- 1GeoSyntheSES Lab, Department of Geography, Programs in Rural Sociology and Ecology, Pennsylvania State University, State College, PA, United States
- 2Department of Nutritional Sciences, School of Public Health, University of Michigan, Ann Arbor, MI, United States
- 3Andean Food Systems and Lead, Andean Initiative, International Potato Center (CIP), Lima, Peru
- 4Diet, Nutrition, and Agrobiodiversity Research Area, Instituto de Investigación Nutricional, Lima, Peru
- 5GeoSyntheSES Lab, Pennsylvania State University, State College, PA, United States
- 6Center for International Earth Science Information Network (CIESIN), The Earth Institute, Columbia University, Palisades, NY, United States
- 7Faculty of Agricultural Sciences, Hermilio Valdizán National University, Huánuco, Peru
- 8Andean Initiative, International Potato Center (CIP), Lima, Peru
- 9Agrobiodiversity Research Area, International Center for Tropical Agriculture (CIAT)—Asia Office, Hanoi, Vietnam
Agrobiodiversity—the biodiversity of food, agriculture, and land use—is essential to U.N. Sustainable Development Goal 2 by providing crucial food and nutritional quality of diets combined with strengthening agroecological sustainability. Focusing on the agrobiodiversity nexus to SDG 2, the current study utilized the interdisciplinary Agrobiodiversity Knowledge Framework (AKF), household-level surveys, and biodiversity sampling of crop fields and home gardens in a case study in Huánuco, Peru, in 2017. Statistical measures estimated agrobiodiversity of crop fields (n = 268 households) and home gardens (n=159 households) based on species richness (3.7 and 10.2 species/household, in fields and gardens, respectively) and evenness (Shannon diversity index; 0.70 and 1.83 in fields and gardens, respectively). Robust results of Poisson and OLS regression models identified several AKF-guided determinants of agrobiodiversity. Estimated species richness and evenness were significantly associated with 12 social-ecological and political-ecological factors from the four AKF thematic axes: farm characteristics and agroecology; diets and nutrition; markets, governance and sociocultural practices; and global change. This study's AKF approach, agrobiodiversity modeling, agroecological characterization, and field-based case study advanced a series of useful research insights, comparisons, and conceptual innovations to address SDG 2. Characterization of nutrient management through soil- and plant-focused cultural practices and livelihood roles distinguished the “keystone agrobiodiversity-and-food space” of multi-species maize fields (maizales) identified in AKF regression and characterization results. This key space furnished crucial food-nutrition and agroecological benefits that can be expanded by overcoming identified barriers. AKF-guided models incorporating key agrobiodiversity-and-food spaces and ecological nutrient management are needed to strengthen SDG 2 strategies.
Introduction
Actively addressing global hunger and malnutrition as urged in U.N. Sustainable Development Goal 2 (“Zero Hunger;” hence SDG 2) requires vital, diverse dietary and nutritional inputs derived from the biodiversity of food, agriculture, and land use (agrobiodiversity). This human-managed biodiversity functions in a nexus role between food-nutrition needs and agroecology. Agrobiodiversity bridges both the access to food and nutrition (Foote et al., 2004; Frison et al., 2011; Fanzo et al., 2013; Jones et al., 2018; Lachat et al., 2018; Williams et al., 2018; CIP, 2019; Zimmerer et al., 2020) and the complex of agroecological, ecosystem-service, adaptive, and social- and political-ecological dynamics (Jackson et al., 2007; Jarvis et al., 2007; Pascual et al., 2011; Zimmerer et al., 2019; Gerits et al., 2021; Labeyrie et al., 2021). Focus on the links of agrobiodiversity to SDG 2 is central to socially just, nutritious food systems (Zimmerer and de Haan, 2020) and sustainable development (Gepts et al., 2012; Kremen et al., 2012; Vandermeer et al., 2018; Martin et al., 2019; Willett et al., 2019; Zimmerer et al., 2021). This study uses this focus to prioritize empowering poor and marginalized indigenous and smallholder populations.
The interdisciplinary Agrobiodiversity Knowledge Framework (AKF; Smale et al., 2006; precursors in Smale, 2006; Zimmerer and de Haan, 2019; Zimmerer et al., 2019, 2020) guides this study's focus on the agrobiodiversity nexus with SDG 2 and agroecological nutrient management. As shown (Figure 1A), the AKF integrates dynamics of: (1) farms and agroecology; (2) food, nutrition, and diets (food practice, food/nutrition security, health, and SDG2); (3) social/socioeconomic and cultural factors (markets, governance, and cultural practices including livelihood facets and biocultural sub-models); and (4) global changes (climate change, national-international markets, programs, and policies). The AKF model guiding this study thus expands predominant farm-environment approaches.
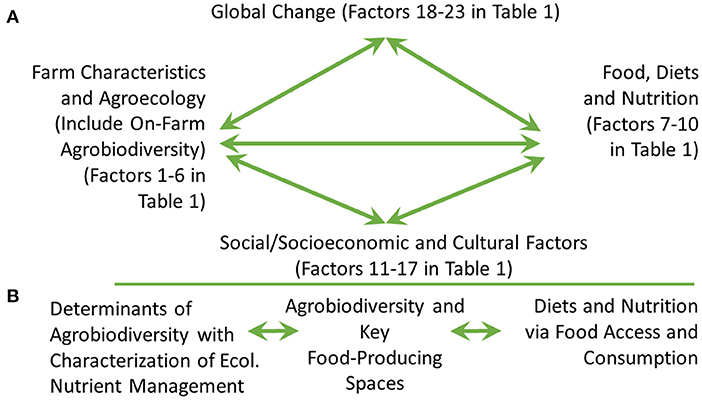
Figure 1. (A) Conceptual model of themes of the Agrobiodiversity Knowledge Framework (AGK) with reference to enumerated factors in Table 1. These AKF themes are linked to the specific factors in Tables 2–4, with the illustrated AKF themes in this figure and the referenced factors serving as the connecting thread through the tables, text, and analysis. (B) Overview of agrobiodiversity-SDG research of this study (left-center) and related project (center-right).
The four AKF themes (Figure 1A) reflect expanding research on agrobiodiversity in relation to dietary, nutrition, and health to address hunger and disease (Foote et al., 2004; Frison et al., 2011; Fanzo et al., 2013; Jones et al., 2018; Lachat et al., 2018; Williams et al., 2018; Downs et al., 2020). The AKF expands emphasis on biodiversity conservation of crop and genetic resources (Brush, 2000; Gepts et al., 2012; Bellon et al., 2015) in conjunction with growing recognition of food and nutrition benefits as well as biocultural dynamics and sustainable development that include human rights and livelihoods (Caillon et al., 2017; Zimmerer and de Haan, 2017, 2019). Additionally, the AKF incorporates agroecological characterization and functions in global-change contexts (e.g., Wood et al., 2015; Zimmerer et al., 2019) including social-ecological and political-ecological transitions (Jackson et al., 2012; Toledo and Barrera-Bassols, 2017; Bottazzi and Boillat, 2021; Goldberg et al., 2021; Labeyrie et al., 2021).
This study addresses questions concerning the nexus roles of agrobiodiversity amid dynamically changing conditions: (1) which AKF-identified factors drawn from social-ecological systems and political ecology (Figure 1A and Table 1) are associated with the variation of agrobiodiversity? (noting the latter are crucial to nutrition outcomes; Jones et al., 2018; Zimmerer et al., 2020) (2) what keystone food-generating spaces contribute to agrobiodiversity outcomes and what influences their occurrence? (3) what is the preliminary characterization of ecological nutrient management in these key spaces? and (4) how can both comparability of results and new concepts of agrobiodiversity be advanced for SDG 2?
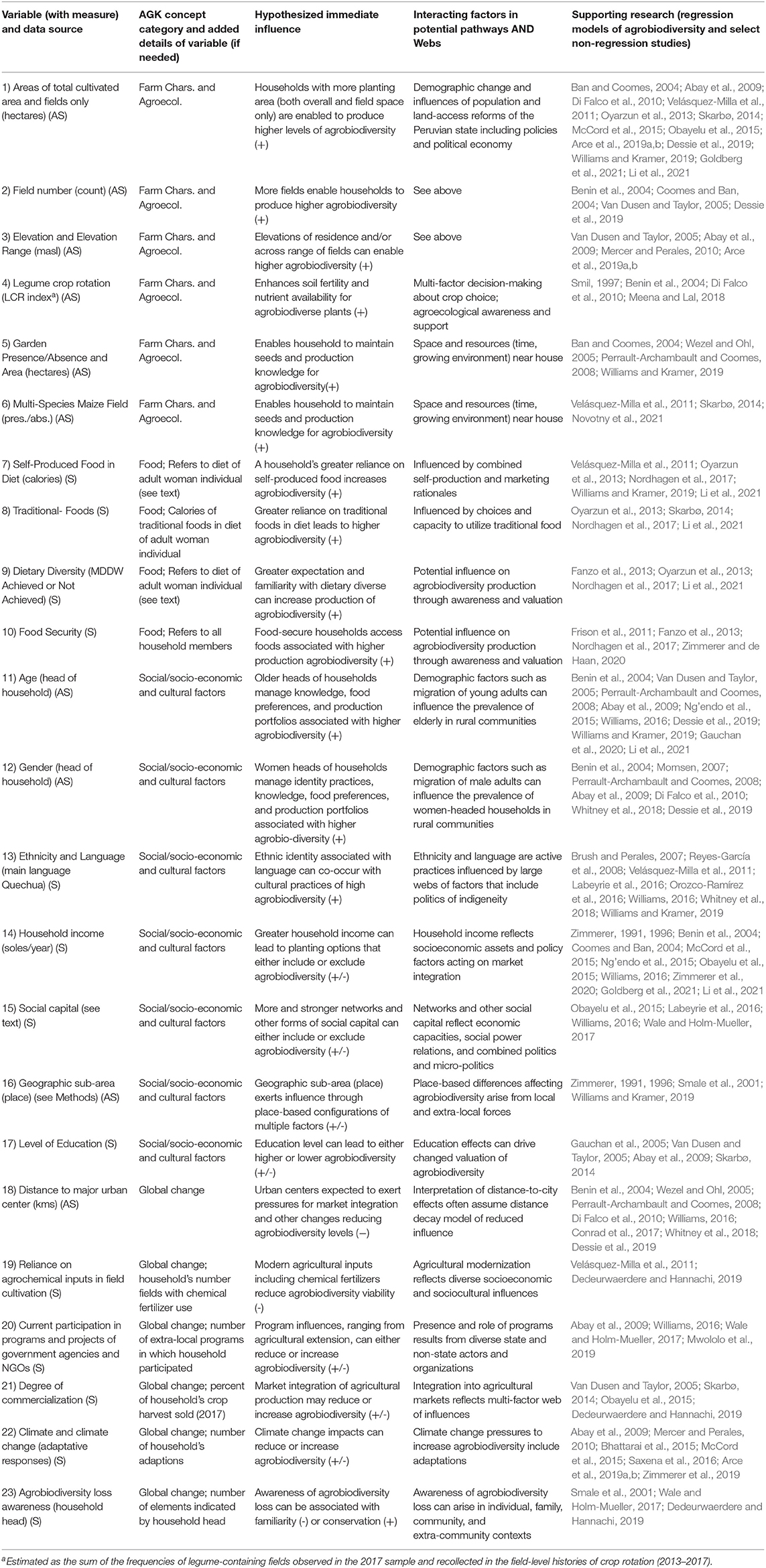
Table 1. Hypothesized social-ecological and political-ecological determinants of agrobiodiversity based on the Agrobiodiversity Knowledge Framework (AKF); data sources for variables refer to survey as “S” and agrobiodiversity sampling as “AS” (descriptions in text).
The overarching goal is to strengthen broad, nimble sustainability-enhancing capacities (sensu Petersen-Rockney et al., 2021) that can generate solutions to SDG-2 by integrating the AKF, socio-ecological modeling, and “keystone agrobiodiversity-and-food spaces” with ecological nutrient management. The study's distinct focus (Figure 1A and thickened arrow on left center of Figure 1B) is integrated with our overarching SDG 2-centered research on dietary diversity and nutrition wellbeing linked to agrobiodiversity (Jones et al., 2018) as well as current food and livelihood struggles (Zimmerer et al., 2020). The relations of this study to overarching research and earlier studies are reflected in the right-center of Figure 1B.
This study uses the AKF and existing research to identify potential social- and political-ecological determinants (Table 1, 1st column) in each of the four AKF themes (Table 1, 2nd column; also Figure 1A). Factors are hypothesized to influence agrobiodiversity via specific processes (3rd column) and examples of conditional interaction webs (4th column). Each factor is rooted in extensive research utilizing agrobiodiversity regression analyses (5th column).
AKF-based design and testing statistical models of social- and political-ecological predictors, as undertaken here, draw on anticipated influences of economics, development, and policy (Van Dusen and Taylor, 2005; Smale, 2006; Smale et al., 2006; Di Falco et al., 2010; Pascual et al., 2011; Rahman and Kazal, 2015; Garduño and Perrings, 2020; Goldberg et al., 2021). AKF-based consideration of model factors draws also from demonstrated influences of culture and society (Williams and Kramer, 2019), social-ecological systems and political ecology (Williams, 2016), and agroecology (see above). The AKF models developed here seeks to engage and advance these approaches.
