- 1Department of Agricultural Economics, Ethiopian Institute of Agricultural Research (EIAR), Addis Ababa, Ethiopia
- 2Socioeconomics Department, The International Maize and Wheat Improvement Center (CIMMYT), Nairobi, Kenya
- 3Department of Rural Development and Agricultural Extension, Haramaya University, Haramaya, Ethiopia
Given the challenges brought about by the increasing frequency of climatic stressors (droughts) and other biotic challenges (pests and diseases), breeding for tolerance to these traits is now seen as an indispensable adjunct to the enhancement of yield potential. Drought tolerant (DT) maize varieties that do well under moderate drought and outperform (or do not underperform) commercial checks under normal rainfall are becoming available. This study examines the role of these maize varieties in mitigating the effects of drought on maize yields in drought-prone areas of eastern Uganda. We estimate the causal impact of these new generations of maize varieties using a multinomial endogenous switching regression treatment effect framework. The average treatment effects of adopting DT maize show that farmers who actually cultivated DT maize achieve 30% more yield than what they would have obtained with non-DT hybrids. Similarly, average treatment effects on the untreated, revealed that farmers who grew non-DT modern and local maize would have 32 and 54% more yield, respectively, if they instead had adopted DT maize. While being superior to all other maize seeds, the magnitudes of the benefits of DT maize varieties were more pronounced in areas with comparatively less rainfall amount providing strong evidence that the yield potential of these varieties is stable across space and a wide range of rainfall conditions. If the genetic gains of these varieties can be secured over the long term, their impacts in improving the resilience of maize farming systems are likely to be considerably large and favorable.
Introduction
Achieving food security and related goals cannot happen without considerable enhancement of agricultural productivity through high yielding crop cultivars. The enhancement of yield potential has been the cornerstone of maize breeding programs for decades and justifiably so (Bänziger et al., 2006; Cairns et al., 2013). Yet, given the challenges brought about by the increasing frequency of climatic stressors (droughts) and other biotic challenges (pests and diseases), breeding for tolerance to these traits is now seen as an indispensable adjunct to the enhancement of yield potential. High-yielding varieties that are susceptible to droughts, pests, or diseases are not likely to impart the needed resilience to the millions of African families who depend on staple crop production under precarious economic and climatic conditions.
Ongoing climatic changes have exceeded farmers' existing adaptive capacities and experiences (Fisher and Carr, 2015). Traditional low-cost practices, such as shifting planting dates, changing crop species, or switching between existing crop varieties, used by African farmers may no longer be adequate to mitigate the negative impacts of weather variability (see Fisher et al., 2015). Projections on the impact of climate change in SSA suggest that in the absence of more climate-resilient crops, drought-induced constraints will not only decrease yield but also amplify the rate at which yield loss will happen (Li et al., 2009; Cairns et al., 2013). Conceivably, drought-related challenges will continue to threaten the region's prospect of achieving food security.
About 40% of the maize area in Africa faces occasional drought stress that may lead to yield losses of about 10–25%. Nearly, a quarter of the maize crop suffers from frequent drought which involves losses extending up to half of the potential harvest (Fisher et al., 2015). Likewise, in Uganda, maize production is predominantly rain fed and, therefore, vulnerable to weather and climatic risks such as extreme temperature and drought [Hartmann et al., 2013; Intergovernmental Panel on Climate Change (IPCC), 2014]. Improving maize production and productivity thus requires dealing with these challenges, not least the threats of drought-induced yield losses or even crop failure (Twinomugisha, 2005; Ekiyar et al., 2010; Nabikolo et al., 2012). Such risk is notable; particularly, because maize is most susceptible to drought stress occurring at the flowering and grain-filling stage which can cause barrenness and serious yield degradation (Magorokosho et al., 2009).
Maize is an important crop among Uganda's staple crop system. It is cultivated by at least 86% of smallholder farmers across all agro-ecologies [United States Agency for International Development (USAID), 2010; Uganda Bureau of Statistics (UBOS), 2013], shared 63% of the area planted to cereals, ranked third after plantain and cassava in average daily calories intake, and a major source of income for most farmers in eastern, northern, and north-western Uganda [Ferris et al., 2008; United States Agency for International Development (USAID), 2010]. Maize productivity in Uganda is three to four times less than the potential [Ministry of Agriculture Animal Industry and Fishery (MAAIF), 2011; Ahmed, 2012]. The overall trend of production, area, and yield shows that yield has either stagnated or declined, and the growth in maize production has primarily been due to area expansion [Uganda Bureau of Statistics (UBOS), 2013]. Efforts at developing and mainstreaming new drought-tolerant varieties are important to ensure resilient maize production systems in the decades to come.
New maize varieties that can withstand drought and achieve yield parity with legacy varieties under normal rainfall conditions offer farmers greater flexibility in adapting to these changes (Lobell et al., 2008; Lybbert and Sumner, 2012). In the last decade or so, the International Maize and Wheat Improvement Center (CIMMYT) has facilitated the development of over 200 such maize varieties. The Drought Tolerant Maize for Africa (DTMA) was such a project.1
Multi-location on-farm trials conducted in eastern and southern Africa by CIMMYT scientists in conjunction with those from the International Institute of Tropical Agriculture (IITA) and national research institutes in 13 SSA countries have demonstrated the strong agronomic performance of these new drought tolerant (DT) maize seeds. The evidence from these trials suggests that these new generations of DT maize varieties can out-yield commercial hybrid checks by 83–137% (controlled drought), 26–47% (random drought), and 25–56% (under optimal rainfall conditions) (Fisher et al., 2015). Additionally, an ex-ante impact assessment suggested that wider adoption of DT maize varieties developed through DTMA can generate US$ 532 million of increased maize grain value under conservative yield improvement. These maize seeds are expected to reduce not only the chances of drought-related harvest failure but also harmful post-failure coping strategies like reducing food consumption, selling assets, or withdrawing children from school (La Rovere et al., 2014).
While researcher-managed trials have largely confirmed that the DT varieties have superior yield advantage, especially, when “all states of nature” (normal rainfall and moderate drought conditions) are considered, the realization of these benefits on farmers' fields under their own management and resource conditions is another matter. It is only when farmers can observe these benefits for themselves under their own growing conditions will they sustainably adopt these varieties. Although the ex-ante assessments based on simulated yields predicted positive impacts of using these varieties on yield potential, food security, and household income, these results remain to be replicated by data retrieved from farmers' fields. The most recent adoption studies (such as those by Tambo and Abdoulaye, 2013; Fisher and Carr, 2015; Fisher et al., 2015) that looked into the level and determinants of DT maize seed adoption, generally did not address the incremental yield or economic impacts of these seeds under farmers' conditions.
This study is designed to offer evidence of the impact of the new DT varieties in reducing susceptibility (potential harm) due to drought in the form of yield penalty based on farmers' own production data to determine if the promise of these varieties is being realized on-farm, i.e., under growing conditions as set by farmers. An important contribution to this study is that our analysis uses plot-level survey data collected from 34 villages in Uganda to examine, for the first time to the best of our knowledge, the causal effect of cultivating DT maize seed on the productivity of maize farmers operating in drought-prone areas of eastern Uganda. The study further evaluates if the cultivation of DT maize had meaningfully larger per unit production of maize when compared to other commercial maize varieties developed for other traits than drought. Finally, using locally observed rainfall data, we spatially correlate the impacts of DT with observed rainfall conditions. This was important to determine the impacts of DT varieties both under adequate and less adequate rainfall. The yield stabilization effect of DT varieties is their key advantage. This study helps to confirm this using farmer survey data as an important check on on-station or researcher managed trials.
