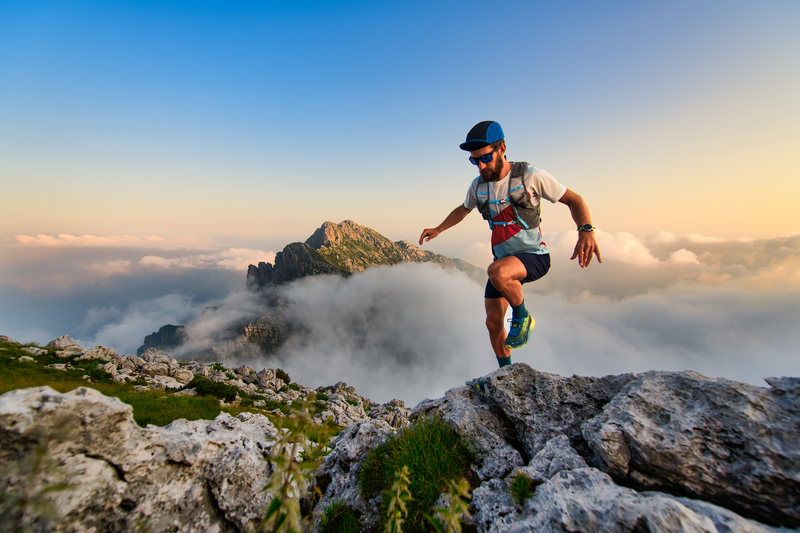
94% of researchers rate our articles as excellent or good
Learn more about the work of our research integrity team to safeguard the quality of each article we publish.
Find out more
ORIGINAL RESEARCH article
Front. Sustain. Food Syst. , 14 November 2022
Sec. Water-Smart Food Production
Volume 6 - 2022 | https://doi.org/10.3389/fsufs.2022.954934
Despite extensive research on farmers' constraints and decisions, technology developers, policymakers and development organizations still encounter difficulties in relating policies to farmers' strategies. Often, the concept of ‘smallholders' is applied as explaining and predicting farmers' decisions—suggesting that specific strategies of farmers can be meaningfully related to their farm size. Our study into farmers' decision-making concerning water transport technologies in Malawi suggests that this way of grouping farmers in policy and development programs does not match actual decision strategies. Using Q-methodology (Q) as a method allowed us to find decision-making patterns without predefining variables that would influence decision-making. We found that farmers within a predefined smallholder group did not decide in the same ways. Furthermore, our results show that decision-making has a clear gender dimension. We argue that Q is able to capture the nuances of farmers' decision-making processes. As such, the methodology potentially provides a useful feed for policy and technology development.
Malawi is a landlocked country between Mozambique, Zambia and Tanzania, facing high climate variability and many agricultural challenges. Its economy predominantly depends on agriculture, with the sector contributing over a third of the Gross Domestic Product on average (SMEC, 2015). Occasionally it is even higher (e.g., 40% in 2013 according to Harrison and Chiroro, 2016). Agriculture covers about 90% of the total domestic exports (OEC, 2020). As such, the agricultural sector supports over three quarters of the population (SMEC, 2015; Harrison and Chiroro, 2016)—or about 14 million people. Unfortunately, production increases have failed to keep up with population growth, resulting in food shortages in times of poor rainfall (FEWS NET, 2012). With this in mind, the Malawi government has developed an active policy to stimulate agricultural production in the country. Strengthening access to water to secure crop growth is a major pillar of this policy, with subsidies and other arrangements in place to facilitate farmers' uptake of new technologies.
When developing and applying policy measures, it is quite reasonable that policymakers and technology developers standardize target farmer groups to a certain extent, in terms of preferences and decision-making. This standardization can be based on the available (extensive) research on farming typologies and the complexity of farmers' adoption of technologies (Tittonell et al., 2010; Wigboldus et al., 2016; Kuehne et al., 2017; Alvarez et al., 2018; Hammond et al., 2020; Llewellyn and Brown, 2020; Montes de Oca Munguia and Llewellyn, 2020; Saengavut and Jirasatthumb, 2021; Sarker et al., 2021). Nonetheless, various agricultural policies still rely on one explanatory factor, land size ownership, to relate to farmers' conditions and decision-making. Examples of this are European payment schemes (European Commission, 2021; Toma et al., 2021), agricultural transformation policies (Fan et al., 2013), agrarian reforms (Cousins, 2013; Scoones et al., 2019), subsidy programs (Mason and Jayne, 2013; Sharma et al., 2015), and credit allocation (Isaga, 2018). Even one of the more famous current policy criteria, the global food security target (SDG2, target 2.3) (Gil et al., 2019; United Nations, 2020), builds on land ownership. Using land size (ownership) to explain farmers' preferences is based on the assumption that farmers owning relatively small or large pieces of land are supposed to make decisions in specific, different ways. Larger farmers are typically perceived as decision makers with commercial interest, whereas smallholders are automatically taken as the opposite.
Imposing unrepresentative labels on a continuum of characteristics, using a single variable to categorize the complex realities of farmers, may actually fail to stimulate take-up of agricultural technologies. Within this frame of reference, we conducted field research to study how Malawian farmers make decisions concerning water transport technologies (WTTs) for agricultural irrigation (van Dijk, 2020)—with our study including gravity irrigation system, watering can, petrol pump, treadle pump, solar pump and the Barsha pump (the latter being a recent innovation, see Intriago Zambrano et al., 2019). Our results, as reported in van Dijk (2020), suggest that land size does not explain or predict how farmers make decisions and cannot represent the diversity of strategies that farmers employ. We found that many Malawian smallholders actually think in a commercial way. Rather than their mindset, their options to act on that decision-making strategy are less. Limited land ownership may constrain concrete options to improve farming, but not necessarily explain how farmers reason about farming.
With these considerations in mind, the objective of this paper is to discuss the spectrum of decision-making strategies that Malawian farmers employ in the adoption of WTT in agriculture. After a brief historical background on farming categorization and typologies, we explain our data collection and analysis strategy in some detail, as we think that the Q-methodology (henceforth Q) is especially promising to bring forward the spectrum of decision-making—or other relevant topics for that matter. Once we have analyzed the qualitative and quantitative data related to decision-making strategies of our respondents, we contrast our findings with the land-ownership labels we criticized above and we explore differences between male and female farmers. After a discussion and brief conclusion on our field study, we suggest how our findings matter for actors in the larger field of agricultural development.
In the last 50 years, many governments, national and international development organizations and NGO's have invested heavily in agricultural development (African Development Fund, 2006). To ensure investments resulted in effective policy formation, numerous efforts have been made to describe farmers and their properties (Collinson, 2000). Categorization (and simplification) of farmers made policies manageable, enabling streamlining and targeting aid initiatives and technological interventions in the agricultural sector. Farm(er)s were classified by land property size to provide a picture of the agricultural resources and their optimal utilization (USDA, 2020). In the 1960s, many studies described resource allocation patterns and productivity, mainly among resource-limited farmers in the “Global South” (Norman, 2002). However, technological recommendations were rarely adopted because they were poorly designed or irrelevant, especially when contrasted against criteria relevant to farmers (Collinson, 2000). The farmer-data extraction approach of these contrasted sharply with later participatory approaches that involve farmers in technological design and development (Norman, 2002).
