- Management School, Hainan University, Haikou, China
Cultivated land protection and quality improvement have become inevitable requirements for alleviating ecological and environmental pressure and sustainable agricultural development. It is of practical significance to explore the differences and causes of cultivated land protection behaviors (CLPB) between smallholders and professional farmers for formulating targeted protection policies and improving their effectiveness. Based on 422 mango farmers' survey data in Hainan Province, this paper explored the internal and external characteristics between smallholders and professional farmers, and used the Fairlie decomposition method to compare and analyze the sources of differences in farmers' CLPB. The results showed that: (1) the CLPB of smallholders and professional farmers differ significantly; (2) the sources of differences in CLPB between smallholders and professional farmers are different, including differences in internal characteristics and differences in external characteristics; (3) differences in internal characteristics are the main cause of the differences in farmer's CLPB, and the contribution of differences in external characteristics was smaller, of which planting years, annual household income and planting scale are the top three factors. It is suggested that differential protection policies should be designed for smallholders and professional farmers, such as guiding smallholders to carry out large-scale operations and improve their organizational level, encouraging and guiding professional farmers to sign long-term contracts to stabilize the land tenure, and formulating subsidy policies for cultivated land protection.
Introduction
Cultivated land is an essential resource for human survival and development, which provides the basic guarantee for food production and security and plays a highly significant role in rural economic development and the ecological environment (Gomiero, 2016; Prăvălie et al., 2021). With increasing population size and urbanization, many high-quality cultivated lands have become urban lands, while cultivated land quality continues to decline due to low adoption of environmentally friendly agricultural production technologies, posing a severe challenge to increased agricultural demand and sustainability (Fazal, 2000; Zhou et al., 2021). At the same time, urbanization and excessive use of fertilizers and pesticides have brought about soil pollution and ecological degradation, further causing a decline in the quality of cultivated land (Jallow et al., 2017; Abass et al., 2018; Liu and Fang, 2021). Therefore, cultivated land protection and quality improvement have become inevitable requirements for alleviating ecological and environmental pressure and achieving sustainable agricultural development.
Since China's “reform and opening up,” significant changes have occurred in socio-economic and cultivated land use. As one of the world's leading agricultural countries, China attaches importance to high-quality agricultural development, and cultivated land protection and quality improvement are important objectives of agricultural modernization (Lu et al., 2019; Su et al., 2019). The Chinese government has acted to protect cultivated lands and improve their quality. As early as 2015, the Chinese government put forward the strategy of “storing grain in the land and storing grain in the technology” and proposed to build 53 million hectares of high-standard cultivated land by 2020 to ensure national food security. The Ministry of Agriculture and Rural Affairs issued the Action Plan for the Protection and Improvement of Cultivated Land Quality in 2017 and the Key Policies for Strengthening and Benefiting Agriculture in 2021, which proposed new rules for cultivated land protection, including cultivated land protection subsidies, high-standard cultivated land construction, and land quality protection and improvement.
Under the household contract responsibility system in China, farmers are the basic unit of cultivated land use and most important participants and stakeholders of cultivated land protection, their land use behavior is the key to the improvement of cultivated land quality (Xue et al., 2021). Unlike the past, which relied mainly on many homogeneous smallholders to engage in agricultural production activities, the diversification of agricultural management subjects has gradually become a necessary basic feature of China's modern agricultural management system, such as the coexistence of family farms, large professional households and smallholders which has become a common phenomenon in China's agricultural production and different farmers play an important role in the protection of cultivated land quality (Lai et al., 2020). As the emerging body of agricultural production in China, professional farmers are mainly characterized by production specialization, which is reflected in the high level of technicalization, scale and organization in the production process. Unlike smallholders, professional farmers are modern farmers with higher educational knowledge level, modern civic competition, strong sense of democracy and cooperation and high anti-risk ability. Since the types of farmers are diversified, different farmers differ significantly in their characteristics and their CLPB may also be different (Lien et al., 2006). Therefore, it is significant to explore the differences in CLPB of different farmers and their influencing factors to formulate targeted cultivated land protection policies and improve their effectiveness.
From the existing studies, scholars have conducted rich research on farmers' CLPB and found that individual and household characteristics significantly affect their protection behavior (Ajewole, 2010; Das and Sahoo, 2012; Ma et al., 2018). However, few studies have been conducted to explain the differences and why different farmers have different CLPB, and only a few scholars have explored the protection behaviors of different farmer types, which is of reference significance to this paper. For example, some studies have shown that scattered smallholders have arbitrariness and blindness in agricultural production, with a general lack of knowledge about green production, and a poor understanding of the hazards of excessive fertilization and arable land protection (Mponela et al., 2016; Liu et al., 2021). Compared to smallholders, large-scale farmers are more inclined to adopt capital-intensive production methods, which are often associated with environmentally friendly technologies, due to labor shortage (Chen et al., 2022). Family farms with relatively better infrastructure conditions and stronger credit capacity are also more inclined to adopt green production practices to meet market demand and improve their agricultural products' quality and brand influence (Shang et al., 2021). This suggests that different types of farmers show significant differences in CLPB. In general, there are still relatively few comparative studies on the protection behaviors of different types of farmers, and the reasons for the differences in farmers' protection behaviors are still unclear.
In view of this, this paper takes mango farmers in Hainan Province as an example, and takes smallholders and professional farmers as research objects, which is different from previous studies that mostly compare smallholders with large-scale farmers or family farms. Based on defining and describing these two types of farmers, the Fairlie decomposition method is applied to explore farmers' differentiated CLPB and the main reasons so as to provide a reference basis for designing relevant incentive policies. This study contributes to the existing literatures in several ways. First, this paper takes professional farmers as the research object, which makes up for the shortage of previous researches focusing on smallholders, large-scale farmers, and family farms. Second, the differences in CLPB between smallholders and professional farmers and the generation mechanism are clarified theoretically, which enriched the theoretical research on the farmers' environmental protection behaviors. Third, while most of the existing studies focus on farmers growing field crops, this paper explores the cultivated land protection behaviors of fruit growers and broadens the research boundary.
