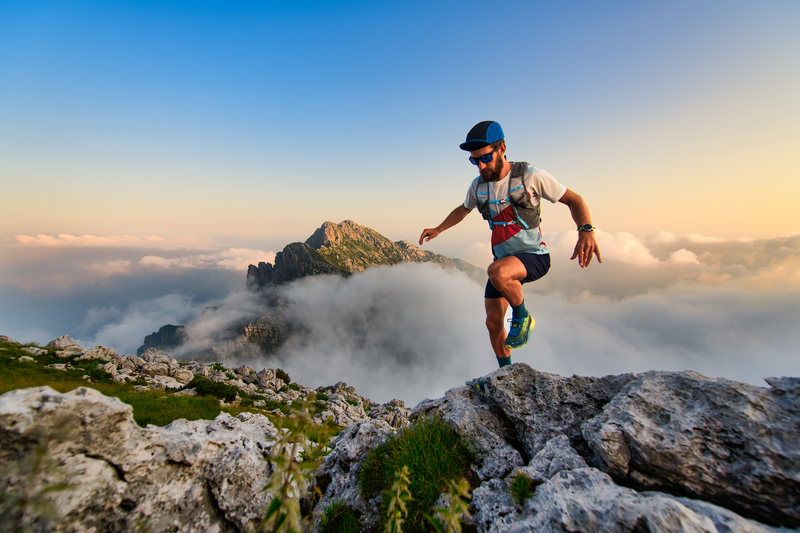
94% of researchers rate our articles as excellent or good
Learn more about the work of our research integrity team to safeguard the quality of each article we publish.
Find out more
MINI REVIEW article
Front. Sustain. Food Syst. , 30 November 2022
Sec. Crop Biology and Sustainability
Volume 6 - 2022 | https://doi.org/10.3389/fsufs.2022.972213
This article is part of the Research Topic Integrated Pest Management Strategies for Sustainable Food Production View all 6 articles
Integrated pest management (IPM) has greatly influenced farming in the past decades. Even though it has been effective, its adoption has not been as large as anticipated. Operational issues regarding crop monitoring are among the reasons for the lack of adoption of the IPM philosophy because control decisions cannot be made unless the crop is effectively and constantly monitored. In this way, recent technologies can provide unique information about plants affected by insects. Such information can be very precise and timely, especially with the use of real-time data to allow decision-making for pest control that can prevent local infestation of insects from spreading to the whole field. Some of the digital tools that are commercially available for growers include drones, automated traps, and satellites. In the future, a variety of other technologies, such as autonomous robots, could be widely available. While the traditional IPM approach is generally carried out with control solutions being delivered throughout the whole field, new approaches involving digital technologies will need to consider adaptations in the concepts of economic thresholds, sampling, population forecast, injury identification, and ultimately the localized use of control tactics. Therefore, in this paper, we reviewed how the traditional IPM concepts could be adapted, considering this ongoing digital transformation in agriculture.
Integrated pest management (IPM) has greatly improved farming. Its theoretical concepts are based on control tactics from the 1940s, conceptualized most thoroughly in the 1950s by Stern et al. (1959). Its major goals have been greatly associated with reducing pest status, accepting tolerable pest levels, conserving natural enemies and environmental quality, and improving growers' profits. Achieving these economic and ecological goals typically requires pest monitoring and the use of the economic injury level (EIL) and economic threshold (ET) for the decisions about pest control.
Predominant reliance on pesticide-based pest control can cause long-term effects on sustainability; thus biological production systems are shifting agricultural practices and research (Ehler, 2006; Goulson, 2020; Bueno et al., 2021; Deguine et al., 2021). Hence, successful IPM programs rely on accurate decision making for implementation of a combination of selective and compatible strategies and tactics for dynamic agricultural systems (Pedigo et al., 1986; Higley and Pedigo, 1996; Stenberg, 2017). Innovative farming technologies, including global positioning systems, various sensors, automated traps, radars, drones, satellites, and robots are shaping new IPM programs (Peterson et al., 2018; Dara, 2019). Using gadgets that connect information through the internet of things (IoT) and artificial intelligence (AI), some farmers or practitioners have remote access to real-time information about crop development and health, insect population, weather, soil moisture, and many other parameters accessed anytime and anywhere.
Digital agriculture is considered an evolution of precision agriculture and is a widespread terminology in the field (Borém, 2020). New digital technologies have a great potential for insect monitoring and local pest management, especially before population outbreaks. Implementing tools for recording and analyzing big datasets from the soil, weather, plants, and insects are shaping modern agriculture (Heege, 2018). Enabling crop field monitoring at the plant level (or sometimes leaf level) can increase the adoption of the most efficient agronomic practices using precise knowledge about the location of infested plants (Greene et al., 1976; Oerke et al., 2010). Radars and automated insect traps provide information about where and when a given pest species arrives in the field (Lima et al., 2020; Preti et al., 2021a,b). Additionally, spectral remote sensing can be used for the detection of individual or groups of plants under attack (Yao et al., 2019; Marston et al., 2022). Digital platforms connecting insect monitoring data, crop development, and weather stations can help analyze area-wide outbreak risks, which can be used to predict population peaks of pests and natural enemies (Bajwa et al., 2003; Wang et al., 2019; Dutta et al., 2020). Some technologies also change the traditional way of delivering control solutions within the fields. Robotic sprayers and dispensers of biocontrol agents attached to drones can selectively deliver products only to the plants that need protection (Iost Filho et al., 2019).
The benefits of digital agriculture can be seen in the precise use of inputs. For instance, crop productivity and environmental resources are optimized if pesticides are precisely applied when and where they are needed, and in the necessary amounts (Corwin, 2013). While the traditional IPM approach is generally carried out with control solutions being delivered throughout the whole field or plots, new approaches involving digital technologies will need to consider adaptations in the concepts of ETs and EILs, sampling, injury identification, population forecasting, and ultimately the localized use of control tactics. Therefore, in this paper, we review how some traditional IPM concepts could be adjusted based on the ongoing digital transformation in agriculture.
