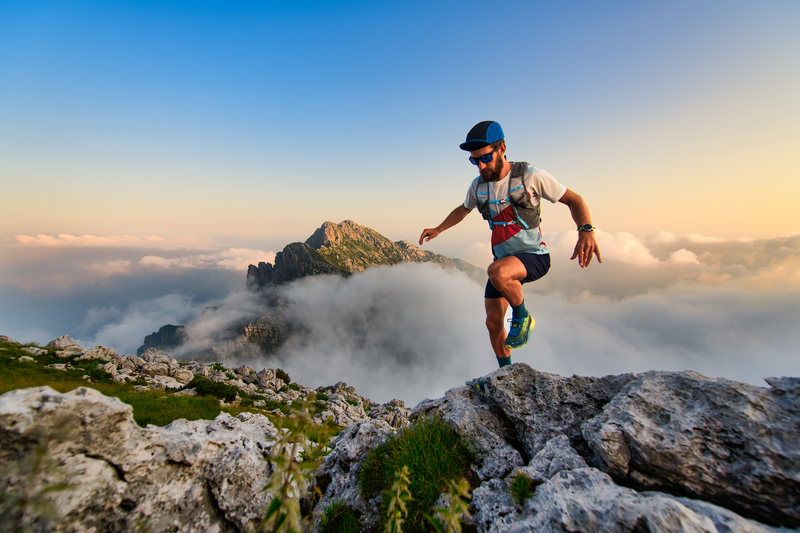
94% of researchers rate our articles as excellent or good
Learn more about the work of our research integrity team to safeguard the quality of each article we publish.
Find out more
ORIGINAL RESEARCH article
Front. Sustain. Food Syst. , 15 June 2022
Sec. Climate-Smart Food Systems
Volume 6 - 2022 | https://doi.org/10.3389/fsufs.2022.702405
Maize cropping systems in Kenya, as is true in many other places in Africa, face multiple biotic and abiotic stressors not least climatic ones. Guided by farmers' priorities, maize breeding programs can contribute to the needed resilience against these changes by developing and mainstreaming new generations of maize varieties adapted to these challenges. Using data from 1,400 farmers and applying a multi-criteria choice analysis, this study reports on smallholder farmers' relative valuation of stress tolerance traits. The results showed that farmers were willing to pay significant premiums for tolerance to drought, striga, low nitrogen (nitrogen use efficiency) and fall army worm infestation, in that order. Large scale incorporation of these traits in legacy varieties as well as new ones, can contribute to enhancing maize system resilience and adaptation to changing growing conditions. For seed systems development, these traits can provide the basis for making strong business cases for the replacement of old varieties with new, stress-adapted ones.
As smallholder farming systems form the majority of maize production in Kenya, it is imperative to enhance their productivity, resilience, and adaptability to climate changes. Part of the toolkit to achieve these aims involves mainstreaming stress- and climate-adapted maize varieties. Maize production in most sub-Saharan African countries has yet to reach its potential due to a combination of factors, including drought, degraded and infertile soils, diseases, pests, weeds, and the limited use of improved seed or chemical fertilizer (International Maize and Wheat Improvement Center; CIMMYT, 2013). An estimated 40% of Africa's maize-growing area faces occasional drought stress, resulting in yield losses of 10–25% (CIMMYT, 2013). A combination of supply and demand factors can also reduce access to modern seeds. For example, the adoption of stress-tolerant maize varieties in eastern and southern Africa was conditioned by their availability, inadequate information, a lack of resources, high seed prices, and their perceived attributes (Fisher et al., 2015).
In recent years, national and international breeding systems have implemented programs to produce stress-tolerant varieties focusing on tolerance to drought, weeds, low soil nitrogen, and insects. For example, Kenya Agricultural and Livestock Research Organization (KALRO), the national research body in Kenya, has implemented a number of such breeding programs. The multi-year and multi-million dollar Drought Tolerant Maize for Africa project (2006–2015), which was implemented in 12 other African countries, is an example. Further, the Improved Maize for African Soils project (2010–15) focused on breeding for efficient nitrogen use.
The Water-Efficient Maize for Africa project (2008–2018) was meant to produce varieties that were suited for moisture-stressed environments, while the Insect-Resistant Maize for Africa project (1999–2004) aimed to promote insect resistance. Recently, the Stress-Tolerant Maize for Africa project (2016–2020) was implemented to consolidate some of the previously mentioned legacy breeding programs to focus on multiple stress-tolerant breeding goals, such as combating drought, invasive, competitive plant species, and low nitrogen, among others. The focus on these stress elements is understandable, as these affect Kenya and other countries in the region in various ways.
Drought is estimated to cause up to a 39% seasonal reduction in yield in much of sub-Saharan Africa (Daryanto et al., 2016). According to CIMMYT (2013), frequent droughts—or those affecting 25% of the maize area—often lead to 50% of losses during the production season. The same study (CIMMYT, 2013) indicated that 40% of the maize area suffers from occasional droughts, with yield losses of 10–25%. An ex-ante assessment of the benefits of diffusion of drought-tolerant maize varieties in 13 African countries, including Kenya, would range from 9 to $1.5 billion (Rovere et al., 2014), revealing the potential economic fallout from unmitigated effects of droughts.
The striga weed (Striga hermonthica), also known by its common name “witch weed,” is a parasitic plant of cereals, including maize. It depletes the host's nutrients by inserting its roots into those of the host's root tissues. The resulting nutrient malnutrition can create extremely low or absent yields depending on the severity of infestation or the host plant's tolerance (Ejeta, 2007). In the 1990s, striga was estimated to impact as much as 50–100 million ha in sub-Saharan Africa (Lagoke et al., 1991; Kanampiu et al., 2018), with consequent yield losses ranging from 20 to 80% (Khan et al., 2006; Ejeta, 2007). Striga's negative effects can be observed in expansive fields over a short period of time due to its diverse seed-dispersal mechanisms, including contaminated crop seeds, farm implements, wind, and animals, among others (Berner et al., 1996; Ejeta, 2007).
