- 1Department of Grassland Science, College of Animal Science, Guizhou University, Guiyang, China
- 2Key Laboratory of Animal Genetics, Breeding and Reproduction in the Plateau Mountainous Region, Ministry of Education, Guizhou University, Guiyang, China
- 3Department of Vehicle Engineering, Guizhou Technological College of Machinery and Electricity, Duyun, China
- 4Guizhou Grassland Technology Experiment and Extension Station, Guiyang, China
Alfalfa (Medicago sativa L.), an important perennial legume forage crop with high nutritional value and forage yield, is widely used in animal husbandry. However, it is very sensitive to aluminum, which severely limits its growth in acidic soils. In this study, we analyzed the genotype variation of each agronomic trait in 44 alfalfa varieties in two acidic soil environments. Then, analysis of variance (ANOVA) of the variance components was performed using the Residual Maximum Likelihood (REML). The best linear unbiased predictor analysis was used to obtain the mean trait of each variety, and the mean values were used to construct the mean matrix of varieties × traits and interaction analysis of varieties × years. The results showed that there was significant (P < 0.05) genotypic variation for each trait of the 44 varieties and the genetic diversity was abundant. The average repeatability (R value) of interannual plant height (PH), stem thickness (ST), number of branches (NS), fresh weight (FW), total fresh weight (TFW), and total dry weight (TDW) was high (0.21–0.34), whereas the genetics were relatively stable. PH, NS, FW, TFW, and dry weight (DW) were positively correlated (P < 0.01) with TDW. Six alfalfa varieties (Algonquin, Xinjiang daye, Trifecta, Vernal, WL354HQ, and Boja) with excellent TDW and TFW were identified in different years, environmental regions, and climatic altitudes. Our research results can provide suggestions and critical information regarding the future improvement and development of new alfalfa strains and varieties that are resistant to acidic soil conditions.
1. Introduction
Alfalfa (Medicago sativa L.), which has a high protein content, high grass yield, and barren tolerance, is the most widely cultivated legume forage crop in the world (Avci et al., 2013; Arshad et al., 2017) and is often called the “King of Forages” (Feng et al., 2022). Worldwide, approximately 320 million hm2 of land are used to cultivate alfalfa (Yuegao et al., 2010), which plays an important role in the development of animal husbandry. Alfalfa grows well in alkaline soils, but its yield decreases along with pH in acidic soils (Dogbatse et al., 2020). Approximately 50% of the world's potentially arable soils are acidic (Kochian et al., 2015), located predominantly in tropical, subtropical, and temperate regions (Ryan and Delhaize, 2010). China's acidic soil area is large, with a total area of ~2.03 × 108 hm2, spanning both tropical and subtropical regions in more than a dozen provinces and regions such as Guizhou, Sichuan, and Jiangxi south of the Yangtze River. Soil acidification is increasing with the use of fertilizers in agriculture and the emission of industrial waste and gases (Guo et al., 2010); in some areas, soil pH has reduced to <5.0 (Von Uexküll and Mutert, 1995; Zeng, 2000). At low pH, metallic aluminum in the soil dissolves in its toxic form and is absorbed by plants (Kinraide, 1991). The tips of plant roots are the most sensitive to aluminum toxicity, which inhibits cell division and elongation (Ciamporová, 2000, 2002). The subsoil may remain acidic despite raising the soil pH with lime, inhibiting root growth and leading to stunted plant growth and an increased susceptibility to drought (Sumner et al., 1986). This has severely limited the adoption of alfalfa in southern China.
Studying genetic diversity is crucial for the development of improved plant varieties, their selection, and exploration of species kinship. Studying plant genetic diversity based on the agronomic traits offers the advantage of being intuitive, simplicity, and practicality (Jia et al., 2019; Ambati et al., 2020). The identification, classification, and screening of plant germplasm resources by agronomic or phenotypic traits and the selection of breeding materials with excellent traits have become a common and effective research method (Kephart et al., 1990; Ta et al., 2020; Hakl et al., 2021). Many plants agronomic traits are quantitative, with a broad and complex genetic basis. Varieties with excellent traits can be screened for starting with their phenotype. However, if the relationship among traits is not clear, the selection of varieties tends to be speculative and ineffective (Yang et al., 2014). Understanding the correlations between traits and predicting them through arbitrary traits can save a lot of breeding resources and is necessary to select new varieties. Previous studies have used agronomic traits to analyze the genetic diversity of alfalfa (Ray et al., 1999; Ta et al., 2020; Hakl et al., 2021), among which stem weight, leaf dry weight, single plant dry weight, and single plant fresh weight have shown rich diversity and large coefficients of variation (Wu et al., 2018b). However, the research conclusions of these studies vary because of the differences in test materials, growth environment, and analysis methods. Talebi et al. (2011) conducted genetic diversity studies on alfalfa germplasm and found that with a wider source of materials, the genetic diversity and Shannon information index were greater and the results more meaningful.
Phenotypic expression and the observed variation in growth and development are influenced by the genotype (G) of a plant, the environment (E), and their interaction (G × E) (Zhu et al., 2009). Temporal adjustments include the effects of year-to-year and harvest time variations, where biotic and abiotic events among years or ripening times between harvests affect agricultural production and quality (Redpath et al., 2021). Dong et al. (2019) conducted a 2-year continuous evaluation of the genotypic variation, phenotype, and genotype correlations of 18 traits in 418 common vetch (Vicia sativa L.) germplasm resources and found that all traits had significant genotypic variation (P < 0.05) as well as genotype interaction (P < 0.05). Redpath et al. (2021) performed phenotypic analysis on various fruit quality-related traits in two consecutive harvests over 2 years at two sites and found that genotype is an important source of variation for most phenotypic characteristics. In addition, year × environment and genotype × year × environment interactions significantly influenced most of the phenotypic traits. Jia et al. (2022) analyzed the productivity of 10 alfalfa cultivars in the Hexi Corridor region for 3 years and found that there were yearly differences in agronomic traits and productivity between varieties. Genetic variation in key traits of other important forages and legumes has also been studied in detail, including that of ryegrass (Lolium perenne L.) (Humphreys, 1991), white clover (Trifolium repens L.) (Jahufer et al., 2002), and yellow sweet clover (Melilotus officinalis L.) (Luo et al., 2016). In recent years, alfalfa resources introduced in China have increased annually, but there are few studies on their agronomic traits and phenotypic genetic diversity in acidic soil areas (Dall'Agnol et al., 1996; Grewal and Williams, 2003; Lakić et al., 2019). Notably, these studies did not note the effects of genotype × year × environment interactions in acidic soil areas, so exploring the effects of alfalfa genotype × year × environment interactions in acidic soils is of great significance for the development of acid tolerant conditions and aluminum tolerant varieties in animal husbandry in southern China.
This study performed a genetic diversity analysis on 9 agronomic traits in 44 alfalfa varieties planted for 2 consecutive years at 2 sites. We pursued the following objectives: (1) estimating the genotype and environmental type variations of key traits, (2) clarifying the interrelationships among these traits, (3) screening for alfalfa varieties resistant to acidic soil conditions in China, and (4) provide reference data and breeding materials for the cultivation of new varieties of acid-resistant aluminum.
2. Materials and methods
2.1. Experimental sites
The experiments were performed at the experimental sites of Guizhou University in Huaxi District, Guiyang City (GY; 106° 39′ 28″ E, 26° 27′ 15″ N, 1,100 m.a.s.l.) and Beiping Village, Huaqiao Town, Shiqiao County, Tongren City (SQ; 108° 20′ 14″ E, 27° 32′ 03″ N, 614 m.a.s.l.), both in Guizhou Province. The monthly precipitation and temperature changes in the two study sites during the experimental period are shown in Figure 1. GY is located in the middle of Guizhou Province, which has a subtropical monsoon humid climate and clear plateau climate characteristics. The annual average temperature at this site is 14.80 °C, whereas the average annual precipitation is 1,347.30 mm (https://data.cma.cn/data/detail/dataCode/A.0012.0001.html, Figure 1). The vegetation primarily comprised natural weeds at an early stage of growth as no crops were planted. SQ is located in the eastern part of Guizhou Province and has a humid central subtropical monsoon climate, with sufficient sunshine and abundant rainfall, an average annual temperature of 17.24°C, and an average annual precipitation of 1,410.05 mm. Before the test, the SQ test point was corn field.
