- Institute of Meteorology and Climate Research, Atmospheric Environmental Research (IMK-IFU), Karlsruhe Institute of Technology, Garmisch-Partenkirchen, Germany
Substantial increases in cereal yields are necessary if a growing global demand for food is to be met without further conversion of natural to agricultural land. However, since in many regions yields are limited by soil nutrient availability, this will increase the requirement for fertilizer inputs, specifically of nitrogen (N). Here we focus on maize cultivation, and investigate the trade-off between yield increases and environmentally harmful N-losses in the form of nitrous oxide (N2O) emissions and nitrate () leaching. We model the evolution of N-losses as yield gaps—the difference between actual and potential yields—are closed. To do this we use the process-based, biogeochemical model LandscapeDNDC to perform global simulations on a 0.5° grid, and evaluate the response of yields and environmental N-losses to changes in N-inputs. Our simulations find current production (circa 2015) of 954 Tg (5.1 Mg/ha), direct and indirect N2O emissions of 416 Gg-N (2.2 kg-N/ha or 0.44 kg-N/Mg) and leaching of 5.9 Tg-N (31.5 kg-N/ha or 6.2 kg-N/Mg). We demonstrate that, under an “optimal” strategy for closing yield gaps, maize yields could be increased by 20–25% with concomitant stable or even slightly decreased yield-scaled N-losses. However, further yield increases would come at an ever accelerating cost in environmentally harmful N losses. This acceleration occurs when yields exceed ~70–80% of their potential.
Introduction
The global demand for cereals is rising, due to an expanding population and changes in dietary preferences (Tilman et al., 2011; Alexandratos and Bruinsma, 2012; Hunter et al., 2017; FAO, 2018). In order to meet this demand, it has been predicted that cereal production will need to increase 25–70% in the period 2015–2050 (Hunter et al., 2017). In principle, the additional production could come either from expanding the total cropland area or from increasing yields. However, while both agricultural expansion and intensification have their associated problems, many authors view intensification as being the lesser of two evils, in terms of biodiversity loss, greenhouse gas emissions and degradation of ecosystems (Godfray et al., 2010; Foley et al., 2011; Tilman et al., 2011; Suh et al., 2020). This has led to a focus on yield gaps—the difference between actual yields and potential yields—and how these can be closed so as to increase crop production without expanding the cultivated area (Cassman, 1999; Cassman et al., 2003; van Ittersum et al., 2013).
There are many possible strategies for closing yield gaps (van Ittersum et al., 2013), but one thing common to all of them is the necessity to supply sufficient nutrients to the crops. One of the most important of these is nitrogen (N), and in many parts of the world N availability is either the limiting factor for crop yields (Mueller et al., 2012), or would quickly become limiting in the case that other influences, such as lack of market accessibility, were ameliorated (Neumann et al., 2010).
The aim of this paper is to quantify the environmental N losses associated with closing yield gaps, in particular the greenhouse gas nitrous oxide (N2O) and the eutrophying water pollutant nitrate (). A further aim is to identify regions where yield gaps could be closed with the lowest impact on the environment. As such, we assume that N fertilizers are generally available and that other potentially limiting nutrients, such as phosphorous, are supplied commensurately with N-inputs. We focus on N2O emissions and leaching, since they are two of the most harmful ways in which N can be lost from agricultural soils. N2O is both a significant greenhouse gas, with 265–298 times the global warming potential per unit mass of carbon dioxide over a 100-year period (Pachauri et al., 2014), and the dominant ozone-depleter of the twenty-first century (Ravishankara et al., 2009). degrades ground and surface water quality, causing large-scale human health effects and eutrophication (Carpenter et al., 1998; WHO, 2017). Additionally, both and ammonia (NH3) can be converted to N2O in the wider environment.
To assess to what extent closing yield gaps would result in additional N losses to the environment, and where the effects would be most pronounced, we focus on one crop, maize. Maize cultivation accounts for 26% of the worldwide harvested area of cereals (circa 2015), has a wide geographical distribution (FAO, 2021) and is dependent on N fertilization. We consider conditions typical of 2015, assuming that similar conditions will prevail in the near future, and use the biogeochemical model LandscapeDNDC to assess both the magnitude of global yield gaps and the effect closing yield gaps would have on N-fertilizer requirements, N2O emissions and leaching.
This is not the first paper to consider the link between yield-gap closing and additional N usage on a global scale (Mueller et al., 2012, 2014; Liu et al., 2018). However, these previous papers have left a number of important points unanswered, which are here addressed: (1) Rather than studying a small number of fixed scenarios for N additions (Mueller et al., 2012; Liu et al., 2018), we here determine continuous N-input response curves at a grid-cell level, including the response of yield, N2O emissions and leaching to additional N fertilizer usage. As a consequence, we are able to study spatially heterogeneous yield-gap closing strategies, including deriving an “optimal” strategy for intensifying maize production with the lowest possible N additions. (2) As opposed to reporting aggregate N losses (Liu et al., 2018), we here explicitly determine N2O emissions and leaching. As such, we focus on the most harmful N losses, and avoid masking these with large-scale but benign losses such as N2 emissions. This allows us to better determine where yield gaps can be closed at low environmental cost.
Methods
Model Setup and Data Inputs
The model LandscapeDNDC (LDNDC) was used to simulate maize production in circa 2015 conditions on a global grid of 0.5° (~50 km close to the equator). LDNDC is a process-based biogeochemical model that simulates major carbon and nitrogen flows, as well as plant growth and water cycling on a sub-daily timestep (Haas et al., 2013). It has been parameterized using a wide variety of site measurements across temperate (Molina-Herrera et al., 2016, 2017), tropical (Kraus et al., 2015, 2016) and savannah (Rahimi et al., 2021) ecosystems, and successfully used to study N2O emissions and leaching (Kiese et al., 2011; Kraus et al., 2015, 2016; Dirnböck et al., 2016; Molina-Herrera et al., 2016, 2017; Houska et al., 2017; Kasper et al., 2019; Schroeck et al., 2019). It performs comparably to other state-of-the-art crop models when simulating maize yields on a global scale (Jägermeyr et al., 2021).
For this study, we used LDNDC version 1.32.3, including the soil-chemistry module MeTrx (Kraus et al., 2015, 2016), the plant growth model PlaMox (Kraus et al., 2016), the hydrology module WatercycleDNDC (Kiese et al., 2011) and the microclimate module CanopyECM (Grote et al., 2009). The strengths of LDNDC include a detailed, process-based description of N cycling, which has been extensively calibrated against measured data for N2O emissions and leaching. The weaknesses include an assumption of homogeneous conditions parallel to the soil surface (i.e., in this study homogeneity within each 0.5 degree grid cell).
Model inputs were largely based on those used in the Global Gridded Crop Model Intercomparison (GGCMI) project (Jägermeyr et al., 2021); the state of the art for global crop modeling. Soil data was taken from the harmonized world soil database (HWSD, 2012). The climate data was taken from the repository of the Inter-Sectoral Impact Model Intercomparison Project (ISIMIP), and in particular the GSWP3-W5E5 product, which provides climate data for the years 1901–2016 (Dirmeyer et al., 2006; Lange, 2019; Cucchi et al., 2020). Air-chemistry data was also adapted from the ISIMIP repository. Monthly, grid-cell-dependent and deposition rates for the year 2015 (Tian et al., 2018a) were scaled to daily values, while atmospheric CO2 concentrations were set to the average 2015 value of 399.9 ppm.