Climate change has expanded striga's range of habitats across maize-growing areas. Mechanical, chemical, and biological control methods are all used to some degree, such as physical weeding, crop rotation, both trap and catch crops, and the use of herbicides and fertilizers (Gressel et al., 2004). However, none have successfully eliminated the striga menace, and especially in Africa. The weed's physiology and adaptability has made it difficult to control, as it has a unique life cycle with broad genetic variation in its reproductive process and it infects a wide range of crops (Ejeta, 2007; Hearne, 2009). Host plant resistance is a promising strategy in controlling striga, and especially when combined with beneficial agronomic practices, including soil nutrient management.
Maize is a nutrient-demanding crop sensitive to nutrient deficiencies, especially nitrogen (Emede and Alika, 2012). Further, large amounts of nutrients are often required to achieve the genetic promise of high yields (Arisede et al., 2020), while many African soils are depleted of macro-nutrients (Drechsel et al., 2001; Pasley et al., 2020). While Smaling et al. (1993) estimated nitrogen (N) depletion due to maize production at 42 kg/N per ha annually, many farmers apply limited nutrients due to a lack of funds to purchase fertilizers, a long-standing issue (Kamara et al., 2006). The fertilizers that are distributed are applied in small quantities. Sheahan and Barrett (2017) estimated that most of Africa only uses ~5 to 12 kg/N fertilizer applications per hectare.
The development of N-efficient maize that can withstand low levels of nitrogen in many African soils is an important contribution to enabling low-input producers to best use the available soil N, in addition to the limited amounts they can afford (Arisede et al., 2020). Maize varieties with high nitrogen use efficiency (NUE) do so through a higher recovery efficiency of N (Arisede et al., 2020), which reduces N losses and provides a cost advantage for farmers who can only afford limited amounts of N, such as the majority of African smallholders (Sheahan and Barrett, 2017). Broadly and in the long run, farmers must be careful, as excessive N applications can increase greenhouse gas emissions. Some estimates suggest that over half of applied N is lost through leaching, and therefore, is not utilized by plants (Arisede et al., 2020).
Globally, nitrogen from fertilizers contribute to 30% of the total nitrous oxide, which contributes to air pollution and the accumulation of greenhouse gases in the atmosphere (Dobermann and Cassman, 2005; Skiba and Rees, 2014). This suggests a strong case for N use efficiency through agronomic and genetic approaches. Therefore, the pathway toward increased input use and productivity, even in low-input-use environments, should involve identifying methods to increase the efficiency of nitrogen use. Subsequently, small-scale farmers with low agricultural inputs can use crop varieties selected and bred for low-N tolerance as a reliable economic method to increase maize yields. This also provides a sustainable approach to mitigate climate change (Badu-Apraku et al., 2018).
The fall armyworm (Spodoptera frugiperda JE Smith)—hereafter “FAW”—is an insect that originated in the Americas, and was first reported in Africa in early 2016 (Goergen et al., 2016). The FAW has the remarkable ability to migrate over long distances on prevailing winds, produce many eggs, and thrive in a variety of hosts and environments (Day et al., 2017; Prasanna et al., 2018). By 2018, it had been identified in 30 countries across the continent, including Kenya, with losses of up to 40% estimated in Ghana and Zambia (Day et al., 2017), 47% in Kenya, and 32% in Ethiopia (Kumela et al., 2019). Further, Prasanna et al. (2018) illustrated the devastating effects of FAW by reporting that the insect can feed on more than 80 different crop species, such as maize, sorghum, rice, sugarcane, pasture grasses, and millet. The Center for Agriculture and Biosciences International, CABI (2017) estimated the FAW's potential economic devastation as 8.3–20.6 million metric tons of crops per year, with impacts in a dozen maize-producing countries in Africa accounting for 21–53% of yield losses in a 3-year period. In monetary terms, these losses amount to up to $6.2 billion.
De Groote et al. (2020) found that over 80% of farmers in Kenya have already been affected by FAW and reported over 30% in yield losses, with variations observed across ecological zones. A range of integrated pest-management practices (IPMs) can be deployed to manage the FAW (Prasanna et al., 2018). While pesticides can be effective, the environmental and health risks demand that appropriate IPMs be promoted, and especially in resource-constrained environments in Africa with little awareness of their safe use and abundant regulatory gaps. Therefore, developing host plant resistance is a crucial element in effective IPMs to manage the FAW. In all cases, IPM strategies that combine good agronomic practices and measures of FAW resistance in maize can be deemed a higher priority than chemical-dependent interventions from a human or environmental health perspective and regardless of farmers' economic or educational circumstances.
Given these challenges, maize breeding programs can provide the necessary resilience by developing and promoting a new generation of maize varieties that are bred to withstand or tolerate these stresses. These efforts should be guided by farmers' priorities for the new varieties to quickly gain broad acceptance. This study was designed to understand the willingness to sacrifice yield for stress-tolerant varieties. We consider the tolerance to four stresses: drought, the striga weed, fall armyworm, and low nitrogen (measured by a lower top-dressing requirement).
The seed markets in Kenya and most of the maize markets in Africa, are characterized by what is typically called low variety turnover (newer varieties are slow to gain market share to replace old ones). The number of varieties that are drought tolerant have increased in recent years. Varieties tolerant to FAW are just beginning to come onto commercial markets. Varieties tolerant to striga and low N have been around for some time. The challenge is that due to a variety of reasons around farmers' decision inertia, weak marketing strategies and low profits for seed companies in investing in new varieties (because it takes a long time to gain market share), new superior varieties remain poorly commercialized.
Regarding prices, seed prices usually do not vary based on traits (Rutsaert and Donovan, 2020). This is a major weakness that is frequently cited as an impediment for introducing new varieties. The experiential nature of new seed varieties often makes many farmers hesitant to buy these new varieties without first observing them in a low-cost way and for a period of time (leading to the slow variety turnover cited above). This also discourages seed companies from investing in the upfront promotional costs, thereby creating a viscous cycle of slow market insertion of new varieties. In focus group discussions, seed price was not mentioned as an attribute that farmers considered when deciding on their seed purchases, reflecting the undifferentiated nature of the market. This is an area that requires careful attention in research and development.