From the mid to late 1970s, the attempt to understand farming phenomena evolved to more inclusive farming systems research (Norman, 2002). A related research methods evolved around the concept of farming styles and typologies (van der Ploeg, 1985, 1994) in the late 1980s, with the more specific aim to understand diversity in farming communities. A farming style encompasses the complex but integrated set of variables, norms, knowledge elements, experiences, etc., that describe the way farming decisions are made. Studying farming styles provides (simplified) representations of community diversity through relatively homogeneous groups of farm types (Alvarez et al., 2018). Despite the evolution in understanding farming systems, and the complexity of its many variables, research findings have largely not been translated into adequate policies, programs and projects. The single variable of farm size is still the dominant variable to classify farmers, mainly because of its relative ease to measure and availability of (access to) data. Farm size is considered to explain differences in technical efficiency, land productivity and income, and a major influence on decision-making behavior (Katongo, 1986; Lund and Price, 1998; Lowder et al., 2016; Rose et al., 2018).
The most common definition (Thapa and Gaiha, 2014; Rapsomanikis, 2015), also used in the World Bank's Rural Development Strategy (World Bank, 2003), defines smallholders as farmers “with a low asset base and operating in<2 hectares of cropland.” What a small or big farm size is, however, is relative to its context. Across different countries, the distribution of farm sizes depends on a number of agro-ecological and demographic conditions, as well as economic and technological factors (FAO, 2015). This contextual influence is nowadays embraced (to certain extent) by FAO's middle-sized farm threshold per country (Rapsomanikis, 2015). In accordance to this threshold, expressed in hectares, smallholders and large farmers are defined as those managing a farm smaller (or equal to) and bigger than that area, respectively. However, as we discuss further below, land size did not explain reasoning on decision-making of any of these farmers. First, we move to the methodology that allowed us to reach that conclusion.
We relied on Q as the research method for this study. The methodology clusters participants according to their ranking of value- and goal-related stimuli (frequently written statements) and thereby creates typologies that encapsulate the diversity of the participants (Pereira et al., 2016). In short, Q groups participants that sort statements in roughly the same way, which results in groups that show similar viewpoints and considerations. As such, Q provides insight into unique viewpoints or perspectives through systematic examination and understanding of an individual's subjectivity. We opted for Q for two reasons. First, it has the ability to capture qualitative aspects of the topic under study, while offering at the same time a robust statistical approach, thereby combining the strengths of qualitative and quantitative methods (Simons, 2013). Second, as a participatory method, Q enables a stronger farmer participation due to its bottom-up construction of what subjectively matters in the adoption of WTTs (Donner, 2001). To clarify the methodological deployment of Q in our study, we describe how we developed its four steps: (1) Research design, (2) Data collection (administration), (3) Analysis (and consequent results), and (4) Interpretation (as described in Zabala et al., 2018).
In order to conduct a Q study successfully, one needs a representative image of the voices and positions around the issue under consideration—or the “concourse.” The content of the concourse determines the quality and reliability of the findings and the identification of the resulting viewpoints. The better the resources from which the concourse is developed, the better it is able to provide a representation of options related to an individuals' subjectivity. We built the concourse on farmers' adoption of WTTs resorting to a two-step approach. First, an initial list of statements was drawn from secondary data sources, i.e., (non)academic literature. Later, in June 2019, we performed semi-structured, tape-recorded on-site interviews with 13 farmers and seven agricultural experts in Malawi. We sampled both farmers and experts through convenience and purposive sampling techniques, based on the network of contacts of the first author, and aiming to cover diverse geographic areas in Malawi. With these interviews, we could validate the initial list, but also gather additional contextualized statements related to the topics within the concourse. Particular attention was paid to understand how the subjectivities surrounding different variables (finances, management, ownership, technology characteristics, etc.) ultimately shape decisions on uptake (or not) of WTTs.
Our initial set of topics in the concourse was reduced through further categorization, deletion and combination of duplicates—using categories of farmer-related, technology-related and contextual variables (Montes de Oca Munguia and Llewellyn, 2020). Our final Q-set counted 34 statements related to farmers' decision-making on WTT uptake, with the set of statements balancing out between categories. This number of statement in a Q-set is within typically accepted ranges (Watts and Stenner, 2012). The statements were initially written in English; they were translated to Chichewa (local Malawian language) by native speakers [Appendix A in Supplementary materials (van Dijk and Intriago Zambrano, 2020)].
The 34 statements fitted with a mesokurtic, 9-point, forced inverted quasi-normal distribution sorting grid—consisting of a −4 to +4, “strongly disagree” to “strongly agree” scale (Table 1). We considered this grid as most useful for two reasons. First, farmers and extensions officers are not necessarily equally knowledgeable on the topic (if they would have been, a platykurtic shape would have been useful). Second, we are not exploring a phenomenon that is fully unfamiliar to our target audience (which would have suggested a leptokurtic shape) (Watts and Stenner, 2012). We provided two positions on each extreme, thus allowing a few elements in the category of most (dis)agreement, as this offered more explanatory information for the later interpretation of factors.
We sampled both farming communities and respondents by means of a purposive sampling technique (Watts and Stenner, 2012). Communities were selected in agricultural areas with several WTTs. Respondents were farmers offering a suitable diversity for three criteria: land size, types of farming (individual or cooperative), and WTTs being used. We reached both communities and respondents through a network of agricultural experts operating in Malawian farming systems. These agricultural experts themselves participated as proxy-respondents on behalf of farmers in their respective districts. This additional participation allowed further study how farmers and experts compare with respect to WTT uptake. Details of the 58-respondent P-set, including category, specific role, gender location and WTTs used, can be found in Appendix B in Supplementary materials (van Dijk and Intriago Zambrano, 2020).
We administered Q exclusively through the face-to-face technique. With farmers, Q was usually conducted right next to their respective houses or farms, whereas for experts Q was mostly conducted in their respective offices. We allowed both individual and collective sorting sessions with farmers. The (few) collective Qs were performed with farming cooperatives, in which several members had group discussions that resulted in a single sort deemed representative of their organization and its internal dynamics. As far as we can see, these different sorts can be compared. We will return to these different Q sorts in the results section.