Maize planting dates were fixed according to the gridded crop calendar developed for the GGCMI project (Jägermeyr et al., 2021). Field management was also set in accordance with the GGCMI project. Tilling was performed 1 day before planting and again 1 day after harvesting, to a depth of 20 cm. Fertilization was split between two applications, with 20% of the total on the planting day, and the remainder after reaching 25% of the growing degree days required for crop maturity. After harvesting, 70% of the crop residuals were removed from the field.
Model Calibration
LDNDC is calibrated against field-scale measurement data for a wide range of different agro-ecosystems (Kraus et al., 2015, 2016; Molina-Herrera et al., 2016; Rahimi et al., 2021). Here, we used the crop calendar to additionally calibrate the cumulative growing degree days required for plants to reach maturity, with the aim of modeling the different maize cultivars grown in different regions. For each grid cell, the growing degree days required for maturity were set to the mean number of growing degree days between planting and harvesting dates in the period 1980–2010. The growing degree days required for other plant developmental stages were adjusted consistently. During the subsequent simulations, harvest was performed not on the date specified by the crop calendar, but at physiological maturity, whose date varied from year to year depending on the climatic conditions during the growing season.
Simulation Runs
We aimed to simulate agricultural conditions typical of 2015, assuming that similar conditions will prevail in the near future, and the input data was chosen accordingly. Nevertheless, we ran the model using climate data for the period 1986–2016, with the first 20 years treated as spin up, and model outputs averaged over the period 2006–2016. The reason for performing a 10-year average, rather than just using 2015 climate data, was to reduce the bias associated with the timing of specific weather events. For example, heavy rainfall shortly after fertilization that results in unusually high leaching.
Baseline simulations were run using gridded N-fertilizer-application-rates data for the year 2015, which was developed for the GGCMI project (Jägermeyr et al., 2021). For subsequent simulations, synthetic N-fertilizer inputs were set to 0, 20, 40, 60, 80, 100, 120, 140, 160, 180, 200, 220, 240, 260, 280, 300, 350, 400, 500, and 600 kg-N/ha, while manure supply was kept fixed at the 2015 level. The balance between urea and ammonium-nitrate synthetic fertilizer was set on a grid-cell basis according to the dataset reported in Nishina et al. (2017). Separate simulations were performed for rain-fed and irrigated water management. Irrigation was assumed to be sufficient to avoid stress associated with lack of water.
Model Outputs, Data Analysis, and Scenarios
Model Outputs
The model outputs considered were yield (Mg/ha), N2O emissions (kg-N/ha), leaching (kg-N/ha) and NH3 volatilization (kg-N/ha). Total N2O emissions were calculated by summing the direct emissions at the site of fertilizer application and indirect emissions associated with conversion of and NH3 to N2O in the wider environment. The indirect emissions were calculated using the emission factors given in the 2019 refinement to the 2006 IPCC guidelines (IPCC, 2019), which stipulate that 1.1% of leached and 1% of volatilized NH3 are converted to N2O. Continuous fertilizer-response curves for each model output and for each grid-cell were constructed by interpolating between simulation results with different N-fertilizer inputs (see Supplementary Figures 1, 2).
Harvested-area data was used to convert the per-hectare model outputs into total production and N-loss values in each grid cell. The harvested areas were based on the MIRCA2000 dataset, which gives rain-fed and irrigated areas for approximately the year 2000 (Portmann et al., 2010). However, since the total harvested area has increased considerably in recent years, from 137 Mha in 2000 to 191 Mha in 2015 (FAO, 2021), areas were scaled on a country-by-country basis to make them consistent with the FAOSTAT statistics for 2015 (FAO, 2021). During this scaling, the relative distribution of cropland within a country and the irrigated fraction within each grid cell were held constant. Only grid cells with >500 ha of harvested area were simulated, both to reduce the computational effort and to avoid skewing map-based visualizations of the results toward regions with very little maize agriculture. As a result, the total simulated area was 188.4 Mha, corresponding to ~99% of the total harvested area. For grid cells containing both rain-fed and irrigated areas, simulation results were combined according to the ratio between the two areas.
Calculated Parameters: Potential Yield, Fractional Yield, and Yield-Scaled N Losses
Two of the most important concepts used throughout the paper are the potential and fractional yields. The potential yield is here defined as the maximum yield obtainable in a grid cell by varying the N-fertilizer amount. This can be easily extracted from the yield vs. N-fertilizer curve, which typically shows saturation behavior at high N input rates (see Supplementary Figures 1, 2 for examples). Potential yields at regional or global scale are determined from those at grid-cell scale by taking a harvested-area weighted average.
The fractional yield measures how close the actual yield of a grid cell is to its potential yield, and is given by:
The fractional yield is thus a function of the N-fertilizer input, and typically increases with increasing N input. It approaches 1 for high N-input rates, due to the actual yield approaching the potential yield. Fractional yields at the regional or global scale are determined from a harvested-area weighted average of grid cells.
N losses in the form of N2O emissions and leaching were put into an agronomic context by calculating yield-scaled losses (kg-N lost per Mg production). The yield-scaled N loss can increase or decrease with increasing N input, depending on whether the yield or the N loss has a stronger response (see Supplementary Figures 1, 2 for examples). Since we are investigating yield-gap closing, of particular interest are the additional N losses (ΔN) and yield (ΔY) that arise from an increase in N-fertilizer input (also ΔN). ΔN/ΔY thus measures the N intensity of additional production. A priori, we expect that ΔN/ΔY will increase as the fractional yield increases. To test this hypothesis, we plotted ΔN/ΔY against fractional yield, where ΔN/ΔY was calculated using ΔY = 0.01 Mg/ha. Grid cells were binned according to their fractional yield (bin width 0.02), and for each bin the 50th (i.e., the median), 10th and 90th percentiles of ΔN/ΔY were determined and plotted against the fractional yield.
Regions
In addition to grid-cell and global scales, we also performed analysis at regional scale. We defined 11 world regions, as shown in Supplementary Figure 3. All of the regions contain a significant fraction of the total global harvested area of maize (>1%) except for Oceania, which has only 0.04 Mha (0.02% of the global total). Regional crop production, N-fertilizer usage, N2O emissions and leaching are shown in Table 1, while regional crop yield, N-fertilizer application rate, yield-scaled N2O emissions and yield-scaled leaching are shown in Table 2.
