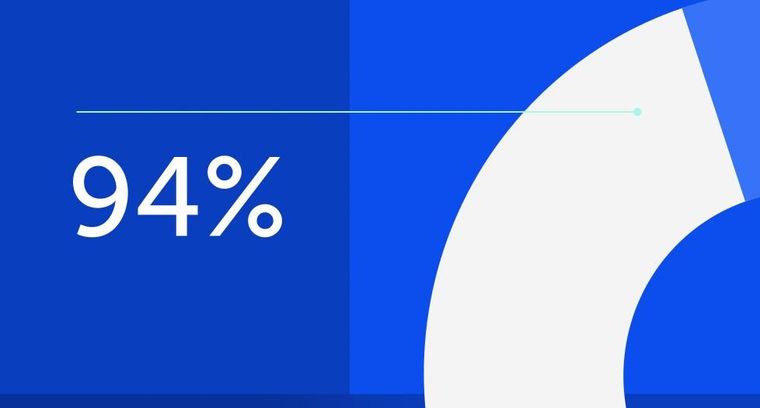
94% of researchers rate our articles as excellent or good
Learn more about the work of our research integrity team to safeguard the quality of each article we publish.
Find out more
ORIGINAL RESEARCH article
Front. Sustain. Food Syst., 24 November 2022
Sec. Climate-Smart Food Systems
Volume 6 - 2022 | https://doi.org/10.3389/fsufs.2022.961034
This article is part of the Research TopicFood-Energy-Water Systems: Achieving Climate Resilience and Sustainable Development in the 21st CenturyView all 21 articles
Introduction: Bangladesh's quest to achieve sustainable development goals has highlighted the need to enhance resilience against the challenges that interconnected food, energy, and water (FEW) nexus systems faces to support human well-being. The government has decided to promote the adoption of competent and cutting-edge technologies that can significantly contribute to balancing energy and water resource utilization in achieving a more sustainable and climate-smart food production system. Hence, scaled-up adoption of solar-powered irrigation systems and recommended fertilizer dose (SIRFD) applications were proposed. This study, to provide practical policy implications, attempts to identify the determinants and impact of SIRFD adoption in water-scarce areas of Bangladesh.
Methods: Determinants of adoption were analyzed using multinomial logistic regression, and the adoption impact was analyzed using treatment effect models.
Results: The results revealed that land typology, soil fertility perception, soil water retention, knowledge, environmental awareness, secondary income, close acquaintance adoption, and cash availability significantly influenced adoption decisions. The treatment effect model result indicated that farmers who adopted both technologies could reduce production costs by 1.36% and obtain an 8.92% higher ROI than non-adopters.
Conclusion: The study findings suggest that policy interventions on scaling up SIRFD adoption require focusing on knowledge development village-based demonstration activities, group farming models backed by micro-finance, and avoiding launching conflicting schemes.
Food, energy, and water (FEW) resource availability are intertwined and rely heavily on each other (Yadav et al., 2021). The food supply chain accounts for 30% of global energy consumption and 92% of the human water footprint. Forty-four percent of global water withdrawals are used for energy production and 70% for food production while 3% of global electricity is required for water provision with around 6% being used for food production (Larsen and Drews, 2019; Yadav et al., 2021). However, globally 828 million people suffer from hunger (WHO, 2022a), 733 million are deprived of access to energy (WHO, 2022b), and 785 million suffer from acute water scarcity (WHO, 2019). Due to combined factors that include global population growth, increased economic development, and rapid climate change, the demand for food, energy, and water resources is projected to increase by 70, 57, and 40%, respectively, over the next 20 years (Sadegh et al., 2020; Yadav et al., 2021). Hence, safeguarding the earth from degradation through sustainable consumption and production, sustainably managed natural resources, and urgent action on climate change at the national, regional, and global levels is warranted.
Attending the FEW nexus can help shed light on cross-sector interdependence with the aim to improve integrated solutions for achieving sustainable development goals. Suppose we aim to build resilience in the infrastructure of agriculture in developing nations, the first step is to establish what practices and input devices can be converted into critically sustainable componential practices. Such critically sustainable practices, in their part in building resilience, serve to guard against the existential threats to agro-development and the fight to achieve freedom from want or needs. However, what does the term “resilience” capture when taken into the world of threat mitigation, human development and agricultural sustainability? Most scholarly articles that articulate resilience in agriculture are limited to stability, robustness, and vulnerability of chemical compositions of inputs to farming (i.e., soil, microorganisms, or genetic makeup of crops). Ecosystem resilience is another prominent topic of academic insight, which investigates biodiversity and how humans can rejuvenate or regenerate such ecosystems against existential threats. However, a specific measure of agro-based resilience requires a viewpoint that encompasses all the aforementioned aspects, with the addition of stressing basic structures and functions for agriculture.
According to the United Nations 2015 Development agenda, disaster vulnerability is reduced as a direct product of well-grounded development (Piece, 2012). From the point of view of the paper, resilience can be defined as “the ability of a system, community or society exposed to hazards to resist, absorb, accommodate to and recover from the effects of a hazard in a timely and efficient manner, including through the preservation and restoration of its essential basic structures and functions” (Piece, 2012).
A great takeaway from this resilience perspective, wrapped within the framework of security aspects is that it allows new technologies in agriculture to have better directionality. Whatever is to be deemed sustainable must not only reduce costs but also preserve and restore what it is functioned to manipulate. This requires shifts in perception, cost and time consciousness, and systematic adaptations to changing environmental factors materializing in the present and possible future. Sustainable agricultural practices must, in the end, build community awareness and optimism toward standardizing efforts to increase productivity while ensuring that resources are plentiful in the future.
Sustainable agriculture is defined as adopting socially acceptable and financially viable farming practices to satisfy human food and fiber needs over the long term and remediate the environment that has been overused or misused by intensified agricultural practices while ensuring the efficient use of non-renewable and on-farm resources (Zilberman et al., 1997; Pretty, 2008; Faroque et al., 2011). This definition suitably begins the conversation of what it takes to ensure that if we farm, we farm to produce enough food, for a time that extends as long as we as humans wish to exist. It also insinuations the place a healthy physical environment takes in keeping humans alive and in balance with other parts of our fragile ecosystems. It does not however apportion specific responsibility over ensuring the ideals it aspires to produce, but it opens the floor for different approaches to exist. Different farming and managerial options that advocate sustainable agriculture practice in the context of FEW include the adoption of efficient water management systems, renewable irrigation technology, soil quality assessment technology, crop diversity practices, recommended doses of fertilizer application, disease-resistant and climate-adjusted varieties, integrated pest management (IPM) practices, agroforestry practices, planting cover crops, and resource conservative scale-appropriate agricultural machinery (Chartzoulakisa and Bertaki, 2015; Mottaleb, 2018; Sunny et al., 2018).
Agriculture is still regarded as the most crucial sector for developing countries like Bangladesh. Agriculture sector employs 38% of the national labor force (The World Bank, 2021) and directly supports 70% of Bangladeshis' livelihood (Imdad, 2021). Due to favorable agro-climatic environments for growing tropical and temperate crops, the government has adopted policies on utilizing available groundwater for irrigation as well as subsidized and intensified fertilizer policies to increase cropping intensity. For instance, the total amount of fertilizer subsidy expenditure has been amplified more than threefold, from BDT 35.34 billion in the fiscal year (FY) 2007-08 to BDT 133.32 billion in FY 2021-22 (FPMU, 2015; The Business Standard, 2022). Likewise, the irrigated area has increased approximately four times in the last three decades (Quddus and Kropp, 2020). These initiatives have escalated the cropping intensity from 183% to 200% (BBS, 2021; Ahmad, 2022) and made it possible for farmers to cultivate over two-thirds of the land twice or more annually (Dey et al., 2017). Rice (Oryza sativa) is the staple food that accounts for around 75% of the total harvested acreage and contributes approximately 95% of the total food grain (Shew et al., 2019; Alam et al., 2021). Studies have suggested that sustainable high yields with high-yielding crops depend entirely on the efficiency of water and fertilizers usage (Sagheb and Hobbi, 2002). Sound water-management practices and balanced fertilization potentially play a vital role in increasing cereal-based systems' productivity, nutrient and water use efficiency, reducing environmental burdens, and increasing economic advantage for farmers (IFC, 2014; Levidow et al., 2014; Ravisankar et al., 2014; Chen et al., 2022).
Over the years, agriculture and agronomic production systems have leaned toward sustainable agriculture to protect against existential challenges threatening humanity's future. However, a shift in perception of human security may need to be created, for instance, to emphasize “security against malnutrition” over general “food security” or seek securely “managed water resources” over the headline of “water security”. This is because the emphasis on general brand security can turn into a futile exercise where those most vocal and influential are positioned better to determine what is and is not a matter of security. In comparison, rebranding sustainable agriculture practices to emphasize matters of building resilient resource management systems may be a better congruent strategy. It is no argument that every nation, would like to realize a situation where agriculturalists can achieve greater yields, using fewer inputs while increasing the resource banks for future development of the sector. With this in mind, simple cost-saving strategies can now be seen as “forward investment liquidity” where long-term costs saved through the introduction of more efficient practices allow for more money left to be used on further developments with long-term advantages. Return on Investment (ROI) thus changes its flavor from a tool to infer the financial practicality of a chosen strategy into one whose favorable outcome justifies the doubling down on building resilient systems.