Sorting sessions were either in English or Chichewa, depending on the preferred language of the respondent—with Chichewa users usually using a fellow farmer or a local extension officer to translate between the user and the researcher. We made sure as best we could that this extra involvement did not influence the sorting itself. When sorting, respondents were asked the umbrella question: What are the most important decision making elements for me as a farmer in adopting water transport technology for irrigation? We offered a four-stage assistance to the participants: (1) pre-sort instructions in English and Chichewa (i.e., researchers, study goal, sorting dynamics), both written and verbally (mandatory in the case of illiterate participants); (2) preliminary sorting with the optional three-pile technique (i.e., conducting a first rough distribution by disagree, neutral, agree criteria), although participants frequently skipped this step, thus engaging directly with the full 9-point sorting grid (as indicated in Table 1); (3) by-step sorting guidance; (4) on-demand clarification and support. Each participant sorted on the sorting grid, drawn on a cardboard, with the 34 statements that were printed and glued on cardboard chips (890 mm × 890 mm) with a randomly assigned number behind. Participants could shuffle the chips on the go around the grid according to their own judgment. We recorded the final distribution, after which we conducted tape-recorded interviews with participants, where they explained their respective choices and reasoning.
We analyzed the dataset of collected sorts with KADE (Ken-Q Analysis Desktop Edition) v1.2.0 (Banasick, 2019), which has the benefit of a non-proprietary GNU General Public License, user-friendly graphical user interface, and cross-platform availability. As factor extraction method, we employed the Principal Component Analysis technique, which we preferred to Centroid Factor Analysis method, due to its single, mathematically-best solution (Watts and Stenner, 2012). We opted to explore different factor-number solutions with respect to the Kaiser-Guttman criterion [Eigenvalue (EV) threshold; EV>1.0] (Watts and Stenner, 2012), composite reliability (rf ≥ 0.94) (Ghazali et al., 2018), representativeness criterion (≥50% P-set loaded) (Hylton et al., 2018), and distinguishing statements (≥5 distinguishing statements at statistical significance p < 0.01) (Cammelli et al., 2019). Retained factors were rotated with the Varimax technique, given its maximum variance solution and fit for a holistic analysis trajectory. We required the majority of common variance to automatically load significant sorts (p < 0.05), resulting in confounded sorts not being taken into account.
We interpreted the retained factors by means of the holistic technique of crib sheets (Watts and Stenner, 2012), which focuses on the examination of factor scores in relation to other statements within the factor, as well as on its comparison with other factors. Crib sheets ensure that the interpretation considers the relative positions of statements within each factor, not just the individual statements in isolation.
We managed to collect a total of 58 valid sorts [Appendix C in Supplementary materials (van Dijk and Intriago Zambrano, 2020)], which usually took 20–40 min each after initial instructions. The sorts came from 49 farmers (either individuals or cooperatives' representatives) and 9 experts. Farmer respondents ranged from poorly-educated farmers operating on ~0.1 ha farms, to well-educated farmers farming on areas up to 5 ha. Table 2 contains details on respondent. Most of the farmers that were initially interviewed during the concourse development were revisited for the sorting and data collection. As such, the extensive qualitative insights collected during the interviews could help explaining their sorting decisions. Besides, many interviewees had expressed an interest in knowing how their input had been translated by us into the puzzle-like Q exercise.
Our data collection created a P-set/Q-set ratio of 1.71; although this ratio is higher than typically, in practice this does not pose further statistical issues (Watts and Stenner, 2012). Given that female farmers usually bear poorer access to resources of all kinds (Poole, 2017; Giordano et al., 2019), we acknowledge that the male-skewed P-set could mean certain form of underrepresentation of the topic under study.
Our exploration of possible analysis trajectories that met the criteria mentioned in section Analysis (Table 3) suggests how different decision-making styles interact with each other and that, depending on the chosen factor solution, respondents can be understood representing views with multiple aspects—which are stressed depending on the factor selected. This is illustrated in the Q maps of the three- and four-factor solutions (Figures 1A,B, respectively) (see Yoshizawa et al., 2016) for details on this aspect. Q maps are based on two axes, with in our case the horizontal axis relating to the theme “support,” and the vertical one the theme “risk.” We aim to show among the “support axis” whether respondents value being “independent” or whether they value “support” (including advice) from others. The “risk axis” spreads from “risk averse” to “risk taker,” depending on how much value participants attached to familiarity and understandability in selecting WTTs. The plotted values are the average scores of those loading in a factor, specifically for the statements related to support and risk. Another theme we explored, but do not depict here, is the cost-effectiveness orientation of the respondents. Details of themes, calculations of scores and plotting of Q maps can be found in Appendix D in Supplementary materials (van Dijk and Intriago Zambrano, 2020).
Figure 1. Q maps of (A) three-factor and (B) four-factor solutions. Gray circles, red squares, blue diamonds and yellow triangles represent factors 1, 2, 3, and 4 (for four-factor solution), respectively. Bubbles with the same colors reflect the clustering of the corresponding loaders regarding the support and risk thematic axes.
The Q maps show the dynamic clustering and (possible) shared viewpoints of the loaders, specifically depending on the analysis trajectories that the researcher(s) aim to select. The bubble around the respective plotted loaders captures the perspectives of each factor, with a factor being a group of respondents that share a similar sorting—even with finding variance within a factor, with some participants even plotting outside the core factor bubble. Furthermore, as factor bubbles overlap, we must also assume that participants of different factors can share similar perspectives on certain decision-making theme. Q maps are to be understood as exploratory tools, with their construction being the choice of the researchers. Q maps do help in rapidly observing factors' distribution and identifying associated discourses.
We concluded that both the three- and four-factor solutions gave enough distinguishing statements for analysis. The “perfect fit” does not exist, but we did consider the four-factor solution as the most interesting fit for the data and field observations [KADE analysis log in Appendix E in Supplementary materials (van Dijk and Intriago Zambrano, 2020)]. It offered us the best trade-off between the maximum variance—its embracement of variety and subjectivity—and the interpretability potential to make meaningful ontologies out of each of the factors (Pereira et al., 2016). The four factors represent 43 of the 58 participants (74% of the sample) and account for 55% of the total variance. Characteristics of factors and factor scores are given in Tables 4, 5, respectively. The remaining 15 participants loaded insignificantly on any or significantly on more than one factor. These confounded loaders are not analyzed further in this text, but it is useful to keep in mind that the variation we report among respondents may be even higher.
Each of the four factors can be considered as a synthetic representative farmer according to key sorting behavior. As such, we did create brief participant typologies to synthesize our findings (Table 6), in line with a common practice in Q methodology (see Fairweather and Keating, 1994; Walter, 1997; Brodt et al., 2006; Burton and Wilson, 2006; Pereira et al., 2016). Actually, it is quite common to assign labels to factors according to key characteristics. The wording of these labels matter. The main risk of using labels is that one could fall back into certain prejudices. This may result in a traditional way of thinking in which certain characteristics are forced upon an individual or group. Such an approach in essence undermines the goal of Q to consider the gathered data in terms of the participants' own (patterns of) responses. Predefined grouping would mean looking for predefined patterns among people, whereas Q builds on the claim that “people and not tests that are the variables” (Coogan and Herrington, 2011, p. 24).