The rest of the paper is organized as follows: in Section Conceptual framework, a conceptual framework related to farmers' cultivated land protection behaviors is developed. Section Materials and methods introduces data sources, sampling descriptions, and modeling methods. A results section follows showing the empirical results. Discussions and policy implications are presented in Section Discussion and the paper ends with conclusion in Section Conclusions.
Conceptual framework
There are two main types of mango farmers in Hainan Province: smallholders and professional farmers. The basic situation of the two types of farmers is shown in Table 1. There are significant differences in characteristics between smallholders and professional farmers, which can be categorized into two aspects: on the one hand, there are differences in external characteristics, which mainly refer to differences in socio-economic characteristics at the household level; on the other hand, there are differences in internal characteristics, mainly referring to the differences farmers' identity, adaptability, responsibility, and sense of belonging.
Smallholders
Smallholders are engaged in agricultural production based on family business units, which is the typical form of mango planting in Hainan. From the national level, the “big country with small farmers” is still the basic national condition of China; smallholders account for more than 98%, and operating cultivated land area accounts for 70% of the total cultivated land area, which is the most basic main body of China's agricultural production.1 Most smallholders from the formation of farmers are Hainan local farmers whose labor input is mainly family members. The education level of smallholders is relatively low, and they tend to base their agricultural production on their own experience, and their production technology level is relatively low. In terms of land characteristics, smallholders generally operate on a small scale, and the degree of land fragmentation is relatively high (Alemu et al., 2017). In terms of the organizational characteristics of farmers, smallholders often show a lack of cooperation or low efficiency of cooperation among themselves, a low degree of organization, and a generally weak ability to resist natural and market risks (Li et al., 2021).
Professional farmers
Scholars have not reached a consensus on the definition and classification of professional farmers (Zhao et al., 2019). According to Zhong et al. (2018), this paper selects agricultural reclamation farmers with vocational characteristics as representatives of professional farmers for analysis. Therefore, professional farmers in this paper refer to growers affiliated with state farms and contract farm land for specialized production by their households. This type of farmer's business form is based on state-owned land and the implementation of the joint production contract as the basis for family farm production under the leadership of state farms. Established in 1952, Hainan State Farm has 47 state farms, making it the third largest farm in China after Xinjiang Production and Construction Corps and Heilongjiang State farms, which has made important contributions to driving and radiating the economic and social development of the surrounding rural areas. In the 1990's, through the reform of the two-tier management system, the state-owned farms established the land contract management right, and the farm workers contracted the farm land for production, thus forming professional farmers. At the national level, the total number of professional farmers has exceeded 20 million by 2021, becoming the emerging main body of China's agricultural production. From the perspective of the formation of farmers, professional farmers are mostly migrants who entered Hainan during the construction of state-owned farms in 1960's and 1970's. In terms of the technical level, since most family members of professional farmers are farm workers, their professional technology and mechanical equipment level are relatively higher (Liu et al., 2021). In terms of land characteristics, professional farmers also contract farm state-owned land through the family joint production responsibility system and tend to carry out agricultural production on a larger scale. In terms of the organizational characteristics of farmers, professional farmers are backed by state-owned farms and tend to be organized by the farms on production materials purchase, technology and variety promotion, and agricultural products marketing, so the degree of organization of professional farmers is higher compared to smallholders.
Since domestic and foreign studies have fully discussed the influence of external characteristics of farmers (such as gender, age, and ethnicity) on CLPB (Ros-Tonen et al., 2019; Belachew et al., 2020; Henriksson et al., 2021), this paper focus on the analysis of farmer's internal differences and how they affecting farmers' CLPB. Based on the descriptions of the two types of farmers above, it is clear that their basic conditions differ significantly. We believe that farmers' different internal characteristics can lead to differentiated cultivated land protection behaviors mainly through the following mechanisms: the first is the heterogeneity of the formation period, which may lead to different geographical identities and adaptations. Smallholders, as local farmers in Hainan, were formed at an earlier period, while professional farmers, as outsiders who came to Hainan during the establishment of state-owned farms, were formed at a later period compared to smallholders, so there are large differences in the geographical identity and adaptability of the two types of farmers (Cai et al., 2017). Second, the technical level of professional farmers is higher than that of smallholders, which may be due to inherent differences in production philosophy (Wang et al., 2021). Third, the nature and status of land ownership differ between smallholders and professional farmers, and thus the sentiment and dependence on land may differ, which may further affect farmers' sense of responsibility for cultivated land protection (Chen, 2013; Ayamga et al., 2016). Fourth, the degree of organization of village collectives and state farms differs, which may affect farmers' access to production materials and marketing of agricultural products, but may ultimately affect their sense of belonging (Mosimane et al., 2012). Base on the above analysis, it is clear that the differences in characteristics of different types of farmers may lead to the internal differences in farmers' characteristics of identity, adaptability, production philosophy, responsibility, and sense of belonging, which may further influence their behavioral attitudes, subjective norms, and perceived behavioral control (Yao et al., 2016), and ultimately lead to differential CLPB (as shown in Figure 1).