Even though various specialists differentially define IPM, its strategies focus on sampling or monitoring procedures, knowing control and non-control levels, and selecting integrated control methods to be used (Kogan, 1998). For most field crops, chemical control through synthetic pesticides is the primary management tactic; however, there is a range of other tactics for pest control, such as biological control, pest-resistant and pest-tolerant cultivars, cultural control (crop rotation, intercropping, trap crops, manipulation of fertilizer and planting calendar, and elimination of crop residues), genetic control (sterile insect technique), and behavioral control (baits, traps, mating disruption techniques), alone or in combination with chemical control (attract-and-kill) (Govaerts et al., 2006; Huang et al., 2014; Alphey and Bonsall, 2018; Neves et al., 2018; Padilha et al., 2018; Blassioli-Moraes et al., 2019; Sharma et al., 2019; Nunes et al., 2020; Preti et al., 2020; Cardé, 2021; Jalli et al., 2021; Kovaleski and Mastrangelo, 2021; Adomako et al., 2022; Parra et al., 2022; Pazini et al., 2022).
References to the adoption of IPM over the last decades are commonly found (McDonald and Glynn, 1994; Farrar et al., 2016; Rossi et al., 2019; Bueno et al., 2021; Rahman, 2022). However, many farmers and practitioners are still reluctant to fully adopt IPM. The adoption of IPM is affected by several barriers, including the need for faster and easier pest sampling procedures, the “commercial pressure” from the agrochemical industry to the intensification of chemical control, and some cultural barriers such as the conviction that non-chemical pest control tactics are inefficient and that chemical pesticides solve all problems (Martins et al., 2016; Bueno et al., 2021). Moreover, one can say that the lack of incentives to use IPM and the complexity of its strategies can hamper the large adoption of this management system (Brewer and Goodell, 2012; Lefebvre et al., 2015).
An essential part of the decision-making process is diagnosing the problems in the field. However, in many cropping systems, thorough scouting is hampered by the lack of resources, both human and technological (Dara, 2019; Iost Filho et al., 2019). This is especially true in large fields, such as soybean, cotton, and maize fields in the Brazilian Central Region, being one of the reasons for the limited adoption of IPM in these areas and the large adoption of calendar-based pest control. New monitoring technology could help change this scenario. For instance, sensors are more widely available for precision fertilizer management and precision weed management than they are for precision pest management.
Strategies from IPM do not strictly apply to every situation, meaning that there are no one-size-fits-all solutions. On the contrary, IPM can be defined as a philosophy that guides the practitioners to use the best tools as appropriate for each situation (Dara, 2019). Regarding the control options, it is noteworthy the importance of not only knowing each tool but knowing how to use them (Peterson et al., 2018). Although a particular pest can be controlled by a given option, this may not be available in all regions, for all crops, at any time, or even at economically viable prices (Dara, 2019). For instance, a chemical pesticide may not be registered for all the crops where it could be used, a biological or behavioral tool may not be affordable in large fields, and a cultural control method cannot be used for the same crop under different cultivation system, among many other scenarios. For achieving the best results, control decisions should be made based on available and affordable control options (Dara, 2019).
Significant technological advances are drastically changing the decision-making components of IPM and expanding its tactical options. The most significant changes relate to applications of geographic information systems, precision agriculture, accuracy of weather-driven computational models for pest prediction, diffusion of real-time information to farmers, and real-time diagnostics. Changes in control tactics also are in substantial transformation, such as the wider use of drones to precisely deliver natural enemies in field crops, boosting the use of biological control (Iost Filho et al., 2019). Thus, all these aspects will be covered in the next sections.
Precision pest management is based on making decisions based on precise subfield-scale information about pest infestations instead of pest density averages at the field scale (Naud et al., 2020). The most significant advantages of precision pest management for crop sustainability are the reduction of pesticide use and the increased use of non-chemical tools targeted to populations in restricted parts of the field. Its central premise is to deliver control solutions only where and when pest populations reach economic levels (Park et al., 2007; Pedigo et al., 2021). Because the spatial distribution of many insects is aggregated (Davis, 1994), precision management can efficiently reduce the number of resources needed in some pest management situations (Park et al., 2007).
Responsive precision pest management programs are based on the creation of subfield management zones (Molin et al., 2015). The zones represent homogeneous field regions according to plant health and development. Thus, the objective of precision pest management is twofold: first, the detection of groups of plants attacked by insects (locating the zones); and second, the localized control on plants injured by insects (Figure 1). Assessing the distribution of insects and injured plants can be done by straightforward tools, such as visual inspection and global positioning technologies (Corwin, 2013). More recently, spectral remote sensing was indicated as a technology that can also identify injured plants (Nansen and Elliott, 2016).
Figure 1. Digital transformation in agriculture, leading to precision pest management (PPM), with the use of digital tools to monitor the fields and identify the regions (hotspots) where pest populations have reached the economic threshold (ET), and the delivery of control solutions only to the hotspots, instead of delivery to the whole area, as in integrated pest management (IPM). Adapted from Molin et al. (2015).
The detection of insect-induced stress using remote sensing is indirect. For example, plant spectral responses are mostly caused by changes in photosynthesis, physical plant structures, and pigments (Moran et al., 1997). The Normalized Difference Vegetative Index (NDVI), proposed by Rouse Jr et al. (1974), was initially used to determine the density of “green” on a patch of land. Its values are typically associated with biotic and abiotic stressors reducing chlorophyll content and leaf cell organization. This index became one of the most used strategies for creating management zones. However, different stressors can cause similar plant physiological responses, making it hard to identify which stressor is contributing to most of the changes in the NDVI values. For instance, how can we differentiate among stressors and create reliable prescription maps in a crop field where some plants are nutritionally deficient and under the attack of pests or plant pathogens simultaneously? Studies regarding this matter indicated the use of more spectral bands (e.g., hyperspectral sensors) and advanced analyzing methods can help selecting specific spectral bands, or spectral regions, for each stressor, such as different pest species, diseases, and nutrient deficiencies (Backoulou et al., 2013, 2015; Iost Filho, 2019; Ma et al., 2019; Nguyen and Nansen, 2020).
The spectral resolution is related to the quality of information produced. The most common sensors include the RGB (red, green, and blue), multispectral sensors (between 3 and 12 non-continuous wide spectral bands), and hyperspectral sensors with hundreds of continuous narrow spectral bands. The RGB sensors are cheap but provide limited spectral information. Multispectral sensors result in more spectral information, are easier to handle, are affordable and lightweight, and are commonly used in agriculture. Hyperspectral sensors are generally better at differentiating subtle differences in canopy reflectance (Yang et al., 2009). However, hyperspectral sensors are larger and heavier, more expensive, and produce more data that require higher storage capability and more time and experience for data analysis.
The spatial resolution plays an essential role in the decision-making process. Spatial resolution refers to the size of the pixels of the image. Field surface is represented by pixels that may vary from some meters in orbital platforms to a few centimeters in ground-based or aerial platforms (Iost Filho et al., 2019). For management purposes, spatial scale is defined by the area being treated. In this way, digital tools might be used for area-wide, whole-field, or site-specific management.