In the recent decade, like other developing countries, Bangladesh has also embraced the idea of sustainable agriculture practice alongside the overarching concept of sustainable development. Therefore, the government has urged promoting improved farm management technology adoption that advocates sustainable intensification while balancing energy and water resource utilization necessary for building a sustainable food production system. This initiative includes up-scaling the adoption of renewable energy-based irrigation and the recommended fertilizer dosage application. Because even though the groundwater availability for irrigation and subsidized fertilizer policies has helped Bangladesh attain near self-sufficiency in rice, it raises the demand for energy and the production cost (Islam et al., 2007; Naher et al., 2015; The Daily Star, 2016; Dey et al., 2017; Alam, 2018; Rahman and Zhang, 2018; Sunny et al., 2018; Das et al., 2020; FPMU, 2020; Kishore et al., 2021). Studies have revealed that compared to less water-stressed areas, the irrigation cost is 7.01% higher, the total return is 6.69% lower, and the fertilizer cost is 4.73% higher in severe water scare regions (Palash et al., 2019). There are two main reasons for this. Firstly, diesel or electricity-based irrigation systems are used in Bangladesh to extract groundwater. The diesel irrigation systems are more expensive in pumping output per liter, and electric-based irrigation systems, though cheaper, draw from an already scarce national electric grid supply. Therefore, the energy constraint in peak irrigation seasons in water-scarce areas exacerbates cost reduction and resource management challenges. More water is needed, and more energy is required to withdraw it. Secondly, subsidized fertilizer prices invigorated farmers' higher fertilizer application tendency (Uddin, 2021; World Bank, 2021), impacting their production costs. Finally, the long-term effects of the diesel irrigation system and unbalanced fertilizer applications negatively impact the environment (Islam et al., 2007; Naher et al., 2015; The Daily Star, 2016; Dey et al., 2017; Alam, 2018; Rahman and Zhang, 2018; Sunny et al., 2018; Das et al., 2020; FPMU, 2020; Kishore et al., 2021).
Solar technology has gained momentum worldwide as a part of strategies to promote climate-friendly, renewable energy-driven implements in production. Studies have suggested that solar energy can either replace or cut down on the farmers' dependency on costly and inaccessible energy sources to power their water distribution facilities. The country can reduce approximately 17,261 tons of carbon dioxide emissions by adopting solar irrigation technology. Farmers can meet irrigation water needs adequately and more efficiently while increasing both the quality and the quantity of the crops and reducing water wastage (Mirta et al., 2011; Hossain and Karim, 2020; Sunny et al., 2022). Due to technological advancement, solar-based technologies are becoming a cheaper alternative to fossil fuels. With the steady increases in diesel prices worldwide, small-scale farmers are increasingly unable to afford the yearly expense of diesel-run water pumps for their irrigation systems. Moreover, there is an expanded burden on the national budget and foreign currency reserves (Sunny et al., 2022). Furthermore, national grid power may seem like a better alternative to diesel fuel, but most rural farmers do not have access to electric grids (Sunny et al., 2022). On the other hand, fertilizer recommendations as a technology combine scientific knowledge and service that shapes the application; skills and techniques are employed toward improving yield and output quality. Thus, although fertilizer recommendations are born out of technological processes that give an output of workable interpretations, the raw information is subsequently organized into unique sets of directions that act as tools rather than general information. These tools guide farmers to apply fertilizer most effectively, in the same manner, that different farm implements can be helpful to a farmer. It is revealed that balanced fertilizer management promotes plant growth, enhances product quality, minimizes inputs, and reduces negative environmental impacts (Chen et al., 2022).
Hence, different programs were launched to popularize the application of balanced fertilizer doses to decrease farmers' production costs and negative environmental impacts (CRI, 2019; Imdad, 2021; Kishore et al., 2021; Shawon and Sourav, 2021). Likewise, initiatives to establish 50,000 solar irrigation pumps by 2025 have been taken to minimize the energy crisis (replacing 10% of conventional energy with renewable energy) and reduce the depletion of fossil fuel reserves while ensuring sustainable water management in the agriculture sectors (Kanojia, 2019; Sajid, 2019; Rana M.d.J. et al., 2021).
However, despite the significant potential of these two technologies, the adoption of solar-powered irrigation systems and recommended fertilizer dose (SIRFD) is slow to develop in Bangladesh (SREDA, 2015; The Daily Star, 2016; Rahman and Zhang, 2018; Sunny et al., 2018; Rana M.d.J. et al., 2021), none of the studies have investigated both technologies' adoption determinants and farm-level impacts simultaneously (Hasanuzzaman et al., 2009; Alam et al., 2011; Choudhury et al., 2013; Hasnat MdA, 2014; Hossain et al., 2015; Sarker and Ghosh, 2017; Mamun et al., 2018; Hossain and Karim, 2020; Rana M.d.J. et al., 2021; Rana J. et al., 2021; Sunny et al., 2022). Therefore, to minimize the research gap, this study first attempts to identify the factors that have influenced farmers' to adopt SIRFD and assesses the impact of the adoption on return on investment (ROI) and production costs by employing treatment effect models to address the selection bias issue (Barreto and Bell, 1994; Coady, 1995; Duflo et al., 2011; Dong et al., 2012; Fanus et al., 2012; Martey et al., 2013; Zhou, 2017; Kumar et al., 2019, 2020; Sanap et al., 2020). The other contribution of this research in literature includes the selection of the empirical approach as we choose treatment effect models to address the selection bias issue. It is expected that the resource-stressed regions should take the study findings as a rationale for policy development to achieve sustainable and environmentally sound food production practices.
To fulfill the research objective we used following steps (Figure 1).
This study spotlights the drought-prone area of the northern region of Bangladesh due to the lower annual rainfall (21.83% lower than the country's average annual rainfall) condition. Lower annual rainfall in the northern part caused surface water scarcity and created a high dependency on groundwater for cultivation and irrigation in these areas (Hossain et al., 2021; Rahman et al., 2022). According to the estimates, among 1.6 million diesel pumps (Prothom Alo, 2021) and 3.20 lakh electricity pumps (Ershadullah, 2021) operating in the country, a significant proportion operates in the northern regions (Hossain et al., 2021).
We used multistage sampling techniques to conduct this research. Therefore, in the first stage, we selected the Dinajpur district as its specific endowments fit our study parameters. Dinajpur is the largest among all 16 districts situated in the northern part, and the district has a tropical wet-dry climate based on the Köppen climate classification. The annual average temperature is 25°C. The average precipitation from November to March is below 20 mm, April and October are below 100 mm, and the remaining 5 months are over 200 mm (Wikipedia, 2022). Due to the low precipitation rate, the district is considered one of the top drought-prone areas in Bangladesh (Afrin et al., 2019; Islam et al., 2022; Rahman et al., 2022). As a result, food insecurity and poverty rates are high (BBS, WFP, 2020). This district is also one of the top districts where more solar irrigation pumps are installed (SREDA, 2022). Besides, information differences on farmers' fertilizer application procedures are found in the existing literature (Jahiruddin et al., 2010; Kobir, 2019; Siddique et al., 2020).
In the second stage, a simple random sampling method was used to select three sub-districts from 13 of the Dinajpur district. The randomly chosen three sub-districts were Birganj, Khanshama, and Kaharol. The combined population of these three sub-districts is 643431 (BBS, 2015). We then used Krejcie and Morgan's (1970) table to determine the optimal sample size. A sample of 384 farmers was determined based on our population size. However, to minimize unexpected errors that may arise from respondents' non-or partial responses, we felt the need to collect an additional 5% of samples. Hence, to sample an equal number of respondents, we finally collected 405 samples, comprising 135 randomly chosen respondent farmers from each sub-district.
Face-to-face semi-structured questionnaire-based interviews were conducted between February and April 2021 to collect data. The Boro season (December to June) was chosen since the maximum rice is produced in this season (BBS, 2020), and irrigation demand is very high (Hossain and Siddique, 2015). The interview schedule was translated into the local language for implementation and pretested before finalization. The interviewed respondents were rice-producing farmers. We also obtained participants' consent before conducting the interview, and all expressed their desire to participate. Our interview schedule included farmers' demographic and socioeconomic characteristics, knowledge level, fee opinion, environmental consciousness, and agroecology-related questions.
The random utility theory (McFadden, 1974) is adopted for this study, which speculates that individuals derive utility from the features of goods or services. This approach combines the deterministic and statistical models of human behavior. It allows eliciting preferences for complex multidimensional goods or services, from which models of (relative) preferences for different attributes of a good or service can be determined (Lancaster, 1966; Manski, 1977; Groothuis-Oudshoorn et al., 2014).
The assumption is that individuals' prefer alternatives when the maximum utility gained from adoption is higher than non-adoption (Hess et al., 2018). Following this theory, the utility that the individual i may derive from adopting an alternative a can be expressed as:
Where, Uia is the net gain from adoption, βi is the function of observable attributes Xia and εia is the error term.
Multinomial logistic regression is considered for testing our propositions because our dependent variable is classified into three categories rather than two and is not continuous. Likewise, this approach requires fewer assumptions than discriminant analysis, and collinearity is assumed to be relatively low. Thus, this approach is reckoned to be more robust in the face of data conditions that might adversely impact discriminant analysis (Hong and Zhu, 2006; El-Habil, 2012). The multinomial logistic regression for this study can be expressed as:
In this equation, p denotes farmers' adoption probability of certain types of technology.
Since the estimated coefficients obtained from multinomial logistic regression are on a log-odds scale, we have also computed the relative risk ratio (RRR) to represent the predicted multiplicative change in the relative risk (that is, the risk of falling into a comparison group relative to the risk of falling into the baseline group) per unit increase in an independent variable (Osborne, 2014; UCLA, 2021).
