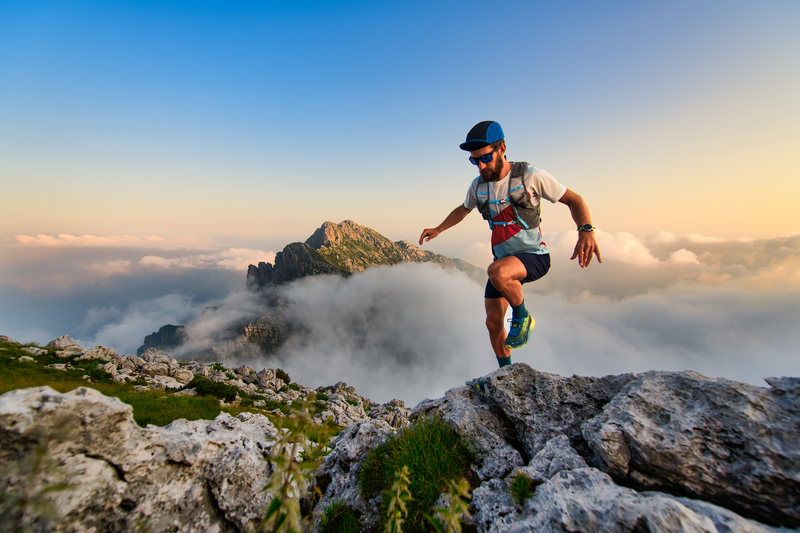
94% of researchers rate our articles as excellent or good
Learn more about the work of our research integrity team to safeguard the quality of each article we publish.
Find out more
ORIGINAL RESEARCH article
Front. Sustain. Food Syst. , 01 December 2023
Sec. Climate-Smart Food Systems
Volume 7 - 2023 | https://doi.org/10.3389/fsufs.2023.1145147
This article is part of the Research Topic Increasing Resilience and Adaptability to Climate Change of Vulnerable Groups in Agriculture View all 12 articles
Smallholder farmers are among the most vulnerable to climate shocks in Sri Lanka. Lack of education and technical skills, poverty, risks inherent to agricultural investments, limited assets, and financial capital are major reasons for low investments in enhancing adaptive capacity. The study explores the use of agricultural technologies in improving smallholder resilience to water-related disasters and their opportunities for recovery. We tested four bundled services to promote climate-smart agriculture practices namely weather index insurance (WII), agronomic advisories dissemination via SMS, weather services, and climate-resilient seeds of maize and rice. The integrated solutions are referred to as Bundled Solutions of Index Insurance with Climate Information and Seed Systems (BICSA) to manage agricultural risks in Sri Lanka. The study conducted the bundled solutions in three agroecological regions spread over five districts and covering more than 2,500 farmers in three cropping periods of Maha and Yala seasons. The results demonstrate that providing bundled solutions significantly protects smallholders against moderate drought events. The satellite-based weather index insurance can offset the long-term consequences of severe yield losses and mitigate the long-term drop in farm productivity. Our findings demonstrate the importance of bundled insurance to mitigate financial risks associated with extreme weather events and enhance resilience to climate change among vulnerable smallholders. It is evident from the study promoting a viable business model among seed companies, insurance companies, and technological partners, along with public institutions such as agricultural extension services can help production-level improvements and develop strategies at both the farm and policy levels that will support a transition to a more resilient farming system.
Over the last two decades, floods and droughts have been identified as two of the most devastating consequences of the climate crisis (Browder et al., 2021). The extreme weather events had serious implications for agriculture and food production, along with a wide range of broader impacts on the livelihood and wellbeing of the affected communities. In the last two decades, floods and drought accounted for 53% of all documented natural disasters, affecting 2.4 billion people and killing 168,000 (CRED, 2020). Droughts and floods have cost US$764 billion in damages in the period 2000–2019, with floods being the most recurrent disaster. Storms have resulted in an additional US$1,390 billion in damages, much of it from storm-related flooding (Browder et al., 2021). Climate change has made extreme weather events more severe by altering the frequency, timing, intensity, and duration, resulting in unprecedented extremes (Amarnath, 2020; Browder et al., 2021). The IPCC (2018) projected increasing global temperatures with higher and longer daily temperature extremes at a global scale. Increasing temperature generally results in an increase in potential evapotranspiration, largely because the water-holding capacity of air is increased (IPCC, 2021). It is highly likely that this development would lead to a change in the hydrologic cycle, including increased atmospheric water vapor and changes in precipitation patterns as well as changes in groundwater and soil moisture.
It has been estimated that by 2050, rising populations in flood-prone lands, climate change, deforestation, loss of wetlands, and rising sea levels are expected to increase the number of people vulnerable to flood disasters to 2 billion (UNU, 2004). At the same time, with changes in the hydrologic cycle, several water-scarce regions are experiencing severe water scarcity. High temperatures will further lead to an increase in land evapotranspiration, creating more conditions of water stress. In contrast to water stress, floods are projected to increase across more than half of the world's region, varying in magnitude across river basins (World Bank, 2016). In addition to these climate change impacts, human-induced factors affect the hydrological systems, and water resources. Most prominent are the land management practices that alter the availability of water resources and increase the risks of floods. Human-induced climate change has contributed to increased agricultural and ecological droughts in some regions due to increased evapotranspiration (IPCC, 2021).
These events and anthropogenic interferences will increase the frequency and intensity of floods and droughts at the regional scale as indicated by the climate model projections (He et al., 2020). This leads to the consideration of developing instruments at the regional scale and local scale to mitigate challenges from extreme weather events. To increase risk resilience at the regional and local level, it is imperative to invest in the exchange of tools, knowledge, and resources to systematically prepare for and respond to floods and droughts (Browder et al., 2021). Integrating different information drawn from existing climate, agriculture, risk, and socioeconomic datasets can increase preparedness for floods and droughts. The various datasets integrated together provide an opportunity for studying climate variabilities and their implications on agriculture and food production. The integration of such heterogeneous datasets can be used to increase risk resilience at the community level.
The development of index-based weather insurance products to compensate agricultural households because of extreme weather events is one such mechanism (Aheeyar et al., 2021; Amarnath et al., 2021). It is a cost-effective way of safeguarding against climate uncertainty, thereby protecting smallholder families from food insecurity and hunger and giving them the confidence to invest in and improve their farming enterprises (Manojkumar et al., 2003; Bryla and Syroka, 2007; Delavallade et al., 2015).
Index-based flood insurance is an innovative approach that integrates different information for designing the insurance product. Remote sensing-based datasets are used for mapping historical and current flood events to determine the spatial extent of the floods. Hydrodynamic models can then be developed to help determine the spatiotemporal variability of flood parameters (Malik and Amarnath, 2021). These models incorporate different time series datasets such as monthly rainfall and frequency, population involved in agriculture, number of people affected historically, flood-affected area, estimated economic loss, and yield loss of the primary crop (Matheswaran et al., 2019). The flood parameters (flood depth and duration) at daily time intervals for a specific period of time can be used to create triggers (Amarnath et al., 2017; Amarnath and Sikka, 2018) that define the insurance scheme. During extreme rainfall events, data provided by local weather stations are matched with the triggers. If the rainfall exceeds the threshold triggers, the farmers enrolled in the program are entitled to a payout. Similar exercises with different datasets, such as evapotranspiration, water deficiency, and water satisfaction index for estimating crop yield, along with the Normalized Difference Vegetation Index (NDVI), can be used for developing an index-based drought insurance scheme (Bucheli et al., 2021). The index-based insurance products can be bundled with local information and advisories such as weather information, loans, crop and water management methods, and fertilizer usage (Mukherjee et al., 2017) for climate-smart transformation to avoid climate disasters.