Materials and methods
Data and description of study area
Data for the present study came from a household survey conducted in Uganda between June and August 2014. The geographical focus was eastern Uganda, where the DT maize seed dissemination activities have been concentrated. The region constitutes 32 districts lying over an area of 39,478.8 hectares covering about 16% of the total area of Uganda. The elevation of the area ranges between 1,075 and 1,524 m above sea level. The region's population is estimated at 9,154,960 of which about 90% lives in rural area. The average household size for the region is about 4.9 vs. 4.7 for the country as a whole [Uganda Bureau of Statistics (UBOS), 2014]. The mean annual rainfall varies from 1,374 to 2,058 mm with a range between 895 and 3,001 mm. The rainfall exhibits significant annual and seasonal variation in the amount and distributional pattern (Kansiime et al., 2013). The region has three distinct agroecological zones (AEZs) viz., Lake Victoria Crescent; Southern and Eastern Lake Kyoga basin; and Mount Elgon high farmlands. These AEZs also capture variability in altitude, soil productivity, cropping system, livestock systems, and land use intensity. Agriculture is the main source of livelihood in the region. Crop production is dominant. The major crops produced in the region are finger millet, maize, rice, sweet potato, and cassava. The region accounted for about 38% of the total maize area and half of the total maize produced in Uganda in 2008/09 [Uganda Bureau of Statistics (UBOS), 2012]. Important livestock includes cattle, small ruminants (goats and sheep), pigs, and poultry (chicken, ducks, turkey). While about 57% of agricultural households face food shortages in certain periods of the year, the Eastern region was reported to have the highest percentage (30%) and the least was for the central region (17%). Households with access to credit are low both at the national (10%) and in the Eastern region (9%). From 31% of the agricultural households using improved seed, the region has the highest share (43.7%) vs. the lowest in the Western region (16.6%) [Uganda Bureau of Statistics (UBOS), 2010]. The Eastern region is subject to the vagaries of climate variability. The effect of climate variability is assumed to be complicated by poorly developed economic and social services and infrastructures, and severe poverty status in the region. Generally, the region is characterized by a combination of acute poverty, vulnerability to drought, floods, landslides, and natural resource degradation (Kansiime et al., 2013).
Unlike many cross-sectional data sets, the current data has information on the spatial heterogeneity of selected districts which we use as a proxy for the temporal dimension of rainfall variability. Multistage sampling was employed to identify sample households for the study. In the first stage, three districts were purposively selected from Lake Victoria Crescent agroecological zones (LVC AEZs) of the eastern region where drought risk is more likely and where the DT maize seeds are disseminated. The LVC AEZ is one of the three distinct AEZs in the eastern region of Uganda which includes the southern and eastern Lake Kyoga basin and Mount Elegon high farmlands (Figure 1). These AEZs are mainly differentiated by the amount of rainfall. According to 40-year (1971–2010) observational rainfall data for the region, there is significant inter-annual rainfall variation within and across AEZs. Particularly, the long-term data show that the LVC exhibits significant intra- and inter-seasonal rainfall variations (Kansiime et al., 2013). The sample districts (Iganga, Tororo, and Bulambuli) are geographically well spread across the LVC AEZ (see Figure 1). Accordingly, they are assumed to account for the spatial heterogeneity as well as the temporal rainfall variability within this AEZ. In the second stage, we used probability proportional to size sampling to select a total of 34 out of 3,119 villages from the study districts. Details of the number of households in each village were acquired from the 2012 Uganda population and housing census of enumeration areas by the Uganda Bureau of Statistics (UBOS). Finally, from each sampled village, 12 households were selected for interview using a simple random sampling technique. The interview involved 696 individuals (householders and their spouses) drawn from a total of 408 sampled households.
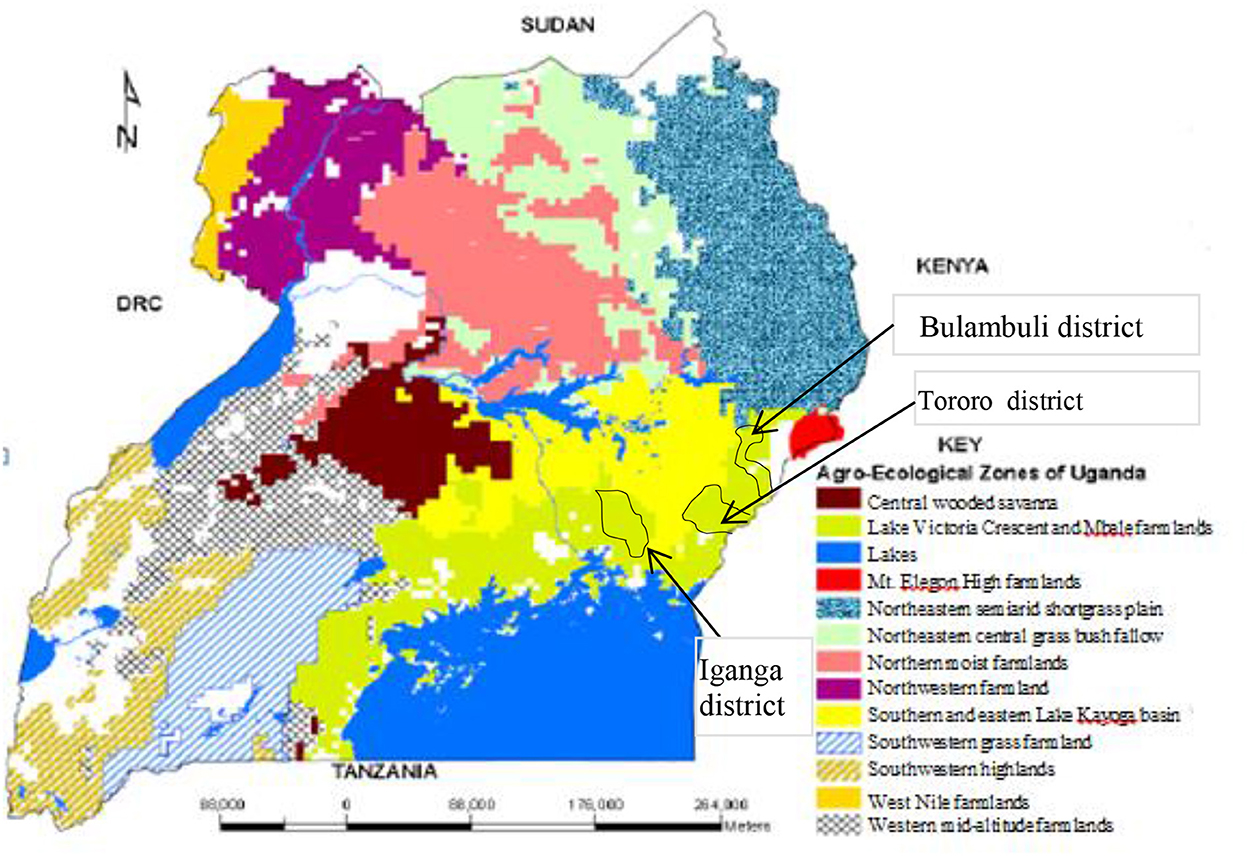
Figure 1. Agro-ecological zones of Uganda, and location of sampled districts. Source: Wasige (2009).
Both household and plot-level data were collected through face-to-face interviews using two structured questionnaires, viz., a household and an individual questionnaire. A village questionnaire was also used to interview key informants [including extension workers, local council chairpersons (an elected head of the village- the lowest administrative level), progressive farmers, and local opinion leaders] regarding village-level variables such as input/output prices, distance to markets, and subjective assessments of rainfall patterns.