This study develops an original approach toward culturally managed “keystone agrobiodiversity-and-food spaces” that can range from crop fields and home gardens to food-generating “wild” spaces (Nabhan, 2012, 2018). Currently, these spaces are being transformed amid intensified livelihood integration with extra-local product and labor markets as well as state- and non-state programs and projects (Zimmerer et al., 2020, 2021). Evolving spatial complexity requires identification and analysis of this dimension of agrobiodiversity's role in SDG 2.
Finally, this study engages expanding agroecological focus on ecological nutrient management (Fonte et al., 2012; Vanek et al., 2020) to offer preliminary results on key agrobiodiversity-and-food spaces. It focuses on field fallow and crop rotation as major management strategies (Arce et al., 2019a,b) as well as principal groups of cultivated and managed plants related to soil-nutrient management (Smil, 1997; Schipanski and Drinkwater, 2012; Pérez-Garcia and del Castillo, 2016; Meena and Lal, 2018). It suggests future linking of agrobiodiversity and agroecological research through integrating the AKF, socio-ecological modeling, and key food spaces with ecological nutrient management.
Materials and Methods
Study Area of Huánuco, Peru: Combined “Bright Spot” and “Hot Spot” of Agrobiodiversity
Huánuco, Peru, is marked by complex relations of food, nutrition, and agrobiodiversity (Figure 2; Malice et al., 2010; Velásquez-Milla et al., 2011; Jones et al., 2018; Zimmerer et al., 2020). Climate, topography, soils, and environmental diversity of Huánuco are representative of valley-upland regions of the Andes Mountains in Peru (Pulgar Vidal, 1996, p. 225) and western South America. The Huánuco Andes extend to ecotones of the Upper Amazon. This “bright spot” of agrobiodiversity (sensu Gould et al., 2021) is also a “hotspot” subject to dynamic agri-food changes including widespread food and nutrition insecurity (Zimmerer et al., 2020) where policy legacies and land privatization (Mayer, 2009) contribute to the urgent need to address SDG 2. Social-ecological and political-ecological drivers of agrobiodiversity change in Huánuco are characteristic of the Andes, Amazon, and global trends (de Haan et al., 2010; Oyarzun et al., 2013; Skarbø, 2014; Arce et al., 2019b; de Haan, 2021; Zimmerer et al., 2021).
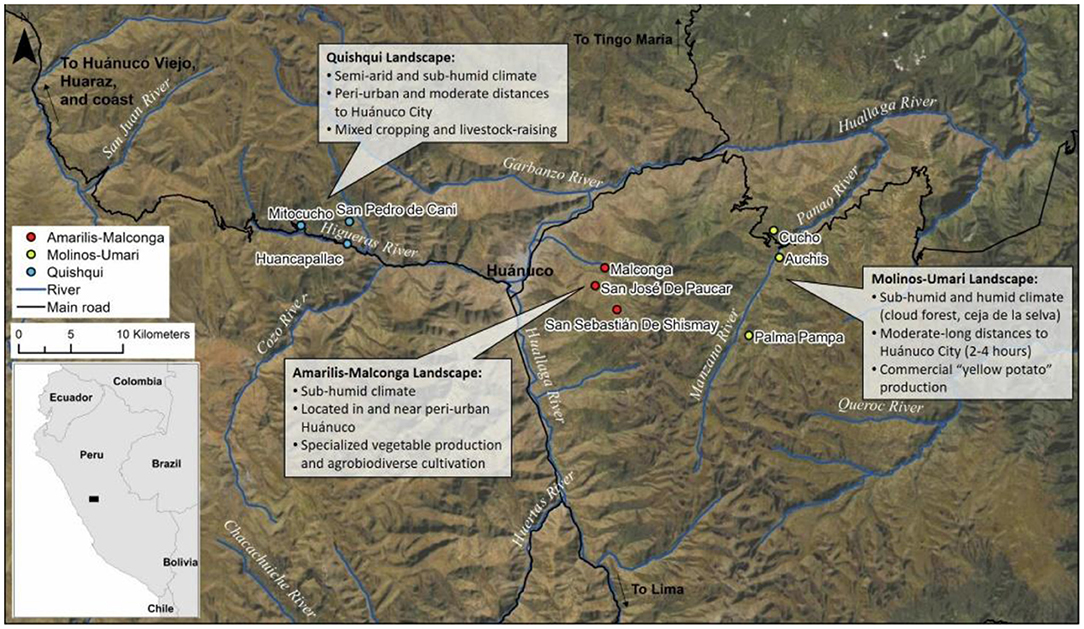
Figure 2. Map of Huánuco study area in Central Peru, with examples of participating study communities in research-focus landscapes (Quishqui, Amarilis-Malconga, Molinos-Umari).
This study was sited in three Huánuco landscapes (Quishqui, Amarilis-Malconga, and Molinos-Umari; Figure 2) that are environmentally and socially distinct. Census and municipal-level data guided the structured-random selection of 10 communities with similar elevation-range characteristics in each landscape. 20 households were then randomly selected to participate out of the 25–40 households in study communities. Eligible households met the following inclusion criteria: (1) members were permanent residents of the household, (2) a woman aged 15–49 years was a household member, and (3) field and/or garden crops had been cultivated by one or more members in 2016–17. For the household survey, we sampled 20 households per community. The selected communities had 25–40 households while extremely small communities were excluded. Institutional human subject approvals (IIN in Lima, Peru, and University of Michigan) guided informed consent and research ethics protocols.
Household Survey
We administered a multi-module household survey to the 600 participating households (April–June 2017) that collected data on potential co-variates with agrobiodiversity. It included modules on sociodemographic characteristics, livelihood assets, food security, dietary intake, and livelihood activities, among other topics.
In addition, a quantitative 24-h recall of food intake of the young or medium-age woman used the multiple-pass method (Gibson, 2005). One hundred women from this sample were randomly selected for a second food intake recall interview after the first interview. From recall data, a 10-food group diet diversity score was used to calculate the Minimum Dietary Diversity for Women (MDD-W) indicator, defined as 1 if the respondent consumed five or more food groups in the previous 24 h and 0 otherwise (Martin-Prevel et al., 2015; FAO, 2016). A 15 g minimum cut-off defined consumption of a given food group. Information on co-variates obtained through above methods is marked with “S” in Table 1 (1st column).
Agrobiodiversity Sampling and Diversity Estimations
One half of surveyed households (n = 300) were randomly selected to participate in sampling agrobiodiversity. Most households cultivated fields (n = 268) and about one half produced gardens (n = 159) as sites for combined market production and home food consumption. The household's principal food-producing spaces were visited with members that participated in sampling (Agrobiodiversity Sampling, AS) using local-name identification and spatial sub-areas. Species-level scientific identification of AS taxa was overseen by local agrobiodiversity experts at the Universidad Nacional Hermilio Valdizan in Huánuco. AS incorporated information on the major food-generating spaces of each household (e.g., rotation history, ownership, and type such as field or garden. These co-variate data are marked “AS” in Table 1 (1st column).
Household-level diversity estimates derived from AS data for fields (n = 268 households) and gardens (n = 159 households) subsequently were used in regression models described below. Our diversity-estimation approach cites specific works since focus on the species level requires general justification (Colwell, 2009: 258; Magurran, 2013; Williams, 2016; Jones, 2017; Zimmerer et al., 2020; Goldberg et al., 2021) and is used specifically to distinguish key food spaces in this study. This species-level focus complements existing agrobiodiversity estimation of cultivars, varieties, and landraces (Smale et al., 2001; Obayelu et al., 2015; Wale and Holm-Mueller, 2017; Dedeurwaerdere and Hannachi, 2019; Gatto et al., 2021), genetic populations (de Haan et al., 2010; Perronne et al., 2017; Arce et al., 2019a,b), and landscapes (Zimmerer et al., 2020), as well as agroecological functional diversity addressed below.
Richness (a count statistic of the number of cultivated and managed food-producing species) and the Shannon diversity index (H = - ln pi) (Colwell, 2009, p. 260)—the latter is an estimate of the relative abundances of species referred to as evenness—were chosen as twin statistical estimates of agrobiodiversity [for statistical formulas, symbols, and rationales see Colwell (2009), Magurran (2013), Smale (2006)]. These diversity measures are widely used individually and in combination for agrobiodiversity research (Benin et al., 2004; Jarvis et al., 2008; Oyarzun et al., 2013; Williams, 2016; Jones et al., 2018; Goslee, 2020; Goldberg et al., 2021) as well as “wild” biodiversity (Colwell, 2009; Hayek and Buzas, 2010). Biodiversity estimates of richness and evenness were visualized on maps and graphs (Figure 5 in Results).
We calculated additional agrobiodiversity estimates using information statistics of the Margalef and Menhinick indices (DMg = (S-1)/(ln N) and DMn = S/√N, respectively) and alternative evenness measures (modified Shannon diversity index, H' = eH and two forms of the Simpson diversity index, D = 1 – and D' = ()−1 (Smale, 2006; Colwell, 2009, p. 260; Magurran, 2013). These additional biodiversity estimates (Supplementary Tables 1, 2) were important though less central to this study and less common in existing agrobiodiversity research. Functional diversity, defined as the diversity of species niches or functions (Villéger et al., 2008; Finney and Kaye, 2017; Blesh, 2018), shown elsewhere as complexly related to agroecological multi-functionality (Blesh, 2018), is treated in this study as important general information. Preliminary distinctions of plant functional groups and nutrient management, described methodologically below, suggests a promising area where future research can more fully integrate functional diversity and agroecology as outlined in the Discussion.
Descriptive Statistics and Regression Analysis
Descriptive statistics assessed the hypothesized predictive factors, with calculations of mean, standard deviation and range of each hypothesized determinant and dependent variable (Table 2 in Results). We then applied multiple regression analysis using Stata statistical software package, version 15.1 (2018; StataCorp) to determine associations of AKF-hypothesized determinants with the four dependent variables (crop species richness of crop fields and home gardens, and Shannon diversity index of crop fields and home gardens).
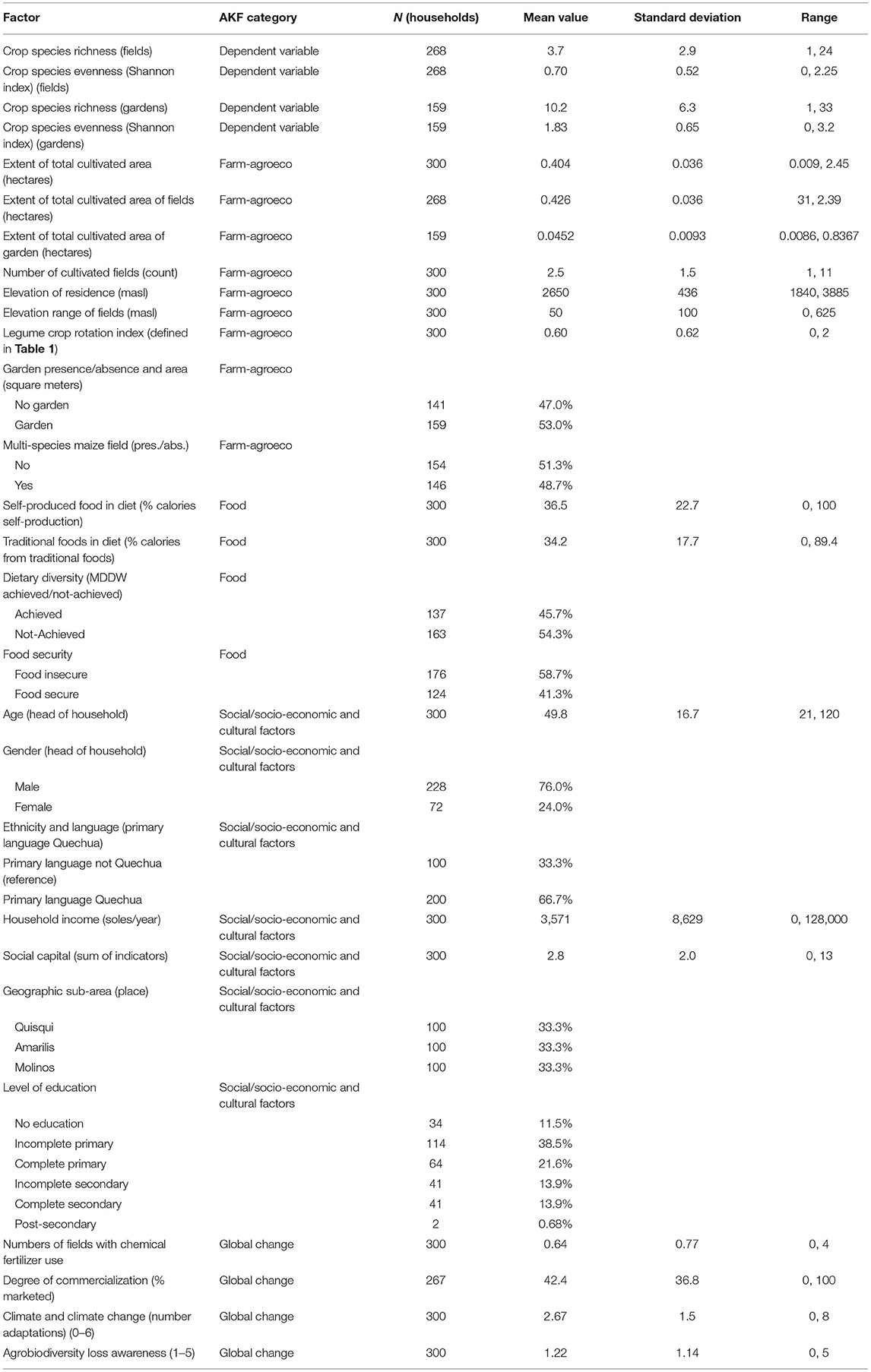
Table 2. Estimations of the social-ecological and political-ecological factors of the Agrobiodiversity Knowledge Framework (AGK) in the sample utilized for the regression analysis of fields.
