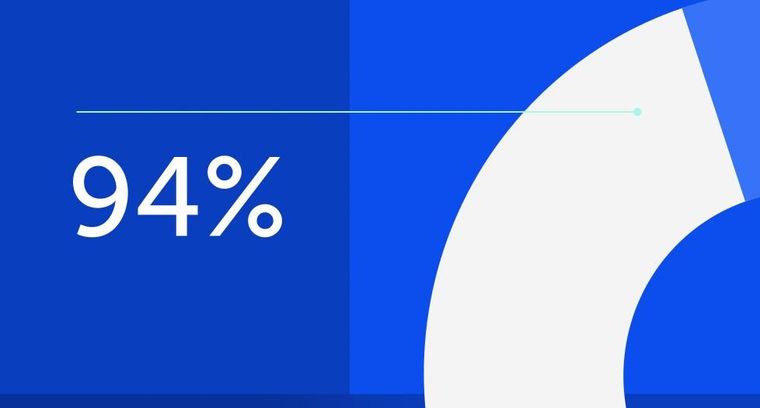
94% of researchers rate our articles as excellent or good
Learn more about the work of our research integrity team to safeguard the quality of each article we publish.
Find out more
PERSPECTIVE article
Front. Sustain. Food Syst., 18 January 2023
Sec. Land, Livelihoods and Food Security
Volume 6 - 2022 | https://doi.org/10.3389/fsufs.2022.1039962
This article is part of the Research TopicImproving Investment in Research and Innovation to Transform Agrifood Systems in the Global SouthView all 13 articles
Agricultural scientists are pursuing sustainable intensification strategies to increase global food availability, but integration from research to impact at the local-level requires knowledge of demographic and human-environment to enhance the adaptive capacity of farmers cultivating <10 ha. Enhancing close collaboration among transdisciplinary teams and these smallholders is critical to co-elaborate policy solutions to ongoing food security crises that are likely to be attuned with local conditions. Human and socio-cultural aspects need to be considered to facilitate both adoption and dissemination of adapted management practices. Despite this well-known need to co-produce knowledge in human systems, we demonstrate the inequality of current agricultural research in smallholder farming systems with heavy focus on a few domains of the sustainable intensification agricultural framework (SIAF), ultimately reducing the overall impact of interventions due to the lack compatibility with prevailing social contexts. Here we propose to integrate agriculture and agronomic models with social and demographic modeling approaches to increase agricultural productivity and food system resilience, while addressing persistent issues in food security. Researchers should consider the scale of interventions, ensure attention is paid to equality and political processes, explore local change interactions, and improve connection of agriculture with nutrition and health outcomes, via nutrition-sensitive agricultural investments.
Globally, agricultural production occurs at vastly different scales, from massive corporate or government owned industrial agricultural farms to small-scale farmers working to produce sufficient food for their families. These small-scale farmers cultivating <10 ha (referred to hereafter as smallholders), often have access to the fewest technologies and financial safety-nets but still produce close to 30% of the world's food (Ricciardi et al., 2018). Here we propose an enhanced, quantitative engagement of agronomists and agricultural development experts with health and social science communities to consider the needs, desires, and behaviors of individuals alongside strategies to increase yields and reduce human labor inputs to quantify context and barriers to agricultural adoption. By incentivizing researchers to extend agricultural technical and conceptual approaches (hereafter ag-approaches) developed in agriculture science to the impacts experienced by households and individuals, we can accelerate the transformation of the global food system (Pretty, 1997).
Farmers employ countless management strategies to hedge some of the risk inherent in areas dependent on variable rainfall or other weather hazards (Bhatta and Aggarwal, 2016; Sibhatu and Qaim, 2017). These strategies are often based on tradition or experience which may, or may not, align with existing scientific knowledge on how to adapt to rapidly changing conditions. Unanticipated and unmitigated climate risk is a primary driver of both short- and long-term food insecurity, as it reduces uptake of new agricultural technologies and can lead to negative livelihood impacts well after a period of climatic stress (Hansen et al., 2022).
There are many ways to develop specific policies that support the transformation of climate information into multi-layered agricultural programs, including anticipatory action and index-based insurance which supports governments and individuals in adopting new agricultural practices (Hansen et al., 2022). For example, the World Food Program's R4 Rural Resilience Initiative provides access to subsidized drought and flood risk insurance products which are triggered with a precipitation index. The program allows vulnerable households to access agricultural insurance while supporting community-led disaster risk reduction and landscape restoration activities (Spiegel and Satterthwaite, 2013). Connecting agricultural technologies to insurance, increased yield or other development goals requires rigorous modeling and assessments of social, economic and productivity outcomes. However, many of these programs offload assessment of performance of their program to models of their choosing, without rigorous insight into their quality, relevance, or connection to livelihood improvements (Saltelli et al., 2020). The failure of agricultural development programs may come from incomplete model selection, unfounded assumptions, lack of quality or quantity of relevant local data, or lack of insight into the context in which the implementation may occur.