Many complexities are reflected in the clustering of the P-set, and these should therefore be interpreted with caution. The interpretation with specific terms might be distorted by bias, affecting the validity and reliability of findings. Factors' multidimensional character, inherent to the concourse and the resulting statements, should not be summarized into one single orientation. To avoid such occurrences, we did not give names to our four factors—but we did provide a set of terms that seem to cover the different considerations reflected in the sorting. These were composed by examining the sorting of statements in relation to other statements within each factor and between factors [crib sheets of Appendix F in Supplementary materials (van Dijk and Intriago Zambrano, 2020)]. The detailed interpretations of the factors are shared in the subsections, using the notation of number of statement and factor score. As an example (29: −4) means that statement 29 was scored −4 (in line with the scores of Table 5).
Factor 1 (F1) has an EV 22.00 and explains 15% of the total rotated variance; 12 participants load significantly in this factor, with the vast majority (n=10) being smallholders, along with one larger farmer and one expert. Most WTTs used in this group are gravity irrigation and watering can, alongside a single loader using a petrol pump.
The idealized loader of F1 expresses a strong focus on WTTs offering high water flowrates (17: +4) and pressure (18: +3)—in contrast to the lower importance given to efficient water use (21: −1), and even ignoring the limitations that specific water sources may pose (23: 0). This farmer has a deep willingness to invest in (25: −4; 7: −3) and seek for a WTT (5: −2), particularly on one that enables the production of cash crops to increase revenues (19: +4). In fact, the ideal F1 respondent would prefer to invest upfront (1: 0) in a more expensive WTT, as long as it is easy to service (13: +3) despite its maintenance cost (14: 0), can be sourced/pooled with other farmers (8: +2) and has low operation costs (9: +1). For this farmer, WTT maneuverability (11: −2), testability (32: −1) and familiarity (33: 0) are less important. These characteristics may perhaps suggest that the F1 farmer is after a solar pump, were it not that the ideal loader expresses the preference to pay for fuel to keep running the WTT (3: +2)—which resembles a petrol pump. This could relate to the observation that most loaders rely on gravity irrigation systems, and may have limited access to sources of information about other available WTTs. This potential conflict in making a choice also shows up in terms of external support. The F1 farmer carefully follows advice provided by local extension officers (27: +3), but also prefers to rely on foreign WTT suppliers (28: −4).
Factor 2 (F2) has an EV of 3.74 and explains 13% of the total rotated variance; 11 farmer participants load significantly in this factor, with eight corresponding to smallholders. It is worth noting that F2 did not load any of the experts. Most WTTs used by respondents correspond to automatic devices such as the petrol, solar and Barsha pumps—even though we also find one user of gravity irrigation, two loaders using watering cans and one case of a treadle pump.
The F2 ideal farmer values WTT cost-effectiveness. In the decision-making process, this farmer is strongly willing to invest (25: −4; 7: −3) and seek for a WTT (5: −2), either individually or in-group (8: 0), paying special attention to the general affordability of the (initial) investment (2: +4; 21: +3), and long-term maintenance (14: +4) and running costs (9: +1; 3: −4) of the WTT. Paying in installments, however, is not of considerable importance for this farmer (1: 0). This could suggest she has enough financial resources to pay for the technology. Another important variable of the cost-effectiveness is the individual ease of use and labor saving ability of the WTT (10: +3). Its capacity to operate automatically is therefore strongly preferred (16: +2). Unlike watering cans—which require substantial human power—the WTT should require little human effort or attention when pumping water. This enables the farmer to focus on other activities such as working on the crops or expanding the farm. F2 farmer attaches relatively low value to familiarity (31: −2; 32: −1; 30: 0; 33: +1), understandability (34: 0), safety (12: −1), or status (15: −2). She is confident enough to adopt the technology, as long as it satisfies the criteria mentioned above. This reasoning can be in close relation with the types of automatic water pumps that many of the loaders are already using.
Factor 3 (F3) has an EV of 3.23 and explains 12% of the total rotated variance; 9 participants load significantly in this factor, with one smallholder, four large farmers and four agricultural experts. The loaded farmers use mechanized WTTs: petrol, pump and Barsha pumps.
The idealized farmer of F3 considers that different biophysical farm conditions influence the appropriateness of a WTT. She is inclined to find the best technology fit (23: +4), which is not necessarily determined by high pressure (18: −3) or flowrate (17: 0). Consequently, the F3 farmer is willing to invest in a more optimal WTT (25: −4; 26: −3; 4: −2), despite high investment costs (6: −4). To cope with possible upfront costs, this farmer prefers to pay in installments (1: +2), either individually or in-group (8: 0). This reduces risks associated to high initial investments and opens up a wider range of technology options. This farmer is also rather risk-averse, expressed in the relatively high value given to familiarity (30: +3; 31: +2; 33: +1), understandability (34: +3) and testability (32: +1) of the WTT, but also in the preference for technologies that are hard to vandalize or steal (12: +4). Moreover, F3 farmers manifest a low attachment to external support (27: 0; 28: −1). This could be a reflection of well-informed farmers, which may fit with their current use of automatic water pumps.
Factor 4 (F4) has an EV of 2.70 and explains 15% of the total rotated variance; 11 participants load significantly in this factor. It is worth remarking that this was the only factor consisting exclusively of smallholders, as it did not load any large farmer or agricultural expert. Gravity irrigation is the prevalent WTT used, followed by a smaller representation of watering can, petrol pump and Barsha pump.
The ideal F4 farmer appears to be influenced by variables involving external support. This support can encompass finances and help with farming inputs, but also knowledge about irrigation management, local markets or different WTT options (28: +4). The decision-making process, for instance, seems to be strongly steered by professional advice given by governmental extension officers (27: +3), and not by community peers or relatives (24: −3). There is the strong willingness to invest in a WTT to irrigate better (25: −4; 22: −4; 4: −2); perhaps this is related to the prevalent use of gravity irrigation among the loaders. However, financial restrictions may limit the availability of choices. Paying for technologies in installments (1: +3)—and even more if pooling financial resources with a group (8: +2)—thus helps in opening a wider range of technology alternatives. Easy maneuverability of the technology (11: +2) may become an enabler in sharing a WTT with a fellow farmers, hence further facilitating in sharing the investment. In contrast, less important decision factors are the ease of operations (10: 0), maintenance costs (14: 0), familiarity (33: 0) and pumping performance (17: 0). Moreover, the use of an automatic (or less human-power demanding) WTT is not considered important (16: −3), possibly due to its linkage with expensive fuels (3: −2) and high upfront costs of the device (9: −1). This farmer seems to value both self-consumption (20: +4) and commercialization (19: +3).
