- 1Enabling Systems Transformation, International Crops Research Institute for the Semi-Arid Tropics, Hyderabad, Telangana, India
- 2Accelerated Crop Improvement, International Crops Research Institute for the Semi-Arid Tropics, Nairobi, Kenya
Groundnut crop is one of the major sources of financial and food security for a large number of Tanzanian smallholder farmers. However, the production of groundnuts in Tanzania is underdeveloped, and yields are reportedly 2.5 to 3 times lower than in other African nations such as Nigeria. There are a number of factors that contribute to lower yields including the cultivation of outdated plant varieties, increased climate variability, the infestation of pests and diseases, and the use of outdated farming techniques. To analyze the scope for increasing groundnut production, this study investigates and compares the technical efficiencies (TEs) and technological gap ratios (TGRs) in Tanzania's four main groundnut-producing regions, namely the Central zone, Lake Zone, Southern zone, Southern highland zone, by using a two-step meta frontier model. We used ICRISAT data collected under the Tropical Legume-III project during 2017–18. Our results show a very low level of technical efficiency of groundnut production in the regions and significant regional differences in TEs, TGRs, and Meta Technical Efficiencies (MTEs). The study identifies a tremendous scope to increase groundnut productivity and production in Tanzania by enhancing its production efficiency and the key drivers that may help harness this potential.
1. Introduction
Groundnuts are the second-most significant crop after soya beans for the production of oil seeds, the thirteenth-most significant crop for human consumption, and the third-most significant crop for the production of edible vegetable oil (Taphee et al., 2015; Upadhyaya and Dwivedi, 2015). The groundnut seed is an excellent nutrient-dense food that provides a high percentage of high-quality vegetable oil (48–50%), as well as protein (26–28%), dietary fiber, minerals (Ca, P, Mg, Zn, and Fe), and vitamins (E, K, and B complex) (Janila et al., 2013), and parts of the crop (haulms) are used as livestock feed. More than a hundred different countries across the tropics, subtropics, and warm temperate zones around the world cultivate groundnuts (Upadhyaya et al., 2012). Despite the fact that the production of groundnuts in East Africa is characterized by low input, low productivity, and inadequate market access (Giliomee, 1994; Carr, 2001), it plays an important role in achieving food security among low-income rural households. Though the production of groundnuts in African countries such as Tanzania takes place on a smaller scale under low input- low output systems (Taru et al., 2010) however it is one of the most significant crops for a large number of smallholder farmers in Tanzania and provides food, nutrition, and income for their households. Tanzania ranked 12th in groundnut production globally, producing 690,000 tons, 4% of Africa's production, and 1.29% of global production in 2020 (FAO, 2022). Tanzania's groundnut production is less developed than that of other African countries like Nigeria and Ethiopia, and Tanzania's yields are significantly lower than those of the other countries in the region. For example, in 2020, groundnut yield in the shell was 690 kg/ha in Tanzania, ranked 93rd, compared with 1,806 kg/ha in Ethiopia (38th) and 1,103 kg/ha in Nigeria (63rd) (FAO, 2022).
The groundnut yield in Tanzania has not increased substantially in the last couple of years; instead, it remained unstable. The groundnut production area (Figure 1) has increased by 754.7%, production by 1,226.92%, but yield increased by only 55.27% over 20 years from 2000 to 2020 [8]. However, the trends indicate that groundnut production is gaining importance in the country, but its growth is led by area growth, not productivity, thus a low efficiency of groundnut production.
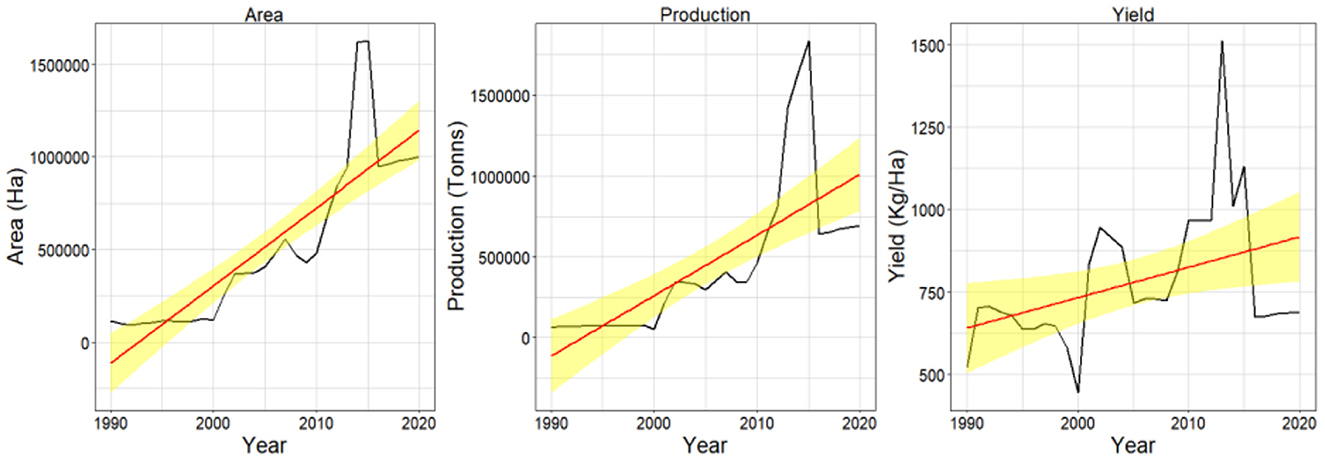
Figure 1. Trends in the groundnut production area, production, and yield in Tanzania from 1990 to 2020.