Prior studies (Asfaw et al., 2011, 2012) have suggested that in the absence of prior intervention data, the best way to assess the impact of technology adoption is to use treatment effect models or an instrumental variable-based regression approach. However, due to difficulties in finding promising valid instrumental variables, this study employed treatment effect models because inappropriate instrumental variables may raise regression analysis pitfalls such as bias or the omitted variable issue (Angrist and Krueger, 2001).
The present study used two different treatment effect models because the adoption decision for this study varies at different levels (0, 1, and 2). Therefore, simple dichotomous treatment status (0,1) may not correctly capture the non-linearity and differential effects across treatment levels (Cattaneo, 2010; Wooldridge, 2010). To examine the impact of technology adoption, we estimate the average treatment effect on the treated (ATT). The ATT estimates the predicted causal effect of the treatment for individuals in the treatment group (Sunny et al., 2022).
The treatment effect models used for this study are regression adjustment (RA), and inverse probability weighted regression adjustment (IPWRA). The RA model, which allows the binary extension to multivalued models, uses the contrasts of the averages of treatment-specific predicted outcomes (Wooldridge, 2010; Stata, 2013). This approach allows estimating the possible outcome of adoption without prior assumptions about the treatment model (Smale et al., 2018). The RA model is a two-step approach (Stata, 2013). Firstly, a separate outcome model is estimated for each treatment level, and secondly, the model uses differences in the potential outcomes (POMs) to evaluate the average treatment effect on the treated (ATT) (Kazal et al., 2020). The ATT for the RA estimator specification can be expressed as (Wooldridge, 2010; Zheng and Ma, 2021):
Where, na is the adopters' number; Ti is the adoption status for farmer i; ra(.) and rna(.) is the projected regression model for the adopters and non-adopters based on observed covariates X and parameters δk = (αk, βk), (k = a, na).
On the other hand, the IPWRA model—known as a double-robust estimator, uses probability weights to deal with the missing-data problem and obtain outcome regression parameters. The adjusted outcome-regression parameters are employed to estimate averages of treatment-level predicted outcomes (Sunny et al., 2022). The contrasts between these averages provide assessments of the treatment effects (Wooldridge, 2010; Stata, 2013). The IPWRA model, also through two steps (the propensity score matching and regression analysis), estimates the average treatment effect on the treated (ATT) (Imbens and Wooldridge, 2009; Sunny et al., 2022). The ATT for the IPWRA estimator can be expressed as (Zheng and Ma, 2021; Sunny et al., 2022):
Where and are the inverse probability-weighted estimator parameters for adopters and non-adopters, respectively, and through the weighted regression methods can be obtained:
The outcome variables for this study are production costs and ROI. The production costs include expenditures on seeds, fertilizer, pesticides, irrigation, and other inputs, which are also measured at Taka/decimal. The ROI is the ratio of net earnings to production costs, defined in accordance with previous studies (Kleemann et al., 2014; Zheng and Ma, 2021).
The dependent variable is a dummy variable equivalent to ‘0' if the farmer did not adopt solar irrigation and fertilizer recommended facilities, ‘1' if a farmer has partially adopted solar irrigation and fertilizer recommended facilities, and ‘2' if the farmer has adopted both solar irrigation and fertilizer recommended facilities.
The explanatory variables for this particular study presented in Table 1 below are chosen from the existing literature on the adoption. The variables include farmers age (Tiwari et al., 2008; Ntshangase et al., 2018), education (Chuchird et al., 2017; Sunny et al., 2018), land typology (Endrias et al., 2013; Reza and Hossain, 2013), farming experience (Sunny et al., 2018; Sarker et al., 2021), household size (Challa and Tilahun, 2014; Araya and Holden, 2018), farm size (Deressa et al., 2011; Ntshangase et al., 2018), knowledge acquisition (Feder and Slade, 1984; Bairagi et al., 2018), off-farm or secondary income (Pandey and Mishra, 2004; Mottaleb et al., 2016), plot fertility (Kassie et al., 2015; Zeng et al., 2018), credit availability (Simtowe and Zeller, 2006; Mottaleb et al., 2016), soil water retention (Albrecht and Ladewig, 1985; Genius et al., 2013), close acquaintances' adoption (Mendola, 2007; Jansson et al., 2017), and environmental awareness (Liu et al., 2013; Irfan et al., 2020).
Table 2 below presents the differences in the characteristics of adopters and non-adopters. Among 405 respondents, 9.63% of the farmers were non-adopters, 52.35% of farmers were partial adopters, and the rest 38.02% have adopted both technologies. The χ2 and F-test result indicates significant differences between these groups based on their farmlands typology, soil water retention condition, knowledge level, and close acquaintances' adoption status. The result shows that among total non-adopters, 58.9% cultivate in mid-high lands, whereas most partial adapters (81.6%) and both adapters (87%) cultivate in mid-low land. The water holding capacity of non-adopters farmland compared to partial and both technology adopters' farmland were also found lower. Close acquaintances of 38.5% of non-adopters, 48.1% of partial, and 66.9% of both-technology adopters have also adopted these technologies. Among the total respondents, only 7.7% of the non-adopters, 12.7% of partial adopters, and 27.9% of adopters possess the proper knowledge of both technologies.
The factors influencing farm households' adoption of solar irrigation facilities were analyzed using multinomial logistic regression, and the results are presented below (Table 3). Since the coefficient result only expresses the direction of change, we also compute the relative risk ratio (RRR) that represents the predicted multiplicative change in the relative risk (that is, the risk of falling into a comparison group relative to the risk of falling into the baseline group) per unit increase in an independent variable (Osborne, 2014; UCLA, 2021). Based on the LR test, our model containing the full set of predictors represents a significant improvement in fit relative to a null model [LR χ2 (IFC, 2014) = 117.23, p < 0.0000], which infers that at least one population slope is non-zero. The calculated variance inflation factor (VIF) value for all the variables is well below the conventional threshold of 10, indicating no severe collinearity (Maddala, 1983).
The negative and significant (p < 0.001) “Land Typology” predictor in Table 3 indicates that farmers cultivating in mid-high land are less likely to fall into partial and both adopters” groups and more likely to be non-adopters compared to farmers cultivating in low-mid land. The RRR value indicates that for each unit increase of this variable, the farmers” risk of falling into the “partial adopters” and “both adopters” categories relative to the risk of belonging to the “non-adopter” category is predicted to change by a factor of 0.16 and 0.12, respectively. This result is meaningful because the Boro rice farming methods necessitate flooded fields (Pearson et al., 2018). The leakage issues on comparatively high land create difficulties in holding ponded water, causing higher wastage of fertilizer and increasing fertilizer use.
Similarly, the “soil fertility perception” predictor is negative and significant (p < 0.001). The relative risk ratio indicates that farmers with the greater belief that their farmland soil is fertile are at a 0.05 and 0.06 times lower risk of falling into the “partial adopters” and “both adopters” categories and at an increased risk of being in the “non-adopters” category. Prior Studies have similarly found that in developing nations, soil fertility perceptions are not fundamentally based upon scientific classifications of soil composition or other lesser visible metrics (e.g., soil nutrient composition) (Desbiez et al., 2004). Being largely informally educated, untrained, and small landowners, a large number of Bangladesh rice farmers' understanding of soil fertility is based on perceived yield increases attributed to increases in fertilizer use. Hence, aspiration to gain higher yields (Rahman and Zhang, 2018), lack of fertilizer application knowledge of hybrid varieties (Huang et al., 2017), and unawareness of the combined impact of adopting improved farming technologies for higher grain yield (Sarker et al., 2014) are responsible for their excessive fertilizer application.
Unlike the other two comparisons, the “Soil Water Retention” predictor was positive and statistically significant (p < 0.001). The result indicates the likelihood of being partial and both adapter groups for farmers is higher if their farmland soil has a higher capacity to hold water. The RRR for “Soil Water Retention” indicates that for each unit increase on this variable, the risk of falling into the “partial adopters” and “both adopters” categories relative to the risk of belonging to the “non-adopter” category is predicted to change by a factor of 16.61 and 24.45 respectively. This result indicates the association between water-holding capacity and soil health. Prior studies have suggested that soil retaining a balanced amount of water can support crop growth and keep soil organic matter alive. Therefore, the nourishment of soils with low water-holding capacity requires more organic and chemical fertilizer usage (Dong et al., 2012). As a result, the likelihood of being in the non-adopters group for farmers cultivating in low water retention land is higher.
The “Knowledge of SIRFD” predictor is positive and significant (p < 0.001). The positive slope suggests that farmers who know about both facilities have a greater possibility of adopting both and a lower probability of being either non-adopters or partial adopters list. The RRR indicates that for each one-unit increase in knowledge level, the relative risk of being in the “both adopters” category (relative to the risk of belonging to the “non and partial” adopters” category) changes by a factor of 9.10. As expected, the result matches prior studies that revealed that better knowledge of technologies positively influences adoption (Kabunga et al., 2012; Bairagi et al., 2018).
The “Environmental Awareness” predictor is negative and significant (p < 0.05), suggesting farmers who know adopting both facilities is beneficial for the environment have less probability of being on both adopters lists and more on either non-adopter or partial adopters lists. The RRR for “Environmental Awareness” indicates that for each one-unit increase in farmers” awareness level, the relative risk of falling into the “both adopters” category compared with the “not-adopters” category is multiplied by a factor of 0.44. This result contradicts our expectations and draws attention to farmers' social and psychological risk factors. It is essential to understand that most farmers in our study area are subsistence farmers. Their technology acceptance decisions are primarily associated with their profit gain aspects and are influenced by the attitude of neighbors, fertilizer dealers, and friends in their immediate environment (Mottaleb et al., 2019).
