina phaseolina in mung bean. Rhizosphere 5, 71–75. doi: 10.1016/j.rhisph.2018.01.001
Shi, W., Li, M., Wei, G., Tian, R., Li, C., Wang, B., et al. (2019). The occurrence of potato common scab correlates with the community composition and function of the geocaulosphere soil microbiome. Microbiome 7, 1–18. doi: 10.1186/s40168-019-0629-2
Shine, M. B., Gao, Q., Chowda-Reddy, R. V., Singh, A. K., Kachroo, P., and Kachroo, A. (2019). Glycerol-3-phosphate mediates rhizobia-induced systemic signaling in soybean. Nat. Commun. 10:5303. doi: 10.1038/s41467-019-13318-8
Silambarasan, S., Logeswari, P., Cornejo, P., and Kannan, V. R. (2019). Role of plant growth–promoting rhizobacterial consortium in improving the Vigna radiata growth and alleviation of aluminum and drought stresses. Environ. Sci. Pollut. Res. 26, 27647–27659. doi: 10.1007/s11356-019-05939-9
Simpson, A. J. G. (2000). The complete genome sequence of the plant pathogen Xylella fastidiosa. Biochem. Soc. Trans. 28:A102. doi: 10.1042/bst028a102b
Singh, N., Singh, V., Rai, S. N., Mishra, V., Vamanu, E., and Singh, M. P. (2022). Deciphering the gut microbiome in neurodegenerative diseases and metagenomic approaches for characterization of gut microbes. Biomed. Pharmacother. 156:113958. doi: 10.1016/j.biopha.2022.113958
Song, C., Von Ahn, S., Rohr, R. P., and Saavedra, S. (2020). Towards a probabilistic understanding about the context-dependency of species interactions. Trends Ecol. Evol. 35, 384–396. doi: 10.1016/j.tree.2019.12.011
Sousa, R. M. S., Mendes, L. W., Antunes, J. E. L., Oliveira, L. M. D. S., Sousa, A. M. D. C. B., Gomes, R. L. F., et al. (2020). Diversity and structure of bacterial community in rhizosphere of lima bean. Appl. Soil Ecol. 150:103490. doi: 10.1016/j.apsoil.2019.103490
Stassen, M. J. J., Hsu, S.-H., Pieterse, C. M. J., and Stringlis, I. A. (2021). Coumarin communication along the microbiome–root–shoot axis. Trends Plant Sci. 26, 169–183. doi: 10.1016/j.tplants.2020.09.008
Stringlis, I. A., Yu, K., Feussner, K., De Jonge, R., Van Bentum, S., Van Verk, M. C., et al. (2018). MYB72-dependent coumarin exudation shapes root microbiome assembly to promote plant health. Proc. Natl. Acad. Sci. U. S. A. 115, E5213–E5222. doi: 10.1073/pnas.1722335115
Su, X., Jing, G., Zhang, Y., and Wu, S. (2020). Method development for cross-study microbiome data mining: challenges and opportunities. Comput. Struct. Biotechnol. J. 18, 2075–2080. doi: 10.1016/j.csbj.2020.07.020
Subramanian, I., Verma, S., Kumar, S., Jere, A., and Anamika, K. (2020). Multi-omics data integration, interpretation, and its application. Bioinform. Biol. Insights 14:117793221989905. doi: 10.1177/1177932219899051
Sun, Y. V., and Hu, Y. J. (2016). Integrative analysis of multi-omics data for discovery and functional studies of complex human diseases. Adv. Genet. 93, 147–190. doi: 10.1016/bs.adgen.2015.11.004
Sweeney, C. J., de Vries, F. T., van Dongen, B. E., and Bardgett, R. D. (2021). Root traits explain rhizosphere fungal community composition among temperate grassland plant species. New Phytol. 229, 1492–1507. doi: 10.1111/nph.16976
Tamaddoni-Nezhad, A., Bohan, D., Milani, G. A., Raybould, A., and Muggleton, S. (2021). “Human-machine scientific discovery,” in Human-like Machine Intelligence. eds. S. Muggleton and N. Chater (Oxford: Oxford University Press), 297–315.
