- 1International Institute of Tropical Agriculture, Kigali, Rwanda
- 2Rwanda Agriculture and Animal Resources Development Board, Rubona, Rwanda
- 3Alliance of Bioversity International and CIAT, Geneva, Switzerland
- 4Alliance of Bioversity International and CIAT, Nairobi, Kenya
- 5Wageningen University, Wageningen, Netherlands
- 6VIAMO, Accra, Ghana
- 7Independent Researcher, Nairobi, Kenya
- 8VIAMO, Kigali, Rwanda
Diet quality is a critical determinant of human health and increasingly serves as a key indicator for food system sustainability. However, data on diets are limited, scattered, often project-dependent, and current data collection systems do not support high-frequency or consistent data flows. We piloted in Rwanda a data collection system, powered by the principles of citizen science, to acquire high frequency data on diets. The system was deployed through an unstructured supplementary service data platform, where respondents were invited to answer questions regarding their dietary intake. By combining micro-incentives with a normative nudge, 9,726 responses have been crowdsourced over 8 weeks of data collection. The cost per respondent was <$1 (system set-up, maintenance, and a small payment to respondents), with interactions taking <15 min. Exploratory analyses show that >70% of respondents consume tubers and starchy vegetables, leafy vegetables, fruits, legumes, and wholegrains. Women consumed better quality diets than male respondents, revealing a sex-based disparity in diet quality. Similarly, younger respondents (age ≤ 24 years) consumed the lowest quality diets, which may pose significant risks to their health and mental well-being. Middle-income Rwandans were identified to have consumed the highest quality diets. Long-term tracking of diet quality metrics could help flag populations and locations with high probabilities of nutrition insecurity, in turn guiding relevant interventions to mitigate associated health and social risks.
Introduction
The triple burden of malnutrition: undernourishment, micronutrient deficiencies, and over nutrition is a global challenge, with almost a billion people experiencing undernutrition and a further 2 billion currently overweight (Global Nutrition Report Independent Expert Group, 2020). Malnutrition has been linked to a multitude of factors, including socio-economic (Reinhardt and Fanzo, 2014), with undernutrition often associated with lower and middle-income countries and overnutrition with high income countries. However, this spectrum is increasingly blurred, with the different forms of malnutrition now observable in the same country, household, and even in the same person (Doak et al., 2000, 2005; Global Nutrition Report Independent Expert Group, 2018).
In low-income countries, consumption of healthy foods is in decline, with simultaneous increases in unhealthy foods (Imamura et al., 2015). In lower-middle income countries (LMICs), increased consumption of both healthy and unhealthy foods has been observed (Imamura et al., 2015). These dynamic patterns are in many cases the manifestation of nutrition transitions, where wealthier and more urban individuals shift towards consumption of processed, sweetened, and salted foods (Popkin, 2015). In Africa, younger, increasingly urban, and wealthier populations have experienced major nutrition transitions over recent decades (Vorster et al., 2011; Steyn and McHiza, 2014; Kinyonki et al., 2020). In Rwanda, for example, 33% of the population remains undernourished (National Institute of Statistics of Rwanda and ICF, 2020), with nutritionally inadequate diets widely prevalent (Marivoet et al., 2020; Arsenault and Olney, 2021). Like many other LMICs however, nutrition transitions are evident in Rwanda, with people moving away from traditional plant-based diets of leafy vegetables, fruits, legumes, and grains. A consequence of such a transition is that the population of overweight children is larger than that suffering wasting (FAO, 2018; World Bank, 2019; Kinyonki et al., 2020).
Global malnutrition and dietary transitions reinforce the need to promote innovative and nutrition-focused food systems (Gómez et al., 2013). To do so, Popkin et al. (2020) argue that new ways of designing and targeting nutrition programmes and policies are needed. These programmes would require concerted efforts to improve the availability, access, frequency, and quality of data on diets. Unfortunately, government and non-government agencies in LMICs generally do not have data collection systems needed for concerted high frequency data collection (Tuffrey and Hall, 2016). When available, data on diets are from small, fragmented, and temporally static samples and globally modelled data diverge dependent on the source and models used and are therefore limited in terms of actionability for program design (Beal et al., 2021).
To address this, innovative systems and tools for dietary data collection are required that provide basic functionality to engage users, whilst having a back-end that can aggregate diet data and generate insights about dietary patterns across groups. Although diet quality tracking software are widely available for smartphone users (e.g., Ferrara et al., 2019). Unfortunately, similar applications are limited for simple phone (non-smartphone) users, which dominate mobile phone ownership outside of advanced economies (Pew Research Center, 2019). Despite this, contextually sensitive technologies and methods exist (e.g., unstructured supplementary service data, interactive voice response, and computer assisted telephone interviews) to generate a sustained and high-frequency data flow from crowd-based data collection systems (Gibson et al., 2017; Lamanna et al., 2019). The harmonisation of these data collection systems with rapid and globally validated diet quality assessment tools (e.g., Herforth et al., 2020a,b), could provide an opportunity for developing and testing a system for high-frequency diet tracking and rapid diet quality assessment. Construction and testing of a crowd-based system constitutes an indispensable and promising step towards robust data-driven policies and interventions for food and nutrition security (e.g., Wells et al., 2019).
