- 1School of Design, Royal Melbourne Institute of Technology University, Melbourne, VIC, Australia
- 2Food Agility CRC Ltd., Ultimo, NSW, Australia
School food programs can improve the nutritional status at community level, and can be a powerful tool to facilitate a transition to sustainable food systems, particularly through their purchasing methods and meal/waste management. Artificial Intelligence (AI) can enable or inhibit transitions to sustainability, due to its capacity to facilitate transformational change and disruption. Thus, AI can have major impacts on achieving the United Nations' Sustainable Development Goals (SDGs), including those related to food systems. This paper focuses on critically exploring different aspects during the implementation of a software project in US schools that used AI techniques to enable agility and provide healthy food options for schoolchildren. Participant observation, semi-structured interviews, and document analysis were used to inform the case study and uncover the new processes developed using the technology. As a key barrier to the effective management of school food programs and nutrition is the administrative load associated with statutory compliance, this case study demonstrates the difference that AI-powered tools can make in alleviating the weight of administrative processes. An Information and Communication Technology (ICT)-enabled boundary spanning framework is used to extend the case study toward an analysis of the systems, boundaries, relationships and perspectives which starts mapping the areas where the private sector, public institutions and civil society can meet to fast-track sustainable transition activities, particularly in the context of food systems. This article presents some possible approaches to facilitating these activities, inviting policy makers to bridge the gap with businesses and use business agility to support common societal goals to achieve sustainable food systems.
Introduction
Transitioning to healthy diets from sustainable food systems depends on changes in food environments, where sustainably produced healthy dietary items are available, affordable, and accessible in different outlets (Herrero et al., 2021). Additionally, intensification, distancing, concentration, and homogenisation weaken communication in food value chains and add constraints to the necessary tightening of feedback loops between ecosystems, actors within the food chains, and consumers needed for sustainable food transitions (Sundkvist et al., 2005). Artificial intelligence has a role to play in meeting the United Nations' Sustainable Development Goals (SDGs) (International Telecommunication Union, 2018; Vinuesa et al., 2020), including those related to food systems, although necessary insight and oversight are needed to ensure transparency, safety, and ethical standards are upheld (Sætra, 2021). Many studies provide theoretical approaches to AI for sustainability and its benefits and impacts on the SDGs (Fisher, 2011; Nishant et al., 2020; Vinuesa et al., 2020; Sætra, 2021). Some authors focus on food systems and food security with approaches to designing transitions using AI responsibly (Camaréna, 2020), informing public health policy on food quality (Bourguet et al., 2013), or using predictive insights to improve their resilience to achieve global food security (How et al., 2020).
Sustainable school food programs have the potential to greatly influence the production and distribution of sustainable food (Goggins and Rau, 2016; Mensah and Karriem, 2021; Perez-Neira et al., 2021), for which the highest environmental impact happens at the food procurement stage (Garcia-Herrero et al., 2021). The role of institutions in supporting menu planning, food safety, and access to healthy food, and building community partnerships is part of a global discourse about eradicating hunger and obesity and other food related diseases (Vogt and Kaiser, 2008; Valaitis et al., 2014; Pagliarino et al., 2021). Sustainable school food programs are also important in the development and strenghtening of the farm-to-institution relationships required to improve the nutritional status of communities and the institutional support for systemic transitions of purchasing methods (Vogt and Kaiser, 2008; Wittman and Blesh, 2017; Aleksejeva and Pelse, 2019; Perez-Neira et al., 2021). National school nutrition programs, influenced by programs such as the Farm to School program, could play a significant role in increasing the connection between fresh, healthy local food producers and education practices at school (Roche and Kolodinsky, 2011; USDA, 2019a; Mensah and Karriem, 2021; Pagliarino et al., 2021; Perez-Neira et al., 2021). These programs are an interface for youth, and are thus important for their engagement in the transition to sustainable food systems (Glover and Sumberg, 2020).
This paper builds on the above theoretical approaches to AI for sustainability and the ethical use of AI for food systems. Emerging research at the intersection of AI, school nutrition and sustainable food systems does not yet provide primary data, which could help inform approaches to policy and transdisciplinary collaboration. This article bridges this gap and investigates a real-life implementation of AI-powered tools to solve issues confronted by school food programs in child nutrition meal planning and administration. It illustrates how the use of AI in the context of a school food program impacting several thousand schools could be extended to wider aspects of institutional food programs for the purpose of sustainable transitions.
The case study takes place in the United States of America (USA) where schools provide subsidized meals to students through a range of programs such as the National School Lunch Program (NSLP), School Breakfast Program (SBP), Special Milk Program (SMP), After School Snack Program (ASSP), and Seamless Summer Option (SSO) (Hopkins and Gunther, 2015). Most programs are run by the United States Department of Agriculture (USDA) in collaboration with state governments and school food authorities (Izumi et al., 2018). The NSLP alone provides free or reduced-fee lunches to more than 30 million students at US public and private schools (USDA, 2019a). With over one in five children in the United States living in food-insecure households (Coleman-Jensen et al., 2020), food programs such as the NSLP play a critical role in the development and long-term health and educational outcomes for low-income children (Baidal and Taveras, 2014; Radday, 2020; Feeding America, 2021).
However, school food authorities face difficulties when implementing and managing these food programs. School leaders are required to provide their full attention to various components not only to meet the Nutrition Standards for School Meals set by the USDA (2019b) but to also maintain sponsorship without neglecting the instructional leadership needed to effectively implement and sustain the program (Rafidi et al., 2012). Reporting guidelines are often complex and time-consuming, involving reporting on the number of meals served, the level of subsidy allocated, and the complete nutritional information of the food, to ensure meals are within the dietary guidelines set by the program (Gleason et al., 2004; USDA, 2015). Errors or delays in reporting can result in the loss of subsidies for school food authorities and gravely impact the families depending on the program for their food and nutritional security. As a result, the stringent reporting and compliance framework often acts as a barrier for the provision of diverse and healthy options that are locally produced and economically attractive (Ralston et al., 2008).
The research objectives are (a) to understand the context for the use of AI-powered tools in school food programs; (b) to understand what the technologies aimed for and delivered; and (c) to develop an informed view of the opportunities and challenges of using AI in that context, and what AI can achieve for the transition to sustainable food systems. The research used mixed methods to collate information about the origins, planning, and implementation of AI for a food program management software company used by a large proportion of schools throughout the United States of America (USA). The research includes semi-structured interviews, participant observation, and document analysis (Section Methodology). Findings include information on the AI tools (and disciplines used), information on nutrition data standardization, results achieved through automation, and the challenges and opportunities faced during the implementation (Section Results). The discussion (Section Discussion) is informed by an analysis of the findings using systems thinking methods. In this section we identify the opportunities for scaling up similar solutions across organizational boundaries for sustainable outcomes and discuss limitations of the research, options for further research, and proposed implications and recommendations for policy and practice. The conclusion (Section Conclusion) summarizes the main findings and reflections.
Methodology
Case Study
Aiming to support both the implementation and the maintenance of federally funded and federally compliant food programs, US-based software company Rayfood Solutions1 offers a suite of products to support schools and school districts to more effectively manage (1) school nutrition programs, (2) food distribution programs, (3) administrative reviews, and (4) food tracking and reporting.
As part of the company's offering of a “software as a service” solution and for a monthly licensing fee, state authorities or local school authorities can access Rayfood's food program management features through a web page without the need for hardware or software infrastructure. Rayfood Solutions have also developed a new range of software features centered around the provision and reporting of nutrition information for child school meals. The information can be used by schools to plan then report about each meal under the USDA's Nutrition Standards for School Meals (USDA, 2019b).
The provision and reporting of these new features triggered the need to initiate a software development project aimed at automatically capturing nutrition and other meal data. Figure 1 presents the existing steps of the menu planning and reporting process taken by the school food authorities and foodservice directors against the steps provided by the new software. The agile and streamlined access, processing, and reporting in the new system is dependent on the availability of over 15,000 menu items nutrition and compliance information.