In summary, seed prices do not vary by traits, this is a weakness of the seed sector in Kenya (and the region). This is the main reason we use yield to assess farmers' valuation of these traits (this information is itself valuable) to inform breeding investments or innovative trait-based commercialization efforts. This paper provides much-needed insights into farmers prioritization of the relevant traits to provide the basis for feedback to seed companies, breeders, policy makers and others to inform maize improvement priorities.
The study was conducted in the mid-altitude, moist, transitional maize-growing areas of western Kenya. The sample was limited to the administrative and agro-ecological characteristics of the region's Busia and Siaya counties. These counties were some of the implementation sites for a stress-tolerant maize breeding and seed-development project conducted by the KALRO and CIMMYT. The dominance of smallholder maize production systems and maize's role as a prominent staple in farmers' crop portfolios were factors also considered in developing the sampling for this study. Finally, we considered the relatively low penetration of stress-tolerant hybrids in these counties' and jurisdictions' seed markets when selecting them for inclusion in this study. Among the four sub-counties selected—Central Alego and Alego-Usonga in Siaya County; and Butula and Samia in Busia County—eight wards were selected: two in Siaya and six in Busia.
The final stage of sampling occurred by randomly selecting villages from each ward based on the sampling principle of probability proportional to size. As more villages were sampled from the larger wards, these wards contributed more to the sample. In each village, 40 households were randomly selected to generate a final random sample of 701 households. Two adults from each household separately participated in the interview, with questions relating to household data answered by the head of the household as the household representative. These questions were not re-administered to the second respondent (typically the spouse or another adult member of the family). After the head of household finished answering their questions and participating in the choice experiment (to be described below), the spouse or other adult in the household with significant maize-production responsibilities participated in the full choice experiment. This second respondent also answered a subset of the other interview questions related to the management of the plots they were responsible for. This process generated a total of 1,400 respondents: 701 were household heads, 419 were spouses, and 280 were other adult household members. Of the 1,400 respondents, 732 (52.3%) were female. The map displayed in Figure 1 summarizes the sampled districts, villages, and households.
This study implemented a choice experiment to elicit farmers' relative preferences for selected yield traits. Diverse literature has discussed choice experiment methods in the transport, health, marketing, and environmental economics fields (Hensher et al., 2005; Louviere et al., 2010; Schipmann and Qaim, 2011; Veettil et al., 2011; Meemken et al., 2017; Ochieng et al., 2017; Wanyama et al., 2019). The farmers in this study were presented with two hypothetical maize varieties with varying levels of the identified traits: yield and the tolerance to drought, striga, low nitrogen, and the FAW. In using a choice experiment approach, we construe farmers' choices of their preferred variety as following a random utility framework, calculated as
where U is the utility of farmer i associated with choice j, V is the deterministic component, and ε is the random element. The choice of j among several alternatives means that the utility derived from j is greater that the utility derived from all other attributes. Assuming Yi is a random variable that indicates the choice made, and given the choice between alternatives j and k, the probability that farmer i chooses alternative j is
Therefore, the utility Uij depends on attributes of (choice) variety j, and farmer i's individual characteristics. Assuming m is a vector of farmer characteristics and A is a vector of the alternative's attributes, it follows that
where β and α are the corresponding vectors of the parameters to be estimated.
We use a mixed logit (MIXL) model as an improvement over the multinomial logit (MNL) and conditional logit (CL) models (McFadden, 1973; Hoffman and Duncan, 1988; McFadden and Train, 2000; Hensher and Greene, 2003). The MNL is appropriate when the focus is on the individual as a unit of analysis, and uses the individual's characteristics as explanatory variables; in contrast, the CL focuses on a set of alternatives for each individual, and the characteristics of these alternatives are used as explanatory variables (Hoffman and Duncan, 1988). The MNL and CL can be calculated as
where M represents the characteristics of individual i, Aij denotes the characteristics of alternative j for individual i, and β and α are the corresponding vectors of parameters that represent the influence of individual characteristics and choice attributes.
A strong assumption for the MNL and CL models is the requirement that the unobserved effects be independently and identically distributed (IID) across the alternatives in the choice set, according to the extreme Type 1 distribution (Dahlberg and Eklöf, 2003). The IID assumption results in a rigid property of “independence from irrelevant alternatives” (IIA; Hoffman and Duncan, 1988; Ben-Akiva and Bierlaire, 1999). The IIA property assumes that the entire population has a homogeneous preference structure that restricts the β to be the same for all members of the population (Holmes and Adamowicz, 2003). Specifically, if εij for all i, then j is the probability that a given individual i chooses alternative j within the choice set Zn, which is given by
The IIA property is difficult to satisfy when only two alternatives are presented (Benson et al., 2016). The IIA requirement can be satisfied by allowing the unobserved part of the utility function to follow a multivariate normal distribution, letting the residuals correlate with each other, and estimating the model with a multinomial probit model (Dahlberg and Eklöf, 2003).