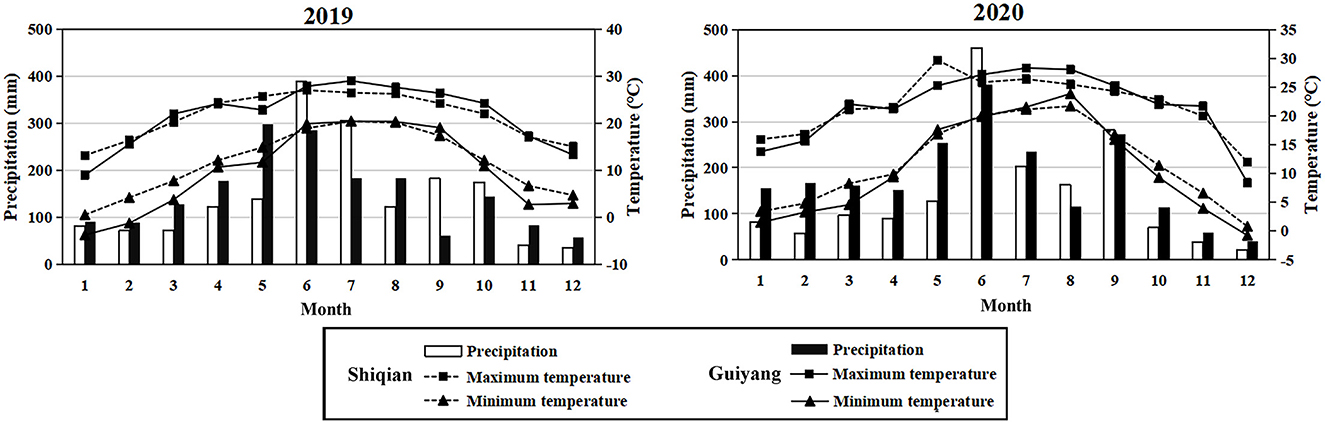
Figure 1. Monthly rainfall, minimum temperature, and maximum temperature in the study areas in 2019 and 2020.
Previously, alfalfa had not been planted at either of the two test sites. Before planting, ~20 cm of surface soil at the two test sites were collected, from which impurities were removed, air-dried, ground through a 2 mm sieve, and used to determine the basal nutrient composition and pH of the soil. Both places have yellow loam soil; SQ has a soil pH of 5.44 and GY has a soil pH of 6.14. The basic nutrients of both soils are detailed in Supplementary Table 1.
This study involved 44 alfalfa varieties, that is, 21 varieties from China; 17 from the United States; 2 each from France and Austria; and 1 each from Poland, the United Kingdom, Mexico, and Guatemala (Supplementary Table 2).
2.2. Experimental design
The experimental layout of the field trial was a randomized complete block design with three replicates, planted on September 27, 2018. The spacing between both plants and rows was 50 cm, and each replicate had 15 individual plants. During the test period, the plants grew naturally, without fertilization or artificial irrigation. When 50% of the plants of each variety in each test site flowered, they were harvested and data were recorded (harvest times are shown in Supplementary Table 3). Trait data for 10 individual plants of each variety were recorded in each replicate, presented in Table 1. Supplementary Table 1 lists the measurement methods used for each trait.
2.3. Data analysis
Using GenStat 21 (2021, VSN International Ltd.) data on the interactions of 9 agronomic traits, different years, and varieties × years of 44 alfalfa varieties cultivated in 2019 and 2020 were analyzed. First, the genotypic variations of each agronomic trait in the 44 alfalfa varieties were analyzed (Dong et al., 2019). Then, analysis of variance (ANOVA) of the variance components was performed using the Residual Maximum Likelihood (REML) (White and Hodge, 1989). The best linear unbiased predictor analysis was used to obtain the mean trait of each variety, and the mean values were used to construct the mean matrix of varieties × traits and interaction analysis of varieties × years.
REML was used to estimate the average repeatability (R) of each trait in each variety using the following equation:
where is the genotype, is the experimental error, nl is the year, and nr is the number of replicates.
The coefficient of variation (CV) indicates the degree of dispersion of each trait and was calculated based on the following equation:
where S represents the standard deviation and X represents the mean.
Phenotypic correlation (rp) between traits was analyzed using GenStat 21 (2021, VSN International Ltd.). Stochastic linear models have been used to analyze inter-varietal variation in alfalfa traits (Nguyen et al., 2016). Pattern analysis combines principal component analysis (PCA) and cluster analysis (Gabriel, 1971; Kroonenberg, 1994) to provide a graphical overview of the data matrix for each trait between different varieties, where the direction vector represents the correlations between traits. A mixed linear model analysis with different varieties as fixed effects was also performed to study the differences in traits between different varieties of alfalfa (Watson et al., 1995).
The genotype main effects and genotype × environment interaction (GGE) model (Yan et al., 2000) was used to evaluate alfalfa yields in the two sites. Analyses were performed using GenStat 21 (2021, VSN International Ltd.).
3. Results
3.1. Yield evaluation of 44 alfalfa varieties
The total dry weight (TDW) of 44 alfalfa varieties was analyzed and ranked using GGE model analysis of G × E (Table 2). The varieties with the five highest yields were, in descending order, Algonquin, Xinjiang daye, Trifecta, Vernal, and Zhongmu No. 1. The varieties with the five lowest yields, in descending order, were Longzhong, Dongmu No. 1, Longdong, CUF 101, and UC-1465.
3.2. Diversity analysis of alfalfa agronomic traits
3.2.1. Genetic diversity of agronomic traits in a single year
Genotypic ANOVA of the agronomic traits of alfalfa varieties in 2019 and 2020 exhibited significant differences in yield between varieties (P < 0.05). Except for plant height (PH) in 2019, the results showed significant (P < 0.05) genotype differences among varieties (Tables 3, 4). Additionally, the average R values of each trait were significantly different. When comparing R values in 2019, those of the stem biomass/leaf biomass ratio (RSL), stem thickness (ST), number of branches (NS), and DW were the highest, at 0.45, 0.34, 0.32 and 0.32, respectively; PH, TDW and total fresh weight (TFW) followed with R values of 0.22, 0.23 and 0.24, respectively; whereas the fresh weight (FW) and dry weight/fresh weight ratio (RDF) R values were the lowest at 0.10 and 0.07, respectively. In 2020, DW and TDW R values were the highest at 0.33 and 0.61, respectively; followed by the FW and NS with R values of 0.2 and 0.22, respectively; TFW, RDF, RSL, ST and PH have lower R values of 0.12, 0.14, 0.14, 0.17 and 0.19, respectively (Table 4). The results showed that DW and TDW are hereditarily stable and less susceptible to environmental influence; thus, they could be used as important reference traits for variety selection. In 2019, FW, TFW, NS, DW, and TDW had high CVs of 29.91, 28.65, 23.40, 28.09, and 28.09%, respectively. The CVs of the RSL, ST, PH, and RDF were relatively small at 6.91, 7.18, 8.71, and 6.48%, respectively (Table 3). In 2020, FW, TFW, NS, DW, and TDW had the highest CV values of 29.37, 26.39, 20.15, 28.81, and 28.86%, respectively. The CVs of PH, ST, RDF, and RSL were relatively low at 17.88, 6.03, 7.66, and 5.06%, respectively (Table 4).