Agronomists and agricultural development entities are doing vital work on developing new, high yielding varieties and agricultural management strategies to more sustainably increase agricultural productivity. These agricultural interventions are being developed with a focus on environmental sustainability (Rockström et al., 2017; Pretty et al., 2018; Peng et al., 2020; Pilling et al., 2020). Ag-approaches can be used to understand how transformation of heterogeneous smallholder systems into those that regularly and consistently produce marketable food and sustainable rural livelihoods can be achieved. Increased agricultural production through intensification needs to be balanced with environmental and social considerations (Hoffmann et al., 2015). Despite impressive technical advancements made in agronomy expertise, downstream impacts on people's lives are difficult to consistently achieve and document, especially in low-income settings (Di Prima et al., 2022). Only a few examples have been documented on communities that have been able to attain long-term behavioral change in smallholder farm management (Cui et al., 2018; Stevenson et al., 2019), despite decades of investment. This is worsened by the critical lack of ground data observations (Saltelli et al., 2020) of agroecological and socioeconomic heterogeneity of smallholders needed to tailor farming practices, as not all practices are universally beneficial (Stevenson et al., 2019).
Social considerations, such as attitudes, preferences, and behaviors of stakeholders in rural areas dominated by smallholder agriculture livelihoods need to be examined while researching sustainable agriculture interventions (Ban et al., 2013). Stakeholders within agriculture settings include not only farmers and their families, but also the community, retailers, input providers, wholesalers and consumers (Brown et al., 2022). Explicitly considering stakeholders during research and planning of sustainable agriculture interventions should allow any suggested interventions to be more realistic and inclusive, informing complex choices by farmers on which crops (Lemos and Morehouse, 2005), with what inputs and with how much investment (Hirsch et al., 2011).
Ag-approaches are integral to developing policy-relevant scenarios to understand the impact of interventions like climate services (Hansen et al., 2007), ag-tech tools (Oyinbo et al., 2020), or agricultural insurance (Osgood et al., 2018). Farm management components that include both tactical and operational decision-making (Fountas et al., 2006) represent key factors where interventions and investments can be planned. Nevertheless, as Siddique et al. (2012) points out, much of the knowledge derived from agronomic crop science has often resulted in reductionist approaches to agricultural management, where a single management factor is modified which results in yield or crop quality improvements. Multiple factors across diverse settings are rarely managed and modified together in ways that simulate the complexity of smallholder systems. If farmers are to benefit from agronomic crop science focused on sustainability, it must be integrated into an overall crop management process, accounting for interactions between factors and incorporation of human, social and economic constraints.
Integrated social and economic models focus on sustainably increasing productivity to produce more food per unit of land (Velten et al., 2015). Although there has been significant effort on sustainable intensification frameworks (Zurek et al., 2016), food security and nutrition (Fanzo et al., 2016), and socioeconomic factors driving food provisioning in smallholder systems (Ritzema et al., 2017), there currently isn't an integrating framework that brings the pieces together to address food security across diverse agroecosystems. This gap is particularly notable because increased yields may not actually result in increased consumption of protein and micronutrients in low-income populations (Firbank, 2012). Moreover, a singular focus on yields (or nutrition alone) can result in unintended consequences including increased environmental and/or social impacts in these deeply integrated socio ecological systems (Zurek et al., 2016). These issues may be intensified because climate variability and change has the potential to transform and degrade agricultural systems if not incorporated and planned for through a wide range of policy, economic, and social system levers (Hansen et al., 2022).
Musumba et al. (2017), proposed five domains (productivity, economic, environment, human condition, and social) to provide indicators for assessing the relative sustainability of an agricultural innovation. To develop innovations with a balanced approach across domains, research needs to be interdisciplinary, yet the lack of integration is conspicuous among Ag-innovations. Researchers working in sustainable development need to more strongly consider non-environmental aspects to agricultural intensification such as social issues, economics (Zurek et al., 2016) issues of equity, poverty alleviation, and gender empowerment (Loos et al., 2014). In the next two sections, we present a review of the literature and the results which provide insight as to how well the sustainable agriculture literature has been able to engage with all five domains. We then proceed to propose a more integrated system that may result in improved outcomes.