With the four factors in mind, we compared our four-factor categorization of farmers to the 0.91 ha middle-sized farm threshold in Malawi (FAO, 2017). According to this threshold, Malawian smallholder farmers operate farms ≤ 0.91 ha, whereas large farmers operate >0.91 ha. In Table 7, the participants are labeled according to these definitions and compared to the factors. We can clearly observe that the predefined groups of smallholder farmers, large farmers (and experts) do not sort in one characteristic way for their labels or roles. The group showing the greatest variety in sorting behavior is the smallholder farmer group, as its members loaded in all four different factors—with several of them highly correlating to factors with commercial mindsets and independent objectives. Large farmers also sorted in different ways, with a more specific focus on either F2, with its focus on cost-effectiveness and labor saving ability, or F3 where participants expressed a focus on risk aversion, independency and context-awareness. Interestingly, about half of the experts ended up in the unloaded group, meaning they loaded insignificantly on one single factor or shared significant sorting behaviors with multiple factors. We can speculate about a possible underlying mismatch between farmers' decision-making rationales compared to the experts' understanding, but a definite claim in this respect is out of the scope of our current work.
Table 7. Overview of the factors' composition with respect to the predetermined labeling of respondents.
What we think we can claim is that farmer decision-making on WTT in Malawi is not rooted in the variable of land size, which is so dominant in policymaking and implementation. This stresses the observation we already made above: with factors representing different possible grouping patterns—compared to typical ways of labeling—we need to be careful with how we (pre)define people. Literature and policies, for instance, tend to illustrate that Malawian smallholder farmers are stagnant and destined to decline, as they do not (yet) think commercially. In contrast, our results show smallholders that show that type of decision-making. We suggest these farmers are willing to move into commercial farming, but may simply not have the means to do so. As such, farm size might have an influence on what farmers (can) do, but would not be the single variable to explain farmer's decision-making.
Women often hold completely different investment priorities than men, with female farmers often value timesaving technologies and male farmers more likely to value technologies that increase productivity (Byanyima, 2015). To explore this gendered decision making, we present the gender distribution in the four respective factors in Table 8—with sorts performed in mixed groups of male and female indicated as well. The table presents the number and percentage of male, female and mixed groups in their respective factors, and the normalized percentages relative to sample size distribution. We encounter male and female farmers in every factor (or decision-making preference), with a high normalized female percentage in F1 (characterized by water volume and group-based WTTs) and F2 (characterized by cost-effective and labor saving technologies). Less females loaded on F3, characterized by independent and risk averse decision-making. While this research is not focused on the impact of gender aspects on farmer decision-making, we should clearly not assume that men and women farmers have the same investment preferences (see van Koppen et al., 2013), nor that all women farmers have the same preference. Understanding the variables that distinguish decision-making between and for different genders, possibly under different conditions, could be very valuable to study in future (Q) research.
Our findings suggest that the decision-making variables surrounding the adoption of WTTs in Malawi is highly diverse. Our Q approach allows us to suggest that regarding these WTTs, it makes sense to distinguish between four participant types, characterized by different distinguishing variables. We do not suggest in any way that these four types to be written in stone, but we do claim that our four factors show that there is considerable diversity with the groups of smallholders, large farmers and experts concerning decision-making on WTT adoption in Malawi. The notion of farming decision making is therefore not exclusively rooted in the variable of land size. We have also suggested that the strategies of female and male farmers may be different in terms of how many male and female farmers are represented in different factors. Our findings are in line with other studies that identified different farmer types as relatively homogeneous groups (Brodt et al., 2006; Pereira et al., 2016; Vander Vennet et al., 2016). Although further background variables were beyond the scope of this work, it would be a valuable addition for further research to explore underpinning reasons, other than land size, why some farmers sorted differently than others.
Again, we do not claim that the four factors that we presented are the perfect—let alone only—representation of participants' subjectivity concerning the adoption of WTTs. We acknowledge that it is challenging, if not impossible, to fully capture the diversity of farming systems in any study, as it is inevitable that the rich set of considerations of actual decision makers is simplified and standardized. With that in mind, we argue that current land-based categorizations of farmers do not support the development of effective policies for agricultural development. When using this definition, one can be labeled as a “smallholder farmer” and yet have (contradictorily) similar decision-making patterns as a “commercial farmer” or an “expert.” As such, land size has to be considered a poor measure to predict farmer decision-making.
A more representative categorization of farmers can be achieved through bottom-up approaches, giving the diversity of decision makers a clearer voice through participatory methods. We argue that Q is particularly suited to explore this representative diversity, as Q allows going beyond a single-variable threshold (i.e., land size) without predefining too strict what the unknown diversity would entail. Whereas, the actual design of the Q sort itself obviously does already reduce the complexity that can be encountered, the analysis promotes a richness in results. Depending on the analysis trajectory the researcher selects, individuals can be “transformed” from one group to another, and how different decision-making styles interact with each other. This suggests that farmer technology decision-making is part of a social dynamic system that is influenced by a wider range of variables, including the perspective of the researcher(s)!
This explicit acknowledgment of social dynamics in itself is in contrast with more traditional top-down thinking of straight-lined technological transfer from governments, development organizations and technology sellers, that was, and often still is, apparent in low-income countries. In this approach, the (smallholder) farmer is still the end-user, a passive actor, a receiver of whatever is done before. Moreover, while other possible variables of influence in decision-making (e.g., uncertainty, capital, costs, performance, etc.) are abundantly recognized and discussed in literature, they are rarely translated into policies.
Once we know that being a smallholder does not mean at all that one is only caring to provide food for one's household, it must mean that smallholders are not to be understood as “those poor farmers who need to be made aware of commercial strategies.” Our results suggest that many Malawian smallholder farmers want to develop themselves to be competitive in the (local) market. In our study, we did discuss with smallholders (or their cooperatives) who have organized themselves, created large and meaningful entities for the local market, and become commercially competitive. However, it often might be the case that smallholders do not have the immediate opportunities to develop those commercially attractive farming strategies—which may partially be the result of not being recognized as capable decision makers within policy programs.
Whereas, we cannot claim that every situation will be similar to what was found for Malawi, we do think that our findings do have a wider relevance for the scholarly and policy communities involved in agricultural development. Smallholder farmers can become the nursery from which successful commercial farmers can develop—and should be valued as such (Aliber and Hall, 2012). Hence, the definition of “smallholder farmer” used in policy should be avoid the strict focus on farm size. Smallholders are not a homogeneous group, but rather a diverse set of farmers with varying characteristics, as Llewellyn and Brown (2020) firmly stress as well. As other studies suggest, there could be alternative underpinning reasons for differences in decision-making (Matshe and Young, 2004; Pannell et al., 2006; Doss et al., 2014; Doss and Meinzen-Dick, 2015; Mutenje et al., 2016; Wheeler et al., 2017). Compressing the meaning of a farmer down into one category that in practice represents multiple characteristics of farmers, hides the importance of the question (who decides) which characteristics are to be included anyway. This is especially relevant when these definitions take center stage in policies, technologies and development programs.