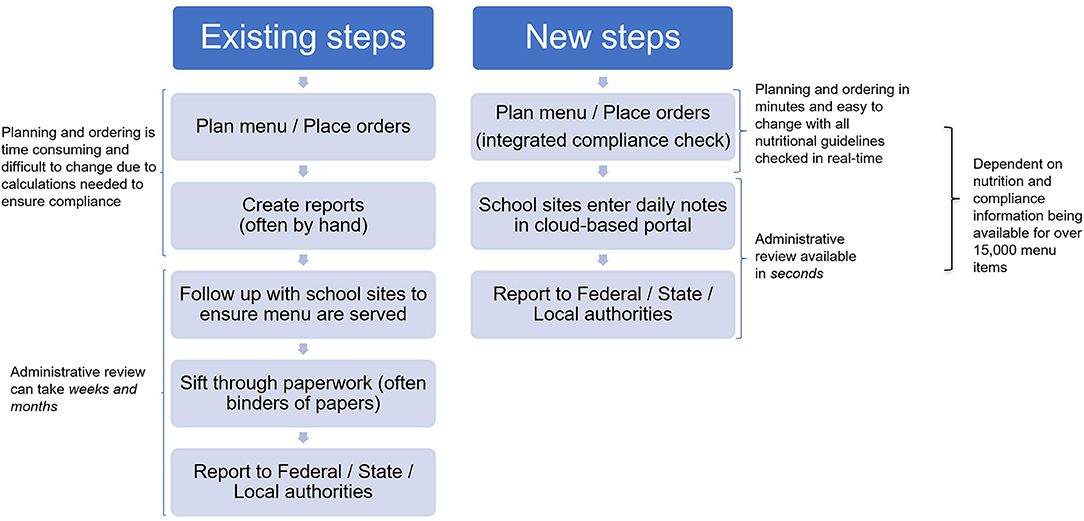
Figure 1. Summary of nutrition planning and reporting steps for foodservice directors and school food authorities.
Initial communications with Rayfood Solutions at the onset of the project provided information on the context, intent, and stakeholders involved. Meals and food products' nutritional data are mostly generated by the food suppliers; therefore, the meal nutrition documents they provide to schools are used for the automated data capture. Rayfood identified the challenge as one requiring accuracy (the nutrition data extracted is used for local, state, and federal compliance reporting to the food programs), speed (the volume of food suppliers and the number of meals or ingredients that they provide represents thousands of items to process), automation (searching in a document then manually entering the data for over 50 fields would be extremely time consuming, rendering the project unviable), and adaptability (food suppliers documents do not follow a standard format and are updated regularly). Rayfood Solutions selected LPB Ltd., a company specializing in document data extraction using AI, to conduct a pilot to test the possibilities of such a project and then implement the project. The data for this article is based on LPB's interviews, participant observations, and documentation as they conducted their pilot test, with input from Rayfood Solutions' project director.
Research Approach
The research approach is summarized in Figure 2 and consists of three main stages: understanding the context, understanding AI, and investigating the opportunities and challenges of AI for transitioning to sustainable food systems. The stages are only in a partial chronological order due to the iterative nature of exploratory case studies. Exploratory case study approaches are used when a “how and why question is being asked about a contemporary set of events over which the investigator has little or no control” (Yin, 2014, p. 9). The first two stages used direct input from document analysis, participant observation, and interviews of LPB. The third stage relied on the conclusion of first two stages and the application of systems thinking and ICT-enabled boundary spanning approaches.
The research is qualitative in nature, relying on document analysis, participant observation, and semi-structured interviews.2 Collecting information from three points of data allows triangulation, using evidence from different sources to corroborate facts and findings (Rowley, 2002; Yin, 2014). Specifically, through these modes of data collection, we have aimed to fulfill the research objectives stated in the introduction.
Systems thinking is the epistemological lens through which the issues of sustainability are addressed and is bounded by the researcher's worldviews of what constitutes sustainable food systems (Abson et al., 2017; Dorninger et al., 2020). The researchers' stance aligns with the Food Agriculture Organization (2018, p. 1) which proposes that a sustainable food system “is a food system that delivers food security and nutrition for all in such a way that the economic, social and environmental bases to generate food security and nutrition for future generations are not compromised”. The analysis includes considerations of the core systems (production, processing, distribution, consumption) and extends to the importance of societal elements (organizations, policies, infrastructures, norms) and the natural elements (soil, air, ecosystems, water, climate).
The whole-system approach (Lovins et al., 2010; Blizzard and Klotz, 2012) helped to identify potential areas for future research where AI can be part of sustainable transition design. The analysis considered the multiple perspectives, relationships, boundaries, and systems at play in this case study (Cabrera et al., 2008). This provided important details to investigate how the implementation of AI for school's food program administration could support transitions to sustainable school food procurement and management programs more broadly.
Finally, a consideration of ICT-enabled boundary spanning was applied to reveal specifically which collaborative forms of governance could be highlighted for solving wicked sustainability problems such as food security (Rittel and Webber, 1973; Termeer et al., 2015). Termeer and Bruinsma (2016, p. 91) define boundary spanning arrangements as “the ensembles of rules, processes and technologies, that deliberately enable interactions between actors across these boundaries”. An ICT-enabled boundary spanning arrangements (IBSA), they argue, can help cross physical, cognitive, and social boundaries and contribute to collaborative solutions to balance socio-technological engineering and to sustainability. In the context of food and nutrition security, IBSA has been identified as a key feature to consider in practice-led redesign of pathways where spanning across social, physical, and cognitive boundaries promises better governance performances (Oostindie et al., 2016). An analysis of ICT-enabled boundary spanning frames the discussion section (Section Discussion) of this paper. The results and discussion can provide insights into pathways made possible by spanning boundaries, engaging stakeholders, and leveraging the AI-powered solutions used in this project.
This exploratory case study investigates the goals of Rayfood Solutions' menu planning automation project, the stakeholders involved, the steps taken to deliver the project, and the lessons learnt, issues that arose, and other opportunities which came from its delivery. In this instance, observing an AI-automation project in a private enterprise to manage an institutional food program offers opportunities for insights into what the current system does and how it could be modified to support sustainable school food procurement and management options.
To that end, a series of unstructured interviews and email conversations conducted with Rayfood Solutions in November–December 2017 helped situate the research and establish background knowledge. Participant observation at LPB Ltd followed in April–May 2019 to gain first-hand knowledge of the activities involved in the automation of the data capture processes (Section Participant Observation). Finally, document analysis (Section Document Analysis) and semi-structured interviews with the management and operation team at LPB Pty Ltd. (Section Semi-Structured Interviews) conducted in October 2019–February 2020 provided details of the data structure, stakeholder engagements, system challenges, and achievements.
Data Collection and Analysis
Participant Observation
Participant observation (Jorgensen, 1989) consisted of observing the work done by the digitalisation operators when conducting quality assurance tasks involved in the data recognition and data classification processes. Recognition and classification are features of AI-powered tools and the participant observation investigated which tools were used to conduct these functions and how they were used. A researcher sat with a team of digitalisation operators and observed while they labeled the information. Labeling is a task conducted when training an algorithm for machine learning and observations revealed what this entailed in the digitisation process. The observation included documenting the series of steps involved in accessing the system, identifying the data extracted, and making assessments of errors and missing data. It also captured conversations conducted in person or via email when operators had questions or encountered difficulties over that period. Understanding the extent and complexity of the task when automating nutrition and meal data extraction can provide indications of how feasible it is and what to expect for other institutions who would want to implement similar tools.
The researcher asked questions about the process, data, and its provenance, and if and how data was transformed [e.g., units needed to be converted from milliliters (ml) to fluid ounces (fl.oz)]. The type of data exchanged and its source can provide bridges to new or additional stakeholders and create new dimensions of information. For instance, capturing location information (where the food originates from) could be used as a filter for locally produced foods. Therefore, it could constitute an avenue of communication between actors in the system and the transition to sustainable procurement preferences.
Document Analysis
Analysis of documentation of Rayfood's new project was conducted between October and December 2019. This included the initial proposal and project specification documents, communications and reports, as well as the project's documents, such as food supplier product specification data sheets and export files once the data had been quality-controlled.
In the interest of generating useful data, the document analysis began by establishing the types and content of the documents flagged for automation: product specification sheets (also called product formulation statements or product information sheets). This helped establish an understanding of the breadth and depth of the data capture activities. Food suppliers to US schools have a regulatory obligation to provide product specification sheets that list all ingredients, quantities, nutritional information, allergens, and certifications (e.g., organic or halal). With no national template for these information sheets, the data can appear in different formats, units, and data fields, making the standardization of the automated capture more complex. For instance, as shown in Figure 3, the amount of each creditable ingredient under the compliance reporting scheme was found in text form (on the left) and in number form (on the right). The sheet on the right also presents the amounts in four different areas on the page. Whether read by a human or by a machine, a high level of attention and logic must be applied to make sense of different formats. In some cases, additional information is available on the sheets on non-nutrition related dimensions (e.g., where food was processed).