The RRR for “Secondary Income” indicates that for each unit increase in off-farm income level, the relative risk of being in the “both adopter” category (relative to the risk of belonging to the “non or partial adopter” category) changes by a factor of 2.63. This result matches a prior study that indicates that adopting new technology requires additional costs (Rahman et al., 2021).
The RRR for “Close Acquaintances adoption” indicates that for each unit increase in close acquaintances adoption level, the relative risk of being in the “partial adopters” and “both adopters” category (relative to the risk of belonging to the “non-adopter” category) changes by a factor of 2.04 and 4.67, respectively. Our findings match other studies (Mendola, 2007; Krishnan and Patnam, 2014) findings, suggesting that close connections influence farmers” new technology acceptance behavior.
Finally, the negative and significant (p < 0.05) “Cash Availability” variable suggest that farmers with available cash during the cropping season have less probability of being on a partial and both adopter list and more on a non-adopter list. The RRR indicates that for each unit increase in farmers” credit availability level, the relative risk of being in the “partial adopters” and the “both adopters” category (relative to the risk of belonging to the “non-adopter” category) changes by a factor of 0.25 and 0.26, respectively. This finding is meaningful because previous studies have revealed that greater access to credit influences fertilizer intensification (Ouattara et al., 2020).
Before finalizing the models, we checked the balancing summary estimates and overlap assumptions. The balancing summary estimates are outlined below (Table 4), which reports the model-adjusted difference in means and ratio of variances between the treated and untreated for each covariate. The result confirms that the balancing property is satisfied as the thresholds for the variance ratio for balanced groups are 0.5 and 2 (Rubin, 2001; Stuart, 2010).
Further, in testing the overlap assumption, we used overlap plots. The graphical diagnostic result in Figure 2 indicates that all the estimated densities had most of their respective masses in regions in which they overlap.
The adoption impact results in Table 5 show that the adoption of both technologies has a significant impact on production cost and ROI. Specifically, farmers who adopted both technologies could reduce production costs by 1.36% and obtain an 8.92% higher ROI than non-adopters.
The result also indicates an association between these technology adaptations and the establishment of a more sustainable food production system that enhances the energy and water sector resiliency from natural and man-made disasters and climate change. The adoption of recommended fertilizer doses helped improve soil health and fertilizer-use efficiency. Due to increased fertilizer-use efficiency, the soil's physical, chemical, and biological constitutions have been enhanced, playing a vital role in improving crop yield. Moreover, solar irrigation adoption helped farmers reduce input costs by significantly reducing electricity usage and fuel costs (diesel and coal) while maintaining diesel or electricity pumps for emergency use only. Likewise, due to longer service life, lower CO2 emissions, and reduced maintenance costs and challenges, these adoptions have added to air and soil environmental protection efforts. In addition, solar-powered irrigation adoption has provided higher groundwater access for irrigation, which is vital for farmers to grow diverse crops, achieving higher crop productivity, improving income, enhancing their coping capacity against climate change risk, and making them more resilient.
Our findings match prior studies documenting the positive impact of these technologies' adoption on yield, input costs, water usage, and energy-saving aspects (Datta et al., 2015; Ramappa et al., 2015; Hossain and Karim, 2020; Sunny et al., 2022). Therefore, sustainable agricultural technologies, such as SIRFD, have the potential to enable a more sustainable supply of food, energy, and water, particularly in water-stressed areas. These outcomes, however, will not be sustained until the barriers to the continuous adoption of these technologies are eliminated or at least reduced.
However, judging how these technology adaptations lead to overall resiliency in the sector against any and most instances of force majeure is problematic. On the one hand, the introduction, development, and propagation of solar technology solely relate to direct cost-benefits of water extraction where it is needed and does not directly indicate how they aid in water saving in the moment or between difficult periods of water shortages. Likewise, the adoption of recommended dosage fertilizer application is also related to site specification and cannot be generalized. On the other hand, introducing these technologies for low-income or developing economies—local and national, may present significant barriers to entry even if the benefits significantly outweigh the costs. However, when combined with increased agency by the farmers toward sustainability and the intention to build existential resilience in farming, those potential cost savings should lead one to consider that savings will allow for the liquidity to develop further efficiencies in water storage systems and more effective technologies. Since adoption is affected by cost-saving strategies that result in greater yield, it is plausible to assert that attitude changes to sustainable practices in rural areas should shift positively. Attitudes shifts to the aforementioned researched practices would not be enough, however. In the long term, trust in objective research, tolerance to the evolution of new and more refined information, and belief in seeking out science ahead of personal contact information will go much further in allowing for resilience in agriculture to take root and adapt to fresh conditions.
Recent decades have seen the popularity of drip irrigation and hydroponic-based farming practices. These technologies can be expensive to set up, especially if the resources needed to be accumulated to develop them are not readily and inexpensively located. To have created some financial freedom to explore more resilient farming practices comes directly from the marriage of cost-saving practices and a motivated intent to plan for between-disaster periods as well as current existential issues. The benefits of precise water and fertilizer management systems cannot be overstated. As time goes on, the soil does not need as much to preserve its richness; water is both more available and in a condition conducive to the needs it is intended for; and farmers are more prepared to see the advantage in thinking more creatively toward resilient sustainability in farming. As a result, policymakers, who are pushed to act by the voices of their constituents, are more likely to develop more viable and contextually driven policies which also encourage the better adoption of improved agro-tech. Again, all this is based upon empowering farmers into positions of greater agency over their contributions to sustainability through nurturing in them a greater motivation to seek, explore, make use of and improve upon resilience-based measures they take to viably improve their production efforts.
As a newly graduated lower-middle-income country, Bangladesh, making progress toward achieving SDGs, has highlighted the need to enhance resilience against the challenges that interconnected food, energy, and water (FEW) nexus systems faces to support its citizen. Hence the government has decided to promote SIRFD technologies to balance energy and water resource utilization to achieve a more sustainable and climate-smart food production system. This study has attempted to reveal critical factors influencing Dinajpur region rice-growing farmers' adoption or non-adoption decisions SIRFD and the adoption impacts on the farm level. The results showed that land typology, soil fertility perception, soil water retention, knowledge of both technology, environment awareness, close acquaintance adoption, and cash availability influence farmers' adoption decisions. The results of the ATT estimates exhibited a positive impact of both technology adoption on production costs and ROI.
The findings of this study have practical policy implications. Firstly, the positive association of SIRFD adoption on production costs and ROI emphasizes the importance of scaling up the adoption process. Hence, policymakers should concentrate on designing more appropriate schemes that are flexible enough to respond to new knowledge gained over time and attractive enough to generate strong demand from farmers. These initiatives include village-level demonstration programs that emphasize the positive impact of combined technology adoption and eco-environmental wellbeing.
Secondly, policymakers must consider heterogeneous group research to provide different farmers' groups with practical support and develop policies that make adopting these two farming technologies more attractive. Likewise, a policy should be initiated for a specific period, and within that time frame, the government should not launch any other policy that conflicts with the existing policy. For instance, region-specific solar irrigation projects should emphasize the installation of solar pumps with a specific capacity so that each site has similar area coverage and water delivery capacity to avoid internal conflicts between service providers. At the same time, introducing an insurance scheme to hedge against the potential production risk may also enhance the adoption process.
Thirdly, knowledge publicity initiatives that aim at providing information on abiotic and biotic factors that affect yield and crop responsiveness, training on water-efficient irrigation methods, and correcting farmers' incorrect fertilizer application timing and quantity are expected to enhance the adoption process. Since most rural families usually involve the other members in the decision process, and if most family members have a knowledge deficiency about the benefits of both technologies, the family may not decide to accept both technologies. Besides, facilitating training on fertilizer application of hybrid varieties that requires less external N inputs under moderate to high soil fertility conditions and the combined impact of adopting improved farming technologies for higher grain yield is expected to increase the adoption rate.
Fourthly, the study indicated that there is room for expanding the role of positively impactful technologies on expanding production and increasing both yield production and positive return on investment. This further implies that SIRFD adoption can significantly increase resilience in farm practices against existentially threatening situations, such as natural and man-made disasters, through the capacity-building mechanisms inherent in positively regarded systems. An ecosystem of farmers who are mindful of the management of shared resources will not follow far behind environmentally friendly and normalized practices. Furthermore, it is not farfetched to observe that as farmers' yields increase (based on SIRFD -like technological progressions), policymakers will have little doubt in pushing hard to adopt policies with the general public and practitioners in agriculture.
Finally, public-private initiatives to introduce the group-farming model backed by microfinance organizations can play a vital role scale up the adoption process. Because group farming model formation will help small farmers pool their resources to create a larger enterprise voluntarily through sharing costs and benefits without forfeiting rights to their owned land.
Despite the valuable data gained, the limitations of this study include a relatively small sample size and less area coverage. A large-scale survey could provide more information. The impact of adoption was measured using one-year cross-section data, and future studies may consider using multiyear panel data for better understanding.
The original contributions presented in the study are included in the article/supplementary material, further inquiries can be directed to the corresponding author.