We conducted two literature reviews focused on representing the most influential knowledge about agricultural interventions. We identify the most cited 50 publications and the most relevant 50 papers of the last 5 years using the methodology of Nagendra et al. (2018). Our objectives were to synthesize the literature on interventions in smallholder farming systems, and to summarize the current state of knowledge and identify the different domains from the Sustainable intensification Assessment Framework (SIAF) (Musumba et al., 2017; Stewart et al., 2018).
A search was conducted on April 21st, 2022 in the Web of Science database using the keywords “Interventions,” “Innovations,” “Agriculture,” “Smallholders,” “Food Security,” “Sustainable Intensification,” and “Modeling”. The results were filtered according to the following criteria:
• Journal Citation Report from the top 50% (quartiles 1 and 2) to measure the probability that the article is influential.
• Article search results were filtered by “highly cited” as provided by the Web of Science, and then ordered in terms of relevance. Only the top 1,000 most highly cited papers were kept.
• From these papers, we then created two groups (1) most cited 50 papers most cited from the 1,000 subset, (2) last 5 years, first 50 papers by relevance from the last 5 years.
• Full-text screening of the 100 resulting papers was done to classify each into the five domains described by the Sustainable intensification Assessment Framework [The Five Domains | Sustainable Intensification Assessment Framework (SIIL) (sitoolkit.com)]. The Supplementary material provide the complete reference and number of citations in the Web of Science for each article.
To analyze the papers' contribution to interdisciplinary research on agriculture and nutrition, we evaluated the 100 papers on whether they included mention of the five domains of sustainable agriculture, as described below:
• Productivity, which focuses on intensification of agriculture by increasing the output per unit input per season or year;
• Economic, which focuses on issues directly related to the profitability of agricultural activities and return on investment;
• Environmental, which focuses on the natural resource base that supports agriculture, including soil, water, natural habitat, and the level of pollution of the surrounding ecosystem resulting from agriculture;
• Human, which pertains to the individual or household, including nutrition status, food security, and capacity to learn and adapt new ways of doing agriculture; and
• Social, which focuses on social interactions including inter-household and cross-social groups in a community or landscape, including the ability to manage conflicts related to agriculture and natural resource management (Musumba et al., 2017).
Figure 1 shows the lack of connection between research realms. There are significant connections between farming and human nutrition, and therefore human health but only a few approaches have tried to capture this interaction (Moberg et al., 2020; Di Prima et al., 2022). Furthermore, most of the publications that involved human domain focused mainly on nutrition, and especially on the calories consumed, disregarding the true complexity of utilization and access to a balanced diet with sufficient micronutrients and diversity (Lobell and Gourdji, 2012; Hasegawa et al., 2018). Lastly, most of the publications, when they included a social assessment, focused on economic concepts of market access and supply chains (Figure 1C; Horbach et al., 2012; Garrett et al., 2017; Ceballos et al., 2020). While Ag-economists focus on the cost/benefit and risk assessments associated with adopting new ag-methods, this kind of agriculture-oriented research is not well connected to broader social science and public health research (Griscom et al., 2017; Meemken et al., 2019; Adegbeye et al., 2020). In summary, research in this domain has neglected the real intricacy of the connections between biological systems and demographic aspects.
Figure 1. In a literature review capturing the 50 most highly cited and the 50 in the past 5 years, we show (A) the number of publications of each domain for the past 5 years (dark blue) and most cited (yellow); (B) the number of publications out of the 100 reviewed (y axis) plotted against the number of domains represented in each paper (x axis); and (C) the five different domains are plotted along a matrix, with the number of publications capturing multiple domains represented by the size and color of the symbol (yellow for most cited, black for publications from the past 5 years).
Researchers in social sciences and public health are considering the ways local food production impacts child health with a focus on expanding social science/health research in ways that consider the climate-food security linkage (Grace, 2017; Cooper et al., 2019). Anthropometric measurement (especially of children—e.g., stunting or wasting) allows for a quantitative assessment of an individual's health at the time of survey. From this information, researchers ascertain the level of undernourishment in a community and can use this information to spatially and temporally evaluate aspects of the food system that can be connected to biophysical data or agricultural models (Phalkey et al., 2015; Shively et al., 2015; Shively, 2017; Randell et al., 2021). Most studies that seek to connect environmental shocks and agriculture to human health use data (aggregated over space and time) on temperature, precipitation and vegetation anomalies, such as drought, heat waves and excessive rainfall (Brown et al., 2014; Randell and Gray, 2019; Sellers and Gray, 2019; Jain, 2020). Understanding how exposure to environmental shocks combined with the timing of exposure of individuals will help development programs to design appropriate interventions to protect human health and wellbeing (Grace et al., 2020; Hunter et al., 2021). This fairly general approach has been especially relevant in the context of climate change as scholars work to consider the linkage between the environment, food systems and health outcomes. However, the use of coarse environmental data fails to engage with the complexities and advancements in agronomic sciences and therefore misses an opportunity to advance cutting-edge science to support climate change adaptation.