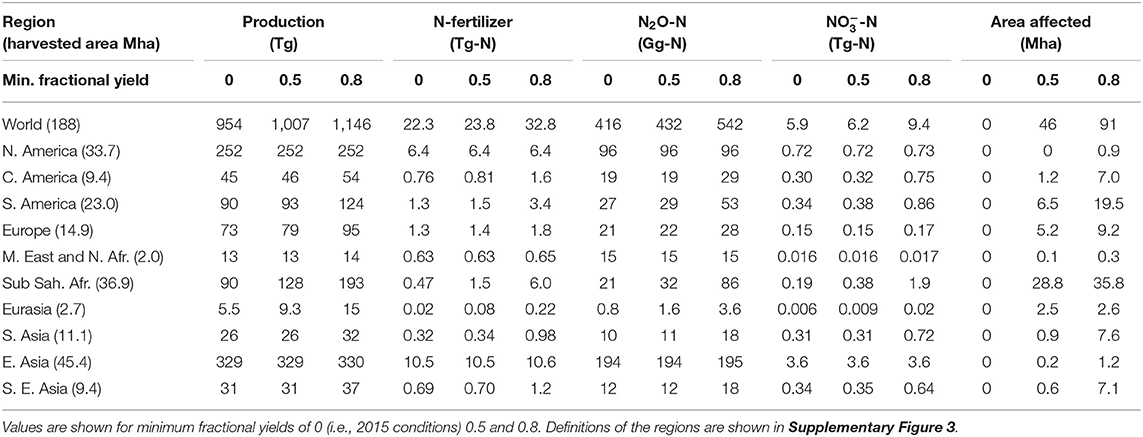
Table 1. The effect of following the “minimum fractional yield” pathway on maize production, N-fertilizer usage, direct and indirect N2O emissions, leaching and the affected harvested area.
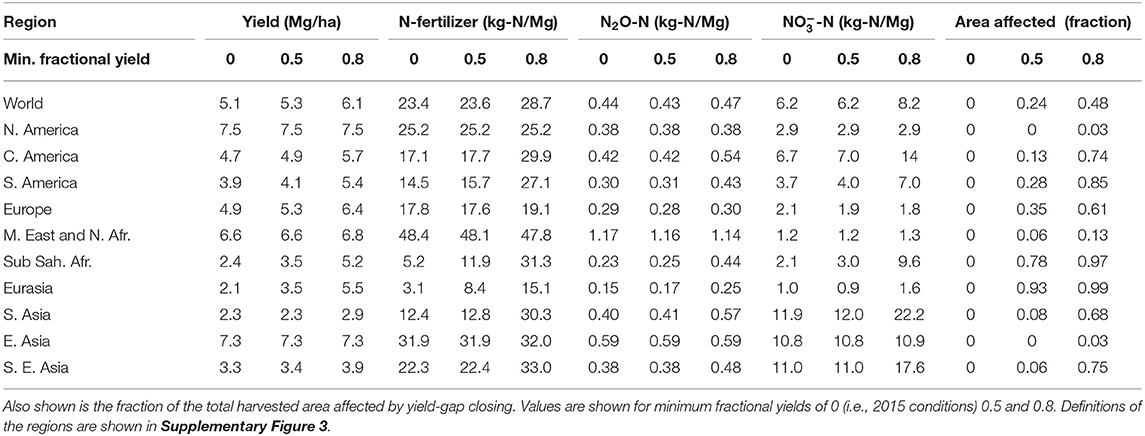
Table 2. The effect of following the “minimum fractional yield” pathway on maize yields, yield-scaled N-fertilizer usage, yield-scaled N2O emissions (direct and indirect) and yield-scaled leaching.
Scenarios
We investigated two scenarios for closing yield gaps, in both cases starting from 2015 conditions:
1) Minimum fractional-yield scenario: A minimum fractional yield is specified, and additional N-fertilizer allocated such that all grid cells attain this minimum. For example, if the minimum fractional yield is 0.5, then a grid cell with a fractional yield of 0.4 would receive sufficient additional N fertilizer to increase its fractional yield to 0.5. A grid cell with a fractional yield of 0.6 would not receive any additional N fertilizer. A yield-gap-closing pathway is defined as gradually increasing the minimum fractional yield from 0 (no closing of yield gaps) to 1 (full closing of yield gaps).
2) “Optimal” scenario: A given amount of additional global production is specified (e.g., 100 Tg), and the minimum amount of additional N-fertilizer allocated in order to reach this production goal. A yield-gap-closing pathway is defined as gradually increasing the additional production goal from 0 Tg to the maximum attainable value of 334 Tg (resulting in a total production of 1,291 Tg).
For the “minimum fractional-yield” scenario, grid cells that would otherwise have a fractional yield below the specified minimum were allocated additional N fertilizer according to their yield vs. N-fertilizer-application-rate curve (see Supplementary Figures 1, 2 for examples). From these curves, one can read off the change in N-application rate required to reach the targeted minimum fractional yield. For the “optimal” scenario, minimization of the additional N-fertilizer input was carried out using parallel-tempering Monte Carlo (Newman and Barkema, 1999). In principle, rather than minimizing N fertilizer usage, we could instead have chosen to minimize either the additional N2O emissions or the additional leaching. However, since it is only possible to minimize one variable, we assumed that minimization of N fertilizer inputs is a good way to reduce all harmful N losses.
Results
Actual and Potential Yields
Total N-fertilizer input, circa 2015, was 25.5 Tg-N, consisting of 22.3 Tg-N of synthetic fertilizer and 3.2 Tg-N of manure. In addition, there was 2.0 Tg-N of atmospheric deposition. This corresponds to an average N-input of 146 kg-N/ha (135 kg-N/ha of synthetic and organic fertilizer). However, there were large regional differences in N-fertilizer usage, as can be seen in Table 1. Using these N-inputs, we simulated a total global maize production of 954 Tg, giving a global average yield of 5.1 Mg/ha, and corresponding to yield-scaled synthetic N-fertilizer usage of 23.4 kgN/Mg.
In conditions of global N sufficiency, we found that production would increase to 1,291 Tg, resulting in an average global yield of 6.9 Mg/ha. Actual production is thus 74% of potential production (i.e., the average global fractional yield is 0.74). Equivalently, maize production could be increased by 35% without increasing the harvested area. However, fractional yields differ significantly between grid cells, as can be seen in Figure 1A. This remains the case at regional scale, where North America (0.97) and East Asia (0.96) have fractional yields close to the maximum, while Sub-Saharan Africa (0.37) and Eurasia (0.30) are far below their potential. Europe (0.66) is split between a higher fractional yield west and lower fractional yield east. Yield-scaled synthetic N-fertilizer usage also shows large differences between grid cells, as shown in Figure 1B. At regional scale it varies from 3.1 kgN/Mg in Eurasia to 48.4 kgN/Mg in the Middle East and North Africa (see Table 2).
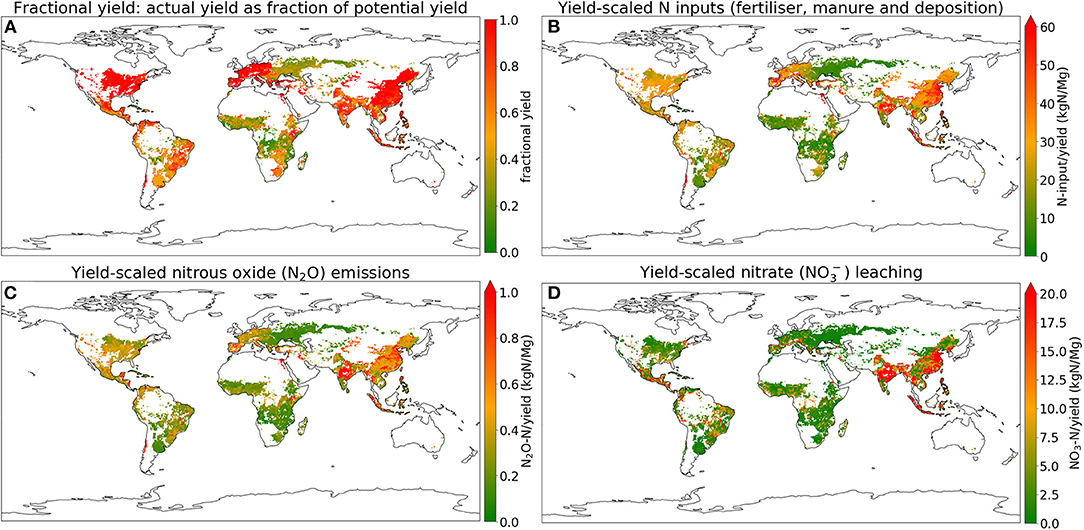
Figure 1. Simulated yields, N inputs and N losses associated with maize cultivation in conditions typical of the year 2015. Simulations were performed on a 0.5° global grid using the biogeochemical model LandscapeDNDC. (A) Fractional yield (actual yield/potential yield, where potential yield is defined as the maximum attainable yield in conditions of nutrient sufficiency). (B) Yield-scaled N-inputs (synthetic fertilizer, manure, and deposition). (C) Yield-scaled nitrous oxide (N2O) emissions (direct and indirect). (D) Yield-scaled nitrate () leaching.