Although remote sensing has proven to be efficient for pest monitoring (Alves et al., 2015; Nansen and Elliott, 2016), the spectral responses vary depending on the location, type, moment, and intensity of the attack (Pinto et al., 2021). An extensive list of plant species, pest species, and the technology used to monitor them is available in Iost Filho et al. (2019). Most of the studies showing that remote sensing is effective for identifying stressed plants were conducted under controlled experimental conditions. Commercial applications of remote sensing necessitate further studies under field conditions, where multiple stressors can be affecting plants simultaneously. Temporal resolution (how often the sensor collects data from the field), repeatability, and accuracy can limit the use of remote sensing. Remote sensing data are usually obtained under different environmental conditions, causing variations that may affect the results and generate unsatisfactory accuracy levels. The quality of information is also related to the data processing methods (Martins et al., 2017). A current challenge for analyzing spectral data is the access to even faster computers and higher capacity data storage gadgets (Caballero et al., 2020).
Remote sensing is typically associated with imaging sensors but accessing information from a distance is not limited to spectral information only. Insect-induced volatiles released in the air can also be detected by real-time sensors (Tholl et al., 2021). Analysis of DNA sequences of insect-borne pathogens and plant fluorescence are becoming more sensitive, inexpensive, and portable for real-time field applications (Donoso and Valenzuela, 2018). Radars represent another remote sensing technology that can provide information about insect-pest dispersal. Studies have shown the potential of using meteorological radars to monitor insect migration, including detailed information like flight activity, trajectory, population size, and species identification (Drake, 2002; Drake et al., 2017; Poffo et al., 2018).
Some of the digital technologies shown here facilitate data-driven decisions from information collected, processed, and transformed into management zones. This process might take some time; thus, the next logical step is the establishment of management decisions as soon as the monitoring data are collected. Technologies that provide crop managers with real-time information rely on an interconnected system of sensors, hardware, software, connectivity web, and applications (sometimes called the ‘internet of things' or IoT) that allow consumers, companies, and government to connect data gadgets in the most diverse environments (Oliveira, 2020).
Smart traps represent a real-time technology to monitor the presence of insect pests in strategic parts of the field. They are an evolution of the traditional methods to monitor pests in commercial attractive traps and periodic in-field inspection, which is a laborious and slow process. A case of success in the evolution of this technology was reported in apple orchards to monitor moths. The first evolution of this process was based on wireless cameras that sent full images for remote classification (Ding and Taylor, 2016). Although this system uses machine learning (ML) to analyze the images collected, it demands time, storage, and processing power because of the size of the full images. Thus, researchers developed a system that processes the picture in situ using a preprocessing algorithm, returns a classification of the insects using an ML algorithm, and sends a notification to the crop manager (Brunelli et al., 2019; Preti et al., 2021a,b). All these steps are done in the hardware located in the trap (i.e., cloudless IoT).
The integration of multilayer information can promote more accurate control decisions. A general multilayer architecture includes the soil and crop layer (the physical component of the agricultural land and the crop present on it) interacting with the rest of the architecture, the actuators layer (with the distribution layer representing the tools to deliver the solutions to specific areas within the field), the sensors layer (representing the tools available to collect data from the soil and plants), a networking layer (connecting all the layers), and, finally, the application layer (with cloud services to process all data and transform them into useful information) (Naud et al., 2020). The integration of data is especially important in the context of pest management because of the dynamics of pest populations in space and time (Venette et al., 2010), as well as the high dynamism of their environmental drivers, such as temperature, radiation, and humidity (Méndez-Vázquez et al., 2019). However, data come at different resolutions, and some data are acquired at a resolution that differs from the scale at which the decision must be made. Therefore, data processing is imperative. Moreover, technically trained interpretation should be capable of delineating biologically and agronomically meaningful zones.
Social and economic questions are raised when digital agriculture projects are planned. For instance, digital agriculture is primarily targeted at large growers because of the cost of investing in technology (Pedersen et al., 2020). Hence, how can these tools, equipment, and systems become more affordable and accessible for small growers?
Various players should be involved in the process of improving digital pest management programs, including companies, researchers, extensionists, farmers, consultants, and regulators. Hence, another social concern is related to data ownership and sharing because of the large number of people working on the different components of the project. Instead of using data in opportunistic ways, end-user license agreements, algorithms, and company policies should aim for the protection of data sovereignty (Fraser, 2019; Hummel et al., 2021). Discussions about data stewardship agreements and open-source formats should increase awareness about the benefits and concerns of contemporary technology services and integration of multilayer data.
Regarding the operational component, a critical question is how precisely can we estimate the infestation level of target pests? One must understand the clear difference between classifying infested or non-infested areas and classifying areas regarding the infestation levels. In other words, it requires different analytical techniques to assess the infestation levels or (at least) the infested/not infested zones. For practical management decisions, some studies relied on the knowledge of EILs for different pests and classified areas within a field as above/below the threshold (Alves et al., 2019; Iost Filho et al., 2022; Marston et al., 2022).
Many aspects such as pest biology and ecology, crop phenology, available control tactics and their costs, weather, crop economic value, and field size should be considered to transform remotely collected infestation data into control decisions. Knowing where the pest populations are found in the field (hotspots) does not necessarily mean that one knows where and when to manage the pest precisely. We should consider that not all populations found in the hotspots will develop into economically damaging populations based on pest biology/ecology and crop economics. Hence, how can we determine the size of the hotspot and intensity of infestation for these hotspots that require treatment? Also, should we consider how accurate and efficient is the delivery of the control solution? These questions worry researchers and producers because different results can be achieved, such as correct/incorrect treatment decisions and correct/incorrect no-treat decisions. Regardless of many studies showing the economic benefits of using precision pest management (Park et al., 2007; Sana, 2020), little is known about the economic impact of incorrect decisions.
Another challenge is related to the adaptation of ETs with the use of digital tools. For example, if technology provides us precise information about the location of a given pest population in the field, should we consider controlling this population even before it reaches ET, and therefore prevent this initial population from spreading over the field? Or, should we continue following the ET guidelines, but controlling the pest at the sub-field level? To answer these, among other questions, it is important to consider the level of injury caused by such a population, its rates of spread, and population growth. Regarding insect-borne plant pathogens (viruses, bacteria such as mollicutes phytoplasmas and spiroplasmas, and fungi), control strategies targeting the vectors should consider that even low populations can transmit the disease throughout the field. In this case, digital tools could improve vector-pest management by enhancing the monitoring of populations at low levels (i.e., early detection).
