- 1Faculty of Entrepreneurship, University of Tehran, Tehran, Iran
- 2Department of Management, Faculty of Social Sciences and Economics, Alzahra University, Tehran, Iran
- 3Zhejiang University City College, Hangzhou, China
Introduction: The main purpose of this study is to extract the rules and patterns governing the behavioral intention of consumers towards the adoption of genetically modified foods (GMFs).
Method: The proposed method is a combination of Rough Set Theory (RST) and Flow Network Graph (FNG). Data was collected from 386 consumers to extract rough rules. 13 rules have been chosen from 289 original rules that were divided into three groups: low, medium, and high intention to use GMFs. They were chosen because of the support values and other indexes that were used in the RST. Eventually, to interpret the performance of the generated rules, FNG were illustrated for each decision-making class, and seven patterns were extracted.
Results: The findings confirm that corporate social responsibilities, consumer concerns, occupational status, and consumer autonomy are more important than other observed dimensions in consumers' decision-making. Moreover, the findings illustrate that combining Rough Set Theory and Flow Network Graph could predict customers' intentions and provide valuable information for policy-makers in related active industries.
Discussion: Based on the analysis outcomes, the most significant factors that affect consumers' intention to use GMFs are: “consumer perception of CSR”; “consumer concerns”; “occupational status”; and “consumer autonomy”. Thus, managers and policymakers must pay more attention to these concepts when they survey consumer intention behavior.
1. Introduction
Food security is one of the most challenging topics globally (Akbari et al., 2022a), and genetically modified foods (GMFs) remain a contentious issue worldwide (Boccia and Punzo, 2021) but the growing population of the world and the increasing demand for food in recent decades have led to the use of new genetic methods in the production of foods called GMFs (Erol, 2021; Ferrari et al., 2021; Owusu and Kwesi Ndzebah Dadzie, 2021). GMFs refers to modifying or improving the genetic structures of foods by the application of modern biotechnological methods. GMFs are applicable in many sectors, such as animal husbandry, the health system, and especially the agriculture and food sectors (Lefebvre et al., 2019; Guo et al., 2020; Erol, 2021). GMFs have a production method that production method of GMFs resembles natural foods. GMFs have a comparative advantage such as resistance to pests and insects, high yield, better nutritional value, etc., which are caused by changes in undesirable genes (Erol, 2021; Ferrari et al., 2021; Hwang and Nam, 2021; Levi, 2022).
Although the utilization of new biotechnology for food production has opened new opportunities for the food industry, raised numerous questions about the potential dangers of producing and consuming such foods (Ortega et al., 2022) and have raised numerous questions about their potential effects, such as allergenicity, increased resistance to antibiotics, destructive environmental effects, and horizontal gene transfer in society. Therefore, the acceptance of GMFs depends on the consumers' perception of the risks and benefits of these food products (Boccia, 2016; Deng and Hu, 2019; Erol, 2021; Hwang and Nam, 2021; Owusu and Kwesi Ndzebah Dadzie, 2021; Ortega et al., 2022). Therefore, despite the potential perceived advantages and disadvantages of GMFs mentioned in the literature, public opinion on GMFs remains largely skeptical and controversial (Miles et al., 2005; Lusk, 2011; Varzakas and Tzanidis, 2015; Boccia et al., 2018; Lefebvre et al., 2019; Guo et al., 2020; Xu et al., 2020; Boccia and Punzo, 2021; Fozouni Ardekani et al., 2021; Owusu and Kwesi Ndzebah Dadzie, 2021; Levi, 2022; Ortega et al., 2022). This is the main reason why the GMFs literature includes conflicting conclusions on consumer response to gene technology and especially to GMFs (Xu et al., 2020; Boccia and Punzo, 2021).
Various research has been done in the field of customer behavior concerning GMFs with different perspectives like consumer response, consumers knowledge, consumer perception, consumer acceptance, consumer trust, risk perception, and corporate social responsibilities (CSR; Deng and Hu, 2019; Ardebili and Rickertsen, 2020; Xu et al., 2020; Ferrari et al., 2021; Fozouni Ardekani et al., 2021; Hwang and Nam, 2021; Vindigni et al., 2022). Although there are several studies on GMFs that include some influential determinants of consumer behavior, still the field of consumer behavior toward GMFs is assumed to be uncertain and this uncertainty makes it difficult to provide solutions for the development of relevant industries (Rodríguez-Entrena and Salazar-Ordóñez, 2016; Guo et al., 2020; Erol, 2021). In this regard, the purpose of this study is to investigate the acceptance of GMFs while considering influential consumer behavior factors such as corporate social responsibilities (CSR), Regulatory Focus Theory (RFT), and Self-Determination Theory (SDT) in order to assist the literature and related industry in clarifying consumer behavior toward GMF. Hence, the combined model of Rough Set Theory (RST) and Flow Network Graph (FNG) is used to generate rules and demonstration that exist among these factors.
This study provides a noticeable contribution to the existing literature on GMFs. Consumers' behavior is considered a noticeable determinant for the future of GMFs and their applications, but the number of studies conducted on this subject is very limited in developing countries (Akbari et al., 2019; Owusu and Kwesi Ndzebah Dadzie, 2021). So, we chose Iran to enrich the related literature on this subject in developing countries. Furthermore, the existing literature on consumer behavior and GMFs includes studies that have investigated the effects of one or some factors on consumer behavior toward GMFs separately. With the means of RST and FNG, not only influential factors were studied together, but also some rules were extracted that have a meaningful role in predicting consumer behavior toward GMFs. Regarding literature, the field of consumer behavior toward GMFs is assumed to be uncertain due to the different results of studies in this field, which makes it difficult to provide solutions for the development of relevant industries (Rodríguez-Entrena and Salazar-Ordóñez, 2016; Guo et al., 2020; Erol, 2021). Here, using RST is a privilege since it is a method that is known for its clear and straightforward outputs, which are demonstrated through rules and show the way. So, predicting consumer behavior toward GMFs by the means of RST is important from a macro perspective since it will help governmental policymakers, farmers, and agricultural biotechnology firms make effective decisions for the future of this industry. Finally, it is also worthwhile to mention that, to the best of our knowledge, none of the previous studies on GMFs used RST for their investigations.
This paper is organized as follows: Section 2 covers a background on the consumers' acceptance of GMFs. The elaboration of RST, FNG, and their combinations are mentioned in the methodology. Section 4 includes the results obtained from the combined model of RST and FNG. Eventually, in Section 5, the conclusion and recommendations for future studies will be delivered.
2. Theoretical background of GMFs
Consumer behavior is one of the influential factors that are important in product success and growth. In this regard, consumer demand is also a key factor shaping the government and firms' strategies or policies and is a determinant for GMFs' acceptance in the market (Deng and Hu, 2019; Ortega et al., 2022). According to Siegrist and Hartmann (2020), due to the lack of knowledge about most novel food production technologies, the term of “black box” is used for consumer demand since it is influenced by simple heuristics and is unstable in different situations.
In the GMF literature, there are plenty of studies that investigate the attractiveness, or better to say acceptance of GMFs due to some determinants like culture, sexuality, age, risks and benefits, concerns, CSR, and knowledge (Siegrist and Hartmann, 2020; Boccia and Punzo, 2021; Fozouni Ardekani et al., 2021; Pruitt et al., 2021; Rabbanee et al., 2021; Zheng and Wang, 2021). Siegrist and Hartmann (2020) showed in their study that cultural issues are related to the acceptance of various risks. In that direction, Soroka et al. (2021) demonstrated that consumer's attitude is different from country to country in the European market. A bulk of studies on GMFs found that Asian consumers were unsure about how to use GMFs because there were only a few studies about them (Siegrist and Hartmann, 2020; Fozouni Ardekani et al., 2021). A study found that education of students about GMF leads to improvement of their scores of knowledge and attitude (Asadi et al., 2021).
According to Siegrist and Hartmann (2020), consumer intentions are also influenced by food neophobia, disgust sensitivity, and cultural values. It is not only true for GMFs but also for novel food technologies. The findings of Fozouni Ardekani et al. (2021) discovered that net benefit and compatibility are significant antecedents of consumers to accept GMFs. Additionally, self-efficacy has an indirect influence on acceptance of GMFs. Akbari et al. (2019) found attitude toward GMFs, trust in GMFs, concerns about GMFs, and CSR the most significant variables of buying intentions for GMFs. Another study confirmed the significant influence of CSR in the relationship between trust in GMFs and consumers' intention toward such products. They have emphasized the role of attitude which is considered the main predictor of intention. Also, they concluded that Iranian consumers have a relatively negative approach to GMFs. Furthermore, they found some differences between males and females for their promotion focus, perceived behavior control, and concerns about choosing GMFs. Attitudes toward GMFs are also determined by environmental concern and objective knowledge (Ferrari et al., 2021). Regarding sexuality effects, Rabbanee et al. (2021) concluded that female consumers in comparison to their male counterparts pose relatively more favorable attitudes and repurchase intention.