The survey covered plot-level information where for each plot, the respondent recounted the names and details of maize varieties cultivated during the 2013/2014 production year. Other plot-level data collected included slope, soil fertility, plot size, irrigation access, plot tenure, erosion incidence, crop production estimates, and input use. Important socioeconomic and demographic variables collected were age (number of the year lived), gender, education (number of school years), family size (number of household members), access to extension service (dummy variable for the source of information), the likelihood of getting credit (dummy variable), and social capital (an index calculated based on the number of groups the respondent is a member out of a list of selected group related to agricultural activity). Moreover, maize network size was defined as the number of other farmers the respondent regularly talks to get information regarding maize farming, the number of progressive farmers in the respondent's village, the number of DT maize seeds known to the respondent, and sources of information on new maize seed were also retrieved from the respondents.2
We collected data on drought shocks based on the number of times the farmer could remember having encountered drought and drought-induced maize harvest loss in the previous 5 years prior to the date of the interview (i.e., 2010–2014). Experiencing frequent episodes of drought may influence farmers' decisions to cultivate DT or other types of maize that withstand droughts and help minimize yield losses.
Data related to rainfall patterns were collected from village key informants based on their subjective assessment of the timeliness, amount, and distribution in the major growing season immediately prior to the date of the survey. We considered farmers' perception of the timeliness (onset and cession), adequacy (whether the amount received is enough to support maize production), and distribution [based on rainfall availability at critical growth stages and extent of dry-spell (based on farmers' estimate of the time interval between any rainy day and the next rainy day) experienced] of rainfall to construct an index variable for the adequacy of rainfall or lack thereof. Perceived responses to each of these items (as “yes” or “no”) were coded as favorable and unfavorable rainfall outcomes and averaged over the number of questions. The index values range from zero to one where one stands for a favorable outcome and zero for the worst. While farmers' decision to cultivate a particular maize seed (i.e., DT or non-DT maize seed) may depend on their expectations regarding rainfall pattern, their perception of the actual rainfall3 pattern may help to explain the performance of a particular type of maize cultivated (Teklewold et al., 2013).
Also, the survey involved the administration of a risk elicitation experiment to measure farmers' risk preferences (risk-taking behavior) using Gneezy and Potters (1997) risk elicitation mechanism. The experiment provided a hypothetical situation that elicits the risk preference of farmers based on actual pay-offs made following: (1) respondents' choice from the combination of high and no-risk maize seeds and (2) probabilistic determination of weather conditions (favorable or unfavorable outcomes) which is based on the toss of a coin. Farmers' risk-taking behavior is important in explaining decisions regarding the cultivation of new maize varieties as a potential adaptation strategy. Although DT maize seeds are developed to deal with drought risk, a decision to switch to new unfamiliar varieties may involve some risk, the potential benefits notwithstanding. Farmers who are more vulnerable to extreme weather events are less likely to use improved varieties as an effective means to cope with drought; instead, they tend to delay it (Cavatassi et al., 2011; Liu, 2013). In this study, we expect that farmers who are averse to risk could decide to cultivate DT maize if their belief in drought tolerance of the variety outweighs the potential risk associated with its being new.
Given that the DT maize seeds are developed for drought tolerance and yield, farmers who have a preference for these two traits are expected to cultivate these varieties. Farmers' preferences for these traits are captured based on whether he/she mentioned them among his/her most preferred traits of maize variety. Social capital may exert behavioral influences in various ways (that is, information sharing, positive externality, resource sharing, and relaxing liquidity constraints through informal credit). The possible roles of farmers' social capital in the decision to grow DT maize are captured using the social capital index constructed based on group membership, roles assumed in each group, the nature of the group composition (homogeneous or diverse), and its functionality.
Conceptual framework
The adoption of new technology is often modeled as a utility-maximizing choice between two or more alternatives. It can be influenced by the characteristic features embodied in the technology and many other factors. Observed adoption choice of agricultural technology (for example, modern crop varieties) is hypothesized to be the end result of a process of preference comparisons by farmers.
In this study, adoption is defined as the reported use of DT maize variety (that is when the farmer exactly mentions the variety by name) on a specific plot managed by the head or spouse of a household during the 2013/14 growing season. Adopting the utility maximization concept, a maize farmer (also referred to as plot manager4)—who has to make decisions regarding what type of maize to grow- has three possible alternatives, which are DT modern maize, non-DT modern maize, and “unimproved” or local maize seed.5 Each one of these categories of choice includes a distinct list of maize varieties. Plots are identified with the type of maize varieties cultivated by matching the name of the varieties reported by farmers with the list under each category. The DT maize seeds are mainly developed for drought tolerance and yield whereas the non-DT modern maize seeds are developed for traits other than drought (mostly higher yields under optimal conditions). The actual choice is assumed to be made based on farmers' utility derived from the adoption of one of these maize varieties.
Farmers' decisions to adopt a particular maize seed are assumed to be driven by maximization of the utility derived from the alternative types of maize seeds. For farmer i to choose any maize type, j, from available alternatives, m, it is required that Uij > Uim, m ≠ j; the expected benefit, Uij, that a farmer derives from the adoption of maize type j (that is, DT, non-DT modern, or local maize) is a latent variable determined by observed household, individual, plot, and location characteristics (Xi), and unobserved characteristics (eij):
Where Xi is a vector of observed exogenous variables; βj is a vector of parameters to be estimated for each type of maize; and eij are unobserved characteristics. Let the choice from among the alternative maize types by a farmer is denoted by an index variable, I, such that:
Equation (2) implies that ith farmer will adopt modern maize type j to maximize his/her expected gain (ηij) if j provides greater benefit than any other alternative type of maize m; that is, if (Bourguignon et al., 2007).
Assuming that eij are independently and identically Gumbel distributed, the probability that farmer i with characteristics X will choose maize type j can be specified by a multinomial logit (MNL) model (McFadden, 1973):
Since MNL is a model where regressors do not vary over choices, coefficients are estimated for any choice. MNL requires identification, thus one of the choices of m maize types (the local maize seed in our case) is treated as the base category (correspondent βm is constrained to equal 0).
Based on the adoption literature, farmers' decisions to adopt a new agricultural technology depend, among others, on socioeconomic, demographic, and institutional factors. Hence, the choice of explanatory variables in this study is made based on a review of past studies on technology adoption and impact in developing countries (these include Feder et al., 1985; Bandiera, 2006; Deressa et al., 2009; Matuschke and Qaim, 2009; Kafle, 2010; Kassie et al., 2011; Asfaw et al., 2012; Teklewold et al., 2013; Fisher and Carr, 2015; Jain et al., 2015). This body of literature indicates that many factors influence adoption and thus affect our outcome variable. The factors are categorized as demographic and farmer characteristics (family size, gender, age, education, risk attitude, preference to drought tolerance, preference to yield, drought risk perception, experience of maize loss due to drought), social and institutional characteristics (social capital, number of progressive farmers in the village, main sources of information, credit access, distance to input market, distance to extension office), maize plot characteristics (plot size, tenure, extent of erosion, soil fertility, slope, irrigation), and geographic characteristics (captured through district dummies).
A fundamental problem when comparing adoption outcomes between individuals is that adoption is not randomly assigned- farmers endogenously select themselves into adopters or non-adopters of particular technologies. So decisions are likely to be influenced by both observed and unobserved characteristics. Unobserved factors that can influence adoption include aptitude, motivation, experience, or other factors, which are not readily observed in the present data. These “other” factors may be correlated with the adoption outcome. As adopters and non-adopters can be systematically different, estimating the effect of adoption without accounting for this implied endogeneity problem would lead to inaccurate results where outcomes are attributed to adoption when in fact significant aspects of the outcome are the result of other factors not accounted for. We apply an endogenous switching regression treatment effects approach to correct for self-selection bias (Dubin and McFadden, 1984) and provide treatment effects that take both observed and unobserved factors into account.
