- Department of Agricultural Economics and Agribusiness Management, Egerton University, Njoro, Kenya
Climate-smart agriculture (CSA) is increasingly becoming important as a sustainable way of increasing agricultural productivity and increasing the resilience of farming systems to climate variability. Moreover, crop diversification strategy plays a vital role in creating resilience against climate-related risks in farm production and enhancing resilience in food systems. While crop diversification intensity acts as a proxy indication of climate risk mitigation strategy, its successful implementation as a climate-smart agricultural practice depends on the ability of the smallholder farmers to allocate the available farm resources efficiently. The study evaluated the effect of crop diversification on variable cost structure (land, labor, capital, fertilizer, and seeds) among smallholder farmers in Western Kenya. We use primary data from 267 randomly selected respondents and apply a translog cost function model to explore the effect of implementing crop diversification strategy on variable cost structure among smallholder farmers. The results showed that indeed practicing crop diversification affects the overall production cost structure. The result showed that the Allen elasticity of substitution (AES) of all combinations of inputs (land and capital, land and fertilizer, land and labor, fertilizer and capital, fertilizer and labor, fertilizer and capital) are positive. These relationships imply that land, labor, fertilizer, and capital substitute each other in crop production. The Morishima elasticities of factor substitution (MES) reveal that the highest degree of substitutability in response to price changes is between capital and fertilizer, land and fertilizer, and labor and fertilizer, implying the intensive nature of crop diversity in terms of land, labor and capital requirements. These findings demonstrate that despite the potential benefits of crop diversification, the trade-off in the total cost of production does matter. Non-accounting for such trade-offs is likely to over-estimate crop diversification benefits and limit its successful practice by smallholder farmers.
Introduction
Agricultural diversification involves allocations of production resources to a wide range of economic activities. Shahbaz et al. (2017) argued that crop diversification is one of the cost-effective risk management strategies to mitigate the uncertainties at the farm level since it affects smallholder farmers' efficiency and economic returns. However, practicing crop diversification entails competition for resources among various crops produced by the farmer that can ultimately positively or negatively affect farm efficiency. Previous studies on crop diversification and technical and allocative efficiency (Haji, 2007; Rahman, 2009; Nandan et al., 2013; Shahbaz et al., 2017; Mzyece et al., 2018) have shown contrasting results indicating that the effect of crop diversification on farm efficiency and economic returns may be country- as well as region-specific. Moreover, Mzyece et al. (2018) results revealed that 1 unit of increase in crop diversification index increases the technical efficiency 0.48 units while a 1-unit increase in crop diversification index reduces variability in income by 0.007 and 0.792 units.
The policy discussion on crop diversification as a climate-smart agricultural practice in the recent past had been a point of interest in the field of agriculture and climate change. In 2019, through the World Bank, the Kenyan government launched a project dubbed the Kenya Climate Smart Agriculture project (KCSA-Project) to improve the agricultural productivity of smallholder farmers through the production of climate-smart crops such as cassava, millet, sorghum, and sweet potato. Despite its main advantage of improving food security and resilience for farmers in the face of climate variability, practicing crop diversification comes with an additional cost of production compared to the mono-cropping farming system (Nandan et al., 2013).
The choice of crop diversification as one of the climate-smart agricultural practices to study was motivated by the growing interest among scientists, policymakers, inter-community development agencies, as well as national government to understand the trade-offs around this practice with resource allocation efficiency and technical efficiency (Ogundari, 2013; Ahmadzai, 2017; Khanal and Mishra, 2017; Mzyece et al., 2018). Previous studies (Di Falco, 2014; Teklewold et al., 2018) reported that adopting agricultural technologies like crop diversification could increase farm revenue and food security among smallholder farmers. Mulwa and Visser (2020) found that a 1-unit increase in crop diversification increases household dietary diversity score (HDDS) by 0.7 points, while it increases monthly per capita expenditure by approximately N$78 in Namibia. However, it can also create trade-offs between farm inputs such as increased demand for labor, agrochemicals, seeds, and other farm inputs. The result implies that the efforts aiming at promoting crop diversification as a climate-smart agricultural technique should evenly center on ways to minimize its effect on the cost of production.