The Rayfood Solutions/LPB Ltd project's documentation also provided information on the origins of the project. It captured the evolution of the project from pilot to implementation, and communications between the implementation team at LPB and Rayfood. The analysis reviewed the intent of the project, as well as the stakeholders involved and their role and insights on the results, challenges, and opportunities facing the project's pilot and implementation. Further analysis looked for words and statements identifying stakeholders and processes up- and downstream from the automated data capture project. Systems, boundaries, perspectives, and relationships provided the context within which the project is situated. Additionally, they helped to build a view of which elements the project is situated without.
Semi-Structured Interviews
Interviews and business process analysis aimed to scope more precisely the extent of the project, identifying the people, processes, and systems involved. Semi-structured interviews were conducted after the roll out of the digitisation project from November 2019 to February 2020 and involved three managers and supervisors from LPB, who were directly involved in design, implementation, and project supervision. The interview subjects were selected based on their ability to provide in-depth knowledge of (1) the processes used when the solution was originally designed, (2) their understanding of how the implementation steps took place, and (3) the nature of the data required by the AI automation project and the challenges faced to ensure data quality and consistent outcomes. The interview questions focused on the project being investigated, how it came about, the participants, what objectives it aimed to deliver, and what documentation exists about it (see Supplementary Material—Questionnaire 1). LPB provided full access to stakeholders, systems, and data. Interviews were analyzed to complete any gaps in the process analysis conducted through the participant observations and the document analysis. Interview data were also reviewed to detect differences in objectives from the original intent (Interview 1 with Rayfood Solutions) to the project achievements (Interview 2 and 3).
Correlations between the documentation analysis, participant observations, and interviews were used to verify the timings and efficiency of the new processes being piloted. Findings from the different methods of data collection and analysis were revisited to generate a system view of the project within its context and the role of AI in creating or hindering opportunities for sustainability transition.
Results
Understanding the Context
Interviewee 1 is the main driver of LPB's project at Rayfood Solutions. With a background as a nutritionist and a dietician and heavily involved in child nutrition in schools, they have a passion for nutrition. Changing the habits of children and their families toward healthy nutrition is the main driver of Interviewee 1's quest for innovation. They and their team have over 20 years of experience managing food nutrition programs in the US. They explained that Rayfood Solutions' goal is to connect the food supply in the country by bringing the production of datasets on nutrition, waste, and children's choice-making and patterns of meals together.
Rayfood Solutions found that some of the biggest challenges related to the wide gap between supply and demand and the lack of predictability and consistency. “The cyclical shortages and overages lead to food loss and food waste” (Interviewee 1) in the absence of proactive and effective design and management of nutrition programs. Background research conducted by Interviewee 1 over 15 years of work in state school districts demonstrated that this inability to provide consistent variety for children often leads to disappointment and disengagement. Children tend to choose what they will eat the day before they go to school. “Not providing access to the menu they wanted is devastating for food nutritionists and children alike” (Interviewee 1).
The Rayfood Solutions project with LPB therefore focused on gathering and extracting nutritional information from food suppliers, including the availability of stock, so that the school menu planning activities could be based on real-time data and provide nutritious and USDA-compliant meal options to children.
Artificial Intelligence for Automation
Automated Data Extraction
Artificial intelligence tools were used to automate the recognition, extraction, and reporting of nutrition data across the over eight thousand school meals' product specification data sheets processed in the initial stages of the project. A total of over 37,000 specifications sheets are estimated to have been converted to USDA-compliant datasets over the lifetime of the Rayfood Solutions project.
Rayfood aimed to process product specification sheets automatically to obtain food items' nutritional information without having to manually search for the data and then manually input it into their systems. The task of extracting the data is complex and the manual process is time consuming and error prone. Through the pilot project, when information is provided (mostly in PDF, Microsoft Word, or Microsoft Excel formats), the new process ensured the content was scanned through an AI-powered process, automatically extracting the information required to inform a menu planning tool for schools.
In this case study, the Advanced Optical Character Recognition3 (OCR) process [a technology used to digitize written text into machine readable data (Keary, 2019)] was supported by image recognition, natural language processing,4 and machine learning, all disciplines powered by AI. This allowed the system to understand where mistakes are made, and to comprehend information wherever it appears in a document, in text or number format. For example, when observing the digitisation operator's screen, it appeared that most of the information on the product specification sheet was individually recognized and extracted (highlighted in green) to the appropriate data fields without human intervention (Figure 4).
The process used image recognition5 to recognize logos and seals and translated them to data when relevant. For example, if a “gluten-free,” “kosher,” or “halal” logo was found, it would be used as part of the dataset to appropriately specify that quality in the food.
The extracted information was displayed in the software for digitisation operators to review, correct errors and missing data, and then save for export in the data compliance system. This was part of a process where the initial stages were supported by the digitisation operators, who were teaching the machine to find the relevant data on product information sheets of any types (Figure 5).
Finally, machine learning6 was used to detect data extraction patterns to sort data into the appropriate fields. Part of the machine learning in the case study was supported by humans who reviewed the fields extracted and ensured data was matched to the appropriate fields (supervised learning). Part of the learning was done directly by the AI, which would detect anomalies in the data when a field extracted did not match the expected format (unsupervised learning). While the machine was learning, digitisation officers managed exceptions raised by the software, or cases where the algorithm could not recognize or find data for a field. They guided the learning until the algorithm could automate all fields extraction and recognition without error. For example, one data field requirement was to identify a Universal Product Code (UPC) in line with the Nutrition Standards for School Meals requirements. In some cases, the AI selected “CASE GTIN” as the UPC because they have similar nomenclature. When training the AI, an operator flagged it and looked to confirm whether “CASE GTIN” was actually the same as the UPC. In this instance, it was not, and needed to be deleted (Supplementary Figure 7).
While the process ensured that there were no data extraction issues, it required additional steps to check the data for compliance to the nutritional standards. The data compliance check was done post-data extraction in a different system where digitisation operators would review any exceptions raised by the system, such as when a type of data was expected but not found, or it was found in an unexpected format. For instance, a “serving size” could have been successfully extracted as 150 g, but appeared as an exception because it needed to be expressed in ounces; in which case the system would raise an exception for the operator or for a Rayfood Solution staff member to confirm what needed to be done.
Data Standardization
A key requirement of the project was to extract and export nutritional information about available meals for the design of school menus and compliance reporting to the food program administrators. This information would be a key enabler for an online platform that Rayfood Solutions was developing as a new service to their customers, to simplify the menu planning and reporting activities.
When Rayfood defined the nutritional information to be extracted from the product specification sheets, they defined “Go-to-market” data fields (called “features” in the project documentation). The data was classified in three types: “Mandatory fields” —data required under the food programs' regulations, implemented in Phase 1; “Good to have” —additional information not required for compliance but highly desirable by users, implemented in Phase 2; and “Phase 3” —the remainder of the data fields to be released once the rest of the solution was in place.