A more robust alignment between agronomists and social science research communities is still needed to create more effective food system investments (Yaro, 2006). Here we propose enhanced, quantitative engagement that considers the needs, desires, and behaviors of individuals alongside strategies to increase yields and reduce human labor inputs to quantify context and barriers to agricultural adoption and connect them to food security outcomes. Therefore, we propose a new approach in agricultural, technical and conceptual research that links agriculture science to health, nutrition, and demographic impacts experienced by households and individuals. This can be achieved by encouraging researchers to Integrate process-oriented crop models with demographic and health models that can enable exploration of the impacts of specific interventions through attention to the biophysical and field management aspects affecting food production (Figure 2).
Figure 2. Traditional demographic approaches integrated with agronomic models. Inputs and outputs are represented by circles; models are represented by squares. Boxes within the models represent model components. Agronomic model components are a simplification of Keating et al. (2003). Demographic approaches components are a simplification of Grace (2017). Red boxes of crop management, labor, resources are all modeling inputs that are targets of direct investment by policy makers.
An integrated quantitative modeling framework that allows the use of information across all five domains in sustainable intensification will enable the identification of barriers, develop new insights and scenarios that could test the likely results of policy changes and intervention investments on food security and nutrition. Figure 2 shows how the community could use interdisciplinary engagement to accelerate planning for investments, highlighted in the models with red coloration. Primary outputs of crop models are estimates of crop yield and biomass at a certain site and year (Holzworth et al., 2018; Hoogenboom et al., 2019). From these, crop models can derive other outputs of interest to the food security community, including total calories, nutrient content, cost, and complexity of the agriculture system (Valin et al., 2014; Grafton et al., 2015). In this context, crop growth models arise as an effective tool to summarize how the biophysical environment affects a community, and focuses these results on human health information such as nutrition and health outcomes. By broadening the focus beyond process-based agronomic interventions, a more holistic approach can be promoted.
Integrated frameworks and models hold the promise to plan, implement and measure outcomes across a variety of contexts. Management factors in process-oriented Ag-Approaches are integral to developing policy-relevant scenarios to understand the impact of interventions and include crop and varietal choice, planting date, fertilizer and manure usage, weeding practices, field preparation, seeding rate, and sowing techniques (Cooper et al., 2009). For example, the impoverished women farming peanuts on undeveloped plots in urban Ouagadougou, Burkina Faso do not use the modern peanut varieties developed by local agronomists—rather, they rely on known and trusted approaches to grow peanuts as quickly and cheaply as possible to sell peanut butter in the market to meet their household budget (Juana et al., 2013). Ag-Approaches can be used to determine if an improved legume introduced to these women farmers will grow well under variable rainfall, high temperature or other weather scenarios, while acknowledging that the woman farmer will not always be able to plant on the idealized planting window. We could also determine if the new legume variety will still perform when the woman farmer loses access to other inputs due to macro considerations such as fuel prices, inflation or drastic changes in the input supply chain, or how it might be affected by changes in labor availability caused by a catastrophic health concern. The woman farmer's decision-making regarding legume choice involves productivity and flexibility simultaneously, among other concerns. If the crop model answers the first well, then investment might help with the second. Models could also show how productivity investments alone without simultaneous health and input investments will doom our woman farmer's cash crop.
Coupling agricultural process models with social and demographic models would enable improved exploration of how to transform low-input subsistence agricultural systems to achieve food security without replicating the unsustainable systems seen elsewhere (Schaller, 1993). This transition requires careful attention to the equity and political processes in the affected communities, the scope and potential of policy interventions, and the practices that result in nutrition and health outcomes. A more integrated modeling framework would allow for the use of modeling scenarios to evaluate potential outcomes and identify the unexpected outcomes that might emerge from biophysical or policy interventions.
Publicly available datasets were analyzed in this study. This data can be found here: https://doi.org/10.6084/m9.figshare.19763044.v1.
MB, KG, ME, AC, IC, and JN conceptualized and wrote the paper. AC and IC conceived and designed the analysis and collected the data. AC, IC, and ME performed the analysis. All authors contributed to the article and approved the submitted version.
This study was supported by the Feed the Future Innovation Lab for Collaborative Research on Sustainable Intensification (SIIL) at Kansas State University through funding United States Agency for International Development (USAID) under the Cooperative Agreement (Grant number AID-OAA-L-14-00006).
Contribution number 23-160-J from the Kansas Agricultural Experiment Station.