Additionally, sensors can be used to monitor host susceptibility to the vector since infected plants tend to become more attractive or acceptable to the vectors (Eigenbrode et al., 2018). An example is the pathosystem composed of Liberibacter- or phytoplasma-infected hosts, which are preferred over non-infected plants for settling by their psyllid vectors (Mayer et al., 2011; Davis et al., 2012). This approach can be understood as the next step in the advancement of precision pest management, where the focus will be on monitoring host susceptibility to pests instead of finding patches of the field already attacked by the pest.
The choice of digital technologies can vary based on the scale of interest (Thomas et al., 2018). Is the primary scale the plant tissue, leaves, individual plants, and canopies? Tissue- and leaf-scale studies are usually conducted in the laboratory as basic research. In contrast, plant- and group-of-plants-scale studies tend to be carried out at the greenhouse and field levels (Barros et al., 2021). With advances in monitoring and control tools, management strategies might be taken at the plant level (or group of plants) in the future. To precisely deliver solutions at such a level for pest management, technologies currently in use for precision nutrient management will need to be adapted.
Weather sensors from IoT devices have great potential for insect forecasting in digital pest management programs (Chen et al., 2022). Although the cost of weather sensors has been decreasing, adding a weather station into each field can still be costly for implementation and maintenance. Field devices can also create difficulties for agricultural machinery practices. For over 20 years, grids of weather stations and numerical weather models have been used in subfield spatio-temporal projections (Russo, 2000; Olantinwo and Hoogenboom, 2014; Lalic et al., 2016; Kim et al., 2020). Therefore, weather data networks and numerical grid datasets are probably the most cost-effective, reliable strategy to forecast the occurrence and distribution of insects (Merrill et al., 2015; Newlands, 2018; Hu et al., 2019; Wang et al., 2019). Integrating weather grid data with current and historical pest scouting data, machine learning technologies, and remote sensing can provide increasingly fine-scale information for IPM.
Ecological modeling of insect movement is another important tool to be considered. Garcia et al. (2021) presented different approaches to model the movement of the cucurbit beetle Diabrotica speciosa Germar (Coleoptera: Chrysomelidae) in intercropping systems, and of the fall armyworm Spodoptera frugiperda (J. E. Smith) (Lepidoptera: Noctuidae) in transgenic (Bt) and conventional maize fields. The information provided in this study can be used for planning actions to delay insect resistance to transgenic crops. Aerobiology simulations can also guide scouting and create threat alerts to pests that use atmosphere to spread (Isard et al., 2007).
Berra (2003), in the chapter “IPM in the future,” predicted that pest forecasting, improved thresholds and monitoring, data storage and retrieval for the recommendation, computer digital imaging technology, and precision farming would indicate directions of possible changes at that time. We see that all of that is shaping today's agriculture. Similarly, we try to predict near future by asking what are the next advances in IPM due to the use of autonomous vehicles (both aerial and ground), plant spectral information, volatiles, and other data connected through digital platforms? Probably, the biggest advance will be related to how fast such data will be analyzed and transformed into management decisions, such as real-time alerts to growers.
Due to the increasing number of tools developed almost daily, it is essential to advance inter- and multidisciplinary research to extract the maximum potential from related areas of science. Areas that need advancements include, but are not limited to, understanding the physiological processes of stressed plants, analysis and processing of data, development of sensors and collection platforms, and optimization of data acquisition and solution delivery tools. Hence, there are opportunities for collaboration on new projects, including researchers from different institutions and countries, and with diverse backgrounds, including computer science, sensors, computer engineering, and entrepreneurship.
Regarding the practical aspects of IPM, it is necessary to understand that the detection of pest populations for making an appropriate decision about control still relies on periodic sampling, which is challenging in some crop systems. Some of the monitoring challenges could be overcome by using digital tools. Another benefit of precision pest management is the natural mortality of pests (e.g., biotic and abiotic mortality factors) in the agricultural ecosystem, which is an important basis of IPM programs, but is often neglected. Hence, control decisions considering management only in the hotspots, instead of whole-field treatments, could help maintain natural enemies in the field, therefore boosting natural mortality. These benefits, among others, could possibly lead to an increase in the adoption of IPM in agriculture.
Conceptualization and writing—review and editing: FI, JP, TA, RK, and PY. Writing—original draft: FI and JP. All authors have read and agreed to the published version of the manuscript.
This study was financed by the Fundação de Amparo e Pesquisa do Estado de São Paulo (FAPESP), Project Nos. 2017/19407-4, 2018/02317-5, 2019/26099-0, and 2019/26145-1.
We would like to thank Dr. Arthur Vieira Ribeiro for his suggestions in the draft. Also, we thank the reviewers who improved this manuscript with their valuable suggestions.
The authors declare that the research was conducted in the absence of any commercial or financial relationships that could be construed as a potential conflict of interest.
All claims expressed in this article are solely those of the authors and do not necessarily represent those of their affiliated organizations, or those of the publisher, the editors and the reviewers. Any product that may be evaluated in this article, or claim that may be made by its manufacturer, is not guaranteed or endorsed by the publisher.
Adomako, J., Yeboah, S., Larbi-Koranteng, S., Kankam, F., Oppong-Sekyere, D., Nboyine, J. A., et al. (2022). “Transgenic plants and its role in insect control,” in New and Future Development in Biopesticide Research: Biotechnological Exploration, eds S. D. Mandal, G. Ramkumar, S. Karthi, and F. Jin (Singapore: Springer), 203–222. doi: 10.1007/978-981-16-3989-0_8
Alphey, N., and Bonsall, M. B. (2018). Genetics-based methods for agricultural insect pest management. Agric. For. Entomol. 20, 131–140. doi: 10.1111/afe.12241
Alves, T. M., Macrae, I. V., and Koch, R. L. (2015). Soybean aphid (Hemiptera: Aphididae) affects soybean spectral reflectance. J. Econom. Entomol. 108, 2655–2664. doi: 10.1093/jee/tov250
Alves, T. M., Moon, R. D., MacRae, I. V., and Koch, R. L. (2019). Optimizing band selection for spectral detection of Aphis glycines Matsumura in soybean. Pest Manag. Sci. 75, 942–949. doi: 10.1002/ps.5198
Backoulou, G. F., Elliott, N. C., Giles, K. L., and Mirik, M. (2015). Processed multispectral imagery differentiates wheat crop stress caused by greenbug from other causes. Comput. Electron. Agric. 115, 34–39. doi: 10.1016/j.compag.2015.05.008
Backoulou, G. F., Elliott, N. C., Giles, K. L., and Rao, M. N. (2013). Differentiating stress to wheat fields induced by Diuraphis noxia from other stress causing factors. Comput. Electron. Agric. 90, 47–53. doi: 10.1016/j.compag.2012.09.013
Bajwa, W. I., Coop, L., and Kogan, M. (2003). Integrated pest management (IPM) and internet-based information delivery systems. Neotrop. Entomol. 32, 373–383. doi: 10.1590/S1519-566X2003000300001
Barros, P. P. S., Iost Filho, F. H., Rosalen, D. L., Martins, G. D., and Di Leo, N. (2021). Monitoring plant health using remote sensing: advances and challenges. Rev. Bras. Cartograf. 73, 489–515. doi: 10.14393/rbcv73n2-56597
Berra, Y. (2003). “IPM in the future,” in Concepts in Integrated Pest Management, eds. R. F. Norris, E. P. Caswell-Chen, and M. Kogan (Upper Saddle River: Pearson), 514–521.