The yield of groundnuts in Tanzania significantly differs not only among different cultivars, but also across the different agroecological zones (Tulole, 2010). The lower yield levels are particularly attributed to unreliable rainfall, diseases and pests, low seed replacement rate, low-yielding varieties, below optimum plant size and population, and outdated agronomic practices like fertilizer broadcasting (NARI, 2010). A low groundnut yield has consequences in terms of poor economic returns, food insecurity, and households' low income, which might affect the sustainability of groundnut cultivation and trapping the farmers in poverty (Tittonell and Giller, 2013). According to different studies, many biotic and abiotic stresses have resulted in Tanzania's low groundnut production (Reddy et al., 2003; Daudi et al., 2018). There is another set of studies where researchers have focused on farm evaluation of potentially high-yielding groundnut cultivars for adoption and adaptation in Tanzania (Tulole, 2010; Daudi et al., 2018; Mwalongo et al., 2020; Lukurugu et al., 2021). The majority of these research efforts have been focused on agricultural technology, groundnut diseases, improved varieties, the climatic conditions that restrict groundnut production, and the contribution of groundnut to household income for eradicating poverty. However, a little emphasis has been given on analyzing the socioeconomic factors that maybe restricting groundnut production and yield among smallholder farmers in Tanzania (Ramadhani et al., 2002; Mangasini et al., 2014; FAO, 2022).
We hardly found any studies addressing the farmers' efficiency of groundnut production in Tanzania. When we talk about efficiency, we're referring to technical efficiency (TE). This refers to the degree of efficiency to which the given inputs are utilized in order to achieve a certain level of output. A farm is considered to have a reasonably high level of technical efficiency if it is able to generate comparatively a greater output while utilizing a given level of inputs (land, labor, capital, and technology, etc.).
Using the stochastic meta-frontier model developed by Huang et al. (2014), this study aims to fill in this knowledge gap by analyzing the socioeconomic challenges faced by smallholder groundnut producers and evaluating the technical efficiency and technology gap ratio1 of its production in various regions of Tanzania. This study analyzes socio-technical factors that limit the efficiency of groundnut production and generates evidence for policymakers at the local and national levels to develop short-and long-term policy responses to address the relevant constraints to improve the efficiency of groundnut production in Tanzania. This paper aims threefold: first is to identify the technical efficiency (TE) and technology gap ratio (TGR) of groundnut production for the four main zones of Tanzania, namely the central, lake, southern, and southern highland zones. Second, identify the factor that influences production and drives the inefficiency of groundnut production. Finally, determine the yield gap due to technical inefficiency and suggests required policy responses.
The rest of the paper is organized as follows. In Section 2, the theoretical model and methodology are described. Section 3 describes the data used and the variables. Following this, the results and discussion of empirical estimations are presented in Section 4. Finally, Section 5 discusses the conclusions and policy implications.
2. Materials and methods
We have used the meta frontier model to analyze the technical efficiency of Tanzania's groundnut production. The modern meta-frontier production function model was familiarized by Battese et al. (2004) and O'Donnell et al. (2008). Their model was estimated by a two-step process: In the first step, they estimated the group-specific frontier by using stochastic frontier (SF) regression. In the second step, they used mathematical programming. However, their second step has no statistical properties of the meta-frontier estimation results due to linear (or quadratic) programming algebraic calculation. Furthermore, no accounting for potentially different production environments facing firms (farm households) can be incorporated into the estimation, not to mention its incapability of isolating idiosyncratic shocks (Mwatawala and Kyaruzi, 2019). To overcome this problem, a new two-step SF method was developed by Huang et al. (2014) in order to estimate the group-specific frontiers and the meta-frontier, respectively, and to break down the various groups' efficiency scores into TE and TGs. The primary distinction between the new two-step SF approach and those of Battese et al. (2004) and O'Donnell et al. (2008) is that the former's second-step estimation of the meta-frontier is based on the SF framework rather than on a mathematical programming technique.
A general conventional stochastic production frontier model with j th production region and the i th decision making unit (DMU) or a firm in the t th period is given by:
Where yjit denotes the output level for farm i in the j th region in the t th period, Xjit is the input vector, Vjit represents the error term and is assumed to be iid as . Ujit representing technical inefficiency and is distributed as i.e., truncated from below at zero and with the mode at , where Zjits are some exogenous variables. A firm's technical efficiency (TE) in production is then defined as:
We employ the methodology developed by Huang et al. (2014) to estimate the stochastic meta-frontier function that encompasses all the frontiers of the k regions. In step 2 we, specify the following SFA:
where the are the predictions from the group frontiers from step 1 in (1).
As discussed in detail in Huang et al. (2014), at a given input level Xijt, the observed output Yjit of the ith farm relative to the meta-frontier consists of three components, that is where is technology gap ratio, is the firm's technical efficiency and is the random noise component.
Then, the two-step approach to estimating the meta-frontier as proposed by Huang et al. (2014) consists of two SFA regressions:
Where is the estimates of the group-specific frontier from the first step in Equation (4). Since the estimates are group-specific, the regression (4) is estimated J times, one for each group (j = 1,2,…,J). These estimates from all J groups are then pooled to estimate (5).
The meta-frontier should be larger than or equal to the group-specific frontier, i.e., , due to the error of estimating . However, the metafrontier should be larger than or equal to the group-specific frontier, The estimated TGR must always be less than or equal to unity,
Where are the estimated composite residuals of Equation (5). The TE of the ith farm to the meta-frontier is equal to the product of the estimate of the TGR in Equation (6) and the individual farm's estimated TE in Equation (2), that is,
In this study we used the Cobb-Douglas production function with cross-section data. The frontier (5) is specified as:
Where Yji is a vector of groundnut outputs, Xjim is a vector of inputs (m = 1, 2, …0.5) by farms (i = 1, 2, ….N) and the Greek letters are parameters to be estimated. Vji and Uji are the random error term in the model.
3. Variables and data description
Our study covers four main groundnut producing areas of Tanzania: the Central, Lake, Southern, and Southern highland zones. Data were collected under the TL III project2 during 2017–18 from 31 districts of the zones as mentioned earlier. In total of 702 randomly selected groundnut producing farm households from the four areas were surveyed under this project.