Although crop diversification plays a vital role in improving food security, income generation, increasing soil fertility, and risk management tool against weather variability, smallholder farmers still face many challenges in producing food in a sustainable and diversified farming system (Teklewold et al., 2013). Possible explanations could be attributed to the lack of farm inputs, high input price, frequent pest and disease outbreaks, and climate variability risks and shocks resulting in higher cost of production. Successful implementation of CSA strategies such as crop diversification in rural developing countries like Kenya requires a proper understanding of trade-offs and their effect on production cost. Crop diversification index was used to measure the extent of crop diversification among smallholder farmers. In measuring the extent of crop diversification, Simpson's Index of diversification (SID) was used. Simpson's index of diversity (SID) was preferred to the Herfindahl index (HI) and Ogive index (OI). This is because SID is an area-based index and measures horizontal diversification in terms of its being proportionate in computation, and it works well when estimating crop diversification. Both HI and OI were found unsuitable for this study since these indices measure diversification in terms of revenue; hence, they are suitable for estimating diversification among livestock and integrated crop-livestock system (Asante et al., 2018). Therefore, this study investigated the trade-offs associated with crop diversification by particularly looking at its effect on the variable cost structure of smallholder farmers in Kisumu County, Kenya. Estimating the effect of crop diversification on variable cost structure could provide critical insights on the decision-making process in resource allocation faced by smallholder farmers.
Furthermore, a better understanding of the role of crop diversification on farm efficiency and resource allocation can also inform policy interventions for practicing crop diversification as part of climate-smart agricultural practice among smallholder farmers. The rest of the paper is organized as follows: in the next section, we present the methodology used to get data and the analytical techniques. In section three we present and discuss the study results, and finally in Section four, we make conclusions and draw implications from the study results.
Materials and Methods
Study Area
The study was conducted in five wards (West Kisumu, Central Kisumu, Kisumu North, North West Kisumu, and South West Kisumu) across Kisumu West Sub County in Kisumu County (Figure 1). The sub-county has a total area of 212.90 km2 with a population of 131,246 people (Kenya National Bureau of Statistics, 2010). The sub-county has a bimodal type of rainfall pattern of long and short rains. The Sub-county lies between longitude 34° 44′ and 34° 54′ East and Latitude 0° 05′ and 0° 14′ North. The annual precipitation ranges between 1,200 and 1,300 mm in different sectors (County Government of Kisumu, 2015). The major crops grown in the region are maize, beans, sweet potato, sorghum, and cassava under rain-fed agriculture.
Data Sources and Sampling
The study was based on a cross-sectional research design whereby primary data were collected using a structured questionnaire comprising both open and closed-ended questions. Following Anderson et al. (2007), a sample of 267 respondents was randomly selected from all five wards to ensure even representation of all farmers in the study area. The sample size was distributed proportionately to the number of households per ward in Kisumu West Sub-County, as presented in Table 1.
Methods
Translog cost function model was used to estimate the effect of crop diversification on the variable cost structure. The cost function was estimated jointly with the cost shares functions using seemingly unrelated analysis. The variable input factors used in the analysis are land, labor, capital, and fertilizer. Consider a farmer whose objective is to produce output (Y) using different combinations of inputs (X) and crop diversification adaptation strategy (Z). Let w be a vector of input prices; is the input price for = 1… n inputs. The model is chosen because of its overall flexibility and limited a priori restriction on substitution possibilities and scale economies (Obare et al., 2003). The cost function in a translog form can be written as:
Labor (L), Fertilizer (F), Land (A), Capital (K), and Seeds (S)
where C is the total cost of production; α0, βi, γij, δij, ϕi, and φi are unknown parameters to be estimated such that γij = γji and δij = δji (a direct consequence of minimization behavior of producers); wi is the factor price, Zi is the crop diversification index, Y is the physical output, and μ is the random error term. The cost function is linearly homogeneous and non-decreasing in w. Satisfying the homogeneity condition requires that: ∑αi = 1, ∑γij = 0 and ϕij = 0, while the requirement of the homothetic condition is that ∑βiY = 0. The translog cost function is flexible because specific features of technology such as returns to scale may be tested by examining the estimated model parameters (Obare et al., 2003; Kumbhakar et al., 2015; Shikuku et al., 2015).