Poisson regressions were fit to main models regressing covariates on cultivated species richness of crop fields and home gardens (Tables 3, 4 in Results). Values are reported as incidence rate ratios (IRR) where a one-unit increase in the independent variable is associated with a percentage increase in the dependent variable based on the IRR (e.g., an IRR of 1.08 equates to an 8% dependent-variable increase). Table 1 describes each independent variable in these models including its hypothesized process of influence on the dependent variables.
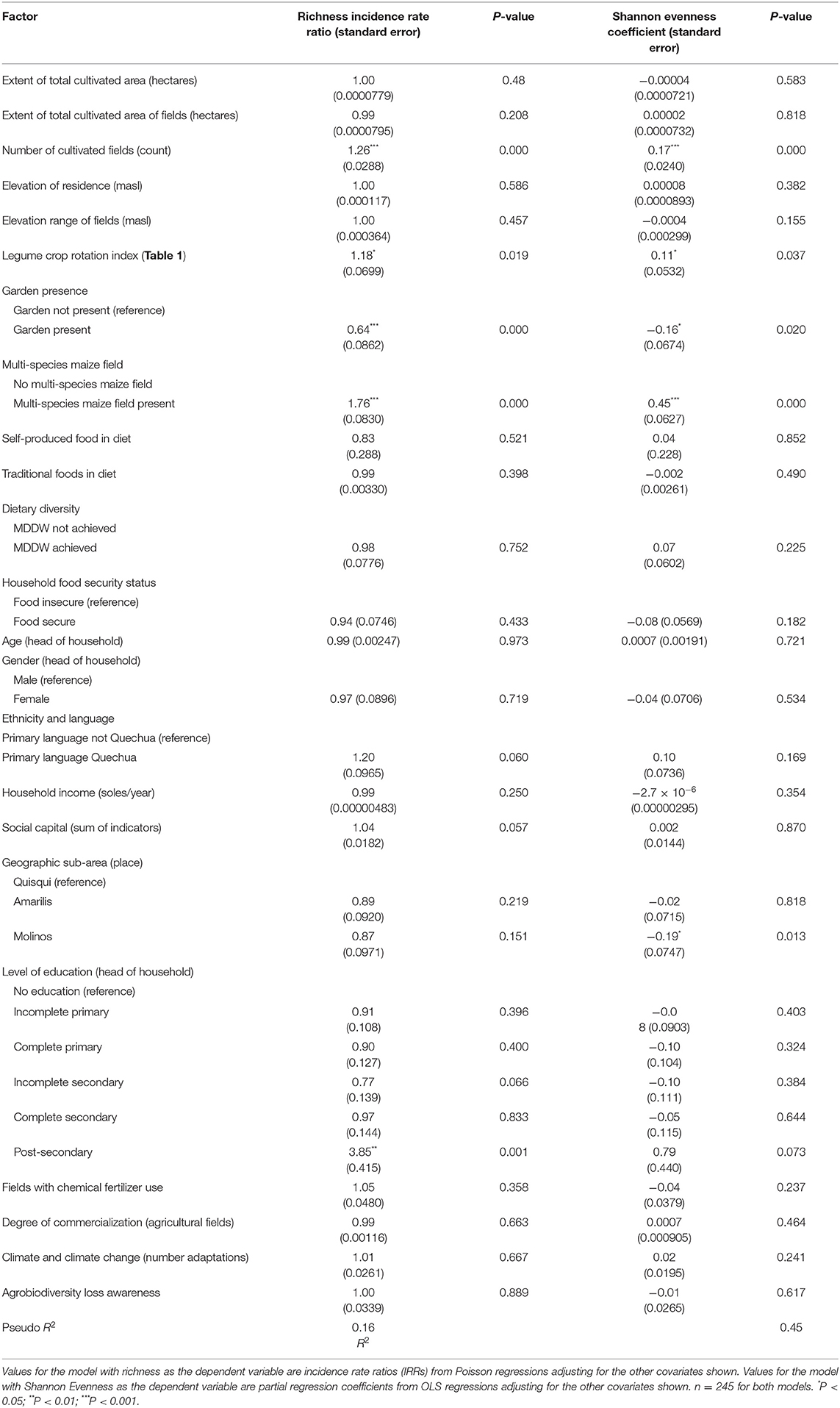
Table 3. Results of multiple regression analyses of the associations of social-ecological and political-ecological factors with crop species agrobiodiversity in fields (Chakras, Parcelas).
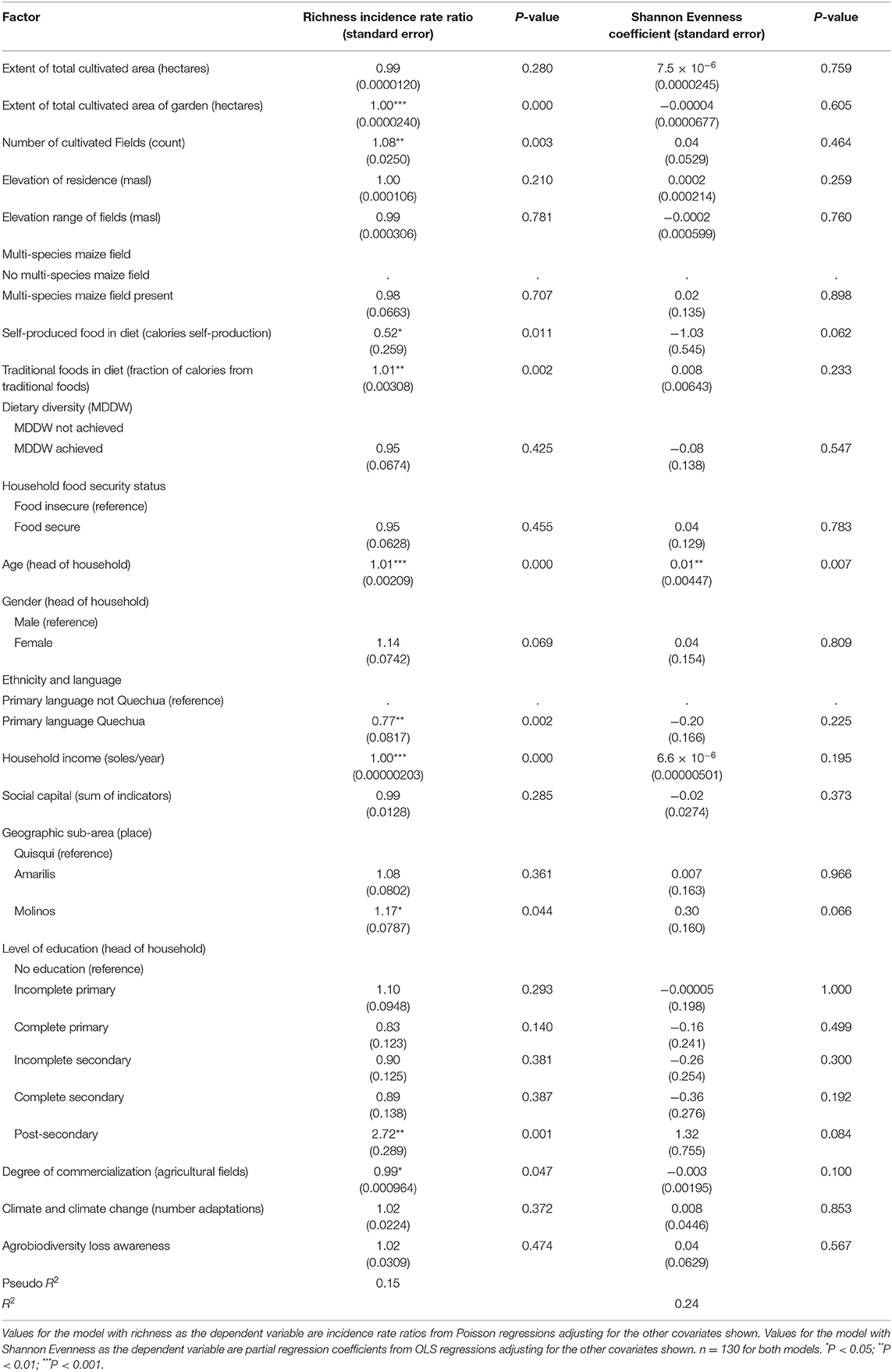
Table 4. Results of multiple regression analyses of the associations of social-ecological and political-ecological factors with managed species agrobiodiversity in gardens (Huertos, Huertas).
Ordinary least-squares regressions were fit to main models regressing covariates on the Shannon diversity index (Tables 3, 4 in Results) in addition to supplementary models using the additional biodiversity indices (Supplementary Tables 1, 2). “Distance” and “current participation in programs” were assessed as AKF-guided independent variables although subsequently omitted since they were found to be statistically insignificant and, due to data limitations, would reduce the utilizable sample of households in regression models. Independent variables used in the garden models differed slightly from the models of crop-field agrobiodiversity, with extent of total garden cultivated area substitute for extent of total cultivated field. The variables “presence of a garden” and “fields with chemical fertilizer use” were omitted in the garden-agrobiodiversity regressions.
Associations of predictor factors and diversity indices were considered consistent with random variability at P > 0.05 (Fisher, 1950, p. 80), with coefficients, Standard Error (SE) and P-values reported in Tables 3, 4 in Results. In addition, a supplement of 16 regression sub-models for grouped AKF factors (farm characteristics and agroecology; diets and nutrition; governance; global change) were estimated for crop fields (Supplementary Tables 3–6) and home gardens (Supplementary Tables 7–10). Breaking out hypothesized factors into sub-models was used to check for possible over-parameterization in the main models. Only factors determined statistically significant in both the main models and supplementary sub-models are reported and discussed below.
Characterization of Keystone Agrobiodiversity-and-Food Spaces
Ecological nutrient management and livelihood roles were estimated for key landscape spaces of agrobiodiversity and food production. Nutrition-focused analysis (Jones et al., 2018; Zimmerer et al., 2020) has signaled the importance of both crop fields (locally chakras or parcelas) and home gardens (kitchen or dooryard gardens, locally huertos). Initial field research involving visits with Huánuco food-growers undertaken in 2017 indicated the potential importance of multi-species maize fields (locally maizales) as an additional distinct type specified further in regression results and fieldwork (Results, Tables 2–4, Figure 8).
Preliminary characterization of ecological nutrient management utilized the AS data to estimate uncultivated fallow (2011–2017), crop rotation (2013–2017), and multi-species plantings (2017). Additionally, AS data enabled categorizing plants in relation to management of soil nutrients and general soil maintenance: (1) nitrogen-fixing legume crops for food and forage; (2) woody vegetation and perennials with generally more extensive root zones; and (3) maize that functions as an extensively rooted annual species. Identification of plant-group categories reflects agroecological, agronomic, and soils research on ecological nutrient management (Smil, 1997; Schipanski and Drinkwater, 2012; Meena and Lal, 2018) as well as research on these plant groups utilized in soil and nutrient management in the Andes (Fonte et al., 2012; Vanek et al., 2020). The total of six above-mentioned agroecological management techniques and plant groups were determined as preliminary estimates feasible using the AS data that had been collected primarily for taxonomic biodiversity estimates. Potential expansion of future social-ecological and political-ecology research on agrobiodiversity to include agroecological methods is described in the Discussion.
Additionally, food and income, which serve major livelihood roles (Arce et al., 2019b; Zimmerer et al., 2020), were characterized based on proportional inputs relative to overall self-produced food and overall farm income, respectively. A group of five key informants knowledgeable about local food, agriculture, and livelihoods rated each farm space from “1 = very important” to “5 = notably unimportant.”
Results
Agrobiodiversity and Descriptive Statistics
Agrobiodiversity sampling (AS) and identification resulted in a total of 92 cultivated species in crop fields (Figure 3). Most frequent among households were maize (Zea mays, maíz; 65.02%), Andean common beans (Phaseolus vulgaris, frejol; 34.98%), potatoes (Solanum tuberosum, papa; 29.37%), Andean squashes (Cucurbita maxima, zapallo, and Cucurbita ficifolia, calabaza; 29.04%), and fava beans (Vicia faba, habas; 18.81%). Home gardens showed 146 species of agriculturally managed plants (Figure 4). Most frequent were onion (Allium cepa, cebolla; 46.47%), oregano (Origanum vulgare, orégano; 40.59%), cilantro (Coriandrum sativum, culantro; 38.24%), peach (Prunus persica, durazno; 34.71%), and chincho (Tagetes elliptica; 32.94%). The species-frequency curves of both crop fields and home gardens were inverse exponential relationships (Figures 3, 4).