Blassioli-Moraes, M. C., Laumann, R. A., Michereff, M. F. F., and Borges, M. (2019). “Semiochemicals for integrated pest management,” in Sustainable Agrochemistry, ed. S. Vaz Júnior (Cham: Springer), 85–112. doi: 10.1007/978-3-030-17891-8_3
Borém, A. (2020). “Nova revolução verde,” in Agricultura Digital, eds D. M. de Queiroz, D. S. M. Valente, F. D. A. de Carvalho Pinto, and A. Borém (Viçosa: Brasil, Oficina de Textos), 9–26.
Brewer, M. J., and Goodell, P. B. (2012). Approaches and incentives to implement integrated pest management that addresses regional and environmental issues. Ann. Rev. Entomol. 57, 41–59. doi: 10.1146/annurev-ento-120709-144748
Brunelli, D., Albanese, A., d'Acunto, D., and Nardello, M. (2019). Energy neutral machine learning based IoT device for pest detection in precision agriculture. IEEE Internet Things. 2, 10–13. doi: 10.1109/IOTM.0001.1900037
Bueno, A. D. F., Panizzi, A. R., Hunt, T. E., Dourado, P. M., Pitta, R. M., and Gonçalves, J. (2021). Challenges for adoption of Integrated Pest Management (IPM): the soybean example. Neotrop. Entomol. 50, 5–20. doi: 10.1007/s13744-020-00792-9
Caballero, D., Calvini, R., and Amigo, J. M. (2020). “Hyperspectral imaging in crop fields: Precision agriculture,” in Data Handling in Science and Technology, vol. 32, ed. J. M. Amigo (Amsterdam, Netherlands: Elsevier), 453–473. doi: 10.1016/B978-0-444-63977-6.00018-3
Cardé, R. T. (2021). “Mating disruption with pheromones for control of moth pests in area-wide management programmes,” in Area-wide Integrated Pest Management: Development and Field Application, eds J. Hendrichs, R. Pereira, and M. J. B. Vreysen (Boca Raton: CRC Press), 779–794. doi: 10.1201/9781003169239-45
Chen, C. J., Li, Y. S., Tai, C. Y., Chen, Y. C., and Huang, Y. M. (2022). Pest incidence forecasting based on internet of things and long short-term memory network. Appl. Soft Comput. 124, 108895. doi: 10.1016/j.asoc.2022.108895
Corwin, D. L. (2013). “Site-specific management and delineating management zones,” in Precision Agriculture for Sustainability and Environmental Protection, ed. M. A. Oliver, T. F. Bishop, and B. P. Marchant (Abingdon: Routledge), 135–157.
Dara, S. K. (2019). The new integrated pest management paradigm for the modern age. J. Integrat. Pest Manage. 10, 1–9. doi: 10.1093/jipm/pmz010
Davis, P. M. (1994). “Statistics for describing populations,” in Handbook of Sampling Methods for Arthropods in Agriculture, eds L. P. Pedigo, and G. D. Buntin (Boca Raton, FL: CRC Press), 33–54. doi: 10.1201/9781003067900-4
Davis, T. S., Horton, D. R., Munyaneza, J. E., and Landolt, P. J. (2012). Experimental infection of plants with an herbivore-associated bacterial endosymbiont influences herbivore host selection behavior. PLOS ONE 7, e49330. doi: 10.1371/journal.pone.0049330
Deguine, J. P., Aubertot, J. N., Flor, R. J., Lescourret, F., Wyckhuys, K. A., and Ratnadass, A. (2021). Integrated pest management: good intentions, hard realities. A review. Agron. Sustain. Dev. 41, 1–35. doi: 10.1007/s13593-021-00689-w
Ding, W., and Taylor, G. (2016). Automatic moth detection from trap images for pest management. Comput Electron Agric. 123, 17–28. doi: 10.1016/j.compag.2016.02.003
Donoso, A., and Valenzuela, S. (2018). In-field molecular diagnosis of plant pathogens: recent trends and future perspectives. Plant Pathol. 67, 1451–1461. doi: 10.1111/ppa.12859
Drake, A. (2002). Automatically operating radars for monitoring insect pest migrations. Insect Sci. 9, 27–39. doi: 10.1111/j.1744-7917.2002.tb00169.x
Drake, V. A., Chapman, J. W., Lim, K. S., Reynolds, D. R., Riley, J. R., and Smith, A. D. (2017). Ventral-aspect radar cross sections and polarization patterns of insects at X band and their relation to size and form. Int. J. Remote Sens. 38, 5022–5044. doi: 10.1080/01431161.2017.1320453
Dutta, J., Dutta, J., and Gogoi, S. (2020). Smart farming: an opportunity for efficient monitoring and detection of pests and diseases. J. Entomol. Zool. Stud. 8, 2352–2359. doi: 10.22271/j.ento.2020.v8.i4ab.7392
Ehler, L. E. (2006). Integrated Pest Management (IPM) definition, historical development and implementation, and the other IPM. Pest Manage. Sci. 62, 787–789. doi: 10.1002/ps.1247
Eigenbrode, S. D., Bosque-Pérez, N. A., and Davis, T. S. (2018). Insect-borne plant pathogens and their vectors: ecology, evolution, and complex interactions. Annu. Rev. Entomol. 63, 169–191. doi: 10.1146/annurev-ento-020117-043119
Farrar, J. J., Baur, M. E., and Elliott, S. F. (2016). Adoption of IPM practices in grape, tree fruit, and nut production in the Western United States. J. Integr. Pest Manag. 7:pmw007. doi: 10.1093/jipm/pmw007
Fraser, A. (2019). Land grab/data grab: precision agriculture and its new horizons. J. Peasant Stud. 46, 893–912. doi: 10.1080/03066150.2017.1415887
Garcia, A. G., Malaquias, J. B., Ferreira, C. P., Tomé, M. P., Weber, I. D., and Godoy, W. A. C. (2021). Ecological modelling of insect movement in cropping systems. Neotrop. Entomol. 50, 321–334. doi: 10.1007/s13744-021-00869-z
Goulson, D. (2020). Pesticides, corporate irresponsibility, and the fate of our planet. One Earth 2, 302–305. doi: 10.1016/j.oneear.2020.03.004
Govaerts, B., Mezzalama, M., Sayre, K. D., Crossa, J., Nicol, J. M., and Deckers, J. (2006). Long-term consequences of tillage, residue management, and crop rotation on maize/wheat root rot and nematode populations in subtropical highlands. Appl. Soil Ecol. 32, 305–315. doi: 10.1016/j.apsoil.2005.07.010
Greene, G. L., Leppla, N. C., and Dickerson, W. A. (1976). Velvetbean caterpillar: a rearing procedure and artificial medium. J. Econ. Entomol. 69, 487–488. doi: 10.1093/jee/69.4.487
Higley, L. G., and Pedigo, L. P. (1996). Economic Thresholds for Integrated Pest Management. Lincoln: University of Nebraska Press.