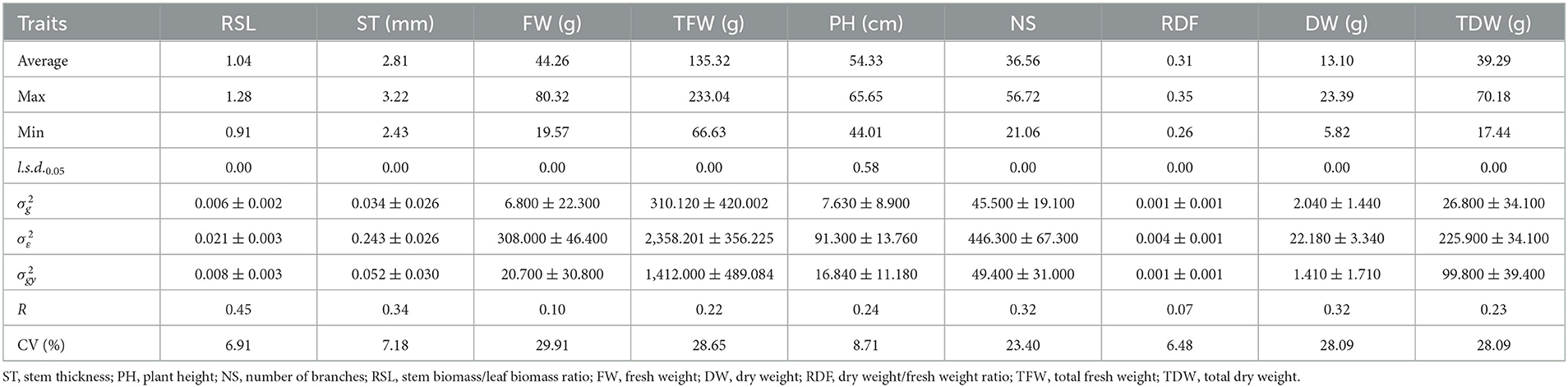
Table 3. Average, maximum, minimum, least significant differences (l.s.d.0.05), estimated genotypic () and experimental error () variance components and associated standard errors (± SE), and mean repeatability (R) estimated from the 44 varieties of alfalfa evaluated in 2019.
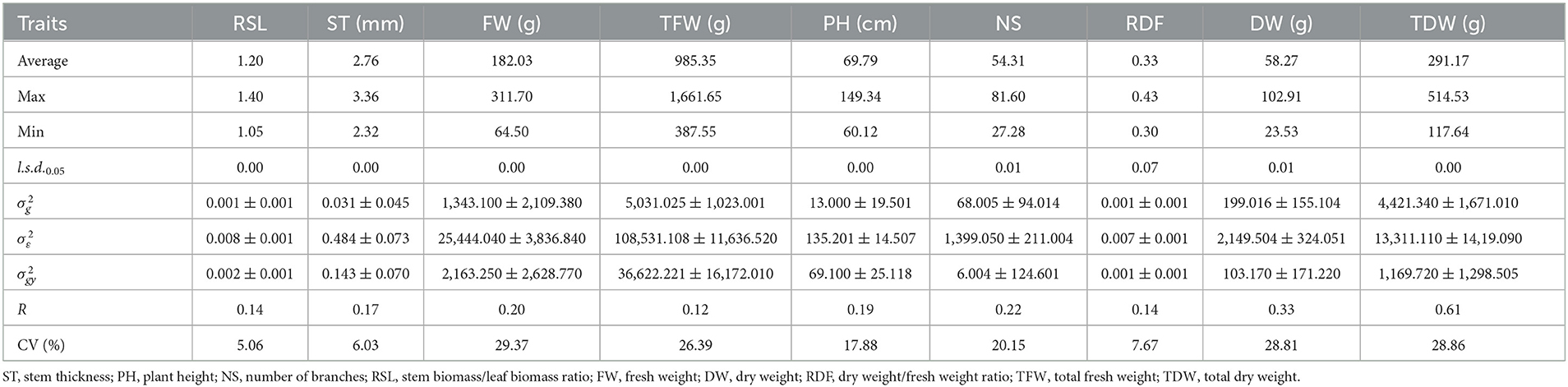
Table 4. Average, maximum, minimum, least significant differences (l.s.d.0.05), estimated genotypic () and experimental error () variance components and associated standard errors (± SE), and mean repeatability (R) estimated from the 44 varieties of alfalfa evaluated in 2020.
3.2.2. Genetic diversity of interannual agronomic traits
The results of variance analysis of the mean value of agronomic traits under the interaction of variety × year show that there were extremely significant (P < 0.01) differences among the nine agronomic traits of 44 alfalfa varieties, excluding NS, indicating rich genotypic variations between them (Table 5). Comparing the R of each trait, ST and NS had high values of 0.31 and 0.34, respectively. RSL, FW, TFW, NS, and TDW had medium R values of 0.25, 0.22, 0.27, 0.28, and 0.21, respectively. RDF and DW had low R values of 0.03. The CVs of all agronomic traits except for RSL were high, ranging from 5.03 to 27.74%, indicating high agronomic trait diversity among alfalfa varieties. In terms of interannual performance, the highest values of DW and TDW were observed in Algonquin (61.45 and 340.82 g, respectively) and the highest values of NS, FW, and TFW were observed in WL354HQ (69.16, 189.72, and 946.60 g, respectively). The highest values of PH, ST, RSL, and RDF was observed in Derby (70.29 cm), Gannong No. 6 (3.06 mm), UC-1465 (1.26), and Xinmu No. 2 (0.37), respectively.
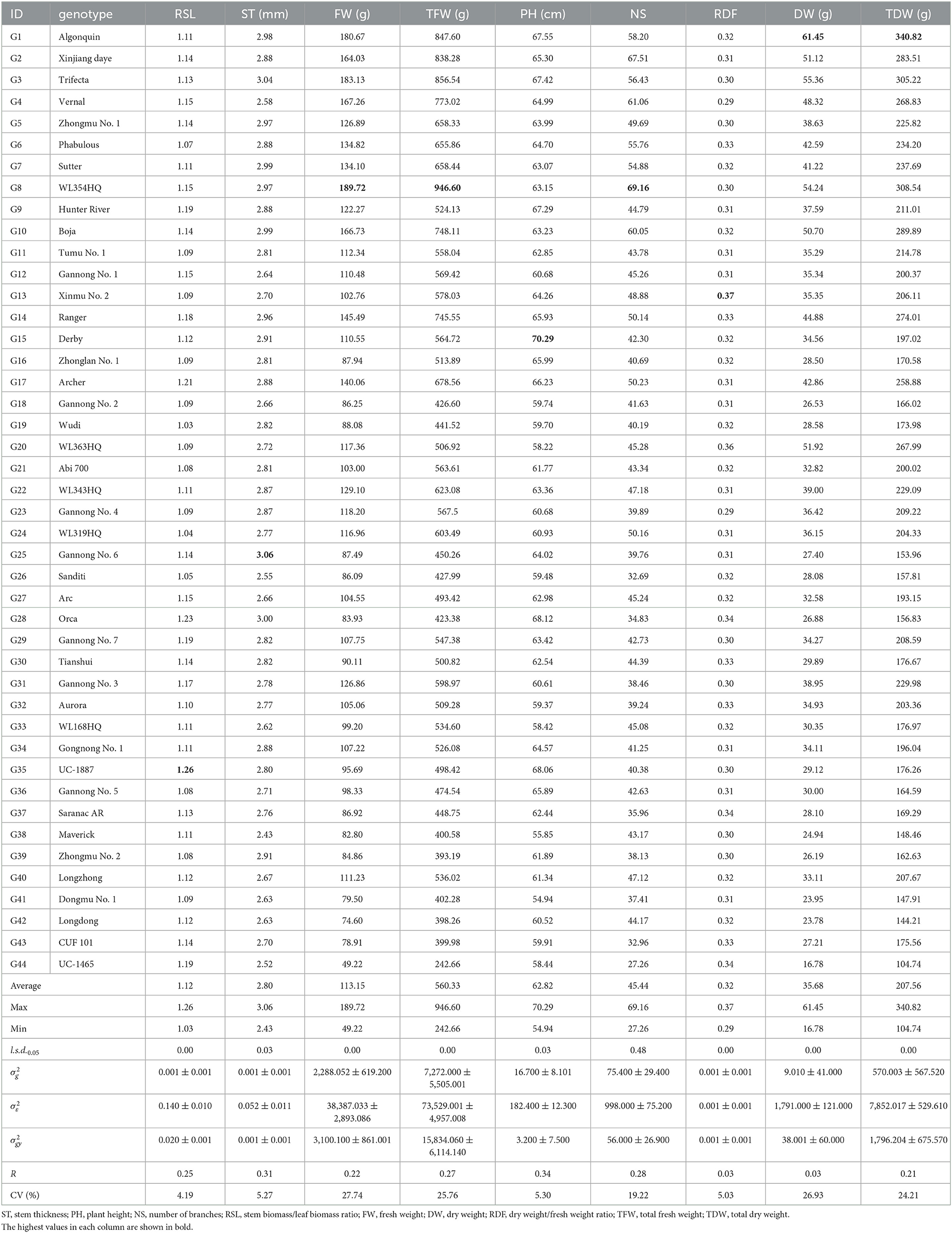
Table 5. Average, maximum, minimum, least significant differences (l.s.d.0.05), estimated genotypic () and experimental error () variance components and associated standard errors (± SE), and mean repeatability (R) estimated from the 44 varieties of alfalfa evaluated in both 2019 and 2020.