Richness of field crops based on agrobiodiversity sampling (AS) with 268 households (Table 2) averaged 3.7 cultivated species/household with the range of 1–24 species. Mean richness of agriculturally management plants in home gardens was 10.2 species per household with a range of 1–33 species (Table 2). Results showed the mean of 7.9 species for all households. This estimated total agrobiodiversity richness, as well as field- and garden-level estimates, did not vary significantly among the three study landscapes. Mapping the species-level richness of agrobiodiversity (combined fields and gardens) illustrated the notable occurrence of household-level variation and the lack of geographic patterning or clustering either within or among the study landscapes (Figure 5). Mean values of the Shannon diversity index were estimated as 0.70 and 1.83 for the cultivated and managed species of crop fields and home gardens, respectively.
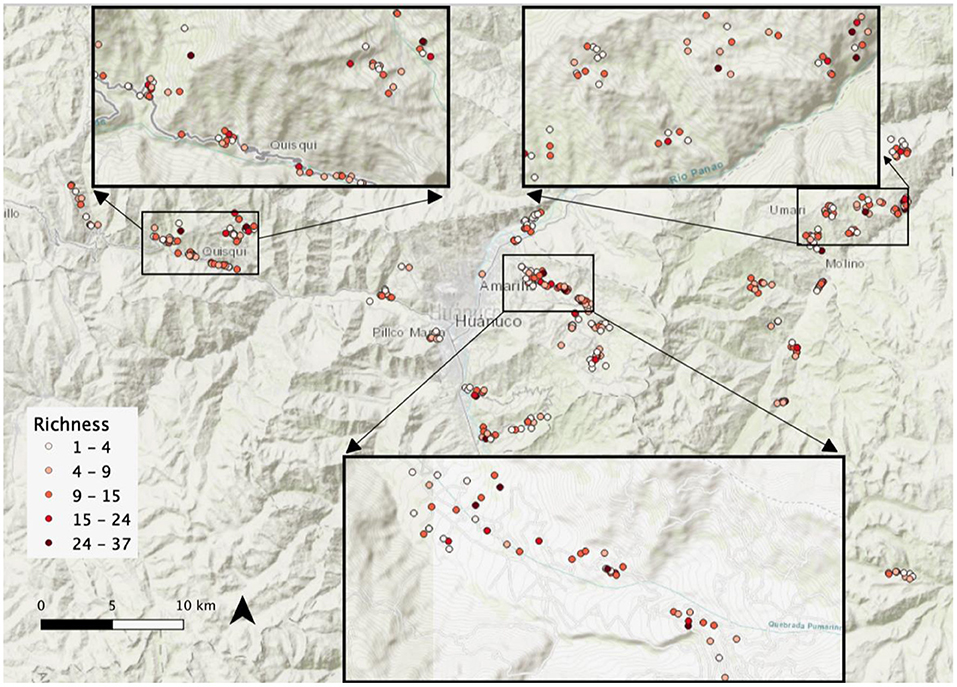
Figure 5. Map of species-level richness of agrobiodiversity (combined richness counts of fields and gardens) among households in Huánuco, Peru.
Results showed the small extents of total cultivated crop-field and home-garden areas (mean values of 0.361 hectares and 0.0925 hectares, respectively) among sampled households. Similarly, low values were estimated regarding food security (i.e., high food insecurity; 58.7%), income, education, and elevation range (Table 2). Estimated household-level capabilities for food production included field numbers (2.5/household), self-produced food in the diet (36.5%), traditional foods in the diet (34.2%), and degree of agricultural commercialization (42.4%) (Table 2). These values reflected limited resource access and hybrid traditional-modern food customs including combined non-market/market linkages.
Certain estimated conditions showed large standard deviations. This high variation occurred in legume crop rotations that were defined as leguminous food and forage crops (mean index value 0.60; standard deviation 0.62) and numbers of fields with chemical fertilizer use (mean value 0.64; standard deviation 0.77). Mean climate change adaptations and elements of agrobiodiversity-loss awareness were low-moderate (2.67 and 1.22, respectively). Sample sizes for results estimated in this paragraph and the preceding varied from 100 to 300 households with most estimates statistically robust. Detailed definitions, data sources, and statistical estimates are given in Tables 1, 2.
Regression Models
Social-ecological and political-ecological factors incorporated in this study's AKF-guided model explained ~16% of variability in the species richness of crop fields (RIRR, Pseudo R2 = 0.16, Table 3). These factors accounted for 45% of the variability in the species evenness of these fields (OLS, R2 = 0.45, Table 3). In the case of home gardens, social-ecological and political-ecological factors of the AKF-guided model explained ~16% of variability in the species richness of these spaces (RIRR, Pseudo R2 = 0.15, Table 4). These factors accounted for 24% of the variability in the species evenness of these fields (OLS, R2 = 0.45, Table 4). Social- and political-ecological factors of the AKF-guided models produced similar results to Tables 3, 4 using other biodiversity measures as the dependent variables (Materials and Methods; Supplementary Tables 1, 2).
Both the species-level richness and evenness of field crops were significantly associated with a set of five factors (Table 3). Field number and presence of a multi-species maize field (maizal) were most significant in the main model of crop fields (P < 0.001; Table 3). These highly significant statistical associations occurred across the range of models using the additional agrobiodiversity measures (Supplementary Tables 1, 2) as well as the AKF sub-model of farm characteristics (Supplementary Table 3).
Field-level richness and Shannon diversity measures showed that legume crop rotation was strongly associated though less significantly (P < 0.05; Table 3). Additional factors, types of association, and significance levels in the main model of crop fields (Table 3) varied among the positive associations of species richness to garden presence (P < 0.001) and post-secondary education (P < 0.01). Field evenness was negatively associated with the presence of a garden (P < 0.05) and the sub-area of the Molinos landscape (P < 0.05).
In home gardens, species-level richness was significantly associated with a set of nine factors and the Shannon evenness measure with one (Table 4, 2nd and 3rd columns). Age of household head was positively associated at highly significant levels with the species richness (P < 0.001) and species evenness of gardens (P < 0.01) (Table 4). Other factors showing high and positive associations with the species richness of home gardens were garden cultivated area (P < 0.001), field number (P < 0.01), traditional food in diet (P < 0.01), Quechua language (P < 0.01), household income (P < 0.001), and extent of post-secondary education (P < 0.01). Additional factors showed significant positive associations albeit at lower statistical levels. These included the sub-area of the Molinos landscape (P < 0.05) and degree of product commercialization (P < 0.05).
Individual social- and political-ecological factors identified as statistically significant represented each the four AKF themes (Figures 6, 7) and offered comparisons to previous agrobiodiversity models (last column of Table 1) as detailed in the Discussion.
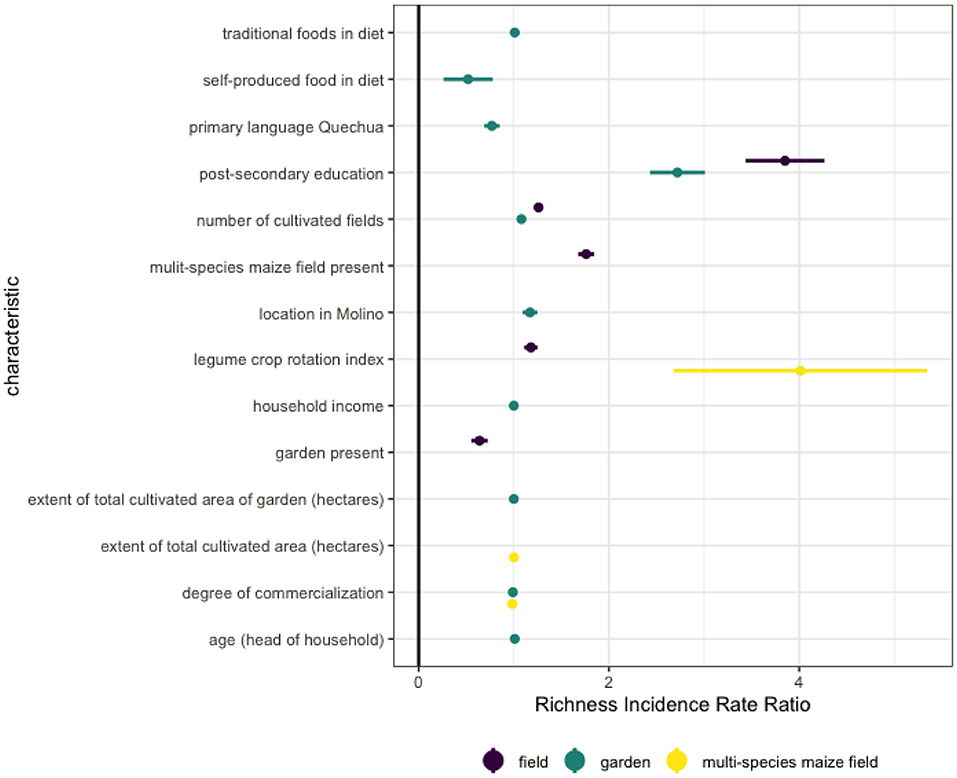
Figure 6. Coefficients and standard errors of significant factors in results of Poisson regression models for richness in agrobiodiversity-and-food spaces (field, garden, multi-species maize fields; each color represents an individual regression model).
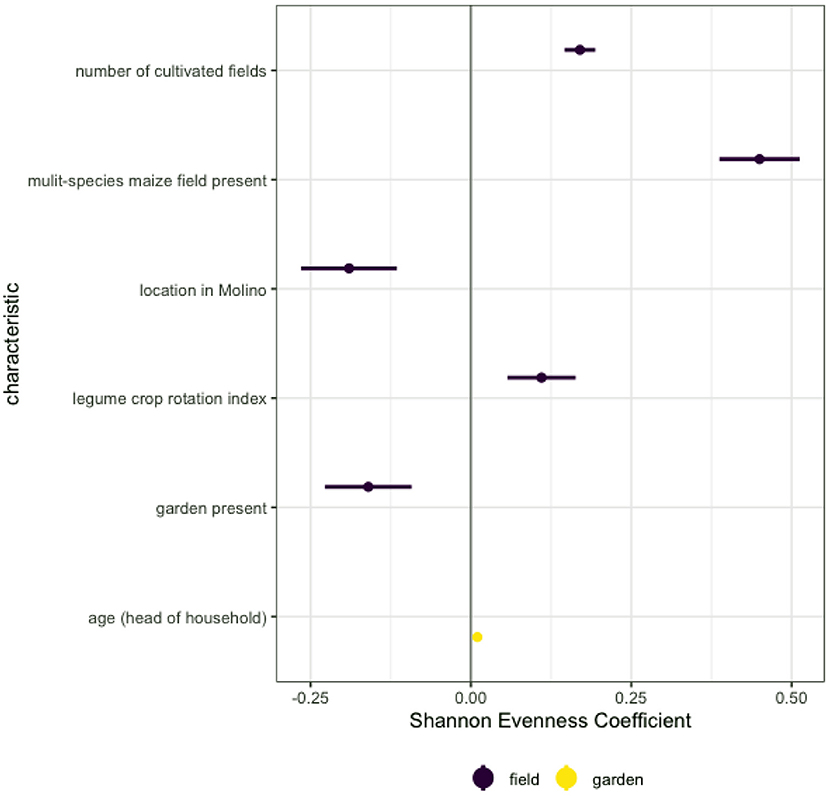
Figure 7. Coefficients and standard errors in regression results on factors in ordinary least squares regression models for Shannon evenness in agrobiodiversity-and-food spaces (field, garden; each color represents an individual regression model).
Agroecological and Livelihood Characterization of Keystone Spaces
Agroecological and livelihood characteristics were compared among keystone agrobiodiversity-and-food spaces comprised of multi-species maize fields, other crop-field types, and home gardens. Identification of multi-species maize fields (maizales) as an additional keystone space was based on above regression results and fieldwork information (Figure 8), including conversations with local people explaining importance. Following above results, further focused analysis of multi-species maize fields as a key space (Supplementary Table 11 and Figure 6) showed the highly positive effect of legume crop rotation (P < 0.001), moderate-high correlation with overall field area (P < 0.01), and negative association with agricultural commercialization (P < 0.01).
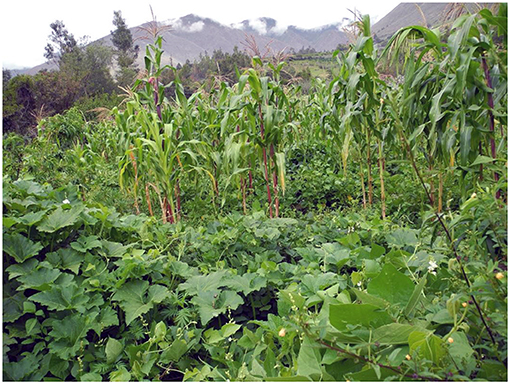
Figure 8. Multi-species maize field (maizal) functioning as “key agrobiodiversity-and-food space” in fieldwork visit (February 2017). Photo credit: Karl Zimmerer.