Data categories defined in the project initiation phase included (Table 1):
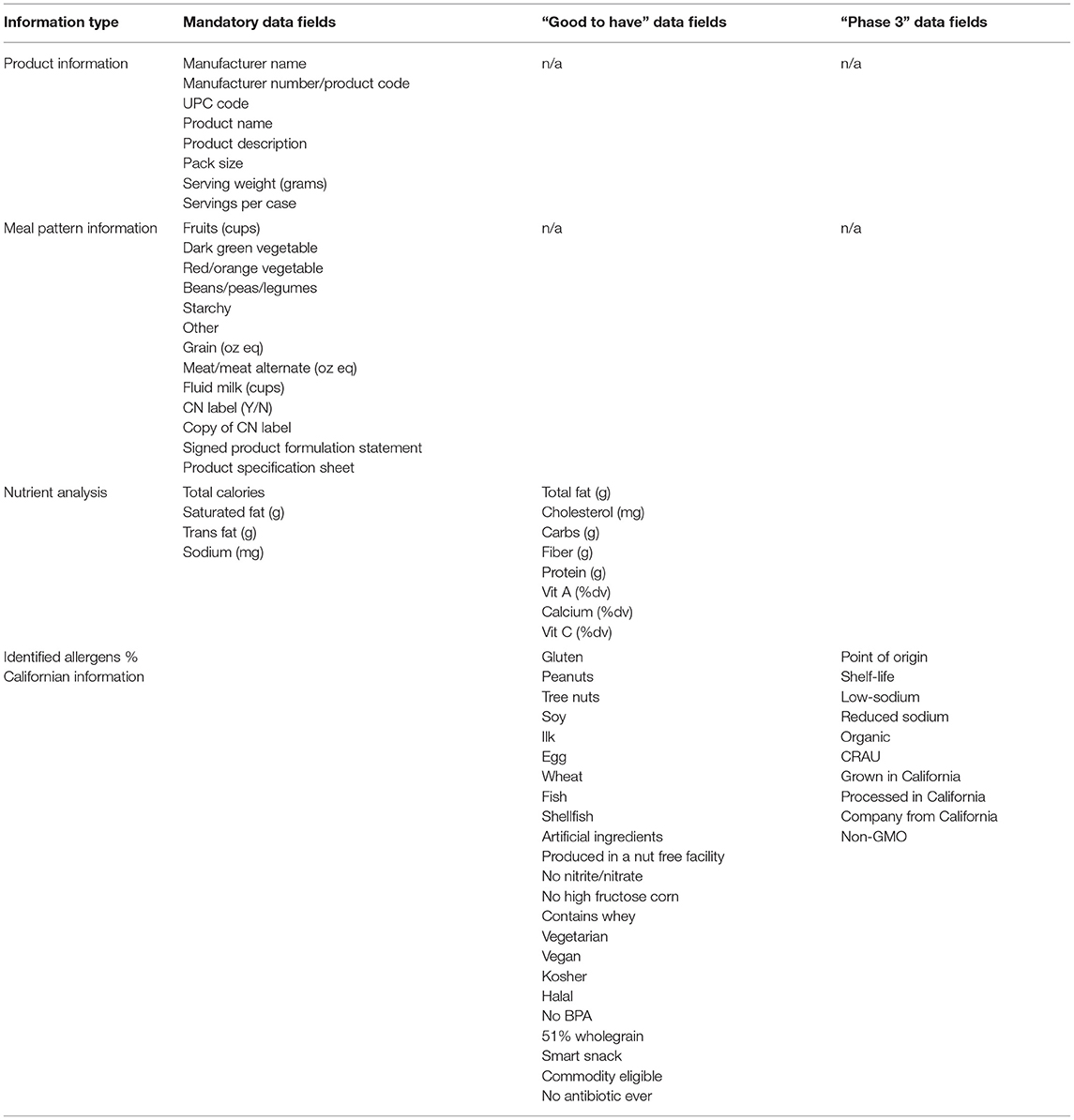
Table 1. Detailed data fields earmarked for automated extraction in the initial project specifications documents. Source: (Project Documentation, 2020).
• Product information
• Meal pattern contribution
• Nutrient analysis
• Identified allergens and Californian information
The product information and meal pattern contribution are fields of data that are required by regulations to be compliant with the food programs. Parts of the nutrient analysis are required by regulations; the rest [total fat (g), cholesterol (mg), carbs (g), fiber (g), protein (g) vitamin A (%DV), and calcium (%DV)] were fields Rayfood Solutions decided to add as additional features that would benefit the school menu planners and food program administrators. Identified allergens were also added as part of the “go-to-market” push to create a competitive advantage for the software solution. Other information fields were highlighted as potential fields to be made available in “Phase 3” of the project.
During the interviews, it appeared that the biggest challenge in the implementation of the AI program was the lack of standardized format in the data. The product specification sheets collated from suppliers did not follow any specific format, and only some suppliers used the USDA templates. There were no naming conventions and supplier information was provided in different formats (Word document, pdf, csv, jpg). This resulted in a large amount of time spent between the digitisation team at LPB and Rayfood Solutions clarifying which keywords to look for and to ensure the extracted data was accurately matched to the data fields requirements. This, in turn, created challenges for the Rayfood team, who had to contact the suppliers and request clarifications on how the fields in their product specifications sheets matched (or did not match) the compliance reporting in the USDA standards.
The lack of standards in the supplier's data also extended to the units used to capture quantities for each food item. For example, product specification sheets indicated quantities in grams (g) but the food program compliance reporting must be done in ounces (oz) or cups. Data conversion tables had to be implemented to ensure the original data, whatever its format and units, could be converted to the format required by the compliance standards. There were also issues with reporting language. For example, when reporting for gluten, there needed to be an agreement that the answer would be “Yes” when the words “gluten free” appeared on the product specification sheet. When training the algorithm, staff could at times say “yes” when they saw “gluten” in the contents or “no” when they saw “gluten free”.
Results of Automation
The interviews and document analysis conducted post-implementation confirmed that the project achieved the goals of automating the capture of child meal nutrition value per meal item for over 2,000 suppliers and dozens of products per supplier on average. The data extracted automatically evolved over time and came to include a range of 30 mandatory fields and 50 additional fields which had never been extracted before (Interview 1, 2, and 3), providing in-depth information on nutritional value of children's meals but also context data about provenance or food miles.
The results of the data analysis and Interview 3 revealed that thousands of supplier data sheets, regularly updated, required between 5 and 10 min per document to extract the data manually. This represented between 160 and 320 h to process the data in a set of 2,000 data sheets (the number of product specification sheets scoped in the initial part of the project). The total project processed between 6,000 and 7,000 data sheets within weeks. At the end of the project and in its current operational form, sets of 200 data sheets are processed within 30–90 s per sheet. This represents a time saving of between 70 and 80% (or over 15 h) per set, compared to processing the updates manually.
The accuracy of the automated extraction ranged between 95 and 98%. The remaining 2–5% is due to gaps in the data, which was either not available on the product specification sheets or unreadable (Interview 3). These gaps nevertheless could be filled by human intervention, with one or two people reviewing any exceptions and making manual corrections if and where required.
The data and the menu planning tool it supports have since been rolled out to schools as a pilot project. Schools can access a vendor portal, which allows menu planners to (1) pick food items and create recipes, (2) plan meals which meet the USDA compliance standards, (3) set meals to a schedule to create menus, (4) connect menus to local sites in the district, (5) track menus to sites in the school district, and (5) automatically generate meal and statutory reports.
Challenges and Opportunities
Training an Algorithm
The participant observation revealed that during the data extraction exercise, the difficulty in training the algorithm lies in the painstaking process of identifying instances where the data needed in a field is presented in many different forms across a range of documents. This renders the automated identification and extraction difficult. As with the UPC code example, the participant observation and document analysis highlighted intense and almost constant communication between the digitisation operators, the digitisation supervisor, and staff of both LPB and Rayfood Solutions. Each field triggered many email conversations to ensure the whole team was up to date with the requirements (Supplementary Figure 8).
On many occasions during our observation of the digitisation operators, the operators engaged in conversations to share knowledge about food and try to make sense of the definitions for the data fields. For example, one of the data was called “dark green vegetables”. In a multicultural environment, the team was often unsure of which vegetable should be considered “dark green” and which to consider “green”. The name of vegetables themselves were unknown to some of the team members; once one person researched it and found photos, they would share the knowledge with others.
The document analysis confirms that a lot of knowledge was therefore created ad-hoc in a multitude of email conversations, specification documents, and clarification statements in team meetings. Interviewee 3 indicated that no single document or library was created to effectively retain the knowledge. Consequently, when stakeholders changed at Rayfood Solutions due to corporate transformations, the business rules and historical knowledge built over the course of the project delivery were lost and had to be re-explained or re-discovered to newly appointed staff.
Managing the Suppliers
The initial interview of a Rayfood Solutions representative indicated that when they embarked on the project, they were focused on the requirements from the schools and the food programs' administrators. They did not expect to have a tool that would put them in a position to advise or manage food suppliers. However, interviews with representatives from LPB indicated that once Rayfood were faced with questions of data validation, data standardization, and consistency of language, the project coordinators realized that they had a new role to play in the supply chain. The process analysis confirmed that the data extraction automated the compliance checks, that is how the food item complied with the food program nutritional standards. As a result, Interviewee 3 indicated that Rayfood were able to contact the suppliers and let them know that their product did not comply and why. This led to a new type of relationship between Rayfood and the food suppliers. Unfortunately, additional information about how the relationships were influenced by the project and what benefits this could lead to was not available in the material.
