- 1Eco-Efficiency Research Group, Agricultural Research and Education Centre Raumberg-Gumpenstein, Irdning-Donnersbachtal, Austria
- 2Life Cycle Assessment (LCA) Research Group, Agroscope, Zurich, Switzerland
Extensive dairy production in less favorable production areas has a long tradition in Austria. Nevertheless, dairy production also contributes considerable environmental impacts (EIs), e.g., greenhouse gas emissions, nutrient losses, and land use. Therefore, 20 organic dairy farms located in the Lungau region in Austria were assessed concerning their EIs via life cycle assessment (LCA). Cumulative exergy demand (CExD), normalized eutrophication potential (EP), aquatic ecotoxicity potential (AE), and global warming potential (GWP) were considered as impact categories to describe the farms' EIs. The farms were part of a pilot project aiming to produce high-quality dairy products and keep production cycles closed within the project region. Consequently, the purchase of key off-farm resources was only possible within the project region. We adapted existing life cycle inventories to account for those regional resource purchases. Subsequently, the EIs of the 20 farms were related to the functional units (FUs) of 1 kg energy-corrected milk (ECM) and 1 ha agricultural area for milk production and compared to a representative model dairy farm (MDF) that was created based on statistical data and average production values of organic Austrian dairy farms. Compared to the MDF, results show an ~58% lower EP per ha and 44% per kg ECM of the Lungau farms. Further, the CExD per ha was about 24% lower due to a lower use of resources caused by the lower production intensity of the Lungau farms. Regarding GWP, Lungau farms are favorable considering 1 ha as the FU, whereas the MDF seems advantageous if 1 kg ECM is used as the FU. However, caused by a high variation of purchased roughage and the lower production intensity, the Lungau farms cause higher AE, regardless of the FU. Overall, we identified three principal production parameters determining the environmental performance of milk production in a closed production cycle in a less favorable area, namely, (1) the stocking rate, (2) the fed concentrate, and (3) the purchased roughage. Using those inputs at moderate intensity, the extensively managed Lungau farms can competitively contribute to producing food, thus highlighting the importance of site-adapted agriculture.
Introduction
In recent years, modern agriculture has been facing a seemingly paradoxical challenge. On the one hand, the world population is expected to grow to 9.7 billion people by 2050 (UN, 2017), implying an increased food demand of about 70% compared to 2005–2007 (FAO, 2012). However, on the other hand, agriculture causes substantial environmental impacts (EIs) and contributes significantly to climate change (FAO, 2018).
Agriculture and especially livestock production currently account for about 24 and 14.5% of global anthropogenic greenhouse gas (GHG) emissions, respectively (FAO, 2018). Foley et al. (2011) identified both agricultural expansion [mainly in the tropics where it replaces forests (Gibbs et al., 2010)] and intensification as major contributors to climate change. Intensification has increased dramatically over the last decades and also caused increased energy use, degradation of aquatic ecosystems, and reduced biodiversity (Matson et al., 1997; Diaz and Rosenberg, 2008; Canfield et al., 2010; Foley et al., 2011).
An approach to decrease GHG emissions of agriculture is through the conversion from conventional to organic production (Lamine and Bellon, 2009). However, studies assessing milk production based on life cycle assessment (LCA) have shown that the reduced input use per kg of milk under organic production is offset by lower milk yields and lower feed conversion ratios, resulting in higher CH4 emissions per kg of milk than that under conventional systems (Tal, 2018; Smith et al., 2019). Conversely, organic systems perform better per unit of agricultural area (Pirlo and Lolli, 2019).
Related to milk production, extensively managed production systems are therefore emphasized to reduce EIs (Haas et al., 2001; Basset-Mens et al., 2009). Such production systems are based on grazing systems, especially on pastures unsuitable for other types of food production, such as arable farming (Foley et al., 2011), and characterized by a decreased use of purchased inputs (e.g., fertilizers, concentrate feed, or energy) and lower stocking rates (Haas et al., 2001; Basset-Mens et al., 2009; Horn et al., 2014).
The resource use and the EIs throughout the life cycles of products or services are generally assessed through LCA (Klöpffer and Grahl, 2009). Thus, LCA helps identify environmental hotspots and allows to derive options to improve the environmental performance of a production system (ISO, 2006a,b). Accordingly, numerous studies have assessed milk production systems through LCA. For a detailed review of recent LCA applications in the dairy sector, see Baldini et al. (2017).
This study aimed to assess the resource use and EIs of milk production of 20 organic dairy farms in a less favorable production area in Austria, which participated in a pilot project that aimed to produce high-quality dairy products and was dedicated to keeping nutrient cycles as closed as possible. Thus, the handling of key inputs is restricted because they have to be purchased from the project region. Therefore, the further goal of the study was to compare the resource use and EIs of the 20 farms to a model dairy farm (MDF) representative for organic milk production in Austria to assess the environmental performance of milk production in a less favorable production area in Austria and its principal determining production parameters.
Materials and Methods
Farm Data and Study Region
A set of 20 organically managed farms was evaluated in 2018 for this study. The farms were part of the pilot project “Reine Lungau,” which aimed to produce high-quality dairy products and was dedicated to keeping nutrient cycles as closed as possible within the project region. For this purpose, all farm inputs that can be produced in the project region must also be purchased from this area, e.g., feedstuffs, animals, and organic fertilizers. According to Austria's farm classification system (Binder et al., 2015), the farms are denoted as dairy farms. A description of key production parameters of the 20 Lungau farms is given in Table 1.
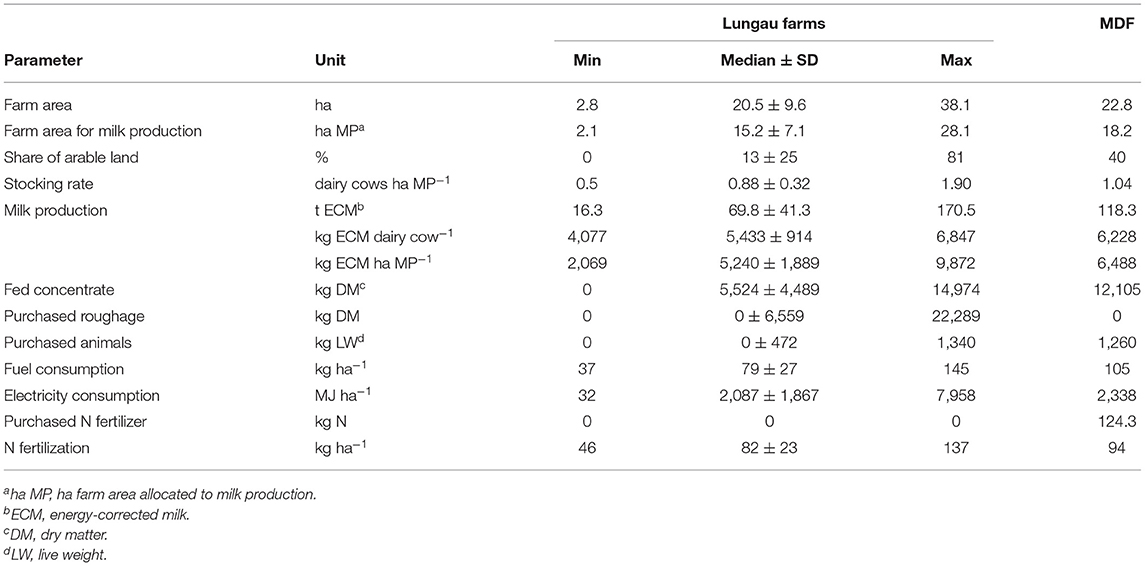
Table 1. Description of key production parameters of the 20 Lungau farms and the model dairy farm (MDF).