3.3. Pattern analysis and phenotypic correlation
3.3.1. Pattern analysis and phenotypic correlation in a single year
In 2019, the PCA showed that principal component 1 explained 50.49% of the total characteristic variation, and principal component 2 explained 16.45% of it (Figure 2A). According to PCA and correlation analyses (Table 6), both TFW and TDW were positively correlated with NS, DW, FW, PH, ST, and RSL (angle between direction vectors <90°, P < 0.01). Additionally, RDF was negatively correlated with DW, FW, TFW, TDW, and NS (angle between directional vectors >90°, P < 0.01). The smallest and largest positive rp were between RSL and DW (0.017) and FW and DW (0.969), respectively. Cluster analysis divided the 44 varieties into 2 groups (Figure 2A, Table 7). Group 1 contained 10 varieties, and group 2 contained 34 varieties. The mean values of RSL, ST, FW, TFW, PH, NS, DW, and TDW in the first group were greater than those in the second group.
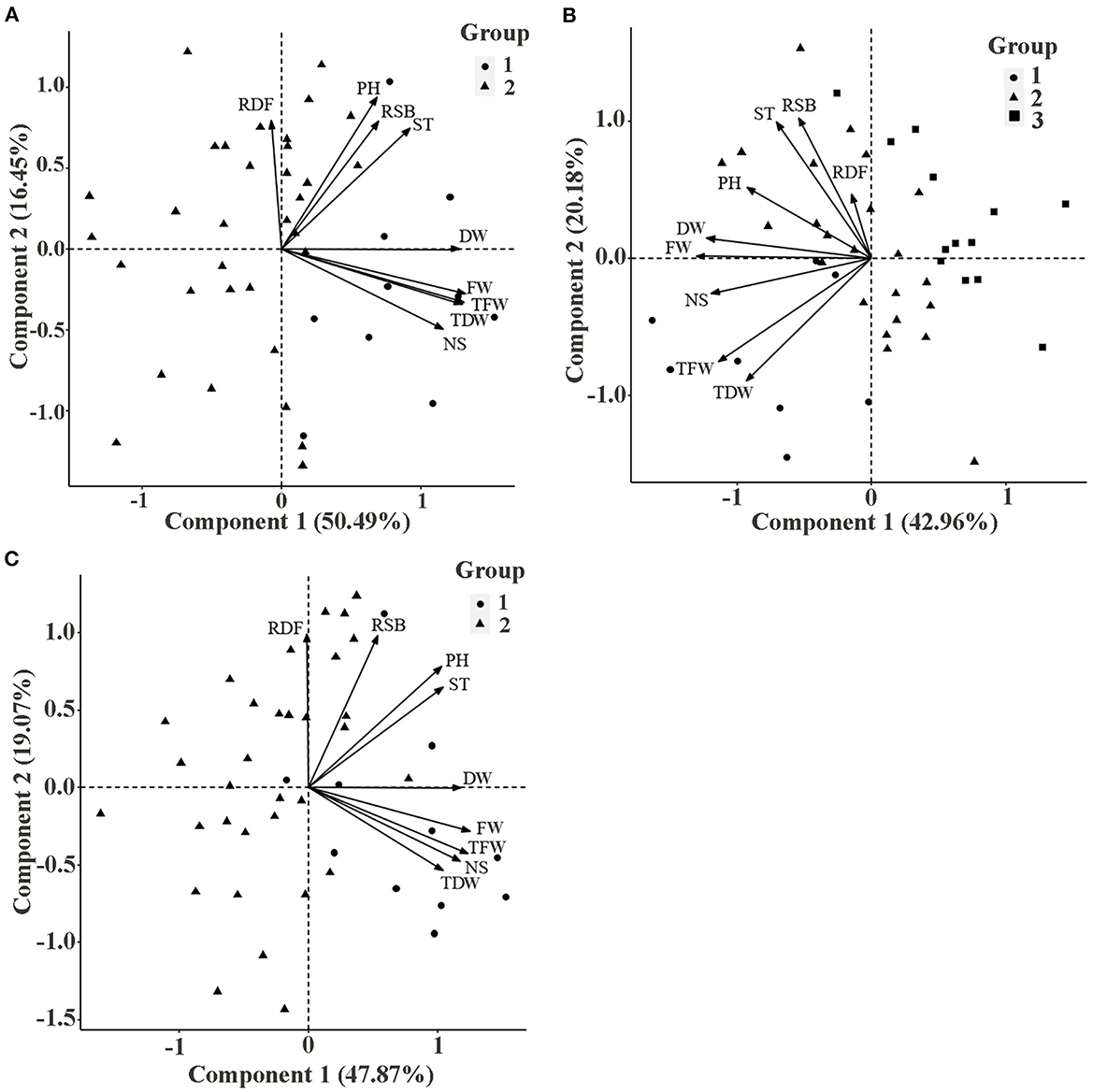
Figure 2. Principal component analysis of 9 agronomic traits of 44 alfalfa varieties in (A) 2019, (B) 2020, and (C) interannual. ST, stem thickness; PH, plant height; NS, number of branches; RSL, stem biomass/leaf biomass ratio; FW, fresh weight; DW, dry weight; RDF, dry weight/fresh weight ratio; TFW, total fresh weight; TDW, total dry weight.
In 2020, PCA showed that principal component 1 explained 42.96% of the total characteristic variation, and principal component 2 explained 20.18% of it (Figure 2B). According to PCA and correlation analyses (Table 8), among the traits, TFW and TDW were positively correlated with PH, DW, FW, and NS (angle between direction vectors <90°, P < 0.01), whereas they were negatively correlated with RSL and RDF (angle between direction vectors >90°, P < 0.05). ST, PH, FW, NS, and DW were significantly positively correlated (P < 0.01). Cluster analysis divided the 44 alfalfa varieties into three groups (Figure 2B, Table 7): group 1 contained 8 varieties, group 2 contained 23 varieties, and group 3 contained 13 varieties. Among them, group 1 had the highest values of TFW, PH, NS, and TDW, which were 1,421.05 g, 95.83 cm, 84, and 421.59 g, respectively. Group 3 had the lowest values for TFW, PH, NS, and TDW (707.81 g, 88.18 cm, 57, and 204.55 g, respectively).