As another approach suggested in literature, the MIXL model (Hall et al., 2004; Hensher et al., 2005; Hole, 2007) can accommodate random taste variations as well as correlations in unobserved factors, which relaxes the IIA assumption (McFadden and Train, 2000; Campbell et al., 2006; Train, 2009). We use the MIXL model to calculate the utility derived by farmer i from choosing maize variety j on choice occasion t, as
where β is the vector of the mean attribute utility weights in the population, ri is the vector of person i's specific deviation from the mean. The random error term εijt is still assumed to be an independently and identically distributed extreme value. The ri can take several distributional forms, such as the multivariate normal of (0, ∑). As one of the variables used in the choice set is the seed price (cost) variable, we assume a log-normal distribution because it allows the price variable to take on a negative sign (Train, 2009; Fiebig et al., 2010). Assuming non-correlation in the attributes, the estimation model takes the form of
where Y is a binary decision variable that assumes a value of one if farmer i chooses variety j in choice scenario t, and zero otherwise; P denotes the price attribute; and A is a vector of other attributes, including yield and the tolerance to drought, the fall armyworm, low nitrogen, and striga. It is possible that the responses in Equation (that is Y) are correlated within households. In our case the MIXL estimation was clustered at household level, and the standard errors are specified as robust.1 For robustness checks, the standard errors are also clustered at village level. While the number of villages in our sample is not enough (16 villages), the results would still provide a good ground for comparison to identify any significant differences. A negative coefficient for γ implies that farmers generally prefer lower seed prices. A positive coefficient for δ implies that another variety of attributes positively influences the selection of a particular variety.
The core of the MIXL model is preference heterogeneity, which can be detected when a statistically significant standard deviation is estimated. Therefore, several interaction terms are included to capture these heterogeneities, as
where M represents a vector of socioeconomic characteristics. The estimates in Equations (7) and (8) can be used to calculate the willingness to pay (WTP) for the selected attributes. This is computed by obtaining the partial derivatives of price (P) relative to other attributes (A) and multiplying this value by −1 (Hole and Kolstad, 2012), as
We also calculate an alternative WTP measure with the yield coefficient instead of the coefficient for the price variable. To distinguish the two, the latter is considered the “willingness to sacrifice yield” (WTSY), and is calculated as
Note that we do not multiply by−1 because the yield coefficient is (intuitively) positive.
Data was collected using a structured choice experiment accompanied by a demographic and farm survey questionnaire. As previously stated, the experiments were conducted separately in each household, with both the household head and the spouse, or another adult household member who independently operated a maize plot. If the household had only one single adult head, with only minor children as the other members, or where only one adult operated a maize plot, then only one member—typically the household head—was interviewed.
A series of 18 focus group discussions (FGDs) were used to identify the key traits to use in the CE. These FGDs were meant to narrow down the key stress tolerance traits of concern, or those most likely to influence farmers' choices of maize varieties. Three FGDs occurred in each host village,2 and two of these meetings were exclusively men or women, with a third mixed; each FGD had 6–12 participants. The aim was to gather information for designing a parsimonious experiment that would not impose an undue cognitive load on the participants. We achieved this following the 18 FGDs by restricting the choices to the following six attributes, as listed in Table 1: seed prices; yield; and tolerance to the four stresses of drought, low nitrogen, striga, and fall armyworm.
Second, the FGDs were also meant to help us determine the reasonable ranges to use without creating choice options that were too complex. Therefore, we aimed to develop a choice experiment that was familiar in the local contexts, but that also used the most important choice criteria. The actual experimental sessions were conducted using the levels estimated from the FGDs; these were designed using the NGENE software suite (ChoiceMetrics, 2012) using a D-efficient design (Hall et al., 2002; ChoiceMetrics, 2012). To facilitate a recognition of the choices, 12 combinations (sessions) generated from NGENE were illustrated in color (Figure 2). The choice experiment was explained to respondents before each session, and the respondent was allowed to ask any clarifying questions. Twelve pages similar to Figure 2 were produced, with each trait combination derived from corresponding NGENE output. The respondent was shown one page at a time; the interviewer held the page with the picture facing the respondent and the interviewer read out the trait combinations. Each respondent was allowed ~30 to 60 s to review the pictures and make a choice. The choice experiment was implemented in the local Swahili language spoken in the research communities and by all the interviewers.
Figure 2. An example of choice experiment session (session 1) comparing traits for two maize varieties.
Table 2 summarizes the demographic data used in the econometric models. As two household members were interviewed, 50% of respondents were heads of household, 30% were spouses, and ~20% were other adult household members. Of the respondents, 48% were male, 42% were younger than 35, and 66% relied on primary agricultural production as the main source of income, or representing 50% or more of their annual income. The respondents' average age (42 years) was slightly less than that often observed among farmers (~50 years). The respondents had an average of 7.5 years of education, or a primary education, with primary school in Kenya involving eight years of education. Overall, youths (those under 35 years) were strongly represented in the maize production sector (42%), but these respondents heavily rely on agriculture. Their low levels of education—or no more than a primary school education on average—would suggest the low accumulation of skills, as one often requires a post-primary education to begin acquiring trade skills. Consequently, the respondents heavily rely on primary agriculture and other forms of low-skilled economic activities.
The upper panel in Table 3 displays the WTP for maize traits.3 In the pooled samples with or without interaction terms,4 the WTPs for the various traits were the same after including interaction effects. The WTP for FAW tolerance (FAT) was slightly higher in Siaya, which is possible if FAWs or similar insect infestations were more prevalent in Siaya. Generally, the WTP for FAT was 79% greater among respondents older than 35 compared to the under-35 cohort. The WTP for yield was the lowest of all traits. A noteworthy observation based on the data displayed in Table 3 is that the differences in the various sub-samples' WTP were not large. Table S1 in the Supplementary Materials notes 13 interaction terms, but only four are significant; this is consistent with weak heterogeneity in preferences and with the largely similar WTPs reported in Table 3.
Table 3. Mean willingness to pay (WTP) and willingness to sacrifice (WTSY) for maize variety attributes, by location and demographics.
The lower panel in Table 3 notes the WTSY estimates; the WTSY for lower seed prices is comparable to that for low N tolerance. In measuring WTSY, we observe that drought tolerance (DT) has the highest WTSY of all traits across locations and demographic categories. The second-highest WTSY was for striga tolerance (ST). Regarding DT, the WTSY increases from that for moderate to full DT. The same occurs for ST, where the WTSY for full ST is twice that of moderate ST. Regarding low N tolerance, we note that the WTSY for moderately low N tolerant is higher than the WTSY for fully low N tolerant. Across all the sub-samples in Table 3, the WTSY for full low N tolerance was an average of 26% smaller than the WTSY for Moderate N tolerance, with a difference of nearly 40% in the pooled sample with interactions.