Proper understanding with the aim to alleviate specific constraints of farmers promises to produce higher benefits compared to implementing and promoting blanket, universal strategies and technologies. Instead of a convenient standardized one-size-fits-all imposing approach—in which it is predefined what farmers need and want based on unrepresentative criteria—policies, investments, innovations and technologies would need to adapt concretely to the different farmer types that are found in their working areas. Adapting measures to country's contexts and respective farmers, can play a critical role in bringing down barriers for farmers to efficiently uptake WTT for irrigation—and possibly allow replacing the metaphor “technology transfer”, often used in policies and development programs, with “technology translation” (Garb and Friedlander, 2014)—or even co-creation. Policies and technology packages do not have to be tailor-made on individual scale, but should recognize multiple, relevant types of farmers who make decisions based on different variables. Recognizing this diversity—which can be fruitfully brought forward by Q—and translating it into contextualized support and technology packages, can encourage sustainable and effective farmer development.
The datasets generated/analyzed for this study can be found in the DataverseNL (https://dataverse.nl/dataset.xhtml?persistentId=doi:10.34894/ZUY803) (van Dijk and Intriago Zambrano, 2020).
The studies involving human participants were reviewed and approved by Human Research Ethics Committee of Delft University of Technology. Written informed consent for participation was not required for this study in accordance with the national legislation and the institutional requirements.
RvD: conceptualization, methodology, investigation, formal analysis, writing—original draft, visualization, and project administration. JI: writing—reviewing and editing, data curation, visualization, and supervision. JD and ME: methodology, writing—reviewing and editing, supervision, and funding acquisition. All authors contributed to the article and approved the submitted version.
Field research expenses from RvD were partially covered by the Lamminga Fund. The work of JI was made possible by a grant from the TU Delft | Global Initiative, a program of the Delft University of Technology to boost Science and Technology for Global Development.
We would like to thank the agricultural experts who facilitated the main author's fieldwork activities in Malawi. Our deepest gratitude to all farmers and experts who participated in this Q research for their time and contribution.
The authors declare that the research was conducted in the absence of any commercial or financial relationships that could be construed as a potential conflict of interest.
All claims expressed in this article are solely those of the authors and do not necessarily represent those of their affiliated organizations, or those of the publisher, the editors and the reviewers. Any product that may be evaluated in this article, or claim that may be made by its manufacturer, is not guaranteed or endorsed by the publisher.
The Supplementary Material for this article can be found online at: https://www.frontiersin.org/articles/10.3389/fsufs.2022.954934/full#supplementary-material
WTT, water transport technology; Q, Q-methodology; EV, Eigenvalue; F1, Factor 1; F2, Factor 2; F3, Factor 3; F4, Factor 4.
African Development Fund (2006). Smallholder Crop Production and Marketing Project: Appraisal Report. Available online at: https://projectsportal.afdb.org/dataportal/VProject/show/P-MW-AAC-001.
Aliber, M., and Hall, R. (2012). Support for smallholder farmers in South Africa: challenges of scale and strategy. Dev. South. Afr. 29, 548–562. doi: 10.1080/0376835X.2012.715441
Alvarez, S., Timler, C. J., Michalscheck, M., Paas, W., Descheemaeker, K., Tittonell, P., et al. (2018). Capturing farm diversity with hypothesis-based typologies: An innovative methodological framework for farming system typology development. PLoS ONE 13, e0194757. doi: 10.1371/journal.pone.0194757
Banasick, S. (2019). KADE: A desktop application for Q methodology. J. Open Source Softw. 4, 1360. doi: 10.21105/joss.01360
Brodt, S., Klonsky, K., and Tourte, L. (2006). Farmer goals and management styles: implications for advancing biologically based agriculture. Agric. Syst. 89, 90–105. doi: 10.1016/j.agsy.2005.08.005
Burton, R. J. F., and Wilson, G. A. (2006). Injecting social psychology theory into conceptualisations of agricultural agency: towards a post-productivist farmer self-identity? J. Rural Stud. 22, 95–115. doi: 10.1016/j.jrurstud.2005.07.004
Byanyima, W. (2015). IFAD Lecture: Winnie Byanyima on the Future of Aid. IFAD Invest. Rural People. Available online at: https://www.ifad.org/en/web/latest/video/asset/39003862 (accessed March 12, 2021).
Cammelli, F., Coudel, E., and de Freitas Navegantes Alves, L. (2019). Smallholders' perceptions of fire in the Brazilian Amazon: exploring implications for governance arrangements. Hum. Ecol. 47, 601–612. doi: 10.1007/s10745-019-00096-6
Collinson, M. P., (ed.). (2000). A History of Farming Systems Research. New York, NY: FAO and CABI Publishing.
Coogan, J., and Herrington, N. (2011). Q methodology: an overview. Res. Second. Teach. Educ. 1, 24−28. Available online at: https://www.uel.ac.uk/sites/default/files/7051.pdf
Cousins, B. (2013). “What is a 'smallholder'?: Class-analytic perspectives on small-scale farming and agrarian reform in South Africa,” in Reforming Land and Resource Use in South Africa: Impact on Livelihoods, eds P. Hebinck and C. Shackleton (Routledge), 86–111. Available online at: https://www.routledge.com/Reforming-Land-and-Resource-Use-in-South-Africa-Impact-on-Livelihoods/Hebinck-Shackleton/p/book/9780415746809
Donner, J. C. (2001). “Using Q-Sorts in Participatory Processes: An Introduction to the Methodology,” in Social Analysis: Selected Tools and Techniques (Washington, DC: Social Development Family of the World Bank), 24–49. Available online at: https://documents.worldbank.org/en/publication/documents-reports/documentdetail/568611468763498929/social-analysis-selected-tools-and-techniques
Doss, C. R., Kim, S., Njuki, J., Hillenbrand, E., and Miruka, M. (2014). Women's Individual and Joint Property Ownership: Effects on Household Decisionmaking (April 30, 2014). IFPRI Discussion Paper 01347. Available at SSRN: https://ssrn.com/abstract=2483968
Doss, C. R., and Meinzen-Dick, R. (2015). Collective action within the household: insights from natural resource management. World Dev. 74, 171–183. doi: 10.1016/j.worlddev.2015.05.001
European Commission (2021). The Basic Payment. European Commission. Available online at: https://ec.europa.eu/info/food-farming-fisheries/key-policies/common-agricultural-policy/income-support/basic-payment_en (accessed February 14, 2022).