Interviewee 2 and 3 mentioned another issue that arose when it was discovered that the product data sheets uploaded by the food suppliers contained what looked like duplicates. The quality control process would automatically flag a specification sheet if it was 99.8% similar to an existing one. However, a number change (for example, 3 spoons of sugar instead of 2) had to be tracked, and meant that the new sheets superseded previous specification sheets. According to Interviewee 3, this issue led to different practices being put in place with the suppliers, such as adding direct comparison of similar files (i.e., field-by-field, submission date), so that the modified product sheets were easily identified.
Disconnection: Intent Lost in Delivery
Looking at design decisions throughout the project, we found that Rayfood Solutions' representative had a vision of what the project was to achieve, but that vision did not transfer across the delivery team (Interview 1). Rayfood Solutions wanted to “connect the food supply…by subsidizing the production of datasets on nutrition, waste, kids picking, and patterns of meals together” (Interview 1). Participant observation and questions on the subject confirmed that the digitisation team involved in the training of AI were focused on data accuracy and the reduction of exceptions; they did not know what the data they were extracting were for.
The lack of shared vision and joint understanding of the project led to missed opportunities in using existing knowledge and skills. For example, as identified earlier, the need for data conversion and issues with units and duplication could have been handled differently and taken advantage of LPB's extensive experience in the area (Interview 2 and 3). There was a lack of clarity about what the automation could achieve and what opportunities it could create, which became more obvious to all participants as the project progressed and they were gaining knowledge of the AI-powered capabilities (Interview 3).
Discussions about food security, nutrition education in children, and sustainability were part of the initial briefings but were not approached again in subsequent communications (Interview 1, specification documents, and email communications). When Rayfood initiated the project, they were in the process of finalizing an agreement with an organization who aim to change local food systems and encourage public institutions to procure food produced through value-driven purchasing standards (Interview 1). This influenced the breadth of data fields flagged for extraction. However, after these initial stages, the document analysis did not find that the aims and values carried by that agreement were mentioned again in the project.
Discussion
Expanding Organizational Boundaries Through ICT-Enabled Collaboration
Termeer and Bruinsma (2016, p. 92) propose that the governance boundaries, which are usually associated with barriers and obstacles, “also reflect junctures that reveal new opportunities for dealing with wicked issues by connecting varied knowledge, resources and ambitions”. These junctures enable the collaborative forms of governance needed across public, private, and civil actors working in “new coalitions, new partnerships, new food markets and relations, new institutional arrangements and new urban-rural relations” (Oostindie et al., 2016, p. 44).
The process analysis outlined for this case study reveals the boundaries between Rayfood Solutions, LPB, the school food suppliers, school food authorities, and government departments. Results indicate that a collaborative form of governance is required for information to flow between Rayfood Solutions, LPB, and the schools' food suppliers. At the juncture of the boundary, school food suppliers started providing nutritional data sheets and receiving advice on gaps in their compliance and in their compliance data (Sections Automated Data Extraction and Data Standardization). Additional data on children's eating patterns and institutional procurement standards could be captured at the juncture with the school food authorities. For example, Figure 1 indicates part of the process is for school food authorities to use the system to collect meal information and manage the inventory of food. Since the data exist, they could be used to capture eating and waste patterns, and to inform procurement strategies. Outside the boundaries are the families benefiting from the programs and the state and federal agencies administering the programs. Figure 6 uses the ICT-boundary spanning approach outlined in Section Research Approach, and takes a systemic view of the project's boundaries, structure, and processes to assess where these opportunities might be.
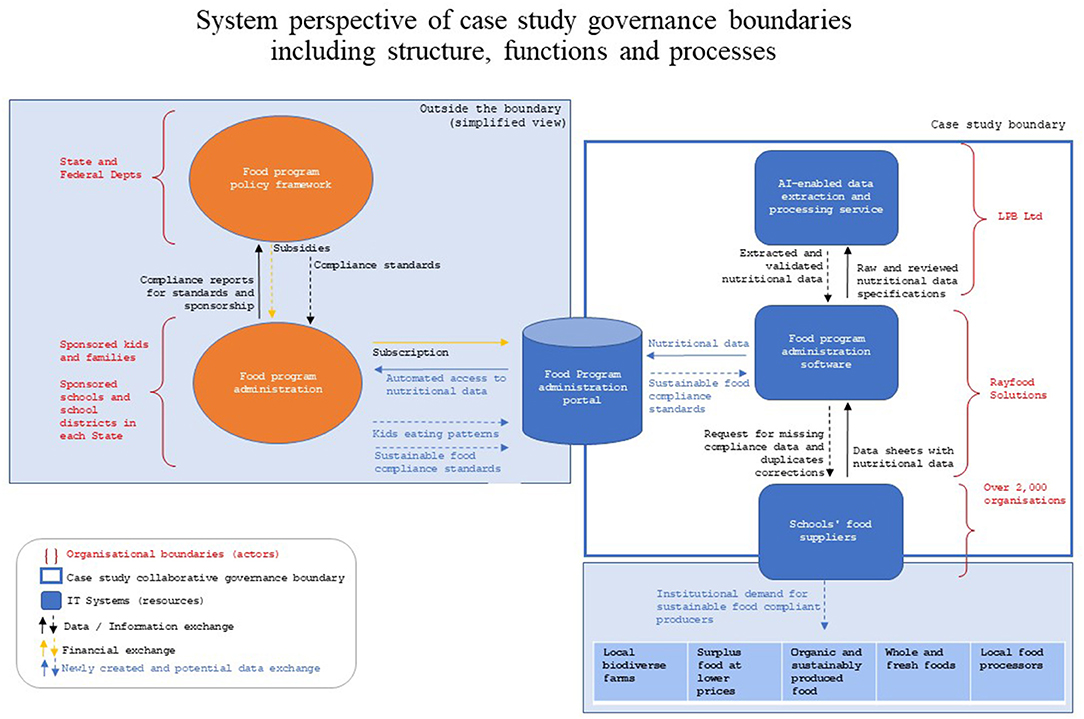
Figure 6. System perspective of case study governance boundaries including structure, functions and processes.
As we discovered in Section Methodology, Rayfood Solutions, through the project implementation, created closer relationships with food suppliers. As a result of collaboratively resolving the data compliance issues discussed in Section Artificial Intelligence for Automation, the search for data clarification uncovered a need for food suppliers to standardize the way they document nutrition information. Rayfood has been able to offer a new service to the suppliers to help them automatically identify the product specification sheets and individual fields that did not meet the statutory nutritional requirements. This is an advantage for Rayfood, who can build trust and share knowledge about nutrition standards with thousands of suppliers. For the food suppliers, it means that their products are not rejected because they were missing the data required for the standards. It is an advantage for the sponsored schools and school districts who can trust that the food items available for the creation of menus are accurately reflecting the ingredients and are in compliance with the food programs. It is also a critical component for the food programs' administrators in federal and state government departments, who can rely on almost real-time information on the type, availability, and nutrient content of food offered to sponsored schools.
Opportunities at the Junctures of the Boundaries
With a view to connect varied forms of knowledge, resources, and ambitions, the knowledge uncovered in this study points to opportunities available to enable sustainability transitions when food suppliers, school food authorities and the private sector collaborate. Food suppliers originate from across the U.S. and provide either raw ingredients or processed foods and drinks. Capturing data about the provenance of the food and ingredients (Table 1) could offer local farmers and food producers a unique value proposition where local and state schools prefer local food producers. The “Californian information” is such an example, allowing Californian schools to select produce that is grown and prepared in California.
The original intent of the project included the view that Rayfood Solutions could offer a set of tools which would cover the gap between supply and demand (Section Understanding the Context). With a few additions to the current processes and systems, automated data extraction could provide options for the rapid detection of surpluses available in the local area that schools could access at a reduced price. This would support efforts to reduce food waste at the production stage, which is a promising strategy for enhancing the sustainability of food systems (Vågsholm et al., 2020). Similarly, where efforts to increase government departments' sustainable procurement practices exist (as in California in this study), access to the data would provide a selection of preferred content, i.e., fresh, locally-produced, vegetables and fruit, direct from the farm. As we have seen earlier, school food authorities (SFA) tend to standardize the food they order to avoid misreporting new food items and jeopardizing the receipt of USDA subsidies (Section Introduction). Automation can help standardize and simplify the way SFA report on compliance rather than standardize the food they offer. This, in turn, could provide agility in the way food menus are created and support a greater diversity in the food accessible to sponsored schools and families. Additionally, the ability to create a localized, biodiverse food supply chain would create systems which are much more resilient to shocks and stresses (Oostindie et al., 2016, p. 43).