Countries in Asia and the Pacific are most vulnerable to water-related disasters, accounting for more than 45% of fatalities and more than 90% of the people affected by disasters (ADB, 2021). In the Asian region, eight countries, the People's Republic of China, India, Indonesia, the Maldives, Myanmar, Pakistan, Sri Lanka, and Thailand, are most affected by floods (ADB, 2013). Therefore, adaptation measures are required to sustain agricultural productivity and enhance the resilience of the agricultural system to climate change. In Sri Lanka, for example, smallholder farmers are among the most vulnerable to natural disasters. Over 27 million people have been affected by floods and droughts, with economic losses estimated at over US$2.62 billion since 1966. Considering weather insurance as a social protection tool along with other advisories, the present study focuses on the application of bundling climate-smart agriculture and risk transfer for agricultural resilience in Sri Lanka. The application of the tool earmarks the integration of global, national, and local datasets for reducing agricultural risk and vulnerability. The study explains the agglomeration of different datasets and digitization in the pathway for developing instruments for increasing agricultural resilience with the help of a case study. The main objective of the study was to provide evidence about the construction and implementation of bundled climate-smart solutions in Sri Lanka. The bundled insurance was tested in five districts—Ampara, Anuradhapura, Kurunegala, Monaragala, and Vavuniya. The distribution of bundled insurance across the farmers provides learnings that lead to key recommendations for scaling up insurance through the utilization of a sustainable business model.
Index-based weather insurance is an alternative adaptation measure with a financial remedy designed based on predetermined weather variables such as rainfall level, temperature, floods, and droughts (Ahmed, 2013; Afriyie-Kraft et al., 2020). The mechanism of index insurance works through triggers derived from weather indices, instead of the direct loss assessments made at the farm level (Ellis, 2017). The triggers of the weather events are set to a defined local or regional weather station (IFAD, 2017a). The premiums for the insurance are usually related to the expected income of the cost of production (Ellis, 2017). All policyholders within a region defined by the weather station receive payouts based on the same contract and measurement according to the set weather station (IFAD, 2017a). Index insurance has a uniform structure, does not require trained experts to check or confirm losses, and thus has comparatively low administrative costs. This helps to avoid loss adjustment by both the insurer and the insured, which lessens risks, enhances trust, and avoids alterations (Shahadat, 2013; Carter et al., 2014; Greatrex et al., 2015). Index insurance can operate as a stand-alone contract or be linked to credit for buying farm inputs, rendering insured farmers more credit-worthy than uninsured ones (Meze-Hausken et al., 2009).
Climate-smart technologies are critical for addressing climate change and variability that help increase agricultural productivity, enhance resilience, and lower emissions. The main constraints in adopting bundled climate-smart solutions are linked to several factors, including the high cost of inputs, lack of credit, limited access to agricultural and climate information services, institutional support, socioeconomic status, and risk-taking behavior (Figure 1). According to Mukherjee et al. (2017) and Chibowa et al. (2020), index insurance can be bundled differently. The study identifies three different ways—(i) credit bundling, (ii) input bundling, and (iii) contract farming and insurance bundling. In credit bundling, loans/credit to farmers are bundled with agricultural insurance. Bundling index-based insurance with credit may help to address these challenges by providing a means to pre-finance or defer premium payments, thus facilitating access to credit (Giné and Yang, 2009; Liu and Myers, 2012). At the same time, insurance can reduce the lending risk of farmers and the credit facility, which, in turn, is expected to increase loan amounts and potentially expand credit to currently underserved farmers (Meyer et al., 2017). Microfinance institutions (MFI) or cooperatives through which farmers access credit and other agricultural services or inputs may also strengthen the take-up of weather index insurance (WII)—which has remained below expectations in many cases—because they can increase access to insurance in rural areas and build on preexisting relationships with farmers. In addition, MFIs and cooperatives can help to reduce administrative costs and thus support the scaling up of WII (Meyer et al., 2017).
Figure 1. Challenges of bundled climate-smart practices among smallholder farmers in enhancing adaptive capacity. Source: Authors.
There are several examples of bundling of index insurances with inputs—(i) subsidized weather index insurance with input loans for fertilizers and modern seed for smallholder farmers in rural Ethiopia (McIntosh et al., 2013; Ahmed et al., 2020); (ii) multi-peril insurance at zero cost if farmers purchase improved seeds (any one of maize, sorghum, soya, and sunflower) (Bulte et al., 2020); and (iii) bundled insurance with seeds (Kilimi Salama in Kenya) and fertilizers (IFFCO Tokio in India). In both the credit- and input-linked insurance bundles, the bundling can take two different forms. In the first scenario, the insurance product is compulsory for the smallholders when using a complementary product or service, such as inputs or credit, and the insurance component may be invisible to the farmer. The second scenario is where the insurance product is voluntary and presented as part of a menu or solution options (Agbon, 2020).
The contract farming and insurance bundling include two sub-forms—buyer collaboration and information and support services. In the buyer collaboration model, the end buyer of the contract (i.e., the company buying the farm output) offers bundled insurance along with loans to the farmers. The insurance in such cases is mandatory along with the credit or inputs (e.g., NWK Agri Services in Zambia). In the second model, the contract buyer offers insurance voluntarily. The insurance provides a set of services that include support services (like weather advisory) to maximize the value proposition to the farmer (example of PepsiCo in India procuring potato from contract farmers). There are other innovations linked to index insurance, for example, linkage with safety nets, where employment opportunities are created for resource-poor farmers to pay for insurance premiums. Farmers are engaged in resource management such as tree plantation, and through their investment in labor, they obtain insurance certificates to guarantee payouts in the event of drought affecting crop production (Brans et al., 2010; OA, 2010). This approach has been tested in northern Ethiopia by Oxfam America, private insurers, cooperative unions/micro-financial institutions, farmers, and government agencies that run the current Productive Safety Net Programs (PSNP) in the country (Tadesse et al., 2015). Besides being bundled with related value-added services, such as agronomic advisory and input loans, many index insurance services have been cross-sold with other types of insurance, such as health or life insurance. This approach provides farmers with comprehensive coverage against a range of shocks and allows providers to cross-subsidize their index insurance services and even educate farmers through trusted partners (Raithatha and Priebe, 2020). For example, Econet's ZFU EcoFarmer Combo provides services to farmers of the Zimbabwe Farmers' Union.
Earth observation plays a crucial role in the application of crop insurance by providing accurate and timely data to assess crop health, monitor agricultural conditions, and facilitate insurance claims processing. Earth observation satellites, equipped with remote sensing instruments, can capture high-resolution imagery of agricultural fields at various intervals throughout the growing season. These data help insurance companies and agricultural experts monitor crop health, detect anomalies, and estimate potential yields (IFAD, 2017b; Omia et al., 2023). By comparing current conditions to historical data, they can assess the impact of weather events and other factors on crop performance (Schauberger et al., 2020).