Differentiating Equation (1) with respect to input prices yields Shephard's lemma
where Si is the cost share of the ith input factor. As a result, the translog cost function yields a cost share equation as follows:
Allen partial elasticities of substitution (AES) between inputs i and j were derived from the cost function as σij = (γij + Si.Sj)/Si.Sj, and . Following Obare et al. (2003) and Shikuku et al. (2015), the respective own and cross price elasticities of demand for individual inputs were calculated as ηii = Si.σii and ηij = Sj.σij, respectively, where σii and σij are the own and cross-price elasticity of demand, respectively. Furthermore, Morishima elasticities of substitution (MES) were also computed because AES do not indicate the curvature ease of substitution as MES which also preserve the significant features of the Hicksian concept in the multifactor situation (Obare et al., 2003). MES also provides sufficient statistics for assessing the effect of changes in the price or quantity ratios on relative factor shares. The MES between factors i and j and vice versa, respectively, are determined as Mij = ηij − ηii and Mji = ηij − ηjj. The cost elasticity with respect to crop diversification (κCZ) is computed as:
where κCZ measures the productivity effect of crop diversification via adjustment in factor demand. The factor adjustment effect is measured by the elasticity of factor shares with respect to crop diversification that is ∂Si/∂ln Z, which is equivalent to the parameter γiZ of the cost share function. Therefore, the elasticity demand for inputs with respect to crop diversification is given as
for all i; i ≠ j.
The value of κiZ obtained in Equation (5) can be positive or negative depending on whether crop diversification practice results into increased or decreased demand for the ith input in crop production.
In order to assess the effect of crop diversification on demand for land, labor, fertilizer, and capital, Equations (1) and (3) were jointly estimated using a seemingly unrelated regression model (SUR). Four outputs were considered: maize, sorghum, cassava, and sweet potato, while the price of seed input was used to normalize all the input prices and the total cost of production, that is, the prices were expressed as relative to maintain linear homogeneity of the cost function. The analysis focused on five variable inputs: land, labor, fertilizer, capital, and seeds. However, to satisfy the adding up condition and maintain the linear homogeneity, the seeds share equation was dropped, and only n-1 equations were linearly independent due to the homogeneity restriction imposed in the model.
The following independent variables were included in the cost-share functions: Labor price (lnwL), Fertilizer price (lnwF), Land price (lnwA), Capital price (lnwK); quantity of maize (lnQmz), the quantity of cassava (lnQCsv), the quantity of sorghum (lnQsgm), and quantity of sweet potato (lnQswpt). All prices and quantities are expressed in natural logarithms. The crop diversification index (SID) was included in the model to capture the effect of crop diversification on the cost shares. Moreover, the cost function also included the interaction between input prices (e.g. lnwL x lnwF), output quantities (e.g. lnQmz x lnQsgm), as well as the input prices and output quantities (e.g. lnQmz x lnwL). The outcome variables were defined as follows:
• Labcostshare is the cost of labor divided by the total cost of production.
• Fertcostshare is the cost of fertilizer divided by the total cost of production.
• Landcostshare is the cost of land divided by the total cost of production.
• Capitalcostshare is the cost of capital divided by production.
The outcome variable in the cost function is lncost, and it is the total cost of producing maize, sorghum, cassava, and sweet potato, and it is expressed in the natural log form.
Description of Variables Used in the Econometric Analysis
In Table 2, the effect of crop diversification on the cost structure variables are presented and subsequently, the postulated casual relationship discussed.