The authors declare that the research was conducted in the absence of any commercial or financial relationships that could be construed as a potential conflict of interest.
All claims expressed in this article are solely those of the authors and do not necessarily represent those of their affiliated organizations, or those of the publisher, the editors and the reviewers. Any product that may be evaluated in this article, or claim that may be made by its manufacturer, is not guaranteed or endorsed by the publisher.
The Supplementary Material for this article can be found online at: https://www.frontiersin.org/articles/10.3389/fsufs.2022.1039962/full#supplementary-material
Adegbeye, M. J., Ravi Kanth Reddy, P., Obaisi, A. I., Elghandour, M. M. M. Y., Oyebamiji, K. J., Salem, A. Z. M., et al. (2020). Sustainable agriculture options for production, greenhouse gasses and pollution alleviation, and nutrient recycling in emerging and transitional nations - An overview. J. Clean. Prod. 242, 118319. doi: 10.1016/j.jclepro.2019.118319
Ban, N. C., Mills, M., Tam, J., Hicks, C. C., Klain, S., Stoeckl, N., et al. (2013). A social-ecological approach to conservation planning: embedding social considerations. Front. Ecol. Environ. 11, 194–202. doi: 10.1890/110205
Bhatta, G. D., and Aggarwal, P. K. (2016). Coping with weather adversity and adaptation to climatic variability: a cross-country study of smallholder farmers in South Asia. Clim. Dev. 8, 145–157. doi: 10.1080/17565529.2015.1016883
Brown, M. E., Escobar, V., Younis, F. M., Carlo, E. S., McGroddy, M. E., Arias, S. D., et al. (2022). Scientist-stakeholder relationships drive carbon data product transfer effectiveness within NASA program. Environ. Res. Lett. 17, 095004. doi: 10.1088/1748-9326/ac87bf
Brown, M. E., Grace, K., Shively, G., Johnson, K., and Carroll, M. (2014). Using satellite remote sensing and household survey data to assess human health and nutrition response to environmental change. Popul. Environ. 36, 48–72. doi: 10.1007/s11111-013-0201-0
Ceballos, F., Kannan, S., and Kramer, B. (2020). Impacts of a national lockdown on smallholder farmers' income and food security: Empirical evidence from two states in India. World Dev. 136, 105069. doi: 10.1016/j.worlddev.2020.105069
Cooper, M. W., Brown, M. E., Hochrainer-Stigler, S., Pflug, G., McCallum, I., Fritz, S., et al. (2019). Mapping the effects of drought on child stunting. Proc. Natl. Acad. Sci. U. S. A. 116, 17219–17224. doi: 10.1073/pnas.1905228116
Cooper, P., Rao, K. P. C., Singh, P., Dimes, J., Traore, P. C. S., Rao, K., et al. (2009). Farming with current and future climate risk: advancing a Hypothesis of Hope'for rainfed agriculture in the semi-arid tropics. J. SAT Agric. Res. 7, 1–19.
Cui, Z., Zhang, H., Chen, X., Zhang, C., Ma, W., Huang, C., et al. (2018). Pursuing sustainable productivity with millions of smallholder farmers. Nature 555, 363–366. doi: 10.1038/nature25785
Di Prima, S., Wright, E. P., Sharma, I. K., Syurina, E., and Broerse, J. E. W. (2022). Implementation and scale-up of nutrition-sensitive agriculture in low- and middle-income countries: a systematic review of what works, what doesn't work and why. Global Food Security 32, 100595. doi: 10.1016/j.gfs.2021.100595
Fanzo, J., Arabi, B., and Haddad, L. (2016). Nutrition and Food Systems. High Level Panel of Experts on Food Security and Nutrition (HLPE). Available online at: https://www.fao.org/fileadmin/user_upload/hlpe/hlpe_documents/PT_Nutrition/Docs/HLPE_Nutrition_Project-Team_9_May_2016.pdf (accessed April 26, 2022).