Econometric estimation strategy
The endogenous switching regression (ESR) framework we used to estimate the impact of adoption while accounting for selection biases involves two stages. In the first stage, farmers' choices of maize types are modeled using the MNL selection model as specified above; and in the second stage of the estimation, the impact of adoption on the outcome variable is evaluated using ordinary least squares (OLS) which includes a selectivity correction term from the first stage.6
The relationship between the outcome variable and the set of exogenous variables Z is estimated for the maize type chosen. The outcome equation for each possible regime j is given as
Where Yij are the outcome variables of the ith farmer in regime j, Z is as defined above, and the error terms (u) are distributed with E(uij|X,Z) = 0 and var . For consistent estimation, Equation (4) will be augmented with plot-specific unobservable characteristics such as land quality that can help in controlling for unobserved heterogeneity among plots. Yij is observed if and only if alternative j is adopted which occurs based on the utility maximization indicated earlier. If the error terms of the selection equation (e) and that of the outcome equations (u) are correlated, unbiased estimation of Equation (4) requires the inclusion of the selection correction terms of the alternative choices as recovered from the MNL models. Bourguignon et al. (2007) show that consistent estimates of the parameter in the above equation can be obtained by estimating the following multinomial endogenous switching regression models:
Where θj is the covariance between e's and u's; and λj is the inverse Mills ratio (IMR) computed from the estimated probabilities in Equation (3) as follows: ; ρ is the correlation coefficient of e's of the selection equation and u's of the outcome equations, and ω represents error terms with an expected value of zero. In the multinomial choice setting, there are J-1 selection correction terms, one for each alternative maize type. The standard errors in Equation (5) are bootstrapped to account for the heteroscedasticity arising from the generated regressor (λj).
For Equation (5) to be identified, it is good practice in empirical analysis to use variables that affect the choice decision but not the outcome variable (as exclusion restrictions) in addition to those automatically generated by the non-linearity of the selection regression. The specification chosen for the outcome equations in Equation (5) follows the common practice in the agricultural economics literature (see, e.g., Solis et al., 2007; Di Falco et al., 2011; Teklewold et al., 2013), allows us to use variables related to information sources, farmer and farm household's characteristics as well as including the number of DT maize varieties known to the farmer as exclusion restriction. The validity of the exclusion restriction is tested based on the significance of these variables in the selection model but not in the outcome equation.
Using the above framework, the average treatment effect on the treated (ATT) which is the average effect of adoption for adopters (DT maize growers), and on the untreated (ATU) which is the average effect of adoption for non-adopters, are both examined by comparing the expected actual and counterfactual outcomes. The computation of the counterfactual and average treatment effects using endogenous switching regression is implemented in the same manner as in Di Falco et al. (2011), Teklewold et al. (2013), and Kassie et al. (2014).
Actual outcomes for
Counterfactual outcomes for
While Equations (6) and (7) represent the actual outcome observed in the sample for adopters and non-adopters, respectively, Equations (8) and (9) are the respective counterfactual outcomes. These conditional expectations are used to compute ATT and ATU. The average adoption effect for adopters (ATT) is calculated as the difference between Equations (6) and (8). The difference between Equations (7) and (9) provides the average adoption effect for non-adopters (ATU). ATT, for example, is shown as follows:
The first term on the right-hand side (RHS) of Equation (10) represents the expected change in adopters' average outcome if adopters' characteristics had the same return (coefficient) as the characteristics of non-adopters. In the second term (on the RHS), λj, is the selection correction term that captures all potential effects of difference in unobserved variables.
The average treatment effect (ATE) can be calculated by taking the difference between the two actual outcomes (Equations 6, 7). In observational studies, ATE gives the treatment effect without accounting for selection bias. The computation of the conditional expectations and treatment effect are summarized in Table 1.
Results and discussions
Descriptive summary
About 77% of the sampled respondents grew modern maize varieties of which 24% used DT maize. The average size of all maize plots was 0.40 hectares; the corresponding figure for plots allocated to all modern and only to DT maize seed was 0.41 and 0.45 hectares, respectively. DT growers tend to cultivate maize on relatively larger plots. The share of maize plots planted to DT maize was about 19%. Comparatively, non-DT modern maize seeds were widely cultivated covering 60% of the maize plots of the sample households. These maize seeds have been part of the seed systems in Uganda since the 1960s (Balirwa, 1992) and their spread might imply that over time the DT maize would also follow similar diffusion trends. At the time of this study, the uptake level of DT maize suggests that it is still at the early diffusion stages (Figure 2).
Table 2 presents descriptive statistics of variables that are used in the empirical models (selection and outcome equation) applied to make casual attribution of adoption of DT maize.
Results from the adoption models
Table 3 presents the estimation results of the first stage (the MNL selection model) of the endogenous switching regression. From the three types of maize used as the categorical dependent variable, local maize is used as the base category. The tests of goodness-of-fit reported at the bottom of the table show the model fits the data reasonably well. The Wald χ2 test statistics (672.26) rejects the hypothesis that the regression coefficients of the explanatory variables are jointly equal to zero (p = 0.00). Accordingly, the result showed that the gender of plot managers, size of the maize plot, preference for the drought tolerance trait, number of DT varieties known to the plot manager, source of information on new maize seed and location dummies, as expected, influenced the probability of adopting DT maize varieties. Male-headed plot managers have a higher adoption probability than their female counterparts.
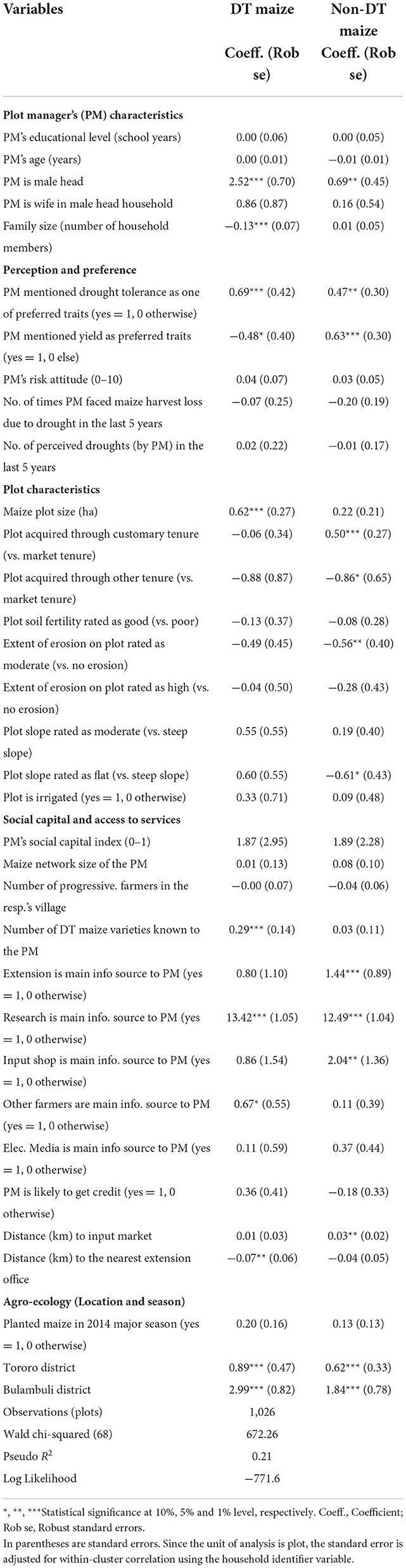
Table 3. Parameter estimates of the determinants of DT and non-DT maize adoption-multinomial logit selection model (Local maize as base category).