GMFs, besides all their new opportunities for the food industry, have led to concerns among consumers due to their perceived potential dangers (Ortega et al., 2022) which have raised numerous questions about their potential side effects, such as allergies, increased resistance to antibiotics, destructive environmental effects, and horizontal gene transfer in society. So, the acceptance of GMFs depends on the risks and benefits that consumers perceive (Boccia, 2016; Deng and Hu, 2019; Erol, 2021; Hwang and Nam, 2021; Owusu and Kwesi Ndzebah Dadzie, 2021; Ortega et al., 2022). Yet, consumers' behavior toward GMFs is vague due to the quite complex technology behind their production (Deng et al., 2019; Palmieri et al., 2020; Siegrist and Hartmann, 2020; Boccia and Punzo, 2021). In this regard, an investigation revealed that a negative approach toward GMFs is related to a lack of knowledge about genetic modifications and their effects (Palmieri et al., 2020). In this case, Ortega et al. (2022) reported that decreasing consumers' fear of novel food technologies improves consumer valuation and acceptance of GMFs.
Consumers would like to make an informed choice. Not only does the existence of labels include product information, but also the amount of time consumers spend reading label information is an important aspect in determining consumers' intentions. It helps consumers in their decisions to buy food products (Macall et al., 2021). Within the scope of GMFs labels, Zheng and Wang (2021) described using a labeling system for differentiating GMFs as important since it is considered one of the most influential factors that drive the buying intention. Similarly, Pruitt et al. (2021) supported consumers knowing that clear health benefits are more accepting of biotechnology products. In addition, Ardebili and Rickertsen (2020) found that information is an important factor that can change attitudes toward GMFs and reduce resistance to these novel technologies over time. To elaborate on the influence of knowledge, Hwang and Nam (2021) showed that higher education and income cause overestimation of actual knowledge level, which subsequently leads to higher risk perception, lower benefit perception, and lower intention to use. Regarding risk perception, Hakim et al. (2020) concluded that risk perception is being moderated by social trust and perceived quality, and as a whole, willingness to pay for GMFs is influenced by price (Hakim et al., 2020; Macall et al., 2021). Finally, Deng and Hu (2019) in this field conclude that safety issues are the main barrier to the acceptance of GMFs by consumers.
Therefore, this paper aims to investigate the acceptance of GMFs considering influential factors of consumer behavior like socio-demographics, CSR, concern, promotion, and prevention focus, autonomy, competence, and relatedness to help the literature and related industry consequently in clarifying consumer behavior toward GMFs. Hence, a combined model of RST and FNG is used to generate rules and demonstrate relations that exist among these factors. For this, the intention to use GMFs was used to measure consumer behavior output because intention influences willingness to fulfill a behavior (Jiang et al., 2016; Fozouni Ardekani et al., 2021). The independent variables cover the determinants of RFT, SDT, and consumers' concerns toward GMFs as psychographic variables. Also, in this study, the determinants of CSR, including social equity, environmental, economic, legal, ethical, and philanthropic factors, determine the role of a social variable in consumers' intention to use GMFs.
As mentioned before, various studies have been conducted in the field of consumer behavior toward GMFs with different perspectives like consumer response, consumer knowledge, consumer perception, consumer acceptance, consumer trust, risk perception, and CSR (Deng and Hu, 2019; Ardebili and Rickertsen, 2020; Xu et al., 2020; Ferrari et al., 2021; Fozouni Ardekani et al., 2021; Hwang and Nam, 2021; Akbari et al., 2022b) and again as it is shown in Table 1, there are several studies on GMFs that include some influential determinants of consumer behavior but still the field of consumer behavior toward GMFs is assumed to be uncertain and this uncertainty makes it difficult to provide solutions for the development of relevant industries (Rodríguez-Entrena and Salazar-Ordóñez, 2016; Guo et al., 2020; Erol, 2021). In this regard, here we are going to investigate the acceptance of GMFs while considering influential consumer behavior factors such as corporate social responsibilities (CSR), Regulatory Focus Theory (RFT), and Self-Determination Theory (SDT) in order to assist the literature and related industry in clarifying consumer behavior toward GMF. For this, we used the combined model of Rough Set Theory (RST) and Flow Network Graph (FNG) in order to generate rules and demonstration that exist among these factors.
3. Research methodology
To meet the purpose of this study, the proposed methodology approach for this research combines RST and FNG. Toward that direction, we consider questionnaire-based survey research according to the practical concept of this research problem that occurs in daily life. This part encompasses (1) variables' selection and item measurement; (2) population and sample; and (3) analytical methods.
3.1. Population and sample
For conducting this survey, the population was consumers in Tehran (Iran's capital) who have experienced at least once consuming GMFs. Since we have assumed the approximate size of the population to be unlimited, the sample size is determined by considering the confidence level of 95% and the usage of the Cochran formula, the sample size is 385. For selecting the study consumers, the multistage stratified random sampling method was used for selecting the study consumers. After pre-processing the data, 386 complete questionnaires in total were collected from GMFs users.
3.2. Variables selection and item measurement
First, based on the literature review, the most important variables have been extracted. Then we assure the validity of the selected variables by inviting experts to interview them, including four university scholars and four industry managers. These managers had an average of over 5 years of experience in GMF firms. The experts were requested to identify influential factors in choosing GMFs and evaluate the importance of selected variables as the main criteria.
In the second step, decision and conditional variables, two types of variables in RST logic, were determined based on the extracted variables in the previous step. It is considered that in RST we could be using both quantitative and qualitative variables simultaneously, which is one of the most important advantages of this approach (Wang et al., 2010). Hence, to determine the rules of consumers' behavior and performance in the GMFs market, 12 conditional variables consisting of demographic, social, and psychographic attributes were considered. We also specified one decision variable (Table 2).
The intention to use GMFs was used to measure consumer behavior output because intention influences willingness to fulfill a behavior (Jiang et al., 2016; Fozouni Ardekani et al., 2021). The independent variables cover gender, age, education, occupation, and knowledge as demographic variables. Also, promotion focus and prevention focus related to RFT; autonomy, relatedness, and competence related to SDT; and consumers' concerns toward GMFs are considered as psychographic variables. Also, in this study, CSR is defined as a social variable in consumers' intention to use GMFs.
In the third step, a questionnaire was designed based on identified variables, and items measured concerning previous studies. As emphasized by scholars (Pino et al., 2016; Alvarado-Herrera et al., 2017), CSR was measured using 26 items by considering social, environmental, legal, philanthropic, and ethical dimensions. Using 15 items that borrow from previous studies (Bett et al., 2010; Kikulwe et al., 2011; Montuori et al., 2012), concerns about GM foods were assessed based on four dimensions (health, ethics, equity, and environment). RFT dimensions, including consumer promotion and prevention focus on GMFs were measured by 10 items, which were derived from Haws et al. (2010). SDT dimensions (autonomy, relatedness, and competence) were measured based on previous literature (Ilardi et al., 1993; Deci and Ryan, 2000; Gagné, 2003) by using 21 items too. Finally, the intention to use GMFs was assessed with a 6-item scale (Kim et al., 2014; Ghali-Zinoubi and Toukabri, 2019). It is worth mentioning the questionnaire dimensions, scored from number 1 (strongly disagree) to number 5 (strongly agree). Moreover, experts verified the face validity of the questionnaire.
3.3. Analytical methods
Finally, and in the last step, our data analysis consists of two phases: (1) decision rules extraction based on RST analysis; and (2) converting the decision rules into FNGs. In the following sections, we offer a detailed overview of these two techniques that we used in this study.
3.3.1. Rough Set Theory (RST)
The RST is based on an important mathematical logic that deals with low-precision, inconsistent and incomplete information and aims to control the inherent ambiguity and uncertainty in decision making (Segovia et al., 2002; Chen, 2009; Ben Sassi et al., 2016; Acharjya and Ahmed, 2021). This theory originates from a simple information model, and its main idea is divided into two parts. The first part refers to the formation of concepts and rules through the classification of relational databases, and the second part refers to the discovery of knowledge through the classification of equivalence relations and approximations. The RST deals with ambiguous information as one of the theories in the field of data analysis and processing. In RST, two precise boundary lines are created to describe inaccurate concepts, which makes the RST a precise mathematical tool for solving ambiguous and uncertain problems. RST is able to solve some noticeable concerns in the research worlds like providing sets descriptions in terms of property values, examining the dependencies (complete or partial) between properties, reducing properties, analyzing the importance of properties, and creating decision rules (Pawlak et al., 1988; Tay and Shen, 2002). In the following, this study will explain some important concepts of this theory.