Fallow and crop rotation were moderately and highly common in multi-species maize fields (35.2 and 86.2%, respectively) and other field types (32.7 and 76.1%, respectively) (Table 5). By contrast, these two practices were applied at low levels in home gardens (1.6 and 4.9%, respectively) (Table 5). Intercropped polycultures (planting areas with more than one food species), crop rotation, and legume crops occurred more commonly multi-species maize fields than in other fields. Multi-species mixtures were also moderately common in other crop fields (48.3%). Plant groups with distinct functional roles and traits—legume food and forage crops; the suite of trees, shrubs, and perennials managed in cultivation; and maize—occurred at different levels in the three key agrobiodiversity-and-food spaces (Table 5).
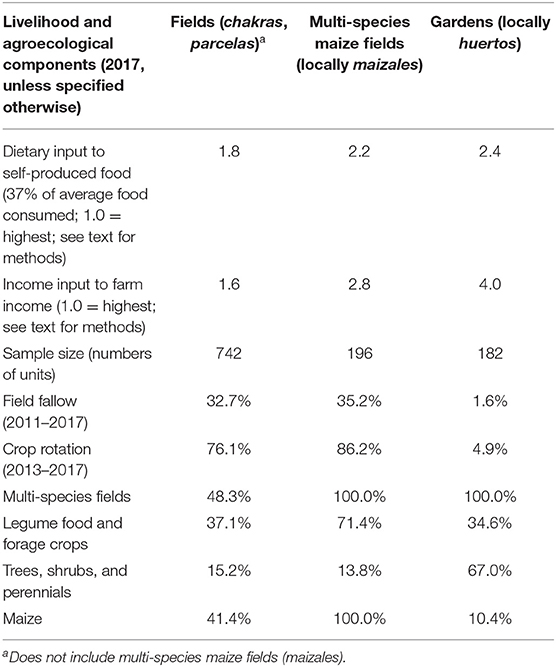
Table 5. Characterization of key farm and food-producing spaces according to livelihood importance and the frequencies of soil and soil-nutrient management (Huánuco, Peru).
Estimation of the frequency of legume crops and edible managed plants was distinctive among multi-species maize fields, crop fields, and home gardens (71.4, 37.1, 34.6%, respectively; Table 5). Leguminous taxa provisioning important foods in the sample consisted of Phaseolus vulgaris (frijol, vainita, or numia), Inga feuilleei (pacay), Caesalpinia spinosa (tara), Pisum sativum (arveja), Vicia faba (haba), Lupinus mutabilis (tarwi), Arachis hypogaea (mani), Inga edulis (guaba), Lens culinaris (lenteja), and (Medicago sativa alfalfa; important as an animal food) as well the widespread ground-covering clover Trifolium spp. (trebol). A total of 51 tree species were managed and cultivated, as well as 20 species of perennial herbs.1
Estimated relative levels of dietary and income importance varied substantially among the key agrobiodiversity-and-food spaces (Table 5). Multi-species maize fields (maizales) typically rated intermediate between crop fields (highest levels) and gardens (lower levels yet still important to diet).
Discussion
The Agrobiodiversity Knowledge Framework (AKF) and Comparative Model Results
This study's use of the interdisciplinary Agrobiodiversity Knowledge Framework (AKF) generates reflection on and comparisons with other SDG 2-relevant research. Overall, the AKF framework was thematically comprehensive, theoretically cohesive, and methodologically innovative in predicting agrobiodiversity that is linked to SDG 2-related nutrition security in our overarching design that integrates the agrobiodiversity analysis here with focus on food, nutrition, and diet research (Figure 1B; Jones et al., 2018; Zimmerer et al., 2020).
Results showed significant associations across each of the four main themes of AKF predictive factors (Figure 1A; agroecology-food/nutrition-governance including sociocultural factors-global change impacts) that provide insights to address SDG 2. First, the AKF-guided models offer a demonstration of agroecological factors (e.g., legume crop rotation; Table 3) positively linked to agrobiodiversity. This linkage combines with results on the importance of leguminous food crops to nutrition wellbeing (Jones et al., 2018). Farm-level, resource-access factors were similarly important. For example, access to a garden both determined agrobiodiversity outcomes as shown in this study while it was also associated with favorable nutrition outcomes (Jones et al., 2018). This study's AKF-guided demonstration of well-proven linkages to nutrition build on initial calls (Jarvis et al., 2007; Hajjar et al., 2008) to provide concrete evidence of agrobiodiversity functions that can address SDG 2.
Second, the AKF shows that food and nutrition can operate as significant predictors (Table 4 and Supplementary Table 8). This AKF result is novel since the predominant view is to treat nutrition, food, and other SDG 2-related conditions solely as outcome variables. Instead, it highlights influential bi-directional interactions in which food and diet can serve as model inputs to explain agrobiodiversity, We urge this insight be built into SDG 2 approaches.
Third, AKF-guided analysis of governance predictors, including socioeconomic factors, were significant in the results (e.g., income; Table 4). Other significant governance factors were sociocultural factors in the changing spatial strategies of food-growing (multi-species maize fields), agrobiodiversity knowledge (Quechua language), and institutional influences (e.g., school-based education; Tables 3, 4). Each of these factors potentially serves as a strong linkage in addressing SDG 2, including other governance factors (such as the potential major influence of seed systems Arce et al., 2019a) and public-good policies (Graddy-Lovelace, 2021).
Fourth, results showed AKF-SDG linkages involving global-change factors in the significant association of the number of fields with agrobiodiversity (Table 3) since the dispersion of fields provides adaptations to weather variation propelled by climate change. At the same time, the resulting higher level of crop diversity has been shown in our related research to predict favorable nutrition outcomes (Jones et al., 2018).
Comparability of model results is needed to advance the use of agrobiodiversity and agroecology to address SDG 2. The AKF supported a much-needed, cohesive approach to interdisciplinary research to enable rigorous cross-case comparisons and policy dimensions ranging from incentives and capacity-building to new initiative such as participatory varietal selection using agrobiodiversity to address SDG 2 (Scurrah et al., 2019). Here we briefly use AKF-guided results to discuss comparisons with other agrobiodiversity studies relevant to SDG 2 approaches and conclude the section by synopsizing this study's model results.
This study's model results show various similarities when compared to related agrobiodiversity studies holding promise for SDG 2 approaches (e.g., Pseudo R2 = 0.1883 of species-level agrobiodiversity in important research linked to food consumption and security in the Northern Ecuadorian Andes; Skarbø, 2014, p. 723). In addition, our results found similar factors to be significance (e.g., field number and education level). Comparisons are partly limited, however, since the other research did not model determinants of evenness or garden-specific agrobiodiversity [see also Velásquez-Milla et al. (2011) on Huánuco agrobiodiversity custodian farmers that identifies similar statistically significant factors but does not report model-level results; Skarbø, 2014]. As a result, this study recommends comparisons to thematically related social- and political-ecological modeling of agrobiodiversity evenness, such as species-level agrobiodiversity in coastal Nicaragua (R2 = 0.34; Williams, 2016, p. 234) whose general similarity suggests SDG 2 promise.
Our model-level results on the social- and political-ecological determination of home garden agrobiodiversity in Huánuco suggest extended geographic comparison to SDG 2-relevant research in the neighboring Amazon region (e.g., Ban and Coomes, 2004, p. 353). Individual factors identified as highly significant in this study (e.g., garden area and gardener experience) were similar (Ban and Coomes, 2004, p. 353), though different statistical techniques and lack of model-level estimation and evenness estimates limit further direct comparison.
Finally, this study's results from regression models account for species richness to a similar degree in both crop-field and home-garden analysis (Pseudo R2 values of 0.16 and 0.15, respectively) while evenness, as measured by the Shannon diversity index, differs substantially among crop fields (R2 = 0.45) and home gardens (R2 = 0.24). Relative species abundance in home gardens is known to vary depending on the complex characteristics of individual households (Ban and Coomes, 2004; Coomes and Ban, 2004; Wezel and Ohl, 2005; Perrault-Archambault and Coomes, 2008; Whitney et al., 2018), which may contribute to the lower results of the evenness model.
Biodiversity Estimations and the Social- and Political-Ecological Factors
This study's estimations of agrobiodiversity levels and specific social- and political-ecological factors offer valuable specific comparisons to SDG 2-related research as well as general research advances. Our result on the species-level richness of crop fields (mean 3.7 species/household) was similar though less than findings in the Ecuadorian Andes (7.22 species/household; Skarbø, 2014, p. 714) whose fields traversed a significantly larger elevation gradient (1,000 meters). This study's results on specifies-level richness of home gardens (mean 10.2 species/household) resembled the richness of cultivated species of fruits, vegetables, and herbs (10.2 species/household) that were grown the Ecuadorian Andes (Skarbø, 2014, p. 714). Furthermore, this study's results on total agrobiodiversity species richness in Huánuco, when summed across the households' crop fields and home gardens, resembled an additional study of the Ecuadorian Andes (Oyarzun et al., 2013, p. 525).
Another useful comparison is to local high-agrobiodiversity custodian farmers in Huánuco (Velásquez-Milla et al., 2011) and high-level agrobiodiversity hotspots elsewhere in the Peruvian Andes (Arce et al., 2019a). The current study complements these others, while our sample design and methods were distinct since we integrated focus on agrobiodiversity's roles in SDG-related diets and nutrition that included the randomized sampling of households (see also Jones et al., 2018; Zimmerer et al., 2020).
Important to highlight as a research advance is this study's demonstration that combined biodiversity richness and evenness estimates are needed to understand agrobiodiversity's nexus role connecting to both food-nutrition, such as SDG 2, and agroecology.
Finally, individual factors determined to be significantly associated with agrobiodiversity in this study (see previous sub-section) conform hypotheses in Table 1 and need to information expanding SDG 2 research globally. Overcoming barriers to link or couple agrobiodiversity access and related SDG 2 benefits for food sovereignty (Zimmerer et al., 2020) will require promoting agroecological management, garden cultivation, and livelihood improvement in addition to income and education as parts of the agendas of social justice and food and nutrition security.
Select factors not showing statistical significance in this study's model results, such as field size and elevation range (Tables 3, 4), help to explain contextual influences. Extremely limited size of cultivated areas in this study (0.404 hectares/household; Table 2) is typical of many places in the Andes (e.g., 0.5-hectare farms are common in the central highlands of Ecuador; Oyarzun et al., 2013, p. 523) and smallholder farming globally (van Vliet et al., 2015). Likewise, this study's result on the limited elevation range among fields (Table 2) did not result in a significant model result though it is consistent with fieldwork observations of the clustering of small-size fields and gardens near residences. Additionally, this study's findings showed the high frequency of global change factors among surveyed households (e.g., climate change adjustments and elements of agrobiodiversity-loss awareness; Table 2), though this study's regression results did not reveal specific statistical associations to these variables.
A Keystone Agrobiodiversity-and-Food Space: Multi-Species Maize Fields
This study's focus on new descriptive, statistical, and conceptual understandings of the role of multi-species maize fields (maizales) as a Keystone agrobiodiversity-and-food space is designed to offer a novel contribution and to build on previously hypothesized functions (see multi-species maize fields, Table 1). Model results demonstrate that multi-species maize fields, which are distinct due to the types and extent of intercropping (see Results, Agroecological and Livelihood Characterization of Key Spaces), hold a high level of significance in agrobiodiversity relationships (Tables 3, 4). Multi-species maize fields comprise a key space of broad significance to the nexus of agrobiodiversity-agroecology-SDG 2.
We propose the concept of “Keystone agrobiodiversity-and-food space” to describe multi-species maize fields (maizales) owing to multi-faceted functions. These fields of Huánuco incorporate widespread inter-plantings of Andean common beans (frejol, Phaseolus vulgaris), Andean squash (most commonly zapallo, Cucurbita maxima), and arracacha (giant Andean carrot or parsnip, Aracacia xanthorrhiza), among other species. Agrobiodiverse maizal assemblages, which are extensive in western South America, are distinct while partly resembling the well-known milpa system of Mexico and Central America that is agrobiodiverse and nutritionally important (Toledo and Barrera-Bassols, 2017; Novotny et al., 2021).
Notable nutrient management techniques characterize the agroecological functions of the multi-species maize field as a “keystone agrobiodiversity-and-food space” with extensive rotation of crops (86.2% of maizales incorporate crop rotation; Table 5). Various agrobiodiverse species depend on seed flows and rotated planting sites that link the maizal system to other fields. For example, Huánuco households undertaking the non-maizal field cropping of Andean maize, Andean common beans and Andean squash species frequently depend on maizal-based seed sources and rotated planting sites, and vice versa. A second major function is continued utilization and development of agrobiodiversity management knowledge. This role is crucial since cultivated area and field number are extremely limited among Huánuco households (Table 2). In this context, the multi-species maize field (maizal) enables the crucial continuation of knowledge and practices of production as well as food processing and consumption that are vital to agrobiodiversity-SDG 2 linkages.