Firbank, L. G. (2012). Commentary: pathways to global sustainable agriculture. Int. J. Agric. Sustainability 10, 1–4. doi: 10.1080/14735903.2012.621747
Fountas, S., Wulfsohn, D., Blackmore, B. S., Jacobsen, H. L., and Pedersen, S. M. (2006). A model of decision-making and information flows for information-intensive agriculture. Agric. Syst. 87, 192–210. doi: 10.1016/j.agsy.2004.12.003
Garrett, R. D., Gardner, T. A., Morello, T. F., Marchand, S., Barlow, J., de Blas, D. E., et al. (2017). Explaining the persistence of low income and environmentally degrading land uses in the Brazilian Amazon. Ecol. Soc. 22, 220327. doi: 10.5751/ES-09364-220327
Grace, K. (2017). Considering climate in studies of fertility and reproductive health in poor countries. Nat. Clim. Chang. 7, 479–485. doi: 10.1038/nclimate3318
Grace, K., Billingsley, S., and Van Riper, D. (2020). Building an interdisciplinary framework to advance conceptual and technical aspects of population-environment research focused on women's and children's health. Soc. Sci. Med. 250, 112857. doi: 10.1016/j.socscimed.2020.112857
Grafton, R. Q., Williams, J., and Jiang, Q. (2015). Food and water gaps to 2050: preliminary results from the global food and water system (GFWS) platform. Food Security 7, 209–220. doi: 10.1007/s12571-015-0439-8
Griscom, B. W., Adams, J., Ellis, P. W., Houghton, R. A., Lomax, G., Miteva, D. A., et al. (2017). Natural climate solutions. Proc. Natl. Acad. Sci. U. S. A. 114, 11645–11650. doi: 10.1073/pnas.1710465114
Hansen, J., List, G., Downs, S., Carr, E. R., Diro, R., Baethgen, W., et al. (2022). Impact pathways from climate services to SDG2 (“zero hunger”): A synthesis of evidence. Clim. Risk Manag. 35, 100399. doi: 10.1016/j.crm.2022.100399
Hansen, J. W., Baethgen, W. E., Osgood, D. E., Ceccato, P. N., and Ngugi, R. K. (2007). Innovations in Climate Risk Management: Protecting and Building Rural Livelihoods in a Variable and Changing Climate. Patancheru: SAT eJournal, ICRISAT.
Hasegawa, T., Fujimori, S., Havlík, P., Valin, H., Bodirsky, B. L., Doelman, J. C., et al. (2018). Risk of increased food insecurity under stringent global climate change mitigation policy. Nat. Clim. Chang. 8, 699–703. doi: 10.1038/s41558-018-0230-x
Hirsch, P. D., Adams, W. M., Brosius, J. P., Zia, A., Bariola, N., and Dammert, J. L. (2011). Acknowledging conservation trade-offs and embracing complexity. Conserv. Biol. 25, 259–264. doi: 10.1111/j.1523-1739.2010.01608.x
Hoffmann, H., Zhao, G., van Bussel, L. G. J., Enders, A., Specka, X., Sosa, C., et al. (2015). Variability of effects of spatial climate data aggregation on regional yield simulation by crop models. Clim. Res. 65, 53–69. doi: 10.3354/cr01326
Holzworth, D., Huth, N. I., Fainges, J., Brown, H., Zurcher, E., Cichota, R., et al. (2018). APSIM next generation: overcoming challenges in modernising a farming systems model. Environ. Model. Software 103, 43–51. doi: 10.1016/j.envsoft.2018.02.002
Hoogenboom, G., Porter, C. H., Boote, K. J., Shelia, V., Wilkens, P. W., Singh, U., et al. (2019). “The DSSAT crop modeling ecosystem,” in Advances in Crop Modeling for a Sustainable Agriculture, eds K. J. Boote (Cambridge: Burleigh Dodds Science Publishing), 173–216.
Horbach, J., Rammer, C., and Rennings, K. (2012). Determinants of eco-innovations by type of environmental impact—The role of regulatory push/pull, technology push and market pull. Ecol. Econ. 78, 112–122. doi: 10.1016/j.ecolecon.2012.04.005
Hunter, L. M., Koning, S., Fussell, E., King, B., Rishworth, A., Merdjanoff, A., et al. (2021). Scales and sensitivities in climate vulnerability, displacement, and health. Popul. Environ. 43, 61–81. doi: 10.1007/s11111-021-00377-7
Jain, M. (2020). The benefits and pitfalls of using satellite data for causal inference. Rev. Environ. Econ. Policy 14, 157–169. doi: 10.1093/reep/rez023
Juana, J., Kahaka, Z., and Okurut, F. (2013). Farmers' perceptions and adaptations to climate change in Sub-Sahara Africa: a synthesis of empirical studies and implications for public policy in African Agriculture. J. Agric. Sci. 5, 121. doi: 10.5539/jas.v5n4p121
Keating, B. A., Carberry, P. C., Hammer, G. L., Probert, M. E., Robertson, M. J., Holzworth, D., et al. (2003). An overview of APSIM, a model designed for farming systems simulation. Eur. J. Agron. 18, 267–288. doi: 10.1016/S1161-0301(02)00108-9
Lemos, M. C., and Morehouse, B. J. (2005). The co-production of science and policy in integrated climate assessments. Glob. Environ. Change 15, 57–68. doi: 10.1016/j.gloenvcha.2004.09.004
Lobell, D., and Gourdji, S. (2012). The influence of climate change on global crop productivity. Plant Physiol. 112, 208298. doi: 10.1104/pp.112.208298
Loos, J., Abson, D. J., Chappell, M. J., Hanspach, J., Mikulcak, F., Tichit, M., et al. (2014). Putting meaning back into “sustainable intensification.” Front. Ecol. Environ. 12, 130157. doi: 10.1890/130157
Meemken, E.-M., Sellare, J., Kouame, C. N., and Qaim, M. (2019). Effects of Fairtrade on the livelihoods of poor rural workers. Nat. Sustain. 2, 635–642. doi: 10.1038/s41893-019-0311-5
Moberg, E., Karlsson Potter, H., Wood, A., Hansson, P.-A., and Röös, E. (2020). Benchmarking the Swedish diet relative to global and national environmental targets—identification of indicator limitations and data gaps. Sustain. Sci. Pract. Policy 12, 1407. doi: 10.3390/su12041407
Musumba, M., Grabowski, P., Palm, C., and Snapp, S. (2017). Guide for the Sustainable Intensification Assessment Framework. Patancheru: SAT eJournal, ICRISAT.