3.1. Main input and output variables
There are five input variables and one output variable in the data used in this analysis. Output includes annual groundnut production per household3. The output is measured in Kg. The Cobb–Douglas production function in the empirical model (7) is specified using the five input variables described next. Farmland use is defined as the size of productive land allocated to groundnut (both owned and rented) in acres; the seed is measured as the total seed (in kg) used in the production, and labor is measured as the total labor day used on the farm for groundnut production, including both family and hired labor. Fertilizer use (in kg)- di-ammonium phosphate (DAP) was used as fertilizer, and the number of oxen was used for plowing. Following Battese et al. (1996), O'Donnell et al. (2008), and Huang et al. (2014), we have used two dummy variables: D1 and D2 for fertilizer use and oxen use, respectively; D1 and D2 are 1 when fertilizer and ox are used in production otherwise 0 to deal with the zero observations for the fertilizer and oxen use input in the data set4.
3.2. Environmental variable
Existing literature and discussion with stakeholders helped us to identify potentially important factors that were used to analyze the efficiency of groundnut production in Tanzania. The empirical study was carried out using a two-step process. During the first stage, a number of environmental variables were taken into consideration in the development of group-specific (regional) and industry-specific (overall) frontiers. This was done in order to investigate the influence that environmental factors have on the effectiveness of groundnut production and the technological gaps. In this analysis, environmental variables were broken down into household- and region-specific variables in order to better understand how they indirectly affect the effectiveness of groundnut production. The former variables were employed in the initial estimation of the group borders in Equation (4), which affects the firm specific (farm household) technical efficiency. On the other hand, the latter kinds of variables were used in the second-step estimation of the meta-frontier in Equation (5), which characterizes the environment that influences the selection of production technologies.
Discussion with stockholders, including researchers, development actors, and farmers, and reviewing existing literature, we considered five firm-specific environmental variables: the Farmer's experience of growing groundnut, the proportion of women participation in agricultural activity in the farm family, the average age of household, average education of household, participation in the technology transfer program. Moreover, we also considered the following three industry (region)-specific environmental variables: the past 10 years' average annual rainfall and market distance from the farm household and a dummy variable for average rainfall, 1 if the annual rainfall is up to 1,000 mm and 0 if it is greater than 1,000 mm.
Table 1 summarizes the sample statistics of four regions, including the output, inputs, and environmental variables for each region.
4. Results and discussion
Groundnut has been one of the most important crops in Tanzania as a source of livelihood and food security for smallholder farmers. However, groundnut production in the country has significantly increased over the past two decades; however, the yields have increased only marginally (Figure 1). The average yield of the Southern Zone, which was highest across zones, was 562 kg per ha, followed by Central Zone (472 kg/ha), Lake Zone (464 kg/ha), and Southern Highland Zone (457/ha) with an overall average 477 kg/ha (Table 2). These current groundnut yields are far lower than those obtained in other countries such as Ethiopia and Kenya, indicating a huge scope to increase the yield and bridge the gaps.
The meta-frontier analysis for estimating the technical efficiency of production is relevant only when different study regions use different technology. If the groundnut production data are collected from a single production frontier, implying that they adopt the same underlying technology, estimating the meta-frontier production function would be unnecessary. To determine whether or not the production frontiers in the four Tanzanian zones are distinct, we used the likelihood ratio (LR) test5. The total of the three log-likelihood function values reported in Table 3 represents the unrestricted log-likelihood function under the null hypothesis as −541.72, whereas the restricted value is −596.96. The degrees of freedom for the chi-square distribution was equal to 48, which is the difference between the numbers of parameters estimated under the two hypotheses, respectively. This leads to the likelihood ratio statistic of 110.49, twice the difference between the unrestricted and the restricted log-likelihood function values. Since the LR test statistic exceeds the associated critical value at the 0.1% level6, the hypothesis is therefore absolutely rejected. This justifies the fact that the four regions are operating under heterogeneous technologies.
4.1. Input elasticities
Table 3 shows the estimation results7 for the group-specific frontier function for four regions, the pooled data model, and the meta-frontier model. Input use for all four individual regions, pooled data, and meta frontier the models exhibit positive (except D1, D2, and land use of the southern zone), and more than 85% of the parameter's estimates are statistically significant at a 10% level.
The land use coefficient was positive and significant except southern zone ranging from 0.3116 in Lake Zone to −0.1275 (not significant) in the southern zone. These positive and significant results indicate that the higher the allocation of land for groundnut by the farm household, the higher would be the production per unit of inputs. This finding aligns with other studies (Huang and Lai, 2017) on Tanzania's groundnut production, possibly due to the economies of scale.
Among other partial production input elasticities fertilizer use in four regions of Tanzania, the pooled data, and the meta frontier, were the biggest, positive, and highly significant at a 1% level and it ranged from 1.0186 in the southern zone to 3.1006 in the lake zone. These results imply that the percentage change in fertilizer use would significantly influence groundnut production more than the other farm inputs. This study is in line with the findings of Taphe et al. (2015), who found a robustly positive correlation between the output of groundnut and the use of agrochemicals by small-scale farmers in the state of Taraba in Nigeria. However, the corresponding dummy D1 for fertilizer use was negatively significant. These two fertilizer results indicate that fertilizer use positively influences groundnuts' productivity. However, the way some Tanzanian farmers are applying fertilizer was not enhancing the production of groundnuts. Most of the smallholder groundnut farmers in Tanzania are using broad-casting methods of fertilizer application which not only increases the cost of production, but the effectiveness of fertilizer becomes low. The micro-dosing fertilizer application method could be one way to improve fertilizer use efficiency and groundnut productivity. Therefore, the capacity building of the farmers on appropriate methods of need-based fertilizer use, improving their access to fertilizers, and appropriate small machines for fertilizer application would contribute to increased productivity.