The younger (under 35) demographic appears to have the highest WTSY for lower seed prices among all categories, mirroring the pooled sample result. Younger farmers may be just starting out and have fewer resources, and their yield considerations are not prioritized, as they lack the complimentary inputs to achieve the varieties' yield potential. As with WTP estimates, the WTSY estimates were fairly similar overall (by visual inspection of Table 3); most differences occurred between men and women, but appear to be generally small. The differences between WTP and WTSY estimates were most discernible when compared based on the geographic variation between Busia and Siaya. Specifically, the sub-sample from Siaya exhibited a higher WTP and WTSY than Busia for DT, FAT, and NUE. This suggests that geographic differences may be at play.
Farmers highly value stress-tolerant traits. Overall, the model estimates indicate that farmers prioritize all the stress-tolerant attributes, and may be willing to pay a premium in terms of higher seed price or sacrifice yield to gain crops with these attributes. The small coefficient estimates for yield compared to the other traits reflect not that yield is a trivial attribute, but that the high yield potential per se has reduced economic value in the presence of drought, striga and fall armyworm infestations, and low N (see Figure 3). It is conceivable that within this limit, lower-yielding varieties with these kinds of attributes may maintain market share as farmers may be willing to accept some yield penalty in favor of stress tolerance. Fortunately, modern breeding techniques have led to maize hybrids with these traits, but that also exhibit comparable or higher yields as legacy hybrids and having stress tolerance traits that the latter may lack.
Our results are broadly consistent with recently published literature. Kassie et al. (2017) found that farmers in Zimbabwe more highly value stress-tolerance traits—or drought tolerance, in their case—even more than such grain characteristics as closed tips, cob size, and flint texture. These authors also conclude that designing and implementing innovative ways of promoting drought-tolerant maize while raising awareness of these issues would enhance their adoption in risk-prone farming communities. Although Sibiya et al. (2013) examined rice crop in South Africa, they discovered that local rice landraces were preferred for their tolerance to abiotic stresses and stable yields.
Regarding impact of seed price on choice of variety, Sibiya et al. (2013) observed that the scarce use of hybrids was attributed to the high seed costs, and that these varieties are not tolerant to acidic, low-nitrogen soils (common issues in their study area in KwaZulu Natal). In our case, the WTSY for seed price was high and ranked after DT and ST (Figure 4). It is often argued that seed price may not be a major determinant of seed demand. However, our study and results by Sibiya et al. (2013) suggest that farmers may be critically sensitive to seed price. If a new variety lacks new traits and its main selling point is not in stress tolerance, farmers may choose more familiar yet lower-yielding varieties due to their less expensive retail price. However, in the local seed markets, there is little trait-based price differentiation. Our conclusion therefore would apply when more sophisticated seed markets emerge, as mimicked in our experiment.
While the local seed markets are particularly not driven by price competition (Rutsaert and Donovan, 2020), it is conceivable that a more nuanced approach to seed markets may be warranted, as seed prices could reflect unique varieties' combinations of attributes. In other words, seed markets should move toward attribute-based pricing. This will provide the chance for farmers to identify the seed product with the desired groups of attributes, which could act as a cornerstone of product differentiation and market segmentation.
Moreover, Kamara et al. (2006) examined geographic variations in preferences in Nigeria. Specifically, these authors observed that farmers in relatively market-driven production systems preferred high-yielding, drought-tolerant varieties, while those in resource-poor areas with low potential preferred earlier-maturing varieties to provide food security during periods of scarcity. Efisue et al. (2008) reported on rice; which although a different crop than maize, is still relevant when discussing farmers' trait preferences. These authors noted that while farmers in irrigated ecologies in Mali preferred high-yielding, long-duration rice varieties, those in the less favorable ecologies preferred tall plants with a short duration. This suggests that market segmentation could be based on geographic segmentation. One exception involves gender, as subtle differences have been observed that could influence marketing within a single geographic area.
Regarding gender preference heterogeneity, Anja et al. (2017) found gender-related differences in variety preferences. In most of the studies reviewed, men exhibited a preference for production- and marketing-related traits, while women focused on production- and use-related traits. However, when men and women faced similar constraints, they tended to mention similar trait preferences. As a general observation, women focused more on traits related to post-harvest processing and food preparation, such as storability, grain characteristics, losses during the decortication process, and the resulting flour's swelling capacity. Women also mentioned various food security-related traits, such as early maturity, the potential for multiple harvests, and production stability.
An apparent duality exists in women's roles in choosing maize varieties. The predominant cultural norms reveal that women are responsible for food processing and preparation, and this is often reflected in how they weigh grain qualities for example. However, women as farmers prefer the same attributes as male farmers. Finally, it is important to recognize that when women express strong preferences for specific consumption traits, those preferences are most likely those expressed by their households, in that this captures the preferences of the women, men and other members in the household. Will only consumption traits matter when targeting women farmers with information on new varieties? It is more likely that this will require a better understanding of who in the household chooses its varieties.
The second consideration will be whether the varieties concerned are primarily for subsistence, in that they are consumed at home, or whether they are intended for the market. Regarding the former, both consumption and stress-tolerance traits will have to be collectively considered in the household. Regarding the latter, the main issue may be stress tolerance and storability, and particularly if farmers store dry grain for some time before sale. As maize grain is often not graded on consumption traits, farmers may pay less attention to these issues if the varieties are primarily meant for market sale.
Maize cropping systems must respond accordingly to biotic and abiotic stressors exacerbated by climatic changes. One way to accomplish this is for maize improvement programs to develop and promote varieties that are simultaneously tolerant to multiple stressors. Consequently, this study examined farmers' valuation of maize traits and how they may prioritize them. We used a choice experiment approach similar to those more broadly used in product development research and applied a mixed-logit model to examine data from 1,400 smallholder farmers responding from two counties in the mid-altitude transitional and mid- to low-potential maize-growing regions in western Kenya. These areas are characterized by smallholder farmers operating low-capital farming enterprises dominated by subsistence concerns and limited commercialization. The results revealed that farmers were willing to pay significant premiums for seed tolerant to drought, striga, low nitrogen (nitrogen use efficiency), and fall armyworm infestation, in that order. The large-scale incorporation of these traits in both legacy and new varieties could enhance the maize system's resilience and adaptation.