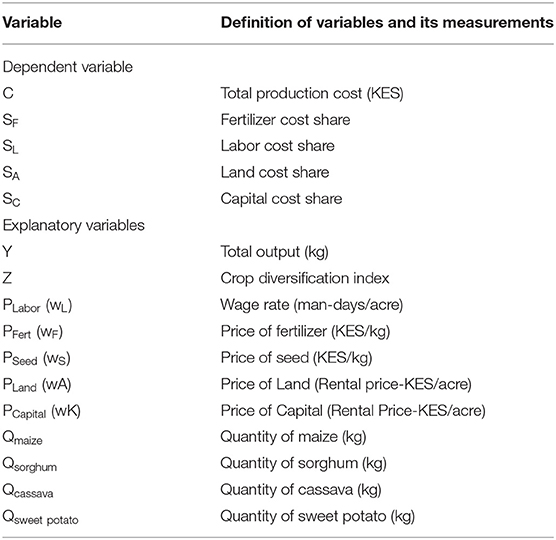
Table 2. Description of model variables for effect of crop diversification on variable cost structure.
Input Prices
Variable costs are essential factors to consider when making farm decisions. Input prices such as land, labor, capital, seeds, and fertilizer are vital factors in practicing crop diversification. It was hypothesized that high input prices negatively influence farm diversification due to high capital requirements.
Crop Diversification Index
This variable shows the extent of crop diversification among the smallholder farmers. It was captured in the model to indicate the effect of practicing crop diversification on the variable cost of production. The study postulated that the larger the extent of crop diversification, the higher the cost of production.
The Total Cost of Production
The variable was included in the translog cost function as a dependent variable. However, it was expected to be influenced by the decision to diversify crop production. The study also postulated that it would affect the variable cost structure of the farm household since crop diversification practice requires additional capital investment.
Labor Cost-Share
This variable was used as the dependent variable in the labor cost share equation. It shows the total cost of labor share in the total cost of production.
Fertilizer Cost-Share
This variable was used as the dependent variable in the fertilizer cost-share equation. It shows the total cost of fertilizer share in the total cost of production.
Land Cost-Share
This variable was used as the dependent variable in the land cost-share equation. It shows the total cost of a land share in the total cost of production.
Capital Cost-Share
This variable was used as the dependent variable in the capital cost-share equation. It shows the total cost of a capital share in the total cost of production.
Quantity Produced
This variable was used as a dependent variable in this study's translog cost function model. It was used as the amount of output per crop.
Results and Discussion
Descriptive Results
The descriptive statistics of the variables used in this study are presented in Table 3.
The results in Table 3 show that the average farm size among the respondents interviewed is 1.71 acres. The results show that most of the farm households hold small pieces of land. However, Rahman (2009) and Amare et al. (2018) reported that successful practice of crop diversification requires more land. The Simpsons' index value of crop diversification on average is 0.42, indicating that the level of crop diversification through the production of climate-smart crops is still low among smallholder farmers in the study area. Asante et al. (2018) reported that a crop diversification index above 0.5 shows that the farmers are relatively doing well in terms of crop diversity. On average, farmers incurred about 22,753/= as total production while the average maize production per acre was 567.15 kg. Most of the farmers attributed this low productivity to variation in weather patterns and pest and disease incidences. The production of climate-smart crops such as sorghum, cassava, and sweet potato is still low, with average yields of 99, 1,690, and 270 kg per acre. The low production can be attributed to inadequate land to produce the crops in large quantities in addition to a low adoption rate. Additionally, most smallholder farmers mainly farm cassava, sweet potato, and sorghum for subsistence purposes.
Empirical Results
To meet the price homogeneity condition of the translog cost function model, seed prize was used to normalize the total cost of production, land, labor, fertilizer, and capital prices. The F-statistic (Prob > F = 0.000) was highly significant at a 1% significance level, showing that the model satisfies the price homogeneity conditions by construction. All the constraints (homotheticity, symmetry, non-negativity, and concavity) imposed were satisfied.