Hu, G., Lu, M., H., Reynolds, D. R., Wang, H. K., Chen, X., et al. (2019). Long-term seasonal forecasting of a major migrant insect pest: the brown planthopper in the Lower Yangtze River Valley. J. Pest Sci. 92, 417–428. doi: 10.1007/s10340-018-1022-9
Huang, S., Wang, L., Liu, L., Fu, Q., and Zhu, D. (2014). Nonchemical pest control in China rice: a review. Agron. Sustain. Dev. 34, 275–291. doi: 10.1007/s13593-013-0199-9
Hummel, P., Braun, M., Tretter, M., and Dabrock, P. (2021). Data sovereignty: a review. Big Data Society 8, 2053951720982012. doi: 10.1177/2053951720982012
Iost Filho, F. H. (2019). Sensoriamento remoto para monitoramento de mosca branca, Bemisia tabaci biótipo B (Hemiptera: Aleyrodidae) em soja. Master's thesis. Piracicaca: University of São Paulo
Iost Filho, F. H., Heldens, W. B., Kong, Z., and Lange, E. S. (2019). Drones: innovative technology for use in precision pest management. J. Econ. Entomol. 113, 1–25. doi: 10.1093/jee/toz268
Iost Filho, F. H., Pazini, J., de, B., Medeiros, A. D., Rosalen, D. L., and Yamamoto, P.T. (2022). Assessment of injury by four major pests in soybean plants using hyperspectral proximal imaging. Agronomy. 12, 1516. doi: 10.3390/agronomy12071516
Isard, S. A., Russo, J. M., and Ariatti, A. (2007). The Integrated Aerobiology Modeling System applied to the spread of soybean rust into the Ohio River valley during September 2006. Aerobiologia 23, 271–282. doi: 10.1007/s10453-007-9073-z
Jalli, M. J., Huusela, E., Jalli, H., Kauppi, K., Niemi, M., Himanen, S., et al. (2021). Effects of crop rotation on spring wheat yield and pest incidence in different tillage systems: a multi-year experiment in Finnish growing conditions. Front. Sustain. Food Syst. 5, 214–228. doi: 10.3389/fsufs.2021.647335
Kim, H. S., Do, K. S., Park, J. H., Kang, W. S., Lee, Y. H., and Park, E. W. (2020). Application of numerical weather prediction data to estimate infection risk of bacterial grain rot of rice in Korea. Plant Path. J. 36, 54–66. doi: 10.5423/PPJ.OA.11.2019.0281
Kogan, M. (1998). Integrated pest management: historical perspectives and contemporary developments. Annu. Rev. Entomol. 43, 243–270. doi: 10.1146/annurev.ento.43.1.243
Kovaleski, A., and Mastrangelo, T. (2021). “Moscasul Programme: first steps of a pilot project to suppress the south american fruit fly in Southern Brazil,” in Area-wide Integrated Pest Management: development and field application, eds J. Hendrichs, R. Pereira, and M. J. B. Vreysen (Boca Raton: CRC Press), 215–230. doi: 10.1201/9781003169239-12
Lalic, B., Francia, M., Eitzinger, J., Podraščanin, Z., and Arsenić, I. (2016). Effectiveness of short-term numerical weather prediction in predicting growing degree days and meteorological conditions for apple scab appearance. Meteorol. Appl. 23, 50–56. doi: 10.1002/met.1521
Lefebvre, M., Langrell, S. R., and Gomez-y-Paloma, S. (2015). Incentives and policies for integrated pest management in Europe: a review. Agron. Sustain Dev. 35, 27–45. doi: 10.1007/s13593-014-0237-2
Lima, M. C. F., Leandro, M. E. D. A., Valero, C., Coronel, L. C. P., and Bazzo, C. O. G. (2020). Automatic detection and monitoring of insect pests—a review. Agriculture. 10, 161. doi: 10.3390/agriculture10050161
Ma, H., Huang, W., Jing, Y., Yang, C., Han, L., Dong, Y., et al. (2019). Integrating growth and environmental parameters to discriminate powdery mildew and aphid of winter wheat using bi-temporal Landsat-8 imagery. Remote Sens. 11, 846. doi: 10.3390/rs11070846
Marston, Z. P., Cira, T. M., Knight, J. F., Mulla, D., Alves, T. M., Hodgson, E. W., et al. (2022). Linear support vector machine classification of plant stress from soybean aphid (Hemiptera: Aphididae) using hyperspectral reflectance. J. Econom. Entomol. 2022:toac077. doi: 10.1093/jee/toac077
Martins, G. D., Galo, M. D. L. B.T., and Vieira, B. S. (2017). Detecting and mapping root-knot nematode infection in coffee crop using remote sensing measurements. IEEE J. Sel. Top. Appl. 10, 5395–5403. doi: 10.1109/JSTARS.2017.2737618
Martins, J. F. S., Silva, F. F., Theisen, G., Mattos, M. L. T., Botta, R. A., and Nunes, C. D. M. (2016). Diagnóstico sobre o manejo de agrotóxicos em áreas orizícolas da Fronteira Oeste do Rio Grande do Sul. Pelotas: Embrapa Clima Temperado.