Circa 2015 N Inputs and Losses
We calculated global direct N2O emissions of 315 Gg-N, corresponding to an average of 1.67 kg-N/ha, or, on a yield-scaled basis, 0.33 kg-N/Mg. Direct N2O emissions thus account for 1.2% of the N contained in synthetic and organic fertilizer inputs to the soil. We determined indirect N2O emissions of 101 Gg-N, corresponding to an average of 0.54 kg-N/ha or 0.11 kg-N/Mg. Summing the direct and indirect emissions results in N2O emissions of 416 Gg-N, corresponding to an average of 2.21 kg-N/ha or 0.44 kg-N/Mg. Figure 1C shows the global distribution of yield-scaled N2O emissions across grid cells, with high values in Western Europe and across Asia. On a regional scale, yield-scaled-emission rates range from 0.15 kgN/Mg in Eurasia to 1.17 kgN/Mg in the Middle East and North Africa (see Table 2).
We simulated total leaching of 5.9 Tg-N, with an average of 31.3 kg-N/ha, or, on a yield-scaled basis, 6.2 kg-N/Mg. Figure 1D shows the distribution of yield-scaled leaching rates across grid cells, and it can be seen that rates are particularly high across South and East Asia. Regional values range from 1.0 kgN/Mg in Eurasia to 11.9 kgN/Mg in South Asia, with East Asia (10.8 kg-N/Mg) and South-East Asia (11.0 kg-N/Mg) also close to the top of the range (see Table 2).
The Effect of Increasing Yields on N Losses
To determine where yield gaps could be closed at the lowest cost in harmful N losses, it is necessary to quantify the additional N-fertilizer requirements and N losses (ΔN) associated with yield increases (ΔY). Figure 2A shows the additional N fertilizer required to increase yields (i.e., ΔN/ΔY), and Figures 2C,D show the ΔN/ΔY's for N2O emissions and leaching. Grid cells with high fractional yields typically require large amounts of additional N fertilizer to drive yield increases, as can be seen by comparing Figures 2A,C,D with Figure 1A. This relationship is quantified in Figure 2B, which shows the dependence of ΔN/ΔY on fractional yield. For fractional yields >0.7–0.8 there is a steep rise in the additional N fertilizer required to increase yields. A similar pattern is seen for N2O emissions and leaching (see Supplementary Figure 4).
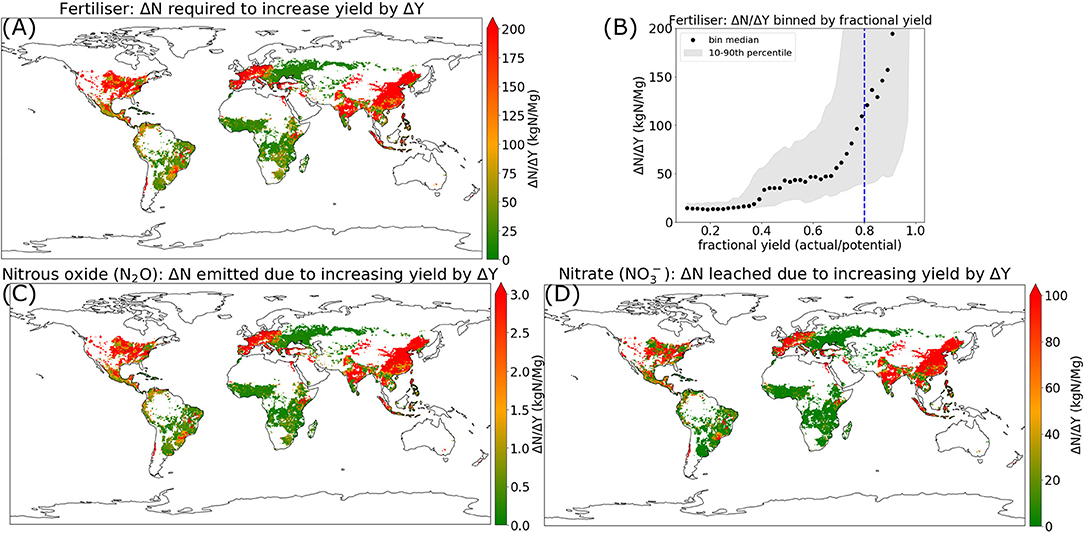
Figure 2. The additional N inputs and losses associated with yield increases, measured as kg's of additional N (ΔN) per Mg of additional production (ΔY). (A) ΔN/ΔY for N-fertilizer inputs. (B) The dependence of ΔN/ΔY for N-fertilizer on the fractional yield. Grid cells are sorted into bins according to their fractional yield (width 0.02). For each bin, the median value of ΔN/ΔY is shown as a black dot and the spread (10th−90th percentiles) by the gray shaded region. (C) ΔN/ΔY for direct and indirect N2O emissions. (D) ΔN/ΔY for leaching.
Targeting Additional N-Inputs at Regions With the Lowest Fractional Yields
Figure 3 shows the effect of following the “minimum fractional yield” scenario for yield-gap closing. Setting a minimum fractional yield of 0.5 results in production increasing to 1.06 times its current value, N-fertilizer requirements to 1.07, direct and indirect N2O emissions to 1.04 and leaching to 1.05. Since the relative increase in production is almost identical to the increase in N-fertilizer requirements and N-losses, there is very little change in the yield-scaled values, as can be seen in Table 2. Further increasing the minimum fractional yield to 0.8 results in production of 1.20 times the current value, N-fertilizer requirements of 1.47, direct and indirect N2O emissions of 1.30 and leaching of 1.58. As a result, there is an increase in yield-scaled N-fertilizer requirements and N-losses, as can be seen in Table 2. Beyond a fractional yield of 0.8, increases in the N-fertilizer requirements and N-losses significantly decouple from production increases, resulting in a rapid acceleration of yield-scaled N-losses.