Another key agrobiodiversity-support function of the Andean multi-species maize field is the concentration of livestock grazing on above-ground plant residues following harvest. This emphasis is crucial to the functioning of ecological nutrient management of agrobiodiversity-containing maizales as well as nearby fields. Home gardens (locally huertos), which are distinct from multi-species maize fields, are also “keystone agrobiodiversity-and-food spaces” though they contain lesser degrees of the linkage functions described above.
Concepts and Barriers for “keystone Agrobiodiversity-and-Food Spaces”
This study's concept of “keystone agrobiodiversity-and-food spaces” is supported by advances in ecological theory extending the keystone species idea from an original food-web focus to other connectivity (e.g., Davic, 2003). An analogous development has occurred in the concept of “cultural keystone species” (Coe and Gaoue, 2020) being expanded to agroecological applications (Nabhan, 2018; Zapico et al., 2020). Use of “keystone” here signifies that addition or loss leads to major changes in occurrence or abundance of other species.
The “keystone agrobiodiversity-and-food spaces” concept is designed to rework the single-taxon definition of a keystone species to one centered on the vital and distinct roles of spatially, culturally, and agroecologically distinct suites of interacting species. Spatial dynamics and influence of food-generating units is traditional in farming and land use (Brookfield, 2001; Mayer, 2018). Changing spatial dynamics of agrobiodiversity pose new challenges and opportunities regarding agroecological sustainability (De Molina et al., 2019). Supporting the positive factors enabling multi-species maize fields (Supplementary Table 11 and Figure 6), such as legume crop rotation, will need to overcome access barriers (e.g., currently multi-species maize fields are linked to larger, less resource-poor farms among indigenous Huánuco smallholders; Supplementary Table 11; Zimmerer et al., 2020).
In sum, the multi-species fields or maizales of Huánuco function as a distinct, locally recognized, and valued agrobiodiversity-and-food space with widespread intercropping, associated agrobiodiversity, and nutrient management. The latter's characterization (Table 5) is preliminary in scope and is intended to stimulate research. We anticipate other “keystone agrobiodiversity-and-food spaces” to include the multi-species, high-agrobiodiversity fields of Andean tuber crops in sectoral fallow management (also known as common field agriculture; Arce et al., 2019b; Vanek et al., 2020). Milpa agriculture of Mexico and Central America is another vital space occurring in highly dynamic contexts (Tamariz, 2022) with key linkages and agroecological functions of agrobiodiversity anticipated to be changing rapidly.
Further Links to Future Research
Finally, this study suggests future research avenues centered on AKF-guided approaches to addressing SDG 2 through agrobiodiversity models integrating one or more emphases of agroecology, biodiversity science, and comparisons with existing studies. It indicates the promising role of “keystone agrobiodiversity-and-food spaces” well-suited to expanded integration with these areas of emphasis. Further new research is needed on functional diversity and multi-functionality in agroecology (Blesh, 2018) suited to SDG 2 goals. New AKF-guided modeling approaches related to SDG 2 goals could involve alternative sampling and statistical methods such as Principal Components Analysis, path analysis, constrained ordination, permanova, systems modeling, and reduced-variable parsimonious models.
Conclusion
Modeling of social- and political-ecological factors using the interdisciplinary Agrobiodiversity Knowledge Framework (AKF, Figure 1A, Table 1) was combined with nutrient management characterization of keystone agrobiodiversity-and-food spaces that linked to our project on improving nutrition, diets, and SDG 2 (Zero Hunger). Focused on a continued case study in Huánuco, Peru, the AKF guided the selection, design, analysis, and interpretation of determinants of agrobiodiversity. The latter was estimated using the biodiversity statistics of species-level richness and Shannon diversity index (as well as five additional biodiversity indices) applied both to crop fields and home gardens.
Model results showed significant associations of the farm and agroecological characteristics of field number, garden area, and legume crop rotation. Other factors identified as significant in agrobiodiversity modeling corresponded to the AKF categories of diet and nutrition; social/socioeconomic and cultural factors (governance); and global change. The AKF model was shown to be thematically comprehensive, conceptually cohesive, and timely in focusing on agrobiodiversity-SDG 2 synergies and communicating new research on dynamic, change-prone agrobiodiversity interactions that are increasingly influential (Zimmerer, 2010; Dwivedi et al., 2013; Baumann, 2022; Tamariz, 2022; Tamariz and Baumann, 2022; Zimmerer et al., 2022).
The study's design enabled comparison to other models and estimation that is crucial to advancing agrobiodiversity knowledge, policy, and initiatives to promote SDG 2. Effectively integrating AKF-guided agrobiodiversity modeling and estimation with SDG 2 research relied on incorporating characterization of ecological nutrient management using the concept of “key agrobiodiversity-and-food spaces.” Characterization focused on soil- and plant-based elements of nutrient management, with results demonstrating the extensive utilization of field fallow, crop rotation, multi-species fields, legume crops, and managed plantings of trees, shrubs, and perennials as well as maize.
The concept of “keystone agrobiodiversity-and-food spaces” is proposed and developed to account for the combined prevalence and functions of crop fields, home gardens, and multi-species maize fields (maizales) in the changing agri-food systems of indigenous smallholders in Peru. Results demonstrated the strong agrobiodiversity associations and ecological nutrient management of each key space with focus on multi-species maize fields. As a key agrobiodiversity-and-food space, the multi-species maize fields are beneficially linked to agrobiodiversity, ecological nutrient management, and food and market capacities, thus offering vital contributions to SDG 2.
Data Availability Statement
The original contributions presented in the study are included in the article/Supplementary Material, further inquiries can be directed to the corresponding author.
Ethics Statement
The studies involving human participants were reviewed and approved by Instituto de Investigacion Nutricional, Lima, Peru. Written informed consent for participation was not required for this study in accordance with the national legislation and the institutional requirements.
Author Contributions
KZ: conceptualization and writing—original draft. HC-K, SH, AJ, KZ, and FP: methodology and supervision. HC-K, SH, AJ, KZ, FP, and MT: investigation. KZ, AJ, SH, RT, CH, and KN: data and analysis. KZ, SH, AJ, and HC-K: writing—review and editing and funding acquisition. RT and CH: visualization. All authors contributed to the article and approved the submitted version.
Funding
This study was supported by the Daniel and Nina Carasso Foundation (00062696).
Conflict of Interest
The authors declare that the research was conducted in the absence of any commercial or financial relationships that could be construed as a potential conflict of interest.
Publisher's Note
All claims expressed in this article are solely those of the authors and do not necessarily represent those of their affiliated organizations, or those of the publisher, the editors and the reviewers. Any product that may be evaluated in this article, or claim that may be made by its manufacturer, is not guaranteed or endorsed by the publisher.
Acknowledgments
The Carasso Foundation funded the main field component of research in Peru (2015–2018). Additional funding was provided through the E. Willard and Ruby S. Miller Professor of Geography designation (2019–2022). Earlier versions of this work were presented to the following: Universidad Nacional Hermilio Valdizan (UNHEVAL) in Huánuco, Peru (2018), the Global Land Project Meeting in Bern, Switzerland (2018), American Association of Geographers annual meeting (2018), Harlan 3 Agrobiodiversity Conference in Montpellier, France (2019), Conference of Latin Americanist Geographers (CLAG Live, 2021), and the annual gathering of the Andean Community of Practice of the McKnight Foundation (2021). Numerous colleagues both in and apart from these meetings, the members of the GeoSyntheSES Lab, and the reviewers and special-issue editors of the journal provided helpful inputs that have been incorporated.
Supplementary Material
The Supplementary Material for this article can be found online at: https://www.frontiersin.org/articles/10.3389/fsufs.2022.734943/full#supplementary-material
Footnotes
1. ^Trees and herbs provided important food and nutrition though the legume crops, as a category, were more important as a source of food and demonstrated determinant of healthy diet and nutrition outcomes among local populations in Huánuco and elsewhere (Jones et al., 2018).
References
Abay, F., Bjørnstad, A., and Smale, M. (2009). Measuring on farm diversity and determinants of barley diversity in Tigray, northern Ethiopia. Momona Ethiop. J. Sci. 1, 44–66. doi: 10.4314/mejs.v1i2.46048
Arce, A., de Haan, S., Burra, D. D., and Ccanto, R. (2019a). Unearthing unevenness of potato seed networks in the high Andes: a comparison of distinct cultivar groups and farmer types following seasons with and without acute stress. Front. Sustain. Food Syst. 2, 1–22. doi: 10.3389/fsufs.2018.00043
Arce, A., de Haan, S., Juarez, H., Burra, D. D., Plasencia, F., Ccanto, R., et al. (2019b). The spatial-temporal dynamics of potato agrobiodiversity in the Highlands of Central Peru: a case study of smallholder management across farming landscapes. Land 8:169. doi: 10.3390/land8110169
Ban, N., and Coomes, O. T. (2004). Home gardens in Amazonian Peru: diversity and exchange of planting material. Geogr. Rev. 94, 348–367. doi: 10.1111/j.1931-0846.2004.tb00177.x
Baumann, M. D. (2022). Agrobiodiversity's caring material practices as a symbolic frame for environmental governance in Colombia's southern Tolima. Geoforum 128, 286–299. doi: 10.1016/j.geoforum.2021.01.002
Bellon, M. R., Gotor, E., and Caracciolo, F. (2015). Conserving landraces and improving livelihoods: how to assess the success of on-farm conservation projects? Int. J. Agric. Sustain. 13, 167–182. doi: 10.1080/14735903.2014.986363
Benin, S., Smale, M., Pender, J., Gebremedhin, B., and Ehui, S. (2004). The economic determinants of cereal crop diversity on farms in the Ethiopian highlands. Agric. Econ. 31, 197–208. doi: 10.1111/j.1574-0862.2004.tb00257.x
Bhattarai, B., Beilin, R., and Ford, R. (2015). Gender, agrobiodiversity, and climate change: a study of adaptation practices in the Nepal Himalayas. World Dev. 70, 122–132. doi: 10.1016/j.worlddev.2015.01.003
Blesh, J. (2018). Functional traits in cover crop mixtures: biological nitrogen fixation and multifunctionality. J. Appl. Ecol. 55, 38–48. doi: 10.1111/1365-2664.13011
Bottazzi, P., and Boillat, S. (2021). Political agroecology in Senegal: historicity and repertoires of collective actions of an emerging social movement. Sustainability 13:6352. doi: 10.3390/su13116352
Brookfield, H. (2001). Exploring Agrodiversity. New York, NY: Columbia University Press. doi: 10.7312/broo10232
Brush, S. B. (2000). Genes in the Field: On-Farm Conservation of Crop Diversity. Washington, DC: Island Press.
Brush, S. B., and Perales, H. R. (2007). A maize landscape: ethnicity and agro-biodiversity in Chiapas Mexico. Agric. Ecosyst. Environ. 121, 211–221. doi: 10.1016/j.agee.2006.12.018
Caillon, S., Cullman, G., Verschuuren, B., and Sterling, E. J. (2017). Moving beyond the human–nature dichotomy through biocultural approaches. Ecol. Soc. 22:27. doi: 10.5751/ES-09746-220427
CIP (2019). Los andes y los alimentos del futuro. 50 Andean Future Foods. Lima: Wust Ediciones. Available online at: https://cipotato.org/publications/los-andes-y-los-alimentos-del-futuro-50-andean-future-foods/ (accessed March 17, 2022).
Coe, M. A., and Gaoue, O. G. (2020). Cultural keystone species revisited: are we asking the right questions? J. Ethnobiol. Ethnomed. 16, 1–11. doi: 10.1186/s13002-020-00422-z
Colwell, R. K. (2009). “Biodiversity: concepts, patterns, and measurement,” in The Princeton Guide to Ecology, ed S. A. Levin (Princeton, NJ: Princeton University Press), 257. doi: 10.1515/9781400833023.257
Conrad, C., Löw, F., and Lamers, J. P. (2017). Mapping and assessing crop diversity in the irrigated Fergana Valley, Uzbekistan. Appl. Geogr. 86, 102–117. doi: 10.1016/j.apgeog.2017.06.016
Coomes, O. T., and Ban, N. (2004). Cultivated plant species diversity in home gardens of an Amazonian peasant village in northeastern Peru. Econ. Bot. 58, 420–434. doi: 10.1663/0013-0001(2004)0580420:CPSDIH2.0.CO;2
Davic, R. D. (2003). Linking keystone species and functional groups: a new operational definition of the keystone species concept. Conserv. Ecol. 7:701r11. doi: 10.5751/ES-00502-0701r11
de Haan, S. (2021). “Community-based conservation of crop genetic resources,” in Plant Genetic Resources, ed M. E. Dulloo (Cambridge: Burleigh Dodds Science), 229–249. doi: 10.4324/9781003180623-13
de Haan, S., Núñez, J., Bonierbale, M., and Ghislain, M. (2010). Multilevel agrobiodiversity and conservation of Andean potatoes in Central Peru. Mt. Res. Dev. 30, 222–231. doi: 10.1659/MRD-JOURNAL-D-10-00020.1
De Molina, M. G., Petersen, P. F., Peña, F. G., and Caporal, F. R. (2019). Political Agroecology: Advancing the Transition to Sustainable Food Systems. Boca Raton, FL: CRC Press. doi: 10.1201/9780429428821
Dedeurwaerdere, T., and Hannachi, M. (2019). Socio-economic drivers of coexistence of landraces and modern crop varieties in agro-biodiversity rich Yunnan rice fields. Ecol. Econ. 159, 177–188. doi: 10.1016/j.ecolecon.2019.01.026
Dessie, A. B., Abate, T. M., Mekie, T. M., and Liyew, Y. M. (2019). Crop diversification analysis on red pepper dominated smallholder farming system: evidence from northwest Ethiopia. Ecol. Process 8, 1–11. doi: 10.1186/s13717-019-0203-7
Di Falco, S., Bezabih, M., and Yesuf, M. (2010). Seeds for livelihood: crop biodiversity and food production in Ethiopia. Ecol. Econ. 69, 1695–1702. doi: 10.1016/j.ecolecon.2010.03.024
Downs, S. M., Ahmed, S., Fanzo, J., and Herforth, A. (2020). Food environment typology: advancing an expanded definition, framework, and methodological approach for improved characterization of wild, cultivated, and built food environments toward sustainable diets. Foods 9:532. doi: 10.3390/foods9040532
Dwivedi, S., Sahrawat, K., Upadhyaya, H., and Ortiz, R. (2013). Food, nutrition and agrobiodiversity under global climate change. Adv. Agron. 120, 1–128. doi: 10.1016/B.978-0-12-407686-0.00001-4
Fanzo, J., Hunter, D., Borelli, T., and Mattei, F. (2013). Diversifying Food and Diets: Using Agricultural Biodiversity to Improve Nutrition and Health. London: Routledge. doi: 10.4324/9780203127261
FAO (2016). Minimum Dietary Diversity for Women: A Guide for Measurement. Rome: FAO Available online at: http://www.fao.org/3/i5486e/i5486e.pdf (accessed June 25, 2021).