The estimated elasticity of groundnut production for seed usage was significant and positive across the zone, and it ranged from 0.1982 in the lake zone to 0.7542 in the Southern Highland zone. After fertilizer use, the seed was the 2nd most crucial factor for ground-nut production in Tanzania. This result is commensurate with Shamsudeen et al. (2011) and Taphe et al. (2015), which found groundnut quantity and seed quality as the essential input factor among the other input variables that increase groundnut output in Sub-Saharan Africa. An increase in the quantity of seeds sown increases the groundnut plant population per acre. A suitable variety and plant stand are especially significant in rainfed regions like Tanzania. This is because, as plant population increases, additional nuts are produced from the added groundnut plants while keeping other factors constant. However, in Tanzania, poor plant stand is often observed in the field mainly due to poor quality of seed and moisture deficit during germination. Moreover, the seed replacement rate was also very low. Most of the farmers have been using locally available low-quality seeds. Due to a lack of knowledge and the absence of a low-cost storage facility, farmers could not store the groundnut seed even if they had a good quality harvest; as a result, they ended up using poor-quality seed.
Labor use was positively significant in all zones, including pooled data and meta frontier, with values ranging from 0.1180 in the central zone to 0.4857 in the southern zone. Labor use was the 3rd most important factor for groundnut production in Tanzania. Since the major allocation of labor and land goes to maize and rice crops, additional labor to the groundnut crop will likely improve its productivity. The findings of Reddy and Bantilan (2012) and Danso-Abbeam et al. (2015) are consistent with our findings that there is a positive correlation between labor and groundnut production. In addition, Asekenye (2012) found that there was a positive correlation between groundnut production and the amount of labor used by groundnut farmers in Kenya. Some of the studies found a positive and not statistically significant relation between labor use and groundnut production in Uganda. On the contrary, some authors have also found a negative relationship between output and labor (Shamsudeen et al., 2011).
Oxen use was positive and significant (except lake zone and pooled data model, where the coefficient was positive but not significant). This finding, then, should be considered in the background of previous empirical investigations that revealed a favorable and significant influence of oxen power usage on output (Hailemariam, 2015; Abate et al., 2019). However, the corresponding dummy D2 was negatively significant (except lake zone, where the sign was negative but not significant). These two results indicate that oxen use positively influenced groundnuts' productivity; however, it suggests a need for a more efficient allocation of oxen resources for groundnut production in all regions except the lake zone of Tanzania.
4.2. Technical efficiency, technology gap ratio, and meta technical efficiency
Table 4 reports the estimated TE Score, TGRs, and MTE scores for the four zones. Evidence from different studies of economic efficiency analysis suggests that worldwide, agricultural production achieves an average technical efficiency of around 70%. This value is significantly lower in many developing and underdeveloped countries (Bravo-Ureta and Pinheiro, 1993; Heshmati and Mulugeta, 1996; Seyoum et al., 1998; Sherlund et al., 2002; Linh, 2012). Our study of groundnut production in Tanzania found that the average group-specific TE score was very low, with an average mean TE score of 0.3035. This result implies that an average groundnut farmer produces 30.35% of the maximum possible (frontier) output, which the most efficient farmers produce in the study regions at a given level of input use. The implication of these results from the policy perspective is significant because, on average, farmers could increase their output by around 70% if all farmers become technically efficient, or they could produce the same output level with a much lower level of input use under the present situation. The average group-specific TE score was highest for the southern zone at 0.5494, ranging from 0.1253 to 0.9999, followed by the southern highland with an average score of 0.5326 ranging from 0.0547 to 0.9999, the lake zone region with an average score of 0.3053 ranging from 0.0220 to 0.9991, and lastly, the central zone had the least TE score of 0.1815 ranging from 0.0229 to 0.9938. The LR test for our model implies that farmers in the different regions do not use the same underlying technologies, and also, the average TE scores of these regions are quite low, ranging from 0.1815 to 0.5494. This result implies that most groundnut farmers lag far behind the technically efficient farmer producers in their region due to poor adoption of technologies and improved practices of production (Figure 2).
The stochastic meta frontier (SMF) estimates the mean value of the technology gap ratio (TGR) score was 0.9268 ranging from 0.6668 to 0.9870, which is not close to 1. A TGR value of 1 denotes a position or circumstance in which the individual regional frontier and the meta-frontier are coincident. It denotes a circumstance in which the most productive farmers in a certain area perform at a level comparable to the most productive farmers on a global (country) scale. A lower average value of TGR and its wider range across zones indicates a substantial difference in the level of technology use among the zones. The Central Zone had an average TGR of 0.9869, ranging from 0.9399 to 1, followed by the Lake Zone, Southern Zone, and Southern Highland Zone, where the average TGR is 0.9219, 0.8162, and 0.8109, respectively. The highest average TGR value for the Central Zone 0.9869 indicates that some farmers in the Central Zone were the technically most efficient farmers across all the zones and produce maximum output per unit of input at the current level of technology as indicated by the meta-frontier (Figure 2). However, its average TE score of 0.1815 was the lowest among the study zones. This shows a big scope for farmers to farmers learning and extensions not only within the zone but also across zones.
The relative MTE scores for different zones were similar to group-specific TE scores. The overall average MTE score is 0.2717 ranging from 0.0214 to 0.9842, which was very low. Overall, Southern Zone was more technically efficient than Southern Highland Zone, Lake Zone, and central zone in producing groundnut in Tanzania as measured by the MTE.
Although the LR test indicates that the four zones are not using the same technology. To examine whether the difference among the efficiency scores across four zones is statistically significant, we calculated the t statistics with the null hypothesis that there is no true mean difference. Based on the values of t statistics the null hypothesis was rejected at the 1% level (except Southern Zone vs. Southern Highland Zone are not significantly different). Thus, it is evident that all technical efficiency scores are not similar across zones (Table 5).
4.3. Determinants of firm and zone-specific efficiency
Table 3 summarizes the farm- and zone-specific technical inefficiency estimates as determined by various environmental variables. If an environmental variable has a negative (positive) coefficient, it suggests that the variable has a positive (negative) influence on the level of technical efficiency.