Opportunities Outside the Boundaries
In this case study, nutrition data (and the decision-making power they offer) is facilitated through the automation of data extraction, the standardization of data reporting, the ability to filter across complex data fields, and newly created relationships within the boundaries of the shared processes, systems, and rules. Looking outside the boundaries of the case study, we consider what the project enables for sustainability among the food program administrators, families and sponsored kids, and government authorities.
The impact of Rayfood and LPB's project was mainly felt by the school food authorities, who are now able to use a portal to simply drag and drop different elements of the menu, and instantly know what is nutritionally missing. They now also have access to recommendations about what other ingredients and items they need to include in their menu to comply with the latest standards for child nutrition. School food authorities are often reluctant to change their menus because it puts the subsidies at risk of being canceled or missed if the new ingredients do not comply with the standards (Ralston et al., 2008). This has caused a lack of agility in how the administrators can introduce new products and how they can take advantage of locally produced food and food surpluses (Interview 1). Rayfood and LPB's intervention can overcome these challenges.
The administration of food programs is often tedious and time consuming, with little room for error. Many schools still manage food orders and menu creation using binders containing the food suppliers' product specification sheets. As we saw previously, these are quickly out of date and many do not follow a standardized format. For administrators, being able to easily change and manage the menu and instantly report to relevant departments with compliant data can save hours and days of reporting every month, resulting in a significant decrease in administrative costs and efforts. Furthermore, the agility gained from the ability to adapt a menu quickly could open opportunities for procurement strategies which could respond more quickly to supply crises and adapt to local availabilities.
Most importantly, being able to provide fresh and diverse menu options to children who often do not have access to food at home, let alone healthy food, has dramatically beneficial consequences for child development, education outcomes, and health (Ashiabi, 2005; Thomas et al., 2019). Furthermore, children who are repeatedly exposed to varied and new foods are more willing to accept them (Baidal and Taveras, 2014) therefore creating a pathway for improving nutrition early in life (Forestell and Mennella, 2007). It is argued here that over time, data about the food offered and the food consumed, delivered through the direct input of school sites could provide insightful information about the uptake of a program, the children's response to local food programs and campaigns (i.e., Farm to School), and more detailed information about food waste. These insights and enabling capabilities could deliver systems where children can select a menu ahead of time and be better engaged in eating healthy foods; thus avoiding the disappointment of not having the food they want when they pick up their lunch.
In a post-COVID-19 world, the movement to cater to customers online and opt for more direct value-chains to minimize COVID-19 contamination (Worstell, 2020), is responding to questions about food security when people are in lockdown, children are not going to school physically (Galanakis, 2020), and dramatically increased demands on food banks (Foodbank, 2020; Fitzpatrick et al., 2021; Gundersen et al., 2021). Meanwhile, food that was supposed to be distributed to schools and institutions is wasted due to vulnerabilities in distribution systems and extreme concentration of supply chains (Pollan, 2020). Access to rich, localized, and real-time data on food availability in a given region could support the better distribution of surpluses, which, in a time of crisis, could also be diverted to the institutions close to the charities that are catering for families in food insecure environments.
Challenges
Cognitive, physical, and social boundaries are a central element of the use of ICT-enabled boundary spanning approaches (Section Research Approach). In the Rayfood and LPB project, differences in meanings, knowledge, and language made collaboration more difficult. Cognitive boundaries were encountered inside the digitisation officer team when they tried to label food items but were unsure of how to categorize them (Section Automated Data Extraction). Cultural differences between the US (where the project nutrition information and food suppliers originated from), and New Zealand and Australia (where the AI implementation team were located), created some level of miscommunication. Despite speaking the same language, names for vegetables could be different between countries (e.g., bell pepper/capsicum, courgette/zucchini, bok choi/Chinese cabbage), and when the team contained staff from different cultural backgrounds, these differences were more pronounced. A positive outcome of the project was that both Australia and New Zealand have strong labor laws protecting the rights of workers. This is not the case for a large proportion of machine learning projects, where the people who are tasked with labeling data (i.e., ghost workers) are often located in poorer countries with little or no labor laws (Mohamed et al., 2020), opening the way for exploitation.
Limitations of the study include the fact the data used to create an overview of the boundaries is the outcome of a project focusing on other research questions, and still deserves specific research in the field. Furthermore, there was only indirect access to the school food authorities and food service directors for the research. Research directly involving this important group could bring relevant and measured information about the differences the system's features can make: for example, how it could accommodate efforts to maintain a balanced budget with options for healthier food, or, how it could support instructional leadership to the school communities and the students.
Finally, being aware of the difference between AI for sustainability and the sustainability of AI, and the implications of this difference, is an important part of how the design of solutions should be conducted. Studies of the sustainability of AI, including life-cycle-based assessments of the equipment used, the increasingly larger energy consumption of data centers, and the further impacts of disposal of electronics, are still few and far between (Jones, 2019; Strubell et al., 2019). More work needs to be done to provide designers of AI for sustainability transitions the tools of relevant data to measure the impacts of what they propose before solutions are implemented, especially in the context of food systems.
Policy and Practice Implications and Recommendations
The application of AI-powered tools to food programs, can extend administrators' and schools' abilities (a) to recognize patterns in meal consumption, (b) to predict surpluses or recommend preferred supply chains, and (c) to optimize meal planning and administration. These improved abilities could support policy objectives aimed at tightening feedback loops to transition to sustainable food systems. Institutions and providers of food programs management software could co-define the critical elements necessary for the systemic adoption of sustainable procurement methods. Elements include (but are not limited to) defining the goals of the system (i.e., sustainable transition of food systems), data necessary for decision-making (e.g., food provenance, nutritional information, costs), and level and forms of feedback (e.g., upcoming surpluses for low-cost options in institutional settings; food waste patterns to school menu planners).
Food program management systems should include the possibility of adding or selecting suppliers based on local settings, thus providing opportunities to create closer relationships between the nutritional status of communities and the procurement of healthy food produced using sustainable practices. Furthermore, the ability to order and redistribute food in local settings could bring more resilience in the supply chain.
At this relatively early stage in the evolution of AI for food systems, there is still time for civil servants, businesses, and charities to be brought together and develop shared problem definition, joint fact-finding, and vision in codesign activities. This can help to take advantage of the opportunities that AI offers, and define what data are needed and how they should be used. However, with the private sector moving quickly to occupy the AI in the food system space, public institutions and civil society need to get involved to guide the scope of solutions toward the achievement of societal goals, including enhancing the sustainability of food systems.
Conclusion
AI processes data and learns from it over time, in a manner that is similar to the human brain. The primary data collected in this study highlights the intricacies of ensuring that data is in the right format for its effective processing, and for facilitating understanding and learning by an AI algorithm. AI-powered solutions can facilitate the adoption of sustainable practices in the planning and administration of current school food programs by providing better decision-making tools and automated access to sustainable food choices. This can be achieved when the private sector and public institutions collaborate on solutions seeking to help responsiveness and agility in meal planning and administration. Overall, this case study demonstrated that ICT-enabled solutions can help bridge the gap between participants in a school food program. Furthermore, the ability to automate the extraction and verification of data to close to real-time can support transition to more sustainable food production systems (especially local ones). The case study also alerts us to the trend of using AI in food systems in school food programs, which is driven primarily by commercial interests. While all of the people involved in the studied project demonstrated a dedication to operate a system that benefits the children, it is not hard to imagine that data and information could quickly evolve toward engines that make personal recommendations based on customer data. For example, Netflix, Facebook, Google, and Amazon use recommendation engines to market theirs and their affiliates' commercial interest in terms of products and services. If this were to be the case, the manner in which the nutrition data are used could either benefit a few commercial interests or society at large.
Data Availability Statement
The original contributions presented in the study are included in the article/Supplementary Material, further inquiries can be directed to the corresponding author/s.