Also, plot managers who receive information about new maize seed varieties from research centers and other farmers, are more likely to cultivate DT maize. Research can provide reliable technical information, but it may not be accessible to many farmers mainly due to limited presence in terms of geographical spread. As expected proximity to the nearest extension service increases the chances of adoption.
Farmers who have a preference for DT traits are expected to cultivate DT maize. The results indicate that compared to the base category, preference for drought tolerance is an important driver for the adoption of DT varieties. This suggests that those who grow DT seeds are well-informed about this unique trait. Moreover, these DT varieties maintain (to a large degree) yield parity with extant hybrids. It stands to reason then that preference for DT trait would be the main differentiation for many farmers in evaluating DT varieties compared to non-DT ones.
Finally, the positive correlation of some location dummies with adoption could be a reflection of agro-ecological factors (like rainfall) as well as other underlying unobserved spatial differences. The reference district (Iganga) received relatively better rainfall (in amount and distribution) over the main growing season (March–June; see Table A1). Comparatively, low rainfall signal at planting time (March) might have prompted a higher likelihood of growing DT maize seeds in Bulambuli and Tororo than in Iganga.
Plot level impacts of DT maize
The results presented in this section are based on the conditional and unconditional average effects of DT maize adoption on expected maize yield. The ATT and ATU are computed using the predicted value of yield following the schema presented in Table 1.
The coefficient estimates from the second stage regression (OLS) are presented in Table A2. It is to be noted that the coefficients on selection correction terms are almost not significant suggesting that our results are unlikely to be driven by selection bias. The regression estimates without the correction terms remain similar but in the interests of brevity, we have not reported the results.
The figures reported in Table 4 are expected values of yield. A simple pairwise comparison of means (ATE) indicates that cultivation of DT maize, on average, gives higher maize yield to adopters than non-adopters who grew either non-DT or local maize. Such comparisons, however, could be misleading as it does not control for observed and unobserved factors that may influence the outcome variable.
We used Equation (10) to estimate the true average adoption effect and compare the expected yield of farmers cultivating DT maize with their counterfactual outcome- if the same farmers had instead cultivated local or non-DT maize seed. The results indicated that both adopters and non-adopters would benefit from adoption. The magnitude of the adoption effect, however, differs when the comparison is between DT vs. non-DT and DT vs. local maize growers. The ATT showed that farmers who actually cultivated DT maize get 30% more yield than what they would have obtained had they instead adopted non-DT maize seed. The corresponding yield effects of adopting DT instead of local maize were four times high. Similarly, ATU revealed that farmers who grew non-DT modern and local maize received 32% and 54% more yield, respectively, if they instead had adopted DT maize. That is, non-adopters would have realized higher productivity if they decide to switch to DT maize.
Spatial variation of the impacts of DT maize
We examined the treatment effect both by location (districts) and rainfall status, the latter was determined using the rainfall index (as described in the data section). Table 5 presents the ATT and ATU of DT adoption against non-DT and local maize by the district. In all the districts, the average yield of DT outperformed that of non-DT and local maize. As would be expected, the magnitude of the ATT was relatively higher against local varieties than non-DT maize varieties. The ATT against local maize indicates a yield advantage ranging from 2.6 times at Tororo to six times at Iganga. The corresponding yield advantage for ATU ranged from 0.5 to 1.5 times more at Tororo and Bulambuli, respectively. The ATT against non-DT growers shows a yield advantage of 52% at Iganga, 18% at Tororo, and 17% at Bulambuli. The ATU (for DT vs. non-DT) had a relatively better yield advantage than the ATT in all the districts suggesting that the adoption of DT would have been even more beneficial to those who grew non-DT (had they grown DT) than those who already adopted DT.
According to the rainfall records from proximate weather stations (Table A1), the amount of rainfall received by each of the study districts during the 2014 main season covered by the survey (that is, March–June 2014) was well below the long-term average (that is, 659 mm) reported by Kansiime et al. (2013). In relative terms, the Iganga district received a higher amount with better distribution during the main season of 2014. Theoretically, the treatment effect should have been pronounced more in the Bulambuli district where the amount and distribution of rainfall received during the surveyed season was relatively less favorable. But this might be an indication that district-level rainfall data adopted from the meteorological stations are less representative of the rainfall distribution under more granular scale conditions at the micro level, given the low density of weather stations in Uganda.
To mitigate this, we tracked rainfall conditions/patterns at a lower level using the rainfall index constructed for each village. The index is then used to categorize the villages into those which had potentially poor rainfall status (with a rainfall index value equal to or < 0.5) and those which had potentially good rainfall status (with an index value greater than 0.5) in the 2014 main season.
Table 6 shows the treatment effect by rainfall status of sample villages. In all cases, be it under poor or good rainfall conditions, DT adoption makes the productivity of adopters (ATT) and non-adopters (ATU) better off. Leaving the substantial yield advantage of DT maize adoption over local maize under the two states of rainfall aside, the treatment effect over non-DT reveals an interesting result. The average treatment effect (ATT) over non-DT maize under poor rainfall conditions was about 417 kg ha−1, that is, cultivation of DT maize offers a yield advantage of 44% over what would have been obtained if the plot was instead planted to non-DT maize. The corresponding ATT under good rainfall conditions has an additional yield advantage of about 21%. This supports the claim that DT maize outperforms other commercial maize (non-DT) more in seasons when the rainfall condition is less favorable.
Despite its inability to account for confounding effects, we further ran ANOVA of the linear prediction of DT yield as a partial metric to compare its performance difference across the three districts. The result suggests a significant (p = 0.00) productivity difference in mean DT maize yield across the three districts. Pairwise yield comparisons between these districts further revealed statistically meaningful differences (Table 7). Bulambuli district- where the highest share (40%) of plots was planted to DT maize varieties- had the highest mean DT yield, and Tororo district had the least.
To help in visualizing the results described above, we summarized these spatial variations in the impact of DT in Figure 3 based on the ATT comparing DT with local maize varieties (Figure 3A) and DT with non-DT maize varieties (Figure 3B). Although the mean ATT is positive and significant across all districts, there are pockets where the impact as measured by ATT was below the sample average. This can be seen in the Tororo district where both the magnitude of the impact is relatively lower (see Tables 5, 6), and the DT coefficient on the district dummy is comparatively smaller (see Table A2). Further examination of the particularities of the Tororo district might be worth looking at to bring out and learn about the underlying causes.