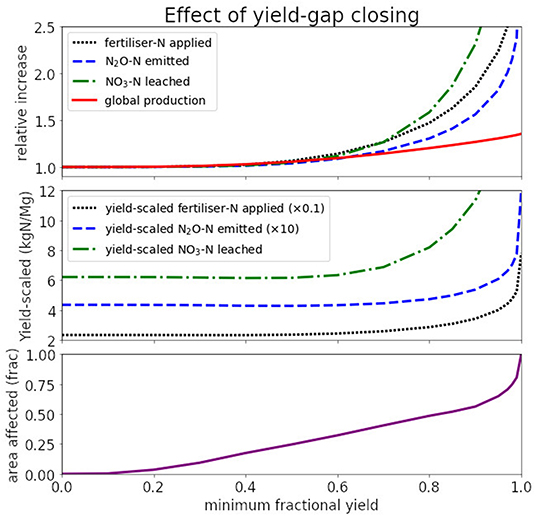
Figure 3. Yield-gap closing following the “minimum fractional yield” scenario. (Top) The evolution of production, N-fertilizer usage, N2O emissions and leaching as the minimum fractional yield is increased. All values are given relative to current (2015) conditions. (Middle) The evolution of yield-scaled N-fertilizer usage, N2O emissions and leaching. (Bottom) The fraction of the total harvested area affected by yield-gap closing.
The environmental cost of yield-gap closing varies considerably between regions (see Tables 1, 2). For example, in South Asia we predict significant additional leaching as yield gaps are closed, resulting in yield-scaled losses of 22.2 kgN/Mg at a minimum fractional yield of 0.8 (compared to a global average of 8.2 kgN/Mg). On the other hand, increasing yields in Eurasia results in relatively little additional N2O emissions or leaching, with yield-scaled losses significantly below the global average even after more than doubling yields.
Optimized Yield-Gap Closing
Figure 4 shows the effect of following the “optimal” yield-gap closing scenario, and how this compares to the “minimum fractional yield” scenario. By focusing additional N-fertilizer on areas with the largest resultant production gains, production can be increased by ~20% (to ~1,150 Tg) without increasing yield-scaled N losses. Increasing production beyond this value results in rapidly accelerating N-fertilizer requirements, N2O emissions and leaching.
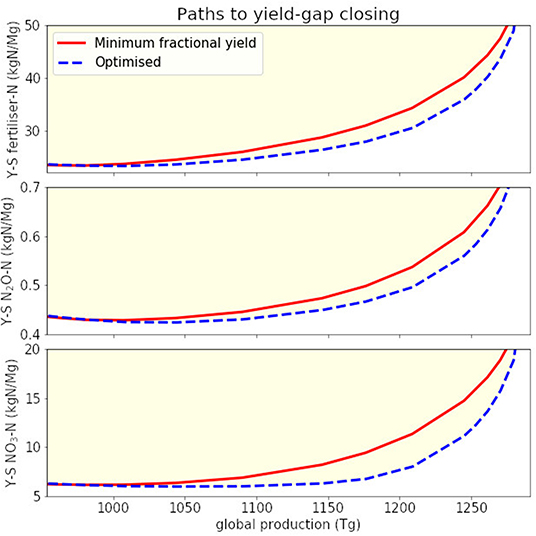
Figure 4. Comparison between the “optimal” (blue, dashed lines) and “minimum fractional yield” (red, solid line) scenarios for closing yield gaps, showing the evolution of yield-scaled N-fertilizer usage and N losses as global maize production is increased. The yellow shaded region shows the space of possible yield-gap closing pathways. (Top) Yield-scaled (Y-S) N-fertilizer usage vs. global production. (Middle) Associated yield-scaled N2O-N emissions. (Bottom) Associated yield-scaled -N leaching.
Discussion
Comparison With Previous Studies
The FAO estimates that global maize production has increased from 592 Tg in 2000 (4.3 Mg/ha) to 1,053 Tg in 2015 (5.5 Mg/ha) (FAO, 2021). Our simulated value of 954 Tg (5.1 Mg/ha) thus matches well to the FAO values. It is worth stressing that LDNDC is not calibrated to reproduce crop yields on a global scale. Instead global crop yields are an emergent feature of a model whose underlying processes are calibrated using field-scale data (see Section Model Calibration). Furthermore, LDNDC has taken part in the Global Gridded Crop Model Intercomparison (GGCMI) project, which compared the performance of 12 global crop models with observed FAO data (Jägermeyr et al., 2021). It was found that LDNDC performs similarly to other state-of-the-art crop models in simulating yield anomalies (i.e., interannual, climate-driven yield variation) for maize at both a global and country level.
There is also good agreement between the potential yield modeled by LDNDC (6.9 Mg/ha on a global scale) and that determined by other studies. Mueller et al. (2012) constructed yield vs. N-fertilizer curves for different climatic zones, and then projected the results across global croplands to find a potential yield for maize of 7.4 Mg/ha under abundant nutrient supply. Similarly, Neumann et al. (2010) used a stochastic method to determine a maximum achievable maize yield of 6.9 Mg/ha. On the other hand, the Global Agro-ecological Zones (GAEZ) project estimates a considerably higher potential yield of 9.6 Mg/ha under optimal management conditions (GAEZ, 2012).
Despite the difference in potential yields between LDNDC and the GAEZ project, we find good agreement in the spatial distribution of fractional yields. Comparing fractional yields at a grid cell level results in a coefficient of determination of r2 = 0.88 (see Supplementary Figure 5). This shows that the two approaches agree as to which regions have the highest agro-climatic potential to increase yields.
Our finding that direct N2O emissions equate to 1.2% of synthetic and organic N-fertilizer inputs can also be compared to previous studies. Del Grosso et al. (2009) found a value of 0.81% for maize using the biogeochemical model DAYCENT, while Gerber et al. (2016) found 0.91% using a non-linear emission factor approach. It should be noted that, unlike in our analysis, these studies corrected for background emissions (i.e., soil N2O emissions under conditions of zero synthetic or organic fertilizer application). More recent results averaged across upland crops have found higher emission rates. For example, Wang et al. (2019) found 1.05% using a non-linear emission factor approach, while Tian et al. (2018b) found 1.8% and Xu et al. (2020) 2.2% using process based models. The LDNDC value lies roughly in the middle of these previous results, suggesting that it provides a reasonable estimate of N2O emissions.
For leaching, the simulated average global loss of 31.3 kgN/ha corresponds to 23% of the synthetic and organic N-fertilizer input. This is very similar to the IPCC tier 1 guideline of 24% (IPCC, 2019). It also matches well with a meta-analysis of global maize cultivation that found 22% (Zhou and Butterbach-Bahl, 2014) and to a previous modeling result of 19% averaged across all crops (Lin et al., 2001).
There is thus close agreement between LDNDC simulations and either data or previous modeling results for actual yields, potential yields, current N2O emissions and current leaching on a global scale. This suggests that LDNDC is able to make robust predictions for yields, N2O emissions and leaching in the case of increased N fertilizer inputs. For N2O emissions and leaching, we are not aware of any previous work that has made such predictions on a global scale.
Yield-Gap Closing at Global, Regional, and Grid-Cell Scales
Yield-Gap Closing at Global Scale
The “optimal” scenario (Figure 4), shows that significant global yield increases are achievable without increasing yield-scaled N-losses. For example, yields could be increased by 20% at a cost of 34% more N-fertilizer use, 23% more N2O emissions and 20% more leaching. However, yield increases beyond ~20–25% would result in fast accelerating losses of N2O and . Comparing our results with the prediction that crop demand will increase by 25–70% in the period 2015–2050 (Hunter et al., 2017), we suggest that the lower end of this range may be achievable without significant additional loading of the global environment with harmful N. However, in the absence of mitigating strategies, yield increases beyond 25% would result in highly non-linear increases in N2O emissions and leaching.