In conclusion, the multi-criteria prioritization data presented here suggests that to mainstream climate-resilient, stress-adapted maize varieties in the country's maize production systems, drought- and striga-tolerant and nitrogen-efficient characteristics must be prioritized in the country's maize-breeding programs. Seed companies will more likely commercialize these stress-adapted varieties if in addition to stress tolerance traits, the preferred consumption traits are integrated into these new varieties, or vice versa.
These trait combinations can provide a basis for seed companies develop their seed businesses by replacing older varieties with those that are new and stress-adapted. In terms of segmentation, an approach that prioritizes geographic market partitioning may be required based on predominant stresses in specific areas. Without balancing these multiple dimensions, it is possible that new stress-adapted varieties may struggle to gain a significant market share. However, creating resilient, well-adapted maize systems will require the widespread commercialization of new generations of maize consistent with farmers' priorities.
The raw data supporting the conclusions of this article will be made available by the authors, without undue reservation.
The studies involving human participants were reviewed and approved by Institutional Research Ethics Committee, International Maize and Wheat Improvement Center. Written informed consent for participation was not required for this study in accordance with the national legislation and the institutional requirements.
PM conceived and designed the study and the experiments, run the surveys and experiments, analyzed and interpreted the data, and wrote the paper. RW analyzed data and wrote MIXL sections of the paper. SA implemented the surveys, reviewed survey instruments, and participated in data analysis and interpretation. VW participated in study design, supervised field implementation, interpreted the data, and wrote discussion sections of the paper. All authors contributed to the article and approved the submitted version.
We gratefully acknowledge funding from the Bill & Melinda Gates Foundation (Grants INV-003439 and OPP1134248) and the United States Agency for International Development (Grant MTO 069033) through the Accelerating Genetic Gains for Maize and Wheat and Stress Tolerant Maize for Africa Projects from under which the research activities were done. We gratefully acknowledge additional support from the CGIAR Research Programs Maize Agri-Food Systems (CRP MAIZE) and Policies, Institutions, and Markets (CRP PIM) for supporting the lead author PM.
The authors declare that the research was conducted in the absence of any commercial or financial relationships that could be construed as a potential conflict of interest.
All claims expressed in this article are solely those of the authors and do not necessarily represent those of their affiliated organizations, or those of the publisher, the editors and the reviewers. Any product that may be evaluated in this article, or claim that may be made by its manufacturer, is not guaranteed or endorsed by the publisher.
The Supplementary Material for this article can be found online at: https://www.frontiersin.org/articles/10.3389/fsufs.2022.702405/full#supplementary-material
1. ^The mixed logit (MIXL) which is meant to take care of heterogeneity uses a group variable that allows the model to be estimated by clustering at the household respondent and household level. We used the variable “group_id” which was generated using three variables household ID (household cluster), respondent type (respondent heterogeneity) and experiment session id (to capture any correlations between experimental sessions).
2. ^These were not the same villages in the final sample, but were located within the same wards.
3. ^The detailed MIXL results are presented in Tables S1–S3 in the Supplementary online Materials to reduce the amount of data presented in the body of the manuscript.
4. ^For each subsample, we ran two models, one with and the other without interaction (heterogeneity) terms.
Anja, C., Weltzien, E., Rattunde, F., and Ashby, J. A. (2017). “Gender differentiation of farmer preferences for varietal traits in crop improvement: evidence and issues,” in Working Paper No. 2. CGIAR Gender and Agriculture Research Network [Cali: CGIAR System Management Office and International Center for Tropical Agriculture (CIAT)].
Arisede, C., Mainassara, Z. A., Jill, C., Amsal, T., Cosmos, M., and Bish. (2020). Low-N stress tolerant maize hybrids have higher fertilizer N recovery efficiency and reduced N-dilution in the grain compared to susceptible hybrids under low N conditions. Plant Prod. Sci. 23, 417–426. doi: 10.1080/1343943X.2020.1746188
Badu-Apraku, B., Fakorede, M. A. B., Annor, B., and Talabi, A. O. (2018). Improvement in grain yield and low-nitrogen tolerance in maize cultivars of three eras. Exp. Agric. 54, 805–823.
Ben-Akiva, M., and Bierlaire, M. (1999). “Discrete choice methods and their applications to short term travel decisions,” in Handbook of Transportation Science (Boston, MA: Springer), 5–33.
Benson, A. R., Kumar, R., and Tomkins, A. (2016). “On the relevance of irrelevant alternatives,” in Proceedings of the 25th International Conference on World Wide Web (Montreal, QC: International World Wide Web Conferences Steering Committee), 963–973.
Berner, D., Carsky, R., Dashiell, K., Kling, J., and Manyong, V. (1996). A land management based approach to integrated Striga hermonthica control in sub-Saharan Africa. Outlook Agric. 25, 157–164.
CABI (2017). How to Identify Fall Armyworm. Plantwise. CABI. pp 4, Available online at: www.plantwise.org/fallarmyworm (accessed July 2nd, 2020).
Campbell, D., Hutchinson, W. G., and Scarpa, R. (2006). Using Discrete Choice Experiments to Derive Individual-Specific WTP Estimates for Landscape Improvements Under Agri-Environmental Schemes: Evidence From the Rural Environment Protection Scheme in Ireland. Working Paper No. 26.2006. FEEM (Fondazione Eni Enrico Mattei). doi: 10.2139/ssrn.884443
CIMMYT (2013). The Drought Tolerant Maize for Africa project. DTMA Brief. Available online at: https://www.cimmyt.org/news/a-decade-of-world-leading-maize-and-wheat-research/. (accessed July 02, 2020).