The farming experience was found to have a mixed sign; only in the Lake Zone did the experience of growing groundnut significantly positively influence the efficiency of groundnut production. However, in the southern zone, the experience of growing groundnut has negatively influenced the efficiency of groundnut production. The empirical evidence from the present study indicates that the farmers' experience of groundnut crop production might not always be an important factor influencing the efficiency of production especially when a crop is cultivated by a large number of farmers in the region/country such as groundnut in Tanzania. That allows the farmers to often learn from their peers. Similar finding was also reported by Kumar et al. (2022) while investigating efficiency of chickpea production in Ethiopia.
Both men and women cultivate and manage groundnut production in the country; however, the production of groundnuts relies heavily on women as the primary source of labor (Tulole, 2010). According to our findings, there is a strong and positive correlation between efficiency and the proportion of women participating in groundnut production. The coefficient estimates for the corresponding variable for all the regions and pooled data are negatively significant (except for the southern highland zone, where the sign is negative but not significant). This result indicates that the farms with the higher number of female participants in groundnut production had significantly higher technical production efficiency. Increased participation of women workers in groundnut production improves technical efficiency in the study areas. The men might have prioritized their time for dominant crops such as maize and rice, and they are often also involved in multiple off-farm activities, and greater engagement of family women might have helped in the timely completion of various groundnut production operations. Similar results have been shown in chickpea production in Ethiopia (Kumar et al., 2022).
The average age of the household members comes out with a significant negative effect on inefficiency; therefore, it could be interpreted as higher average age having a positive influence on on-farm production efficiency. We have considered the age as the average of the family members older than 18 years. This might be due to the low participation of younger members in groundnut production. Therefore, those households with a higher proportion of younger members may likely be relatively inefficient. These results are similar to other previous studies (Khan et al., 2022).
Formal education was found to have significantly enhanced the efficiency of groundnut production. Farmers with more education may have had easier access to and utilization of pertinent information from a variety of sources about crop production technology, inputs, prices, and market demand. The educated farmers were thus technically more efficient than those who did not attain education. These results agree with the other research work (Ali and Khan, 2014; Kebede et al., 2014; Mahgoup et al., 2017; Alemu et al., 2018; Dessale, 2019), which found a positive relationship between technical efficiency and years of education. According to the findings of these research, a rise in human capital boosts agricultural output. This is because farmers with higher levels of education are more innovative; they are better able to recognize, analyze, and react to new information; and they are better able to adopt newer technology, such as fertilizers, pesticides, planting materials, and improved agronomic methods much more quickly than their less educated counterparts.
Lastly, the training and participation of farmers in the technology dissemination program strongly and positively influenced groundnut production efficiency with high significance for all the zones and pooled data. This finding is supported by similar other studies undertaken in Sub Saharan Africa (Dibba et al., 2018; Anang et al., 2022). This finding shows the relevance of research and agricultural advisory services in enhancing groundnut production in Tanzania. It also highlights the necessity for policy assistance to create appropriate participatory technology development and dissemination initiatives to bridge yield gaps in groundnut to contribute to food security.
We also assessed region-specific determinants of technical efficiency. The market distance from the farmers' agricultural fields, average rainfall, and a dummy variable for rainfall8 up to 1,000 mm per year as one and zero otherwise. Distance to market from the agricultural field shows positive but not significant, implying shorter distance positively influences efficiency. Average rainfall shows positive and significant, implying that higher rainfall will badly affect groundnut production and efficiency in Tanzania. The negative influence of rainfall may be due to the negative impact of heavy rainfall events on groundnut production. This relationship was more clearly revealed from the dummy variable for rainfall up to 1,000 mm, which indicated that the zones with rainfall up to 1,000 mm per annum attained higher efficiency of groundnut production compared to higher rainfall regions. Although the groundnut is cultivated in Tanzania in the regions that have annual rainfall between 450 mm to 1,200 mm. However, the present evidence indicating a low efficiency of groundnut production in the region with more than 1,000 mm annual rainfall might be due to occurrence of water logging that may have adverse effect on yields (Tian et al., 2021).
5. Conclusion and policy implication
This study of technical efficiency analysis using the stochastic meta-frontier approach9 clearly shows that a large proportion of Tanzanian groundnut farmers were producing much lower outputs from a given level of inputs use or using more inputs to get the same level of output, compared to the best performing farmers in their respective zones. The evidence shows that groundnut farmers in all four zones were using available groundnut production technology sub-optimally at around a 30% level of technical efficiency. At the same time, the maximum value of TGR is 1 for the central zone, and the lake zone indicates that some farmers in these two zones, which on average were least technically efficient, were producing groundnut at their optimum level concerning the meta frontier. As a result, there is a tremendous opportunity to increase groundnut production in these zones by approximately twice as much if the technical efficiency of crop production can be improved. There is a need for a different strategy for the Central and Lake zones, where the majority of the farmers are least efficient in groundnut production, and the Southern and the southern highland zones, where farmers are relatively more efficient in groundnut production, may be willing to make more investment on groundnut technologies. According to our findings, improving the effectiveness of Tanzania's groundnut production might be accomplished by reorienting gender priorities at the farm level within the traditional framework of a male-dominated extension system. To increase groundnut production sustainably, it is necessary to refocus training efforts on women and conduct training that is gender-friendly (by modifying the approach, time, and location of training). Knowledge-based interventions that support women farmers and educate them on how much and how to apply each type of input would help Tanzania realize its full potential in groundnut production. Enabling farmers' participation in technology dissemination programs was a key factor in enhancing the efficiency of groundnut production in all the zones. Therefore, the policy supports the design of appropriate participatory technology development and dissemination programs that can significantly contribute to bridging the yield gaps in groundnut crops in the country. Other significant elements that contribute to enhancing output performance include access to manpower, small agricultural machinery, and oxen. To increase groundnut output, farmers' access to better seeds and fertilizers was crucial. However, there is a need to improve farmers' awareness of the proper use of fertilizers. Encouraging farmer or community-level seed production together with appropriate lowest-cost storage systems such as hermetic bags or structures could be one of the effective strategies for enhancing the access and adoption of improved cultivars. There is a need to undertake a crop suitability analysis; the zones with annual rainfall up to 1,000 mm were found to be more efficient; therefore, the high rainfall region should get low priority for groundnut production. It is possible to increase the efficiency of groundnut production to a great extent by expanding farmers' access to improved seeds, providing extension support that is need-based and gender-responsive, and encouraging farmers to participate in programmes that develop new technologies.