The farms are located in the Lungau region, which complies with the district of Tamsweg and is part of the federal province of Salzburg (Figure 1). According to Huber and Arnberger (2021), the region is characterized by forests, alpine pastures, extensive grassland, lakes, and wetlands, and the agricultural sector is dominated by a high proportion of small-scale organic farms. In addition, Figure 1 indicates that some parts of the study region are also related to arable land, which is mainly used to grow cereals like barley, triticale, or rye for concentrate feed or to cultivate potatoes as cash crop. Generally, unfavorable natural landscape conditions occur in the Lungau region, with low mean annual values of precipitation (774 mm) and temperature (5.2°C) (ZAMG, 2021) and a short vegetation period (180°C−220 days depending on altitude) (Schaumberger and Formayer, 2008).
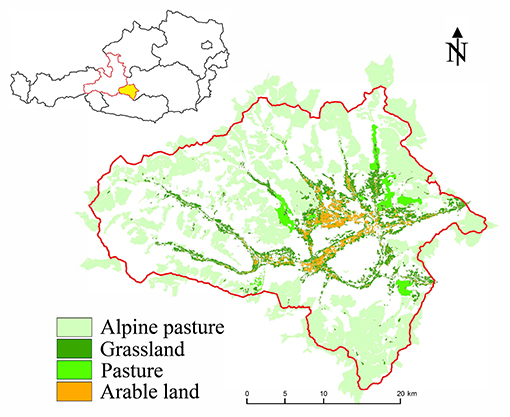
Figure 1. Location of the study region (Lungau) in Austria and agricultural land use based on CORINE land cover data (CLC, 2020).
Model Dairy Farm
In order to have a production system to serve as a representative reference for Austrian organic dairy farms, we compiled output and input data for an MDF. We derived the data from (i) national databases and complemented additional data based on (ii) specific models and (iii) expert judgments. Regarding national databases (i), we selected the total number of organic dairy farms in 2018 and calculated average values to be considered as inventory. Animal categories and numbers, the farm area, the grown crops, and types of grassland uses were derived from the Austrian Integrated Administration and Control System (IACS) (EC, 2021). Output parameters like the yield of milk, crops, and grassland stem from the annual report from the Austrian federal ministry of agriculture, regions, and tourism on the situation of the Austrian agriculture and forestry (BMNT, 2019). (ii) The feed ration was calculated in two steps: first, the amounts of on-farm roughage and concentrate were calculated based on the given grown crops and grassland types. In step two, we adapted the feed ration with purchased (off-farm) concentrate according to the given milk yield. (iii) Expert judgments were used to define the share of pasture intake in the feed ration and estimate the used infrastructure (buildings, equipment, and machinery). Key production parameters of the MDF are also given in Table 1.
LCA
Definition of Goal and Scope
This study aims to assess the resource use and EIs related to milk production of 20 extensively managed organic dairy farms from the Lungau region in Austria. Further, the resource use and EIs of the Lungau farms are compared to the resource use and EIs of a MDF, which is modeled as described in Section MDF and depicts the production system of the average Austrian organic dairy farm.
According to dairy farms being multifunctional systems that, besides producing food, also generate income for farmers and provide environmental services (O'Brien et al., 2012; Grassauer et al., 2021), we consider two functional units in this study: (1) 1 kg of energy-corrected milk (ECM) and (2) 1 ha of agricultural land allocated to milk production (ha MP).
The scope of the study comprises on-farm activities as well as upstream processes related to purchased inputs (off-farm). The considered system boundaries of the Lungau farms are set at the farm gate (i.e., from cradle to farm gate) and are depicted in Figure 2. We considered the whole farm area assigned to milk production as the physical limit and one calendar year as the temporal limit of the system under study. According to the regulations of the pilot project, feedstuffs (except mineral feed, cattle salt, and feeding lime), animals, and organic fertilizers must only be sourced from the study region. Other inputs (energy carriers, buildings and equipment, machinery, and seed) can be purchased according to the applicable Austrian organic production regulations (BIO-AUSTRIA, 2021). Due to limited data availability, we could not take the production and application of cleaning and disinfection agents and veterinary drugs into account.
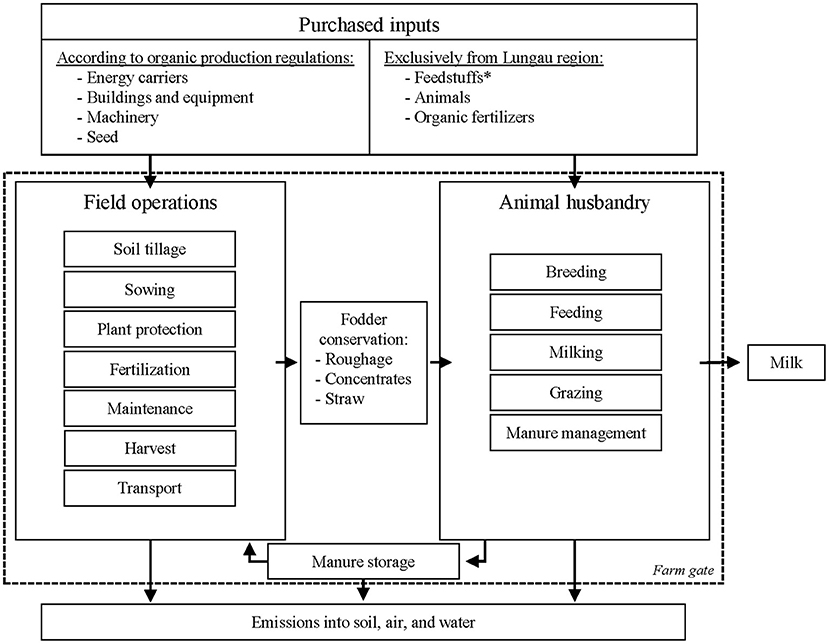
Figure 2. System description of the dairy farms from the Lungau region. *Except for mineral feed, cattle salt, and feeding lime.
The applied allocation procedure to assign the EIs and resource use to the product group milk production is based on physical and monetary criteria and follows a hierarchical process as described in Pedolin et al. (2021):
I. If possible, the whole impact was assigned to the product group milk production via causal relation (i.e., indirect emissions from the milking parlor are fully assigned to milk production). In these cases, no allocation is necessary.
II. If an impact could not be assigned causally, the allocation was based on physical criteria (i.e., livestock units for animal products and farm area for cop products).
III. If physical criteria were not sensible (e.g., when allocating between multiple diverse product groups), monetary criteria were used. If the allocation was necessary, the following distinction was made.
IV. Direct field emissions: one allocation key per field (i.e., one allocation factor for each potential product group).
V. Indirect emissions from energy carriers, buildings and equipment, machinery, seed, feedstuffs, animals, organic fertilizers, and direct animal emissions: one allocation factor for each potential product group.
Life Cycle Inventory
The life cycle inventory (LCI) stage of an LCA comprises the compilation and quantification of the inputs and outputs of a given product throughout its life cycle (ISO, 2006a). Direct emissions from on-farm activities were assessed through several models that were adapted to Austrian conditions by Herndl et al. (2015): (i) Emissions of phosphorous were estimated based on the work from Prasuhn (2006) and cover PO deposits into surface waters through soil erosion, drainage, and surface run-off, and into groundwater by leaching. (ii) The leaching of nitrate was assessed based on Richner et al. (2014), which consider the monthly mineralization of nitrogen depending on the soil type, tillage activities and fertilization rates, and the nitrogen uptake from different crops and grassland types. (iii) The accumulation of heavy metals in soil and water was computed based on the methodology from Freiermuth (2006). The model was refined by adding values for heavy metal contents in Austrian soils (Umweltbundesamt, 2004) and Austrian heavy metal deposition rates (Zechmeister et al., 2009). (iv) Emissions related to animal husbandry cover the enteric fermentation of ruminants (CH4) and emissions from the stable (NH3), exercise area (NH3, CH4), and pasture (NH3, CH4, N2O, NOx, NO). (v) Finally, emissions from manure management (NH3, N2O, NOx, CH4) and manure application (NH3, N2O, NOx, P, NO) were considered. Both (iv) and (v) were assessed based on Menzi et al. (1997) (NH3), Nemecek and Kägi (2007) (NOx), and IPCC Tier 2 models (Dong et al., 2006) (N2O, CH4). The assessed direct emissions from on-farm activities (excluding the accumulation of heavy metals in soil and water) of the 20 Lungau farms and the MDF are presented in Supplementary Table 1.