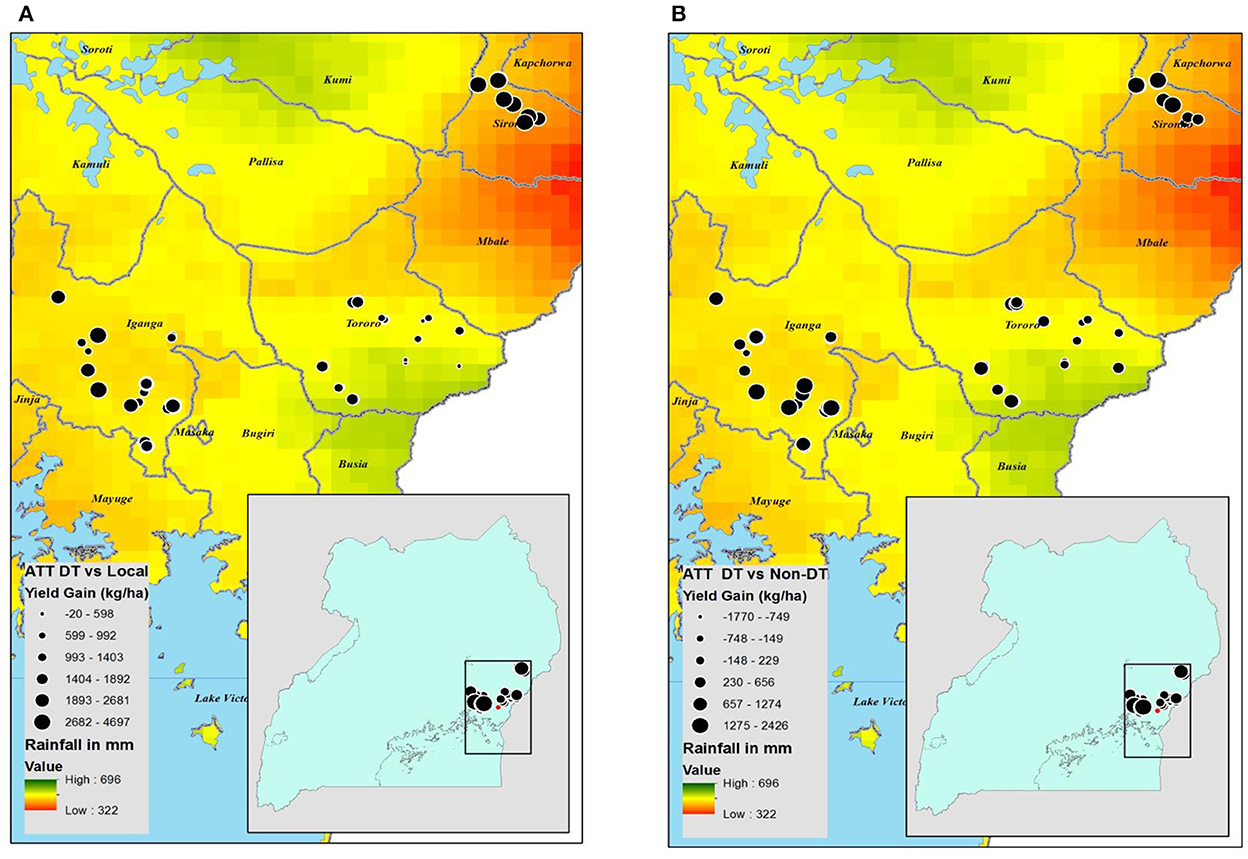
Figure 3. (A) Spatial distribution of ATT (average treatment effects) of DT maize compared to local varieties. (B) Spatial distribution of ATT (average treatment effects) of DT maize compared to all non-DT varieties.
Discussion
This study evaluates the potential impact of maize varieties developed for drought tolerance. These varieties are said to stabilize maize yield under drought conditions thereby offering maize farmers living in drought-prone areas a greater possibility to adapt to climate change. The study utilizes cross-sectional household and plot-level data collected from 696 plot managers of randomly selected 408 sample households. The finding of this study revealed that compared to plots planted to non-DT/local maize varieties, those planted to DT maize had superior yield performance both across locations and under varying rainfall conditions. Particularly, putting the superior yield performance of DT over non-DT maize varieties in favorable rainfall conditions aside, the more pronounced yield performance observed when the rainfall conditions were less favorable provides evidence that the yield potential of these varieties is stable across space and a wide range of rainfall conditions. Irrespective of locations and rainfall conditions, the magnitude of the yield impact of cultivating DT maize over non-DT maize, which is about 30% larger, is consistent with the previously reported experimental results which showed that DTs offer a yield advantage of 26–47% (random drought), and 25–56% (under optimal rainfall conditions) over commercial (non-DT modern) maize seeds (Fisher et al., 2015). As expected, the corresponding ATT (yield effect of adopting DT instead of local maize) was found many times larger. Likewise, it is not only the farmers who already started cultivating DT maize who reap the benefit but also farmers who grew non-DT modern and local maize would have potentially received 32 and 54% more yield benefits, respectively, if they instead had adopted DT maize. That is, non-adopters would have realized higher productivity if they decide to switch to DT maize. The results generally confirm the direct role and prospect of DT maize adoption in reducing susceptibility to drought, thereby improving food security and welfare status of maize farm households- as higher yields are likely to translate to a greater household food supply, sellable surplus, and better crop income, all else equal.
Farmers' decision to cultivate DT maize tends to follow rainfall signals. Spatial differences observed in technology adoption across districts are partly the results of variable rainfall on-set signals across sample districts. Teklewold et al. (2013) and Kassie et al. (2014) also observed spatial differences in technology adoption induced by various factors. The DT impacts observed in each of the districts showed that the adoption of DT would have been even more beneficial to those who grew non-DT (had they grown DT) than those who already adopted DT. This implies that those not currently using DT maize would greatly benefit from them once the factors preventing these farmers from adopting them are removed.
The impact analysis based on the rainfall condition indicated that cultivation of DT maize offers more yield advantage over non-DT maize during relatively poor rainfall conditions than a favorable one (44 vs. 21%). This supports the claim that DT maize outperforms other commercial maize (non-DT) more in seasons when the rainfall condition is less favorable. In fact, the DT maize varieties are meant to ensure stable yield across variable rainfall conditions.
The fact that DT maize seeds are cultivated by only about a fifth of the sample households is suggestive of an initial diffusion cycle. The relatively wider cultivation of other commercial non-DT maize varieties which had long been in the seed system suggests that over time the DT maize would follow a similar trend as most of the barriers would be removed. In addition, a higher potential benefit for non-adopters should they cultivate DT maize suggest the barriers to adoption are not occasioned by the low benefits of DT varieties, but by the interplay of factors such as access to information and ready availability of retail supplies of seed. For example, compared to the female head plot managers, higher adoption probability associated with plot managers who are male household heads could be an indication of variable access to key resources (land, capital, information, and so on) that can influence adoption decisions. Such gender-linked resource constraints which resulted in variable adoption status is also observed in earlier studies (Doss and Morris, 2001; Smale, 2011; Fisher and Kandiwa, 2014). Interventions to improve adoption might require, among others, appreciating and accommodating such gender-linked differences through affirmative actions in favor of female farmers.
The implication is that public policy institutions involved in agricultural development may need to provide necessary supportive actions which can strengthen and leverage public extension services to promote these new generations of varieties. Government should underwrite programs for technology promotion and dissemination at early stages to provide the basis for the private sector (particularly input dealers) to enter into retailing these varieties. Promising policy mix to speed up adoption may include exposing farmers to these technologies through networks of field demonstrations and using farmers' social networks as most of the sample farmers relied on their fellow farmers for information. Distribution of sample seeds for farmers to experience the benefit and improving local availability of seed at affordable prices can accelerate the uptake of the DT maize varieties.
Although this study presents evidence that the benefits of DT maize are commensurate or better than existing non-DT maize seeds, the results are based on cross-sectional analysis which offers a snapshot and associational effects. In the future, panel data analysis will be needed to truly capture the dynamics and account for unobserved heterogeneities that underpin DT maize variety adoption and its corresponding impact.
Data availability statement
The datasets presented in this article are not readily available to ensure anonymity and observe the protocols that may be required from CIMMYT, the institution that was leading the project in the generation of the data. Requests to access the datasets should be directed to the corresponding author.
Author contributions
EH: material preparation, data collection, methodology, data analysis, and writing—original draft. PM: writing-part of the first draft and supervision. FB, AB, EH, and PM: conceptualization. FB, AB, and PM: writing—review and editing. All authors contributed to the study conception and design, improvised the previous versions of the manuscript, read, and approved the final manuscript.