3.3.1.1. Indiscernibility of objects
A basic concept of the RST philosophy is its ability in knowledge classification. One of the important approaches to classifying knowledge is indiscernibility, which is a means for eliciting and finding facts from imperfect data through creating discretized attributes and eliminating redundant and irrelative ones. In the table IS = (U, Q, V, F), accept that Q = (C ∪ D) and (C ∩ D) = ∅, where C is a set of condition attributes, and D is a set of decision attributes. Let P ⊆ Q which makes an indiscernibility relation IND (P) is an equivalence relation for any (Han et al., 1999; Wang et al., 2010; Zhang et al., 2016). The indiscernibility relation IND (P) is defined as:
U/IND(P) = {C1, C2, … CK} is the partition of U by P, and every Ci is an equivalence class. For∀X ∈ U, the equivalence class for X in relation U/IND(P) can be conceptualize as:
3.3.1.2. Set approximation
As stated earlier, the purpose of applying RST is to extract and define rules from vague and incomplete data. Thus, the RST deals with two main concepts: Information system and upper and lower approximations (Pawlak, 2002).
U is assumed to be a reference set defining a finite set of other factors (actions). A also represents a finite set of non-attributes (type of service), such that for each attribute a∈ A there is a function a: U → Va in which Va is the sum of all possible values of attribute a that extends to the domain a is famous. Accordingly, the information system is S = (U, A). Each subset B of A, B ⊆ A, defines a binary relation I (B) in U and is described as:
Where a (x) specifies the value of the attribute “a” for the element “x.” Therefore, S = (U, A) is an information system in which X ⊆ U and B ⊆ A. For each X ⊆ U, there are two approximations under the heading of lower B * (X) and upper B * (X) as follows:
3.3.1.3. Attribute reduction
At this stage, some attributes may be seen as redundant in the information table, which means they are irrelative or unnecessary, and their elimination won't affect essential classificatory information. Two noticeable concepts in this stage are attribute reduction and core. Given A and B ⊆ Q a reduct is a minimal set of attributes such that IND (A) = IND (B). The intersection of all reducts, Core(A) = ∩ RED(A) is mentioned as the core of A. The core includes a group of all the central attributes in the decision table (Wang et al., 2010; Zhang et al., 2016).
3.3.1.4. Decision rules induction
The decision table leads to two sets of attributes: condition attributes C and decision attributes D. The decision table S is the exact decision rule if C→D. The set of decision rules that result from the decision table are considered the entrants feeding the decision analysis. Regarding Pawlak (2004) the process of capturing decision rules from a set of initial data is known as induction. The formula for an induced decision rule is: IF-condition(s)-THEN-decision(s). The decision rule represents a relationship between a set of conditions and a conclusion or a decision (Wang et al., 2010).
3.3.2. Flow Network Graph (FNG)
The FNG is an approach proposed by Fulkerson and Ford (1962). The path-dependent relationship of each branch in a flow network, which is produced by the decisions' rules of RST, is interpreted through FNG. Branches of the FNG are considered as decision rules and describe a decision algorithm. The resultant model is applied to capture and define the nature of decision processes within FNGs rather than a description of flow optimization (Pawlak, 2002). According to Pawlak (2004), FNGs and decision algorithms' relation can be explained as follows.
An FNG is a directed acyclic finite graph G = (V, β, h) where V is a set of nodes, β ⊆ v2 is a set of directed branches, h:β → R+ is a flow function, and R+ is the set of non-negative real numbers. Although the flow of a branch (x, y) ∈ β is donated by h(x, y): then x is an input of y is an output of x. The input and output of a graph G are defined as I(G) = {x ∈ V|J(x) ≠ ∅ and (G) = {x ∈ V|O(x) ≠ ∅. For every node x in the FNG, inflow is defined as h+(y) = Σx∈I(y)h(x, y) and outflow is defined as is h−(y)= Similarly, the inflow, and outflow of the whole FNG can be definite as h+ (G) = Σx∈I(x) h−(x). And h− (G) = Σx∈o(Gx) h+(x), respectively. We assume that, for any node x in an FNG, G, h+ (x) = h− (x) = h(G). The measure the strength of every branch (x, y) in an FNG, G = (V, β, h). We define the strength p( x , y) = h(x, y)|r(G), where 0 ≤ p(x, y) ≤ 1. The strength of the branch expresses the ratio of the total flow through the branch. Every branch (x, y) of an FNG, G is associated with the certainty and the coverage coefficients. The certainty and coverage of every branch are outlined as cer(x, y) = p(x, y)|p(x) and cov(x, y) = p(x, y)|p(y). Respectively, where p(x, y) = h(x, y)|h(G), p(x) = h(x)|h(G), and p(y) = h(y)|h(G) are normalized through flow, and p(x) ≠ ∅, p(y) ≠ ∅ and ≤ p(x, y) ≤ 1. The certainty coefficient shows the outflow distribution between outputs of a node, and the coverage coefficient exhibits the quality of inflow distribution between inputs of the node. These coefficients' task is to explain some properties of the flow distribution among the branches in the whole FNG. That's why FNG is considered an important tool for modeling flow information derived from a set of decision rules (Wang et al., 2010; Lin et al., 2011). At the end of this section, Figure 1 presents the research design for this study.
4. Results
Within the scope of RST, after preparing the data, an information table can be constructed to carry out further analysis (Table 3). The first outcomes extracted from the coded information table based on rough sets analysis were the description of equivalence classes, the approximation of the decision classes, and the quality of classifications. It is considered that the classification accuracy is used to represent the percentage of correctly classified and the approximation accuracy is used to describe the degree of intention to use GMFs.
As illustrated in Table 4, our samples are categorized into three classes, (1) low, (2) medium, and (3) high intention to use GMFs. To display the rigor and robustness of results, the accuracy of classifications has been shown for the three decision classes. In this study, we have a total of 377 equivalence classes. Furthermore, in terms of consumers' intention to use GMFs, the results indicate high accuracy equal to 1 for all three decision classes. It means correct classification of all samples.
To get the reduced attribute sets and increase the classification rate, the existing algorithms in RST analysis are employed to determine the core attributes and reduce conditional attributes. Due to the result of 1.0 for the value of the positive region, the reduced set include all 12 attributes {x1, x2, x3, x4, x5, x6, x7, x8, x9, x10, x11, and x12} and there is no redundant attribute in our analysis. Furthermore, since the core includes the essential and most influential attributes, our results display the importance of these 12 variables in anticipating consumer intent to use GMFs.
4.1. Decision-making rules extraction
Based on the analysis of the rough set, 289 logical rules have been extracted from the informational table, of which 116 rules belong to the low intention class, 76 rules belong to the medium intention class, and 97 rules belong to the high intention class. But, many of these rules don't have adequate support factors with regard to their capability to distinguish consumers' characteristics amongst the decision-making classes. Thus, based on the previous research (Wang et al., 2010; Lin et al., 2011; Chen and Tsai, 2016; Mahavarpour et al., 2019) it has been regarded as the threshold value for some indexes to find the higher quality rules. For rule reduction, after consulting with experts, we consider a threshold value with minimum condition attributes ≥ 3. Also, we considered the percentage of training data or support value ≥ 5% for each decision class. So, we only interpreted 13 rules (7, 2, and 4 rules in the low, medium, and high intention classes, respectively; see Table 5).
In the following, by exploring the relationships between consumer factors and intention to use GMFs, we offer a detailed overview of decision rules for consumers' characteristics regarding low, medium, and high intention to consume GMFs (Tables 6–8, respectively). To emphasize the validity of extracted rules, the coefficients of support, certainty, strength, and coverage associated with each rule are shown in tables too.
The three top-ranking variables in the decision rules tables consist of (1) CSR (x6) with frequency occurrences of 11 and a percentage of 84.62%, (2) concerns (x7) with a frequent occurrence of 9, and a percentage of 69.23%, and (3) autonomy (x10) with a frequent occurrence of 7, and a percentage of 53.85%. In the following, we illustrate the main and priorities of variables for each decision class (the three top-ranking variables for each class; Table 9).
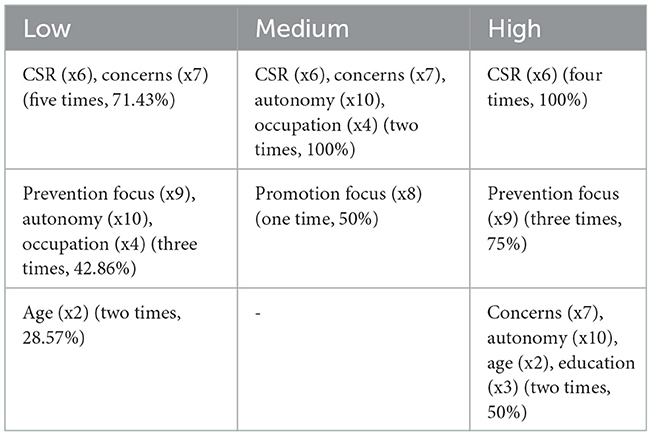
Table 9. Top-3 ranking of the variables in three decision classes based on frequencies and percentages.
4.2. Converting the decision rules into FNGs
An FNG was used in this study to interpret the decision rules and examine the influences of the dimensions and attributes listed in Tables 6–8. The cause-and-effect relationship between variables and consumer intention to use GMFs is depicted in Figures 2–4. The diagrams also show the important meanings and how they influenced people's desire to use GMFs.