Indirect, off-farm emissions from upstream processes related to purchased inputs were estimated through eco-inventories from the SALCA database (Gaillard and Nemecek, 2009) and ecoinvent database version 3.5 (Weidema et al., 2013). However, as shown in Figure 2, some purchased inputs (feedstuffs, animals, and organic fertilizer) must stem from the Lungau region according to the regulations of the pilot project. Thus, we adapted existing Swiss eco-inventories for organic agriculture. More specifically, we adapted eco-inventories for the purchase of barley grain, wheat grain, rye grain, grass silage, hay, and calves. The adaptations included a reduction of the transportation effort to account for transportation just inside the study region and reduction in the amount of fertilizer, agricultural machinery, and irrigation based on primary data to reflect the extensive management. A comparison of the EIs of the existing and adapted eco-inventories is given in Supplementary Table 2.
Life Cycle Impact Assessment
Building on the LCI, the life cycle impact assessment (LCIA) transforms the direct and indirect emissions and resource use into several EIs (EC, 2010). According to Nemecek et al. (2011), there are three dimensions of EIs which represent different management options: (i) resource management, (ii) nutrient management, and (iii) pollutant management. Based on these dimensions and following the rationale of Grassauer et al. (2021), we selected the following EIs to be considered within this study: (i) cumulative exergy demand (CExD) (Bösch et al., 2007; Alvarenga et al., 2013), (ii) global warming potential 100 years (GWP) (IPCC, 2013), (iii) normalized eutrophication potential (EP) (Hauschild and Potting, 2005), and (iv) aquatic ecotoxicity potential (AE) (Guinée et al., 2001; Kägi et al., 2008; Hayer et al., 2010).
CExD is a comprehensive energy-based indicator for resource demand that aggregates different forms of energy resources into a single indicator (Bösch et al., 2007). GWP is based on the cumulative radiative forcing of various substances over a time horizon of 100 years and gives values relative to those for the reference gas CO2 (IPCC, 2013). EP comprises eutrophication indicators for aquatic and terrestrial N eutrophication and aquatic P eutrophication (Hauschild and Potting, 2005). Using average European emissions from 2004, these eutrophication indicators are normalized, aggregated, and measured in person year−1 (Laurent et al., 2011). Finally, AE assesses the effects of the accumulation of heavy metals in the soil and water.
Due to its integration of several LCIA methods and its specific reflection of the agricultural sector (Gaillard and Nemecek, 2009), SALCA 1.12 was selected as the impact assessment method in this study. The computation of the LCIA was done with SimaPro Developer software version 9.0.0.49 (Pré Consultants, 2019).
Interpretation
For interpretation, the LCA results were broken down into ten different sources: land use, fertilizers, and field emissions; animal husbandry; buildings and equipment; machinery; energy carriers; seed; purchased animals; purchased roughage; purchased concentrate; and other inputs. Further, the LCA results were related to the two functional units as described in Section Definition of Goal and Scope.
Results
The absolute values of the four considered EIs (CExD, GWP, EP, and AE) comprising direct and indirect emissions of the 20 Lungau farms and the MDF are given in Supplementary Table 3.
Figure 3 shows the contribution of the ten different sources to the four considered EIs (CExD, GWP, EP, and AE) of the Lungau farms compared to the MDF. The bar of the Lungau farms shows the mean EIs of the 20 farms under study.
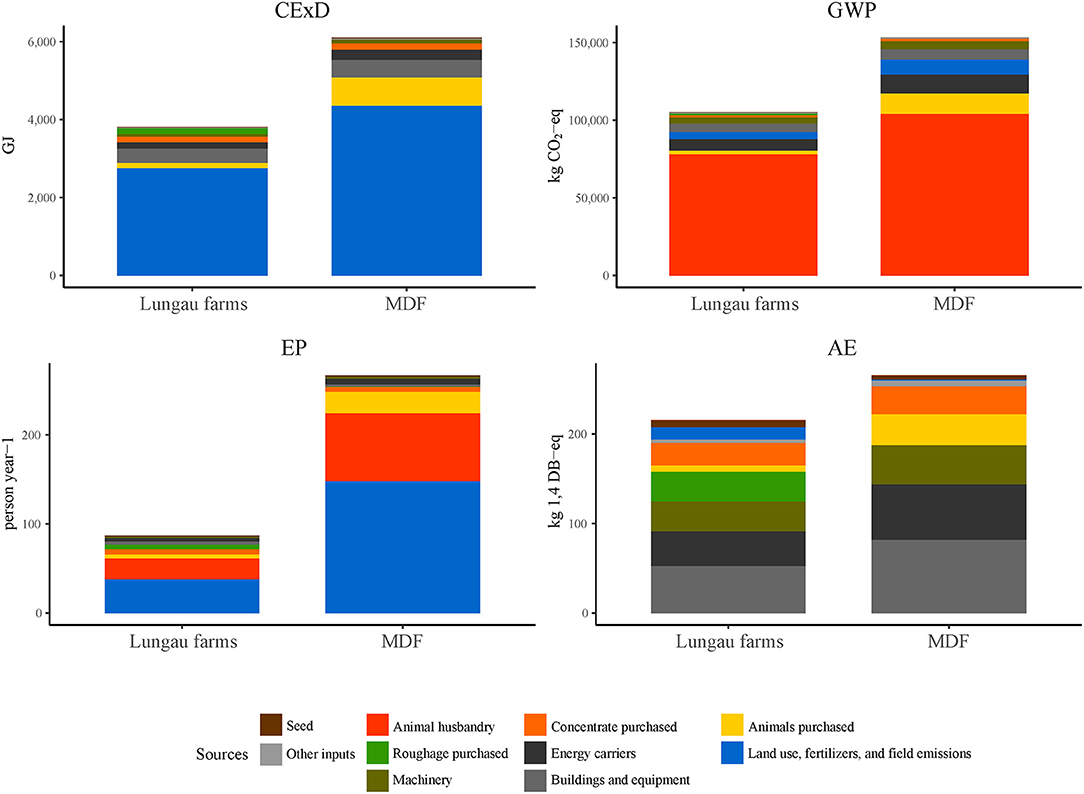
Figure 3. Mean absolute EIs [cumulative exergy demand (CExD), global warming potential 100 years (GWP), normalized eutrophication potential (EP), and aquatic ecotoxicity potential (AE)] of the 20 Lungau farms compared to the model dairy farm (MDF), showing the contribution of the ten different sources.
Compared to the MDF (6,100 GJ), the Lungau farms have a 38% lower CExD (3,800 GJ). The main contributor to CExD is land use, fertilizers, and field emissions, with a share of 72% in both bars. The purchased animals cause another 12% of CExD on the MDF but contribute only 3% on the Lungau farms.
Regarding GWP, the difference between Lungau farms (105,134 kg CO2-eq) and the MDF (153,242 kg CO2-eq) is 31%. Emissions from animal husbandry are the highest contributing source to GWP, with shares of 74% for Lungau farms and 68% on the MDF. Again, emissions from purchased animals contribute another 9% of GWP on the MDF but only 2% on the Lungau farms. Another considerable source of GWP is energy carriers (comprising fuel and electricity consumption), adding 7 and 8% of GWP to the Lungau farms and the MDF, respectively.
The highest difference between Lungau farms and the MDF was found for EP. Whereas, the Lungau farms cause a total of 87 person year−1, the MDF causes 267 person year−1, resulting in a difference of 67%. This difference is mainly caused by lower contributions of critical sources of EP. For example, land use, fertilizers, and field emissions contribute 55% to EP on the MDF and 44% within the Lungau farms. Similarly, eutrophication through animal husbandry causes 29% of EP on the MDF and 26% on the Lungau farms.