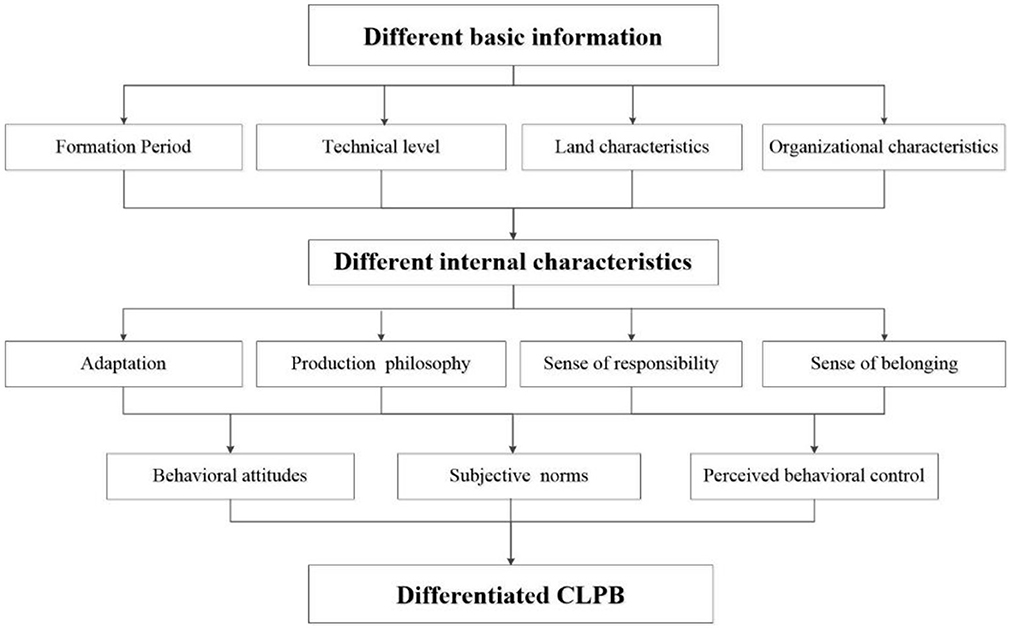
Figure 1. The formation of internal characteristics differences and their influence on farmers' CLPB.
Materials and methods
Data source
The data in this paper come from the field research conducted by the research team in 2020 in Dongfang, Ledong, and Sanya cities of Hainan Province for mango growers, as shown in Figure 2. Mango as an important tropical fruit, coupled with Hainan's suitable light and heat conditions, gradually become one of the main products of Hainan agriculture. The sample was selected because Ledong, Dongfang, and Sanya are important mango planting bases in Hainan. At the end of 2019, the mango planting area in these three cities and counties accounted for 86.5% of the total planting area in Hainan Province, which is representative of the study. The survey used a combination of staged sampling and a random sampling method. In the first stage, three townships were selected from each city or county; in the second stage, two to three administrative villages were randomly selected from each township; in the third stage, 20–25 mango growers were randomly selected as survey subjects. The questionnaire survey mainly includes basic information about individual farmers and families, mango production and operation, etc. A total of 449 questionnaires were distributed, 27 invalid and incomplete questionnaires were excluded, and finally, 422 valid questionnaires were obtained, with an efficiency rate of 93.99%.
The basic characteristics of the sample growers are shown in Table 2. Mango growers whose household heads are over 50 years old account for 54.97% of the total sample, and those whose heads are 35–50 years old account for nearly 40%; the education level of household heads is mostly junior high school or below, accounting for 83.42%, but there are a few household heads with a college education or above. 8.29% of mango farmers have an annual household income of < 100,000; 68.96% of the full sample are mango farmers with an agricultural labor force ratio higher than 0.75, indicating that most of the surveyed sample are mainly engaged in agriculture; 72.27% of mango farmers have been cultivating for more than 10 years, indicating that most of the sample have long-term cultivation experience; 63.74% of mango farmers have a planting scale of fewer than 20 mu, and 13.03% have a planting scale of more than 40 mu. The sample generally shows good representativity.
Variable settings
Explained variable
The explained variables in this paper are the protection behaviors of mango farmers, which correspond to the questions “Do you use organic fertilizer?” and “Do you use soil testing and fertilization technology?” If the respondent farmers answered in the affirmative, they were considered to have CLPB and the explanatory variable was assigned a value of 1, otherwise a value of 0. It should be noted that the organic fertilizer referred to is a commercial organic fertilizer made from livestock and poultry manure, animal and plant residues, and other resources after fermentation and maturation. The soil testing and fertilization technology are tested by enterprises, agricultural stores, soil fertilization institutes, or fruit stations, mainly including the five steps of “soil testing, formulation, fertilization, supply, and guidance.”
Explanatory variable
Based on existing studies, we selected personal characteristics of household heads, household characteristics, and production and management characteristics as the main explanatory variables affecting mango farmers' CLPB, as shown in Table 3.
Firstly, the household head, as the leading decision-maker in household production and operation, has an important influence on CLPB. Personal characteristics of the household head, such as age, education level, perception of fertilizer pollution, planting years, and internet use, often influence the decision-making (Adnan et al., 2017; Tey et al., 2017; Rahman and Zhang, 2018; Haile et al., 2019; Ma et al., 2020).
Second, the agricultural labor proportion reflects farmers' dependence on agricultural production. Households with a higher proportion of agricultural labor rely more on agricultural production, which may prompt them to apply organic fertilizers that help improve cultivated land quality (Waithaka et al., 2007; Noll et al., 2014; Teshome et al., 2016). Annual household income directly impacts farming households' access to organic fertilizers, and in general, households with high-income level have more capital to invest in green production (Nastis et al., 2019). At the same time, farmers' CLPB is also easily influenced by social relationships and surrounding neighbors, for example, farmers with more interactions and stronger neighborhood effects are more likely to obtain information about cultivated land protection and thus promote their implementation of CLPB (Tsusaka et al., 2015; Zeweld et al., 2018; Qiao et al., 2022). Therefore, four variables, namely the proportion of agricultural labor, annual household income, number of interactions, and neighborhood effect, were selected to investigate the effects of household characteristics.
Furthermore, farmers with larger planting scale and more plots of cultivated land may need to invest more labor and transportation costs if they implement CLPB, which may negatively affect their CLPB to a certain extent (Kaliba et al., 2000). Extension services and support subsidies provide technical and financial support to farmers, respectively, which can reduce the pressure on farmers to conserve their land and thus increase their likelihood of implementing CLPB (Boz, 2016; Zhang et al., 2022). Therefore, this paper also selects the variables of planting scale, number of land plots, extension services and support subsidies to study the influence of production and management characteristics on their CLPB (Abhilash and Singh, 2009; Qiao et al., 2022).