Mayer, C. J., Vilcinskas, A., and Gross, J. (2011). Chemically mediated multitrophic interactions in a plant-insect vector-phytoplasma system compared with a partially nonvector species. Agric. For. Entomol. 13, 25–35. doi: 10.1111/j.1461-9563.2010.00495.x
McDonald, D. G., and Glynn, C. J. (1994). Difficulties in measuring adoption of apple IPM: a case study. Agric. Ecosyst. Environ. 48, 219–230. doi: 10.1016/0167-8809(94)90104-X
Méndez-Vázquez, L. J., Lira-Noriega, A., Lasa-Covarrubias, R., and Cerdeira-Estrada, S. (2019). Delineation of site-specific management zones for pest control purposes: exploring precision agriculture and species distribution modeling approaches. Comput. Electron. Agric. 167, 101–105. doi: 10.1016/j.compag.2019.105101
Merrill, S. C., Holtzer, T. O., Peairs, F. B., and Lester, P. J. (2015). Validating spatiotemporal predictions of an important pest of small grains. Pest Manag. Sci. 71, 131–138. doi: 10.1002/ps.3778
Molin, J. P., do Amaral, L. R., and Colaço, A. (2015). Agricultura de precisão. Viçosa, Brasil: Oficina de textos.
Moran, M. S., Inoue, Y., and Barnes, E. M. (1997). Opportunities and limitations for image based remote sensing in precision crop management. Remote Sens. Environ. 61, 319–346. doi: 10.1016/S0034-4257(97)00045-X
Nansen, C., and Elliott, N. (2016). Remote sensing and reflectance profiling in entomology. Ann. Rev. Entomol. 61, 139–158. doi: 10.1146/annurev-ento-010715-023834
Naud, O., Taylor, J., Colizzi, L., Giroudeau, R., Guillaume, S., Bourreau, E., et al. (2020). “Support to decision-making,” in Agricultural Internet of Things and Decision Support for Precision Smart Farming, eds A. Castrignanò, G. Buttafuoco, R. Khosla, A. Mouazen, D. Moshou, and O. Naud (London, UK: Academic Press), 183–224. doi: 10.1016/B978-0-12-818373-1.00004-4
Neves, R. C. D. S., Torres, J. B., Barros, E. M., and Vivan, L. M. (2018). Boll weevil within season and off-season activity monitored using a pheromone-and-glue reusable tube trap. Sci. Agric. 75, 313–320. doi: 10.1590/1678-992x-2016-0450
Newlands, N. K. (2018). Model-based forecasting of agricultural crop disease risk at the regional scale, integrating airborne inoculum, environmental, and satellite-based monitoring data. Front. Environ. Sci. 6, 63. doi: 10.3389/fenvs.2018.00063
Nguyen, H. D., and Nansen, C. (2020). Hyperspectral remote sensing to detect leaf miner-induced stress in bok choy and spinach according to fertilizer regime and timing. Pest Manag. Sci. 76, 2208–2216. doi: 10.1002/ps.5758
Nunes, M. Z., Baronio, C. A., Schutze, I. X., Bernardi, D., Arioli, C. J., Machota Junior, R., et al. (2020). Toxicity of baits and their effects on population suppression of Anastrepha fraterculus (Diptera: Tephritidae): implications for field management. Environ. Entomol. 49, 638–644. doi: 10.1093/ee/nvaa035
Oerke, E. C., Gerhards, R., Menz, G., and Sikora, R. A. (2010). Precision Crop Protection-The Challenge and Use of Heterogeneity. Dordrecht: Springer. doi: 10.1007/978-90-481-9277-9
Olantinwo, R., and Hoogenboom, G. (2014). “Weather-based pest forecasting for efficient crop protection,” in Integrated Pest Management (New York, NY: Academic Press), 59–78. doi: 10.1016/B978-0-12-398529-3.00005-1
Oliveira, H. S. (2020). “Internet das coisas para agricultura,” in Agricultura Digital, eds D. M. de Queiroz, D. S. M. Valente, F. D. A. de Carvalho Pinto, and A. Borém (Viçosa: Brasil, Oficina de Textos), 216–238.
Padilha, A. C., Arioli, C. J., Boff, M. I. C., Rosa, J. M., and Botton, M. (2018). Traps and baits for luring Grapholita molesta (Busck) adults in mating disruption-treated apple orchards. Neotrop. Entomol. 47, 152–159. doi: 10.1007/s13744-017-0517-z
Park, Y. L., Krell, R. K., and Carroll, M. (2007). Theory, technology, and practice of site-specific insect pest management. J. Asia-Pacific Entomol. 10, 89–101. doi: 10.1016/S1226-8615(08)60337-4
Parra, J. R. P., Garcia, A. G., Diniz, A. J. F., and Bento, J. M. S. (2022). Sustainability in Brazilian citriculture: three decades of successful biological control of insect pests. Front. Agron. 4, 874188. doi: 10.3389/fagro.2022.874188
Pazini, J., de, B., Grützmacher, A. D., Seidel, E. J., Padilha, A. C., Silva, F. F., et al. (2022). Field assessment of Oryzophagus oryzae (Coleoptera: Curculionidae) preference and performance on selected rice cultivars. J. Econ. Entomol. 115, 671–681. doi: 10.1093/jee/toac003
Pedersen, S. M., Pedersen, M. F., Ørum, J. E., Fountas, S., Balafoutis, A. T., van Evert, F. K., et al. (2020). “Economic, environmental and social impacts,” in Agricultural Internet of Things and Decision Support for Precision Smart Farming, eds A. Castrignanò, G. Buttafuoco, R. Khosla, A. Mouazen, D. Moshou, O. Naud. (London, UK: Academic Press), 183–224. doi: 10.1016/B978-0-12-818373-1.00006-8
Pedigo, L. P., Hutchins, S. S. H., and Higley, L. G. (1986). Economic injury levels in theory and practice. Annu. Rev. Entomol. 31, 341–368. doi: 10.1146/annurev.en.31.010186.002013
Pedigo, L. P., Rice, M. E., and Krell, R. K. (2021). Entomology and Pest Management. Long Grove, IL: Waveland Press.