Ethics Statement
The studies involving human participants were reviewed and approved by RMIT University Human Research Ethics Committee/College of Human Ethics Advisory Network (CHEAN A 21722). The participants provided their written informed consent to participate in this study.
Author Contributions
The author confirms being the sole contributor of this work and has approved it for publication.
Funding
This study was supported through the Australian Government Research Training Program Scholarship. This project was partially supported by funding from Food Agility CRC Ltd., funded under the Commonwealth Government CRC Program. The CRC Program supports industry-led collaborations between industry, researchers and the community.
Conflict of Interest
The author declares that the research was conducted in the absence of any commercial or financial relationships that could be construed as a potential conflict of interest.
Publisher's Note
All claims expressed in this article are solely those of the authors and do not necessarily represent those of their affiliated organizations, or those of the publisher, the editors and the reviewers. Any product that may be evaluated in this article, or claim that may be made by its manufacturer, is not guaranteed or endorsed by the publisher.
Acknowledgments
The author would like to thank the participants in the USA, New Zealand, and Australia who so generously contributed to this research project. We would also like to acknowledge the reviewers for their support. This study took place within the context of a wider project investigating the role of artificial intelligence in the design of transitions to sustainable food systems. It is one of four case studies which examines applications of AI found in different parts of food systems and will be used to inform the approach to designing food systems transition activities using AI in subsequent phases of the larger project.
Supplementary Material
The Supplementary Material for this article can be found online at: https://www.frontiersin.org/articles/10.3389/fsufs.2022.743810/full#supplementary-material
Footnotes
1. ^Company names in this article are pseudonyms.
2. ^Data collected in this case study included information about people and from people and organisations; therefore, research ethics approval was sought and obtained from the RMIT University Research Ethics Committee (CHEAN A 21722).
3. ^Combined with AI, Advanced OCR means that data is simultaneously captured and the content comprehended (Keary, 2019). This is different from basic OCR data extraction processes, which use templates to define the coordinates of the text to capture. If the document format changes slightly (for example, an invoice number which used to be on the top left corner is now in the bottom right corner), then the OCR accuracy declines, and important data may be omitted.
4. ^Natural language processing is the AI discipline that is used to make meaning out of extracted text. It is done by the algorithm reading the text, deciphering it, and understanding how it relates to the extracted data.
5. ^Image recognition identifies objects or features in an image or video.
6. ^Machine learning is a branch of AI and computer science which focuses on the use of data and algorithms to imitate the way that humans learn, gradually improving its accuracy (IBM Cloud Education, 2020).
References
Abson, D. J., Fischer, J., Leventon, J., Newig, J., Schomerus, T., Vilsmaier, U., et al. (2017). Leverage points for sustainability transformation. Ambio 46, 30–39. doi: 10.1007/s13280-016-0800-y
Aleksejeva, L., and Pelse, M. (2019). “Procurement of organic food by Latvian schools,” in Economic Science for Rural Development 2019, ed A. Auzina, Economic Science for Rural Development, Latvia Uiversity Life Sciences & technologies, LIELA IELA 2, JELGAVA, LV-3001, LATVIA, 305–311.
Ashiabi, G. (2005). Household food insecurity and children's school engagement. J. Children Poverty 11, 3–17. doi: 10.1080/1079612042000333027
Baidal, J. A. W., and Taveras, E. M. (2014). Protecting Progress against childhood obesity — the national school lunch program. New Engl. J. Med. 371, 1862–1865. doi: 10.1056/NEJMp1409353
Blizzard, J. L., and Klotz, L. E. (2012). A framework for sustainable whole systems design. Design Stud. 33, 456–479. doi: 10.1016/j.destud.2012.03.001
Bourguet, J.-R., Thomopoulos, R., Mugnier, M.-L., and Abecassis, J. (2013). An artificial intelligence-based approach to deal with argumentation applied to food quality in a public health policy. Expert Syst. Appl. 40, 4539–4546. doi: 10.1016/j.eswa.2013.01.059
Cabrera, D., Colosi, L., and Lobdell, C. (2008). Systems thinking. Eval. Program Plann. 31, 299–310. doi: 10.1016/j.evalprogplan.2007.12.001
Camaréna, S. (2020). Artificial Intelligence in the design of transition to sustainable food systems. J. Clean. Prod. 271, 122574. doi: 10.1016/j.jclepro.2020.122574
Coleman-Jensen, A., Rabbit, M. P., Gregory, C. A., and Singh, A. (2020). Household Food Security in the United States in 2019, Economic Research Service. Available online at: https://www.ers.usda.gov/publications/pub-details/?pubid=99281 (accessed May 24, 2020).
Dorninger, C., Abson, D. J., Apetrei, C. I., Derwort, P., Ives, C. D., Klaniecki, K., et al. (2020). Leverage points for sustainability transformation: a review on interventions in food and energy systems. Ecol. Econ. 171, 106570. doi: 10.1016/j.ecolecon.2019.106570
Feeding America (2021). The National School Lunch Program (NSLP). Available online at: https://www.feedingamerica.org/take-action/advocate/federal-hunger-relief-programs/national-school-lunch-program (accessed June 2, 2021).
Fisher, D. H. (2011). Computing and AI for a sustainable future. IEEE Intell. Syst. 26, 14–18. doi: 10.1109/MIS.2011.98
Fitzpatrick, K. M., Harris, C., Drawve, G., and Willis, D. E. (2021). Assessing food insecurity among US adults during the COVID-19 pandemic. J. Hunger Environ. Nutr. 16, 1–18. doi: 10.1080/19320248.2020.1830221
Food Agriculture Organization (2018). Sustainable Food Systems – Concept and Framework, Rome, Italy. http://www.fao.org/3/ca2079en/CA2079EN.pdf
Forestell, C. A., and Mennella, J. A. (2007). Early determinants of fruit and vegetable acceptance. Pediatrics 120, 1247–1254. doi: 10.1542/peds.2007-0858
Galanakis, C. M. (2020). The food systems in the era of the coronavirus (COVID-19) pandemic crisis. Foods 9, 523. doi: 10.3390/foods9040523
Garcia-Herrero, L., Costello, C., De Menna, F., Schreiber, L., and Vittuari, M. (2021). Eating away at sustainability. Food consumption and waste patterns in a US school canteen. J. Cleaner Prod. 279, 123571. doi: 10.1016/j.jclepro.2020.123571
Gleason, P., Hulsey, L., and Burghardt, J. (2004). Evaluation of the National School Lunch Program Application/Verification Pilot Projects Volume V: Analysis of Applications, Alexandria, VA.
Glover, D., and Sumberg, J. (2020). Youth and food systems transformation. Front. Sustain. Food Syst. 4, 1–15. doi: 10.3389/fsufs.2020.00101
Goggins, G., and Rau, H. (2016). Beyond calorie counting: assessing the sustainability of food provided for public consumption. J. Clean. Prod. 112, 257–266. doi: 10.1016/j.jclepro.2015.06.035
Gundersen, C., Hake, M., Dewey, A., and Engelhard, E. (2021). Food insecurity during COVID-19. Appl. Econ. Perspect. Policy 43, 153–161. doi: 10.1002/aepp.13100
Herrero, M., Hugas, M., Lele, U., Wira, A., and Torero, M. (2021). Shift to healthy and sustainable consumption patterns - a paper on action track 2 - UN food systems summit 2021. Scientific Group for the UN Food Systems Summit, 1–26.
Hopkins, L. C., and Gunther, C. (2015). A historical review of changes in nutrition standards of USDA child meal programs relative to research findings on the nutritional adequacy of program meals and the diet and nutritional health of participants: Implications for future research and the summ. Nutrients 7, 10145–10167. doi: 10.3390/nu7125523
How, M. L., Chan, Y. J., and Cheah, S. M. (2020). Predictive insights for improving the resilience of global food security using artificial intelligence. Sustainability. 12, 6272. doi: 10.3390/su12156272
IBM Cloud Education (2020). Machine Learning, 12p. Avialble online at: https://www.ibm.com/cloud/learn/machine-learning (accessed June 16, 2021).
International Telecommunication Union (2018). The AI for Good Global Summit: AI for UN Sustainable Development Goals. International Telecommunication Union (2018), no. 15–17. Available online at: https://www.itu.int/en/ITU-T/AI/2018/Pages/default.aspx (accessed July 1, 2021).