The results showed that the coefficient of crop diversification index is significant in the cost function and labor cost share function. The estimated parameters of the homogeneous cost functions using seemingly unrelated regression analysis are presented in Table 4.
The results show that six out of nine coefficients (labor price, capital price, land price, maize quantity, cassava quantity, and crop diversification) significantly influences the labor cost share function at p ≤ 0.05. Five out of nine coefficients (labor price, capital price, land price, capital price, and maize quantity) significantly influence the land cost share and capital cost share function at p ≤ 0.05. Furthermore, the crop diversification effect coefficient on production cost (0.0806, p ≤ 0.05) significantly influences the labor cost share function only. A possible explanation is that more labor is required in a diversified farming system, hence crop diversification's effect on production costs. Similarly, Chhatre et al. (2016) found that including horticultural crops in crop portfolios among smallholder farmers increases both labor and capital requirement resulting in increased cost of production in India.
The elasticities of substitution were calculated at mean levels of input shares because of the variation in input share levels. Allen elasticities of substitution are shown in Table 5.
The AES between all combinations of inputs [Labor and Fertilizer (16.84), Labor and Land (9.75), Labor and Capital (15.97), Land and Fertilizer (74.15), Fertilizer and Capital (113.66), and Capital and Land (69.14)] are all positive as postulated. The result suggests that labor, land, fertilizer, and capital substitute each other in crop production.
The Morishima elasticities of factor substitution (MES) are presented in Table 6.
The study findings reveal that the highest degree of substitutability is in response to price changes between fertilizer and capital (11.21), land and fertilizer (11.21), and labor and fertilizer (10.29). The study results imply that an increase in a unit price of land, labor, capital, and fertilizer results into substitution between these inputs. Moreover, the substitution effect varies across individual farm households which practice crop diversification at different levels with different crop mix portfolios. The MES of Labor by land (5.97) is higher than the MES of Land by labor (1.67), which confirms that to improve the extent of crop diversification, as a climate-smart agricultural practice among smallholder farmers, more land is needed (Rahman, 2009). This study finding corroborates the results of Amare et al. (2018) who found that the probability of practicing crop diversification through production of climate-smart crops increases with an increase in farm size. More land is required to enable smallholder farmers to produce crops such as cassava and sweet potatoes on different portions to improve productivity. This is because these crops cannot be intercropped with other crops. Besides, additional land will require more labor; Branca et al. (2021) found that high investment costs mainly hinder smallholder farmers' practice of climate-smart agriculture, more so labor due to increase on-farm workforce requirements.
Furthermore, Senyolo et al. (2018) argue that the requirement of more labor and the initial cost of investment coupled with the intensity of management associated with CSA may reduce the likelihood of smallholder farmers adopting these practices as a mitigation strategy for climate variability. To improve the uptake and upscale practice of CSA, Branca et al. (2021) suggested that investment programs for climate-smart agriculture should encourage youth participation in agriculture. Moreover, the MES of Land by Fertilizer (11.21) is higher than that of Fertilizer by Land (7.36) due to production of maize crop all season round in the study area that have resulted in soil fertility depletion.
The derived price elasticities of factor demand are shown in Table 7.
The results show that own-price elasticity labor (−0.02), fertilizer (−0.13), land (−0.23), and capital (−0.16) are negative while cross-price elasticity Labor-fertilizer (10.26), Labor-Land (5.94), Labor-Capital (9.73) Fertilizer-Labor (1.64), Fertilizer-Land (7.23), Fertilizer-Capital (11.08), Land-Labor (1.44), Land-Capital (10.24), Land-Fertilizer (10.98), Capital-Labor (1.51), Capital-Land (6.54), and Capital-Fertilizer (10.75) are positive as expected and are in line with the economic theory. The positive cross-price elasticities imply that as the extent of crop diversification increases across individual farm households, the demand for land, labor, capital, and fertilizer increases proportionately.