Model settings
The Oaxaca-Blinder decomposition method (“O-B decomposition method”) is used by scholars at home and abroad to analyze the sources of difference between groups. The method can decompose the sources of difference between groups into observable differences in characteristics (the explainable part) and unobservable differences in coefficients (the unexplainable part), and then determine the main causes of difference (Blinder, 1973; Oaxaca, 1973). However, the early O-B decomposition was based on linear regression models with continuous explained variables and did not apply to models with discrete explained variables. In view of this, Nielsen and Bauer extended the O-B decomposition to discrete explained variable models (Nielsen, 1998; Bauer and Sinning, 2008), making it applicable to the decomposition of Logit models. However, the model can only analyze the explainable component as a whole and fails to refine the contribution of differences to specific explanatory variables. Further, Fairlie improved the extended O-B decomposition to allow the analysis of the extent to which each explanatory variable in the explainable component contributes to the difference, which is also known as the “Fairlie decomposition” (Fairlie, 2005). The explained variable in this paper is “whether to adopt CLPB,” which is a discrete binary variable and thus requires the Fairlie decomposition method. Firstly, this paper uses a binary logit model to estimate the factors influencing farmers' CLPB. The specific model form is as follows:
In Equation 1, the yi is the explained variable (whether to adopt CLPB); F denotes the cumulative distribution function of the logistic distribution; zi is a linear combination of explanatory variables, mainly including personal characteristics of household heads, household characteristics, and production and management characteristics; δ is the estimated coefficients affecting the explained variables. The probability distribution of farmers' CLPB can be expressed as follows:
Further, based on the estimation results of the Logit model, an extended O-B decomposition was used to perform a primary decomposition, which decomposes the sources of differences in CLPB between the two types of farmers into an explainable part (differences in external characteristics as referred to in the text) and an unexplainable part (differences in internal characteristics as referred to in the text). The decomposition method is as follows:
In Equation 3, and represents the mean value of CLPB of Professional Farmers and smallholders, respectively; indicates the difference in CLPB between professional farmers and smallholders; Zpi and Zsi denotes the explanatory variables corresponding to professional farmers and smallholders, respectively; Np and Ns represents the sample size of professional farmers and smallholders respectively; and are the estimated coefficients of the explanatory variables for the professional farmers and smallholders, respectively. The difference between the first two terms on the right-hand side of Equation 3 is the explainable part of the difference in CLPB between professional farmers and smallholders, i.e., the difference in CLPB caused by differences in the personal, household and production characteristics of the household head; the difference between the last two terms is the unexplainable part of the difference in CLPB between professional farmers and smallholders. An alternative expression for Equation 3 is as follows:
The difference between Equations 3 and 4 is the choice of a different benchmark. Equation 3 is based on the estimated coefficients for professional farmers, while Equation 4 is based on the estimated coefficients for smallholders. It can be seen that the choice of different benchmarks may yield different analytical results, with the problem of index benchmarking, i.e., whether the estimated coefficients of smallholders or professional farmers are used as a benchmark, the unexplained component is over- or underestimated. Therefore, this paper draws on the solution proposed by Newmark to calculate the estimated coefficients for the entire sample with the following equation:
Finally, to obtain the contribution of each explanatory variable in the explainable component to the source of differences, the paper further decomposed according to Fairlie. For the sake of exposition, it is assumed that Np = Ns and there are only two explanatory variables in the explainable part XA and XB. Then the differences contribution of the explainable part of Equation 5 is expressed as Equation 6:
In Equation 6, is the full sample of farmers estimated coefficient of the constant term c in the Logit model. It can be obtained by decomposition calculation: variables XA and XB respective contributions to the sources of variation are as follows (Fagbamigbe et al., 2021):
Results
Analysis of the differences in the characteristics of smallholders and professional farmers
To further compare the differences in CLPB and each explanatory variable between smallholders and professional farmers, descriptive statistics and mean difference tests were conducted separately for the two types of farmers, as shown in Table 4. It can be seen that the mean values of CLPB between smallholders and professional farmers are 0.292 and 0.403, respectively, and are significant at the 5% level. In terms of the personal characteristics of the household head, there were significant differences in planting years between the two types of farmers at a significant level of 1%, while there were no significant differences in age, education, chemical fertilizer pollution cognition, and internet usage. In terms of household characteristics, there were significant differences in annual income between the two types of farmers at a significant level of 1%, while the share of agricultural labor, number of interactions, and neighborhood effects did not differ significantly. In terms of the production and management characteristics, there were significant differences in planting scale at a significant level of 1%, while there were no significant differences in the number of land plots, extension services, and support subsidies.
Analysis of factors influencing farmers' CLPB
This paper used Stata (Version 15.0, created by Stata Corp LLC in Texas, USA) software for model estimation, as shown in Table 5. To exclude the possible co-linearity problem among the explanatory variables, this paper adopts the variance inflation factor method to conduct the multiple co-linearity tests. The test results show that each variable's Vif (variance inflation factor) is < 5, indicating no problem with multicollinearity.
Firstly, in terms of personal characteristics, the education level has a significant positive effect on CLPB of smallholders, professional farmers and full sample farmers, probably because more educated farmers have a higher awareness of the ecological services, social security value, as well as a stronger ability to learn land protection techniques. Planting years had a significant positive effect on CLPB for the smallholders and total sample farmers, while age, chemical fertilizer pollution cognition and internet use were not significant in the estimated coefficients for all three groups of samples, indicating that they had no significant effect on CLPB. Secondly, in terms of the household characteristics, the share of agricultural labor and annual income had a significant positive effect on CLPB of smallholders and total sample farmers, which indicated that farmers with more agricultural labor in the household and higher total income had more positive CLPB (Nastis et al., 2019). Neighborhood effect had a significant positive effect on CLPB of professional farmers, while number of interactions was not significant in the estimated coefficients of all three sample groups. Furthermore, in terms of production and management characteristics, planting scale has a significant negative effect on CLPB of smallholders, professional and full sample farmers, the possible reason is that the larger the planting scale, the more labor and transportation costs may be required to implement protection behavior, which negatively affects their protection behavior to some extent (Kaliba et al., 2000). Extension services significantly positively affected the CLPB of professional farmers and full sample of farmers. It may be due to the fact that professional farmers with well-developed infrastructure can apply the technologies provided by extension services more efficiently in practice, while smallholder farmers may lack the conditions for application (Liu et al., 2021). The number of land plots and support subsidies were insignificant in the estimated coefficients of all three groups of samples. It may be because farmers with many land plots are more dependent on agricultural production, prompting them to improve cultivated land quality, which offsets inconvenient transportation's negative impact on farmers' CLPB. In addition, the capital, time and labor costs of CLPB are very high, and limited support subsidies can hardly cover the costs for farmers, so subsidies have no significant impact.