Izumi, B. T., Bersamin, A., Shanks, C. B., Grether-Sweeney, G., and Murimi, M. (2018). The US national school lunch program: a brief overview. Japanese J. Nutr. Diet. 76, S126–S132. doi: 10.5264/eiyogakuzashi.76.S126
Jones, N. (2019). How to stop data centres from gobbling up the world's electricity. Nature 561, 163–166. doi: 10.1038/d41586-018-06610-y
Jorgensen, D. L. (1989). Participant Observation: A Methodology for Human Studies. Los Angeles: SAGE Publications.
Keary, T. (2019). AI and OCR: How Optical Character Recognition Is Being Revitalised. Information Age. Available online at: https://www.information-age.com/optical-character-recognition-tools-ocr-ai-123479324/ (accessed June 16, 2021).
Lovins, A., Bendewald, M., and Kinsley, M. (2010). Factor Ten Engineering Design Principles, Basalt, CO. Available online at: https://rmi.org/insight/factor-ten-engineering-design-principles (accessed July 17, 2021).
Mensah, C., and Karriem, A. (2021). Harnessing public food procurement for sustainable rural livelihoods in South Africa through the national school nutrition programme: a qualitative assessment of contributions and challenges. Sustainability 13, 13838. doi: 10.3390/su132413838
Mohamed, S., Png, M. T., and Isaac, W. (2020). Decolonial AI: decolonial theory as sociotechnical foresight in artificial intelligence. Philos Technol. 33, 659–684. doi: 10.1007/s13347-020-00405-8
Nishant, R., Kennedy, M., and Corbett, J. (2020). Artificial intelligence for sustainability: challenges, opportunities, and a research agenda. Int. J. Inf. Manage. 53, 102104. doi: 10.1016/j.ijinfomgt.2020.102104
Oostindie, H., Hebinck, P., Villarreal, G., Hebinck, A., Grando, S., and Galli, F. (2016). Practice-Led FNS Redesigning in Europe, Report EU KBBE.2013.2.5-01 Grant Agreement no: 613532, Pisa, Italy.
Pagliarino, E., Santanera, E., and Falavigna, G. (2021). Opportunities for and limits to cooperation between school and families in sustainable public food procurement. Sustainability 13, 8808. doi: 10.3390/su13168808
Perez-Neira, D., Simon, X., and Copena, D. (2021). Agroecological public policies to mitigate climate change: public food procurement for school canteens in the municipality of Ames (Galicia, Spain). Agroecol Sustain Food Syst. 45, 1528–1553. doi: 10.1080/21683565.2021.1932685
Pollan, M. (2020). The Sickness in Our Food Supply | by Michael Pollan | The New York Review of Books. The New York review of Books. Available online at: https://www.nybooks.com/articles/2020/06/11/covid-19-sickness-food-supply (accessed May 24, 2020).
Radday, K. (2020). Dissertation Development of the National School Lunch Program From an Agricultural Support to a Child Welfare Program, Notre Dame of Mayrland University.
Rafidi, J. A., Sanders, M., and Member, C. (2012). Implementing an Effective and Efficient System to Manage the National School Lunch Program ub a Private PREK-12 School: An Action Research Study, Capella University.
Ralston, K., Newman, C., Clauson, A., Guthrie, J., and Buzby, J. (2008). The National School Lunch Program: Background, Trends, and Issues. Available online at: https://ageconsearch.umn.edu/record/56464 (accessed June 3, 2021).
Rittel, H. W. J., and Webber, M. M. (1973). Dilemmas in a general theory of planning. Policy Sci. 4, 155–169. doi: 10.1007/BF01405730
Roche, E., and Kolodinsky, J. M. (2011). Overcoming barriers to providing local produce in school lunches in Vermont. J. Agric. Food Syst. Commun. Develop. 1, 89–97. doi: 10.5304/jafscd.2011.013.012
Rowley, J. (2002). Using case studies in research. Manage. Res. News 25, 16–27. doi: 10.1108/01409170210782990
Sætra, H. S. (2021). AI in context and the sustainable development goals: factoring in the unsustainability of the sociotechnical system. Sustainability 13, 1–19. doi: 10.3390/su13041738
Strubell, E., Ganesh, A., and McCallum, A. (2019). “Energy and policy considerations for deep learning in NLP,” in 57th Annual Meeting of the Association for Computational Linguistics, Florence, Italy.
Sundkvist, Å., Milestad, R., and Jansson, A. (2005). On the importance of tightening feedback loops for sustainable development of food systems. Food Policy 30, 224–239. doi: 10.1016/j.foodpol.2005.02.003
Termeer, C., and Bruinsma, A. (2016). ICT-enabled boundary spanning arrangements in collaborative sustainability governance. Curr. Opin. Environ. Sustain. 18, 91–98. doi: 10.1016/j.cosust.2015.11.008
Termeer, C., Dewulf, A., Breeman, G., and Stiller, S. J. (2015). Governance capabilities for dealing wisely with wicked problems. Adm. Soc. 47, 680–710. doi: 10.1177/0095399712469195
Thomas, M. M. C., Miller, D. P., and Morrissey, T. W. (2019). Food insecurity and child health. Pediatrics 144, e20190397. doi: 10.1542/peds.2019-0397
USDA (2015). Report of School Program Operations. Available online at: https://fns-prod.azureedge.net/sites/default/files/cn/FNS-10.pdf (accessed October 21, 2019).
USDA (2019a). National School Lunch Program (NSLP) Fact Sheet. USDA Food and Nutrition Service. Available online at: https://www.fns.usda.gov/nslp/nslp-fact-sheet (accessed February 4, 2020).
USDA (2019b). Nutrition Standards for School Meals. Technical Assistance and Guidance. Available online at: https://www.fns.usda.gov/cn/nutrition-standards-school-meals (accessed November 9, 2020).
Vågsholm, I., Arzoomand, N. S., and Boqvist, S. (2020). Food security, safety, and sustainability—getting the trade-offs right. Front. Sustain. Food Syst. 4, 1–14. doi: 10.3389/fsufs.2020.00016
Valaitis, R. F., Hanning, R. M., and Herrmann, I. S. (2014). Programme coordinators' perceptions of strengths, weaknesses, opportunities and threats associated with school nutrition programmes. Public Health Nutr. 17, 1245–1254. doi: 10.1017/S136898001300150X
Vinuesa, R., Langhans, S. D., Azizpour, H., Leite, I., Balaam, M., Dignum, V., et al. (2020). The role of artificial intelligence in achieving the sustainable development goals. Nat. Commun. 11, 1–10. doi: 10.1038/s41467-019-14108-y
Vogt, R. A., and Kaiser, L. L. (2008). Still a time to act: a review of institutional marketing of regionally-grown food. Agric. Hum. Values 25, 241–255. doi: 10.1007/s10460-007-9106-9
Wittman, H., and Blesh, J. (2017). Food sovereignty and fome zero: connecting public food procurement programmes to sustainable rural development in Brazil. J. Agrarian Change 17, 81–105. doi: 10.1111/joac.12131
Worstell, J. (2020). Ecological resilience of food systems in response to the COVID-19 crisis. J. Agric. Food Syst. Commun. Develop. 9, 1–8. doi: 10.5304/jafscd.2020.093.015
Keywords: food security, sustainable food systems, artificial intelligence, systems thinking, information management, school food programs, child nutrition
Citation: Camaréna S (2022) Artificial Intelligence (AI) for Sustainable Institutional Food Systems: Implementation of AI Tools for School Nutrition Program Management in the United States of America. Front. Sustain. Food Syst. 6:743810. doi: 10.3389/fsufs.2022.743810
Received: 19 July 2021; Accepted: 13 April 2022;
Published: 02 June 2022.
Edited by:
Patrick Meyfroidt, Catholic University of Louvain, BelgiumReviewed by:
Andrew Mitchell, De Montfort University, United KingdomAlexandros Gasparatos, The University of Tokyo, Japan
Copyright © 2022 Camaréna. This is an open-access article distributed under the terms of the Creative Commons Attribution License (CC BY). The use, distribution or reproduction in other forums is permitted, provided the original author(s) and the copyright owner(s) are credited and that the original publication in this journal is cited, in accordance with accepted academic practice. No use, distribution or reproduction is permitted which does not comply with these terms.
*Correspondence: Stéphanie Camaréna, c3RlcGhhbmllLmNhbWFyZW5hQHN0dWRlbnQucm1pdC5lZHUuYXU=