Earth observation satellites equipped with remote sensing sensors, such as optical and radar instruments, can monitor crops from space (Omia et al., 2023). The increased availability of open-source data to seamlessly access historical to current satellite images can help insurers to track vegetation health and growth patterns and identify potential risks such as pest infestations, diseases, or drought stress. Such regular monitoring of the insured areas can help insurers make informed decisions about insurance coverage and identify areas requiring additional support. In recent years, the advanced capabilities of earth observation data, combined with advanced data analytics and machine learning algorithms, can be used to estimate crop yields accurately (Elavarasan et al., 2018; Virnodkar et al., 2020). This information is essential for setting appropriate insurance premium rates and determining insurance payouts in the event of crop losses. Accurate yield estimation reduces the risk of overpaying or underpaying claims.
Furthermore, earth observation data are regularly used to study the historical to current weather patterns and identify climate hotspots for insurers to develop tailored parametric index insurance products to ensure wider adoption of risk transfer mechanisms to changing climate conditions. The study highlights key satellite data products, for example, satellite-derived products such as Climate Hazards Group Infrared Precipitation with Stations (CHIRPS), Global Precipitation Measurement (GPM), Soil Moisture and Ocean Salinity (SMOS), Soil Moisture Active Passive (SMAP), and Normalized Difference Vegetation Index (NDVI)/Enhanced Vegetation Index (EVI), and high-resolution data from the European Space Agency (ESA) Sentinel-1 and Sentinel-2 and the United States Geological Survey (USGS) Landsat will be used for the monitoring and claim assessment. Index insurance is increasingly being recognized as an innovative approach to eliminating issues in the existing indemnity-based approach. By harnessing the power of earth observation and combining it with advanced analytics and data-driven methodologies, crop insurance companies can enhance their risk management capabilities, provide timely and accurate insurance services to farmers, and contribute to the resilience of agriculture in the face of climate change and other environmental challenges.
Sri Lanka is a tropical island nation and is becoming increasingly vulnerable to climate change, which significantly impacts the country's food production, people's livelihood, and natural ecosystem. As Sri Lanka is located in the Indian Ocean south of India, it receives rainfall through four monsoon seasons, such as First Inter Monsoon (FIM—March to April), Southwestern Monsoon (SWM—May to September), Second Inter Monsoon (SIM—October to November), and Northeastern Monsoon (NEM—December to February). There are two major cropping seasons, Yala (May to August) and Maha (September to March), which coincide with the four rainy seasons, and crop damage is regularly reported due to frequent droughts and floods during the south-west and north-east monsoon. Rainfall distribution of the country is the central pillar of agroecological classification, and there are three climatic zones—wet, dry, and intermediate zones (Figure 2). These zones are further classified based on the elevation of land, soil type, and agricultural conditions, resulting in 24 agroecological regions (Panabokke, 1996).
Figure 2. Map shows study sites piloted during three (2020–2021, 2021–2022 Maha, and 2021 Yala) cropping seasons. Source: Authors.
The evaluation of BISCA was first tested through a pilot study conducted in the north-central province during the Maha season. The pilot study included maize-growing farmers selected through purposive sampling from the Dunumadalawa Gramaniladari Division (GND) and Galenbindunuwewa Divisional Secretariat Division (DSD) in the Anuradhapura District. As the sampling was non-probabilistic, care was taken to reduce bias through stratification, proper consultation, and benchmarking. Sri Lanka is administratively divided into provinces, districts, divisional districts, and GNDs at the lowest level. The decision of the sample size was mainly governed by the presence of marginal farmers (cultivating < 0.25 acres) estimated through a stratification approach. The other benchmark apart from being a marginal farmer that was used for selection was agricultural income.
In Anuradhapura district, Galenbindunuwewa Divisional District was selected as maize cultivation is the main livelihood in this division, where more than 55,000 farmers cultivate an area of over 10,000 acres (Hiru News, 2021). Smallholder farmers (farmers cultivating 0.25 acre to 20 acres) are approximately 11,809 (Economic Census, 2013-14). According to the Economic Census (2013-14), 46.1% (5,432) are small farmers operating on land < 0.25 acres. The distribution of these marginal farmers across the Divisional District was unavailable from secondary data sources; hence, crop production data at different GNDs were used. Grama Niladhari Divisions Statistics (2020) for Anuradhapura district indicates that out of the 41 GN divisions, 38 GN divisions have crop cultivation as an economic activity. This implies that, on average, there are 143 farmers engaged in crop cultivation. Therefore, given this background, 100 farmers for controlled evaluation were considered an appropriate sample size from the Dunumadalawa Gramaniladari Division, where most farmers primarily relied on rain for maize cultivation.
The 100 marginal farmers were selected based on the information provided by District Agricultural Officers (DAOs) and consultation with farmers' organizations at the local level identified by the DAOs. The main criterion for selecting these 100 small farmers was their agricultural income. The Household Income and Expenditure survey (2012-13) shows that the mean household income from agriculture was LKR 5,856. As this income is not representative of the present situation, this household income was inflated with an appropriate deflator to reach a representative income. The Economic Statistics of Sri Lanka (2022) shows that between 2016 and 2022, the price deflator had doubled. The benchmark was, therefore, set to LKR 12,000 as monthly agricultural income to select the farmers in the pilot study and accommodate the inflation between the periods.
Following the successful implementation of the pilot study, the BICSA program was scaled to dry zones in the five districts of the north and eastern parts of the country—Ampara, Anuradhapura, Monaragala, Kurunegala, and Vavuniya (Figure 2). These districts have similar climatic variabilities, and smallholder farmers face severe challenges in sustaining a stable agricultural income. The particular sites within the districts were selected using the stratification process through consultation with the DAOs, and farmers were identified by the farmers' organization through benchmarking (with agricultural income) during the pilot study. The second (1,321 farmers for the Yala season 2021) and third phase (1,000 farmers for the Maha season 2021–2022) of implementation covered farmers engaged in maize and rice cultivation. The distribution of the farmers across the districts is shown in Tables 6, 7.
The primary focus of the business model was to provide an integrated one-stop-shop service delivery model for smallholder farmers to promote climate-smart bundled solutions that accelerate, de-risk, and scale services and business models with direct climate-resilient impacts for transformative adaptation. Hence, the insurance was bundled with climate-resilient seeds for smallholder adaptation, advisory based on climate and weather information, and a package of practices on agronomy, fertilizers, and water management. The model ensured that it is digitally enabled (using satellite data and mobile networks) and indicates the potential for scaling up digital platform-based bundled insurance products in the country.
In this section, we present our main results, focusing on piloting bundled climate insurance solutions among smallholder farmers offered with drought-resistant crop varieties, weather index insurance, and crop advisories to evaluate the future scaling potential of bundled products.
The scaling of the BICSA covering maize and rice farmers with the four components of the bundled solutions resulted in the provision of approximately 2,572,538.22 million rupees (USD 13,891) as compensation to eligible farmers based on the deficit and excess rainfall triggers across the pilot districts covering the 2021 Yala and 2021–2022 Maha seasons (Tables 6, 7). Our findings of comprehensive risk management are consistent with other case studies evaluated in India, Kenya and Zambia on the benefits of bundling with climate insurance products, such as weather-based insurance combined with agricultural inputs, which can significantly enhance resilience and reduce the vulnerability of farmers to climate-induced shocks (Mukherjee et al., 2017; Bulte et al., 2020). The study also demonstrates that bundling these solutions helps farmers make informed decisions based on weather forecasts and insurance coverage, leading to improved crop management and reduced climate risks.