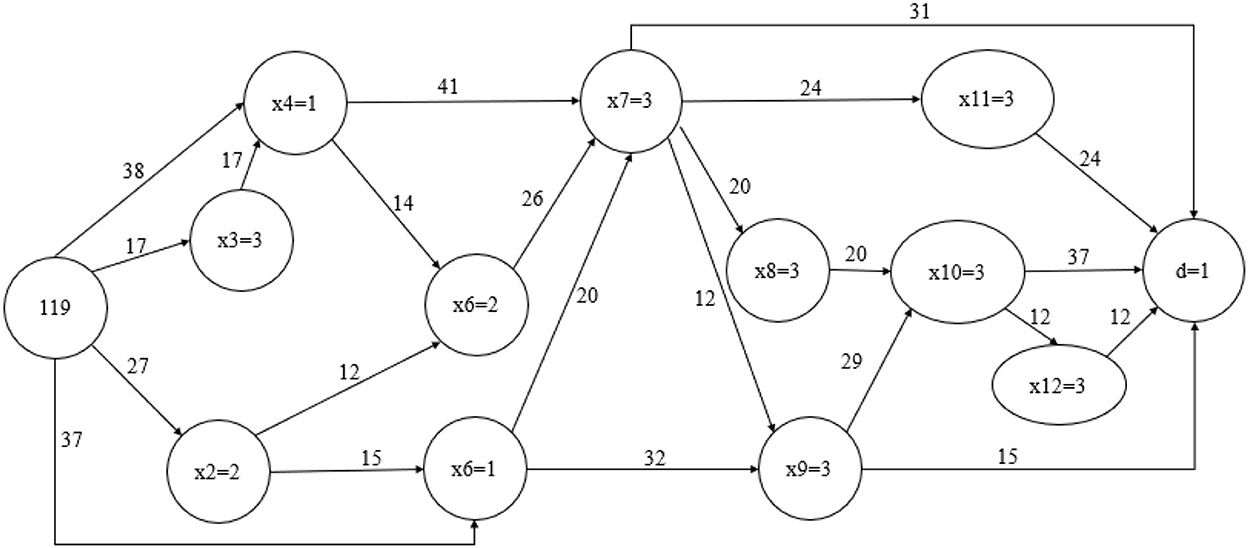
Figure 2. Decision flow graph and rule-set of low intention to use GMFs class. x2 = 2 means that consumer's age is from 30 to 39; x3 = 3 means that consumer has master degree; x4 = 1 means that consumer is student; x6 = 1 means that consumer's perception of CSR is low; x6 = 2 means that consumer's perception of CSR is medium; x7 = 3 means that consumer's concerns about GMFs is high; x8 = 3 means that consumer's promotion focus toward GMFs is high; x9 = 3 means that consumer's prevention focus toward GMFs is high; x10 = 3 means that consumer's autonomy to choose GMFs is high; x11 = 3 means that consumer's competence to choose GMFs is high; x12 = 3 means that consumer's relatedness to choose GMFs is high; d = 1 means that consumer's intention to use GMFs is low.
Based on the descriptions and concepts of RST and FNGs described in the former section, decision rules could be displayed as flow graphs (Figures 2–4). It can be claimed that each branch represents a decision rule in flow graphs or decision algorithms (Lin et al., 2011; Chin et al., 2018).
Regarding the results of RST analysis and generated decision rules, 116 decision rules that supported a low intention to use GMFs were identified, which indicates the firms following these rules may encounter a lack of consumers' intention to consume GMFs, which probably lead to their failure. In this study, 97 and 76 decision rules led to medium and high intentions to use GMFs, which indicates that companies who use these rules in their consumer engagement efforts can make people more likely to think about GMFs.
Within the scope of the FNGs, the coefficients of support, certainty, strength, and coverage explain some properties of flow distribution among the branches. However, to simplify the FNGs, we only show the support coefficients in Figures 2–4 and omit the other coefficients.
The total inflow of the graph in the low intention class is 119, which is the total of the supports related to the decision rules in Table 5. Based on the FNG in Figure 2, the importance of condition attributes could be identified. According to the number of supports, the top-4 determinants of consumers' low intention to use GMFs are: (1) consumer's concerns about GMFs are high (67 supports); (2) the occupational status of the consumer is student (55 supports); (3) consumer's perception of CSR is low (52 supports); and (4) the consumer's autonomy to choose GMFs is high (49 supports).
Looking at Figure 3, we find that in the low intention class, the total inflow of the graph is 11. According to the number of supports, the top-4 determinants of consumers' medium intention to use GMFs with equal support (support = 11) are: (1) the occupational status of the consumer is self-employed; (2) the consumer's perception of CSR is medium; (3) the consumer's concerns about GMFs are high; and (4) the consumer's autonomy to choose GMFs is medium. Finally, the total inflow of the graph in the high intention class is 31, which is the total of the supports related to the decision rules in Table 7.
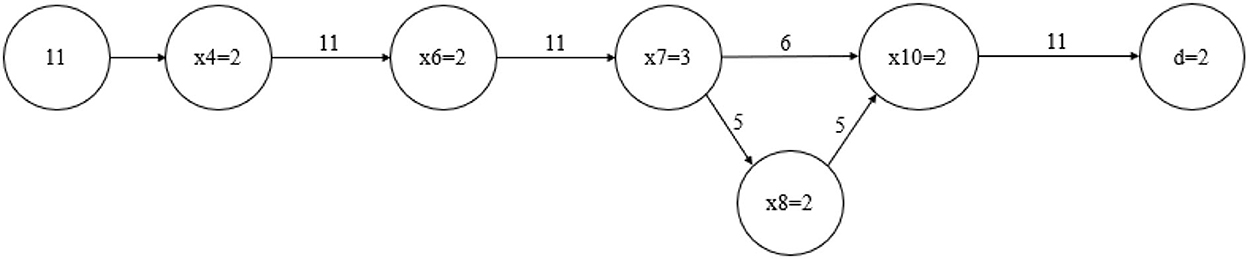
Figure 3. Decision flow graph and rule-set of medium intention to use GMFs class. x4 = 2 means that consumer is self-employed; x6 = 2 means that consumer's perception of CSR is medium; x7 = 3 means that consumer's concerns about GMFs is high; x8 = 2 means that consumer's promotion focus toward GMFs is medium; x10 = 2 means that consumer's autonomy to choose GMFs is medium; d = 2 means that consumer's intention to use GMFs is medium.
Based on the FNG in Figure 4, the importance of condition attributes could be identified. According to the number of supports, the top-4 determinants of consumers' high intention to use GMFs are: (1) consumer's perception of CSR is high (31 supports); (2) consumer's age is 30–39 (16 supports); (3) consumer's education is diploma (16 supports); and the fourth triggering determinant for this class is: consumer's prevention focus toward GMFs is medium (16 supports).
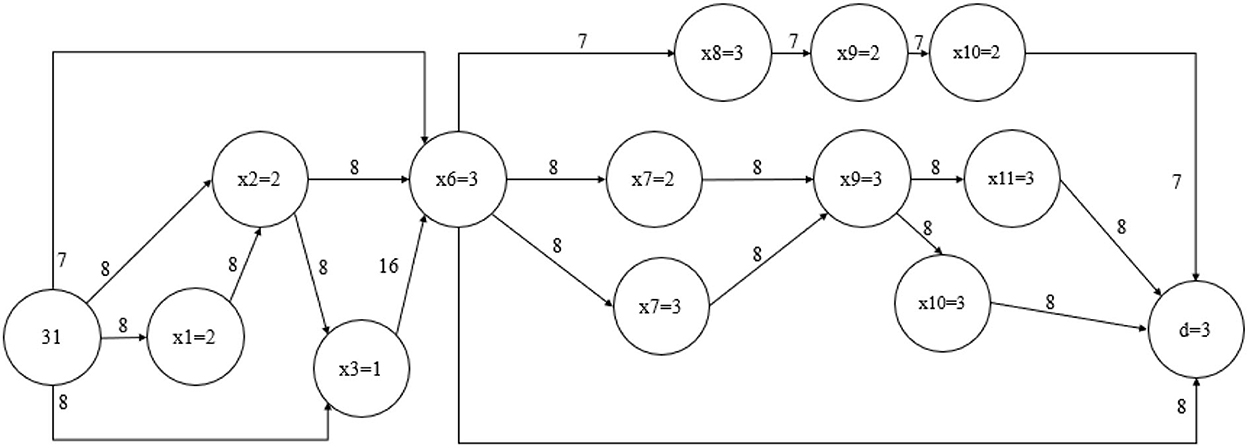
Figure 4. Decision flow graph and rule-set of high intention to use GMFs class. x1 = 2 means that consumer is female; x2 = 2 means that consumer's age is from 30 to 39; x3 = 1 means that consumer has diploma; x6 = 3 means that consumer's perception of CSR is high; x7 = 2 means that consumer's concerns about GMFs is medium; x7 = 3 means that consumer's concerns about GMFs is high; x8 = 3 means that consumer's promotion focus toward GMFs is high; x9 = 2 means that consumer's prevention focus toward GMFs is medium; x9 = 3 means that consumer's prevention focus toward GMFs is high; x10 = 2 means that consumer's autonomy to choose GMFs is medium; x10 = 3 means that consumer's autonomy to choose GMFs is high; x11 = 3 means that consumer's competence to choose GMFs is high; d = 3 means that consumer's intention to use GMFs is high.