Funding
This study was financed by the Bill & Melinda Gates Foundation (BMGF) through the Drought Tolerant Maize for Africa project implemented by the International Maize and Wheat Improvement Center (CIMMYT). BMGF mainly offered the financial support and did periodic check ins. CIMMYT led and coordinated the project across 13 African countries. Stress Tolerant Maize for Africa (Grant number OPP1134248) and Accelerating Genetic Gains for Maize and Wheat in Africa (Grant number INV-003439).
Acknowledgments
The authors wish to acknowledge in particular, Monica Fisher, for her insight and support in the design and implementation of the Uganda survey, and collaborations from others including Woinshet Asnake, Wiliam Ekere, Mywish Meridia, Daniel Kwemoi, Godfrey Asea, and the survey team for collaboration on the design and implementation of the Uganda survey. We are also indebted to Yosef Alemayehu for preparing the maps and Girma Mamo for his valuable input in the responses addressed to issues raised by reviewers. Our thanks are due to the respondents at the study sites. We are also grateful to the reviewers for their useful insights which we used to elevate the quality of this study.
Conflict of interest
The authors declare that the research was conducted in the absence of any commercial or financial relationships that could be construed as a potential conflict of interest.
Publisher's note
All claims expressed in this article are solely those of the authors and do not necessarily represent those of their affiliated organizations, or those of the publisher, the editors and the reviewers. Any product that may be evaluated in this article, or claim that may be made by its manufacturer, is not guaranteed or endorsed by the publisher.
Footnotes
1. ^The DTMA project was implemented by the International Maize and Wheat Improvement Center (CIMMYT) in conjunction with International Institute of Tropical Agriculture (IITA) and national research institutes in 13 African countries including Uganda.
2. ^Progressive farmers are those who achieve higher agricultural yields and earn higher agricultural profits than other farmers. These farmers are also usually the first in the village to adopt new technologies.
3. ^Actual rainfall data are preferable for the purpose, but reliable data that are villagespecific are scarce in most developing countries, including Uganda.
4. ^Plot manager, also referred to as farmer, refers to the head or spouse in a household who made decision (had management access) regarding what type of maize variety to cultivate on a given household plot.
5. ^In this study unless specified, modern maize refers to all improved maize seed- DT or non-DT modern maize. In addition, the terms “DT maize” and “non-DT maize” are used in subsequent sections to denote DT modern maize and non-DT modern maize, respectively. While modern maize seeds are products of formal breeding process, the local ones are results of farmers own long years of selection.
6. ^Bourguignon et al. (2007) using Monte-Carlo experiments, show that selection bias correction based on multinomial logit model can provide consistent and efficient estimates of the selection process and a reasonable correction for the outcome equations, even when the assumption of the independence of irrelevant alternatives (IIA) is not achieved.
References
Ahmed, M. (2012). Analysis of incentives and disincentives for maize in Uganda. Technical Notes Series. Rome: MAFAP, FAO.
Asfaw, S., Shiferaw, B., Simtowe, F., and Lipper, L. (2012). Impact of modern agricultural technologies on smallholder welfare: evidence from Tanzania and Ethiopia. Food Policy 37, 283–295. doi: 10.1016/j.foodpol.2012.02.013
Balirwa, E. K. (1992). Maize Research and Production in Uganda. Available online at: http://pdf.usaid.gov/pdf_docs/PNABT623.pdf (Accessed September 30, 2020).
Bandiera, O. (2006). Social networks and technology adoption in Northern Mozambique. Econ. J. 116, 869–902. doi: 10.1111/j.1468-0297.2006.01115.x
Bänziger, M., Setimela, P. S., Hodson, D., and Vivek, B. (2006). Breeding for improved drought tolerance in maize adapted to Southern Africa. Agric. Water Manage. 80, 212–224. doi: 10.1016/j.agwat.2005.07.014
Bourguignon, F., Fournier, M., and Gurgand, M. (2007). Selection bias corrections based on the multinomial logit model: Monte-Carlo comparisons. J. Econ. Surveys 21, 174–205. doi: 10.1111/j.1467-6419.2007.00503.x
Cairns, J. E., Hellin, J., Sonder, K., Araus, J. L., MacRobert, J. F., Thierfelder, C., et al. (2013). Adapting maize production to climate change in sub-Saharan Africa. Food Secur. 5, 345–360. doi: 10.1007/s12571-013-0256-x
Cavatassi, R., Lipper, L., and Narloch, U. (2011). Modern variety adoption and risk management in drought prone areas : insights from the sorghum farmers of eastern Ethiopia. Agric. Econ. 42, 279–292. doi: 10.1111/j.1574-0862.2010.00514.x
Deressa, T. T., Hassan, R. M., Ringler, C., Alemu, T., and Yesuf, M. (2009). Determinants of farmers' choice of adaptation methods to climate change in the Nile Basin of Ethiopia. Glob, Environ. Change 19, 248–255. doi: 10.1016/j.gloenvcha.2009.01.002
Di Falco, S., Veronesi, M., and Yesuf, M. (2011). Does adaptation to climate provide food security? A micro-perespective from Ethiopia. Am. J. Agric. Econ. 93, 829–846. doi: 10.1093/ajae/aar006
Doss, C. R., and Morris, M. L. (2001). How does gender affect the adoption of agricultural innovations? The case of improved maize technology in Ghana. Agric. Econ. 25, 27–39. doi: 10.1016/S0169-5150(00)00096-7
Dubin, J., and McFadden, D. (1984). An econometric analysis of residential electric appliance holdings and consumption. Econometricia 52, 345–362. doi: 10.2307/1911493
Ekiyar, V., Jumbe, C., and Mangisoni, J. Mkwambisi. (2010). “Climate change impacts to agriculture in Uganda's semi arid areas, farmers' means of adaptation: an application of cross-section data,” in Presentesd on the Second RUFORUM Biennial Meeting, 20–24 September 2010 (Entebbe).
Feder, G., Just, E. R., and Zilberman, D. (1985). Adoption of agricultural innovations in developing countries: a survey. Econ. Dev. Cult. Change 33, 255–298. doi: 10.1086/451461
Ferris, S., Engoru, P., Wood, M., and Kaganzi, E. (2008). “Making market information services work better for the poor in Uganda,” in Paper presented on workshop on Collective Action and Market Access for Smallholders, 2–5 October 2006 (Cali: International Food Policy Research Institute).
Fisher, M., Abate, T., Lunduka, R. W., Asnake, W., Alemayehu, Y., and Madulu, R. B. (2015). Drought tolerant maize for farmer adaptation to drought in sub-Saharan Africa: determinants of adoption in eastern and southern Africa. Clim. Change 133, 283–299. doi: 10.1007/s10584-015-1459-2
Fisher, M., and Carr, E. R. (2015). The influence of gendered roles and responsibilities on the adoption of technologies that mitigate drought risk : the case of drought-tolerant maize seed in eastern Uganda. Glob. Environ. Change 35, 82–92. doi: 10.1016/j.gloenvcha.2015.08.009
Fisher, M., and Kandiwa, V. (2014). Can agricultural input subsidies reduce the gender gap in modern maize adoption? Evidence from Malawi. Food Policy 45, 101–111. doi: 10.1016/j.foodpol.2014.01.007
Gneezy, U., and Potters, J. (1997). An experiment on risk taking and evaluation periods. Q. J. Econ. 112, 631–645. doi: 10.1162/003355397555217
Hartmann, D. L., Tank, a. M. G. K., and Rusticucci, M. (2013). IPCC Fifth Assessment Report, Climatie Change 2013: The Physical Science Basis. IPCC.
Intergovernmental Panel on Climate Change (IPCC) (2014). Climate Change 2014: Impacts, Adaptation and Vulnerability. Part B: Regional Aspects. Contribution of Working Group II to the Fifth Assesment Reports of IPCC. Cambridge University Press, 688.