Though there were no significant variations in the average groundnut yield in four zones, we found a significant difference in TEs across regions. Following Moreira and Bravo-Ureta (2010), it may be suggested that adaptive research for development may provide the best outcomes for the less efficient areas/farmers far away from the meta frontiers. The research that helps in adapting the improved technologies and practices from the better performing areas and zones may contribute to increased efficiency of production. This would be a rational course of action to take in order to facilitate advancement toward the Meta Frontier. This would need strengthening of the agricultural extension system by improving the knowledge of the extension partners on different aspects of the groundnut value chain. And the policies are needed that provide incentives for the dissemination of accessible technology to remote places and farmers with low efficiency. It also emerges from the study that encouraging “farmer to farmer” extension that enables sharing of learnings and knowledge of the efficient farmers available in each zone, would considerably improve Tanzania's groundnut production efficiency.
Data availability statement
The raw data supporting the conclusions of this article will be made available by the authors, without undue reservation.
Author contributions
AD: conceptualization, methodology, validation, coding, formal analysis, investigation, data curation, and writing—original draft. SK: conceptualization, writing—review and editing, supervision, project administration, and funding acquisition. NG: writing—review and editing. All authors have read and agreed to the published version of the manuscript.
Funding
This research was funded by BMGF through Tropical Legumes-III (Grant ID-OPP1114827) and CRP Grain Legumes and Dryland Cereals (GLDC) through CGIAR Fund Donors and bilateral funding agreements.
Acknowledgments
The authors thank Chris Ojiewo for providing access to primary farm household data of Tanzania.
Conflict of interest
The authors declare that the research was conducted in the absence of any commercial or financial relationships that could be construed as a potential conflict of interest.
Publisher's note
All claims expressed in this article are solely those of the authors and do not necessarily represent those of their affiliated organizations, or those of the publisher, the editors and the reviewers. Any product that may be evaluated in this article, or claim that may be made by its manufacturer, is not guaranteed or endorsed by the publisher.
Footnotes
1. ^A production technology gap is a difference between the productivity achieved through the best technology represented in the meta frontier and the productivity achieved through chosen sub-technology represented in the group-specific frontier for a region. The technological gap ratio is the ratio of a certain farm's production frontier to the meta-frontier. (Mwatawala and Kyaruzi, 2019).
2. ^The goal of the Tropical Legumes programmes was to increase the amount of legumes produced and assist smallholder farmers in improving their standard of living. The project was carried out in three stages: TL I (from 2007 to 2011), TL II (from 2012 to 2014), and TL III (2015–2019). The project's operations were carried out in Sub-Saharan Africa's Burkina Faso, Ghana, Mali, Niger, Nigeria, Senegal, Ethiopia, Kenya, Malawi, Mozambique, Tanzania, Uganda, and Zimbabwe as well as South Asia's India and Bangladesh. The International Center for Tropical Agriculture (CIAT), International Institute of Tropical Agriculture (IITA), and International Crop Research Institute for the Semi-Arid Tropics (ICRISAT) worked with NARS partners to carry out this research, which was funded by the Bill & Melinda Gates Foundation. For more details, Available online at: https://www.icrisat.org/12-years-of-research-on-tropical-legumes/.
3. ^We added output from all the plots if a household has more than one plot.
4. ^Technically fertilizer use and oxen use data are not used directly in the model specifically, it is used in the form of Ln(max(fertilizer, 1−D1)) for fertilizer and Ln(max(ox, 1 − D2)) for oxen use.
5. ^The LR statistic is defined by , where ln LHo is the value of the log-likelihood function for the stochastic frontier estimated by pooling the data for all groups under the homoscedastic variance component, and ln LH1 is the sum of the values of the log-likelihood functions for the separate group frontiers (Battese et al., 1996, 2004; Rao and Coelli, 2004; Huang et al., 2014) with a Chi-square (χ2) distribution with degrees of freedom , where dim(.) is the dimension of the parameter (Rao, 2004).
6. ^Table value of Chi-square (χ2) distribution with degrees of freedom 48 at 0.1% level is 84.03.
7. ^We use the “frontier” package in R developed by Coelli and Henningsen (2013).
8. ^Annual rainfall required for groundnut production in Tanzania is 750-1,200 mm depending on the soil types and drainability.
9. ^Production frontiers are defined by the model and within the sample values. This implies that there may be techniques of production not practiced by any of the farmers in the sample, which could yield a much higher output for the same input level.
References
Abate, T. M., Dessie, A. B., and Mekie, T. M. (2019). Technical efficiency of smallholder farmers in red pepper production in North Gondar zone Amhara regional state, Ethiopia. J. Econ. Struct. 8, 1–18. doi: 10.1186/s40008-019-0150-6
Alemu, M. D., Tegegne, B., and Beshir, H. (2018). Technical efficiency in Teff (Eragrostisteff) production: the case of smallholder farmers in Jamma district, South Wollo Zone, Ethiopia. J. Agric. Econ. Rural Develop. 4, 513–519.
Ali, S., and Khan, M. (2014). Technical efficiency of wheat production in district Peshawar, Khyber Pakhtunkhwa, Pakistan. Sarhad J. Agric. 30, 433–441.