Dahlberg, M., and Eklöf, M. (2003). Relaxing the IIA Assumption in Locational Choice Models: A Comparison Between Conditional Logit, Mixed Logit, and Multinomial Probit Models. Uppsala: Nationalekonomiska institutionen.
Daryanto, S., Wang, L., and Jacinthe, P.-A. (2016). Global synthesis of drought effects on maize and wheat production. PLoS ONE 11, e0156362. doi: 10.1371/journal.pone.0156362
Day, R., Abrahams, P., Bateman, M., Beale, T., Clottey, V., Cock, M., et al. (2017). Fall armyworm: impacts and implications for Africa. Outlooks Pest Manag. 28, 196–201.
De Groote, H., Kimenju, S. C., Munyua, B., Palmas, S., Kassie, M., and Bruce, A. (2020). Spread and impact of fall armyworm (Spodoptera frugiperda JE Smith) in maize production areas of Kenya. Agriculture, Ecosystems and Environment. 292, 106804.
Dobermann, A., and Cassman, K. G. (2005). Cereal area and nitrogen use efficiency are drivers of future nitrogen fertilizer consumption. Sci. China Life Sci. 48, 745–758.
Drechsel, P., Kunze, D., and de Vries, F. P. (2001). Soil nutrient depletion and population growth in sub-Saharan Africa: a Malthusian nexus? Popul Environ. 22, 411–423. doi: 10.1023/A:1006701806772
Efisue, A., Tongoona, P., Derera, J., Langyintuo, A., Laing, M., and Ubi, B. (2008). Farmers' Perceptions on rice varieties in Sikasso region of Mali and their implications for rice breeding. J. Agron. Crop Sci. 194, 393–400. doi: 10.1111/j.1439-037X.2008.00324.x
Ejeta, G. (2007). “The Striga scourge in Africa: a growing pandemic,” in Integrating New Technologies for Striga Control: Towards Ending the Witch-Hunt, eds G. Ejeta, and J. Gressel (Singapore: World Scientific), 3–16.
Emede, T. O., and Alika, J. E. (2012). Variation in agronomic characters among high and low nitrogen S2 maize (Zea mays L) lines grown in high and low nitrogen environments. Maydica. 57, 139–146.
Fiebig, D. G., Keane, M. P., Louviere, J., and Wasi, N. (2010). The generalized multinomial logit model: Accounting for scale and coefficient heterogeneity. Mark. Sci. 29, 393–421.
Fisher, M., Abate, T., Lunduka, R. W., Asnake, W., Alemayehu, Y., and Madulu, R. B. (2015). Drought tolerant maize for farmer adaptation to drought in sub-Saharan Africa: determinants of adoption in eastern and southern Africa. Clim. Change 133, 283–299. doi: 10.1007/s10584-015-1459-2
Goergen, G., Kumar, P. L., Sankung, S. B., Togola, A., and Tamò, M. (2016). First report of outbreaks of the fall armyworm Spodoptera frugiperda (JE Smith)(Lepidoptera, Noctuidae), a new alien invasive pest in West and Central Africa. PloS one. 11, e0165632.
Gressel, J., Hanafi, A., Head, G., Marasas, W., Obilana, A. B., Ochanda, J., et al. (2004). Major heretofore intractable biotic constraints to African food security that may be amenable to novel biotechnological solutions. Crop Prot. 23, 661–689.
Hall, J., Kenny, P., King, M., Louviere, J., Viney, R., and Yeoh, A. (2002). Using stated preference discrete choice modelling to evaluate the introduction of varicella vaccination. Health Econ. 11, 457–465. doi: 10.1002/hec.694
Hall, J., Viney, R., Haas, M., and Louviere, J. (2004). Using stated preference discrete choice modeling to evaluate health care programs. J. Bus. Res. 57, 1026–1032. doi: 10.1016/S0148-2963(02)00352-1
Hensher, D. A., and Greene, W. H. (2003). The mixed logit model: the state of practice. Transportation 30, 133–176. doi: 10.1023/A:1022558715350
Hensher, D. A., Rose, J. M., and Greene, W. H. (2005). Applied Choice Analysis: A Primer. New York, NY: Cambridge University Press.
Hoffman, S. D., and Duncan, G. J. (1988). Multinomial and conditional logit discrete-choice models in demography. Demography 25, 415–427. doi: 10.2307/2061541
Hole, A. R.. (2007). Fitting mixed logit models by using maximum simulated likelihood. Stata J. 7, 388–401. doi: 10.1177/1536867X0700700306
Hole, A. R., and Kolstad, J. R. (2012). Mixed logit estimation of willingness to pay distributions: a comparison of models in preference and WTP space using data from a health-related choice experiment. Empir. Econ. 42, 445–469. doi: 10.1007/s00181-011-0500-1
Holmes, T. P., and Adamowicz, W. L. (2003). “Attribute-based methods,” in A Primer on Nonmarket Valuation (Dordrecht : Springer), 171–219.
Kamara, A., Kureh, I., Menkir, A., Kartung, P., Tarfa, B., and Amaza, P. (2006). Participatory onfarm evaluation of the performance of drought tolerant maize varieties in the Guinea Savannas of Nigeria. J. Food Agric. Environ. 4, 192–196.
Kanampiu, F., Makumbi, D., Mageto, E., Omanya, G., Waruingi, S., Musyoka, P., et al. (2018). Assessment of management options on striga infestation and maize grain yield in Kenya. Weed Sci. 66, 516–524. doi: 10.1017/wsc.2018.4
Kassie, G. T., Abdulai, A., Greene, W. H., Shiferaw, B., Abate, T., Tarekegne, A., et al. (2017). Modeling preference and willingness to pay for drought tolerance (DT) in maize in rural Zimbabwe. World Dev. 94, 465–477. doi: 10.1016/j.worlddev.2017.02.008
Khan, Z. R., Pickett, J. A., Wadhams, L. J., Hassanali, A., and Midega, C. A. (2006). Combined control of Striga hermonthica and stemborers by maize–Desmodium spp. intercrops. Crop Prot. 25, 989–995.