Conversely, the lowest difference was found for AE at 19%, with Lungau farms emitting a mean of 216 kg 1,4 DB-eq and the MDF causing 266 kg 1,4 DB-eq. The main sources of AE were buildings and equipment with 24% within Lungau farms and 31% on the MDF, energy carriers with 18% on the Lungau farms, and 23% on the MDF.
In Figure 4, the EIs were related to the two considered functional units (i.e., kg ECM on the y-axis and ha MP on the x-axis) and presented as double boxplots with the colored dots showing the median values of the Lungau farms (blue) and the values of the MDF (orange), and the gray area indicating the upper and lower quartiles of the Lungau farms.
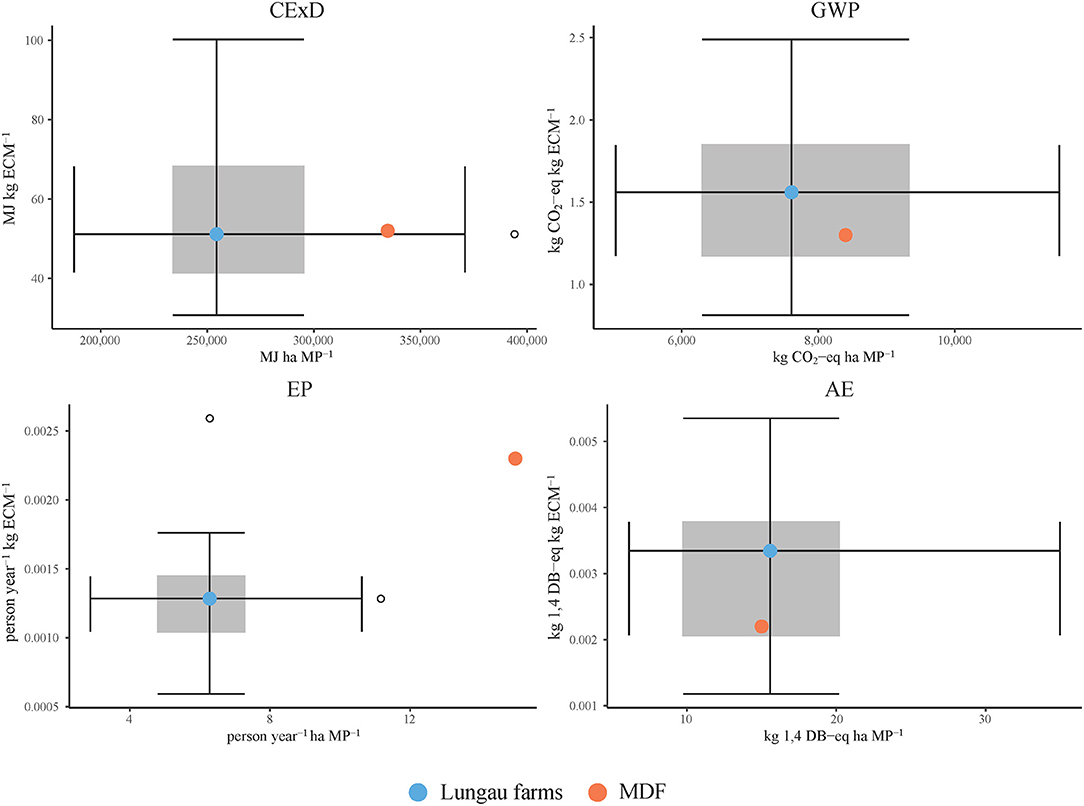
Figure 4. Double boxplots of the EIs per kg ECM (y-axis) and ha MP (x-axis) with the colored dots indicating the median values of the Lungau farms (blue) and the values of the MDF (orange), and the gray area indicating the upper and lower quartiles of the Lungau farms.
Per kg ECM, the Lungau farm's CExD ranges from 31 to 100 MJ with a median of 51 MJ, which is slightly lower than the MDF with 52 MJ. However, when expressed per ha MP, the difference in CExD is higher (24%), with Lungau farms having a demand of 254,426 MJ (with a range of 187,561 to 394,123 MJ) and the MDF demanding 334,663 MJ.
For GWP, we found that the favorable farming system depends on the considered functional unit. Expressed per kg ECM, the MDF seems favorable with 1.3 kg CO2-eq compared to the Lungau farms with a median of 1.6 and a range of 0.8–2.5 kg CO2-eq. Conversely, when considering ha MP as the functional unit, the Lungau farms emitted 7,609 kg CO2-eq (with a range of 5,035–11,533 CO2-eq), around 9% less than the MDF (8,401 kg CO2-eq).
Considering EP and regardless of the functional unit, the Lungau farms cause a considerably lower EP. The difference is roughly 44% per kg ECM, with the Lungau farms emitting a median of 0.0013 person year−1 (with a range of 0.0006–0.0026 person year−1), whereas the MDF emits 0.0023 person year−1. Per ha MP, the Lungau farms emit 2.9–11.2 person year−1 with a median of 6.3 person year−1, which results in a difference of 58% compared to the MDF (15 person year−1).
The favorable farming system regarding AE is the MDF, but the differences show a high margin depending on the considered FU. Per kg ECM, the MDF emits 0.0022 kg 1,4 DB-eq, which is 34% lower than the median value of the Lungau farms (0.0033 kg 1,4 DB-eq). Considering 1 ha MP as the functional unit, the AE (kg 1,4 DB-eq) amounts to 15 for the MDF and a median of 15.6 for the Lungau farms, which equals a difference of only 4%.
A summary of the EIs is given in Figure 5, which presents normalized environmental profiles of the Lungau farms (blue) and the MDF (orange) per (a) kg ECM and (b) ha MP with the respective normalized values. We present the median values for the Lungau farms, and the EIs were normalized to the maximum value to indicate EIs, where further action of the Lungau farms might be needed.
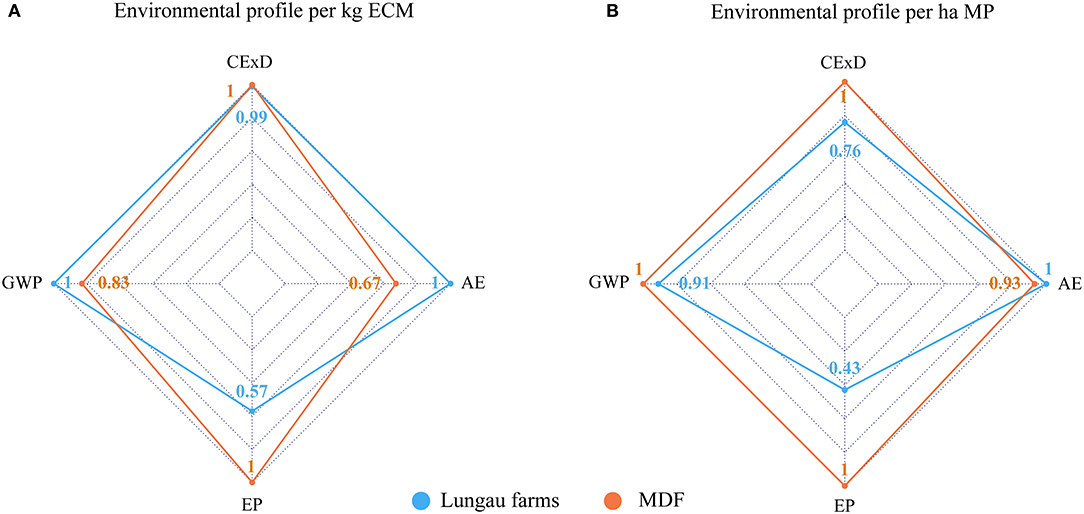
Figure 5. Normalized environmental profiles of the Lungau farms (blue) and the MDF (orange) per (A) kg ECM and (B) ha MP.
Per kg ECM, the Lungau farms are favorable regarding CExD and EP, whereas the MDF is beneficial concerning GWP and AE. Conversely, considering the functional unit of 1 ha MP, the Lungau farms perform better regarding three out of four EIs (i.e., CExD, GWP, and EP).