FAS and MSR planned and designed the research. FAS analyzed and interpreted the data. FAS and TTPK wrote the first draft. TTPK, WP, and HZ critically reviewed the manuscript that went through multiple revisions by FAS, TTPK, and MR. All authors read and approved the final manuscript.
The Zhejiang Philosophy and Social Science Planning Project (Grant No. 19NDJC109YB) and the Zhejiang Provincial Basic Public Welfare Research Project (Grant No. LY20G030005) funded this research.
The authors are grateful to the farmers of the study area, Md. Mamun Hossain from Dinajpur and Nahid Ferdous Dristy of the Housing and Building Research Institute (HBRI) for their time and assistance.
The authors declare that the research was conducted in the absence of any commercial or financial relationships that could be construed as a potential conflict of interest.
All claims expressed in this article are solely those of the authors and do not necessarily represent those of their affiliated organizations, or those of the publisher, the editors and the reviewers. Any product that may be evaluated in this article, or claim that may be made by its manufacturer, is not guaranteed or endorsed by the publisher.
Afrin, R., Hossain, F., and Mamun, S. A. (2019). Analysis of drought in the northern region of bangladesh using standardized precipitation index (SPI). Environ. Sci. Nat Resour. (2019) 11, 199–216. doi: 10.3329/jesnr.v11i1-2.43387
Alam, M. A. U. (2018). The effect of the ‘subsidy on fertilizer' on food prices in bangladesh and policy options. J. Econ. Sustain. Dev. 9, 200–208.
Alam, M. J., Huylenbroeck, G. V., Buysse, J., Begum, I. A., and Rahman, S. (2011). Technical efficiency changes at the farm-level: a panel data analysis of rice farms in Bangladesh. Afr J Bus Manag. 5, 5559–66. doi: 10.5897/AJBM11.329
Alam, M. J., Mahmud, A. A., Islam, Md,.A., Hossain, M.d.F., Ali, Md,.A., Dessoky, E. S., et al. (2021). Crop diversification in rice—based cropping systems improves the system productivity, profitability and sustainability. Sustainability. 13, 6288. doi: 10.3390/su13116288
Albrecht, D. E., and Ladewig, H. (1985). Adoption of irrigation technology: the effects of personal, structural, and environmental variables. J Rural Soc Sci. 3, 26–41.
Angrist, J. D., and Krueger, A. B. (2001). Instrumental variables and the search for identification: from supply and demand to natural experiments. J. Econ. Perspect. 15, 69–85. doi: 10.1257/jep.15.4.69
Araya, G. B., and Holden, S. T. (2018). The Impact of Ethiopia's Productive Safety Net Program on Fertilizer Adoption by Small Holder Farmers in Tigray, Northern Ethiopia. In: Vancouver, British Columbia: International Association of Agricultural Economists (IAAE). (2018) p. 29. Available online at: https://ageconsearch.umn.edu/record/277051
Asfaw, S., Shiferaw, B., Simtowe, F., and Haile, M. (2011). Agricultural technology adoption, seed access constraints and commercialization in Ethiopia. J Dev Agric Econ. 3, 436–77.
Asfaw, S., Shiferaw, B., Simtowe, F., and Lipper, L. (2012). Impact of modern agricultural technologies on smallholder welfare: evidence from Tanzania and Ethiopia. Food Policy. 37, 283–95. doi: 10.1016/j.foodpol.2012.02.013
Bairagi, S., Bhandari, H., Das, S. B., and Mohanty, S. (2018). Impact of Submergence-Tolerant Rice Varieties on Smallholders Income and Expenditure: Farm-Level Evidence from Bangladesh. Selected Paper prepared for presentation in Agricultural and Applied Economics Association Annual Meeting 2018. Washington, D.C.: Selected Paper prepared for presentation presented at 2018. Agricultural and Applied Economics Association Annual Meeting.
Barreto, H. J., and Bell, M. A. (1994). Assessing risk associated with N fertilizer recommendations in the absence of soil tests. Fertil Res. 40, 175–83. doi: 10.1007/BF00750463
BBS (2015). Population and Housing Census 2011, Zila Report: Dinajpur. Dhaka, Bangladesh: Bangladesh Bureau of Statistics (BBS) p. 558. Available From: http://203.112.218.65:8008/WebTestApplication/Userfiles/Image/PopCenZilz2011/Zila_Dinajpur.pdf (accessed Oct 10, 2020).
BBS (2020). Estimate of Major Crops (2019-2020). Available online at: http://www.bbs.gov.bd/Site/Page/453af260-6aea-4331-B4a5-7b66fe63ba61/ Agriculture (accessed Aug 12, 2021).
BBS (2021). Statistical Yearbook Bangladesh 2021. 41st ed. Dhaka, Bangladesh: Bangladesh Bureau of Statistics (BBS) 584 p. Available online at: http://bbs.Portal.gov.bd/Sites/Default/Files/Files/bbs.Portal.gov.bd/Page/B2db87 58_8497_412c_A9ec_6bb299f8b3ab/2022-06-15-10-49-3cf641425dd693f9e954 de5ae9470775.pdf(accessed July 4, 2022).
BBS WFP. (2020). Poverty Maps of Bangladesh 2016. Dhaka, Bangladesh: Bangladesh Bureau of Statistics (BBS) and World Food Program (WFP) p. 57. Available online at: http://bbs.Portal.gov.bd/Sites/Default/Files/Files/bbs.Portal.gov.bd/Page/56 95ab85_1403_483a_Afb4_26dfd767df18/2021-02-22-16-57-C64fb3d272175e7efe a0b02de6a23eaa.pdf (accessed Jun 10, 2022)
Cattaneo, M. D. (2010). Efficient semiparametric estimation of multi-valued treatment effects under ignorability. J. Econom. 155, 138–54. doi: 10.1016/j.jeconom.2009.09.023
Challa, M., and Tilahun, U. (2014). Determinants and impacts of modern agricultural technology adoption in West Wollega: the case of Gulliso District. J. Biol. Agric. Healthc. 4, 63–77.
Chartzoulakisa, K., and Bertaki, M. (2015). Sustainable water management in agriculture under climate change. Agric. Agric. Sci. Procedia. 4, 88–98. doi: 10.1016/j.aaspro.2015.03.011
Chen, X., Chen, X., Jiao, J., Zhang, F., Chen, X., Li, G., et al. (2022). Towards Balanced Fertilizer Management in South China: Enhancing Wax Gourd (Benincasa hispida) Yield and Produce Quality. Sustainability. 14, 5646. doi: 10.3390/su14095646
Choudhury, A. T. M. A., Saleque, M. A., Zaman, S. K., Bhuiyan, N. I., Shah, A. L., Rahman, M. S., et al. (2013). Nitrogen fertilizer management strategies for rice production in Bangladesh. Pak. J. Sci. Ind. Res. 56, 167–74. doi: 10.52763/PJSIR.BIOL.SCI.56.3.2013.167.174
Chuchird, R., Sasaki, N., and Abe, I. (2017). Influencing Factors of the Adoption of Agricultural Irrigation Technologies and the Economic Returns: A Case Study in Chaiyaphum Province Thailand. Sustain MDPI Open Access J. (2017) 9, 9. doi: 10.3390/su9091524
Coady, D. P. (1995). An empirical analysis of fertilizer use in Pakistan. Economica. (1995). 62, 213–34. doi: 10.2307/2554904
CRI (2019). Bangladesh: towards achieving food security 2009-2019. Dhaka, Bangladesh: Centre for Research and Information (CRI). Available online at: https://cri.org.bd/Publications/
Das, N. K., Chakrabartty, J., Dey, M., Gupta, A. K. S., and Matin, M. (2020). A present energy scenario and future energy mix of Bangladesh. Energy Strategy Rev. 32, 100576. doi: 10.1016/j.esr.2020.100576
Datta, V., Mondal, D. K., Ghosh, S., Mukherjee, R., Chattopadhyay, K. S., Chakrabarti, S., et al. (2015). Adoption of Recommended Doses of Fertilizers on Soil Test Basis by Farmers. New Delhi: Ministry of Agriculture, Government of India. p. 126. Available online at: https://www.visvabharati.ac.in/file/Final-Report-179.pdf
Deressa, T. T., Hassan, R. M., and Ringler, C. (2011). Perception of and adaptation to climate change by farmers in the Nile basin of Ethiopia. J. Agric. Sci. 149, 23–31. doi: 10.1017/S0021859610000687
Desbiez, A., Matthews, R., Tripathi, B., and Ellis-Jones, J. (2004). Perceptions and assessment of soil fertility by farmers in the mid-hills of Nepal. Agric Ecosyst Environ. 103, 191–206. doi: 10.1016/j.agee.2003.10.003
Dey, N. C., Saha, R., Parvez, M., Bala, S. K., Islam, A. K. M. S., Paul, J. K., et al. (2017). Sustainability of groundwater use for irrigation of dry-season crops in northwest Bangladesh. Groundw Sustain Dev. 4, 66–77. doi: 10.1016/j.gsd.2017.02.001
Dong, W., Zhang, X., Wang, H., Dai, X., Sun, X., Qiu, W., et al. (2012). Effect of different fertilizer application on the soil fertility of paddy soils in red soil region of southern China. PLoS ONE. 7, e44504. doi: 10.1371/journal.pone.0044504
Duflo, E., Kremer, M., and Robinson, J. (2011). Nudging farmers to use fertilizer: theory and experimental evidence from Kenya. Am. Econ. Rev. 101, 2350–90. doi: 10.1257/aer.101.6.2350
El-Habil, A. M. (2012). An application on multinomial logistic regression model. Pak J Stat Oper Res. 8, 271–91. doi: 10.18187/pjsor.v8i2.234
Endrias, G., Ayalneh, B., Belay, K., and Elias, E. (2013). Determinants of farmers' decision on soil fertility management options for maize production in Southern Ethiopia. Am J Exp Agric. 3, 226–39. doi: 10.9734/AJEA/2013/2299
Ershadullah, M. (2021). Solar irrigation pumps: Transforming to smart irrigation and improving agriculture in Bangladesh. (2021). Available online at: https://smartwatermagazine.com/blogs/md-ershadullah/solar-irrigation-pumps-transforming-smart-irrigation-and-improving-agriculture
Fanus, A., Aregay, F., and Minjuan, Z. (2012). Impact of irrigation on fertilizer use decision of farmers in china: a case study in weihe river basin. J Sustain Dev. 5, 74–82. doi: 10.5539/jsd.v5n4p74
Faroque, M. A. A., Kashem, M. A., and Bilkis, S. E. (2011). Sustainable agriculture: a challenge in Bangladesh. Int. J. Agric. Res. Innov. Technol. 1:1–8. doi: 10.3329/ijarit.v1i1-2.13922
Feder, G., and Slade, R. (1984). The acquisition of information and the adoption of new Technology. Am. J. Agric. Econ. 66, 312–20. doi: 10.2307/1240798
FPMU (2015). National Food Policy Plan of Action and Country Investment Plan. Dhaka, Bangladesh: Food Planning and Monitoring Unit (FPMU) p. 231. Available online at: https://Fpmu.Gov.Bd/Agridrupal/Sites/Default/Files/File/MR-2015/ Monitoring%20Report%202015.Pdf (accessed July 4, 2022).