Fairweather, J. R., and Keating, N. C. (1994). Goals and management styles of New Zealand farmers. Agric. Syst. 44, 181–200. doi: 10.1016/0308-521X(94)90160-H
Fan, S., Brzeska, J., Keyzer, M., and Halsema, A. (2013). From Subsistence to Profit: Transforming Smallholder Farms. Washington, DC: International Food Policy Research Institute (IFPRI). doi: 10.2499/9780896295582
FAO (2015). A Data Portrait of Smallholder Farmers: An Introduction to a Dataset on Small-Scale Agriculture, Vol. 6. Available online at: http://www.fao.org/family-farming/detail/en/c/385074/
FAO (2017). Small family farms data portrait: Basic information document-Methodology and Data Description. Rome. Available online at: http://www.fao.org/fileadmin/user_upload/smallholders_dataportrait/docs/Data_portrait_variables_description_new2.pdf
FEWS NET (2012). MALAWI Food Security Outlook Update. Available online at: https://fews.net/southern-africa/malawi/food-security-outlook-update/december-2012
Garb, Y., and Friedlander, L. (2014). From transfer to translation: using systemic understandings of technology to understand drip irrigation uptake. Agric. Syst. 128, 13–24. doi: 10.1016/j.agsy.2014.04.003
Ghazali, M. H., Shah, S. A., and Mahmood, M. I. (2018). Factor analysis of a novel scoring-based instrument on forecasting Malaysian travelers' behavioral preparedness for travel-related infectious diseases. Int. J. Travel Med. Glob. Heal. 6, 54–63. doi: 10.15171/ijtmgh.2018.11
Gil, J. D. B., Reidsma, P., Giller, K., Todman, L., Whitmore, A., and van Ittersum, M. (2019). Sustainable development goal 2: Improved targets and indicators for agriculture and food security. Ambio 48, 685–698. doi: 10.1007/s13280-018-1101-4
Giordano, M., Barron, J., and Ünver, O. (2019). “Water scarcity and challenges for smallholder agriculture,” in Sustainable Food and Agriculture, eds C. Campanhola and S. Pandey (Rome: FAO; Elsevier), 75–94.
Hammond, J., Rosenblum, N., Breseman, D., Gorman, L., Manners, R., van Wijk, M. T., et al. (2020). Towards actionable farm typologies: Scaling adoption of agricultural inputs in Rwanda. Agric. Syst. 183, 102857. doi: 10.1016/j.agsy.2020.102857
Harrison, E., and Chiroro, C. (2016). Small-Scale Irrigation in Malawi: Challenges and Opportunities, Vol. 4. Available online at: https://cidt.org.uk/wp-content/uploads/2016/02/Malawi-brief.pdf
Hylton, P., Kisby, B., and Goddard, P. (2018). Young people's citizen identities: a Q-methodological analysis of English youth perceptions of citizenship in Britain. Societies 8, 121. doi: 10.3390/soc8040121
Intriago Zambrano, J. C., Michavila, J., Arenas Pinilla, E., Diehl, J. C., and Ertsen, M. W. (2019). Water lifting water: a comprehensive spatiotemporal review on the hydro-powered water pumping technologies. Water 11, 1677. doi: 10.3390/w11081677
Isaga, N. (2018). Access to bank credit by smallholder farmers in Tanzania: a case study. Afrika Focus 31, 241–256. doi: 10.1163/2031356X-03101013
Katongo, K. (1986). Decision Making Behavior of Small Scale Farming Households: The Case of Zambia. MSc thesis, Department of Economics, Iowa State University, Iowa. p. 74. doi: 10.31274/rtd-180813-6518
Kuehne, G., Llewellyn, R., Pannell, D. J., Wilkinson, R., Dolling, P., Ouzman, J., et al. (2017). Predicting farmer uptake of new agricultural practices: a tool for research, extension and policy. Agric. Syst. 156, 115–125. doi: 10.1016/j.agsy.2017.06.007
Llewellyn, R. S., and Brown, B. (2020). Predicting adoption of innovations by farmers: what is different in smallholder agriculture? Appl. Econ. Perspect. Policy 42, 100–112. doi: 10.1002/aepp.13012
Lowder, S. K., Skoet, J., and Raney, T. (2016). The number, size, and distribution of farms, smallholder farms, and family farms worldwide. World Dev. 87, 16–29. doi: 10.1016/j.worlddev.2015.10.041
Lund, P., and Price, R. (1998). The measurement of average farm size. J. Agric. Econ. 49, 100–110. doi: 10.1111/j.1477-9552.1998.tb01254.x
Mason, N. M., and Jayne, T. S. (2013). Fertiliser subsidies and smallholder commercial fertiliser purchases: crowding out, leakage and policy implications for zambia. J. Agric. Econ. 64, 558–582. doi: 10.1111/1477-9552.12025
Matshe, I., and Young, T. (2004). Off-farm labour allocation decisions in small-scale rural households in Zimbabwe. Agric. Econ. 30, 175–186. doi: 10.1111/j.1574-0862.2004.tb00186.x
Montes de Oca Munguia, O., and Llewellyn, R. (2020). The adopters versus the technology: which matters more when predicting or explaining adoption? Appl. Econ. Perspect. Policy 42, 80–91. doi: 10.1002/aepp.13007
Mutenje, M., Kankwamba, H., Mangisonib, J., and Kassie, M. (2016). Agricultural innovations and food security in Malawi: Gender dynamics, institutions and market implications. Technol. Forecast. Soc. Change 103, 240–248. doi: 10.1016/j.techfore.2015.10.004
Norman, D. W. (2002). “The farming systems approach: a historical perspective,” in 17th Symposium of the International Farming Systems Association (Lake Buena Vista, FL), 1–12. Available online at: https://www.researchgate.net/publication/251791709_The_farming_systems_approach_A_historical_perspective
OEC (2020). Malawi. OEC. Available online at: https://oec.world/en/profile/country/mwi (accessed October 5, 2020).
Pannell, D. J., Marshall, G. R., Barr, N., Curtis, A., Vanclay, F., and Wilkinson, R. (2006). Understanding and promoting adoption of conservation practices by rural landholders. Aust. J. Exp. Agric. 46, 1407. doi: 10.1071/EA05037
Pereira, M. A., Fairweather, J. R., Woodford, K. B., and Nuthall, P. L. (2016). Assessing the diversity of values and goals amongst Brazilian commercial-scale progressive beef farmers using Q-methodology. Agric. Syst. 144, 1–8. doi: 10.1016/j.agsy.2016.01.004
Poole, N. (2017). Smallholder Agriculture and Market Participation. Rugby: Practical Action Publishing.