Anang, B. T., Dokyi, E. O., Asante, B. O., and Donkoh, S. A. (2022). Technical efficiency of resource-poor maize farmers in northern Ghana. Open Agric. 7, 69–78. doi: 10.1515/opag-2022-0075
Asekenye, C. (2012). An analysis of productivity gaps among smallholder Groundnut farmers in Uganda and Kenya. Master's Theses. University of Connecticut Graduate School.
Battese, G. E., Malik, S. J., and Gill, M. A. (1996). An investigation of technical inefficiencies of production of wheat farmers in four districts of Pakistan. J. Agric. Econ. 47, 37–49. doi: 10.1111/j.1477-9552.1996.tb00670.x
Battese, G. E., Rao, D. S., and O'donnell, C. J. (2004). A metafrontier production function for estimation of technical efficiencies and technology gaps for firms operating under different technologies. J. Produc. Analy. 21, 91–103. doi: 10.1023/B:PROD.0000012454.06094.29
Bravo-Ureta, B. E., and Pinheiro, A. E. (1993). Efficiency analysis of developing country agriculture: a review of the frontier function literature. Agric. Resour. Econ. Rev. 22, 88–101. doi: 10.1017/S1068280500000320
Carr, S. J. (2001). Changes in African smallholder agriculture in the twentieth century and the challenges of the twenty first. African Crop Sci. J. 9, 331–338. doi: 10.4314/acsj.v9i1.27655
Coelli, T., and Henningsen, A. (2013). Frontier: Stochastic Frontier Analysis. R package version 1.1-0. Available online at: https://CRAN.R-Project.org/package=frontier (accessed October 14, 2021).
Danso-Abbeam, G., Dahamani, A. M., and Bawa, G. A. (2015). Resource-use-efficiency among smallholder groundnut farmers in Northern Region, Ghana. Am. J. Exper. Agric. 6, 290. doi: 10.9734/AJEA/2015/14924
Daudi, H., Shimelis, H., Laing, M., Okori, P., and Mponda, O. (2018). Groundnut production constraints, farming systems, and farmer-preferred traits in Tanzania. J. Crop Improve. 32, 812–828. doi: 10.1080/15427528.2018.1531801
Dessale, M. (2019). Analysis of technical efficiency of small holder wheat-growing farmers of Jamma district, Ethiopia. Agric. Food Secur. 8, 1–8. doi: 10.1186/s40066-018-0250-9
Dibba, L., Zeller, M., and Diagne, A. (2018). The Impact of Agricultural Training on the Technical Efficiency of Smallholder Rice Producers in the Gambia. Mod. Concep. Dev. Agrono. 2, 537. doi: 10.31031/MCDA.2018.02.000537
FAO. (2022). Crops and livestock products. License: CC BY-NC-SA 3.0 IGO. Available online at: https://www.fao.org/faostat/en/#data/QCL (accessed August 28, 2022).
Giliomee, J. H. (1994). “Integrated pest management in the African context,” in African Crop Science Conference Proceedings 346–349.
Hailemariam, L. (2015). Technical efficiency in teff production: The case of Bereh District, Oromia National Regional State, Ethiopia. Master's Thesis. Haramaya University, Ethiopia.
Heshmati, A., and Mulugeta, Y. (1996). Technical efficiency of the Ugandan matoke farms. Appl. Econ. Lett. 3, 491–494. doi: 10.1080/758540813
Huang, C. J., Huang, T. H., and Liu, N. H. (2014). A new approach to estimating the metafrontier production function based on a stochastic frontier framework. J. Product. Analy. 42, 241–254. doi: 10.1007/s11123-014-0402-2
Huang, C. J., and Lai, H. P. (2017). A note on the likelihood ratio test on the equality of group frontiers. Econ. Lett. 155, 5–8. doi: 10.1016/j.econlet.2017.02.037
Janila, P., Nigam, S. N., Pandey, M. K., Nagesh, P., and Varshney, R. K. (2013). Groundnut improvement: use of genetic and genomic tools. Front. Plant Sci. 4, 23. doi: 10.3389/fpls.2013.00023
Kebede, T., Berhane, G., and Gebru, M. (2014). Technical efficiency in teff production by small scale farmers in Tigray. Int. J. Res. 4, 85–97.
Khan, S., Shah, S. A., Ali, S., Ali, A., Almas, L. K., and Shaheen, S. (2022). Technical efficiency and economic analysis of rice crop in khyber pakhtunkhwa: a stochastic frontier approach. Agriculture 12, 503. doi: 10.3390/agriculture12040503
Kumar, S., Das, A., Hauser, M., Muricho, G., Degefu, T., Fikre, A., et al. (2022). Estimating the potential to close yield gaps through increased efficiency of chickpea production in Ethiopia. Food Secur. 14, 1241–1258. doi: 10.1007/s12571-022-01285-w
Linh, V. H. (2012). Efficiency of rice farming households in Vietnam. Int. J. Develop. Issues. 11, 60–73. doi: 10.1108/14468951211213868
Lukurugu, G. A., Mponda, O. K., Akpo, E., Monyo, E. S., Nzunda, J., Daudi, H., et al. (2021). “Groundnut seed production and distribution through multi-stakeholder platforms in southern region of Tanzania,” in Enhancing Smallholder Farmers' Access to Seed of Improved Legume Varieties Through Multi-stakeholder Platforms (Singapore: Springer) 9–30. doi: 10.1007/978-981-15-8014-7_2
Mahgoup, O. B., Ali, A. E. S., and Mirghani, A. O. (2017). Technical efficiency analysis of groundnut production in the gezira scheme, Sudan. Int. J. Sci. Res. Publications 7, 6103.
Mangasini, A. K., Mwanahawa, L. M., Arbogast, G. M., and Neema, P. K. (2014). Socio economic Factors Limiting Smallholder Groundnut Production in Tabora Region. Research report 14/1. Available online at: https://www.repoa.or.tz/wp-content/uploads/2 (accessed July 27, 2020).