Using the estimated parameters of the translog cost model, the demand elasticity of labor (11.89) was positive while that of fertilizer (−76.61), land (−60.51), and capital (−197.63) was negative (Table 8). This result suggests that as a farm household increases the extent of crop diversification through production of climate-smart crops (sorghum, cassava, and sweet potato), the labor demand increases proportionately (i.e., positive value). This confirms the labor-intensive nature of crop diversification. Similarly, Zerssa et al. (2021) found that inadequate labor supply, lack of technical knowhow, and shortage of funds are the major factors influencing the adoption of climate-smart agricultural practices, the mitigation strategy to climate variability in Ethiopia. On the other hand, the demand elasticity of land and capital were negative implying that as farm households increase the intensity of crop diversity, the demand for land, capital, and fertilizer decreases. A possible explanation could be that due to intercropping of crops such as maize and sorghum, the demand for land and capital decreases. Furthermore, the demand elasticity of fertilizer decreases with a unit increase in extent of crop diversification due to variation in soil type and fertility level. Moreover, in line with the demand elasticity of fertilizer, Ogundari (2013) analyzed crop diversification and technical efficiency in food crop production in Nigeria and found that the demand elasticity of fertilizer was positive, implying that fertilizer use is region-specific.
A clear implication of the study findings is that promoting crop diversification as a climate-smart agricultural practice to climate risks among smallholder farmers in Western Kenya results in additional production costs among the resource-constrained farmers. Therefore, to improve crop diversification among the farmers, the government and developmental organizations should improve credit access through low-interest loans and grants to enhance the financial stability of the farmers. Similar results were reported by Shikuku et al. (2015), who argued that increased demand for extra cost hinders the uptake of CSA practices such as mulching and crop diversification. Moreover, these findings demonstrate that despite the potential benefits of crop diversification, the trade-off in the total cost of production does matter. Non-accounting for such trade-offs is likely to over-estimate crop diversification benefits and limit its successful practice by smallholder farmers. However, the long-term solutions to improve its practice will call for its practice along with complementary practices such as minimum or zero tillage to reduce the increased production cost that comes with the uptake of crop diversification.
Conclusion and Policy Implications
We used cross-sectional data to evaluate smallholder farmers' climate-smart crop diversification cost structure in Western Kenya. The research question was motivated by the difficulties faced by policymakers in promoting crop diversification as a climate-smart agricultural practice due to its effect on the production cost structure. However, the successful adoption of any CSA practice requires adequate knowledge and understanding of the trade-off and effect of each option.
The study result showed that, indeed, practicing crop diversification as a climate-smart agricultural practice increases the cost of production due to additional land, labor, and capital required relative to production of maize only under mono-cropping farming system. Furthermore, the study finding implies that farmers who diversified their farming system by producing all four crops (maize, sorghum, cassava, and sweet potatoes) need to incur an additional cost of production. These additional costs of production involve cost of hiring land, labor costs, fertilizer costs, and cost of seeds. Although the cost of seed was found to have little impact on increment of cost of production, due to climate change, most of the smallholder farmers were found changing to certified and drought-tolerant maize varieties compared to local varieties. The additional cost of production prevents most of the smallholder farmers from up-scaling the practice of crop diversification as a climate-smart agricultural practice.
Although crop diversification has the potential of improving resiliency in food systems, its effect on cost of production needs to be weighed against its potential benefits. Therefore, ignoring such trade-offs in implementing crop diversification might not only overestimate its benefits as climate-smart strategy but can also limit its successful adoption and up-scaling among the resource-constrained smallholder farmers. Based on the study results, a clear policy implication is that there is need for the Kenyan government to divert more agricultural resources in promoting crop diversification as climate-smart agricultural practice for reduced climate variability effect and improved resilience in agri-food systems among smallholder farmers. Moreover, policymakers should consider formulating policies that reduce farmers' financial burdens in implementing crop diversification adaptation strategy. Therefore, policies aimed at increasing farmers' financial liquidity level are highly recommended to cater for the increased production cost.