5. Discussion and implication
This research used a new approach to predict consumers' intention to use GMFs in a developing country by combining RST and FNGs. The reason behind that is to respond to the research gap that has been addressed by the scholars (Rodríguez-Entrena and Salazar-Ordóñez, 2016; Guo et al., 2020; Erol, 2021) to realize hidden information in consumer characteristics and predict the decision rules of consumers' intention to use GMFs. This approach, is specifically useful for the classification of multi-attribute problems, as well as suited for the problems of business success or failure prediction (Dimitras et al., 1999). Moreover, as emphasized by Cheng et al. (2013) the FNGs like a bridge could connect the pathway of decision rules and the degree of their interdependency. As such, this study utilized these features to extract more influential factors and important patterns of consumers' intention to use GMFs.
By considering the results of the FNG, it was found that consumers' perception of CSR is the factor that has been influential in all degrees of consumers' intention to use GMFs. Moreover, it can be claimed that consumers' concerns, occupational status, and autonomy are other sensitive factors that affect consumer behavior toward GMFs. So, concerning the results of the present study, these factors influence consumer decision-making toward GMFs consumption more than other influential factors. Additionally, the influence of consumers' age, education, and prevention focus has been predicted to reinforce consumers' intention. A summary of these dimensions, derived from the FNGs, is given in Figure 5. It is worth noting that the lines represent the relationships between the variables.
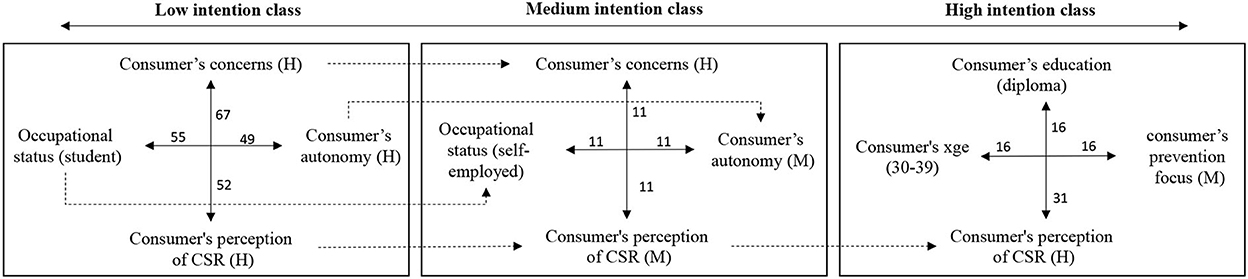
Figure 5. Extended map of the most important dimensions in three classes. H = high, M = medium. Means the relationships between variables in each class.
Means the relationships between the variables in different classes.
This study also discovered multiple interesting and considerable patterns from our data as following:
Pattern 1: the greater the consumers' perception of CSR, the greater the chance that they will use GMFs (94 supports).
In this respect, other scholars (van der Wees and Moonen, 2011; Pino et al., 2016; Boccia and Covino, 2021) referred to CSR initiatives that have a powerful effect on consumer decisions. Furthermore, in many developing and developed countries, the emphasis on biotechnological foods has recently increased (Alphonce et al., 2020), which has led to the growth of social problems. These social problems mainly focus on challenges such as the environment, ethics, philanthropy, and the law.
Pattern 2: consumers with high concerns about GMFs probably fall into the low or medium intention class of using GMFs (78 supports).
With the evolution of the GMFs domain, one of the vital concepts is consumers' concerns about GMFs. The influence of consumers' concerns on the adoption of GMFs has been studied in prior research to some extent. For example, findings by scholars (Hakim et al., 2020; Davies et al., 2021; Pakseresht et al., 2021; Zheng and Wang, 2021) reveal that consumers' concerns positively affect consumer adoption of GMFS. Additionally, findings from Frewer et al. (2011) confirm that those foods characterized as being “bioactive” boost particular concerns, including ethical concerns, uncontrolled use, and unpredictable effects.
Pattern 3: consumers with the occupational status of students usually fall into the low intention class, while self-employed consumers usually fall into the medium intention class (66 supports).
One of the most fundamental elements of GMFs is their focus on and interest in individuals with higher incomes. The finding obtained by Chege and Groote (2008), Zheng and Wang (2021), and Waterfield et al. (2020), suggest that the high-income category of consumers were more likely to purchase GMFs. The reason behind this is consumers' income levels could moderate attitudes toward bioengineered food (Levi, 2022).
Pattern 4: the degree of autonomy is higher for low intention consumers rather than for consumers with medium intention to use GMFs (60 supports).
The SDT is one of the most important theories that has imported the psychological concepts from the GMFs literature to explore consumers' characteristics toward these biotechnological foods (Rubel and Streiffer, 2005; Mwathi, 2015; Martinez-Ribaya and Areal, 2020). In this study, the authors claim consumers' autonomy to choose GMFs has a greater effect on consumers' adoption compared to other elements in SDT. Similarly, Siipi and Uusitalo (2011) support that consumers' autonomy will be a crucial driver for the adoption of GMFs. Moreover, other patterns that have fewer support values are listed below:
Pattern 5: consumers' aged 30–39 are more likely to be in the high intention class (16 supports).
Pattern 6: high intention consumers usually have a medium level of prevention focus (16 supports).
Pattern 7: diploma consumers usually fall into the high intention class (16 supports).
5.1. Theoretical implication
Given the growth of the GMFs topic, consumer behavioral predictions in the form of decision rules and important patterns that occur in this study could be noticed for their strength and capability to represent the importance of variables. This research contributes to the current belief among scholars (Chen and Tsai, 2016; Bai et al., 2019; Mahavarpour et al., 2021; Pelissari et al., 2021) that the generated rules can provide favorable strategies which can recognize consumers' perception-based attributes. In more precise terms, it provides insights into how relationships are described by consumer characteristics and their behaviors. However, this paper is the first effort to consider this direction toward GMFs.
We also contribute to the GMFs literature by predicting the degree to which consumer characteristics, such as demographic, social, and psychographic variables, influence the consumer's intention to use GMFs. The findings illustrate that consumers' perception of CSR plays a vital role in consumer decision-making toward GMFs adoption. These results support preceding literature that suggests CSR is associated with consumer reaction toward GMFs (Pino et al., 2016; Boccia and Covino, 2021; Rabbanee et al., 2021). Prior research on consumer behavior toward GMFs mainly focused on the effects of one or a few rare factors separately (Deng and Hu, 2019; Jiang and Zhang, 2021; McKenzie et al., 2021; Rabbanee et al., 2021; Levi, 2022; Sleboda and Lagerkvist, 2022), while in this study, important influential factors were studied together and the analysis of their effects simultaneously to determine priorities. Consequently, we point to consumers' concerns, occupational status, and consumer autonomy as other important aspects of consumer decision-making toward GMFs. Furthermore, this study adds to the current belief among scholars (Chen et al., 2019; Pakseresht et al., 2021) that the “food-orientation” function is an important direction for future research and it would be beneficial to identify influential factors regarding GMFs acceptance.
5.2. Managerial implication
The results of this study, especially the consumers' intentions illustrated in the FNGs would be useful for the GMFs firms to understand how their marketing efforts are affected by consumers' demographic, social, and psychographic variables. Concerning the findings, managers must take into consideration that it is not only one characteristic that is related to a low or high intention of using GMFs. As previously demonstrated, there are relatively complex with significant interaction relationships between variables that managers could realize the entire conditions of the relationship among mentioned variables from the consumer's perspective and its effect on a favorable decision making, particularly in the developing countries context.
Based on the analysis outcomes, the most significant factors that affect consumers' intention to use GMFs are: “consumer perception of CSR;” “consumer concerns;” “occupational status;” and “consumer autonomy.” Thus, managers and policymakers must pay more attention to these concepts when they survey consumer intention behavior. In addition, GMFs companies can use the combined RST and an FNG of their CRM database to create an integrated system for predicting consumer behavior in order to expand appropriate operations and decisions.
6. Conclusion
This study combined the RST and FNG to construct network graphs of consumers' intentions to use GMFs. Current research extracts decision rules from the initial data based on RST. Moreover, by considering the extracted rules, non-linear relationships to analyze the influence of consumer characteristics on the intention to use GMFs have been provided by the FNG. Therefore, in this study, the researchers, looked at possible routes and useful information about how likely people were to eat GMFs.