Moreira, V. H., and Bravo-Ureta, B. E. (2010). Technical efficiency and metatechnology ratios for dairy farms in three southern cone countries: a stochastic meta-frontier model. J. Produc. Analy. 33, 33–45. doi: 10.1007/s11123-009-0144-8
Mwalongo, S., Akpo, E., Lukurugu, G. A., Muricho, G., Vernooy, R., Minja, A., et al. (2020). Factors influencing preferences and adoption of improved groundnut varieties among farmers in Tanzania. Agronomy 10, 1271. doi: 10.3390/agronomy10091271
Mwatawala, H. W., and Kyaruzi, P. P. (2019). An exploration of factors affecting groundnut production in central tanzania: empirical evidence from Kongwa District, Dodoma Region. Int. J. Progr. Sci. Technol. 14, 120–130.
NARI. (2010). Naliendele Agricultural Research Institute, Ministry (NARI). Agricultural research Annual Report. Government printer, Dares salaam, Tanzania.
O'Donnell, C. J., Rao, D. S., and Battese, G. E. (2008). Metafrontier frameworks for the study of firm-level efficiencies and technology ratios. Empir. Econ. 34, 231–255. doi: 10.1007/s00181-007-0119-4
Ramadhani, T., Otsyina, R., and Franzel, S. (2002). Improving household incomes and reducing deforestation using rotational woodlots in Tabora district, Tanzania. Agric. Ecosyst. Environ. 89, 229–239. doi: 10.1016/S0167-8809(01)00165-7
Rao, D. P., and Coelli, T. J. (2004). Catch-up and convergence in global agricultural productivity. Indian Econ. Rev. 39, 123–148.
Rao, D. S. (2004). The country-product-dummy method: A stochastic approach to the computation of purchasing power parities in the ICP. CEPA Working Paper Series No. 03/2004.
Reddy, A. A., and Bantilan, M. C. S. (2012). Competitiveness and technical efficiency: Determinants in the groundnut oil sector of India. Food Policy 37, 255–263. doi: 10.1016/j.foodpol.2012.02.004
Reddy, T. Y., Reddy, V. R., and Anbumozhi, V. (2003). Physiological responses of groundnut (Arachis hypogea L.) to drought stress and its amelioration: a critical review. Plant Growth Regul. 41, 75–88. doi: 10.1023/A:1027353430164
Seyoum, E. T., Battese, G. E., and Fleming, E. M. (1998). Technical efficiency and productivity of maize producers in eastern Ethiopia: a study of farmers within and outside the Sasakawa-Global 2000 project. Agric. Econ. 19, 341–348. doi: 10.1111/j.1574-0862.1998.tb00536.x
Shamsudeen, A., Donkoh, S. A., and Sienso, G. (2011). Technical efficiency of groundnut production in West Mamprusi District of Northern Ghana. J. Agric. Biol. Sci. 2, 71–77.
Sherlund, S. M., Barrett, C. B., and Adesina, A. A. (2002). Smallholder technical efficiency controlling for environmental production conditions. J. Develop. Econ. 69, 85–101. doi: 10.1016/S0304-3878(02)00054-8
Taphe, B. G., Agbo, F. U., and Okorji, E. C. (2015). Resource productivity and technical efficiency of small scale groundnut farmers in Taraba State, Nigeria. J. Biol. Agric. Healthcare 5, 25–35.
Taphee, G. B., Jongur, A. A. U., Giroh, D. Y., and Jen, E. I. (2015). Analysis of Profitability of Groundnut Production in Northern Part of Taraba State, Nigeria. Int. J. Compu. Applic. 125, 34–39. doi: 10.5120/ijca2015905545
Taru, V. B., Kyagya, I. Z., and Mshelia, S. I. (2010). Profitability of groundnut production in Michika local government area of Adamawa state, Nigeria. J. Agric. Sci. 1, 25–29. doi: 10.1080/09766898.2010.11884650
Tian, L. X., Zhang, Y. C., Chen, P. L., Zhang, F. F., Li, J., Yan, F., et al. (2021). How does the waterlogging regime affect crop yield? A global meta-analysis. Front. Plant Sci. 12:634898. doi: 10.3389/fpls.2021.634898
Tittonell, P., and Giller, K. E. (2013). When yield gaps are poverty traps: The paradigm of ecological intensification in African smallholder agriculture. Field Crops Res. 143, 76–90. doi: 10.1016/j.fcr.2012.10.007
Tulole, L. B. (2010). The groundnut client oriented research in Tabora, Tanzania. Afr. J. Agric. Res. 5, 356–362.
Upadhyaya, H. D., and Dwivedi, S. L. (2015). “Global perspectives on groundnut production, trade, and utilization: Constraints and opportunities,” in National Seminar on Technologies for Enhancing Oilseeds Production Through NMOOP (Hyderabad: PJTSAU).
Keywords: groundnut production, Tanzania, technical efficiency, stochastic meta-frontier, technology gap
Citation: Das A, Kumar S and Ganga Rao NVPR (2023) Potential for increasing groundnut production in Tanzania by enhancing technical efficiency: A stochastic meta-frontier analysis. Front. Sustain. Food Syst. 7:1027270. doi: 10.3389/fsufs.2023.1027270
Received: 24 August 2022; Accepted: 14 March 2023;
Published: 03 April 2023.
Edited by:
Francis Tetteh, Council for Scientific and Industrial Research (CSIR), GhanaReviewed by:
Chrilukovian Wasike, Maseno University, KenyaA Amarender Reddy, National Institute of Agricultural Extension Management (MANAGE), India
Copyright © 2023 Das, Kumar and Ganga Rao. This is an open-access article distributed under the terms of the Creative Commons Attribution License (CC BY). The use, distribution or reproduction in other forums is permitted, provided the original author(s) and the copyright owner(s) are credited and that the original publication in this journal is cited, in accordance with accepted academic practice. No use, distribution or reproduction is permitted which does not comply with these terms.
*Correspondence: Shalander Kumar, ay5zaGFsYW5kZXJAY2dpYXIub3Jn