Peterson, R. K. D., Higley, L. G., and Pedigo, L. P. (2018). Whatever happened to IPM? Am. Entomol. 64, 146–150. doi: 10.1093/ae/tmy049
Pinto, J., Powell, S., Peterson, R., Rosalen, D., and Fernandes, O. (2021). Detection of defoliation injury in peanut with hyperspectral proximal remote sensing. Remote Sens. 12, 3828. doi: 10.3390/rs12223828
Poffo, D. A., Beccacece, H. M., Caranti, G. M., Comes, R. A., Drewniak, M. E., Martina, A., et al. (2018). Migration monitoring of Ascia monuste (Lepidoptera) and Schistocerca cancellata (Orthoptera) in Argentina using RMA1 weather radar. ISPRS J. Photogramm. Remote Sens. 145, 340–348. doi: 10.1016/j.isprsjprs.2018.05.011
Preti, M., Knight, A. L., and Angeli, S. (2020). Improved monitoring of Grapholita molesta (Lepidoptera: Tortricidae) in stone fruit orchards with a pheromone-kairomone combination lure. Insects 11, 412. doi: 10.3390/insects11070412
Preti, M., Moretti, C., Scarton, G., Giannotta, G., and Angeli, S. (2021b). Developing a smart trap prototype equipped with camera for tortricid pests remote monitoring. Bull. Insectol. 74, 147–160. Available online at: http://www.bulletinofinsectology.org/pdfarticles/vol74-2021-147-160preti.pdf
Preti, M., Verheggen, F., and Angeli, S. (2021a). Insect pest monitoring with camera-equipped traps: strengths and limitations. J. Pest Sci. 94, 203–217. doi: 10.1007/s10340-020-01309-4
Rahman, M. S. (2022). Farmers' perceptions of integrated pest management (IPM) and determinants of adoption in vegetable production in Bangladesh. Int. J. Pest Manag. 68, 158–166. doi: 10.1080/09670874.2020.1807653
Rossi, V., Sperandio, G., Caffi, T., Simonetto, A., and Gilioli, G. (2019). Critical success factors for the adoption of decision tools in IPM. Agronomy 9, 710. doi: 10.3390/agronomy9110710
Rouse Jr, J. W., Haas, R. H., Deering, D. W., Schell, J. A., and Harlan, J. C. (1974). Monitoring the Vernal Advancement and Retrogradation (green wave effect) of Natural Vegetation (No. E75–10354).
Russo, J. M. (2000). “Weather forecasting for IPM,” in Emerging Technologies for Integrated Pest Management: Concepts, Research, and Implementation, eds G. G. Kennedy and T. B. Sutton (St. Paul, MN: American Phytopathological Society), 453–73.
Sana, R. S. (2020). “Estudo de caso SLC,” in Agricultura Digital, eds D. M. de Queiroz, D. S. M. Valente, F. D. A. de Carvalho Pinto, and A. Borém (Viçosa: Brasil, Oficina de Textos), 326–343.
Sharma, A., Shrestha, G., and Reddy, G. V. P. (2019). Trap crops: how far we are from using them in cereal crops? Ann. Entomol. Soc. Am. 112, 330–339. doi: 10.1093/aesa/say047
Stenberg, J. A. (2017). A conceptual framework for integrated pest management. Trends in Plant. Sci. 22, 759–769. doi: 10.1016/j.tplants.2017.06.010
Stern, V. M., Smith, R. F., Van Den Bosch, R., and Hagen, K. S. (1959). The integrated control concept. Hilgardia 29, 81–101. doi: 10.3733/hilg.v29n02p081
Tholl, D., Hossain, O., Weinhold, A., Röse, U. S., and Wei, Q. (2021). Trends and applications in plant volatile sampling and analysis. Plant J. 106, 314–325. doi: 10.1111/tpj.15176
Thomas, S., Kuska, M. T., Bohnenkamp, D., Brugger, A., Alisaac, E., Wahabzada, M., et al. (2018). Benefits of hyperspectral imaging for plant disease detection and plant protection: a technical perspective. J. Plant Dis. Protect. 125, 5–20. doi: 10.1007/s41348-017-0124-6
Venette, R. C., Kriticos, D. J., Magarey, R. D., Koch, F. H., Baker, R. H., Worner, S. P., et al. (2010). Pest risk maps for invasive alien species: a roadmap for improvement. BioScience 60, 349–362. doi: 10.1525/bio.2010.60.5.5
Wang, H. H., Grant, W. E., Elliott, N. C., Brewer, M. J., Koralewski, T. E., Westbrook, J. K., et al. (2019). Integrated modelling of the life cycle and aeroecology of wind-borne pests in temporally-variable spatially-heterogeneous environment. Ecol. Model. 399, 23–38. doi: 10.1016/j.ecolmodel.2019.02.014
Yang, C., Everitt, J. H., Bradford, J. M., and Murden, D. A. L. E. (2009). Comparison of airborne multispectral and hyperspectral imagery for estimating grain sorghum yield. Trans. ASABE 52, 641–649. doi: 10.13031/2013.26816
Keywords: integrated pest management (IPM), precision pest management, precision agriculture (PA), crop protection, food system, pesticide reduction, early detection and prevention
Citation: Iost Filho FH, Pazini JdB, Alves TM, Koch RL and Yamamoto PT (2022) How does the digital transformation of agriculture affect the implementation of Integrated Pest Management? Front. Sustain. Food Syst. 6:972213. doi: 10.3389/fsufs.2022.972213
Received: 18 June 2022; Accepted: 08 November 2022;
Published: 30 November 2022.
Edited by:
Surendra K. Dara, Oregon State University, United StatesReviewed by:
Roger D. Magarey, North Carolina State University, United StatesCopyright © 2022 Iost Filho, Pazini, Alves, Koch and Yamamoto. This is an open-access article distributed under the terms of the Creative Commons Attribution License (CC BY). The use, distribution or reproduction in other forums is permitted, provided the original author(s) and the copyright owner(s) are credited and that the original publication in this journal is cited, in accordance with accepted academic practice. No use, distribution or reproduction is permitted which does not comply with these terms.
*Correspondence: Fernando Henrique Iost Filho, ZmVybmFuZG9oaW9zdEB1c3AuYnI=
†These authors have contributed equally to this work and share first authorship
Disclaimer: All claims expressed in this article are solely those of the authors and do not necessarily represent those of their affiliated organizations, or those of the publisher, the editors and the reviewers. Any product that may be evaluated in this article or claim that may be made by its manufacturer is not guaranteed or endorsed by the publisher.
Research integrity at Frontiers
Learn more about the work of our research integrity team to safeguard the quality of each article we publish.