Terrat, S., Horrigue, W., Dequietd, S., Saby, N. P. A., Lelièvre, M., Nowak, V., et al. (2017). Mapping and predictive variations of soil bacterial richness across France. PLoS One 12, 5–8. doi: 10.1371/journal.pone.0186766
Thomson, B. C., Tisserant, E., Plassart, P., Uroz, S., Griffiths, R. I., Hannula, S. E., et al. (2015). Soil conditions and land use intensification effects on soil microbial communities across a range of European field sites. Soil Biol. Biochem. 88, 403–413. doi: 10.1016/j.soilbio.2015.06.012
Thrall, P. H., Hochberg, M. E., Burdon, J. J., and Bever, J. D. (2007). Coevolution of symbiotic mutualists and parasites in a community context. Trends Ecol. Evol. 22, 120–126. doi: 10.1016/j.tree.2006.11.007
Tikhonov, G., Opedal, Ø. H., Abrego, N., Lehikoinen, A., de Jonge, M. M. J., Oksanen, J., et al. (2020). Joint species distribution modelling with the r-package Hmsc. Methods Ecol. Evol. 11, 442–447. doi: 10.1111/2041-210X.13345
Todeschini, V., Aitlahmidi, N., Mazzucco, E., Marsano, F., Gosetti, F., Robotti, E., et al. (2018). Impact of beneficial microorganisms on strawberry growth, fruit production, nutritional quality, and volatilome. Front. Plant Sci. 9, 1–22. doi: 10.3389/fpls.2018.01611
Toubali, S., Tahiri, A., Anli, M., Symanczik, S., Boutasknit, A., Ait-El-Mokhtar, M., et al. (2020). Physiological and biochemical behaviors of date palm vitroplants treated with microbial consortia and compost in response to salt stress. Appl. Sci. 10:8665. doi: 10.3390/app10238665
Trivedi, P., Batista, B. D., Bazany, K. E., and Singh, B. K. (2022). Plant–microbiome interactions under a changing world: responses, consequences and perspectives. New Phytol. 234, 1951–1959. doi: 10.1111/nph.18016
Turrini, A., Avio, L., Giovannetti, M., and Agnolucci, M. (2018). Functional complementarity of arbuscular mycorrhizal fungi and associated microbiota: the challenge of translational research. Front. Plant Sci. 9:1407.
Ullah, A., Farooq, M., Nadeem, F., Rehman, A., Hussain, M., Nawaz, A., et al. (2020). Zinc application in combination with zinc solubilizing Enterobacter sp. MN17 improved productivity, profitability, zinc efficiency, and quality of Desi chickpea. J. Soil Sci. Plant Nutr. 20, 2133–2144. doi: 10.1007/s42729-020-00281-3
Vacher, C., Cordier, T., and Vallance, J. (2016). Phyllosphere fungal communities differentiate more thoroughly than bacterial communities along an elevation gradient. Microb. Ecol. 72, 1–3. doi: 10.1007/s00248-016-0742-8
Van Buyten, E., and Höfte, M. (2013). Pythium species from rice roots differ in virulence, host colonization and nutritional profile. BMC Plant Biol. 13:203. doi: 10.1186/1471-2229-13-203
Venkataram, S., Kuo, H.-Y., Hom, E. F. Y., and Kryazhimskiy, S. (2023). Mutualism-enhancing mutations dominate early adaptation in a two-species microbial community. Nat. Ecol. Evol. 7, 143–154. doi: 10.1038/s41559-022-01923-8
Vieira-silva, S., Gonçalves, P. J., and Falony, G. (2021). Benchmarking microbiome transformations favors experimental quantitative approaches to address compositionality and sampling depth biases. Nat. Commun. 12:3562. doi: 10.1038/s41467-021-23821-6
Vorholt, J. A. (2012). Microbial life in the phyllosphere. Nat. Rev. Microbiol. 10, 828–840. doi: 10.1038/nrmicro2910
Wang, Z., Chen, Z., Kowalchuk, G. A., Xu, Z., Fu, X., and Kuramaeb, E. E. (2021). Succession of the resident soil microbial community in response to periodic inoculations. Appl. Environ. Microbiol. 87, 1–16. doi: 10.1128/AEM.00046-21
Wang, L., Chen, M., Lam, P. Y., Dini-Andreote, F., Dai, L., and Wei, Z. (2022). Multifaceted roles of flavonoids mediating plant-microbe interactions. Microbiome 10, 1–13. doi: 10.1186/s40168-022-01420-x