3.3.2. Interannual agronomic trait pattern analysis and phenotypic correlation
Between 2019 and 2020, TFW and TDW were positively correlated with PH, DW, FW, NS, and ST (angle between direction vectors <90°) and negatively correlated with RSL and RDF (angle between direction vectors >90°) (Figure 2C). PCA showed that principal component 1 explained 47.87% of the total characteristic variation, and principal component 2 explained 19.07% of it. Cluster analysis divided the 44 alfalfa varieties into two groups (Figure 2C, Table 7), with group 1 containing 11 varieties and group 2 containing 33 varieties. The mean values of all traits in group 1 were greater than those of group 2, and the mean values of TFW, PH, NS, and TDW in group 1 were 764.26 g, 76.51 cm, 61, and 224.03 g, respectively. The same trend was observed in the agronomic traits across years (Figure 3), as varieties with high FW, DW, TFW, and TDW (Algonquin, Xinjiang daye, Trifecta, Vernal, WL354HQ, and Boja) also had relatively high ST, PH, and NS. This result was the same as that shown in Figure 2C.
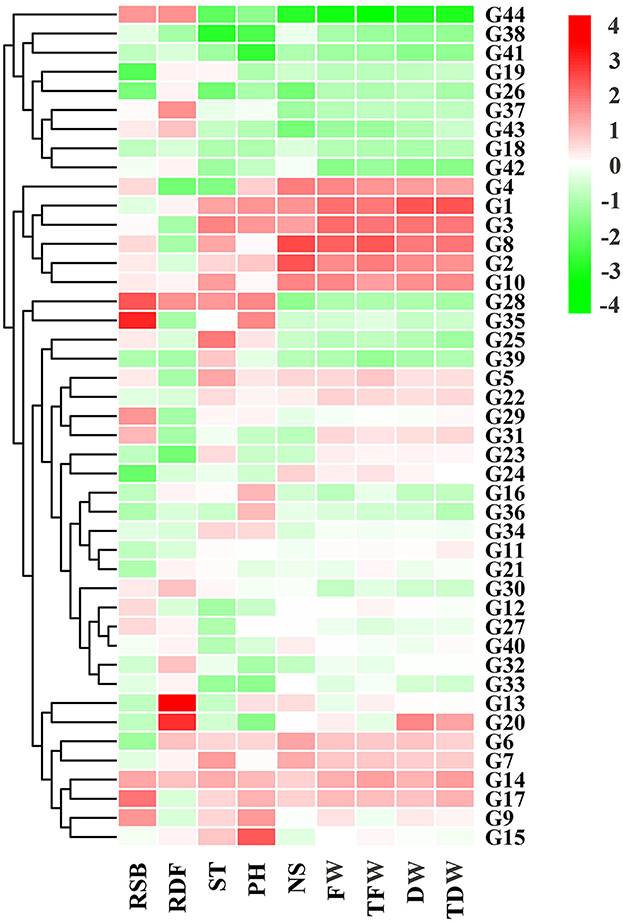
Figure 3. Heatmap showing 9 agronomic traits of 44 alfalfa varieties cultivated between 2019 and 2020. ST, stem thickness; PH, plant height; NS, number of branches; RSL, stem biomass/leaf biomass ratio; FW, fresh weight; DW, dry weight; RDF, dry weight/fresh weight ratio; TFW, total fresh weight; TDW, total dry weight.
The rp coefficients between the agronomic traits of the 44 alfalfa varieties, shown in Table 9, further demonstrate the correlation between the indicators in Figure 2C. TFW and TDW were positively correlated with PH, DW, FW, and NS (P < 0.01) and negatively correlated with RSL and RDF, reaching a significant correlation only with RDF. The smallest positive rp belonged to the PH and RSL correlation, whereas the largest belonged to the TDW and TFW correlation.
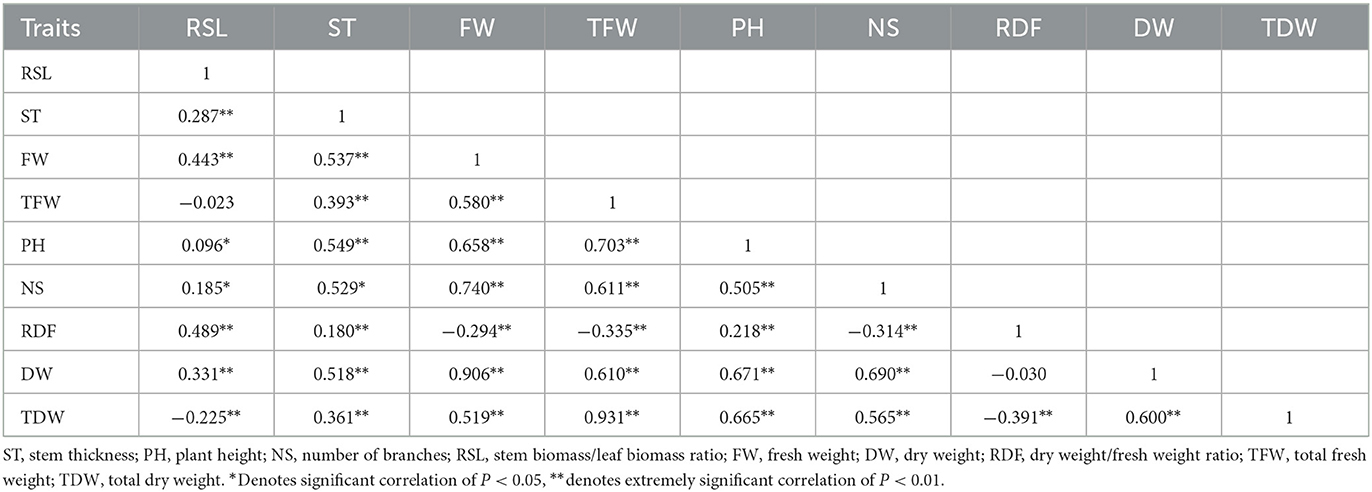
Table 9. Correlation coefficients of phenotypes (rp) among 44 varieties of alfalfa in both 2019 and 2020.
4. Discussion
Genetic variations in plants are formed by long-term natural selection and endows them with relatively stable and rich phenotypic and genotypic traits (Wu et al., 2018a). In this study, 5 alfalfa varieties (Algonquin, Xinjiang daye, Trifecta, Vernal, and Zhongmu No. 1) with strong adaptability and high grass yield were screened through GGE evaluation of the dry weights of 44 alfalfa varieties at multiple locations over 2 years. Jia et al. (2022) conducted a 3-year study on yield and agronomic traits of 10 alfalfa varieties in the Hexi Corridor region. Through the analysis of grass yield, they found that the yield of Xinjiang daye (11,416 kg/ha) was lower than that of Gannong No. 5 (13,923 kg/ha). However, in this study, Xinjiang daye showed good adaptability and high relative yields compared with Gannong No. 5. Zhao et al. (2022) conducted a 1-year adaptability study on 7 alfalfa varieties in Chifeng area, China, the total hay yield of each crop was in the order of Algonquin (12,859.40 kg/ha), Golden Empress (12,341.31 kg/ha), WL168(11,928.95 kg/ha), 55V12(10,258.90, Sanditi (9,394.60 kg/ha), Hunt River (8,782.25 kg/ha) and 54V09 (8,422.43 kg/ha). This was quite different from the performance of Algonquin, WL168 and Sanditi in this study, which may be due to a combination of genes and the environment (Dungey et al., 2018) as the same variety will show significant phenotypic variation across different environments. Thus, the above discrepancies in results may be caused by differences among the varieties, test-site climate (especially temperature and precipitation), and soil physical and chemical properties.