Discussion
In Figure 3, we compared the mean value of the absolute EIs of the Lungau farms with the MDF broken down into ten different sources and found the values of the Lungau farms to be lower for each of the considered EIs. To set the EIs of the MDF into perspective, we compared them to 12 Austrian organic dairy farms from the study of Grassauer et al. (2021), who assessed the EIs of organic dairy farms distributed to all of Austria under similar system boundaries (Table 2). The comparison revealed that the MDF is below the mean but within the range of the EIs.
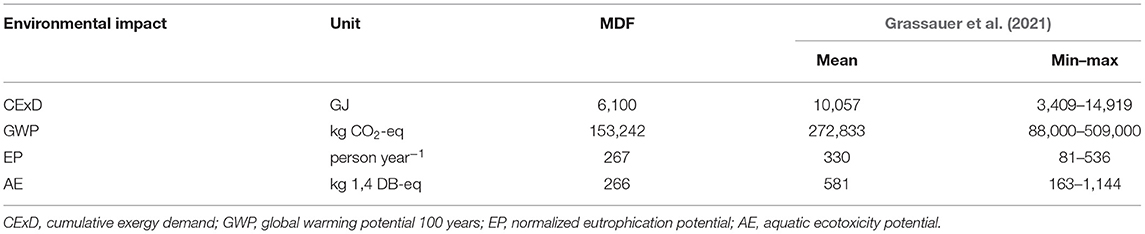
Table 2. Comparison of the absolute EIs of the MDF with EIs of 12 Austrian organic dairy farms from Grassauer et al. (2021).
Looking at the CExD, the most apparent difference between the Lungau farms and the MDF is the source land use, fertilizers, and field emissions, which usually account for a high share of CExD in agricultural production systems (Dewulf et al., 2005; Alvarenga et al., 2013). The difference results from the median Lungau farm cultivating less farm area than the MDF (20.5 and 22.8 ha, respectively; Table 1), which indicates the small-structured agriculture common in the inner-alpine region of Austria (BMNT, 2019).
Regarding GWP, the primary source is animal husbandry which accounts for emissions from manure management (N2O, CH4) and enteric fermentation in ruminants (CH4), with the majority originating from the latter (Ogino et al., 2007; Dick et al., 2015; Doltra et al., 2018; Gislon et al., 2020). Therefore, the lower GWP from animal husbandry of the Lungau farms is caused by the lower stocking rates (0.88 and 1.04 dairy cows ha MP−1 for Lungau farms and MDF, respectively; Table 1), which lead to less enteric fermentation and lower emissions from manure management (Dong et al., 2006).
The lower stocking rate of the Lungau farms also explains the difference in the source animal husbandry regarding EP by causing less aquatic and terrestrial N eutrophication through ammonia (NH3) and nitrogen oxides (NOx) (Menzi et al., 1997; Nemecek and Kägi, 2007), respectively. Further, the lower stocking rate of the Lungau farms leads to a lower N fertilization per farm area (Table 1), which, in turn, lowers the emissions from the source land use, fertilizers, and field emissions through reduced N2O from direct field emissions (Dong et al., 2006) and lower NO from manure application (Richner et al., 2014). Another reason for the higher EP value from land use, fertilizers, and field emissions of the MDF is the purchase of 124.3 kg N fertilizer and the related indirect fertilizer production emissions (Herndl et al., 2015).
The AE comprises the accumulation of heavy metals in water and soil (Freiermuth, 2006). A relevant difference between the Lungau farms and the MDF is the share of emissions from the source energy carriers. Since the production of energy carriers, especially diesel, is considered a major contributor to heavy metal emissions (Berlin, 2002), the difference can be explained by the lower fuel consumption of the Lungau farms, which is caused by a lower share of arable land (Table 1). Another significant difference in AE arises from the import of heavy metals through the purchase of roughage which is practiced by some of the Lungau farms but does not happen at the MDF (Table 1).
Due to the crucial importance of the choice of the LCIA method (Hauschild et al., 2013), we conducted uncertainty analyses of the calculated EIs in order to improve the transparency and robustness of the obtained results (Guo and Murphy, 2012). Considering the statistical distribution of all upstream processes, we conducted a Monte Carlo simulation with 1,000 iterations for all 20 Lungau farms and the MDF. The results of the uncertainty analyses are shown in Supplementary Figure 1 and indicate the highest uncertainties for the AE. This can be explained by a certain degree of uncertainty regarding heavy metal emissions (Pizzol et al., 2011a,b). Due to a high degree of confidence in LCA results related to resource-related EIs and climate change (Niero et al., 2014), the remaining impact categories (CExD, EP, and GWP) show considerably lower uncertainty.
As depicted in Figure 4, the CExD per kg ECM of the Lungau farms ranges from 31 to 100 MJ, with a median of 51 MJ. Since the CExD is highly related to land use (Dewulf et al., 2005; Alvarenga et al., 2013), this variation can be explained by the milk production yield per ha MP. We conducted a correlation analysis between the two parameters and found that the CExD per kg ECM correlates highly significant with the milk production yield per ha MP (Pearson's r = −0.80; p = 2.76e−05). Per 1 ha MP, the CExD of the Lungau farms amounts to a median of 254,426 MJ and the MDF reaches 334,663 MJ. Due to limited evidence in the literature, we compared these values with the work from Huysveld et al. (2015), who assessed the resource use of an intensively managed (10,542 kg fat- and protein-corrected milk (FPCM) per cow) Belgian model dairy farm and reported a CExD of 28.3 MJ kg FPCM−1. According to our recalculation, this results in a CExD of 545,266 MJ ha MP−1. This value is significantly higher than the values obtained in this study (254,426 and 334,663 MJ ha MP−1 for Lungau farms and MDF, respectively) and can be related to the management intensity as producing more milk in the same area leads to a higher exergy demand per area.
The median value of GWP per kg ECM of the Lungau farms (1.6 kg CO2-eq) is 19 % higher compared to the MDF (1.3 kg CO2-eq). These values are comparable with the results of Hersener et al. (2011), who analyzed the GWP of Swiss organic dairy farms over 3 years under similar system boundaries and reported a median of 1.4 kg CO2-eq per kg milk (with a range of 1.2–2 kg CO2-eq). However, the range of GWP per kg ECM in this study is significantly higher (0.8–2.5 kg CO2-eq) and especially the lower bound seems hard to attain. Nonetheless, Cederberg and Flysjö (2004) reported a mean value of 0.94 kg CO2-eq kg ECM−1 within six Swedish organic dairy farms. Moreover, it should be noted that these low values only occur on Lungau farms with a large share of intensive continuous grazing, which is known to reduce GWP on ruminant keeping farms (Alemu et al., 2017). The range of GWP per ha MP of the Lungau farms (5,035–11,533 kg CO2-eq) corresponds to the findings of Bystricky et al. (2015), who reported a range of 5,000–12,000 kg CO2-eq ha−1 of 12 Austrian organic dairy farms under similar system boundaries.
Due to limited evidence in the literature, the values and ranges of EP both per kg ECM and per ha MP were compared to the findings of Grassauer et al. (2021), who reported median values of 0.0025 (within a range of 0.0017–0.0035) and 10.3 (within a range of 7.6–11.4) person year−1 per kg ECM and ha MP, respectively. The significantly lower EP values of the Lungau farms can mainly be related to lower stocking and N-fertilization rates as manure application and nitrate leaching are the main contributors to EP (O'Brien et al., 2012).
The values of AE per kg ECM (0.0033 and 0.0022 kg 1,4 DB-eq for the Lungau farms and the MDF, respectively) are substantially higher compared to the findings of Arsenault et al. (2009), who assessed Canadian dairy farms of two farming systems (confinement and pasture-based) and reported values of 0.0014 and 0.0013 kg 1,4 DB-eq, respectively. Although the Canadian farms operated conventional, Arsenault et al. (2009) did not consider the use of pesticides, which could have led to values 50 times higher (Knudsen et al., 2019). It should further be noted that Arsenault et al. (2009) assessed intensively managed dairy farms with milk yield averages well over 9,000 kg cow−1 leading to lower AE per kg milk. Although the difference in AE per ha MP between the Lungau farms and the MDF seems negligible, there is a considerable variation of values ranging from 6.1 to 35 kg 1,4 DB-eq, which can be attributed to the high variance of purchased roughage (0–22.3 t DM; see Table 1).