Finally, any efforts aiming to promote a wide-scale practice of crop diversification should equally focus on ways to minimize its effect on the total cost of production. Therefore, farm households practicing crop diversification should consider choosing crop mix combinations that reduce the total cost of production and effect of climate risks. Moreover, policies should also address labor constraints associated with crop diversification; for example, participation in groups to increase social capital and boost collective action among smallholder farmers is recommended.
Data Availability Statement
The raw data supporting the conclusions of this article will be made available by the authors, without undue reservation.
Author Contributions
All authors listed have made a substantial, direct, and intellectual contribution to the work and approved it for publication.
Funding
This work was funded by African Economic Research Consortium (AERC) based in Nairobi, Kenya.
Author Disclaimer
The views expressed here are those of the authors and do not necessarily reflect the views of AERC.
Conflict of Interest
The authors declare that the research was conducted in the absence of any commercial or financial relationships that could be construed as a potential conflict of interest.
Publisher's Note
All claims expressed in this article are solely those of the authors and do not necessarily represent those of their affiliated organizations, or those of the publisher, the editors and the reviewers. Any product that may be evaluated in this article, or claim that may be made by its manufacturer, is not guaranteed or endorsed by the publisher.
Acknowledgments
The authors are grateful to the African Economic Research Consortium (AERC) for funding the research.
References
Ahmadzai, H. (2017). Crop Diversification and Technical Efficiency in Afghanistan: Stochastic Frontier Analysis (No. 17/04). CREDIT Research Paper.
Amare, M., Mavrotas, G., and Edeh, H. (2018). Farmers' Crop Choice Decisions: Trends and Determinants in Nigeria andUganda. IFPRI-Discussion Papers (1716).
Anderson, D. R., Sweeny, J. D., Williams, T. A., Freeman, J., and Shoesmith, E. (2007). Statistics for Business and Economics. London: Thomson Learning.
Asante, B. O., Villano, R. A., Patrick, I. W., and Battese, G. E. (2018). Determinants of farm diversification in integrated crop–livestock farming systems in Ghana. Renew. Agric. Food Syst. 33, 131–149. doi: 10.1017/S1742170516000545
Branca, G., Braimoh, A., Zhao, Y., Ratii, M., and Likoetla, P. (2021). Are there opportunities for climate-smart agriculture? Assessing costs and benefits of sustainability investments and planning policies in Southern Africa. J. Cleaner Prod. 278, 123847. doi: 10.1016/j.jclepro.2020.123847
Chhatre, A., Devalkar, S., and Seshadri, S. (2016). Crop diversification and risk management in Indian agriculture. Desicion 43, 167–179. doi: 10.1007/s40622-016-0129-1
County Government of Kisumu. (2015). County Fact Sheet: Vital Statistics. County Government of Kisumu, Kenya.
Di Falco, S. (2014). Adaptation to climate change in Sub-Saharan agriculture: assessing the evidence and rethinking the drivers. Eur. Rev. Agric. Econ. 41, 405–430. doi: 10.1093/erae/jbu014
Haji, J. (2007). Production efficiency of smallholders' vegetable-dominated mixed farming system in Eastern Ethiopia: a non-parametric approach. J. Afr. Econ. 16, 1–27 doi: 10.1093/jae/ejl044
Kenya National Bureau of Statistics. (2010). The 2009 Kenya Population and Housing Census, Vol. 1. Nairobi: Kenya National Bureau of Statistics.
Khanal, A. R., and Mishra, A. K. (2017). Enhancing food security: food crop portfolio choice in response to climatic risk in India. Global Food Secur. 12, 22–30. doi: 10.1016/j.gfs.2016.12.003
Kumbhakar, S. C., Wang, H. J., and Horncastle, A. P. (2015). A Practitioner's Guide to Stochastic Frontier Analysis Using Stata. Cambridge: Cambridge University Press. doi: 10.1017/CBO9781139342070
Mulwa, C. K., and Visser, M. (2020). Farm diversification as an adaptation strategy to climatic shocks and implications for food security in northern Namibia. World Dev. 129, 104906. doi: 10.1016/j.worlddev.2020.104906
Mzyece, A., Zereyesus, Y. A., and Shanoyan, A. (2018). “Does Crop Diversification Involve A Trade-Off Between Technical Efficiency And Income Stability For Farmers In Zambia?,” in Paper presented at the 2018 Agricultural & Applied Economics Association Annual Meeting (Washington, DC).