Agronomic traits such as RSL, ST, FW, TFW, PH, NS, RDF, DW, and TDW are key indicators used to evaluate both the quality (Bhattarai et al., 2020; Jia et al., 2022; Sayed et al., 2022) and productivity of alfalfa varieties (Singer et al., 2017). However, previous studies on alfalfa have primarily focused on agronomic traits in a single place or year (Tucak et al., 2008; Inostroza et al., 2021) as well as the development of simple sequence repeat primers (Flajoulot et al., 2005; He et al., 2020) and transcriptome analysis (Chen et al., 2011; Liu et al., 2017). In this study, phenotypic and genotypic variation analyses of 9 agronomic traits in 44 varieties of alfalfa grown in acidic soil for 2 years showed that there was abundant genotypic variation in these agronomic traits. Overall, ST, PH, RSL, FW, NS, TFW, and TDW had relatively high R values (0.21–0.34, Tables 2–4), which indicate that the trait is mainly affected by genetic factors, is less susceptible to environmental factors, and has more stable genetics (Gonçalves et al., 2020). Generally, the genetic traits ST, PH, RSL, FW, NS, TFW, and TDW are genetically more stable than RDF and DW. Dong et al. (2019) found that the R values of ST, PH, and DW of 418 common vetch germplasms in 2015 and 2016 ranged 0.89–0.95, which is much larger than the R values of those traits seen in this study. This may be because vetch is a self-pollinating plant with more conserved and stable genetics, whereas alfalfa is a cross-pollinating plant with greater variation and susceptibility to environmental influence (Bagavathiannan and Van Acker, 2009).
Plant yield is an important indicator of forage production performance and is mainly affected by dry weight (Sayed et al., 2022). El-Hifny et al. (2019) found that alfalfa genotypes exhibited a high total variability in PH and pasture yield. The forage yield of alfalfa depends mainly on PH and tillering (Monirifar, 2011). This was also verified in this study, where visualization of interannual data showed that high FW and DW were usually accompanied by higher pH and NS (Tables 3–5, Figure 3). Studies have shown that PH is positively correlated with alfalfa yield; therefore, PH can be used as an indicator for selecting the best genotype and predicting high yield in hybridization and breeding programs (Davis and Buker, 1966; Tucak et al., 2008). The RSL, fresh yield, and dry yield are important indicators for evaluating the palatability of forage, which affects forage yield. However, when the RSL is too high, it indicates that the stem yield is too large and the leaf yield is relatively reduced. Although the overall yield increases with high RSL, the protein content is low and the palatability is poor. Wu et al. (2018a) conducted a genetic diversity analysis on seven agronomic traits, including PH, FW, and DW, and the results showed that the CV of each trait was >10%. The CV of each trait in this study was high (>5%, RSL CV <5% only for 2019–2020, Tables 3–5), which could be regarded as producing large variation (Bendjama and Ramdani, 2022), indicating that the agronomic traits in this study are rich in genetic diversity. The richer the genetic diversity of a species, the greater its ability to adapt to its environment and its evolutionary potential (Nevo et al., 2012). Under the interaction between varieties × environment, the traits showed large differences, which was also conducive to the study of alfalfa variety selection and introduction adaptability in the later stage.
Previously, the correlation between agronomic traits and yield as well as their contribution rate were used to determine whether agronomic traits could be used as reference indicators for germplasm selection (Monirifar, 2011; Jia et al., 2022). In this study, PCA and related analysis results showed that PH, NS, FW, TFW, DW, and TDW all had a significant positive correlation, and the higher the RSL, the better the yield performance. This may be a consequence of higher stem/leaf ratio being accompanied by a higher PH and NS. These results are consistent with those reported by other researchers (Kephart et al., 1990; Ray et al., 1999; Avci et al., 2018). The results showed that PH, DW, FW, and NS can be used as the main target traits for breeding, and it is necessary to increase the selection of target traits in the process of variety selection. However, the contribution of alfalfa agronomic traits varied across locations and years. In the first year of planting (2019), the ecological niche requirements of alfalfa were sufficiently met, and the available resources promoted vegetative growth (Weiner et al., 2009; Zhu et al., 2019); that is, agronomic traits were proportional to DW and FW. In the second year (2020), the competition between communities increased, the varieties obtained more resources by increasing PH, the proportion of stems increased, the resources were more inclined toward reproductive growth, RSL gradually increased, and RDF increased. Also taking into account the interannual data analysis, Algonquin, Xinjiang daye, Trifecta, Vernal, WL354HQ, and Boja varieties performed well in terms of TDW and TFW in different years, environmental regions, and climatic altitudes (Tables 3–5). Thus, these varieties (genotypes) can be used in future projects to improve and develop new strains and varieties of alfalfa that can tolerate acidic soil conditions.
5. Conclusion
The agronomic traits of alfalfa are of great significance to the selection and preservation of excellent varieties, and dry weight plays a decisive role in yield. There were significant (P < 0.05) genotypic variations and genetic diversities in the traits of 44 alfalfa cultivars. Compared with other traits, PH, ST, NS, FW, TFW, and TDW R values were higher, and their genetics were relatively stable, suggesting they could be used as reference indicators for alfalfa variety selection. Genotypes Algonquin, Xinjiang daye, Trifecta, Vernal, WL354HQ, and Boja performed well under various climatic and environmental conditions. They can be used as commercial varieties as well as in projects to improve and develop new alfalfa strains that tolerate the acidic soil conditions in the south of China.
Data availability statement
The datasets presented in this study can be found in online repositories. The names of the repository/repositories and accession number(s) can be found in the article/Supplementary material.
Author contributions
RD and CC conceived the experiments. ZT, ZY, ZL, BL, YH, XW, FY, and SW performed the experiments. ZT, ZY, and RD analyzed the data. RD and ZT wrote this manuscript. All authors have contributed to the manuscript and approved the submitted version.
Funding
This research was supported by the National Natural Science Foundation of China (32060392), Guizhou Province Science and Technology Projects (Qian Ke He Zhi Cheng [2020]1Y074 and QKHPTRC-CXTD [2022]011), Qian Ke He Cheng Guo ([2022] Zhong Dian 005), and the GZMARS-Forage Industry Technology System of Guizhou Province.
Acknowledgments
We thank Professor Yanrong Wang for providing the alfalfa seeds.
Conflict of interest
The authors declare that the research was conducted in the absence of any commercial or financial relationships that could be construed as a potential conflict of interest.
Publisher's note
All claims expressed in this article are solely those of the authors and do not necessarily represent those of their affiliated organizations, or those of the publisher, the editors and the reviewers. Any product that may be evaluated in this article, or claim that may be made by its manufacturer, is not guaranteed or endorsed by the publisher.