Wang, W. X., Zhang, F., Chen, Z. L., Liu, J., Guo, C., He, J. D., et al. (2017). Responses of phytohormones and gas exchange to mycorrhizal colonization in trifoliate orange subjected to drought stress. Arch. Agron. Soil Sci. 63, 14–23. doi: 10.1080/03650340.2016.1175556
Wei, Z., Gu, Y., Friman, V. P., Kowalchuk, G. A., Xu, Y., Shen, Q., et al. (2019). Initial soil microbiome composition and functioning predetermine future plant health. Sci. Adv. 5, 1–12. doi: 10.1126/sciadv.aaw0759
Wei, Y., Lan, G., Wu, Z., Chen, B., Quan, F., Li, M., et al. (2022). Phyllosphere fungal communities of rubber trees exhibited biogeographical patterns, but not bacteria. Environ. Microbiol. 24, 3777–3790. doi: 10.1111/1462-2920.15894
Wolfgang, A., Taffner, J., Guimarães, R. A., Coyne, D., and Berg, G. (2019). Novel strategies for soil-borne diseases: exploiting the microbiome and volatile-based mechanisms toward controlling meloidogyne-based disease complexes. Front. Microbiol. 10:1296. doi: 10.3389/fmicb.2019.01296
Xia, T., Hemert, J. V., and Dickerson, J. A. (2010). OmicsAnalyzer: a cytoscape plug-in suite for modeling omics data. Bioinformatics 26, 2995–2996. doi: 10.1093/bioinformatics/btq583
Xiao, E., Ning, Z., Xiao, T., Sun, W., and Jiang, S. (2021). Soil bacterial community functions and distribution after mining disturbance. Soil Biol. Biochem. 157:108232. doi: 10.1016/j.soilbio.2021.108232
Xiao, L., Zhang, F., and Zhao, F. (2022). Large-scale microbiome data integration enables robust biomarker identification. Nat. Comput. Sci. 2, 307–316. doi: 10.1038/s43588-022-00247-8
Xu, L., Pierroz, G., Wipf, H. M.-L., Gao, C., Taylor, J. W., Lemaux, P. G., et al. (2021). Holo-omics for deciphering plant-microbiome interactions. Microbiome 9:69. doi: 10.1186/s40168-021-01014-z
Xu, Q., Fu, H., Zhu, B., Hussain, H. A., Zhang, K., Tian, X., et al. (2021). Potassium improves drought stress yolerance in plants by affecting root morphology, root exudates, and microbial diversity. Meta 11:131. doi: 10.3390/metabo11030131
Xu, Y., Ge, Y., Song, J., and Rensing, C. (2020). Assembly of root-associated microbial community of typical rice cultivars in different soil types. Biol. Fertil. Soils 56, 249–260. doi: 10.1007/s00374-019-01406-2
Xu, N., Zhang, Z., Shen, Y., Zhang, Q., Liu, Z., Yu, Y., et al. (2022). Compare the performance of multiple binary classification models in microbial high-throughput sequencing datasets. Sci. Total Environ. 837:155807. doi: 10.1016/j.scitotenv.2022.155807
Xu, J., Zheng, H. J., Liu, L., Pan, Z. C., Prior, P., Tang, B., et al. (2011). Complete genome sequence of the plant pathogen Ralstonia solanacearum strain Po82. J. Bacteriol. 193, 4261–4262. doi: 10.1128/JB.05384-11
Yang, Y., Luo, W., Zhang, W., Mridha, M. A., Wijesinghe, S. N., McKenzie, E. H. C., et al. (2023). Cladosporium species associated with fruit trees in Guizhou Province, China. J. Fungi 9:250. doi: 10.3390/jof9020250
Yang, H., Zheng, Y., Yang, Z., Wang, Q.-C., Lü, P.-P., Hu, H.-W., et al. (2023). Bacterial communities in the phyllosphere are distinct from those in root and soil, and sensitive to plant species changes in subtropical tree plantations. FEMS Microbiol. Ecol. 99:fiad033. doi: 10.1093/femsec/fiad033
Yergeau, É., Quiza, L., and Tremblay, J. (2020). Microbial indicators are better predictors of wheat yield and quality than N fertilization. FEMS Microbiol. Ecol. 96, 1–13. doi: 10.1093/femsec/fiz205
Yi, Y., Lin, C., Wang, W., and Song, J. (2021). Habitat and seasonal variations in bacterial community structure and diversity in sediments of a shallow lake. Ecol. Indic. 120:106959. doi: 10.1016/j.ecolind.2020.106959
Yogendra, K. N., Kushalappa, A. C., Sarmiento, F., Rodriguez, E., and Mosquera, T. (2015). Metabolomics deciphers quantitative resistance mechanisms in diploid potato clones against late blight. Funct. Plant Biol. 42, 284–298. doi: 10.1071/FP14177