It can be seen that there are significant differences in the external characteristics that influence the CLPB of smallholders and professional farmers. This suggests that the causes of differences in CLPB between the two types of farmers are different in their explainable parts (differences due to external characteristics). Further discrepancy decomposition is required to explore the reasons for the above discrepancies.
Decomposition of differences in CLPB
In this paper, we decompose the causes of the difference in CLPB between smallholders and professional farmers by the Fairlie decomposition method. The method requires an equal sample size in the comparison group, using the farmer category with the smaller sample size in the comparison group as a benchmark, and an equal subsample from the farmer category with the larger sample size in the comparison group for analysis by random sampling. To avoid over-reliance of the analysis results on a single sub-sample, each group of comparisons in this paper was repeated 100 times as described above. In addition, when using the Fairlie decomposition method for analysis, the same variable may be estimated with slightly different results depending on the order in which it is placed. To solve the above problem, we randomly collected 100 sets of subsamples while randomly sorting the variables 100 times, then decomposed them and calculated the mean value of the different contributions of each variable as the final result. Table 6 reports the specifics of the decomposition of differences in CLPB between the two types of farmers.
In the comparison between smallholders and professional farmers, the total difference between the two types of farmers' CLPB was 0.112, using the estimated coefficient of the full sample as a benchmark. The explainable part of the difference was 0.029, accounting for 26.11%; the unexplainable part was 0.083, accounting for 73.89%. Further decomposition of the explainable part shows that planting years, annual income and planting scale are the main factors influencing the difference in CLPB between the two types of farmers, and their contribution rates are above 10%, among which planting years and annual income increase the difference in CLPB between the two types of farmers by 11.03 and 27.34%, respectively, and planting scale decreases the difference in CLPB between the two types of farmers by 30.77%. From the above analysis results, it is clear that the difference in internal characteristics accounts for 73.89% of the difference in CLPB between the two types of farmers. In comparison, the difference in external characteristics accounts for 26.11%. The contribution of planting years, annual income, and planting scale are greater because professional farmers have more experience in planting, higher household capital endowment, and larger planting scale.
Robustness test
In order to verify the reliability of the above findings, the robustness test was conducted by replacing the index benchmark. The estimated coefficients of smallholders and professional farmers were used as the new index benchmark of the model to replace the index benchmark in the original model, and then the regression analysis was conducted again. The results are shown in Table 6. The estimation results using the coefficients of smallholders and professional farmers as benchmarks are similar to those of the full sample of farmers, indicating that the above results are robust.
Discussion
Protecting the quality of cultivated land is an inevitable requirement for alleviating ecological and environmental pressure and achieving sustainable agricultural development. For this reason, the Chinese government has introduced many policies on cultivated land protection. Effectively implementing cultivated land protection policies depends greatly on farmers' acceptance of the policies. However, various types of farmers in China have different characteristics, and their acceptance of cultivated protection policies differs. As representatives of agricultural operation subjects, smallholders, and professional farmers play an important role in protecting cultivated land quality. Therefore, it is essential to study the differences in CLPB and the reasons between smallholders and professional farmers to formulate differentiated protection policies.
Previous studies on farmers' cultivated land protection provide a good reference for this paper. However, two points remain to be explored: first, there are still relatively few comparative studies on different types of farmers' CLPB, and the reasons for the differences in their behaviors are still unclear; second, in the existing studies, scholars have more often explored the differences among smallholder and large-scale farmers, but relatively few studies have been conducted on professional farmers, who are an important component. Therefore, this paper focuses on the differences and reasons of CLPB between smallholders and professional farmers. It is found that smallholders' and professional farmers' external and internal characteristics are different, which leads to the great difference in CLPB. Secondly, this paper discusses the influencing factors of CLPB, and analyzes the factors that cause the difference and their contributions.
Based on the main findings, this paper puts forward the following policy recommendations: (1) As the most basic subject of agricultural production in China, smallholders' participation in cultivated land protection is currently low, so attention should be paid to the design of cultivated land protection policies for smallholders to guide them to implement large-scale operation and improve their organization. At the same time, smallholders' awareness of cultivated land protection can be improved by increasing the propaganda related to cultivated land protection. (2) Due to the particularity of land lease, professional farmers have weak awareness of farmland protection, so they should be guided to sign long-term contracts to stabilize the land use right. Professional farmers should also be treated as local residents regarding agricultural subsidies, technology promotion, children's education and other aspects, to improve regional identity and adaptability, and ultimately improve their CLPB. (3) It is necessary to formulate subsidy policies related to cultivated land protection, provide differentiated compensation to smallholders and professional farmers, and effectively improve the endogenous power of cultivated land protection. In addition, farmers' literacy and agricultural technology can be improved through rural night schools, short-term training, and internet usage.
Additionally, several aspects are worthy of further discussion for the current work. For example, other types of farmers could be included for comparative studies, or more factors influencing farmers' CLPB can be included. In view of this, a more completed and targeted questionnaire could be designed in future studies to explore more in-depth.