The data section on developing weather index insurance using precipitation data from CHIRPS provides rainfall amounts and distribution since 1981. The study gathers ground-based weather data from meteorological stations in the insured areas. These data serve as ground truth for calibrating and validating satellite-based observations (Table 1). Insurers use these data to set rainfall thresholds that trigger insurance payouts for drought or excess rainfall events. The study uses earth observation data from USGS Landsat and ESA Sentinel-2 images to provide insights into crop health and growth based on the Normalized Difference Vegetation Index (NDVI) to evaluate the historical insurance claim. NDVI values are often used as one of the indices triggering insurance payouts in agriculture-related index insurance products. The study developed a survey form using the Open Data Kit (ODK) for efficient enrollment of weather index insurance products among farmers.
The bundle intends to address both the challenges of farmers' adoption of climate-smart agriculture and strengthening partnerships among public–private actors, namely, seed companies, climate information services, and insurance companies. BICSA supports (1) improving access to climate-resilient seeds of maize and rice varieties; (2) improving farmers' access to climate-smart information services; (3) promoting the use of agronomic practices (e.g., tillage, water management advisories, and pest and weed management); and (4) access to weather index insurance to mitigate financial losses from floods and drought (Figure 3).
Figure 3. Components of climate-smart bundled solutions. Source: https://ccafs.cgiar.org/news/bundled-agricultural-insurance-solutions-climatarians-gambit.
The most important thing for cultivation is to purchase seeds that give a good yield in any climatic condition, and because of adverse acclimate variability experienced in recent times, it is more important for the farmers to use seeds that are resistant to climate. As part of BICSA, we provide climate-resilient seeds (paddy and maize) to farmers for better yields even during floods and droughts. As the BICSA pilot project was conducted to cover different climatic zones, the seed varieties of rice crops, namely, BG 352, BG 300, BG 358, and AT 362, were distributed to the farmers. Hybrid Maize—JET 999 was provided to the farmers as climate-resilient seeds for maize farmers.
Climate and agronomical advisories are more important for farmers to manage their crops safely through the efficient use of water and fertilizers. To provide the climate advisories, freely available climate forecasts were used 5 days in advance. Climate forecast information on rainfall, temperature, and wind speed is provided to farmers twice a week through SMS. Table 2 shows how the forecasted rainfall data are converted into information. For agronomical advisory services, from the beginning of cultivation to the time of harvest, the agricultural specialist works closely with the farmers. The information required for their agricultural problems, such as fertilizer use, herbicide, and pesticide application time, is provided to the farmers each week.
The precipitation data required for the development of the WII product were obtained by CHIRPS rainfall products and extracted from 30 years of daily precipitation data pertaining to the study area covered by the CHIRPS pixels. WII product was designed by insurance companies using the daily rainfall data given below.
Rainfall was used to design our Meteorological Insurance (WII) products, and a single WII was designed to cover both droughts and floods, which were caused by extreme wet and dry conditions. The area considered for WII product implementation uses monthly rainfall data for approximately 30 years of historical rainfall data, which is used to determine the trigger values for drought and flood for each month of the particular season (Yala and Maha). Thus, the claim is determined for each millimeter of precipitation that increases or decreases from the trigger value determined for drought and flood. For example, as shown in Table 3, the WII trigger values for drought and flood in Nainamadu village in Vavuniya district in May, June, and July are 300 mm, 200 mm, and 200 mm (flood) and 20 mm, 2 mm, and 15 mm (drought), respectively. The increase and decrease of each millimeter of rainfall relative to the trigger values is calculated relative to the maximum payout of the maximum insurance coverage. The example shown in Table 3 pays 382.50 rupees per millimeter reduction of rainfall in May during the drought in Nainamadu, which is as high as 1,377 rupees per millimeter in June because the gap between the trigger and stop-loss is approximately only 2 mm.
Table 3. Weather index insurance design for excess and deficit rainfall among pilot districts in Sri Lanka.
Climate and agronomy advisories were provided to the enrolled farmers via SMS throughout two consecutive seasons (Yala 2021 and Maha 2021–2022) (Table 4). The climate advisories on short-term weather forecast information (rainfall, temperature, and wind speed) were provided 5 days in advance to the farmers weekly (Monday and Thursday) in Sinhala and Tamil. On the other hand, agronomy advisories were issued biweekly (on Thursdays). A focus group discussion was carried out to understand the need for specific information that was to be incorporated into the advisory. A total of 17,161 for the Yala 2021 pilot and 23,100 advisories for the Maha season 2021/2022 were issued in the two consecutive seasons, respectively.
The bundled insurance was implemented as a micro-model (Figure 4) where the implementing partners, namely, (i) International Water Management Institute (IWMI); (ii) Sanasa General Insurance Company Limited, Sri Lanka; (iii) Department of Agrarian Development, Sri Lanka; and (iv) CIC Agri Businesses (Pvt) Ltd., Sri Lanka, co-designed and co-implemented across pilot districts in Sri Lanka. The program was an insurer-led model with the insurance company designing the insurance product, underwriting, and taking care of regulatory compliance. IWMI provided analysis of remote sensing and weather data (historical rainfall and forecasts), which formed inputs for the weather index. The insurance contract design was further tested and adjusted before the insurance staff, agents, and other delivery channels were trained. Table 5 provides a summary of the roles and responsibilities of partners for scaling BICSA solutions.
Table 7. District-wise summary of farmer enrollment and insurance claim for the 2021–2022 Maha season.
In the 2021 Yala season, insurance was provided with a 2,500 LKR premium for all the districts, and at the end of the season, the farmers received insurance claims covering all the districts, but in the 2021–2022 Maha season, the farmers in Monaragala and Vavuniya districts did not receive the insurance claims because the area did not overcome the insurance triggers kept for both drought and flood disasters. The paddy seeds provided to the farmers during the 2021–2022 Maha season are BG 352, BG 300, BG 358, and AT 362. Hybrid Maize—JET 999 was provided as climate-resilient seeds. In terms of insurance claims received by farmers in both seasons, the maximum value of 2,651.17 LKR was received by the farmers of Vavuniya in the 2021 Yala season, while the farmers of Ampara received the minimum insurance claim of 850 LKR. However, it is evident that there has been significant insurance coverage for farmers in both seasons.
The above figure indicates that farmers' enrollment in the Maha season has gained momentum from the previous season (Figure 5). Farmer leaders informed that farmers enrolled in the previous season had received claims which induced other farmers to enroll in the following season. Following the implementation of the bundled insurance, feedback from the farmers enrolled in the program was collected to better understand the areas of improvement. Most of the farmers appreciated the initiation of the weather advisories as they depend on rain for cultivation (program implemented in intermediate and dry agro-climatic zones), and the forecasts had helped them in planning ahead. For example, one of the registered farmers in Monaragala district mentioned,
“…I had to harvest the groundnuts and was planning to pump water from a great distance because of the lack of rain. But according to the weather forecast you gave; it was said that it would rain in the coming days. So I delayed the harvest of the groundnuts for about 2 days. It actually rained as informed by the climate forecast. I did not need to pump water, so I was able to save a lot of money” (20 April, 2021).
Another farmer in Ampara district indicated, “…We look forward to participating in your program during the Maha season. About 385 farmers in our village joined the Yala season program, and it would be great if they could be involved in the Maha season as well.”