Supplementary material
The Supplementary Material for this article can be found online at: https://www.frontiersin.org/articles/10.3389/fsufs.2023.1144061/full#supplementary-material
References
Ambati, D., Phuke, R. M., Vani, V., Sai Prasad, S. V., Singh, J. B., Patidar, C. P., et al. (2020). Assessment of genetic diversity and development of core germplasm in durum wheat using agronomic and grain quality traits. Cereal Res. Commun. 48, 375–382. doi: 10.1007/s42976-020-00050-z
Arshad, M., Feyissa, B. A., Amyot, L., Aung, B., and Hannoufa, A. (2017). MicroRNA156 improves drought stress tolerance in alfalfa (Medicago sativa) by silencing SPL13. Plant Sci. 258, 122–136. doi: 10.1016/j.plantsci.2017.01.018
Avci, M., Hatipoglu, R., Çinar, S., and Kiliçalp, N. (2018). Assessment of yield and quality characteristics of alfalfa (Medicago sativa L.) cultivars with different fall dormancy rating. Legum. Res. 41, 369–373. doi: 10.18805/LR-364
Avci, M. A., Ozkose, A., and Tamkoc, A. (2013). Determination of yield and quality characteristics of alfalfa (Medicago sativa L.) varieties grown in different locations. J. Anim. Vet. Adv. 12, 487–490. doi: 10.3923/javaa.2013.487.490
Bagavathiannan, M. V., and Van Acker, R. C. (2009). The biology and ecology of feral alfalfa (Medicago sativa L.) and its implications for novel trait confinement in North America. Crit. Rev. Plant Sci. 28, 69–87. doi: 10.1080/07352680902753613
Bendjama, A., and Ramdani, S. (2022). Genetic variability of some agronomic traits in a collection of wheat (Triticum turgidum L. sp. pl.) genotypes under South Mediterranean growth conditions. Ital. J. Agron. 17, 1976. doi: 10.4081/ija.2021.1976
Bhattarai, S., Biswas, D., Fu, Y. B., and Biligetu, B. (2020). Morphological, physiological, and genetic responses to salt stress in alfalfa: a review. Agronomy 10, 577. doi: 10.3390/agronomy10040577
Chen, Q., Zhang, X. D., Wang, S. S., Wang, Q. F., Wang, G. Q., Nian, H. J., et al. (2011). Transcriptional and physiological changes of alfalfa in response to aluminium stress. J. Agric. Sci. 149, 737–751. doi: 10.1017/S0021859611000256
Ciamporová, M. (2000). Diverse responses of root cell structure to aluminium stress. Plant and Soil 226, 113. doi: 10.1023/A:1026468403157
Ciamporová, M. (2002). Morphological and structural responses of plant roots to aluminium at organ, tissue, and cellular levels. Biol. Plant. 45, 161–171. doi: 10.1023/A:1015159601881
Dall'Agnol, M., Bouton, J. H., and Parrott, W. A. (1996). Screening methods to develop alfalfa germplasms tolerant of acid, aluminum toxic soils. Crop Sci. 36, 64–70. doi: 10.2135/cropsci1996.0011183X003600010011x
Davis, R. L., and Buker, R. J. (1966). Relationship among seedling height, greenhouse regrowth, fall height, and spring height in Medicago sativa L. 1. Crop Sci. 6, 201–204. doi: 10.2135/cropsci1966.0011183X000600020029x
Dogbatse, J. A., Arthur, A., Padi, F. K., Konlan, S., Quaye, A. K., Owusu-Ansah, F., et al. (2020). Influence of acidic soils on growth and nutrient uptake of cocoa (Theobroma cacao L.) varieties. Commun. Soil Sci. Plant Anal. 51, 2280–2296. doi: 10.1080/00103624.2020.1822384
Dong, R., Shen, S. H., Jahufer, M. Z., Dong, D. K., Luo, D., Zhou, Q., et al. (2019). Effect of genotype and environment on agronomical characters of common vetch (Vicia sativa L.). Genet. Resour. Crop Evol. 66, 1587–1599. doi: 10.1007/s10722-019-00789-3
Dungey, H. S., Dash, J. P., Pont, D., Clinton, P. W., Watt, M. S., and Telfer, E. J. (2018). Phenotyping whole forests will help to track genetic performance. Trends Plant Sci. 23, 854–864 doi: 10.1016/j.tplants.2018.08.005
El-Hifny, Z. M., Bakheit, B. R., Hassan, M. S., and El-Rady, A. (2019). Forage and seed yield variation of alfalfa cultivars in response to planting date. SVU-Int. J. Agric. Sci. 1, 21–33. doi: 10.21608/svuijas.2019.67079
Feng, Y., Shi, Y., Zhao, M., Shen, H., Xu, L., Luo, Y., et al. (2022). Yield and quality properties of alfalfa (Medicago sativa L.) and their influencing factors in China. Eur. J. Agron. 141, 126637. doi: 10.1016/j.eja.2022.126637
Flajoulot, S., Ronfort, J., Baudouin, P., Barre, P., Huguet, T., Huyghe, C., et al. (2005). Genetic diversity among alfalfa (Medicago sativa) cultivars coming from a breeding program, using SSR markers. Theor. Appl. Genet. 111, 1420–1429. doi: 10.1007/s00122-005-0074-4
Gabriel, K. R. (1971). The biplot graphic display of matrices with application to principal component analysis. Biometrika 58, 453–467. doi: 10.1093/biomet/58.3.453
Gonçalves, G., Ferreira-Gomes, R. L., Lopes, Â., and Vieira, P. (2020). Adaptability and yield stability of soybean genotypes by REML/BLUP and GGE biplot. Crop Breed. Appl. Biot. 20, e282920217. doi: 10.1590/1984-70332020v20n2a33
Grewal, H. S., and Williams, R. (2003). Liming and cultivars affect root growth, nodulation, leaf to stem ratio, herbage yield, and elemental composition of alfalfa on an acid soil. J. Plant Nutr. 26, 1683–1696. doi: 10.1081/PLN-120022381
Guo, J. H., Liu, X. J., Zhang, Y., Shen, J. L., Han, W. X., Zhang, W. F., et al. (2010). Significant acidification in major chinese croplands. Science 327, 1008–1010. doi: 10.1126/science.1182570
Hakl, J., Kunzová, E., Tocauerová, Š., Menšík, L., Mrázková, M., and Pozdíšek, J. (2021). Impact of long-term manure and mineral fertilization on yield and nutritive value of lucerne (Medicago sativa) in relation to changes in canopy structure. Eur. J. Agron. 123, 126219. doi: 10.1016/j.eja.2020.126219
He, F., Long, R., Zhang, T., Zhang, F., Wang, Z., Yang, X., et al. (2020). Quantitative trait locus mapping of yield and plant height in autotetraploid alfalfa (Medicago sativa L.). Crop J. 8, 812–818. doi: 10.1016/j.cj.2020.05.003
Humphreys, M. O. (1991). A genetic approach to the multivariate differentiation of perennial ryegrass (Lolium perenne L.) populations. Heredity 66, 437–443. doi: 10.1038/hdy.1991.53
Inostroza, L., Espinoza, S., Barahona, V., Gerding, M., Humphries, A., del Pozo, A., et al. (2021). Phenotypic diversity and productivity of Medicago sativa subspecies from drought-prone environments in mediterranean type climates. Plants 10, 862. doi: 10.3390/plants10050862
Jahufer, M. Z. Z., Cooper, M., Ayres, J. F., and Bray, R. A. (2002). Identification of research to improve the efficiency of breeding strategies for white clover in Australia-a review. Aust. J. Agr. Res. 53, 239–257. doi: 10.1071/AR01110
Jia, X., Zhang, Z., and Wang, Y. (2022). Forage yield, canopy characteristics, and radiation interception of ten alfalfa varieties in an arid environment. Plants 11, 1112. doi: 10.3390/plants11091112
Jin, L., Zhao, L., Wang, Y., Zhou, R., Song, L., Xu, L., et al. (2019). Genetic diversity of 324 cultivated tomato germplasm resources using agronomic traits and InDel markers. Euphytica 215, 69. doi: 10.1007/s10681-019-2391-8
Kephart, K. D., Buxton, D. R., and Hill, R. R. (1990). Digestibility and cell-wall components of alfalfa following selection for divergent herbage lignin concentration. Crop Sci. 30, 207. doi: 10.2135/cropsci1990.0011183X003000010045x
Kinraide, T. B. (1991). Identity of the rhizotoxic aluminium species. PSILPH. 717–728. doi: 10.1007/978-94-011-3438-5_80
Kochian, L. V., Piñeros, M. A., Liu, J., and Magalhaes, J. V. (2015). Plant adaptation to acid soils: the molecular basis for crop aluminum resistance. Annu. Rev. Plant Biol. 66, 571–598. doi: 10.1146/annurev-arplant-043014-114822