Conclusions
Based on the field research data of mango farmers in Hainan Province, this paper analyzed the differences and sources of differences in CLPB between smallholders and professional farmers using the Logit model and Fairlie decomposition method, and the following conclusions were obtained: Firstly, the mean values of CLPB of smallholders and professional farmers were 0.292 and 0.403, respectively, and were significant at the 5% level, showing the adoption ratio of organic fertilizer and soil testing and fertilizer application by the two types of farmers was relatively low. Secondly, education has a significant positive impact on both smallholders and professional farmers, while planting scale significantly negatively impacts the two types of farmers. Thirdly, there are differences in CLPB between smallholders and professional farmers, including differences in external characteristics based on observable factors such as family socio-economic characteristics and internal characteristics based on unobservable factors such as sense of identity, adaptability, responsibility, and belonging. Fourthly, 73.89% of the reasons for the differences in CLPB between smallholders and professional farmers are due to internal characteristics, while the remaining 26.11% are due to differences in external characteristics, of which planting years, annual household income and planting scale and planting scale contribute most.
Data availability statement
The raw data supporting the conclusions of this article will be made available by the authors, without undue reservation.
Author contributions
TX and HC: conceptualization and formal analysis. HC and DQ: methodology. TX and YJ: software. TX, HC, DQ, and FW: validation and writing—review and editing. TX and DQ: investigation and project administration. DQ and FW: resources and supervision. HC and YJ: data curation. TX, HC, and YJ: writing—original draft preparation. DQ: funding acquisition. All authors have read and agreed to the published version of the manuscript.
Funding
This research was supported by the National Natural Science Foundation of China (Nos. 72003054 and 72103052), National Banana Industry Technical System Industrial Economic Position (No. CARS-31-15), Hainan Provincial Natural Science Foundation of China (Nos. 720RC576 and 722RC630), and the Youth Project of Humanities and Social Science Foundation of the Ministry of Education (No. 19XJC790011).
Conflict of interest
The authors declare that the research was conducted in the absence of any commercial or financial relationships that could be construed as a potential conflict of interest.
Publisher's note
All claims expressed in this article are solely those of the authors and do not necessarily represent those of their affiliated organizations, or those of the publisher, the editors and the reviewers. Any product that may be evaluated in this article, or claim that may be made by its manufacturer, is not guaranteed or endorsed by the publisher.
Footnotes
1. ^Data from the Third Agricultural Census of China.
References
Abass, K., Adanu, S. K., and Agyemang, S. (2018). Peri-urbanisation and loss of arable land in Kumasi Metropolis in three decades: Evidence from remote sensing image analysis. Land Use Pol. 72, 470–479. doi: 10.1016/j.landusepol.2018.01.013
Abhilash, P. C., and Singh, N. (2009). Pesticide use and application: An Indian scenario. J. Hazard. Mater. 165, 1–12. doi: 10.1016/j.jhazmat.2008.10.061
Adnan, N., Nordin, S. M., Rahman, I., and Noor, A. (2017). Adoption of green fertilizer technology among paddy farmers: A possible solution for Malaysian food security. Land Use Pol. 63, 38–52. doi: 10.1016/j.landusepol.2017.01.022
Ajewole, O. C. (2010). Farmer's response to adoption of commercially available organic fertilizers in Oyo state, Nigeria. Afri. J. Agri. Res. 5, 2497–2503. Available online at: https://www.researchgate.net/publication/267939287
Alemu, G. T., Berhanie Ayele, Z., and Abelieneh Berhanu, A. (2017). Effects of land fragmentation on productivity in Northwestern Ethiopia. Adv. Agri. 2017, 4509605. doi: 10.1155/2017/4509605
Ayamga, M., Yeboah, R. W. N., and Ayambila, S. N. (2016). An analysis of household farm investment decisions under varying land tenure arrangements in Ghana. J. Agri. Rural Dev. Trop. Subtrop. 117, 21–34. Available online at: https://kobra.uni-kassel.de/handle/123456789/2015120349476
Bauer, T. K., and Sinning, M. (2008). An extension of the Blinder–Oaxaca decomposition to nonlinear models. Adv. Statist. Anal. 92, 197–206. doi: 10.1007/s10182-008-0056-3
Belachew, A., Mekuria, W., and Nachimuthu, K. (2020). Factors influencing adoption of soil and water conservation practices in the northwest Ethiopian highlands. Int. Soil Water Conservat. Res. 8, 80–89. doi: 10.1016/j.iswcr.2020.01.005
Blinder, A. S. (1973). Wage discrimination: Reduced form and structural estimates. J. Hum. Resour. 1973, 436–455. doi: 10.2307/144855
Boz, I. (2016). Effects of environmentally friendly agricultural land protection programs: Evidence from the Lake Seyfe area of Turkey. J. Integr. Agri. 15, 1903–1914. doi: 10.1016/S2095-3119(15)61271-0
Cai, J., Liao, H., Qiu, D., Yu, Y., and Luo, D. (2017). Urban integration and influence mechanism for city farmers in the reform of household registration: An investigation based on 472 “New Citizens” in the Main Urban Area of Chongqing. Hum. Geogr. 32, 46–52. doi: 10.13959/j.issn.1003-2398.2017.02.007