Nagendra, H., Bai, X., Brondizio, E. S., and Lwasa, S. (2018). The urban south and the predicament of global sustainability. Nat. Sustain. 1, 341–349. doi: 10.1038/s41893-018-0101-5
Osgood, D., Powell, B., Diro, R., Farah, C., Enenkel, M., Brown, M., et al. (2018). Farmer perception, recollection, and remote sensing in weather index insurance: an Ethiopia case study. Remote Sensing 10, 1887. doi: 10.3390/rs10121887
Oyinbo, O., Chamberlin, J., and Maertens, M. (2020). Design of digital agricultural extension tools: perspectives from extension agents in Nigeria. J. Agric. Econ. 71, 798–815. doi: 10.1111/1477-9552.12371
Peng, B., Guan, K., Tang, J., Ainsworth, E. A., Asseng, S., Bernacchi, C. J., et al. (2020). Towards a multiscale crop modelling framework for climate change adaptation assessment. Nat. Plants 6, 338–348. doi: 10.1038/s41477-020-0625-3
Phalkey, R. K., Aranda-Jan, C., Marx, S., Höfle, B., and Sauerborn, R. (2015). Systematic review of current efforts to quantify the impacts of climate change on undernutrition. Proc. Natl. Acad. Sci. 112, E4522–E4529. doi: 10.1073/pnas.1409769112
Pilling, D., Bélanger, J., and Hoffmann, I. (2020). Declining biodiversity for food and agriculture needs urgent global action. Nat. Food 1, 144–147. doi: 10.1038/s43016-020-0040-y
Pretty, J., Benton, T. G., Bharucha, Z. P., Dicks, L. V., Flora, C. B., Godfray, H. C. J., et al. (2018). Global assessment of agricultural system redesign for sustainable intensification. Nat. Sustain. 1, 441–446. doi: 10.1038/s41893-018-0114-0
Pretty, J. N. (1997). The sustainable intensification of agriculture. Nat. Resour. Forum 21, 247–256. doi: 10.1111/j.1477-8947.1997.tb00699.x
Randell, H., Grace, K., and Bakhtsiyarava, M. (2021). Climatic conditions and infant care: implications for child nutrition in rural Ethiopia. Popul. Environ. 42, 524–552. doi: 10.1007/s11111-020-00373-3
Randell, H., and Gray, C. (2019). Climate change and educational attainment in the global tropics. Proc. Natl. Acad. Sci. 116, 8840–8845. doi: 10.1073/pnas.1817480116
Ricciardi, V., Ramankutty, N., Mehrabi, Z., Jarvis, L., and Chookolingo, B. (2018). How much of the world's food do smallholders produce? Global Food Security 17, 64–72. doi: 10.1016/j.gfs.2018.05.002
Ritzema, R. S., Frelat, R., Douxchamps, S., Silvestri, S., Rufino, M. C., Herrero, M., et al. (2017). Is production intensification likely to make farm households food-adequate? A simple food availability analysis across smallholder farming systems from East and West Africa. Food Security 9, 115–131. doi: 10.1007/s12571-016-0638-y
Rockström, J., Williams, J., Daily, G., Noble, A., Matthews, N., Gordon, L., et al. (2017). Sustainable intensification of agriculture for human prosperity and global sustainability. Ambio 46, 4–17. doi: 10.1007/s13280-016-0793-6
Saltelli, A., Bammer, G., Bruno, I., Charters, E., Di Fiore, M., Didier, E., et al. (2020). Five ways to ensure that models serve society: a manifesto. Nature 582, 482–484. doi: 10.1038/d41586-020-01812-9
Schaller, N. (1993). The concept of agricultural sustainability. Agric. Ecosyst. Environ. 46, 89–97. doi: 10.1016/0167-8809(93)90016-I
Sellers, S., and Gray, C. (2019). Climate shocks constrain human fertility in Indonesia. World Dev. 117, 357–369. doi: 10.1016/j.worlddev.2019.02.003
Shively, G., Sununtnasuk, C., and Brown, M. (2015). Environmental variability and child growth in Nepal. Health Place 35, 37–51. doi: 10.1016/j.healthplace.2015.06.008