The adaption of six eco-inventories (i.e., barley grain, wheat grain, rye grain, grass silage, hay, and calves) as presented in Supplementary Table 2 shows differences up to 13% depending on the considered EI. On the one hand, these differences are caused by the amended transport distances, which only have minor importance on eco-inventories of organic feedstuffs and are subject to high uncertainty (Nemecek and Kägi, 2007). On the other hand, the reduced application of fertilizers leads to lower resource use (CExD) and a reduced accumulation of heavy metals (AE). However, when upscaled to the farm level, the differences in the EIs were even lower.
Summarizing the EIs related to the two considered FUs, as shown in Figure 5, and besides the unfavorable natural landscape conditions in the study region, we identify three principal production parameters describing the closed regional production:
1. The stocking rate influences (i) the milk yield per ha MP and, therefore, the CExD, (ii) indirectly the N fertilization, which further affects the EP, and (iii) the GWP per ha MP.
2. The fed concentrate is highly correlated with the milk yield per cow, thus influencing all EIs considering one kg ECM as the functional unit.
3. The purchased roughage leads to an import of heavy metals, therefore affecting the AE.
The Lungau farms operate at a lower level regarding two of the three mentioned production parameters (the high range of purchased roughage is due to different site conditions and limitations of pasture and concentrate). This moderate use of inputs is consistent with the overall unfavorable natural landscape conditions in the Lungau region, and the comparison with the average Austrian organic dairy farm (operating on a more intensive level regarding inputs) showed that such extensive production systems can competitively contribute to producing food and providing environmental services by performing site-adapted agriculture.
Conclusions
This study assessed the resource use and EIs of 20 Austrian organic dairy farms located in the Lungau region and compared them with an average Austrian organic model dairy farm to determine the main factors influencing the environmental performance of milk production in a closed regional production system. Considering 1 kg ECM as the functional unit (FU), results of farm LCAs indicated that the Lungau farms are favorable regarding CExD and EP, whereas the MDF emitted lower values of GWP and AE. However, we also related the EIs to one ha MP as the second considered FU, which led to the Lungau farms being favorable in three out of four categories (CExD, GWP, and EP). Therefore, we conclude that the choice of FU is crucial when comparing different production systems, thus highlighting the integration of multiple FUs and taking the multifunctionality of agriculture into account. Further, we identified three principal management parameters determining the environmental performance of milk production in a closed production cycle in a less favorable area, namely, (1) the stocking rate, (2) the fed concentrate, and (3) the purchased roughage. Using these inputs at moderate intensity, the extensively managed Lungau farms can competitively contribute to producing food and providing environmental services from an environmental point of view. Therefore, the unfavorable natural landscape conditions in the Lungau region and the extensive management with moderate use of inputs highlight the importance of site-adapted agriculture.
Data Availability Statement
The datasets presented in this study can be found in online repositories. The names of the repository/repositories and accession number(s) can be found below: doi: 10.5281/zenodo.5709472.
Author Contributions
FG: conceptualization, data curation, methodology, visualization, and writing—original draft. MH: conceptualization, methodology, and writing—review and editing. LI: software, methodology, and writing—review and editing. GG: writing—review and editing and supervision. All authors contributed to the article and approved the submitted version.
Funding
This research was supported by the project Circular Agronomics funded by the European Union as part of the Horizon 2020 Research and Innovation Programme under Grant Agreement No. 773649.
Conflict of Interest
The authors declare that the research was conducted in the absence of any commercial or financial relationships that could be construed as a potential conflict of interest.
Publisher's Note
All claims expressed in this article are solely those of the authors and do not necessarily represent those of their affiliated organizations, or those of the publisher, the editors and the reviewers. Any product that may be evaluated in this article, or claim that may be made by its manufacturer, is not guaranteed or endorsed by the publisher.
Acknowledgments
The authors want to thank Dr. T. Guggenberger for successfully leading the demanding data collectionprocess.
Supplementary Material
The Supplementary Material for this article can be found online at: https://www.frontiersin.org/articles/10.3389/fsufs.2022.817671/full#supplementary-material
References
Alemu, A. W., Janzen, H., Little, S., Hao, X., Thompson, D. J., Baron, V., et al. (2017). Assessment of grazing management on farm greenhouse gas intensity of beef production systems in the Canadian Prairies using life cycle assessment. Agricul. Syst. 158, 1–13. doi: 10.1016/j.agsy.2017.08.003
Alvarenga, R. A. F., Dewulf, J., Van Langenhove, H., and Huijbregts, M. A. J. (2013). Exergy-based accounting for land as a natural resource in life cycle assessment. Int. J. Life Cycle Assessm. 18, 939–947. doi: 10.1007/s11367-013-0555-7
Arsenault, N., Tyedmers, P., and Fredeen, A. (2009). Comparing the environmental impacts of pasture-based and confinement-based dairy systems in Nova Scotia (Canada) using life cycle assessment. Int. J. Agricul. Sustain. 7, 19–41. doi: 10.3763/ijas.2009.0356
Baldini, C., Gardoni, D., and Guarino, M. (2017). A critical review of the recent evolution of Life Cycle Assessment applied to milk production. J. Cleaner Produc. 140, 421–435. doi: 10.1016/j.jclepro.2016.06.078
Basset-Mens, C., Ledgard, S., and Boyes, M. (2009). Eco-efficiency of intensification scenarios for milk production in New Zealand. Ecol. Econ. 68, 1615–1625. doi: 10.1016/j.ecolecon.2007.11.017
Berlin, J. (2002). Environmental life cycle assessment (LCA) of Swedish semi-hard cheese. Int. Dairy J. 12, 939–953. doi: 10.1016/S0958-6946(02)00112-7
Binder, J., Fensl, F., and Gahleitner, G. (2015). Das österreichische Klassifizierungssystem für land- und forstwirtschaftliche Betriebe basierend auf dem Standardoutput. AWI - Bundesanstalt für Agrarwirtschaft, Vienna, Austria.
BIO-AUSTRIA (2021). Produktionsrichtlinien. Available online at: https://www.bio-austria.at/app/uploads/2021/06/Richtlinien_2021_Juni_AKTUELL_neueSchrift_klein.pdf (accessed 22, 09, 2021).
BMNT (2019). Grüner Bericht. Die Situation der österreichischen Land- und Forstwirtschaft. Bundesministerium für Nachhaltigkeit und Touismus, Vienna, Austria.
Bösch, M. E., Hellweg, S., Huijbregts, M. A. J., and Frischknecht, R. (2007). Applying cumulative exergy demand (CExD) indicators to the ecoinvent database. Int. J. Life Cycle Assessm. 12:181. doi: 10.1065/lca2006.11.282
Bystricky, M., Herndl, M., and Baumgartner, D. (2015). Umweltwirkungen der Projektbetriebe, Abschlusstagung des Projektes FarmLife. Höhere Bundeslehr- und Forschungsanstalt für Landwirtschaft Raumberg-Gumpenstein, HBLFA Raumberg-Gumpenstein, Austria, 41–52.
Canfield, D. E., Glazer, A. N., and Falkowski, P. G. (2010). The evolution and future of earth's nitrogen cycle. Science 330, 192–196. doi: 10.1126/science.1186120
Cederberg, C., and Flysjö, A. (2004). Life Cycle Inventory of 23 Dairy Farms in South-Western Sweden. Swedish Institute for Food and Biotechnology. Gothenburg, Sweden, 63.
CLC (2020). Corine Land Cover (CLC). Version 2020_20u1. Available online at: https://land.copernicus.eu/pan-european/corine-land-cover/clc2018 (accessed June 01, 2021).