The “optimal” scenario minimizes the additional N losses associated with yield gap closing, but is conceptually complicated due to the requirement to perform a global optimization. As such, it is useful to compare it to the conceptually simpler “minimum fractional yield” scenario, which uses a single variable—the fractional yield—to determine where yields could be increased at low environmental cost (see Figure 4). For a yield increase of 20%, which can be achieved by setting a minimum fractional yield of 0.8, the “optimal” scenario results in considerably less additional N losses. Relative to the year 2015, the “minimum fractional yield” scenario requires 47% more N fertilizer and results in 30% more N2O emissions and 58% more leaching (vs. 34, 23, and 20% for the “optimal” scenario). This makes it clear that, while the fractional yield gives a first idea as to where yields could be increased at the lowest environmental cost, other factors, such as soil type, are also important.
Yield-Gap Closing at Regional Scale
The effect of yield-gap closing on N losses is regionally heterogeneous, as can be seen in Figure 2. Sub-Saharan Africa is the region with the most to gain in terms of production, with the potential to more than double yields (Table 1). However, this would increase N2O emissions by a factor 4 and leaching by a factor 10. While yield-scaled N2O emissions would remain below the global average (Table 2), leaching would exceed the global average, likely resulting in significant pollution of surface and ground water.
Sub-Saharan Africa provides an opportunity to compare our predictions for N2O emissions with previous work. Leitner et al. (2020) performed a meta-analysis of maize cultivation experiments in the region, finding average emissions of 0.27 kg-N/ha under current conditions, and predicting 1.05 kg-N/ha with 50% yield-gap closing and 1.64 kg-N/ha with 75% yield-gap closing. The comparable LDNDC results for direct N2O emissions are 0.45, 1.06, and 1.45 kgN/ha. These results are in reasonable agreement, despite the different methodologies used, i.e., statistical upscaling vs. process based model. However, it is worth noting that the predictions for absolute N2O emissions are very different, due to the different assumptions for the harvested area.
Europe and Eurasia show the most benign effect of yield-gap closing, with yield-scaled N2O emissions and leaching remaining significantly below the global average (Table 2). However, the harvested areas are relatively small (8 and 1.4% of the global total). South Asia is the least favorable region for yield-gap closing, with low potential yields and high susceptibility to leaching. Yields in North America and East Asia are already close to their potential maximum, and there is thus little scope for increasing yield.
Yield-Gap Closing at Grid-Cell Scale
Grid cells in which the N-loss intensity of additional production (i.e., ΔN/ΔY) is less than the global average are shown in Figure 5. These are the grid cells in which yield-gap closing would result in a reduction in global yield-scaled N2O emissions and leaching. Eastern-Europe, especially north and west of the Black Sea, Western Africa, especially Ghana, Togo, Benin and Nigeria, and parts of Central and Eastern Africa stand out as having both low values of ΔN/ΔY and significant harvested areas of maize.
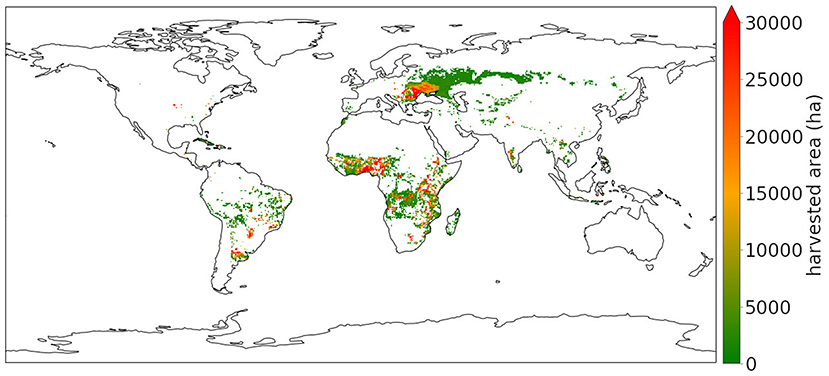
Figure 5. Grid cells in which yield increases come at the lowest cost in additional N losses. Those grid cells for which yield increases would reduce the global average yield-scaled N losses of both N2O and are colored according to their harvested areas (i.e., grid cells for which ΔN/ΔY for N2O emissions is less than the global average value of 0.44 kg-N/Mg and for leaching is <6.2 kg-N/Mg).
On the other hand, the western side of Central America, North India, Indonesia and the Philippines have significant harvested areas of maize and relatively low fractional yields (<0.8), but yield-gap closing would result in high additional leaching. In these regions, ΔN/ΔY exceeds twice the global average for yield-scaled leaching (i.e., ΔN/ΔY > 12.4 kgN/Mg). A similar analysis for N2O emissions shows that yield-gap closing in South-East Brazil would lead to high additional emissions (i.e., ΔN/ΔY >0.88 kgN/Mg).
Possibilities for Further Study
To develop realistic and practical recommendations for how yield gaps could be closed most effectively, our scenarios would also need to take into account economic and political factors, such as the affordability of additional N-inputs or the development of regional food demand. This would clearly make an interesting area for further study, but would require coupling our biogeochemical model to one or more socio-economic models. Other areas that should be addressed in further studies include the effects of climate-change scenarios on yield-gap closing, exploring how far it is possible to balance decreases of N-inputs in N-surfeit regions against increases in N-deficit regions and determining to what extent yield gaps could be closed via non-synthetic N-inputs, such as organic soil amendments or biological nitrogen fixation.
Conclusion
This paper has quantified the additional N-inputs necessary to close yield gaps in maize cultivation, and the consequent N2O emissions and leaching. It is clear that the additional N-inputs necessary for yield-gap closing will always result in increased N2O emissions and leaching. However, by targeting those areas with the highest yield response to additional N-fertilizer application, the additional N losses can be minimized, allowing production to be increased without increasing yield-scaled N2O emissions and leaching. The areas most suitable for additional N fertilizer include Eastern Europe, Western Africa and parts of Central and Eastern Africa. As a result, we predict that significant global increases in maize production of 20–25% are possible without increasing the intensity of N losses.
Data Availability Statement
The raw data supporting the conclusions of this article will be made available by the authors, without undue reservation.
Author Contributions
AS carried out the simulations and analysis. DK made the necessary adjustments to the biogeochemical model Landscape DNDC. All the authors contributed to the study design and to writing the paper and approved the submitted version.
Funding
The authors acknowledge funding by the German Federal Ministry of Education and Research (BMBF) under the Make our Planet Great Again—German Research Initiative, Grant Number 306060, implemented by the German Academic Exchange Service (DAAD).
Conflict of Interest
The authors declare that the research was conducted in the absence of any commercial or financial relationships that could be construed as a potential conflict of interest.
Publisher's Note
All claims expressed in this article are solely those of the authors and do not necessarily represent those of their affiliated organizations, or those of the publisher, the editors and the reviewers. Any product that may be evaluated in this article, or claim that may be made by its manufacturer, is not guaranteed or endorsed by the publisher.
Acknowledgments
We thank Christoph Müller and Jonas Jägermeyr for supplying the phase-3 GGCMI crop calendar, N-manure data and N-fertilizer data.