FPMU (2020). National Food and Nutrition Security Policy (NFNSP) - Draft Copy (English). Food Planning and Monitoring Unit (FPMU), Ministry of Food Bangladesh. Available online at: https://Fpmu.gov.bd/Agridrupal/Policy-and-Planning-Frameworks
Genius, M., Koundouri, P., Nauges, C., and Tzouvelekas, V. (2013). Information transmission in irrigation technology adoption and diffusion: social learning, extension services, and spatial effects. Am. J. Agr Econ. 96, 328–44. doi: 10.1093/ajae/aat054
Groothuis-Oudshoorn, C. G., Fermont, J. M., van Til, J. A., and IJzerman, M. J. (2014). Public stated preferences and predicted uptake for genome-based colorectal cancer screening. Med. Inform Decis Mak. 14, 18. doi: 10.1186/1472-6947-14-18
Hasanuzzaman, M., Nahar, K., Alam, M. M., Hossain, M. Z., and Islam, M. R. (2009). Response of transplanted rice to different application methods of urea fertilizer. Int. J. Sustain Agric. 1, 1–5.
Hasnat, MdA, Hasan, MN, and Hoque, N. (2014). A brief study of the prospect of hybrid solar irrigation system in Bangladesh. Khulna, Bangladesh: Khulna University of Engineering and Technology (KUET). (2014) p. 140285–6. Available online at: https://www2.kuet.ac.bd/icmiee2014/wp-content/uploads/2015/02/ICMIEE-PI-140285.pdf (accessed October 10, 2020).
Hess, S., Daly, A., and Batley, R. (2018). Revisiting consistency with random utility maximisation: theory and implications for practical work. Theory Decis. (2018) 84, 181–204. doi: 10.1007/s11238-017-9651-7
Hong, W., and Zhu, K. (2006). Migrating to internet-based e-commerce: factors affecting e-commerce adoption and migration at the firm level. Inf. Manage. 43, 204–21. doi: 10.1016/j.im.2005.06.003
Hossain, M., Bari, N., and Miah, S. U. (2021). Opportunities and challenges for implementing managed aquifer recharge models in drought-prone Barind tract, Bangladesh. Appl. Water Sci. Vol. 11, 15. doi: 10.1007/s13201-021-01530-1
Hossain, M., and Karim, A. (2020). Does Renewable Energy Increase Farmers' Well-being? Evidence from Solar Irrigation Interventions in Bangladesh. Tokyo: Asian Development Bank Institute (ADBI) p. 20. Available online at: https://www.adb.org/sites/default/files/publication/574811/adbi-wp1096.pdf (Apr 08, 2021).
Hossain, M. A., Hassan, M. S., Mottaleb, M. A., and Hossain, M. (2015). Feasibility of solar pump for sustainable irrigation in Bangladesh. Int. J. Energy Environ. Eng. Vol. 6, 147–55. doi: 10.1007/s40095-015-0162-4
Hossain, M. A., and Siddique, M. N. A. (2015). Water-A limiting resource for sustainable agriculture in Bangladesh. EC Agric. 1, 124–37.
Huang, M., Jiang, P., Shan, S., Gao, W., Ma, G., Zou, Y., et al. (2017). Higher yields of hybrid rice do not depend on nitrogen fertilization under moderate to high soil fertility conditions. Rice. 10, 5. doi: 10.1186/s12284-017-0182-1
IFC (2014). Impact of Efficient Irrigation Technology on Small Farmers. (2014). Available online at: https://www.ifc.org/wps/wcm/connect/1f630d98-dabc-41e4-9650-b8809d620664/Impact+of+Efficient+Irrigation+Technology+on+Small+Farmers+-+IFC+Brochure.pdf?MOD=AJPERES&CVID=lKbEzwG
Imbens, G. W., and Wooldridge, J. M. (2009). Recent developments in the econometrics of program evaluation. J. Econ. Lit. 47, 5–86. doi: 10.1257/jel.47.1.5
Irfan, M., Zhao, Z. Y., Rehman, A., Ozturk, I., and Li, H. (2020). Consumers' intention-based influence factors of renewable energy adoption in Pakistan: a structural equation modeling approach. Environ Sci Pollut Res Vol. 28, 432–45. doi: 10.1007/s11356-020-10504-w
Islam, M. N., Ishida, A., and Taniguchi, K. (2007). Market reform and farm demand for inorganic fertilizers in Bangladesh. Int J Agric Res. 2, 325–337. doi: 10.3923/ijar.2007.325.337
Islam, S. M. S., Islam, K. M. A., and Mullick, M. R. A. (2022). Drought hot spot analysis using local indicators of spatial autocorrelation: an experience from Bangladesh. Environ. Chall. 6, 13. doi: 10.1016/j.envc.2021.100410
Jahiruddin, M., Islam, M. R., and Miah, M. A. M. (2010). Constraints of Farmers' Access to Fertilizer for Food Production. Dhaka, Bangladesh: Food Planning and Monitoring Unit (FPMU). p. 44. Available online at: http://fpmu.gov.bd/agridrupal/sites/default/files/Final_Technical_Report_ (CF_03).pdf (accessed Jun 9, 2018).
Jansson, J., Pettersson, T., Mannberg, A., Brännlund, R., and Lindgren, U. (2017). Adoption of alternative fuel vehicles: Influence from neighbors, family and coworkers. Transp Res. Part Transp. Environ. 54, 61–73. doi: 10.1016/j.trd.2017.04.012
Kabunga, N. S., Dubois, T., and Qiam, M. (2012). Heterogeneous information exposure and technology adoption: the case of tissue culture bananas in Kenya. Agric Econ. 43, 473–85. doi: 10.1111/j.1574-0862.2012.00597.x
Kassie, M., Teklewold, H., Jaleta, M., Marenya, P., and Erenstein, O. (2015). Understanding the adoption of a portfolio of sustainable intensification practices in eastern and southern Africa. Land use policy. 42, 400–11. doi: 10.1016/j.landusepol.2014.08.016
Kazal, M. M. H., Rahman, M. S., and Rayhan, S. J. (2020). Determinants and impact of the adoption of improved management practices: case of freshwater prawn farming in Bangladesh. Aquac Rep. 18, 100448. doi: 10.1016/j.aqrep.2020.100448
Kishore, A., Alvi, M., and Krupnik, T. J. (2021). Development of balanced nutrient management innovations in South Asia: perspectives from Bangladesh, India, Nepal, and Sri Lanka. Glob. Food Secur. 28, 100464. doi: 10.1016/j.gfs.2020.100464
Kleemann, L., Abdulai, A., and Buss, M. (2014). Certification and access to export markets: Adoption and return on investment of organic-certified pineapple farming in Ghana. World Dev. 64, 79–92. doi: 10.1016/j.worlddev.2014.05.005
Kobir, M. R. (2019). Disproportionate Fertiliser Use Affecting Soil Health. The Business Standard. (2019) p. 4.