Kumela, T., Simiyu, J., Sisay, B., Likhayo, P., Mendesil, E., Gohole, L., et al. (2019). Farmers' knowledge, perceptions, and management practices of the new invasive pest, fall armyworm (Spodoptera frugiperda) in Ethiopia and Kenya. Int. J. Pest Manag. 65, 1–9.
Lagoke, S. T. O., Parkinson, V., and Agunbiade, R. M. (1991). “Parasitic weeds and control methods in Africa,” in Combating Striga in Africa: Proceedings of an International Workshop on Striga, ed S. K. Kim (Ibadan: IITA), 3–14.
Louviere, J. J., Flynn, T. N., and Carson, R. T. (2010). Discrete choice experiments are not conjoint analysis. J. Choice Modell. 3, 57–72. doi: 10.1016/S1755-5345(13)70014-9
McFadden, D.. (1973). Conditional Logit Analysis of Qualitative Choice Behavior. Berkeley, CA: Institute of Urban and Regional Development.
McFadden, D., and Train, K. (2000). Mixed MNL models for discrete response. J. Appl. Econometr. 15, 447–470. doi: 10.1002/1099-1255(200009/10)15:5<447::AID-JAE570>3.0.CO;2-1
Meemken, E. M., Veettil, P. C., and Qaim, M. (2017). Toward improving the design of sustainability standards—a gendered analysis of farmers' preferences. World Dev. 99, 285–298. doi: 10.1016/j.worlddev.2017.05.021
Ochieng, D. O., Veettil, P. C., and Qaim, M. (2017). Farmers' preferences for supermarket contracts in Kenya. Food Policy 68, 100–111. doi: 10.1016/j.foodpol.2017.01.008
Pasley, H. R., Camberato, J. J., Cairns, J. E., Zaman-Allah, M., Das, B., and Vyn, T. J. (2020). Nitrogen rate impacts on tropical maize nitrogen use efficiency and soil nitrogen depletion in eastern and southern Africa. Nutrient Cycl. Agroecosyst. 116, 1–12. doi: 10.1007/s10705-020-10049-x
Prasanna, B. M., Huesing, J. E., Eddy, R., and Peschke, V. M. (2018). Fall Armyworm in Africa: A Guide for Integrated Pest Management, 1st Edn. Mexico, CDMX: CIMMYT.
Rovere, R. L., Abdoulaye, T., Kostandini, G., Guo, Z., Mwangi, W., MacRobert, J., et al. (2014). Economic, production, and poverty impacts of investing in maize tolerant to drought in Africa: an ex-Ante assessment. J. Dev. Areas 48, 199–225. doi: 10.1353/jda.2014.0016
Rutsaert, P., and Donovan, J. (2020). Sticking with the old seed: input value chains and the challenges to deliver genetic gains to smallholder maize farmers. Outlook Agric. 49, 39–49.
Schipmann, C., and Qaim, M. (2011). Supply chain differentiation, contract agriculture, and farmers' marketing preferences: the case of sweet pepper in Thailand. Food Policy 36, 667–677. doi: 10.1016/j.foodpol.2011.07.004
Sheahan, M., and Barrett, C. B. (2017). Ten striking facts about agriculture input use in Sub-Saharan Africa. Food Policy 67, 12–25. doi: 10.1016/j.foodpol.2016.09.010
Sibiya, J., Tongoona, P., Derera, J., and Makanda, I. (2013). Farmers' desired traits and selection criteria for maize varieties and their implications for maize breeding: a case study from KwaZulu-Natal Province, South Africa. J. Agric. Rural Dev. Tropics Subtropics 114, 39–49. Available online at: http://nbn-resolving.de/urn:nbn:de:hebis:34-2013030542599
Smaling, E. M. A., Stoorvogel, J. J., and Windmeijer, P. N. (1993). Calculating soil nutrient balances in Africa at different scales. Fertil Res. 35, 237–250. doi: 10.1007/BF00750642
Veettil, P. C., Speelman, S., Frija, A., Buysse, J., and Van Huylenbroeck, G. (2011). Complementarity between water pricing, water rights and local water governance: a Bayesian analysis of choice behaviour of farmers in the Krishna river basin, India. Ecol. Econ. 70, 1756–1766. doi: 10.1016/j.ecolecon.2011.04.018
Keywords: Africa, climate change adaptation, climate-ready maize varieties, maize-genetic improvement, resilience, smallholder farming systems
Citation: Marenya PP, Wanyama R, Alemu S and Woyengo V (2022) Building Resilient Maize Production Systems With Stress-Adapted Varieties: Farmers' Priorities in Western Kenya. Front. Sustain. Food Syst. 6:702405. doi: 10.3389/fsufs.2022.702405
Received: 29 April 2021; Accepted: 05 May 2022;
Published: 15 June 2022.
Edited by:
Todd Andrew Crane, International Livestock Research Institute (ILRI), KenyaReviewed by:
Nathaniel Jensen, Independent Researcher, Morris, NY, United StatesCopyright © 2022 Marenya, Wanyama, Alemu and Woyengo. This is an open-access article distributed under the terms of the Creative Commons Attribution License (CC BY). The use, distribution or reproduction in other forums is permitted, provided the original author(s) and the copyright owner(s) are credited and that the original publication in this journal is cited, in accordance with accepted academic practice. No use, distribution or reproduction is permitted which does not comply with these terms.
*Correspondence: Paswel Phiri Marenya, cC5tYXJlbnlhQGNnaWFyLm9yZw==
Disclaimer: All claims expressed in this article are solely those of the authors and do not necessarily represent those of their affiliated organizations, or those of the publisher, the editors and the reviewers. Any product that may be evaluated in this article or claim that may be made by its manufacturer is not guaranteed or endorsed by the publisher.
Research integrity at Frontiers
Learn more about the work of our research integrity team to safeguard the quality of each article we publish.