Shively, G. E. (2017). Infrastructure mitigates the sensitivity of child growth to local agriculture and rainfall in Nepal and Uganda. Proc. Natl. Acad. Sci. U. S. A. 114, 903–908. doi: 10.1073/pnas.1524482114
Sibhatu, K. T., and Qaim, M. (2017). Rural food security, subsistence agriculture, and seasonality. PLoS ONE 12, e0186406. doi: 10.1371/journal.pone.0186406
Siddique, K. H. M., Johansen, C., Turner, N. C., Jeuffroy, M.-H., Hashem, A., Sakar, D., et al. (2012). Innovations in agronomy for food legumes. A review. Agron. Sustain. Dev. 32, 45–64. doi: 10.1007/s13593-011-0021-5
Spiegel, A., and Satterthwaite, D. (2013). The R4 Rural Resilience Initiative—Protecting Livelihoods in a Changing Climate. The Geneva Reports 65. Available online at: https://web.actuaries.ie/sites/default/files/erm-resources/ga_2013_geneva_report_7.pdf#page=73 (accessed June 6, 2022).
Stevenson, J., Vanlauwe, B., Macours, K., Johnson, N., Krishnan, L., Place, F., et al. (2019). Farmer adoption of plot- and farm-level natural resource management practices: between rhetoric and reality. Global Food Security 20, 101–104. doi: 10.1016/j.gfs.2019.01.003
Stewart, Z. P., Middendorf, B. J., Musumba, M., Grabowski, P., Palm, C., Snapp, S., et al. (2018). sitoolkit.com©. Feed the Future Innovation Lab for Collaborative Research on Sustainable Intensification, Kansas State University, Manhattan, KS, United States.
Valin, H., Sands, R. D., Van der Mensbrugghe, D., Nelson, G. C., Ahammad, H., Blanc, E., et al. (2014). The future of food demand: understanding differences in global economic models. Agric. Econ. 45, 51–67. doi: 10.1111/agec.12089
Velten, S., Leventon, J., Jager, N., and Newig, J. (2015). What is sustainable agriculture? A systematic review. Sustain. Sci. Pract. Policy 7, 7833–7865. doi: 10.3390/su7067833
Yaro, J. A. (2006). Is deagrarianisation real? A study of livelihood activities in rural northern Ghana. J. Mod. Afr. Stud. 44, 125–156. doi: 10.1017/S0022278X05001448
Keywords: integrated modeling, demography, sustainable agriculture, framework, agronomy
Citation: Brown ME, Carcedo AJP, Eggen M, Grace KL, Neff J and Ciampitti IA (2023) Integrated modeling framework for sustainable agricultural intensification. Front. Sustain. Food Syst. 6:1039962. doi: 10.3389/fsufs.2022.1039962
Received: 08 September 2022; Accepted: 28 December 2022;
Published: 18 January 2023.
Edited by:
P. V. Vara Prasad, Kansas State University, United StatesReviewed by:
Humnath Bhandari, International Rice Research Institute, BangladeshCopyright © 2023 Brown, Carcedo, Eggen, Grace, Neff and Ciampitti. This is an open-access article distributed under the terms of the Creative Commons Attribution License (CC BY). The use, distribution or reproduction in other forums is permitted, provided the original author(s) and the copyright owner(s) are credited and that the original publication in this journal is cited, in accordance with accepted academic practice. No use, distribution or reproduction is permitted which does not comply with these terms.
*Correspondence: Molly E. Brown, bWJyb3duNTJAdW1kLmVkdQ==; Ignacio A. Ciampitti,
Y2lhbXBpdHRpQGtzdS5lZHU=
Disclaimer: All claims expressed in this article are solely those of the authors and do not necessarily represent those of their affiliated organizations, or those of the publisher, the editors and the reviewers. Any product that may be evaluated in this article or claim that may be made by its manufacturer is not guaranteed or endorsed by the publisher.
Research integrity at Frontiers
Learn more about the work of our research integrity team to safeguard the quality of each article we publish.