Finney, D. M., and Kaye, J. P. (2017). Functional diversity in cover crop poly-cultures increases multifunctionality of an agricultural system. J. Appl. Ecol. 54, 509–517. doi: 10.1111/1365-2664.12765
Fonte, S. J., Vanek, S. J., Oyarzun, P., Parsa, S., Quintero, D. C., Rao, I. M., et al. (2012). Pathways to agroecological intensification of soil fertility management by smallholder farmers in the Andean highlands. Adv. Agron. 116, 125–184. doi: 10.1016/B978-0-12-394277-7.00004-X
Foote, J. A., Murphy, S. P., Wilkens, L. R., Basiotis, P. P., and Carlson, A. (2004). Dietary variety increases the probability of nutrient adequacy among adults. J. Nutr. 134, 1779–1785. doi: 10.1093/jn/134.7.1779
Frison, E. A., Cherfas, J., and Hodgkin, T. (2011). Agricultural biodiversity is essential for a sustainable improvement in food and nutrition security. Sustainability 3, 238–253. doi: 10.3390/su3010238
Garduño, G. L., and Perrings, C. (2020). The on-farm diversity of maize cultivars and landraces in the Lacandon region of Chiapas, Mexico. Dev. Agric. Econ. 12, 113–121. doi: 10.5897/JDAE2020.1170
Gatto, M., de Haan, S., Laborte, A., Bonierbale, M., Labarta, R., and Hareau, G. (2021). Trends in varietal diversity of main staple crops in Asia and Africa and implications for sustainable food systems. Front. Sust. Food Syst. 5:12. doi: 10.3389/fsufs.2021.626714
Gauchan, D., Joshi, B. K., Sthapit, S., and Jarvis, D. I. (2020). Traditional crops for household food security and factors associated with on-farm diversity in the Mountains of Nepal. J. Agri. Environ. 21, 31–43. Available online at: https://hdl.handle.net/10568/109041 (accessed March 17, 2022).
Gauchan, D., Smale, M., Maxted, N., Cole, M., Sthapit, B. R., Jarvis, D., et al. (2005). Socioeconomic and agroecological determinants of conserving diversity on-farm: the case of rice genetic resources in Nepal. Nepal Agri. Res. J. 6, 89–98. doi: 10.3126/narj.v6i0.3370
Gepts, P., Famula, T. R., Bettinger, R. L., Brush, S. B., Damania, A. B., McGuire, P. E., et al. (2012). Biodiversity in Agriculture: Domestication, Evolution, and Sustainability. Cambridge: Cambridge University Press. doi: 10.1017/CBO9781139019514
Gerits, F., Messely, L., Reubens, B., and Verheyen, K. (2021). A social–ecological framework and toolbox to help strengthening functional agrobiodiversity-supported ecosystem services at the landscape scale. Ambio 50, 360–374. doi: 10.1007/s13280-020-01382-0
Goldberg, Z. A., Powell, B., and Ouarghidi, A. (2021). Determinants of smallholder maintenance of crop diversity in Morocco's High Atlas Mountains. Hum. Ecol. 49, 453–465. doi: 10.1007/s10745-021-00248-7
Goslee, S. C. (2020). Drivers of agricultural diversity in the contiguous United States. Front. Sust. Food Syst. 4:75. doi: 10.3389/fsufs.2020.00075
Gould, R., Harp, B. D., Nackerman, C., and Maibach, E. (2021). Developing equitable health and climate solutions: Insights from the field. Environ. Res. Lett. 16:011002. doi: 10.1088/1748-9326/abca66
Graddy-Lovelace, G. (2021). Farmer and non-farmer responsibility to each other: negotiating the social contracts and public good of agriculture. J. Rural Stud. 82, 531–541. doi: 10.1016/j.jrurstud.2020.08.044
Hajjar, R., Jarvis, D. I., and Gemmill-Herren, B. (2008). The utility of crop genetic diversity in maintaining ecosystem services. Agric. Ecosyst. Environ. 123, 261–270. doi: 10.1016/j.agee.2007.08.003
Hayek, L. A., and Buzas, M. (2010). Surveying Natural Populations: Quantitative Tools for Assessing Biodiversity, 2nd Edn. New York, NY: Columbia University Press. doi: 10.7312/haye14620
Jackson, L. E., Pascual, U., and Hodgkin, T. (2007). Utilizing and conserving agrobiodiversity in agricultural landscapes. Agric. Ecosyst. Environ. 121, 196–210. doi: 10.1016/j.agee.2006.12.017
Jackson, L. E., Pulleman, M. M., Brussaard, L., Bawa, K. S., Brown, G. G., Cardoso, I. M., et al. (2012). Social-ecological and regional adaptation of agrobiodiversity management across a global set of research regions. Glob. Environ. Change 22, 623–639. doi: 10.1016/j.gloenvcha.2012.05.002
Jarvis, D. I., Brown, A. H., Cuong, P. H., Collado-Panduro, L., Latournerie-Moreno, L., Gyawali, S., et al. (2008). A global perspective of the richness and evenness of traditional crop-variety diversity maintained by farming communities. Proc. Natl. Acad. Sci. U. S. A. 105, 5326–5331. doi: 10.1073/pnas.0800607105
Jarvis, D. I., Padoch, C., and Cooper, H. D. (2007). Managing Biodiversity in Agricultural Ecosystems. New York, NY: Columbia University Press. doi: 10.7312/jarv13648
Jones, A. D. (2017). Critical review of the emerging research evidence on agricultural biodiversity, diet diversity, and nutritional status in low-and middle-income countries. Nutr. Rev. 75, 769–782. doi: 10.1093/nutrit/nux040
Jones, A. D., Creed-Kanashiro, H., Zimmerer, K. S., de Haan, S., Carrasco, M., Meza, K., et al. (2018). Farm-level agricultural biodiversity in the Peruvian Andes is associated with greater odds of women achieving a minimally diverse and micronutrient adequate diet. J. Nutr. 148, 1625–1637. doi: 10.1093/jn/nxy166
Kremen, C., Iles, A., and Bacon, C. (2012). Diversified farming systems: an agroecological, systems-based alternative to modern industrial agriculture. Ecol. Soc. 17:444. doi: 10.5751/ES-05103-170444
Labeyrie, V., Antona, M., Baudry, J., Bazile, D., Bodin, Ö., Caillon, S., et al. (2021). Networking agrobiodiversity management to foster biodiversity-based agriculture. A review. Agron. Sustain. Dev. 41, 1–15. doi: 10.1007/s13593-020-00662-z
Labeyrie, V., Thomas, M., Muthamia, Z. K., and Leclerc, C. (2016). Seed exchange networks, ethnicity, and sorghum diversity. Proc. Natl. Acad. Sci. U. S. A. 113, 98–103. doi: 10.1073/pnas.1513238112
Lachat, C., Raneri, J. E., Smith, K. W., Kolsteren, P., Van Damme, P., Verzelen, K., et al. (2018). Dietary species richness as a measure of food biodiversity and nutritional quality of diets. Proc. Natl. Acad. Sci. U. S. A. 115, 127–132. doi: 10.1073/pnas.1709194115
Li, C., Chen, X., Jiang, A., Lee, M. B., Mammides, C., and Goodale, E. (2021). Socioeconomic determinants of crop diversity and its effect on farmer income in Guangxi, Southern China. Agriculture 11, 336. doi: 10.3390/agriculture11040336
Malice, M., Bizoux, J. P., Blas, R., and Baudoin, J. P. (2010). Genetic diversity of Andean tuber crop species in the in situ microcenter of Huanuco, Peru. Crop Sci. 50, 1915–1923. doi: 10.2135/cropsci2009.09.0476
Martin, A. R., Cadotte, M. W., Isaac, M. E., Milla, R., Vile, D., and Violle, C. (2019). Regional and global shifts in crop diversity through the Anthropocene. PLoS ONE 14:e0209788. doi: 10.1371/journal.pone.0209788
Martin-Prevel, Y., Allemand, P., Wiesmann, D., Arimond, M., Ballard, T., Deitchler, M., et al. (2015). Moving Forward on Choosing a Standard Operational Indicator of Women's Dietary Diversity. Rome: FAO. Available online at: http://www.fao.org/3/a-i4942e.pdf (accessed June 25, 2021).
Mayer, E. (2009). Ugly Stories of the Peruvian Agrarian Reform. Durham: Duke University Press. doi: 10.1215/9780822390718
Mayer, E. (2018). The Articulated Peasant: Household Economies in the Andes. London: Routledge. doi: 10.4324/9780429496820
McCord, P. F., Cox, M., Schmitt-Harsh, M., and Evans, T. (2015). Crop diversification as a smallholder livelihood strategy within semi-arid agricultural systems near Mount Kenya. Land Use Policy 42, 738–750. doi: 10.1016/j.landusepol.2014.10.012
Meena, R. S., and Lal, R. (2018). “Legumes and sustainable use of soils,” in Legumes for Soil Health and Sustainable Management, eds R. S. Meena, A. Dup, G. S. Yadav, and R. Lal (Singapore: Springer), 1–31. doi: 10.1007/978-981-13-0253-4_1
Mercer, K. L., and Perales, H. R. (2010). Evolutionary response of landraces to climate change in centers of crop diversity. Evol. Appl. 3, 480–493. doi: 10.1111/j.1752-4571.2010.00137.x
Momsen, J. H. (2007). Gender and agrobiodiversity: introduction to special issue. Singap. J. Trop. Geogr. 28, 1–6. doi: 10.1111/j.1467-9493.2006.00272.x
Mwololo, H. M., Nzuma, J. M., Ritho, C. N., and Aseta, A. (2019). Is the type of agricultural extension services a determinant of farm diversity? Evidence from Kenya. Dev. Stud. Res. 6, 40–46. doi: 10.1080/21665095.2019.1580596
Nabhan, G. P. (2012). Where Our Food Comes From: Retracing Nikolay Vavilov's Quest to End Famine. Washington, DC: Island Press.
Nabhan, G. P. (2018). Food From the Radical Center. Washington, DC: Island Press. doi: 10.5822/978-1-61091-920-3
Ng'endo, M., Keding, G. B., Bhagwat, S., and Kehlenbeck, K. (2015). Variability of on-farm food plant diversity and its contribution to food security: a case study of smallholder farming households in Western Kenya. Agroecol. Sustain. Food Syst. 39, 1071–1103. doi: 10.1080/21683565.2015.1073206
Nordhagen, S., Pascual, U., and Drucker, A. G. (2017). Feeding the household, growing the business, or just showing off? Farmers' motivations for crop diversity choices in Papua New Guinea. Ecol. Econ. 137, 99–109. doi: 10.1016/j.ecolecon.2017.02.025
Novotny, I. P., Tittonell, P., Fuentes-Ponce, M. H., López-Ridaura, S., and Rossing, W. A. (2021). The importance of the traditional milpa in food security and nutritional self-sufficiency in the highlands of Oaxaca, Mexico. PLoS ONE 16:e0246281. doi: 10.1371/journal.pone.0246281
Obayelu, O. A., Akintunde, O. O., and Obayelu, A. E. (2015). Determinants of on-farm cassava biodiversity in Ogun State, Nigeria. Int. J. Biodivers. Sci. Ecosyst. Serv. Manag. 11, 298–308. doi: 10.1080/21513732.2015.1071282
Orozco-Ramírez, Q., Ross-Ibarra, J., Santacruz-Varela, A., and Brush, S. (2016). Maize diversity associated with social origin and environmental variation in Southern Mexico. Heredity 116, 477–484. doi: 10.1038/hdy.2016.10
Oyarzun, P. J., Borja, R. M., Sherwood, S., and Parra, V. (2013). Making sense of agrobiodiversity, diet, and intensification of smallholder family farming in the highland Andes of Ecuador. Ecol. Food Nutr. 52, 515–541. doi: 10.1080/03670244.2013.769099
Pascual, U., Narloch, U., Nordhagen, S., and Drucker, A. G. (2011). The economics of agrobiodiversity conservation for food security under climate change. Econ. Agrar. Recur. Nat. 11, 191–200. Available online at: https://hdl.handle.net/10568/35788 (accessed March 17, 2022).