Jain, M., Naeem, S., Orlove, B., Modi, V., and DeFries, R. S. (2015). Understanding the causes and consequences of differebtial decision-making in adaptation research: adapting to delayed monsoon onset in Gujarat, India. Glob. Environ. Change 31, 98–109. doi: 10.1016/j.gloenvcha.2014.12.008
Kafle, B. (2010). Determinants of adoption of improved maize varieties in developing countries: a review. Int. Res. J. Appl. Basic Sci. 1, 1–7. Avaiable online at: http://ecisi.com/wp-content/uploads/2012/01/1-71.pdf (accessed December 25, 2020).
Kansiime, M. K., Wambugu, S. K., and Shisanya, C. A. (2013). Perceived and actual rainfall trends and variability in eastern Uganda: implications for community preparedness and response. J. Nat. Sci. Res. 3, 179–194. Available online at: https://www.researchgate.net/publication/301351273 (accessed December 20, 2020).
Kassie, M., Shiferaw, B., and Muricho, G. (2011). Agricultural technology, crop income, and poverty alleviation in Uganda. World Dev. 39, 1784–1795. doi: 10.1016/j.worlddev.2011.04.023
Kassie, M., Teklewold, H., Marenya, P., Jaleta, M., and Erenstein, O. (2014). Production risks and food security under alternative technology choices in Malawi: application of a multinomial endogenous switching regression. J. Agric. Econ. 66, 640–659. doi: 10.1111/1477-9552.12099
La Rovere, R., Abdoulaye, T., Kostandini, G., Guo, Z., Mwangi, W., MacRobert, J., et al. (2014). Economic, production, and poverty impacts of investing in maize tolerant to drought in Africa: an ex-ante assessment. J. Dev. Areas 48, 199–225. doi: 10.1353/jda.2014.0016
Li, Y., Ye, W., Wang, M., and Yan, X. (2009). Climate change and drought: a risk assessment of crop-yield impacts. Clim. Res. 39, 31–46. doi: 10.3354/cr00797
Liu, E. M. (2013). Time to change what to sow: risk preferences and technology adoption decisions of cotton farmers in China. Rev. Econ. Stat. 95, 1386–1403. doi: 10.1162/REST_a_00295
Lobell, D. B., Burke, M. B., Tebaldi, C., Mastrandrea, M. D., Falcon, W. P., and Naylor, R. L. (2008). Prioritizing climate change adaptation needs for food security in 2030. Science 319, 607–610. doi: 10.1126/science.1152339
Lybbert, T. J., and Sumner, D. A. (2012). Agricultural technologies for climate change in developing countries: policy options for innovation and technology diffusion. Food Policy 37, 114–123. doi: 10.1016/j.foodpol.2011.11.001
Magorokosho, C., Vivek, B., and MacRobert, J. (2009). Characterization of Maize Germplasm in Eastern and Southern Africa: Results of the 2008 Regional Trials Coordinated by CIMMYT. Harare: CIMMYT.
Matuschke, I., and Qaim, M. (2009). The impact of social networks on hybrid seed adoption in India. Agric. Econ. 40, 493–505. doi: 10.1111/j.1574-0862.2009.00393.x
McFadden, D. (1973). “Conditional logit analysis of qualitative choice behavior,” in Frontiers in Econometrics, ed P. Zarembka (New York, NY: Academic Press), 105–142.
Ministry of Agriculture Animal Industry and Fishery (MAAIF) (2011). National Agriculture Policy. Kampala: Ministry of Agriculture Animal Industry and Fishery (MAAIF).
Nabikolo, D., Bashaaasha, B., Mangheni, M. N., and Majaliwa, J. G. M. (2012). Determinants of climate change adaptation among male and female headed farm households in Eastern Uganda. Afr. Crop Sci. J. 20:203. Available online at: https://www.ajol.info/index.php/acsj/article/view/81767 (accessed December 11, 2020).
Smale, M. (2011). “Does household headship affect demand for hybrid maize seed in Kenya? An exploratory analysis based on 2010 survey data,” in MSU International Development Working Paper No. 115 (East Lansing, MI: Michigan State University).
Solis, D., Uretta, B. E., and Bravo, Q.uiroga, R.E. (2007). Soil conservation and technical efficiency among hillside farmers in Central America: a switching regression model. Austr. J. Agric. Resour. Econ. 51, 491–510. doi: 10.1111/j.1467-8489.2007.00394.x
Tambo, J. A., and Abdoulaye, T. (2013). Smallholder farmers' perceptions of and adaptations to climate change in the Nigerian savanna. Region. Environ. Change 13, 375–388. doi: 10.1007/s10113-012-0351-0
Teklewold, H., Kassie, M., Shiferaw, B., and Kohlin, G. (2013). Cropping system diversi- fication, conservation tillage and modern seed adoption in Ethiopia: impacts on household income, agricultural chemical use and demand for labor. Ecol. Econ. 93, 85–93. doi: 10.1016/j.ecolecon.2013.05.002
Twinomugisha, B. (2005). A Content Analysis Reports on Climate Change Impacts, Vulnerability and Adaptation in Uganda. Kampala: DENIVA.
Uganda Bureau of Statistics (UBOS). (2010). Summary Report on Uganda Census of Agriculture 2008/2009. Kampala: Uganda Bureau of Statistics (UBOS).
Uganda Bureau of Statistics (UBOS) (2012). Statistical Abstracts. Kampala: Uganda Bureau of Statistics (UBOS).
Uganda Bureau of Statistics (UBOS) (2013). Statistical Abstracts. Kampala: Uganda Bureau of Statistics (UBOS).
Uganda Bureau of Statistics (UBOS). (2014). National Population and Housing Census 2014: Provisional Result. Kampala: Uganda Bureau of Statistics (UBOS).
United States Agency for International Development (USAID) (2010). Market Assessment and Baseline Study Of Staple Foods. Country Report. Kampala: United States Agency for International Development (USAID).
Wasige, J. E. (2009). Assessment of the Impact of Climatechange and Climate Variability on Crop Production in Uganda. End of Project Technical Report Submitted to START/US National Science Foundation. Kampala: Department of Soil Science; Makarere University. Available online at: http://start.org/download/gec08/wasige-final.pdf
Appendix

Table A1. Monthly rainfall totals (in mm) of 2014 for the study districts from the proximate weather station.
Keywords: drought, maize, multinomial switching regression, impact, Uganda
Citation: Habte E, Marenya P, Beyene F and Bekele A (2023) Reducing susceptibility to drought under growing conditions as set by farmers: The impact of new generation drought tolerant maize varieties in Uganda. Front. Sustain. Food Syst. 6:854856. doi: 10.3389/fsufs.2022.854856
Received: 14 January 2022; Accepted: 15 November 2022;
Published: 19 January 2023.
Edited by:
Vandana Jaiswal, Institute of Himalayan Bioresource Technology (CSIR), IndiaReviewed by:
Paul Isolo Mukwaya, Makerere University, UgandaBjorn Van Campenhout, International Food Policy Research Institute, United States
Copyright © 2023 Habte, Marenya, Beyene and Bekele. This is an open-access article distributed under the terms of the Creative Commons Attribution License (CC BY). The use, distribution or reproduction in other forums is permitted, provided the original author(s) and the copyright owner(s) are credited and that the original publication in this journal is cited, in accordance with accepted academic practice. No use, distribution or reproduction is permitted which does not comply with these terms.
*Correspondence: Endeshaw Habte, ZW5kaGFidGUmI3gwMDA0MDtnbWFpbC5jb20=
†These authors have contributed equally to this work and share first authorship