Chen, S. (2013). Change of farmers' emotion on land in the economic transition: A perspective of farmers' disparity. China Land Sci. 27, 35–41. doi: 10.13708/j.cnki.cn11-2640.2013.06.007
Chen, Y., Fu, X., and Liu, Y. (2022). Effect of farmland scale on farmers' application behavior with organic fertilizer. Int. J. Env. Res. Pub. He. 19, 4967. doi: 10.3390/ijerph19094967
Das, A. B., and Sahoo, D. (2012). Farmers' educational level and agriculture productivity: A study of tribals of KBK districts of Odisha. Int. J. Educ. Econ. Dev. 3, 363–374. doi: 10.1504/IJEED.2012.052312
Fagbamigbe, A. F., Adebola, O. G., Dukhi, N., Fagbamigbe, O. S., and Uthman, O. A. (2021). Exploring the socio-economic determinants of educational inequalities in diarrhoea among under-five children in low- and middle-income countries: a Fairlie decomposition analysis. Arch. Public. Health. 79, 114. doi: 10.1186/s13690-021-00639-8
Fairlie, R. W. (2005). An extension of the Blinder-Oaxaca decomposition technique to logit and probit models. J. Econ. Soc. Measur. 30, 305–316. doi: 10.3233/JEM-2005-0259
Fazal, S. (2000). Urban expansion and loss of agricultural land-a GIS based study of Saharanpur City, India. Environ. Urban. 12, 133–149. doi: 10.1177/095624780001200211
Gomiero, T. (2016). Soil degradation, land scarcity and food security: Reviewing a complex challenge. Sustainabil. Basel 8, 281. doi: 10.3390/su8030281
Haile, K. K., Tirivayi, N., and Tesfaye, W. (2019). Farmers' willingness to accept payments for ecosystem services on agricultural land: The case of climate-smart agroforestry in Ethiopia. Ecosyst. Serv. 39, 100964. doi: 10.1016/j.ecoser.2019.100964
Henriksson, R., Vincent, K., Archer, E., and Jewitt, G. (2021). Understanding gender differences in availability, accessibility and use of climate information among smallholder farmers in Malawi. Climate Dev. 13, 503–514. doi: 10.1080/17565529.2020.1806777
Jallow, M. F., Awadh, D. G., Albaho, M. S., Devi, V. Y., and Thomas, B. M. (2017). Pesticide risk behaviors and factors influencing pesticide use among farmers in Kuwait. Sci. Tot. Environ. 574, 490–498. doi: 10.1016/j.scitotenv.2016.09.085
Kaliba, A. R., Verkuijl, H., and Mwangi, W. (2000). Factors affecting adoption of improved maize seeds and use of inorganic fertilizer for maize production in the intermediate and lowland zones of Tanzania. J. Agri. Appl. Econ. 32, 35–47. doi: 10.1017/S1074070800027802
Lai, Z., Chen, M., and Liu, T. (2020). Changes in and prospects for cultivated land use since the reform and opening up in China. Land Use Pol. 97, 104781. doi: 10.1016/j.landusepol.2020.104781
Li, M., Yan, X., Guo, Y., and Ji, H. (2021). Impact of risk awareness and agriculture cooperatives' service on farmers' safe production behavior: Evidences from Shaanxi Province. J. Clean. Prod. 312, 127724. doi: 10.1016/j.jclepro.2021.127724
Lien, G., Flaten, O., Jervell, A. M., Ebbesvik, M., Koesling, M., and Valle, P. S. (2006). Management and risk characteristics of part-time and full-time farmers in Norway. Appl. Econ. Perspect. Pol. 28, 111–131. doi: 10.1111/j.1467-9353.2006.00276.x
Liu, B., and Fang, Y. (2021). The nexus between rural household livelihoods and agricultural functions: Evidence from China. Agriculture 11, 241. doi: 10.3390/agriculture11030241
Liu, Y., Guo, P., Ma, Z., and Hu, C. (2021). Research on the influencing factors of the willingness of new professional farmers to participate in skills training and its promotion during the development of urban modern agriculture. Converter 2021, 199–210. doi: 10.17762/converter.281
Lu, H., Xie, H., Lv, T., and Yao, G. (2019). Determinants of cultivated land recuperation in ecologically damaged areas in China. Land Use Pol. 81, 160–166. doi: 10.1016/j.landusepol.2018.10.052
Ma, W., Abdulai, A., and Goetz, R. (2018). Agricultural cooperatives and investment in organic soil amendments and chemical fertilizer in China. Am. J. Agri. Econ. 100, 502–520. doi: 10.1093/ajae/aax079
Ma, W., Grafton, R. Q., and Renwick, A. (2020). Smartphone use and income growth in rural China: Empirical results and policy implications. Electr. Commerce Res. 20, 713–736. doi: 10.1007/s10660-018-9323-x
Mosimane, A., Breen, C., and Nkhata, B. (2012). Collective identity and resilience in the management of common pool resources. Int. J. Commons. 6, 344–362. doi: 10.18352/ijc.298
Mponela, P., Tamene, L., Ndengu, G., Magreta, R., Kihara, J., and Mango, N. (2016). Determinants of integrated soil fertility management technologies adoption by smallholder farmers in the Chinyanja Triangle of Southern Africa. Land Use Pol. 59, 38–48. doi: 10.1016/j.landusepol.2016.08.029
Nastis, S. A., Mattas, K., and Baourakis, G. (2019). Understanding farmers' behavior towards sustainable practices and their perceptions of risk. Sustainabil. Basel 11, 1303. doi: 10.3390/su11051303
Nielsen, H. S. (1998). Discrimination and detailed decomposition in a logit model. Econ. Lett. 61, 115–120. doi: 10.1016/S0165-1765(98)00155-4
Noll, D., Dawes, C., and Rai, V. (2014). Solar community organizations and active peer effects in the adoption of residential PV. Energy Pol. 67, 330–343. doi: 10.1016/j.enpol.2013.12.050
Oaxaca, R. (1973). Male-female wage differentials in urban labor markets. Int. Econ. Rev. 1973, 693–709. doi: 10.2307/2525981
Prăvălie, R., Patriche, C., Borrelli, P., Panagos, P., Roşca, B., Dumitraşcu, M., et al. (2021). Arable lands under the pressure of multiple land degradation processes. A global perspective. Environ. Res. 194, 110697. doi: 10.1016/j.envres.2020.110697