Supplementary Material
The Supplementary Material for this article can be found online at: https://www.frontiersin.org/articles/10.3389/fsufs.2022.736394/full#supplementary-material
References
Alexandratos, N., and Bruinsma, J. (2012). World Agriculture Towards 2030/2050: the 2012 Revision. ESA Working paper No. 12–03. Rome: FAO.
Carpenter, S. R., Caraco, N. F., Correll, D. L., Howarth, R. W., Sharpley, A. N., and Smith, V. H. (1998). Nonpoint pollution of surface waters with phosphorus and nitrogen. Ecol. Appl. 8, 559–568. doi: 10.1890/1051-0761(1998)008[0559:NPOSWW]2.0.CO;2
Cassman, K. G.. (1999). Ecological intensification of cereal production systems: yield potential, soil quality, and precision agriculture. Proc. Natl. Acad. Sci. 96, 5952–5959. doi: 10.1073/pnas.96.11.5952
Cassman, K. G., Dobermann, A., Walters, D. T., and Yang, H. (2003). Meeting cereal demand while protecting natural resources and improving environmental quality. Ann. Rev. Environ. Resour. 28, 315–358. doi: 10.1146/annurev.energy.28.040202.122858
Cucchi, M., Weedon, G. P., Amici, A, Bellouin, N., Lange, S., Müller Schmied, H., et al. (2020). WFDE5: bias-adjusted ERA5 reanalysis data for impact studies. Earth Syst. Sci. Data 12, 2097–2120. doi: 10.5194/essd-12-2097-2020
Del Grosso, S. J., Ojima, D. S., Parton, W. J., Stehfest, E., Heistemann, M., DeAngelo, B., et al. (2009). Global scale DAYCENT model analysis of greenhouse gas emissions and mitigation strategies for cropped soils. Global Planet. Change 67, 44–50. doi: 10.1016/j.gloplacha.2008.12.006
Dirmeyer, P. A., Gao, X., Zhao, M., Guo, Z., Oki, T., and Hanasaki, N. (2006). GSWP-2: multimodel analysis and implications for our perception of the land surface. Bull. Am. Meteorol. Soc. 87, 1381–1398. doi: 10.1175/BAMS-87-10-1381
Dirnböck, T., Kobler, J., Kraus, D., Grote, R., and Kiese, R. (2016). Impacts of management and climate change on nitrate leaching in a forested karst area. J. Environ. Manag. 165, 243–252. doi: 10.1016/j.jenvman.2015.09.039
Foley, J. A., Ramankutty, N., Brauman, K. A., Cassidy, E. S., Gerber, J. S., Johnston, M., et al. (2011). Solutions for a cultivated planet. Nature 478, 337–342. doi: 10.1038/nature10452
Gerber, J. S., Carlson, K. M., Makowski, D., Mueller, N. D., de Cortazar-Atauri, I. G., et al. (2016). Spatially explicit estimates of N2O emissions from croplands suggest climate mitigation opportunities from improved fertilizer management. Global Change Biol. 22, 3383–3394. doi: 10.1111/gcb.13341
Godfray, C., Beddington, J. R., Crute, I. R., Haddad, L., Lawrence, D., Muir, J. F., et al. (2010). Food security: the challenge of feeding 9 billion people. Science 327, 812–818. doi: 10.1126/science.1185383
Grote, R., Anne-Violette, L., Rambal, S., Staudt, M., Zimmer, I., and Schnitzler, J. P. (2009). Modelling the drought impact on monoterpene fluxes from an evergreen mediterranean forest canopy. Oecologia 160, 213–223. doi: 10.1007/s00442-009-1298-9
Haas, E., Klatt, S., Fröhlich, A., Kraft, P., Werner, C., Kiese, R., et al. (2013). LandscapeDNDC: A process model for simulation of biosphere-atmosphere-hydrosphere exchange processes at site and regional scale. Landscape Ecol. 28, 615–636. doi: 10.1007/s10980-012-9772-x
Houska, T., Kraus, D., and Kiese, R, Breuer, L. (2017). Constraining a complex biogeochemical model for CO2 and N2O emission simulations from various land uses by model-data fusion. Biogeosciences 14, 3487–3508. doi: 10.5194/bg-14-3487-2017
Hunter, M. C., Smith, R. G., Schipanski, M. E., Atwood, L. W., and Mortensen, D. A. (2017). Agriculture in 2050: recalibrating targets for sustainable intensification. BioScience 67, 386–391. doi: 10.1093/biosci/bix010
HWSD (2012). FAO/IIASA/ISRIC/ISSCAS/JRC, 2012. Harmonized World Soil Database (Version 1.2). Rome: FAO; Laxenburg: IIASA.
IPCC (2019). 2019 Refinement to the 2006 IPCC Guidelines for National Greenhouse Gas Inventories, eds E. Calvo Buendia, K. Tanabe, A. Kranjc, J. Baasansuren, M. Fukuda, S. Ngarize, A. Osako, Y. Pyrozhenko, P. Shermanau, and S. Federici (Switzerland: IPCC).
Jägermeyr, J., Müller, C., Ruane, A. C., Elliott, J., Balkovic, J., Castillo, O., et al. (2021). Climate impacts on global agriculture emerge earlier in new generation of climate and crop models. Nature Food. 2, 873–885. doi: 10.5194/egusphere-egu22-3011
Kasper, M., Foldal, C., Kitzler, B, Haas, E., Strauss, P., Eder, A., et al. (2019). N2O emissions and NO3 leaching from two contrasting regions in Austria and influence of soil, crops and climate: a modelling approach. Nutr. Cycl. Agroecosyst. 113, 95–111. doi: 10.1007/s10705-018-9965-z
Kiese, R., Heinzeller, C., Werner, C., Wochele, S., Grote, R., and Butterbach-Bahl, K. (2011). Quantification of nitrate leaching from german forest ecosystems by use of a process oriented biogeochemical model. Environ. Pollut. 159, 3204–3214. doi: 10.1016/j.envpol.2011.05.004
Kraus, D., Weller, S., Klatt, S., Haas, E., Wassmann, R., Kiese, R., et al. (2015). A new landscapeDNDC biogeochemical module to predict CH4 and N2O emissions from lowland rice and upland cropping systems. Plant Soil 386, 125–149. doi: 10.1007/s11104-014-2255-x
Kraus, D., Weller, S., Klatt, S., Santabárbara, I., Haas, E., Wassmann, R., et al. (2016). How well can we assess impacts of agricultural land management changes on the total greenhouse gas balance (CO2, CH4 and N2O) of tropical rice-cropping systems with a biogeochemical model? Agric. Ecosyst. Environ. 224, 104–115. doi: 10.1016/j.agee.2016.03.037
Lange, S.. (2019). WFDE5 over Land Merged with ERA5 over the Ocean (W5E5). V. 1.0. GFZ Data Services. doi: 10.5880/pik.2019.023
Leitner, S., Pelster, D. E., Werner, C., Merbold, L., Baggs, E. M., Mapanda, F., et al. (2020). Closing maize yield gaps in sub-saharan africa will boost soil N2O emissions. Curr. Opin. Environ. Sustainab. 47, 95–105. doi: 10.1016/j.cosust.2020.08.018
Lin, B.-L., Sakoda, A., Shibasaki, R., and Suzuki, M. (2001). A modelling approach to global nitrate leaching caused by anthropogenic fertilisation. Water Res. 35, 1961–1968. doi: 10.1016/S0043-1354(00)00484-X
Liu, W., Yang, H., Folberth, C., Müller, C., Ciais, P., Abbaspour, K. C., et al. (2018). Achieving high crop yields with low nitrogen emissions in global agricultural input intensification. Environ. Sci. Technol. 52, 13782–13791. doi: 10.1021/acs.est.8b03610
Molina-Herrera, S., Haas, E., Grote, R., Kiese, R., Klatt, S., Kraus, D., et al. (2017). Importance of soil NO emissions for the total atmospheric NOx budget of saxony, Germany. Atmosp. Environ. 152, 61–76. doi: 10.1016/j.atmosenv.2016.12.022
Molina-Herrera, S., Haas, E., Klatt, S., Kraus, D., Augustin, J., Magliulo, V., et al. (2016). A modeling study on mitigation of N2O emissions and NO3 leaching at different agricultural sites across europe using landscapeDNDC. Sci. Total Environ. 553, 128–140. doi: 10.1016/j.scitotenv.2015.12.099
Mueller, N. D., Gerber, J. S., Johnston, M., Ray, D. K., Ramankutty, N., and Foley, J. A. (2012). Closing yield gaps through nutrient and water management. Nature 490, 254–257. doi: 10.1038/nature11420
Mueller, N. D., West, P. C., Gerber, J. S., MacDonald, G. K., Polasky, S., and Foley, J. A. (2014). A tradeoff frontier for global nitrogen use and cereal production. Environ. Res. Lett. 9, 054002. doi: 10.1088/1748-9326/9/5/054002
Neumann, K., Verburg, P. H., Stehfest, E., and Müller, C. (2010). The yield gap of global grain production: a spatial analysis. Agric. Syst. 103, 316–326. doi: 10.1016/j.agsy.2010.02.004
Newman, M., and Barkema, G. (1999). Monte Carlo Methods in Statistical Physics. Oxford: Oxford University Press.