Figure 5. Increase in farmers' enrollment in different villages during the Maha season. Source: Authors.
The farmers were of the view that weather advisories should increase the forecast period and the precision for improved decision-making process.
“…and it would be very good if you could give us information by increasing the forecast period. The weather forecast you provide is often correct, but as far as I can remember, there were times when it went wrong” (a farmer from Ampara district, on 20 April 2021).
“…it would be great if we could increase the number of frames for next season…” (a farmer from Kurunegala district, on 15 March 2021).
“…In my experience, I say weather and agricultural advice are really important for the success of cultivation. I think it would be better if I could provide weather forecast for more than 5 days” (a farmer from Monaragala district, on 18 December 2021).
The farmers also mentioned that mobile services should improve as it is the medium to access the services in their respective areas. Farmers in Ampara stated, “…we need a better telephone network. Otherwise, we will not receive the text messages you send…” Along with network improvement, proper enrollment was also cited as an essential consideration during the registration for the advisories. One of the farmers in Kurunegala district mentioned, “…most farmers in our area have not received that advisory SMS….”
The enthusiasm from the farmers and the farmer leaders on the weather advisories and their feedback that the logistics of the registration and the mobile networks need improvement implies a potential uptake of the bundled insurance.
Insurers and technical service providers1 (TSP) are primary leaders of the index insurance value chain. In the insurer-led model, insurance companies provide the service. The insurer designs and underwrites the product. The insurer model can include partners such as aggregators2/mobile money providers or TSPs. Aggregators are usually involved in targeting potential customers and marketing the service. TSPs are sometimes included in the partnership to provide specialist assistance in service design and monitoring the index trigger. Premium payments and claim payouts are carried out by aggregators either through cash-based collection or mobile money where available. In some cases, TSPs are well-equipped to design the product, but as they do not have insurance licenses, they require an insurance partner. TSP-led services target farmers directly or through distribution partners (such as mobile network-based operators (MNOs), input suppliers/aggregators, microfinance institutions (MFIs), and non-governmental organizations (NGOs).
One of the extensions suitable for scaling up these models is the inclusion of a “catalytic” micro-agent downstream of the value chain. The “farmer leaders” or farmers with more influence within the community can be considered for extending the service chain to the last mile. The uptake of agricultural insurance is arguably low due to different confounding factors like lack of awareness about insurance products, low financial literacy of the farmers, and low penetration of the insurance companies or other financial services in the rural areas. Even though farmers are aware of insurance, insufficient knowledge and understanding of financial services and their mechanisms imply that farmers might not immediately trust the service provider. For farmers aware of the insurance, the likeliness of using the insurance service increases with their understanding of the modality of the service and the value it provides. Traditionally, insurers take the help of insurance agents at the local level to reach the farmers. Using the farmer leaders within the community helps gain the trust of smallholder farmers and overcome the problem of lower financial literacy (Rosenstock et al., 2020). Accordingly, in such communities where influential farmers exist, training them with insurance products by the insurers for enrolling other farmers can be a strategy for scaling. The inclusion of these influential farmers in the business model can be increased through the identification of farmers willing to take up rural-level enterprises, and the leader of the value chain (insurer/TSP/MNO) needs to incentivize such operations by enrolling other farmers (Greatrex et al., 2015; Long et al., 2017; Prager et al., 2021). Over time and with the increasing expertise of such enterprises, delivery of bundled solutions can be included.
Compilation of different datasets through the digitized platform can be used for scaling up insurance utilizing different business models. One such example is the utilization of mobile-based technology for reaching customers. The MNO business model has gained prominence in many low- and middle-income countries. Apart from the insurer- or the TSP-led model, the MNO-led model is an emerging approach where the existing assets of the mobile company are used to reach the subscribed customers under the network. For example, Econet in Zimbabwe and Safaricom in Kenya are MNO-led insurances that use their assets to offer index insurance to farmers. Both these MNOs use their digital platforms to provide service to smallholder farmers. In this model, the technical functions (such as regulatory compliance, underwriting policy administration, index trigger monitoring, and claims management) usually remain with the insurer/TSP. This might hinder the MNO from leading the value chain, especially in situations where the insurance sector is not competitive, and there exists opportunities for insurance companies to grow their customer base and discontinue the contract (partnership with the MNO).
Utilizing MNOs to register and locate farmers and using mobile money to collect premiums and payout claims can be possible strategies for upscaling. Precise locations of farmers are critical to increase scalability and reduce basis risk for index insurance. Automating the collection of user locations through mobile networks (Global Positioning System) can overcome this challenge. In case automated service cannot be used, the mobile networks of insurance agents can be used for face-to-face registering of the users. For bundling insurance with climate and crop advisory services, mobile networks [usually through Unstructured Supplementary Service Data (USSD), Short Message Service (SMS), and Interactive Voice Response (IVR)] offer valuable opportunities. However, in MNO-led models, call center crop and climate advisories customized to the needs of the farmer (regarding special agro-climatic zones) can improve the demand for insurance products (Long et al., 2017; Groot et al., 2019; Prager et al., 2021). Additionally, such call centers can be targeted toward educating customers about the need for insurance, any disputes related to the insurance, and providing solutions on call for effective service delivery. Using digital platforms reduces the transaction costs for enrollment and has a huge potential in Sri Lanka. It has been estimated by Rambukwella et al. (2020) that in Sri Lanka, approximately a company spends LKR 20 on administration costs to earn a premium of LKR 100. Digital innovation for index insurance can reduce operation costs by 30% in Sri Lanka.
The scaling up of bundled insurance depends on different institutions playing different roles. The following Table 8 presents recommendations on the roles and responsibilities of different stakeholders.
The scaling program should have an approach that embeds targeted outreach, inclusivity, and capacity building of farmers. This would ensure that the program reaches smallholder farmers, women farmers, and marginalized communities often most vulnerable to climate impacts (Greatrex et al., 2015). Community leaders, agricultural extension agents, and farmers' organizations should be included in outreach campaigns to increase program awareness and participation. Similarly, the agricultural department, through the extension agents, should provide comprehensive training programs for farmers that encompass climate-smart agricultural practices, proper use of drought-resistant seeds, and understanding of index insurance. These training sessions should focus on sustainable farming techniques, efficient water management, and the benefits of using climate-resilient seeds.
Climate change induces more severe extreme weather events by altering the frequency, timing, intensity, and duration. Floods and droughts are the two most devastating consequences of the climate crisis, with a huge impact on agriculture. Smallholder farmers are most vulnerable and face a range of shocks and challenges beyond their control. These shocks have a drastic impact and affect their incomes and livelihoods. Extreme climate events are often coupled with unexpected agricultural events, such as market and price fluctuations or pest and disease infestations, and non-agricultural events, like health problems, increasing the helplessness of the smallholders. Catering to these challenges, national, regional, and local data must be integrated to build instruments that increase the preparedness for floods and droughts. Index-based agricultural insurance is one of the innovative approaches that integrate different information and is perceived to help tackle the challenges of extreme weather events. With the help of remote sensing, historical weather datasets, spatial maps of flooding extent, and socioeconomic data, hydrodynamic models can be prepared, indicating spatiotemporal variability of flood parameters. In addition to index insurance, bundling the insurance credit facilities, inputs (seeds, fertilizers), and crop and weather services is gaining importance as it provides holistic mitigation measures. The value addition through agronomic services, agricultural inputs (seeds, fertilizers), and credit helps smallholders and supports scaling up of the insurance services, reducing the associated risks of agriculture.