Qiao, D., Li, W., Zhang, D., Yan, Y., and Xu, T. (2022). How do you want to restore?–Assessing the public preferences and social benefits of ecological restoration for natural rubber plantation in China. Front. Environ. Sci. 2022, 92. doi: 10.3389/fenvs.2022.823778
Rahman, K., and Zhang, D. (2018). Effects of fertilizer broadcasting on the excessive use of inorganic fertilizers and environmental sustainability. Sustainability 10, 759. doi: 10.3390/su10030759
Ros-Tonen, M. A., Bitzer, V., Laven, A., de Leth, D. O., Van Leynseele, Y., and Vos, A. (2019). Conceptualizing inclusiveness of smallholder value chain integration. Curr. Opin. Environ. Sustainabil. 41, 10–17. doi: 10.1016/j.cosust.2019.08.006
Shang, H., Yi, X., Yin, C., Chen, Y., and Zhang, Z. (2021). How does the stability of land management right (SLMR) affect family farms' cultivated land protection and quality improvement behavior (CLPQIB) in China? Land. 10, 1052. doi: 10.3390/land10101052
Su, M., Guo, R., and Hong, W. (2019). Institutional transition and implementation path for cultivated land protection in highly urbanized regions: A case study of Shenzhen, China. Land Use Pol. 81, 493–501. doi: 10.1016/j.landusepol.2018.11.015
Teshome, A., De Graaff, J., and Kassie, M. (2016). Household-level determinants of soil and water conservation adoption phases: Evidence from North-Western Ethiopian highlands. Environ. Manag. 57, 620–636. doi: 10.1007/s00267-015-0635-5
Tey, Y. S., Li, E., Bruwer, J., Abdullah, A. M., Brindal, M., Radam, A., et al. (2017). Factors influencing the adoption of sustainable agricultural practices in developing countries: A review. Environ. Eng. Manag. J. 16, 34. doi: 10.30638/eemj.2017.034
Tsusaka, T. W., Kajisa, K., Pede, V. O., and Aoyagi, K. (2015). Neighborhood effects and social behavior: The case of irrigated and rainfed farmers in Bohol, the Philippines. J. Econ. Behav. Org. 118, 227–246. doi: 10.1016/j.jebo.2015.04.022
Waithaka, M. M., Thornton, P. K., Shepherd, K. D., and Ndiwa, N. N. (2007). Factors affecting the use of fertilizers and manure by smallholders: The case of Vihiga, western Kenya. Nutr. Cycl. Agroecosyst. 78, 211–224. doi: 10.1007/s10705-006-9087-x
Wang, X., Zhang, J., He, K., and Li, W. (2021). Place attachment, environmental cognition and organic fertilizer adoption of farmers: Evidence from rural China. Environ. Sci. Pollut. Res. 28, 41255–41267. doi: 10.1007/s11356-021-13509-1
Xue, Y., Guo, J., Li, C., Xu, X., Sun, Z., Xu, Z., et al. (2021). Influencing factors of farmers' cognition on agricultural mulch film pollution in rural China. Sci. Tot. Environ. 787, 147702. doi: 10.1016/j.scitotenv.2021.147702
Yao, L., Zhao, M., and Xu, T. (2016). Study on residing village leaders' competence and effectiveness of poverty alleviation under precision match perspective. J. Nanjing Agri. Univ. 16, 86–95. Available online at: https://kns.cnki.net/kcms2/article/abstract?v=8hgYgJxiWjmhxLSSpauTNPPmV_iN1t_16jYnXd-G5UK4Ugwb0fz5n5UinYK-OL5Rze17wOAvFAyRmO8bEQqeHebQahq5Tinn2a9soPPj7CtVczkb65yWdTkNIBDAo0t4&uniplatform=NZKPT&language=CHS
Zeweld, W., Van Huylenbroeck, G., Tesfay, G., Azadi, H., and Speelman, S. (2018). Impacts of socio-psychological factors on actual adoption of sustainable land management practices in dryland and water stressed areas. Sustainabil. Basel 10, 2963. doi: 10.3390/su10092963
Zhang, Y., Lu, X., Zhang, M., Ren, B., Zou, Y., and Lv, T. (2022). Understanding farmers' willingness in arable land protection cooperation by using fsQCA: Roles of perceived benefits and policy incentives. J. Nat. Conserv. 68, 126234. doi: 10.1016/j.jnc.2022.126234
Zhao, D., Chen, Y., Parolin, B., and Fan, X. (2019). New Professional Farmers' Training (NPFT): A multivariate analysis of farmers' participation in lifelong learning in Shaanxi, China. Int. Rev. Educ. 65, 579–604. doi: 10.1007/s11159-019-09790-5
Zhong, Z., Qi, J., Shi, B., and Zhang, D. (2018). Do professional farmers have higher production efficiency? —Evidence from natural rubber growers in Yunnan and Hainan Provinces. J. Agrotech. Econ. 2018, 40–51. doi: 10.1080/00036846.2018.1543941
Keywords: cultivated land protection behaviors, difference, smallholders, professional farmers, Fairlie decomposition
Citation: Xu T, Chen H, Ji Y, Qiao D and Wang F (2023) Understanding the differences in cultivated land protection behaviors between smallholders and professional farmers in Hainan Province, China. Front. Sustain. Food Syst. 7:1081671. doi: 10.3389/fsufs.2023.1081671
Received: 27 October 2022; Accepted: 11 January 2023;
Published: 27 January 2023.
Edited by:
Cosmas Parwada, Zimbabwe Open University, ZimbabweReviewed by:
Grace Kanonge Mafaune, University of KwaZulu-Natal, South AfricaWanglin Ma, Lincoln University, New Zealand
Copyright © 2023 Xu, Chen, Ji, Qiao and Wang. This is an open-access article distributed under the terms of the Creative Commons Attribution License (CC BY). The use, distribution or reproduction in other forums is permitted, provided the original author(s) and the copyright owner(s) are credited and that the original publication in this journal is cited, in accordance with accepted academic practice. No use, distribution or reproduction is permitted which does not comply with these terms.
*Correspondence: Dan Qiao, cWlhb2RhbjExMjQmI3gwMDA0MDsxMjYuY29t; Fang Wang,
d2FuZ2Zhbmc3OTA4MTYmI3gwMDA0MDsxMjYuY29t