Rapsomanikis, G. (2015). The Economic Lives of Smallholder Farmers: An Analysis Based on Household Data From Nine Countries. FAO. Available online at: http://www.fao.org/family-farming/detail/en/c/385065/
Rose, D. C., Keating, C., and Morris, C. (2018). Understand How to Influence Farmers' Decision-Making Behaviour: A Social Science Literature Review. Warwickshire. Available online at: https://ahdb.org.uk/knowledge-library/understand-how-to-influence-farmers-decision-making-behaviour
Saengavut, V., and Jirasatthumb, N. (2021). Smallholder decision-making process in technology adoption intention: implications for Dipterocarpus alatus in Northeastern Thailand. Heliyon 7, e06633. doi: 10.1016/j.heliyon.2021.e06633
Sarker, M. R., Galdos, M. V., Challinor, A. J., and Hossain, A. (2021). A farming system typology for the adoption of new technology in Bangladesh. Food Energy Secur. 10, 1–18. doi: 10.1002/fes3.287
Scoones, I., Murimbarimba, F., and Mahenehene, J. (2019). Irrigating zimbabwe after land reform: the potential of farmer-led systems. Water Altern. 12, 88–106. Available online at: https://opendocs.ids.ac.uk/opendocs/handle/20.500.12413/14338
Sharma, S., Moerenhout, T., and Tripathi, S. (2015). Rationalizing Energy Subsidies in Agriculture: A Scoping Study of Agricultural Subsidies in Haryana, India. Winnipeg, MB. Available online at: https://www.iisd.org/publications/rationalizing-energy-subsidies-agriculture-scoping-study-agricultural-subsidies
Simons, J. (2013). An introduction to Q methodology. Nurse Res. 20, 28–32. doi: 10.7748/nr2013.01.20.3.28.c9494
SMEC (2015). National Irrigation Master Plan and Investment Framework. Available online at: http://doi.gov.mw/wp-content/uploads/2020/07/Irrigation_Master-Plan-Main_Report.pdf
Thapa, G., and Gaiha, R. (2014). “Smallholder farming in Asia and the Pacific: challenges and opportunities,” in New Directions for Smallholder Agriculture, eds P. Hazell and A. Rahman (Oxford: Oxford University Press), 69–114. Available online at: http://www.oxfordscholarship.com/view/10.1093/acprof:oso/9780199689347.001.0001/acprof-9780199689347
Tittonell, P., Muriuki, A., Shepherd, K. D., Mugendi, D., Kaizzi, K. C., Okeyo, J., et al. (2010). The diversity of rural livelihoods and their influence on soil fertility in agricultural systems of East Africa - a typology of smallholder farms. Agric. Syst. 103, 83–97. doi: 10.1016/j.agsy.2009.10.001
Toma, I., Redman, M., Czekaj, M., Tyran, E., Grivins, M., and Sumane, S. (2021). Small-scale farming and food security - policy perspectives from Central and Eastern Europe. Glob. Food Sec. 29, 100504. doi: 10.1016/j.gfs.2021.100504
United Nations (2020). Goal 2: Zero Hunger. Sustainable Development Goals. Available online at: https://www.un.org/sustainabledevelopment/hunger/ (accessed August 12, 2020).
USDA (2020). USDACensus of Agriculture Historical Archive. USDACensus Agriculture Historical Archive. Available online at: http://agcensus.mannlib.cornell.edu/AgCensus/homepage.do (accessed February 17, 2020).
van der Ploeg, J. D. (1985). Patterns of farming logic, structuration of labour and impact of externalization: changin dairy farming in Northern Italy. Sociol. Ruralis 25, 5–25. doi: 10.1111/j.1467-9523.1985.tb00751.x
van der Ploeg, J. D. (1994). “Styles of farming: an introductory note on concepts and methodology,” in Endogenous Regional Development in Europe: Theory, Method and Practice, eds J. D. van der Ploeg and H. J. de Haan (Luxemburg), 7–30. Available online at: https://library.wur.nl/WebQuery/wurpubs/28143
van Dijk, R. (2020). Unraveling Decision-Making in Irrigated Farming in Malawi: Using Q Methodology to Develop Typologies on Water Transport Technology Adoption. Available online at: http://resolver.tudelft.nl/uuid:e72a4bb1-0aa1-457a-9c29-699937311cba
van Dijk, R., and Intriago Zambrano, J. C. (2020). Replication Data for: Q-Methodology and Farmers' Decision-Making. DataverseNL. Available online at: https://dataverse.nl/privateurl.xhtml?token=4f396f46-3f15-4fdd-9ac6-4c219ab1813e (accessed October 7, 2020).
van Koppen, B., Hope, L., and Colenbrander, W. (2013). Gender Aspects of Small-Scale Private Irrigation in Africa. Colombo: International Water Management Institute.
Vander Vennet, B., Schneider, S., and Dessein, J. (2016). Different farming styles behind the homogenous soy production in southern Brazil. J. Peasant Stud. 43, 396–418. doi: 10.1080/03066150.2014.993319
Walter, G. (1997). Images of success: how Illinois farmers define the successful farmer. Rural Sociol. 62, 48–68. doi: 10.1111/j.1549-0831.1997.tb00644.x
Watts, S., and Stenner, P. (2012). Doing Q Methodological Research: Theory, Method and Interpretation. London: SAGE Publications Ltd.
Wheeler, S. A., Zuo, A., Bjornlund, H., Mdemu, M. V., van Rooyen, A., and Munguambe, P. (2017). An overview of extension use in irrigated agriculture and case studies in south-eastern Africa. Int. J. Water Resour. Dev. 33, 755–769. doi: 10.1080/07900627.2016.1225570
Wigboldus, S., Klerkx, L., Leeuwis, C., Schut, M., Muilerman, S., and Jochemsen, H. (2016). Systemic perspectives on scaling agricultural innovations. A review. Agron. Sustain. Dev. 36, 46. doi: 10.1007/s13593-016-0380-zs
World Bank (2003). Reaching the Rural Poor: A Renewed Strategy for Rural Development. Washington, DC: World Bank. Available online at: http://hdl.handle.net/10986/14084
Yoshizawa, G., Iwase, M., Okumoto, M., Tahara, K., and Takahashi, S. (2016). Q workshop: an application of Q methodology for visualizing, deliberating and learning contrasting perspectives. Int. J. Environ. Sci. Educ. 11, 6277–6302. Available online at: http://www.ijese.net/makale/795.html
Keywords: farmer decision-making, farmer typology, Q-methodology, water transport, technology adoption
Citation: van Dijk R, Intriago Zambrano JC, Diehl JC and Ertsen MW (2022) Q-methodology and farmers' decision-making. Front. Sustain. Food Syst. 6:954934. doi: 10.3389/fsufs.2022.954934
Received: 10 June 2022; Accepted: 26 October 2022;
Published: 14 November 2022.
Edited by:
Yanjun Shen, University of Chinese Academy of Sciences, ChinaReviewed by:
Yiorgos Gadanakis, University of Reading, United KingdomCopyright © 2022 van Dijk, Intriago Zambrano, Diehl and Ertsen. This is an open-access article distributed under the terms of the Creative Commons Attribution License (CC BY). The use, distribution or reproduction in other forums is permitted, provided the original author(s) and the copyright owner(s) are credited and that the original publication in this journal is cited, in accordance with accepted academic practice. No use, distribution or reproduction is permitted which does not comply with these terms.
*Correspondence: Juan Carlo Intriago Zambrano, ai5jLmludHJpYWdvemFtYnJhbm9AdHVkZWxmdC5ubA==
Disclaimer: All claims expressed in this article are solely those of the authors and do not necessarily represent those of their affiliated organizations, or those of the publisher, the editors and the reviewers. Any product that may be evaluated in this article or claim that may be made by its manufacturer is not guaranteed or endorsed by the publisher.
Research integrity at Frontiers
Learn more about the work of our research integrity team to safeguard the quality of each article we publish.