Dewulf, J., Van Langenhove, H., and Van De Velde, B. (2005). Exergy-based efficiency and renewability assessment of biofuel production. Environ. Sci. Tech. 39, 3878–3882. doi: 10.1021/es048721b
Diaz, R. J., and Rosenberg, R. (2008). Spreading dead zones and consequences for marine ecosystems. Science 321, 926–929. doi: 10.1126/science.1156401
Dick, M., Abreu da Silva, M., and Dewes, H. (2015). Life cycle assessment of beef cattle production in two typical grassland systems of southern Brazil. J. Cleaner Product. 96, 426–434. doi: 10.1016/j.jclepro.2014.01.080
Doltra, J., Villar, A., Moros, R., Salcedo, G., Hutchings, N. J., and Kristensen, I. S. (2018). Forage management to improve on-farm feed production, nitrogen fluxes and greenhouse gas emissions from dairy systems in a wet temperate region. Agricul. Syst. 160, 70–78. doi: 10.1016/j.agsy.2017.11.004
Dong, H., Mangino, J., McAllister, T. A., Hatfield, J. L., Johnson, D. E., Lassey, K. R., et al. (2006). Emissions from Livestock and Manure Management, in IPCC Guidelines for National Greenhouse Gas Inventories. Volume 4: Agriculture, Forestry and Other Land Use (Hayama: Institute for Global Environmental Strategies). Available online at: https://www.ipcc-nggip.iges.or.jp/public/2006gl/pdf/4_Volume4/V4_10_Ch10_Livestock.pdf
EC (2010). International Reference Life Cycle Data System (ILCD) Handbook - General guide for Life Cycle Assessment - Provisions and Action Steps. Publications Office of the European Union, Luxembourg,Luxembourg.
EC (2021). Integrated Administration and Control System (IACS). Available online at: https://ec.europa.eu/info/food-farming-fisheries/key-policies/common-agricultural-policy/financing-cap/financial-assurance/managing-payments_en (accessed October 11, 2021).
FAO (2018). FAO's Work on Climate Change. United Nations Climate Change Conference. Available online at: http://www.fao.org/3/CA2607EN/ca2607en.pdf (accessed May 20, 2021).
Foley, J. A., Ramankutty, N., Brauman, K. A., Cassidy, E. S., Gerber, J. S., Johnston, M., et al. (2011). Solutions for a cultivated planet. Nature 478, 337–342. doi: 10.1038/nature10452
Freiermuth, R. (2006). Modell zur Berechnung der Schwermetallflüsse in der Landwirtschaftlichen Ökobilanz - SALCA-Schwermetall (Zurich: Agroscope FAL Reckenholz), 38.
Gaillard, G., and Nemecek, T. (2009). Swiss Agricultural Life Cycle Assessment (SALCA): An Integrated Environmental Assessment Concept for Agriculture, AgSAP Conference (Netherlands: Egmond aan Zee), 134–135.
Gibbs, H. K., Ruesch, A. S., Achard, F., Clayton, M. K., Holmgren, P., Ramankutty, N., et al. (2010). Tropical forests were the primary sources of new agricultural land in the 1980s and 1990s. Proc. Natl. Acad. Sci. U.S.A. 107:16732. doi: 10.1073/pnas.0910275107
Gislon, G., Ferrero, F., Bava, L., Borreani, G., Prà, A. D., Pacchioli, M. T., et al. (2020). Forage systems and sustainability of milk production: feed efficiency, environmental impacts and soil carbon stocks. J. Cleaner Produc. 260:121012. doi: 10.1016/j.jclepro.2020.121012
Grassauer, F., Herndl, M., Nemecek, T., Guggenberger, T., Fritz, C., Steinwidder, A., et al. (2021). Eco-efficiency of farms considering multiple functions of agriculture: concept and results from Austrian farms. J. Cleaner Product. 297:126662. doi: 10.1016/j.jclepro.2021.126662
Guinée, J. B., Gorrée, M., Heijungs, R., Huppes, G., Kleijn, R., de Koning, A., et al. (2001). Life Cycle Assessment - An Operational Guide to the ISO Standards. Ministry of Housing, Spatial Planning and Environment (VROM) and Centre of Environmental Sciences (CML), Den Haag and Leiden, Netherlands.
Guo, M., and Murphy, R. J. (2012). LCA data quality: sensitivity and uncertainty analysis. Sci. Total Environ. 435–436, 230–243. doi: 10.1016/j.scitotenv.2012.07.006
Haas, G., Wetterich, F., and Köpke, U. (2001). Comparing intensive, extensified and organic grassland farming in southern Germany by process life cycle assessment. Agricul. Ecosyst. Environ. 83, 43–53. doi: 10.1016/S0167-8809(00)00160-2
Hauschild, M. Z., Goedkoop, M., Guinée, J., Heijungs, R., Huijbregts, M., Jolliet, O., et al. (2013). Identifying best existing practice for characterization modeling in life cycle impact assessment. Int. J. Life Cycle Assess. 18, 683–697. doi: 10.1007/s11367-012-0489-5
Hauschild, M. Z., and Potting, J. (2005). Spatial Differentiation in Life Cycle Impact Assessment – The EDIP. Methodology, Environmental news n. 80. The Danish Ministry of the Environment, Environmental Protection Agency, Copenhagen, Denmark.
Hayer, F., Bockstaller, C., Gaillard, G., Mamy, L., Nemecek, T., and Strassemeyer, J. (2010). Multi-criteria comparison of eco-toxicity models focused on pesticides, in 7th Int. Conf. on LCA in the Agri-Food Sector, ed Notarnicola (Bari), B., , 305–310.
Herndl, M., Baumgartner, D., Steinwidder, A., Guggenberger, T., Fasching, C., Gaillard, G., et al. (2015). Einzelbetriebliche Ökobilanzierung landwirtschaftlicher Betriebe in Österreich. Höhere Bundeslehr- und Forschungsanstalt für Landwirtschaft Raumberg-Gumpenstein, Irdning-Donnersbachtal, Austria, 99.
Hersener, J.-L., Baumgartner, D. U., Dux, D., Aeschbacher, U., Alig, M., Blaser, S., et al. (2011). Zentrale Auswertung von Ökobilanzen landwirtschaftlicher Betriebe (ZA-ÖB). Foschungsanstalt Agroscope Reckenholz-Tänikon ART, Zurich/Ettenhausen, Switzerland.
Horn, M., Steinwidder, A., Pfister, R., Gasteiner, J., Vestergaard, M., Larsen, T., et al. (2014). Do different cow types respond differently to a reduction of concentrate supplementation in an Alpine low-input dairy system? Livestock Sci. 170, 72–83. doi: 10.1016/j.livsci.2014.10.006
Huber, M., and Arnberger, A. (2021). Factors influencing the level of local participation in planning and management of the planned Salzburger Lungau andamp; Kärntner Nockberge Biosphere Reserve in Austria. Sustainability 13:9685. doi: 10.3390/su13179685
Huysveld, S., Van linden, V., De Meester, S., Peiren, N., Muylle, H., Lauwers, L., et al. (2015). Resource use assessment of an agricultural system from a life cycle perspective – a dairy farm as case study. Agricul. Syst. 135, 77–89. doi: 10.1016/j.agsy.2014.12.008
IPCC (2013). Climate Change. The Physical Science Basis. Working Group I Contribution to the Fifth Assessment Report of the Intergovernmental Panel on Climate Change. Cambridge University Press, Cambridge, United Kingdom and New York, USA.
ISO (2006a). ISO 14040:2006 Environmental Management - Life cycle assessment - Principles and Framework. International Organization for Standardization, Geneva, Switzerland.
ISO (2006b). ISO 14044:2006 Environmental Management - Lyfe cycle assessment - Requirements and Guidelines. International Organization for Standardization, Geneva, Switzerland.