The present study based in Sri Lanka is an example of such an implementation of bundled insurance. The bundled insurance was implemented in five districts—Ampara, Anuradhapura, Monaragala, Kurunegala, and Vavuniya. Due to the increasing impact of climate change on crops in the dry zone of Sri Lanka, the dry zone was covered during the implementation and upscaling of the insurance products. The business model implemented is insurer-led, whereby seeds and climate advisory were bundled with the insurance product. The implementation included 1,321 farmers for the Yala season 2021 and 1,000 farmers for the Maha season 2021–2022, with approximately 30,000 weather advisories to the farmers during this period. Following the implementation of the bundled insurance, a follow-up on the assistance received by the farmers from the project was conducted. The feedback from the farmers indicated a willingness to take up bundled insurance in future. It has also been realized through the implementation that partnerships are crucial driving factors for the growth of index insurance. This includes insurers, TSPs, aggregators, extension agencies, agro-dealers, seed companies, and government agencies.
Based on this experience, the study recommends different mechanisms for scaling up the market for insurance products. This includes targeting farmers' uptake through community-based influential farmers willing to establish rural enterprises for enrolling farmers with insurance products. The index insurance value chain for agriculture requires the expertise of different agents in delivering the product. Insurers are required to design the product, regulatory compliance, and underwriting; TSPs provide data for designing and monitoring the trigger, and aggregators use their reach to farmers and cooperatives to promote and market the product. With digitization across the value chain, MNOs increasingly become potential competitors for upscaling bundled insurance. MNOs can locate farmers either through GPS location-based systems or through the use of mobile networks of rural agents registering farmers. Through MNOs, mobile cash is a facility that can be targeted for premium payment and claims settlement. The MNOs can also introduce call center facilities dedicated to weather and crop facilities and provide solutions on insurance premiums, claims, and related procedures. To sustain the process, it is envisioned that MNOs need to formulate business-to-business (B2B) partnerships to deliver index insurance services at scale as B2B allows sell of bundled value-added services.
The original contributions presented in the study are included in the article/supplementary material, further inquiries can be directed to the corresponding author.
GA conceptualized the study and contributed to overall project coordination. SG and NA provided technical backstopping on data analysis and imagery. GA, AT, and NA drafted the manuscript. GA was involved in funds sourcing and NA was involved in field activity arrangements. All authors contributed to the article and approved the submitted version.
This study was originally funded by the CGIAR Research Program on Climate Change, Agriculture, and Food Security (CCAFS). The authors would like to thank all funders who support the CGIAR Initiative on Climate Resilience research through their contributions to the CGIAR Trust Fund: www.cgiar.org/funders.
The authors would like to thank officials of Sanasa General Insurance Pvt. Ltd., the Department of Agrarian Development, and CIC Seed Pvt. Ltd., for their valuable support in implementing the BICSA program in Sri Lanka. We would like to thank all funders who supported this research through their contributions to the CGIAR Trust Fund.
The authors declare that the research was conducted in the absence of any commercial or financial relationships that could be construed as a potential conflict of interest.
All claims expressed in this article are solely those of the authors and do not necessarily represent those of their affiliated organizations, or those of the publisher, the editors and the reviewers. Any product that may be evaluated in this article, or claim that may be made by its manufacturer, is not guaranteed or endorsed by the publisher.
1. ^Companies that provide administrative and technical input for technical services which might include the insurer's responsibility of designing the insurance, with the exception of ensuring regulatory compliance and underwriting. This is primarily due to the reason that the TSPs lack insurance licenses.
2. ^An aggregator here refers to companies involved in agribusiness, seed manufacturing.
ADB (2013). The rise of natural disasters in Asia and the Pacific: Learning from ADB's experience. Mandaluyong City, Philippines: Asian Development Bank. Available online at: https://www.adb.org/sites/default/files/evaluation-document/36114/files/rise-natural-disasters-asia-pacific.pdf (accessed May 20, 2022).
ADB (2021). Disaster Resilience in Asia: A Special Supplement of Asia's Journey to Prosperity: Policy, Market and Technology Over 50 Years. Manila: Asian Development Bank. Available online at: https://www.adb.org/sites/default/files/publication/703556/disaster-resilience-asia.pdf (accessed March 23, 2023).
Afriyie-Kraft, L., Zabel, A., and Damnyag, L. (2020). Index-based weather insurance for perennial crops: a case study on insurance supply and demand for cocoa farmers in Ghana. World Dev. Perspect. 20, 100237. doi: 10.1016/j.wdp.2020.100237
Agbon, A. (2020). Bundling of insurance with agricultural inputs: strategy for enhancing access and distribution. Presentation at NIRSAL webinar. Theme: Deepening agricultural insurance penetration in Nigeria: opportunities and strategies. Available online at: https://www.corprojectsng.com/corprojects/wp-content/uploads/2020/06/Bundling-of-Insurance-with-Agricultural-Inputs-June-18th-2020.pdf (accessed June 5, 2022).
Aheeyar, M., Samarakoon, K., and de Silva, S. (2021). Bundled weather index insurance pilot for drought-affected areas in Sri Lanka: reaching marginal farmers. IWMI Working Paper 201, International Water Management Institute (IWMI). doi: 10.5337/2021.233
Ahmed, S., Gommes, R., McIntosh, C., and Sarris, A. (2020). The impact of commercial rainfall index insurance: Experimental evidence from Ethiopia. Am. J. Agric. Econ. 102, 1154–1176. doi: 10.1002/ajae.12029
Ahmed, T. (2013). S coping report: Current status of index-based insurance in Bangladesh. WorldFish, Penang, Malaysia Project Report. (38). Available online at: http://icccad.net/wp-content/uploads/2015/12/Scoping-Report-Current-Status-of-IBI-in-Bangladesh.pdf (accessed May 12, 2022).
Amarnath, G. (2020). Mainstreaming technology provides key solutions for disaster risk mitigation. Available online at: https://www.iwmi.cgiar.org/2020/03/mainstreaming-technology-provides-key-solutions-for-disaster-risk-mitigation/ (accessed April 15, 2022).
Amarnath, G., Malik, R. P. S., and Taron, A. (2021). Scaling up Index-based Flood Insurance (IBFI) for agricultural resilience and flood-proofing livelihoods in developing countries. Colombo, Sri Lanka: International Water Management Institute (IWMI). 68p. (IWMI Research Report 180). doi: 10.5337/2021.213
Amarnath, G., Matheswaran, K., Alahacoon, N., Malik, R. P. S., and Sikka, A. (2017). Investing in disaster resilience: Risk transfer through flood insurance in South Asia. Paper presented at the international biennial conference, World Bosai Forum, Japan.
Amarnath, G., and Sikka, A. (2018). “Satellite data offers new hope for flood-stricken farmers in India,” in Asia Insurance Review 80–82.
Brans, M. V., Tadesse, M., and Takama, T. (2010). “Community-based solutions to the climate crisis in Ethiopia,” in Climate Change Adaptation and International Development. Making Development Cooperation More Effective, eds. R. Fujikura, M. Kawanishi (Earthscan, UK: Routledge).