Nishina, K., Ito, A., Hanasaki, N., and Hayashi, S. (2017). Reconstruction of spatially detailed global map of NH$_4+$ and NO$_3−$ application in synthetic nitrogen fertilizer. Earth Syst. Sci. Data 9, 149–162. doi: 10.5194/essd-9-149-2017
Pachauri, R. K., Allen, M. R., Barros, V. R., Broome, J., Cramer, W., Christ, R., et al. (2014). Climate Change 2014: Synthesis Report. Contribution of Working Groups I, II and III to the Fifth Assessment Report of the Intergovernmental Panel on Climate Change. Geneva: IPCC.
Portmann, F. T., Siebert, S., and Döll, P. (2010). MIRCA2000-global monthly irrigated and rainfed crop areas around the year 2000: a new high-resolution data set for agricultural and hydrological modeling. Global Biogeochem. Cycles 24, 435. doi: 10.1029/2008GB003435
Rahimi, J., Ago, E. E., Ayantunde, A., Berger, S., Bogaert, J., Butterbach-Bahl, K., et al. (2021). Modelling gas exchange and biomass production in west african sahelian and sudanian ecological zones. Geosci. Model Dev. Discuss. 2021, 1–39. doi: 10.5194/gmd-2020-417
Ravishankara, A. R., Daniel, J. S., and Portmann, R. W. (2009). Nitrous oxide (N2O): the dominant ozone-depleting substance emitted in the 21st century. Science 326, 123–125. doi: 10.1126/science.1176985
Schroeck, A. M., Gaube, V., Haas, E., and Winiwarter, W. (2019). Estimating nitrogen flows of agricultural soils at a landscape level - a modelling study of the upper enns valley, a long-term socio-ecological research region in Austria. Sci. Total Environ. 665, 275–289. doi: 10.1016/j.scitotenv.2019.02.071
Suh, S., Johnson, J. A., Tambjerg, L., Sim, S., Broeckx-Smith, S., Reyes, W., et al. (2020). Closing yield gap is crucial to avoid potential surge in global carbon emissions. Global Environ. Change 63, 102100. doi: 10.1016/j.gloenvcha.2020.102100
Tian, H., Yang, J., Lu, C., Xu, R., Canadell, J. G., Jackson, R. B., et al. (2018a). The global N2O model intercomparison project. Bull. Am. Meteorol. Soc. 99, 1231–1251. doi: 10.1175/BAMS-D-17-0212.1
Tian, H., Yang, J., Xu, R., Lu, C., Canadell, J., Davidson, E., et al. (2018b). Global soil nitrous oxide emissions since the pre-industrial era estimated by an ensemble of terrestrial biosphere models: magnitude, attribution and uncertainty. Global Change Biol. 25, 514. doi: 10.1111/gcb.14514
Tilman, D., Balzer, C., Hill, J., and Befort, B. L. (2011). Global food demand and the sustainable intensification of agriculture. Proc. Natl. Acad. Sci. 108, 20260–20264. doi: 10.1073/pnas.1116437108
van Ittersum, M. K., Cassman, K. G., Grassini, P., Wolf, J., Tittonell, P., and Hochman, Z. (2013). Yield gap analysis with local to global relevance-a review. Field Crops Res. 143, 4–17. doi: 10.1016/j.fcr.2012.09.009
Wang, Q., Zhou, F., Shang, Z., Ciais, P., Winiwarter, W., Jackson, R. B., et al. (2019). Data-driven estimates of global nitrous oxide emissions from croplands. Natl. Sci. Rev. 7, 441–452. doi: 10.1093/nsr/nwz087
WHO (2017). Guidelines for Drinking-Water Quality: Fourth Edition Incorporating First Addendum. Geneva: World Health Organization.
Xu, R., Tian, H., Pan, S., Prior, S. A., Feng, Y., and Dangal, S. R. S. (2020). Global N2O emissions from cropland driven by nitrogen addition and environmental factors: comparison and uncertainty analysis. Global Biogeochem. Cycles 34, e2020GB006698. doi: 10.1029/2020GB006698
Keywords: yield-gap, N2O emissions, NO3 leaching, maize, global
Citation: Smerald A, Fuchs K, Kraus D, Butterbach-Bahl K and Scheer C (2022) Significant Global Yield-Gap Closing Is Possible Without Increasing the Intensity of Environmentally Harmful Nitrogen Losses. Front. Sustain. Food Syst. 6:736394. doi: 10.3389/fsufs.2022.736394
Received: 05 July 2021; Accepted: 20 March 2022;
Published: 14 April 2022.
Edited by:
Amos P. K. Tai, The Chinese University of Hong Kong, ChinaReviewed by:
Deepranjan Sarkar, Banaras Hindu University, IndiaNikolaos Vasileios Paranychianakis, Technical University of Crete, Greece
Jia Mao, The Chinese University of Hong Kong, China
Copyright © 2022 Smerald, Fuchs, Kraus, Butterbach-Bahl and Scheer. This is an open-access article distributed under the terms of the Creative Commons Attribution License (CC BY). The use, distribution or reproduction in other forums is permitted, provided the original author(s) and the copyright owner(s) are credited and that the original publication in this journal is cited, in accordance with accepted academic practice. No use, distribution or reproduction is permitted which does not comply with these terms.
*Correspondence: Andrew Smerald, andrew.smerald@kit.edu