Krejcie, R. V., and Morgan, D. W. (1970). Determining sample size for research activities. Educ. Psychol. Meas. 38, 607–10. doi: 10.1177/001316447003000308
Krishnan, P., and Patnam, M. (2014). Neighbors and extension agents in ethiopia: who matters more for technology adoption? Am. J. Agric. Econ. 96, 308–27. doi: 10.1093/ajae/aat017
Kumar, V., Hundal, B. S., and Kaur, K. (2019). Factors affecting consumer buying behaviour of solar water pumping system. Smart Sustain Built Environ. 8, 351–64. doi: 10.1108/SASBE-10-2018-0052
Kumar, V., Syan, A. S., Kaur, A., and Hundal, B. S. (2020). Determinants of farmers, decision to adopt solar powered pumps. Int. J. Energy Sect Manag. (2020) 14, 707–27. doi: 10.1108/IJESM-04-2019-0022
Lancaster, K. (1966). A new approach to consumer theory. J. Polit. Econ. 74, 132–57. doi: 10.1086/259131
Larsen, M. A. D., and Drews, M. (2019). Water use in electricity generation for water-energy nexus analyses: the European case. Sci. Total Environ. 651, 2044–2058. doi: 10.1016/j.scitotenv.2018.10.045
Levidow, L., Zaccaria, D., Maia, R., Vivas, E., Todorovic, M., Scardigno, A., et al. (2014). Improving water-efficient irrigation: prospects and difficulties of innovative practices. Agric Water Manag. 146, 84–94. doi: 10.1016/j.agwat.2014.07.012
Liu, W., Wang, C., and Mol, A. P. J. (2013). Rural public acceptance of renewable energy deployment: the case of Shandong in China. Appl. Energy. 102, 1187–96. doi: 10.1016/j.apenergy.2012.06.057
Maddala, G. S. (1983). Limited-dependent and qualitative variables in econometrics. New York: Cambridge University Press. p. 401. doi: 10.1017/CBO9780511810176
Mamun, M. A. A., Haque, M. M., Saleque, M. A., Khaliq, Q. A., Karim, A. J. M. S., Karim, M. A., et al. (2018). Evaluation of different fertilizer management guidelines for boro rice T cultivation in south central coastal region of Bangladesh. Ann. Agrar. Sci. 16, 466–75. doi: 10.1016/j.aasci.2018.05.005
Manski, C. F. (1977). The structure of random utility models. Theory Decis Vol. 8, 229–54. doi: 10.1007/BF00133443
Martey, E., Wiredu, A. N., Etwire, P. M., Fosu, M., Buah, S. S. J., Bidzakin, J., et al. (2013). Fertilizer adoption and use intensity among smallholder farmers in northern ghana: a case study of the AGRA soil health project. Sustain Agric Res. 3, 24–36. doi: 10.5539/sar.v3n1p24
McFadden, D. (1974). “Chapter Four: Conditional logit analysis of qualitative choice behavior,” in Frontiers in Econometrics, Zarembka, P. New York: Academic Press. (1974). Available online at: https://eml.berkeley.edu/reprints/mcfadden/zarembka.pdf (accessed Oct 12, 2021).
Mendola, M. (2007). Agricultural technology adoption and poverty reduction: A propensity-score matching analysis for rural Bangladesh. Food Policy. 32, 372–93. doi: 10.1016/j.foodpol.2006.07.003
Mirta, A., Alam, M. F., and Yashodha, Y. (2011). Solar Irrigation in Bangladesh A Situation Analysis Report. Colombo, Sri Lanka: International Water Management Institute (IWMI) p. 39. Available online at: https://solar.iwmi.org/wp-content/uploads/sites/43/2021/09/BANGLADESH-SITUATION-ANALYSIS-REPORT_final-version-2.pdf doi: 10.5337/2021.216 (Mar 03, 2021).
Mottaleb, K., Krupnik, A., Timothy, J, and Erenstein, O. (2016). Factors associated with small-scale agricultural machinery adoption in Bangladesh: Census findings. J Rural Stud. 46, 155–68. doi: 10.1016/j.jrurstud.2016.06.012
Mottaleb, K. A. (2018). Perception and adoption of a new agricultural technology: evidence from a developing country. Technol. Soc. 55, 126–135. doi: 10.1016/j.techsoc.2018.07.007
Mottaleb, K. A., Rahut, D. B., and Erenstein, O. (2019). Small businesses, potentially large impacts: The role of fertilizer traders as agricultural extension agents in Bangladesh. J. Agribus Dev Emer. Econ. (2019) 9, 109–24. doi: 10.1108/JADEE-08-2017-0078
Naher, U. A., Shah, A. L., Sarkar, M. I. U., Islam, S. M., Ahmed, M. N., Panhwar, Q. A., et al. (2015). “Fertilizer consumption scenario and rice production in Bangladesh,” in Advances in Tropical Soil Science, Vol. 3, eds H. Jol and S. Jusop (Universiti Putra Malaysia Press), 81–98. Available online at: https://www.researchgate.net/profile/Md-Imran-Sarkar/publication/305442920_Fertilizer_Consumption_Scenario_and_Rice_Production_in_Bangladesh/links/6173b4173c987366c3cf6782/Fertilizer-Consumption-Scenario-and-Rice-Production-in-Bangladesh.pdf
Ntshangase, N. L., Muroyiwa, B., and Sibanda, M. (2018). Farmers' perceptions and factors influencing the adoption of no-till conservation agriculture by small-scale farmers in zashuke, kwazulu-natal province. Sustainability. 10, 555. doi: 10.3390/su10020555
Osborne, J. W. (2014). Best Practices in Logistic Regression. 1st ed. Los Angeles. SAGE Publications. (2014) 488 p. doi: 10.4135/9781483399041
Ouattara, N., Xiong, X., Traore, L., Turvey, C. G., and Sun, R. (2020). Does credit influence fertilizer intensification in rice farming? Empirical evidence from Côte D'Ivoire. Agronomy. 10, 1063. doi: 10.3390/agronomy10081063
Palash, M. S., Rahman, M. W., Amin, M. R., Mainuddin, M., and Jalilov, S. M. (2019). Water stress effect on the factors of production of irrigated rice in northwest regions of Bangladesh. Bangladesh J Agric Econ. 40, 43–56. doi: 10.22004/ag.econ.304093
Pandey, V. L., and Mishra, V. (2004). Adoption of zero tillage farming : evidences from Haryana and Bihar. SSRN Electron J. 2004, 17. doi: 10.2139/ssrn.529222
Pearson, K. A., Millar, G. M., Norton, G. J., and Price, A. H. (2018). Alternate wetting and drying in Bangladesh: Water-saving farming practice and the socioeconomic barriers to its adoption. Food Energy Secur. 7, e00149. doi: 10.1002/fes3.149
Piece, T. T. (2012). Disaster Risk and Resilience. New York: United Nations Department of Economic and Social Affairs (UN-DESA) p. 13. Available online at: https://www.un.org/en/development/desa/policy/untaskteam_undf/them_tp.shtml (accessed Oct 19, 2020).
Pretty, J. (2008). Agricultural sustainability: concepts, principles and evidence. Philos. Trans. R Soc. Lond. B Biol. Sci. 02, 447–65. doi: 10.1098/rstb.2007.2163
Prothom Alo (2021). Boro and Rabi crops Farmers should be given subsidy on diesel. Prothom Alo. p. 2.
Quddus, A., and Kropp, J. D. (2020). Constraints to agricultural production and marketing in the lagging regions of Bangladesh. Sustainability. (2020). 12, 3956. doi: 10.3390/su12103956
Rahman, K. M. A., and Zhang, D. (2018). Effects of fertilizer broadcasting on the excessive use of inorganic fertilizers and environmental sustainability. Sustainability. 10, 759. doi: 10.3390/su10030759
Rahman, M. S., Kazal, M. M. H., Rayhan, S. J., and Manjira, S. (2021). Adoption determinants of improved management practices and productivity in pond polyculture of carp in Bangladesh. Aquac Fish. 8, 96–101. doi: 10.1016/j.aaf.2021.08.009
Rahman, S. M., Faruk, M.d.O., Rahman, Md,.H., and Rahman, S. M. (2022). Drought index for the region experiencing low seasonal rainfall: an application to northwestern Bangladesh. Arab J. Geosci Vol. (2022) 15, 15. doi: 10.1007/s12517-022-09524-2
Ramappa, K. B., Kannan, E., and Lavanya, B. T. (2015). Adoption of recommended doses of fertilizers on soil test basis by farmers in Karnataka. Bangalore, India: Agricultural Development and Rural Transformation Centre Institute for Social and Economic Change. Available online at: https://www.researchgate.net/publication/306262602_Adoption_of_Recommended _Doses_of_Fertilizers_on_Soil_Test_Basis_by_Farmers_in_Karnataka (accessed Sep 18, 2018).
Rana, J., Kamruzzaman, M., Oliver, M. H., and Akhi, K. (2021). Financial and factors demand analysis of solar powered irrigation system in Boro rice production: a case study in Meherpur district of Bangladesh. Renew Energy. 167, 433–9. doi: 10.1016/j.renene.2020.11.100
Rana, M.d.J., Kamruzzaman, M., Oliver, Md,.M. H., and Akhi, K. (2021). Influencing factors of adopting solar irrigation technology and its impact on farmers' livelihood. a case study in Bangladesh. Future Food J Food Agric Soc. 9, 14. doi: 10.17170/kobra-202110144898
Ravisankar, N., Gangwar, B., and Prasad, K. (2014). Influence of balanced fertilization on productivity and nutrient use efficiency of cereal based cropping systems. Indian J Agric Sci. 84, 248–254.
Reza, S., and Hossain, E. (2013). Factors affecting farmers' decisions on fertilizer use: a case study of rajshahi district in Bangladesh. Bangladesh J Polit Econ. 29, 211–21.
Rubin, D. B. (2001). Using propensity scores to help design observational studies: application to the tobacco litigation. Health Serv Outcomes Res Methodol. 2, 169–88. doi: 10.1023/A:1020363010465
Sadegh, M., AghaKouchak, A., Mallakpour, I., Huning, L. S., Mazdiyasni, O., Niknejad, M., et al. (2020). Data and analysis toolbox for modeling the nexus of food, energy, and water. Sustain Cities Soc. 61, 102281. doi: 10.1016/j.scs.2020.102281
Sagheb, N., and Hobbi, M. S. (2002). Field evaluation of urea fertilizer and water use efficiency by tomato under trickle fertigation and furrow irrigation in the Islamic Republic of Iran. Austria: International Atomic Energy Agency (IAEA) p. 3–13. Available online at: https://www-pub.iaea.org/MTCD/Publications/PDF/te_1266_prn.pdf
Sajid, E. (2019). Solar irrigation holds promise for low-cost farming. The Business Standard. (2019) p. 7.