Browder, G. A., Nunez Sanchez, B., Jongman, N., Engle, E., van Beek, M., Castera Errea, et al. (2021). An EPIC Response : Innovative Governance for Flood and Drought Risk Management. Washington, DC: World Bank. Available online at: https://openknowledge.worldbank.org/handle/10986/35754 (accessed April 2, 2023).
Bryla, E., and Syroka, J. (2007). Developing index-based insurance for agriculture in developing countries. United Nations Sustainable Development Innovation Briefs (2). New York: United Nations Department of Economic and Social Affairs. https://www.un.org/esa/sustdev/publications/innovationbriefs/no2.pdf (accessed May 20, 2022).
Bucheli, J., Dalhaus, T., and Finger, R. (2021). The optimal drought index for designing weather index insurance. Eur. Rev. Agric. Econ. 48, 573–597. doi: 10.1093/erae/jbaa014
Bulte, E., Cecchi, F., Lensink, R., Marr, A., and van Asseldonk, M. (2020). Does bundling crop insurance with certified seeds crowd-in investments? Experimental evidence from Kenya. J. Econ. Behav. Organ. 180, 744–757. doi: 10.1016/j.jebo.2019.07.006
Carter, M., Janvry, D. A., Sadoulet, E., and Sarris, A. (2014). Index-based weather insurance for developing countries: A review of evidence and a set of propositions for up-scaling. TRB Special Report 312: Transportation Investments in Response to Economic Downturns. Washington, D.C.
Chibowa, T. K., Synnevag, G., Maonga, B., and Mainje, M. (2020). “Gender differentiation in the adoption of climate smart agriculture technologies and level of adaptive capacity to climate change in Malawi,” in Climate impacts on agricultural and natural resource sustainability in Africa, eds. B. R. Singh, A. Safalaoh, A. A. Nyambilila, L. O. Eik, B. K. Sitaula, and R. Lal (Cham: Springer International Publishing), 507–26. doi: 10.1007/978-3-030-37537-9_29
CRED (2020). Natural Disasters 2019. Brussels: CRED. Available online at: https://emdat.be/sites/default/files/adsr_2019.pdf (accessed March 23, 2023).
Delavallade, C., Dizon, F., Hill, R. V., and Petraud, J. P. (2015). Managing risk with insurance and savings: Experimental evidence for male and female farm managers in West Africa. IFPRI Discussion Paper. Washington, DC: International Food Policy Research Institute (IFPRI). Available online at: https://www.ifpri.org/publication/managing-risk-insurance-and-savings-experimental-evidence-male-and-female-farmmanagers (accessed April 2, 2022).
Economic Census (2013-14). Sri Lanka Statistical Reports. Department of Census and Statistics. Available online at: http://www.statistics.gov.lk/Agriculture/StaticalInformation/new/EconomicCensus2013-14-AgricuturalEnumeration-BasicReports-AnuradhapuraDistrict (accessed April 12, 2021).
Economic Statistics of Sri Lanka (2022). Department of Census and Statistics, Ministry of Finance, Economics Stabilization and National Policies. Available online at: http://www.statistics.gov.lk/Publication/Economic-Statistics-2022 (accessed May 14, 2023).
Elavarasan, D., Vincent, D. R., Sharma, V., Zomaya, A. Y., and Srinivasan, K. (2018). Forecasting yield by integrating agrarian factors and machine learning models: a survey. Computers Electron. Agricul. 155, 257–282. doi: 10.1016/j.compag.2018.10.024
Ellis, E. (2017). Farmers' willingness to pay for crop insurance: evidence from eastern Ghana. Int. J. Agric. Manage. Dev. 7, 447–463. doi: 10.18488/journal.aefr.2017.77.700.721
Giné, X., and Yang, D. (2009). Insurance, credit, and technology adoption: Field experimental evidence from Malawi. J. Dev. Econ. 89, 1–11. doi: 10.1016/j.jdeveco.2008.09.007
Grama Niladhari Divisions Statistics (2020). Anuradhapura District. Department of Census and Statistics, Ministry of Economic Policies and Plan Implementation. Available online at: http://www.statistics.gov.lk/Resource/en/Population/GND_Reports/2020/Anuradhapura.pdf (accessed April 22, 2022).
Greatrex, H., Hansen, J. W., Garvin, S., Diro, R., Blakeley, S., Le Guen, M., et al. (2015). Scaling up index insurance for smallholder farmers: Recent evidence and insights. CCAFS Report No. 14. Copenhagen, Denmark: CGIAR Research Program on Climate Change, Agriculture and Food Security (CCAFS).
Groot, A., Bolt, J., Jat, H. S., Jat, M. L., Kumar, M., Agarwal, T., et al. (2019). Business models of SMEs as a mechanism for scaling climate smart technologies: the case of Punjab, India. J. Cleaner Prod. 210, 1109–1119. doi: 10.1016/j.jclepro.2018.11.054
He, X., Pan, M., Wei, Z., Wood, E. F., and Sheffield, J. (2020). A global drought and flood catalogue from 1950 to 2016. Bull. Am. Meteorol. Soc. 101, E508–E535. doi: 10.1175/BAMS-D-18-0269.1
Hiru News (2021). Maize farmers inconvenienced without a fixed price. Available online at: https://www.hirunews.lk/english/267573/maize-farmers-inconvenienced-without-a-fixed-price-video (accessed September 5, 2022).
Household Income and Expenditure survey (2012-13). Final Report. Department of Census and Statistics. Ministry of Policy Planning Economic Affairs, Child Youth and Cultural Affairs Sri Lanka. Available online at: efaidnbmnnnibpcajpcglclefindmkaj/http://www.statistics.gov.lk/Resource/en/IncomeAndExpenditure/HouseholdIncomeandExpenditureSurvey2012-2013FinalReport.pdf (accessed May 11, 2022).
IFAD (2017a). Remote sensing for index insurance An overview of findings and lessons learned for smallholder agriculture. Available online at: https://www.ifad.org/documents/38714170/40239486/RemoteSensing_Overview_2017.pdf/3b074eff-d3c5-4d45-9e1d-a505ee9aee0f?t=1522059339000 (accessed May 5, 2022).
IFAD (2017b). Remote Sensing for Index Insurance: Findings and Lessons Learned for Smallholder Agriculture. Available online at: https://docs.wfp.org/api/documents/WFP-0000023503/download/ (accessed April 15, 2023).
IPCC (2018). “An IPCC Special Report on the impacts of global warming of 1.5°C above pre-industrial levels and related global greenhouse gas emission pathways, in the context of strengthening the global response to the threat of climate change, sustainable development, and efforts to eradicate poverty” in Global Warming of 1.5°C, eds V., Masson-Delmotte, P. Zhai, H.-O. Pörtner, D. Roberts, J. Skea, P.R. Shukla, A. Pirani, W. Moufouma-Okia, C. Péan, R. Pidcock, S. Connors, J. B. R. Matthews, Y. Chen, X. Zhou, M. I. Gomis, E. Lonnoy, T. Maycock, M. Tignor, and T. Waterfield (Intergovernmental Panel on Climate Change).