Pérez-Garcia, O., and del Castillo, R. F. (2016). The decline of the itinerant milpa and the maintenance of traditional agrobiodiversity: Crops and weeds coexistence in a tropical cloud forest area in Oaxaca, Mexico. Agric. Ecosyst. Environ. 228, 30–37. doi: 10.1016/j.agee.2016.05.002
Perrault-Archambault, M., and Coomes, O. T. (2008). Distribution of agrobiodiversity in home gardens along the Corrientes River, Peruvian Amazon. Econ. Bot. 62, 109–126. doi: 10.1007/s12231-008-9010-2
Perronne, R., Makowski, D., Goffaux, R., Montalent, P., and Goldringer, I. (2017). Temporal evolution of varietal, spatial and genetic diversity of bread wheat between 1980 and 2006 strongly depends upon agricultural regions in France. Agr. Ecosyst. Environ. 236, 12–20. doi: 10.1016/j.agee.2016.11.003
Petersen-Rockney, M., Baur, P., Guzman, A., Bender, S. F., Calo, A., Castillo, F., et al. (2021). Narrow and brittle or broad and nimble? Comparing adaptive capacity in simplifying and diversifying farming systems. Front. Sustain. Food Syst. 5:56. doi: 10.3389/fsufs.2021.564900
Pulgar Vidal, J. (1996). Geografía del Peru: Las ocho regiones naturales del Perú, la regionalización transversal, la sabiduría tradicional, 10th Edn. Lima: PEISA.
Rahman, S., and Kazal, M. M. H. (2015). Determinants of crop diversity in the regions of Bangladesh (1990–2008). Singap. J. Trop. Geogr. 36, 83–97. doi: 10.1111/sjtg.12086
Reyes-García, V., Vadez, V., Martí, N., Huanca, T., Leonard, W. R., and Tanner, S. (2008). Ethnobotanical knowledge and crop diversity in swidden fields: a study in a native Amazonian society. Hum. Ecol. 36, 569–580. doi: 10.1007/s10745-008-9177-2
Saxena, A. K., Fuentes, X. C., Herbas, R. G., and Humphries, D. L. (2016). Indigenous food systems and climate change: Impacts of climatic shifts on the production and processing of native and traditional crops in the Bolivian Andes. Front. Public Health 4. doi: 10.3389/fpubh.2016.00020
Schipanski, M. E., and Drinkwater, L. E. (2012). Nitrogen fixation in annual and perennial legume-grass mixtures across a fertility gradient. Plant Soil 357, 147–159. doi: 10.1007/s11104-012-1137-3
Scurrah, M., Ccanto, R., and Bonierbale, M. (2019). “Participatory varietal selection in the Andes,” in Farmers and Plant Breeding: Current Approaches and Perspectives, eds O. T. Westengen and T. Winge (London: Routledge), 8. doi: 10.4324/9780429507335-8
Skarbø, K. (2014). The cooked is the kept: factors shaping the maintenance of agro-biodiversity in the Andes. Hum. Ecol. 42, 711–726. doi: 10.1007/s10745-014-9685-1
Smale, M. (2006). “Concepts, metrics and plan of the book,” in Valuing Crop Biodiversity: On-farm Genetic Resources and Economic Change, ed M. Smale (Rome: Biodiversity International), 1–16. doi: 10.1079/9780851990835.0001
Smale, M., Bellon, M. R., and Aguirre Gomez, J. A. (2001). Maize diversity, variety attributes, and farmers' choices in Southeastern Guanajuato, Mexico. Econ. Dev. Cult. Change 50, 201–225 doi: 10.1086/340010
Smale, M., Lipper, L., and Koundouri, P. (2006). “Scope, limitations and future directions,” in Valuing Crop Biodiversity: On-farm Genetic Resources and Economic Change, ed M. Smale (Rome: Biodiversity International) 280–295. doi: 10.1079/9780851990835.0280
Smil, V. (1997). Some unorthodox perspectives on agricultural biodiversity: the case of legume cultivation. Agr. Ecosyst. Environ. 62, 135–144. doi: 10.1016/S0167-8809(96)01138-3
Tamariz, G. (2022). Agrobiodiversity conservation with illegal-drug crops: an approach from the prisons in Oaxaca, Mexico. Geoforum 128, 300–311. doi: 10.1016/j.geoforum.2020.10.012
Tamariz, G., and Baumann, M. D. (2022). Agrobiodiversity change in violent conflict and post-conflict landscapes. Geoforum 128, 217–222. doi: 10.1016/j.geoforum.2021.11.010
Toledo, V. M., and Barrera-Bassols, N. (2017). Political agroecology in Mexico: a path toward sustainability. Sustainability 9:268. doi: 10.3390/su9020268
Van Dusen, M. E., and Taylor, J. E. (2005). Missing markets and crop diversity: evidence from Mexico. Environ. Dev. Econ. 10, 513–531. doi: 10.1017/S1355770X05002317
van Vliet, J. A., Schut, A. G., Reidsma, P., Descheemaeker, K., Slingerland, M., van de Ven, G. W., et al. (2015). De-mystifying family farming: features, diversity and trends across the globe. Glob. Food Sec. 5, 11–18. doi: 10.1016/j.gfs.2015.03.001
Vandermeer, J., Aga, A., Allgeier, J., Badgley, C., Baucom, R., Blesh, J., et al. (2018). Feeding Prometheus: an interdisciplinary approach for solving the global food crisis. Front. Sustain. Food Syst. 2:39. doi: 10.3389/fsufs.2018.00039
Vanek, S. J., Meza, K., Ccanto, R., Olivera, E., Scurrah, M., and Fonte, S. J. (2020). Participatory design of improved forage/fallow options across soil gradients with farmers of the Central Peruvian Andes. Agr. Ecosyst. Environ. 300:106933. doi: 10.1016/j.agee.2020.106933
Velásquez-Milla, D., Casas, A., Torres-Guevara, J., and Cruz-Soriano, A. (2011). Ecological and socio-cultural factors influencing in situ conservation of crop diversity by traditional Andean households in Peru. J. Ethnobiol. Ethnomedicine 7, 1–20. doi: 10.1186/1746-4269-7-40
Villéger, S., Mason, N. W. H., Mouillot, D., and Mason, H. (2008). New multidimensional functional diversity indices for a multifaceted framework in functional ecology. Ecol. 89, 2290–2301. doi: 10.1890/07-1206.1
Wale, E., and Holm-Mueller, K. (2017). Explaining the Ethiopian farmers' perceptions on potential loss of traditional crop varieties: a principal components regression analysis. J. Dev. Areas 51, 377–395. doi: 10.1353/jda.2017.0108
Wezel, A., and Ohl, J. (2005). Does remoteness from urban centres influence plant diversity in homegardens and swidden fields? a case study from the Matsiguenka in the Amazonian rain forest of Peru. Agrofor. Syst. 65, 241–251. doi: 10.1007/s10457-005-3649-9
Whitney, C. W., Luedeling, E., Tabuti, J. R., Nyamukuru, A., Hensel, O., Gebauer, J., et al. (2018). Crop diversity in homegardens of southwest Uganda and its importance for rural livelihoods. Agric. Human Values 35, 399–424. doi: 10.1007/s10460-017-9835-3
Willett, W., Rockström, J., Loken, B., Springmann, M., Lang, T., Vermeulen, S., et al. (2019). Food in the Anthropocene: the EAT–Lancet Commission on healthy diets from sustainable food systems. Lancet 393, 447–492. doi: 10.1016/S0140-6736(18)31788-4
Williams, N. E. (2016). The political ecology of 'ethnic' agricultural biodiversity maintenance in Atlantic Nicaragua. J. Polit. Ecol. 23, 223–245. doi: 10.2458/v23i1.20210
Williams, N. E., Carrico, A. R., Edirisinghe, I., and Champika, P. J. (2018). Assessing the impacts of agrobiodiversity maintenance on food security among farming households in Sri Lanka's dry zone. Econ. Bot. 72, 196–206. doi: 10.1007/s12231-018-9418-2
Williams, N. E., and Kramer, D. B. (2019). Agricultural biodiversity maintenance in a coastal socio-ecological system: the pearl lagoon basin, Nicaragua. Hum. Ecol. 47, 111–120. doi: 10.1007/s10745-018-0042-7
Wood, S. A., Karp, D. S., DeClerck, F., Kremen, C., Naeem, S., and Palm, C. A. (2015). Functional traits in agriculture: agrobiodiversity and ecosystem services. Trends Ecol. Evol. 30, 531–539. doi: 10.1016/j.tree.2015.06.013
Zapico, F. L., Dizon, J. T., Fernando, E. S., Borromeo, T. H., McNally, K. L., and Hernandez, J. E. (2020). Upland rice: cultural keystone species in a Philippine Traditional Agroecosystem. Trends Ecol. Evol. 17, 93–104. doi: 10.37801/ajad2020.17.2.6
Zimmerer, K. S. (1991). Labor shortages and crop diversity in the southern Peruvian sierra. Geogr. Rev. 81, 414–432. Available online at: https://www.jstor.org/stable/215608 (accessed March 17, 2022).
Zimmerer, K. S. (1996). Changing Fortunes: Biodiversity and Peasant Livelihood in the Peruvian Andes. Berkeley, CA: University of California Press.
Zimmerer, K. S. (2010). Biological diversity in agriculture and global change. Annu. Rev. Environ. Resour. 35, 137–166. doi: 10.1146/annurev-environ-040309-113840
Zimmerer, K. S., and de Haan, S. (2017). Agrobiodiversity and a sustainable food future. Nat. Plants 3, 1–3. doi: 10.1038/nplants.2017.47
Zimmerer, K. S., and de Haan, S. (2019). Agrobiodiversity: Integrating Knowledge for a Sustainable Future. Cambridge: MIT Press. doi: 10.7551/mitpress/11989.001.0001
Zimmerer, K. S., and de Haan, S. (2020). Informal food chains and agrobiodiversity need strengthening—not weakening—to address food security amidst the COVID-19 crisis in South America. Food Secur. 12, 891–894. doi: 10.1007/s12571-020-01088-x
Zimmerer, K. S., de Haan, S., Jones, A. D., Creed-Kanashiro, H., Tello, M., Amaya, F. P., et al. (2020). Indigenous smallholder struggles in Peru: nutrition security, agrobiodiversity, and food sovereignty amid transforming global systems and climate change. J. Lat. Am. Geogr. 19, 74–111. doi: 10.1353/lag.2020.0072
Zimmerer, K. S., de Haan, S., Jones, A. D., Creed-Kanashiro, H., Tello, M., Carrasco, M., et al. (2019). The biodiversity of food and agriculture (agrobiodiversity) in the Anthropocene: research advances and conceptual framework. Anthropocene 25:100192. doi: 10.1016/j.ancene.2019.100192
Zimmerer, K. S., Duvall, C. S., Jaenicke, T. C., Minaker, L. M., Reardon, T., and Seto, K. C. (2021). Urbanization and agrobiodiversity: leveraging a key sustainability nexus. One Earth 4, 1557–1568. doi: 10.1016/j.oneear.2021.10.012
Keywords: agricultural biodiversity, agroecology, Sustainable Development Goal 2, Agrobiodiversity Knowledge Framework, key agrobiodiversity-and-food spaces, political ecology, social-ecological systems, Peru
Citation: Zimmerer KS, Jones AD, de Haan S, Creed-Kanashiro H, Tubbeh RM, Hultquist C, Tello Villavicencio MN, Plasencia Amaya F and Nguyen KT (2022) Integrating Social-Ecological and Political-Ecological Models of Agrobiodiversity With Nutrient Management of Keystone Food Spaces to Support SDG 2. Front. Sustain. Food Syst. 6:734943. doi: 10.3389/fsufs.2022.734943
Received: 01 July 2021; Accepted: 03 March 2022;
Published: 31 March 2022.
Edited by:
Meagan Schipanski, Colorado State University, United StatesReviewed by:
Selena Ahmed, Montana State University, United StatesAidee Guzman, University of California, Berkeley, United States
Copyright © 2022 Zimmerer, Jones, de Haan, Creed-Kanashiro, Tubbeh, Hultquist, Tello Villavicencio, Plasencia Amaya and Nguyen. This is an open-access article distributed under the terms of the Creative Commons Attribution License (CC BY). The use, distribution or reproduction in other forums is permitted, provided the original author(s) and the copyright owner(s) are credited and that the original publication in this journal is cited, in accordance with accepted academic practice. No use, distribution or reproduction is permitted which does not comply with these terms.
*Correspondence: Karl S. Zimmerer, ksz2@psu.edu