Nandan, B. R. I. J., Sharma, B. C., and Kumar, A. N. I. L. (2013). Mitigating food security options through climate resilient mustard-maize based intercropping sequences for North-western-Himalayas. J. Food Secur. 1, 58–64. doi: 10.12691/JFS-1-2-8
Obare, G. A., Omamo, S. W., and Williams, J. C. (2003). Smallholder production structure and rural roads in Africa: the case of Nakuru District, Kenya. Agric. Econ. 28, 245–254. doi: 10.1111/j.1574-0862.2003.tb00141.x
Ogundari, K. (2013). Crop diversification and technical efficiency in food crop production: a study of peasant farmers in Nigeria. Int. J. Soc. Econ. 40, 267–287. doi: 10.1108/03068291311291536
Rahman, S. (2009). Whether crop diversification is a desired strategy for agricultural growth in Bangladesh? Food Policy 34, 340-349. doi: 10.1016/j.foodpol.2009.02.004
Senyolo, M. P., Long, T. B., Blok, V., and Omta, O. (2018). How the characteristics of innovations impact their adoption: an exploration of climate-smart agricultural innovations in South Africa. J. Cleaner Prod. 172, 3825–3840. doi: 10.1016/j.jclepro.2017.06.019
Shahbaz, P., Boz, I., and Haq, S. U. (2017). Determinants of crop diversification in mixed cropping zone of Punjab Pakistan. Direct Research. J. Agric. Food Sci 5, 360–366.
Shikuku, K. M., Laderach, P., Winowiecki, L., and Mwongera, C. (2015). A Cost Function Analysis of Trade-Offs Within Climate Smart Agriculture: Does Mulching Save the Cost of Crop Production Among Smallholder Farmers in Uganda? (No. 1008-2016-80240). Milan: International Association of Agricultural Economist.
Teklewold, H., Gebrehiwot, T., and Bezabih, M. (2018). Climate smart agricultural practices and gender differentiated nutrition outcome: an empirical evidence from Ethiopia. World Dev. 122, 38–53. doi: 10.1016/j.worlddev.2019.05.010
Teklewold, H., Kassie, M., Shiferaw, B., and Köhlin, G. (2013). Cropping system diversification, conservation tillage and modern seed adoption in Ethiopia: Impacts on household income, agrochemical use and demand for labor. Ecol. Econom. 93, 85–93. doi: 10.1016/j.ecolecon.2013.05.002
Keywords: crop diversification, cost structure, climate-smart, resilience, translog cost function
Citation: Awiti HA, Gido EO and Obare GA (2022) Smallholder Farmers Climate-Smart Crop Diversification Cost Structure: Empirical Evidence From Western Kenya. Front. Sustain. Food Syst. 6:842987. doi: 10.3389/fsufs.2022.842987
Received: 24 December 2021; Accepted: 21 February 2022;
Published: 31 March 2022.
Edited by:
Hupenyu Allan Mupambwa, Sam Nujoma Marine and Coastal Resources Research Center (SANUMARC), NamibiaReviewed by:
Patrick Nyambo, University of Fort Hare, South AfricaBinganidzo Muchara, University of South Africa, South Africa
Copyright © 2022 Awiti, Gido and Obare. This is an open-access article distributed under the terms of the Creative Commons Attribution License (CC BY). The use, distribution or reproduction in other forums is permitted, provided the original author(s) and the copyright owner(s) are credited and that the original publication in this journal is cited, in accordance with accepted academic practice. No use, distribution or reproduction is permitted which does not comply with these terms.
*Correspondence: Hezbon Akelo Awiti, YWtlbGxvYXdpdHRpQGdtYWlsLmNvbQ==