According to the analysis of RST, and considering the support values and other indexes introduced in the Rough Set Theory, 13 rules have been selected from 289 initial rules in three segmented classes. Using the RST analysis, in the first class (low intention to use GMFs), seven decision rules from 116 rules were selected; in the second class (medium intention to use GMFs), two decision rules from 76 rules were selected; and in the third class (high intention to use GMFs), four decision rules from 97 rules were selected. Using the FNG approach, the total inflow of the graph and the top-4 determinants of consumers' intention to use GMFs in all three decision classes were identified and described. Moreover, by combining these two methods and investigating all three classes, the main rules and patterns governing the behavioral intention of consumers toward GMFs adoption were identified. The overall results confirm that corporate social responsibilities, consumer concerns, occupational status, and consumer autonomy are more important than other observed dimensions in consumers' decision-making. Moreover, findings illustrate that combining Rough Set Theory and Flow Network Graph could predict customers' intention and provide valuable information for policy-makers in related active industries.
7. Limitation and future research
Like any other study, this research has some limitations that we must acknowledge for the direction of future research. (Christofi et al., 2021) use the term “tri-axial” including theory, context, and methodology, to signify that future research is positioned along these axes. Concerning the results of the present study, we suggest fruitful areas for further advancement of future GMFs research based on these three axes.
7.1. Axis 1: Theory
First, although a few theories (such as the Theory of Planned Behavior, Innovation Diffusion Theory, Theory of Reasoned Action, Social Representations Theory, and Theory of the Risk) have been explored by previous scholars (Prati et al., 2012; Kim et al., 2014; Li and Bautista, 2020; Fozouni Ardekani et al., 2021; Rabbanee et al., 2021), the lack of a coherent and explicit theoretical base by considering novel approaches feels in GMFs research. This study examined a number of theory-related issues during. Similarly, to find more attitudes about consumer behavior in GMFs, we propose future research to investigate an extended variety of theories like protected motivation theory, theory of social power, uses and gratifications theory, social interdependence theory, and other theoretical concepts from different related disciplines such as sociology, psychology, and economics, which lead to empowering GMFs literature in the theory section.
Second, regarding the result of this study, future research could explore the ways that firms could maximize the positive consequences that occur from GMFs activity in society and contribute to reinforcing insight toward corporate's social responsibility. In addition, according to the literature themes, there is a lack of consideration on investigating consumers' attitudes toward both pre-and-post GMFs consumption stages. Thus, according to this gap, and considering that by exploring the relationships between critical factors within the pre-and-post consumption stages, deeper insight will be detected from consumer behavior, we encourage future studies to investigate this issue.
7.2. Axis 2: Context
Understanding the driving forces that can impact the evolution of the present-day situation is vital for future research on GMFs (Gurau and Ranchhod, 2016). Since society represents the general framework in which these changes will happen, these factors' recognition must be accomplished through the survey and analysis of human perceptions. As a result, other researchers are encouraged to empirically examine and compare the research model of this study in various geographical, social, institutional, and economic conditions of human beings. Also, it is valuable to compare the attitudes of consumers toward GMFs in developed and developing countries with each other.
7.3. Axis 3: Methodology
As regards the methodology, this research is one of the first to combine the RST and FNG to predict consumer behavior in the GMFs section. According to scholars (Chen and Tsai, 2016; Li et al., 2018; Tsai and Yeh, 2019; Moghadam et al., 2021; Pelissari et al., 2021), the application of RST at the decision-making level is suitable for prediction and theory building. Hence, we propose our methodology to other scholars too, to use our method for different samples and compare the extracted rules. Finally, future researchers are proposing to combine RST with other approaches like neural networks or fuzzy logic.
To sum up, the lack of a coherent and explicit theoretical base by considering novel approaches feels in GMFs research. Also, understanding the driving forces of consumers' attitudes toward GMFs is vital for future research on GMFs which can be accomplished through the survey and analysis of human perceptions. Table 10 presents more future suggestions in the form of research questions based on these three identified axis in order to complete more the GMFs literature.
Data availability statement
The raw data supporting the conclusions of this article will be made available by the authors, without undue reservation.
Author contributions
MA, NM, and FM: methodology. NM and FM: software. MA, NM, FM, and PM: analysis, writing—original draft, and writing—review and editing. MA: investigation and project administration. All authors contributed to the article and approved the submitted version.
Conflict of interest
The authors declare that the research was conducted in the absence of any commercial or financial relationships that could be construed as a potential conflict of interest.
Publisher's note
All claims expressed in this article are solely those of the authors and do not necessarily represent those of their affiliated organizations, or those of the publisher, the editors and the reviewers. Any product that may be evaluated in this article, or claim that may be made by its manufacturer, is not guaranteed or endorsed by the publisher.
References
Acharjya, D. P., and Ahmed, N. S. S. (2021). Tracing of online assaults in 5G networks using dominance based rough set and formal concept analysis. Peer-to-Peer Network. Appl. 14, 349–374. doi: 10.1007/s12083-020-00983-6
Akbari, M., Foroudi, P., Shahmoradi, M., Padash, H., Parizi, Z. S., Khosravani, A., et al. (2022a). The evolution of food security: where are we now, where should we go next? Sustainability 14, 63634. doi: 10.3390/su14063634
Akbari, M., Fozouni Ardekani, Z., Pino, G., and Maleksaeidi, H. (2019). An extended model of Theory of Planned Behavior to investigate highly-educated Iranian consumers' intentions towards consuming genetically modified foods. J. Clean. Prod. 227, 784–793. doi: 10.1016/j.jclepro.2019.04.246
Akbari, M., Maleksaeidi, H., Ardekani, Z. F., Pino, G., Padash, H., and Mofrad, H. (2022b). Consumer intention toward genetically modified food : a moderated mediation model. J. Agri. Sci. Technol. 24, 291–304. Available online at: http://jast.modares.ac.ir/article-23-49636-en.html
Alphonce, R., Waized, B. M., and Larsen, M. N. (2020). Consumer preference for novelty in processed foods: a developing country perspective. J. Agribus. Develop. Emerg. Econ. 2019, 36. doi: 10.1108/JADEE-03-2019-0036
Alvarado-Herrera, A., Bigne, E., Aldas-Manzano, J., and Curras-Perez, R. (2017). A scale for measuring consumer perceptions of corporate social responsibility following the sustainable development paradigm. J. Bus. Ethics 140, 243–262. doi: 10.1007/s10551-015-2654-9
Ardebili, A. T., and Rickertsen, K. (2020). Personality traits, knowledge, and consumer acceptance of genetically modified plant and animal products. Food Qual. Pref. 80, 103825. doi: 10.1016/j.foodqual.2019.103825
Asadi, P., Pourhossein, M., Marasi, M. R., and Esfandiari, Z. (2021). Effect of education on knowledge, attitude, and practices of students of Isfahan University of Medical Sciences, Iran, toward genetically modified foods. J. Health Syst. Res. 17, 7–15. doi: 10.22122/jhsr.v17i1.1402
Bai, C., Shi, B., Liu, F., and Sarkis, J. (2019). Banking credit worthiness: evaluating the complex relationships. Omega 83, 26–38. doi: 10.1016/j.omega.2018.02.001
Ben Sassi, D., Frini, A., Ben Abdessalem Karaa, W., and Kraiem, N. (2016). A competitive intelligence solution to predict competitor action using K-modes algorithm and rough set theory. Proc. Comput. Sci. 96, 597–606. doi: 10.1016/j.procs.2016.08.240
Bett, C., Ouma, J. O., and De Groote, H. (2010). Perspectives of gatekeepers in the Kenyan food industry towards genetically modified food. Food Pol. 35, 332–340. doi: 10.1016/j.foodpol.2010.01.003