Zancarini, A., Westerhuis, J. A., Smilde, A. K., and Bouwmeester, H. J. (2021). Integration of omics data to unravel root microbiome recruitment. Curr. Opin. Biotechnol. 70, 255–261. doi: 10.1016/j.copbio.2021.06.016
Zarraonaindia, I., Owens, S. M., Weisenhorn, P., West, K., Hampton-Marcell, J., Lax, S., et al. (2015). The soil microbiome influences grapevine-associated microbiota. MBio 6, 1–10. doi: 10.1128/mBio.02527-14
Zhang, J., Liu, W., Bu, J., Lin, Y., and Bai, Y. (2023). Host genetics regulate the plant microbiome. Curr. Opin. Microbiol. 72:102268. doi: 10.1016/j.mib.2023.102268
Zhang, W., Luo, X., Mei, Y. Z., Yang, Q., Zhang, A. Y., Chen, M., et al. (2022). Priming of rhizobial nodulation signaling in the mycosphere accelerates nodulation of legume hosts. New Phytol. 235, 1212–1230. doi: 10.1111/nph.18192
Zhang, G., Wei, G., Wei, F., Chen, Z., He, M., Jiao, S., et al. (2021). Dispersal limitation plays stronger role in the community assembly of fungi relative to bacteria in rhizosphere across the arable area of medicinal plant. Front. Microbiol. 12, 1–16. doi: 10.3389/fmicb.2021.713523
Zheng, D., Liwinski, T., and Elinav, E. (2020). Interaction between microbiota and immunity in health and disease. Cell Res. 30, 492–506. doi: 10.1038/s41422-020-0332-7
Zhou, X., Wang, J.-T., Wang, W.-H., Tsui, C. K. M., and Cai, L. (2021). Changes in bacterial and fungal microbiomes associated with tomatoes of healthy and infected by Fusarium oxysporum f. sp. lycopersici. Microb. Ecol. 81, 1004–1017. doi: 10.1007/s00248-020-01535-4
Zhuang, X., McPhee, K. E., Coram, T. E., Peever, T. L., and Chilvers, M. I. (2012). Rapid transcriptome characterization and parsing of sequences in a non-model host-pathogen interaction; pea-Sclerotinia sclerotiorum. BMC Genomics 13, 1–19. doi: 10.1186/1471-2164-13-668
Zolti, A., Green, S. J., Sela, N., Hadar, Y., and Minz, D. (2020). Correction to: the microbiome as a biosensor: functional profiles elucidate hidden stress in hosts. Microbiome 8:101. doi: 10.1186/s40168-020-00883-0
Keywords: horticulture, sustainable production, holobiont, holo-omic analysis, microbiome, host-microbiome fidelity
Citation: Ait-El-Mokhtar M and Baslam M (2023) Holo-omic applications to unveil microbiome shaping sustainable horticultural production. Front. Sustain. Food Syst. 7:1151367. doi: 10.3389/fsufs.2023.1151367
Received: 26 January 2023; Accepted: 17 May 2023;
Published: 05 June 2023.
Edited by:
Riyaz Zafar Sayyed, PSGVP Mandal’s Arts, Science and Commerce College, IndiaReviewed by:
Sugitha Thankappan, Karunya Institute of Technology and Sciences, IndiaCopyright © 2023 Ait-El-Mokhtar and Baslam. This is an open-access article distributed under the terms of the Creative Commons Attribution License (CC BY). The use, distribution or reproduction in other forums is permitted, provided the original author(s) and the copyright owner(s) are credited and that the original publication in this journal is cited, in accordance with accepted academic practice. No use, distribution or reproduction is permitted which does not comply with these terms.
*Correspondence: Marouane Baslam, bW1tLmJhc2xhbUBnbWFpbC5jb20=
Disclaimer: All claims expressed in this article are solely those of the authors and do not necessarily represent those of their affiliated organizations, or those of the publisher, the editors and the reviewers. Any product that may be evaluated in this article or claim that may be made by its manufacturer is not guaranteed or endorsed by the publisher.
Research integrity at Frontiers
Learn more about the work of our research integrity team to safeguard the quality of each article we publish.