IPCC (2021). “Contribution of working group I to the sixth assessment report of the intergovernmental panel on climate change” in Climate Change 2021: The Physical Science Basis, eds V, Masson-Delmotte, P. Zhai, A. Pirani, S. L. Connors, C. Péan, S. Berger, N. Caud, Y. Chen, L. Goldfarb, M. I. Gomis, M. Huang, K. Leitzell, E. Lonnoy, J. B. R. Matthews, T. K. Maycock, T. Waterfield, O. Yelekçi, R. Yu, and B. Zhou (Cambridge; New York, NY: Cambridge University Press). doi: 10.1017/9781009157896
Liu, Y., and Myers, R. J. (2012). The dynamics of insurance demand under liquidity constraints and insurer default risk. IFPRI Discussion Papers 1174. Washington, USA, International Food Policy Research Institute.
Long, T. B., Blok, V., and Poldner, K. (2017). Business models for maximizing the diffusion of technological innovations for climate-smart agriculture. Int. Food Agribus. Manag. Rev. 20, 5–23. doi: 10.22434/IFAMR2016.0081
Malik, R. P. S., and Amarnath, G. (2021). Economics of Index-based Flood Insurance (IBFI): scenario analysis and stakeholder perspectives from South Asia. IWMI Working Paper 199. Colombo, Sri Lanka, International Water Management Institute (IWMI), 34. doi: 10.5337/2021.228
Manojkumar, M., Sreekumar, B., and Ajithkumar, G. S. (2003). Crop insurance scheme: a case study of banana farmers in Wayanad district. Discussion Paper No. 54. Thiruvananthapuram, Kerala, India: Kerala Research Programme on Local Level Development, Centre for Development Studies. Available online at: http://www.cds.ac.in/krpcds/publication/downloads/54.pdf (accessed May 5, 2022).
Matheswaran, K., Alahacoon, N., Pandey, R., and Giriraj, A. (2019). Flood risk assessment in South Asia to prioritize flood index insurance applications in Bihar, India. Geomat. Nat. Hazards Risk 10, 26–48. doi: 10.1080/19475705.2018.1500495
McIntosh, C., Sarris, A., and Papadopoulos, F. (2013). Productivity, credit, risk, and the demand for weather index insurance in smallholder agriculture in Ethiopia. Agric. Econ. 44, 399–417. doi: 10.1111/agec.12024
Meyer, R., Hazell, P., and Varangis, P. (2017). Unlocking smallholder credit: does credit-linked agricultural insurance work? International Labour Organization and International Finance Organization. Available online at: https://openknowledge.worldbank.org/handle/10986/31437 (accessed May15, 2022).
Meze-Hausken, E., Patt, A., and Fritz, A. (2009). Reducing climate risk for micro-insurance providers in Africa: A case study of Ethiopia. Global Environ. Change. 19, 66–73. doi: 10.1016/j.gloenvcha.2008.09.001
Mukherjee, P., Pandey, M., and Prashad, P. (2017). Bundling to Make Agriculture Insurance Work. Geneva, Switzerland: International Labour Organization. Available online: http://www.impactinsurance.org/publications/mp47 (accessed April 15, 2022).
OA (2010). Horn of Africa Risk Transfer for Adaptation, (HARITA). HARITA progress report: January-June, 2010. Available at: http://www.oxfamamerica.org/publications/harita-progress-report-jan-jun-2010 (accessed May 25, 2022).
Omia, E., Bae, H., Park, E., Kim, M. S., Baek, I., Kabenge, I., et al. (2023). Remote sensing in field crop monitoring: a comprehensive review of sensor systems, data analyses and recent advances. Remote Sens. 15:354. doi: 10.3390/rs15020354
Panabokke, C. R. (1996). Soils and agroecological environments of Sri Lanka. Colombo, Sri Lanka: NARESA. xvi, 220 (Natural resources series no. 2).
Prager, S. D., Byandaga, L., and Kagabo, D. M. (2021). “Scaling and sustaining CIS and CSA through Bundled Business Models,” in AICCRA Info Note, 13.
Raithatha, R., and Priebe, J. (2020). Agricultural insurance for smallholder farmers: Digital innovations for scale. GSMA. Available online at: https://www.gsma.com/mobilefordevelopment/wp-content/uploads/2020/05/Agricultural_Insurance_for_Smallholder_Farmers_Digital_Innovations_for_Scale.pdf (accessed May 10, 2022).
Rambukwella, R., Vidanapathiraba, R., Champika, J., and Priyadarshana, D. (2020). Performance of weather Index (WII) Scheme in Sri Lanka. Research Report No: 231. Hector Kobbekaduwa Agrarian Research and Training Institute. Available online at: http://harti.gov.lk/images/download/reasearch_report/new1/report_no_231.pdf (accessed April 2, 2023).
Rosenstock, T. S., Lubberink, R., Gondwe, S., Manyise, T., and Dentoni, D. (2020). Inclusive and adaptive business models for climate-smart value creation. Curr. Opin. Environ. Sustain. 42, 76–81. doi: 10.1016/j.cosust.2019.12.005
Schauberger, B., Jagermeyr, J., and Gornott, C. (2020). A systematic review of local to regional yield forecasting approaches and frequently used data resources. Eur. J. Agron. 120:126153. doi: 10.1016/j.eja.2020.126153
Shahadat, H. (2013). Problems and prospects of weather index based crop insurance for rural farmers in Bangladesh. Dev. Country Stud. 3, 208–220.
Tadesse, M. A., Shiferaw, B. A., and Erenstein, O. (2015). Weather index insurance for managing drought risk in smallholder agriculture: lessons and policy implications for sub-Saharan Africa. Agric. Econ. 26, 1–21. doi: 10.1186/s40100-015-0044-3
UNU (2004). Two Billion People Vulnerable to Floods by 2050: Number Expected to Double or More in Two Generations. News Release. Tokyo, UNU. Available online at: https://archive.unu.edu/update/archive/issue32_2.htm (accessed May 25, 2022).
Virnodkar, S. S., Pachghare, V. K., Patil, V. C., and Jha, S. K. (2020). Remote sensing and machine learning for crop water stress determination in various crops: a critical review. Precision Agric. 21, 1121–1155. doi: 10.1007/s11119-020-09711-9
Keywords: climate-smart agriculture, drought-prone farmers, extension services, weather index insurance, public-private partnership, Sri Lanka
Citation: Amarnath G, Taron A, Alahacoon N and Ghosh S (2023) Bundled climate-smart agricultural solutions for smallholder farmers in Sri Lanka. Front. Sustain. Food Syst. 7:1145147. doi: 10.3389/fsufs.2023.1145147
Received: 16 January 2023; Accepted: 26 October 2023;
Published: 01 December 2023.
Edited by:
Robert Martin Rees, Scotland's Rural College, United KingdomReviewed by:
Munyaradzi Chitakira, University of South Africa, South AfricaCopyright © 2023 Amarnath, Taron, Alahacoon and Ghosh. This is an open-access article distributed under the terms of the Creative Commons Attribution License (CC BY). The use, distribution or reproduction in other forums is permitted, provided the original author(s) and the copyright owner(s) are credited and that the original publication in this journal is cited, in accordance with accepted academic practice. No use, distribution or reproduction is permitted which does not comply with these terms.
*Correspondence: Giriraj Amarnath, YS5naXJpcmFqQGNnaWFyLm9yZw==
Disclaimer: All claims expressed in this article are solely those of the authors and do not necessarily represent those of their affiliated organizations, or those of the publisher, the editors and the reviewers. Any product that may be evaluated in this article or claim that may be made by its manufacturer is not guaranteed or endorsed by the publisher.
Research integrity at Frontiers
Learn more about the work of our research integrity team to safeguard the quality of each article we publish.