Boccia, F. (2016). Consumer perception: an analysis on second generation genetically modified foods. Nutr. Food Sci. 46, 637–646. doi: 10.1108/NFS-03-2016-0035
Boccia, F., and Covino, D. (2021). Corporate social responsibility and biotechnological foods: an experimental study on consumer's behaviour. Nutr. Food Sci. 2021, 293. doi: 10.1108/NFS-10-2021-0293
Boccia, F., Covino, D., and Sarnacchiaro, P. (2018). Genetically modified food versus knowledge and fear: a Noumenic approach for consumer behaviour. Food Res. Int. 111, 682–688. doi: 10.1016/j.foodres.2018.06.013
Boccia, F., and Punzo, G. (2021). A choice experiment on consumer perceptions of three generations of genetically modified foods. Appetite 161, 105158. doi: 10.1016/j.appet.2021.105158
Chege, S., and Groote, H. D. (2008). Consumer willingness to pay for genetically modified food in Kenya. Agri. Econ. 38, 35–46. doi: 10.1111/j.1574-0862.2007.00279.x
Chen, L. F., and Tsai, C. T. (2016). Data mining framework based on rough set theory to improve location selection decisions: a case study of a restaurant chain. Tour. Manag. 53, 197–206. doi: 10.1016/j.tourman.2015.10.001
Chen, R., Liu, M. W., Guan, Y., and Zheng, Y. (2019). Female responses to genetically modified foods: effects of the menstrual cycle and food risk concerns. J. Bus. Res. 6, 39. doi: 10.1016/j.jbusres.2019.06.039
Chen, W. S. (2009). Analysis of a customer satisfaction survey using Rough Sets theory: a manufacturing case in Taiwan. Asia Pacific J. Market. Logist. 21, 93–105. doi: 10.1108/13555850910926263
Cheng, J.-W., Chiu, W.-L., and Tzeng, G.-H. (2013). Do impression management tactics and/or supervisor–subordinate guanxi matter? Knowl. Based Syst. 40, 123–133. doi: 10.1016/j.knosys.2012.12.002
Chin, Y. C., Su, W. Z., Chen, S. C., Hou, J., and Huang, Y. C. (2018). Exploring users' self-disclosure intention on social networking applying novel soft computing theories. Sustainability 10, 113928. doi: 10.3390/su10113928
Christofi, M., Pereira, V., Vrontis, D., Tarba, S., and Thrassou, A. (2021). Agility and flexibility in international business research: a comprehensive review and future research directions. J. World Bus. 56, 101194. doi: 10.1016/j.jwb.2021.101194
Davies, G., Gorman, R., McGlacken, R., and Peres, S. (2021). The social aspects of genome editing: publics as stakeholders, populations and participants in animal research. Lab. Anim. 2021, 23677221993157. doi: 10.1177/0023677221993157
Deci, E. L., and Ryan, R. M. (2000). The “what” and “why” of goal pursuits: human needs and the self-determination of behavior. Psychol. Inq. 11, 227–268. doi: 10.1207/S15327965PLI1104_01
Deng, H., and Hu, R. (2019). A crisis of consumers' trust in scientists and its influence on consumer attitude toward genetically modified foods. Br. Food J. 121, 2454–2476. doi: 10.1108/BFJ-07-2018-0476
Deng, H., Hu, R., Pray, C., and Jin, Y. (2019). Perception and attitude toward GM technology among agribusiness managers in China as producers and as consumers. Sustainability 11, 51342. doi: 10.3390/su11051342
Dimitras, A. I., Slowinski, R., Susmaga, R., and Zopounidis, C. (1999). Business failure prediction using rough sets. Eur. J. Operat. Res. 114, 263–280. doi: 10.1016/S0377-2217(98)00255-0
Erol, A. (2021). Genetically modified foods from islamic law perspective. J. Agri. Environ. Ethics 34, 1–14. doi: 10.1007/s10806-021-09845-4
Ferrari, L., Baum, C. M., Banterle, A., and De Steur, H. (2021). Attitude and labelling preferences towards gene-edited food: a consumer study amongst millennials and Generation Z. Br. Food J. 123, 1268–1286. doi: 10.1108/BFJ-09-2020-0820
Fozouni Ardekani, Z., Akbari, M., Pino, G., Zúñiga, M. Á., and Azadi, H. (2021). Consumers' willingness to adopt genetically modified foods. Br. Food J. 123, 1042–1059. doi: 10.1108/BFJ-04-2019-0260
Frewer, L. J., Bergmann, K., Brennan, M., Lion, R., Meertens, R., Rowe, G., et al. (2011). Consumer response to novel agri-food technologies: Implications for predicting consumer acceptance of emerging food technologies. Trends Food Sci. Technol. 22, 442–456. doi: 10.1016/j.tifs.2011.05.005
Fulkerson, D. R., and Ford, L. R. (1962). Flows in Networks. Princeton, NJ: University Press Princeton.
Gagné, M. (2003). The role of autonomy support and autonomy orientation in prosocial behavior engagement. Motivat. Emot. 27, 199–223. doi: 10.1023/A:1025007614869
Ghali-Zinoubi, Z., and Toukabri, M. (2019). The antecedents of the consumer purchase intention: sensitivity to price and involvement in organic product: moderating role of product regional identity. Trends Food Sci. Technol. 90, 175–179. doi: 10.1016/j.tifs.2019.02.028
Guo, Q., Yao, N., and Zhu, W. (2020). How consumers' perception and information processing affect their acceptance of genetically modified foods in China: a risk communication perspective. Food Res. Int. 137, 109518. doi: 10.1016/j.foodres.2020.109518
Gurau, C., and Ranchhod, A. (2016). The futures of genetically-modified foods: global threat or panacea? Futures 83, 24–36. doi: 10.1016/j.futures.2016.06.007
Hakim, M. P., Zanetta, L. D. A., de Oliveira, J. M., and da Cunha, D. T. (2020). The mandatory labeling of genetically modified foods in Brazil: consumer's knowledge, trust, and risk perception. Food Res. Int. 132, 109053. doi: 10.1016/j.foodres.2020.109053
Han, Z., Zhang, Q., and Wen, F. (1999). A survey on rough set theory and its application. Kongzhi Lilun Yu Yingyong 16, 322–333.
Haws, K. L., Dholakia, U. M., and Bearden, W. O. (2010). An assessment of chronic regulatory focus measures. J. Market. Res. 47, 967–982. doi: 10.1509/jmkr.47.5.967
Hwang, H., and Nam, S. J. (2021). The influence of consumers' knowledge on their responses to genetically modified foods. GM Crops Food 12, 146–157. doi: 10.1080/21645698.2020.1840911
Ilardi, B. C., Leone, D., Kasser, T., and Ryan, R. M. (1993). Employee and supervisor ratings of motivation: main effects and discrepancies associated with job satisfaction and adjustment in a factory setting 1. J. Appl. Soc. Psychol. 23, 1789–1805. doi: 10.1111/j.1559-1816.1993.tb01066.x
Jiang, C., Zhao, W., Sun, X., Zhang, K., Zheng, R., and Qu, W. (2016). The effects of the self and social identity on the intention to microblog: an extension of the theory of planned behavior. Comput. Hum. Behav. 64, 754–759. doi: 10.1016/j.chb.2016.07.046
Jiang, D., and Zhang, G. (2021). Marketing clues on the label raise the purchase intention of genetically modified food. Sustainability 13, 9970. doi: 10.3390/su13179970
Kikulwe, E. M., Wesseler, J., and Falck-Zepeda, J. (2011). Attitudes, perceptions, and trust. Insights from a consumer survey regarding genetically modified banana in Uganda. Appetite 57, 401–413. doi: 10.1016/j.appet.2011.06.001
Kim, Y. G., Jang, S. Y., and Kim, A. K. J. (2014). Application of the theory of planned behavior to genetically modified foods: moderating effects of food technology neophobia. Food Res. Int. 62, 947–954. doi: 10.1016/j.foodres.2014.03.057
Lefebvre, S., Cook, L. A., and Griffiths, M. A. (2019). Consumer perceptions of genetically modified foods: a mixed-method approach. J. Consumer Market. 36, 113–123. doi: 10.1108/JCM-12-2016-2043
Levi, S. (2022). Living standards shape individual attitudes on genetically modified food around the world. Food Qual. Pref. 95, 104371. doi: 10.1016/j.foodqual.2021.104371
Li, J., Fang, H., and Song, W. (2018). Sustainability evaluation via variable precision rough set approach: a photovoltaic module supplier case study. J. Clean. Prod. 192, 751–765. doi: 10.1016/j.jclepro.2018.04.248
Li, L., and Bautista, J. R. (2020). Incorporating communication factors in the theory of planned behavior to predict Chinese university students' intention to consume genetically modified foods. Int. J. Commun. 14, 2338–2359.
Lin, C. S., Tzeng, G. H., and Chin, Y. C. (2011). Combined rough set theory and flow network graph to predict customer churn in credit card accounts. Expert Syst. Appl. 38, 8–15. doi: 10.1016/j.eswa.2010.05.039
Lusk, J. L. (2011). “Consumer preferences for genetically modified food,” in Frontiers of Economics and Globalization, Vol. 10, Issue 2011, eds C. A. Carter, G. Moschini, and I. Sheldon (Bingley: Emerald Group Publishing Ltd), 243–262. doi: 10.1108/S1574-8715(2011)0000010015
Macall, D. M., Williams, C., Gleim, S., and Smyth, S. J. (2021). Canadian consumer opinions regarding food purchase decisions. J. Agri. Food Res. 3, 100098. doi: 10.1016/j.jafr.2020.100098
Mahavarpour, N., Hoseinzade Shahri, M., Abdolvand, N., and Babaei, M. A. (2021). Rules governing the behavioral intentions of instagram users to participate in marketing crowdsourcing contest based on the ruff set theory (case study: motosel consumers). Consum. Behav. Stud. J. 8, 132–159.