Kroonenberg, P. M. (1994). The TUCKALS line: a suite of programs for three-way data analysis. Comput. Stat. Data Anal. 18, 73–96. doi: 10.1016/0167-9473(94)90133-3
Lakić, Ž., Antić, M., Durdić, I., and Popović, V. (2019). Morphological characteristics of alfalfa genotypes tolerant to low soil pH. Genetika-Belgrade 51, 907–922. doi: 10.2298/GENSR1903907L
Liu, W., Xiong, C., Yan, L., Zhang, Z., Ma, L., Wang, Y., et al. (2017). Transcriptome analyses reveal candidate genes potentially involved in Al stress response in alfalfa. Front. Plant Sci. 8, 26. doi: 10.3389/fpls.2017.00026
Luo, K., Jahufer, M. Z. Z., Wu, F., Di, H., Zhang, D., Meng, X., et al. (2016). Genotypic variation in a breeding population of yellow sweet cover (Melilotus officinalis). Front. Plant Sci. 7, 972. doi: 10.3389/fpls.2016.00972
Monirifar, H. (2011). Path analysis of yield and quality traits in alfalfa. Not. Bot. Horti Agrobo. 39, 190–195. doi: 10.15835/nbha3926324
Nevo, E., Fu, Y. B., Pavlicek, T., Khalifa, S., Tavasi, M., and Beiles, A. (2012). Evolution of wild cereals during 28 years of global warming in Israel. PNAS. 109, 3412–3415. doi: 10.1073/pnas.1121411109
Nguyen, L., Lang, D., van Gessel, N., Beike, A. K., Menges, A., Reski, R., et al. (2016). Evolutionary processes as models for exploratory design. In Bio. Res. Arch. Build. Construct. 8, 295–318. doi: 10.1007/978-3-319-46374-2_15
Ray, I. M., Townsend, M. S., Muncy, C. M., and Henning, J. A. (1999). Heritabilities of water-use efficiency traits and correlations with agronomic traits in water-stressed alfalfa. Crop Sci. 39, 494–498. doi: 10.2135/cropsci1999.0011183X0039000200032x
Redpath, L. E., Gumpertz, M., Ballington, J. R., Bassil, N., and Ashrafi, H. (2021). Genotype, environment, year, and harvest effects on fruit quality traits of five blueberry (Vaccinium corymbosum L.) cultivars. Agronomy 11, 1788. doi: 10.3390/agronomy11091788
Rudgers, J. A., Hallmark, A., Baker, S. R., Baur, L., Hall, K. M., Litvak, M. E., et al. (2019). Sensitivity of dryland plant allometry to climate. Funct. Ecol. 33, 2290–2303. doi: 10.1111/1365-2435.13463
Ryan, P. R., and Delhaize, E. (2010). The convergent evolution of aluminium resistance in plants exploits a convenient currency. Funct. Plant Biol. 37, 275–284. doi: 10.1071/FP09261
Sayed, M. R., Alshallash, K. S., Safhi, F. A., Alatawi, A., ALshamrani, S. M., Dessoky, E. S., et al. (2022). Genetic diversity, analysis of some agro-morphological and quality traits and utilization of plant resources of alfalfa. Genes 13, 1521. doi: 10.3390/genes13091521
Singer, S. D., Hannoufa, A., and Acharya, S. (2017). Molecular improvement of alfalfa for enhanced productivity and adaptability in a changing environment. Plant Cell Environ. 41, 1955–1971. doi: 10.1111/pce.13090
Sumner, M. E., Shahandeh, H., Bouton, J., and Hammel, J. (1986). Amelioration of an acid soil profile through deep liming and surface application of gypsum. Soil Sci. Soc. Am. J. 50, 1254–1258. doi: 10.2136/sssaj1986.03615995005000050034x
Ta, H. T., Teixeira, E. I., Brown, H. E., and Moot, D. J. (2020). Yield and quality changes in lucerne of different fall dormancy ratings under three defoliation regimes. Eur. J. Agron. 115, 126012. doi: 10.1016/j.eja.2020.126012
Talebi, M., Hajiahmadi, Z., and Rahimmalek, M. (2011). Genetic diversity and population structure of four Iranian alfalfa populations revealed by sequence-related amplified polymorphism (SRAP) markers. JCSB. 14, 173–178. doi: 10.1007/s12892-011-0030-6
Tucak, M., Popovic, S., Bolaric, S., and Kozumplik, V. (2008). Agronomic evaluation of alfalfa genotypes under ecological conditions of eastern croatia. Cereal Res. Commun. 36, 651–654. Available online at: https://www.jstor.org/stable/90002788
Von Uexküll, H. R., and Mutert, E. (1995). Global extent, development and economic impact of acid soils. Plant Soil 171, 1–15. doi: 10.1007/BF00009558
Watson, S. L., DeLacy, I. H., Podlich, D. W., and Basford, K. E. (1995). GEBEI: an analysis package using agglomerative hierarchical classificatory and SVD ordination procedures for genotype × environment data. Centre for Statistics Research Report 57. Department of Agriculture, The University of Queensland, Australia.
Weiner, J., Campbell, L. G., Pino, J., and Echarte, L. (2009). The allometry of reproduction within plant populations. J. Ecol. 97, 1220–1233. doi: 10.1111/j.1365-2745.2009.01559.x
White, T. L., and Hodge, G. R. (1989). Predicting breeding values with applications in forest tree improvement. Forest. Sci. 33, 1. doi: 10.1007/978-94-015-7833-2
Wu, X. M., Guo, P., Chi, H. W., Fang, Z. H., Shi, Y. H., Wang, Y. Q., et al. (2018a). Diversity analysis of phenotypic traits and quality characteristics of alfalfa (Medicago sativa) introducted from abroad germplasm resources. J. Plant Genet. Resour. C. 19, 103–111. doi: 10.13430/j.cnki.jpgr.2018.01.012
Wu, Y. H., Fan, Z. L., Li, J., Guo, J. H., Guo, Y. K., and Wang, Y. L. (2018b). Phenotypic diversity of seeds and fruits in natural populations of Acer ginnala Maxim in China. Guihaia 38, 795–803. doi: 10.11931/guihaia.gxzw201711041
Yan, W., Hunt, L. A., Sheng, Q., and Szlavnics, Z. (2000). Cultivar evaluation and mega-environment investigation based on the GGE biplot. Crop Sci. 40, 597. doi: 10.2135/cropsci2000.403597x
Yang, K. M., Li, G. Q., Guo, S. J., and Qiao, L. (2014). Genetic diversity and association analysis of agronomic traits with SSR in a natura. J. Nuclear Agric. Sci. 9, 1576–1584. doi: 10.11869/j.issn.100-8551.2014.09.1576
Yuegao, H., Cash, D., Kechang, L., Suqin, W., Ping, Z., and Rong, G. (2010). Global status and development trends of alfalfa. Alfalfa management guide for Ningxia 1–14. United Nations Food Agriculture Organization. Rome.
Zeng, X. B. (2000). Acidification of red soils and control methods. Chin. J. Soil Sci. 31, 111–113. doi: 10.19336/j.cnki.trtb.2000.03.005
Zhao, M. Q. E., Wang, Z. J., Bao, J., Du, S., Hao, J. F., Yan, X., et al. (2022). Comprehensive performance evaluation of seven alfalfa varieties in Chifeng area. Heilongjiang Anim. Sci. Vet. Med. 652, 94–101+139. doi: 10.13881/j.cnki.hljxmsy.2021.09.0209
Keywords: Medicago sativa, acidic soil, agronomic traits, genetic diversity, genotypic variation
Citation: Tian Z, Yang Z, Lu Z, Luo B, Hao Y, Wang X, Yang F, Wang S, Chen C and Dong R (2023) Effect of genotype and environment on agronomical characters of alfalfa (Medicago sativa L.) in a typical acidic soil environment in southwest China. Front. Sustain. Food Syst. 7:1144061. doi: 10.3389/fsufs.2023.1144061
Received: 13 January 2023; Accepted: 09 February 2023;
Published: 01 March 2023.
Edited by:
Yuan Li, Lanzhou University, ChinaReviewed by:
Erick Amombo, University of Chinese Academy of Sciences (CAS), ChinaKai Luo, Hainan University, China
Copyright © 2023 Tian, Yang, Lu, Luo, Hao, Wang, Yang, Wang, Chen and Dong. This is an open-access article distributed under the terms of the Creative Commons Attribution License (CC BY). The use, distribution or reproduction in other forums is permitted, provided the original author(s) and the copyright owner(s) are credited and that the original publication in this journal is cited, in accordance with accepted academic practice. No use, distribution or reproduction is permitted which does not comply with these terms.
*Correspondence: Rui Dong, cmRvbmdAZ3p1LmVkdS5jbg==