Sanap, S., Bagal, S., and Pawar, D. (2020). Factors affecting farmer's decision of adoption of solar powered pumps. Eur J Mol Clin Med. 7, 3762–73.
Sarker, K. K., Roy, D. K., Hossain, A., and Islam, M. M. (2014). Effect of different levels of fertilizer and irrigation on the yield of wheat under raised bed system. Agric For Fish. 3, 23–7. doi: 10.11648/j.aff.s.2014030601.14
Sarker, M. N. I., and Ghosh, H. R. (2017). Techno-economic analysis and challenges of solar powered pumps dissemination in Bangladesh. Sustain Energy Technol Assess. 20, 33–46. doi: 10.1016/j.seta.2017.02.013
Sarker, M. R., Galdos, M. V., Challinor, A. J., and Hossain, A. (2021). A farming system typology for the adoption of new technology in Bangladesh. Food Energy Secur. 10, E287. doi: 10.1002/fes3.287
Shawon, A. S., and Sourav, D.S. (2021). Budget FY22: Agriculture gets Tk759 crore higher allocation. Dhaka Tribune, First page. Available online at: https://www.dhakatribune.com/bangladesh/2021/06/03/budget-fy22-agriculture-gets-tk759-crore-higher-allocation
Shew, A. M., Morat, A. D., Putman, B., Nally, L. L., and Ghosh, A. (2019). Rice intensification in Bangladesh improves economic and environmental welfare. Environ. Sci. Policy. 95, 46–57. doi: 10.1016/j.envsci.2019.02.004
Siddique, M. N. E. A., de Bruyn, L. L., Guppy, C. N., and Osanai, Y. (2020). Temporal variations of soil organic carbon and pH at landscape scale and the implications for cropping intensity in rice-based cropping systems. Agronomy. 11, 59. doi: 10.3390/agronomy11010059
Simtowe, F., and Zeller, M. (2006). The impact of access to credit on the adoption of hybrid maize in Malawi: an empirical test of an agricultural household model under credit market failure. MRPA. Available online at: https://Mpra.ub.uni-Muenchen.de/45/ (accessed Apr 29, 2018).
Smale, M., Assima, A., Kergna, A., Thériault, V., and Weltzien, E. (2018). Farm family effects of adopting improved and hybrid sorghum seed in the Sudan Savanna of West Africa. Food Policy. 74, 162–71. doi: 10.1016/j.foodpol.2018.01.001
SREDA (2015). Scaling Up Renewable Energy in Low Income Countries (SREP), Investment Plan for Bangladesh. Sustainable and Renewable Development Authority (SREDA). Available online at: https://www.Climateinvestmentfunds.org/Sites/cif_enc/Files/Bangladesh_ Srep_ip_Final.pdf (accessed Jan 01, 2018).
SREDA (2022). National Database of Renewable Energy. Renewableenergy.gov.bd. 2022. Available online at: http://www.Renewableenergy.gov.bd/Index.php?id=01&I=4&s=&ag=&di=&ps=1&sg=&fs=&ob=1&Submit=Search (accessed February 4, 2022).
Stata (2013). Stata Treatment-effects Reference Manual: Potential Outcomes/Counterfactural Outcomes-Release 16. College Station T. X.: StataCorp LLC; (2013) p. 160. Available online at: https://www.stata.com/manuals13/te.pdf
Stuart, E. A. (2010). Matching methods for causal inference: a review and a look forward. Stat Sci. 25, 1–21. doi: 10.1214/09-STS313
Sunny, F. A., Fu, L., Rahman, M. S., and Huang, Z. (2022). Determinants and impact of solar irrigation facility (SIF) adoption: a case study in Northern Bangladesh. Energies. 15, 2460. doi: 10.3390/en15072460
Sunny, F. A., Huang, Z., and Karimanzira, T. T. P. (2018). Investigating key factors influencing farming decisions based on soil testing and fertilizer recommendation facilities (STFRF)—a case study on rural Bangladesh. Sustainability. 10, 4331. doi: 10.3390/su10114331
The Business Standard (2022). Budget 2022-23: Govt to increase fertiliser subsidy to ensure food security. The Business Standard. p. 1.
The World Bank (2021). Employment in agriculture (% of total employment) (modeled ILO estimate) - Bangladesh. Available online at: https://data.worldbank.org/indicator/SL.AGR.EMPL.ZS?locations=BD (accessed Sep 11, 2021).
Tiwari, K. R., Sitaula, B. K., Nyborg, I. L. P., and Paudel, G. S. (2008). Determinants of Farmers adoption of improved soil conservation technology in a middle mountain watershed of Central Nepal. Environ Manage. (2008) 42, 210–22. doi: 10.1007/s00267-008-9137-z
UCLA (2021). Multinomial Logistic Regression | Stata Annotated Output. UCLA: Statistical Consulting Group. Available online at: https://stats.oarc.ucla.edu/stata/output/multinomial-logistic-regression/ (accessed Mar 12, 2020).
WHO (2019). 1 in 3 people globally do not have access to safe drinking water – UNICEF, WHO. Available online at: https://www.who.int/news/item/18-06-2019-1-in-3-people-globally-do-not-have-access-to-safe-drinking-water-unicef-who (accessed June 19, 2022).
WHO (2022a). UN Report Global Hunger Numbers Rose to as Many as 828 Million in 2021. Available online at: https://www.who.int/news/item/06-07-2022-un-report–global-hunger-numbers-rose-to-as-many-as-828-million-in-2021 (accessed August 10, 2022).
WHO (2022b). Report COVID-19 Slows Progress Towards Universal Energy Access. Available online at: https://www.who.int/news/item/01-06-2022-report--covid-19-slows-progress-towards-universal-energy-access (accessed August 16, 2022).
Wikipedia (2022). Dinajpur District Bangladesh. In: Wikipedia, the free encyclopedia. Available online at: https://en.wikipedia.org/wiki/Dinajpur_District,_Bangladesh
Wooldridge, J. M. (2010). Econometric Analysis of Cross Section and Panel Data. Second Edition. Cambridge, Massachusetts: MIT. Press. (2010) 1064 p.
World Bank (2021). Fertilizer consumption (kilograms per hectare of arable land) - Bangladesh. The World Bank IBRD-IDA. Available online at: https://data.worldbank.org/indicator/AG.CON.FERT.ZS (accessed Sep 12, 2021).
Yadav, K., Geli, H. M. E., Cibils, A. F., Hayes, M., Fernald, A., Peach, J., et al. (2021). An integrated food, energy, and water nexus, human well-being, and resilience (FEW-WISE) framework: new Mexico. Front Environ Sci. 9, 667018. doi: 10.3389/fenvs.2021.667018
Zeng, D., Alwang, J., Norton, G., Jaleta, M., Shiferaw, B., Yirga, C., et al. (2018). Land ownership and technology adoption revisited: Improved maize varieties in Ethiopia. Land Use Policy. 72, 270–9. doi: 10.1016/j.landusepol.2017.12.047
Zheng, H., and Ma, W. (2021). Smartphone-based information acquisition and wheat farm performance: insights from a doubly robust IPWRA estimator. Electron. Commer. Res. (2021) 1–26. doi: 10.1007/s10660-021-09481-0
Zhou, D. (2017). The acceptance of solar water pump technology among rural farmers of northern Pakistan: a structural equation model. Cogent Food Agric. 3, 1280882. doi: 10.1080/23311932.2017.1280882
Keywords: sustainable agriculture, solar irrigation, recommended fertilizer dose, impact study, treatment effect models, sustainable farming, determinants, adoption
Citation: Sunny FA, Karimanzira TTP, Peng W, Rahman MS and Zuhui H (2022) Understanding the determinants and impact of the adoption of technologies for sustainable farming systems in water-scarce areas of Bangladesh. Front. Sustain. Food Syst. 6:961034. doi: 10.3389/fsufs.2022.961034
Received: 03 June 2022; Accepted: 07 November 2022;
Published: 24 November 2022.
Edited by:
Graham Jewitt, IHE Delft Institute for Water Education, NetherlandsReviewed by:
Isahaque Ali, Universiti Sains Malaysia (USM), MalaysiaCopyright © 2022 Sunny, Karimanzira, Peng, Rahman and Zuhui. This is an open-access article distributed under the terms of the Creative Commons Attribution License (CC BY). The use, distribution or reproduction in other forums is permitted, provided the original author(s) and the copyright owner(s) are credited and that the original publication in this journal is cited, in accordance with accepted academic practice. No use, distribution or reproduction is permitted which does not comply with these terms.
*Correspondence: Wenhuan Peng, cHdodWFuQDEyNi5jb20=
†These authors have contributed equally to this work
Disclaimer: All claims expressed in this article are solely those of the authors and do not necessarily represent those of their affiliated organizations, or those of the publisher, the editors and the reviewers. Any product that may be evaluated in this article or claim that may be made by its manufacturer is not guaranteed or endorsed by the publisher.
Research integrity at Frontiers
Learn more about the work of our research integrity team to safeguard the quality of each article we publish.