Kägi, T., Bockstaller, C., Gaillard, G., Hayer, F., Mamy, L., and Strassemeyer, J. (2008). Multicriteria comparison of RA and LCA toxicity methods with focus on pesticide application strategies, in Proceedings of the 6th International Conference on LCA in the Agri-Food Sector – Towards a sustainable management of the Food chain, eds Nemecek, T., and Gaillard, G., (Zurich: Agroscope ART), 169–177.
Klöpffer, W., and Grahl, B. (2009). Ökobilanz (LCA). Ein Leitfaden für Ausbildung und Beruf. Weinheim: WILEY-VCH. doi: 10.1002/9783527627158
Knudsen, M. T., Dorca-Preda, T., Djomo, S. N., Peña, N., Padel, S., Smith, L. G., et al. (2019). The importance of including soil carbon changes, ecotoxicity and biodiversity impacts in environmental life cycle assessments of organic and conventional milk in Western Europe. J. Cleaner Produc. 215, 433-443. doi: 10.1016/j.jclepro.2018.12.273
Lamine, C., and Bellon, S. (2009). Conversion to organic farming: a multidimensional research object at the crossroads of agricultural and social sciences. A review. Agronomy Sustain. Dev. 29, 97–112. doi: 10.1051/agro:2008007
Laurent, A., Olsen, S.I., and Hauschild, M.Z. (2011). Normalization in EDIP97 and EDIP2003: updated European inventory for 2004 and guidance towards a consistent use in practice. Int. J. Life Cycle Assessment 16, 401–409. doi: 10.1007/s11367-011-0278-6
Matson, P. A., Parton, W. J., Power, A. G., and Swift, M. J. (1997). Agricultural intensification and ecosystem properties. Science 277, 504–509. doi: 10.1126/science.277.5325.504
Menzi, H., Frick, R., and Kaufmann, R. (1997). Ammoniak-Emissionen in der Schweiz: Ausmass und technische Beurteilung des Reduktionspotentials., Schriftenreihe der FAL. FAL Reckenholz, Zurich, Switzerland, p. 107.
Nemecek, T., Dubois, D., Huguenin-Elie, O., and Gaillard, G. (2011). Life cycle assessment of Swiss farming systems: I. Integrated and organic farming. Agricul. Syst. 104, 217–232. doi: 10.1016/j.agsy.2010.10.002
Nemecek, T., and Kägi, T. (2007). Life Cycle Inventories of Agricultural Production Systems. Agrosope Reckenholz-Tänikon Research Station ART, Zurich and Dubendorf, Switzerland.
Niero, M., Pizzol, M., Bruun, H.G., and Thomsen, M. (2014). Comparative life cycle assessment of wastewater treatment in Denmark including sensitivity and uncertainty analysis. J. Cleaner Product. 68, 25–35. doi: 10.1016/j.jclepro.2013.12.051
O'Brien, D., Shalloo, L., Patton, J., Buckley, F., Grainger, C., and Wallace, M. (2012). A life cycle assessment of seasonal grass-based and confinement dairy farms. Agricul. Syst. 107, 33–46. doi: 10.1016/j.agsy.2011.11.004
Ogino, A., Orito, H., Shimada, K., and Hirooka, H. (2007). Evaluating environmental impacts of the Japanese beef cow–calf system by the life cycle assessment method. Animal Sci. J. 78, 424–432. doi: 10.1111/j.1740-0929.2007.00457.x
Pedolin, D., Six, J., and Nemecek, T. (2021). Assessing between and within product group variance of environmental efficiency of swiss agriculture using life cycle assessment and data envelopment analysis. Agronomy 11:1862. doi: 10.3390/agronomy11091862
Pirlo, G., and Lolli, S. (2019). Environmental impact of milk production from samples of organic and conventional farms in Lombardy (Italy). J. Cleaner Product. 211, 962–971. doi: 10.1016/j.jclepro.2018.11.070
Pizzol, M., Christensen, P., Schmidt, J., and Thomsen, M. (2011a). Eco-toxicological impact of “metals” on the aquatic and terrestrial ecosystem: a comparison between eight different methodologies for Life Cycle Impact Assessment (LCIA). J. Cleaner Produc. 19, 687–698. doi: 10.1016/j.jclepro.2010.12.008
Pizzol, M., Christensen, P., Schmidt, J., and Thomsen, M. (2011b). Impacts of “metals” on human health: a comparison between nine different methodologies for Life Cycle Impact Assessment (LCIA). J. Cleaner Product. 19, 646–656. doi: 10.1016/j.jclepro.2010.05.007
Prasuhn, V. (2006). Erfassung der PO4-Austräge für die Ökobilanzierung - SALCA-Phosphor. Zurich: Agroscope FAL Reckenholz. 20.
Richner, W., Oberholzer, H.-R., Freiermuth Knuchel, R., Huguenin, O., Ott, S., Nemecek, T., et al. (2014). Modell zur Beurteilung der Nitratauswaschung in Ökobilanzen – SALCA-NO3. Unter Berücksichtigung der Bewirtschaftung (Fruchtfolge, Bodenbearbeitung, N-Düngung), der mikrobiellen Nitratbildung im Boden, der Stickstoffaufnahme durch die Pflanzen und verschiedener Bodeneigenschaften. Version 2.0, Juni. Agroscope Science Nr. 5. Agroscope, Zurich, Switzerland, 28.
Schaumberger, A., and Formayer, H. (2008). Räumliche Modellierung der thermischen Vegetationsperiode für Österreich, 10. (Vienna: Österreichischer Klimatag), 76–78.
Smith, L. G., Kirk, G. J. D., Jones, P. J., and Williams, A. G. (2019). The greenhouse gas impacts of converting food production in England and Wales to organic methods. Nat. Commun. 10:4641. doi: 10.1038/s41467-019-12622-7
Tal, A. (2018). Making conventional agriculture environmentally friendly: moving beyond the glorification of organic agriculture and the demonization of conventional agriculture. Sustainability 10:1078. doi: 10.3390/su10041078
Umweltbundesamt (2004). Siebenter Umweltkontrollbericht. Kapitel 4.3 Boden. Umweltbundesamt, Vienna, Austria, 303–322.
UN (2017). World Population Prospects. The Revision. Key Findings and Advance Tables. New York, NY: United Nations. p. 53.
Weidema, B. P., Bauer, C., Hischier, R., Mutel, C., Nemecek, T., Reinhard, J., et al. (2013). Overview and Methodology. Data quality guideline for the ecoinvent database version 3. Ecoinvent Report 1(v3) (St. Gallen: The ecoinvent Centre), 169.
ZAMG (2021). Klimamittel - Zentralanstalt für Meteorologie und Geodynamik. Available online at: https://www.zamg.ac.at/cms/de/klima/informationsportal-klimawandel/daten-download/klimamittel (accessed November 11, 2021).
Keywords: dairy production, extensive agriculture, regionality, site-adapted agriculture, life cycle assessment
Citation: Grassauer F, Herndl M, Iten L and Gaillard G (2022) Environmental Assessment of Austrian Organic Dairy Farms With Closed Regional Production Cycles in a Less Favorable Production Area. Front. Sustain. Food Syst. 6:817671. doi: 10.3389/fsufs.2022.817671
Received: 18 November 2021; Accepted: 18 January 2022;
Published: 08 March 2022.
Edited by:
August Bonmatí, Institute of Agrifood Research and Technology (IRTA), SpainReviewed by:
Viviane Barros, State University of Ceará, BrazilEdilene Pereira Andrade, Institute of Agrifood Research and Technology (IRTA), Spain
Copyright © 2022 Grassauer, Herndl, Iten and Gaillard. This is an open-access article distributed under the terms of the Creative Commons Attribution License (CC BY). The use, distribution or reproduction in other forums is permitted, provided the original author(s) and the copyright owner(s) are credited and that the original publication in this journal is cited, in accordance with accepted academic practice. No use, distribution or reproduction is permitted which does not comply with these terms.
*Correspondence: Florian Grassauer, florian.grassauer@raumberg-gumpenstein.at