Mahavarpour, N., Sajadian, F., and Haghighinasab, M. (2019). Extracting the rules governing the behavioral intentions of Iranian users of Mobile Tourism Applications using individual factors based on the Rough Set theory. Tour. Develop. 7, 83–99. doi: 10.22034/jtd.2018.104061.1221
Martinez-Ribaya, B., and Areal, F. J. (2020). Is there an opportunity for product differentiation between GM and non-GM soya-based products in Argentina? Food Control 109, 106895. doi: 10.1016/j.foodcont.2019.106895
McKenzie, K., Metcalf, D. A., and Saliba, A. (2021). Validation of the Food Technology Neophobia Scale in a Chinese sample using exploratory and confirmatory factor analysis. Food Qual. Pref. 89, 104148. doi: 10.1016/j.foodqual.2020.104148
Miles, S., Ueland, Ø., and Frewer, L. J. (2005). Public attitudes towards genetically-modified food. Br. Food J. 107, 246–262. doi: 10.1108/00070700510589521
Moghadam, M. R. S., Govindan, K., Dahooie, J. H., Mahvelati, S., and Meidute-Kavaliauskiene, I. (2021). Designing a model to estimate the level of university social responsibility based on rough sets. J. Clean. Prod. 324, 129178. doi: 10.1016/j.jclepro.2021.129178
Montuori, P., Triassi, M., and Sarnacchiaro, P. (2012). The consumption of genetically modified foods in Italian high school students. Food Qual. Pref. 26, 246–251. doi: 10.1016/j.foodqual.2012.05.004
Mwathi, N. (2015). A review of key regulatory issues for genetically modified foods in Kenya. Int. J. Hum. Rights Constitut. Stud. 3, 31–45. doi: 10.1504/IJHRCS.2015.069239
Ortega, D. L., Lin, W., and Ward, P. S. (2022). Consumer acceptance of gene-edited food products in China. Food Qual. Pref. 95, 104374. doi: 10.1016/j.foodqual.2021.104374
Owusu, R., and Kwesi Ndzebah Dadzie, S. (2021). Heterogeneity in consumer preferences for organic and genetically modified food products in Ghana. Afri. J. Agri. Resour. Econ. 16, 112–125. doi: 10.53936/afjare.2021.16(2).08
Pakseresht, A., Edenbrandt, A. K., and Lagerkvist, C. J. (2021). Genetically modified food and consumer risk responsibility: the effect of regulatory design and risk type on cognitive information processing. PLoS ONE 16, e0252580. doi: 10.1371/journal.pone.0252580
Palmieri, N., Simeone, M., Russo, C., and Perito, M. A. (2020). Profiling young consumers' perceptions of GMO products: a case study on Italian undergraduate students. Int. J. Gastron. Food Sci. 21, 100224. doi: 10.1016/j.ijgfs.2020.100224
Pawlak, Z. (2002). Rough set theory and its applications. J. Telecommun. Informat. Technol. 3, 7–10. Available online at: https://yadda.icm.edu.pl/baztech/element/bwmeta1.element.baztech-article-BPS2-0018-0017
Pawlak, Z. (2004). Decisions rules and flow networks. Eur. J. Operat. Res. 154, 184–190. doi: 10.1016/S0377-2217(03)00004-3
Pawlak, Z., Wong, S. K. M., and Ziarko, W. (1988). Rough sets: probabilistic vs. deterministic approach. Int. J. Man Machine Stud. 29, 81–95. doi: 10.1016/S0020-7373(88)80032-4
Pelissari, R., Oliveira, M. C., Abackerli, A. J., Ben-Amor, S., and Assumpção, M. R. P. (2021). Techniques to model uncertain input data of multi-criteria decision-making problems: a literature review. Int. Transact. Operat. Res. 28, 523–559. doi: 10.1111/itor.12598
Pino, G., Amatulli, C., De Angelis, M., and Peluso, A. M. (2016). The influence of corporate social responsibility on consumers' attitudes and intentions toward genetically modified foods: evidence from Italy. J. Clean. Prod. 112, 2861–2869. doi: 10.1016/j.jclepro.2015.10.008
Prati, G., Pietrantoni, L., and Zani, B. (2012). The prediction of intention to consume genetically modified food: test of an integrated psychosocial model. Food Qual. Pref. 25, 163–170. doi: 10.1016/j.foodqual.2012.02.011
Pruitt, J. R., Melton, K. M., and Palma, M. A. (2021). Does physical activity influence consumer acceptance of gene edited food? Sustainability 13, 1–12. doi: 10.3390/su13147759
Rabbanee, F. K., Afroz, T., and Naser, M. M. (2021). Are consumers loyal to genetically modified food? Evidence from Australia. Br. Food J. 123, 803–819. doi: 10.1108/BFJ-11-2019-0832
Rodríguez-Entrena, M., and Salazar-Ordóñez, M. (2016). “Consumer behavior regarding genetically modified foods,” in Genetically Modified Organisms in Food (Issue 1975). eds R. R. Watson, V. R. Preedy (Amsterdam: Elsevier Inc), 6. doi: 10.1016/B978-0-12-802259-7.00016-6
Rubel, A., and Streiffer, R. (2005). Respecting the autonomy of European and American consumers: defending positive labels on GM foods. J. Agri. Environ. Ethics 18, 75–84. doi: 10.1007/s10806-004-3079-0
Segovia, M., Gil, J., Heras, A., Vilar, J. L., and Arellano, A. (2002). Using Rough Sets to Predict Insolvency of Spanish Non-Life Insurance Companies. Madrid: Universidad Complutense de Madrid, Facultad de Ciencias Económicas y Empresariales
Siegrist, M., and Hartmann, C. (2020). Consumer acceptance of novel food technologies. Nat. Food 1, 343–350. doi: 10.1038/s43016-020-0094-x
Siipi, H., and Uusitalo, S. (2011). Consumer autonomy and availability of genetically modified food. J. Agri. Environ. Ethics 24, 147–163. doi: 10.1007/s10806-010-9250-x
Sleboda, P., and Lagerkvist, C.-J. (2022). Tailored communication changes consumers' attitudes and product preferences for genetically modified food. Food Qual. Pref. 96, 104419. doi: 10.1016/j.foodqual.2021.104419
Soroka, A., Mazurek-Kusiak, A. K., and Trafialek, J. (2021). Organic food in the diet of residents of the Visegrad Group (V4) Countries—reasons for and barriers to its purchasing. Nutrients 13, 4351. doi: 10.3390/nu13124351
Tay, F. E. H., and Shen, L. (2002). Economic and financial prediction using rough sets model. Eur. J. Operat. Res. 141, 641–659. doi: 10.1016/S0377-2217(01)00259-4
Tsai, C.-A., and Yeh, C.-C. (2019). Understanding the decision rules for 3D printing adoption. Technol. Anal. Strategic Manag. 31, 1104–1117. doi: 10.1080/09537325.2019.1584287
van der Wees, B. J., and Moonen, J. M. (2011). “The potential of in-train crowdsourcing,” in 24th Bled Econference: Efuture: Creating Solutions For The Individual, Organisations And Society, eds N. Wickramasinghe, U. Lechner, A. Pucihar, J. Gricar, and M. Babnik (Maribor: University of Maribor), 68–83.
Varzakas, T., and Tzanidis, T. (2015). “Genetically modified foods: risk assessment, legislation, consumer behavior, and ethics,” in Encyclopedia of Food and Health, 1st edn. eds B. Caballero, P. M. Finglas, F. Toldrá (Amsterdam: Elsevier Ltd), 2. doi: 10.1016/B978-0-12-384947-2.00348-2
Vindigni, G., Peri, I., Consentino, F., Selvaggi, R., and Spina, D. (2022). Exploring consumers' attitudes towards food products derived by new plant breeding techniques. Sustainability 14, 105995. doi: 10.3390/su14105995
Wang, C. H., Chin, Y. C., and Tzeng, G. H. (2010). Mining the R&D innovation performance processes for high-tech firms based on rough set theory. Technovation 30, 447–458. doi: 10.1016/j.technovation.2009.11.001
Waterfield, G., Kaplan, S., and Zilberman, D. (2020). Willingness to pay vs. willingness to vote: consumer and voter avoidance of genetically modified foods. Am. J. Agri. Econ. 102, 505–524. doi: 10.1002/ajae.12001
Xu, R., Wu, Y., and Luan, J. (2020). Consumer-perceived risks of genetically modified food in China. Appetite 147, 104520. doi: 10.1016/j.appet.2019.104520
Zhang, Q., Xie, Q., and Wang, G. (2016). A survey on rough set theory and its applications. CAAI Trans. Intellig. Technol. 1, 323–333. doi: 10.1016/j.trit.2016.11.001
Keywords: genetically modified foods (GMFs), Flow Network Graphs, Rough Set Theory, decision analysis, intention
Citation: Akbari M, Mahavarpour N, Moshkdanian F and Maroufkhani P (2023) Modeling adoption of genetically modified foods: Application of Rough Set Theory and Flow Network Graph. Front. Sustain. Food Syst. 6:992054. doi: 10.3389/fsufs.2022.992054
Received: 23 July 2022; Accepted: 05 December 2022;
Published: 04 January 2023.
Edited by:
Giuseppe Di Vita, University of Turin, ItalyCopyright © 2023 Akbari, Mahavarpour, Moshkdanian and Maroufkhani. This is an open-access article distributed under the terms of the Creative Commons Attribution License (CC BY). The use, distribution or reproduction in other forums is permitted, provided the original author(s) and the copyright owner(s) are credited and that the original publication in this journal is cited, in accordance with accepted academic practice. No use, distribution or reproduction is permitted which does not comply with these terms.
*Correspondence: Morteza Akbari, bW9ydGV6YWFrYmFyaUB1dC5hYy5pcg==