We aim to develop a generic and widely applicable data collection system that leverages on the application of citizen science and digital tools for high frequency collection of diet data. Specifically, we look to provide learning from the development, testing, and application of this system in Rwanda, whilst providing provisional results on the trends in diet quality across Rwandan society. The goal of this system being to generate a robust system that is easily adapted to national dietary and technological conditions to generate insights on diet quality to support evidenced-based policy making and interventions.
Methods
Study Area
The piloting of this system was conducted in Rwanda (Figure 1), a Central African country of around 13 million people. The Rwandan population is largely below 40 years old (National Institute of Statistics Rwanda, 2021). In 2018, at least 17% of the population experienced moderate or severe food insecurity (National Institute of Statistics Rwanda, 2021). In 2016, 56% of the population was living below the international poverty line (National Institute of Statistics Rwanda, 2021), with median household incomes of $2.57 (World Bank, 2021a). Mobile phone penetration is high, with around 76 mobile phone subscriptions per 100 inhabitants (Rwanda Utilities Regulatory Authority, 2020), with 3G mobile network being accessible to 94% of the population (Rwanda Utilities Regulatory Authority, 2019).
Diet Quality Questionnaire
To generate insights on diet quality across Rwanda, we applied the Diet Quality Questionnaire (DQ-Q) (Herforth et al., 2020a). The DQ-Q is already being applied globally, with the Gallup Polling organisation including it in diet module of their global polling (Herforth et al., 2020b).
The DQ-Q captures consumption information for 26 food groups (including both healthy and unhealthy food items) by asking 29 binary questions. The DQ-Q requires respondents to answer either “yes” or “no” to whether they consumed, during the past 24 h, example food items presented in the corresponding question. For more information on the DQ-Q and its global application, please visit: https://www.globaldietquality.org/.
To make the DQ-Q contextually relevant to Rwanda, it was adapted to national dietary conditions through identification of recognisable and widely available food items for each food group. To do this, we collaborated with the developers of DQ-Q and local governmental authorities. The DQ-Q was translated to Kinyarwanda (Rwanda's official language). The full questionnaire (in English and Kinyarwanda) is available in Supplementary Material 1.
From the outputs of the DQ-Q, 12 indicators can be generated. The indicators reflect global dietary recommendations from international authorities (Table 1). These indicators were validated by Herforth et al. (2020a) against equivalent quantitative amounts to align with these global dietary recommendations. The outputs of DQ-Q can also be aggregated into three summary indicators (Global Dietary Recommendation score, Global Dietary Recommendation—Healthy score, and Global Dietary Recommendation—Limit score) which are constructed through the presence of relevant food groups (Table 1). For further information on the construction and validation of the indicators see Herforth et al. (2020a).
Platform Design
We deployed the DQ-Q collection system using unstructured supplementary service data (USSD). USSD is a free, text-based protocol that allows for two-way exchanges of information (up to 140 characters in Rwanda). The text-based nature of USSD offers flexibility for deployment on both feature and smartphones and deployment in (almost) any country with mobile phone coverage. An example of the USSD-based DQ-Q is shown in Figure 2. The system was developed and maintained by the social enterprise VIAMO (www.viamo.io), who have extensive background in developing mobile phone-based systems in LMICs, including Rwanda.
Sampling
Potential respondents for the DQ-Q were sourced from a toll-free information service database (*845#), maintained by VIAMO in collaboration with MTN Rwanda (a telecommunications company). The information service has been live for more than 4 years and provides information on agriculture, health, finance, legal aid, nutrition, youth, news, and weather. It can be accessed by all MTN Rwanda customers who have 8 free calls to the service each month.
The 845-service database contains prospective respondents' mobile number, age, and sex. This information was collected during a voluntary registration process for the service, not related to this project. The information for more than 1.2 million verified individuals (~9% of the Rwandan population) are held in the database, with around 80% consenting to be contacted for further information or to participate in surveys. Respondents from all districts (sub-national level—administrative level 2) of Rwanda are present in the database.
To track spatial and temporal trends of diet quality, an initial schedule of 28 weekly data collections was developed starting in early August 2021. Data will be collected until February 2022. A representative sample of ~2,500 respondents for each weekly data collection was calculated (confidence interval of 1.96 and confidence limits of 95%). However, due to budget limitations, this was reduced to 1,800 individuals. Our experience of similar USSD-based surveys (e.g., Adewopo et al., 2021) has shown that most contacted individuals will not perform the survey, with response rates around 20% (Solano-Hermosilla et al., 2020). To address this and secure a representative sample, per data collection moment, 10,000 individuals were contacted in each weekly data collection moment.
To ensure sex-balanced responses, a 50-50 split in responses was programmed. This meant that once 50% of the required respondents were received to be male or female, no further submissions from that sex group could be submitted. To ensure spatially distributed and representative responses, we implemented a population-based weighting of respondents. To do this, the DQ-Q was sent proportionally to individuals in each district, based upon the population of that district, relative to the national. Once the required number of responses from each district was submitted, no further submissions from that district were accepted.
The contacted cohort of 10,000 individuals was randomly selected per week, considering the following requirements: (i) respondents provided consent to be contacted; and (ii) respondents provided information on their sex and district when consenting to be contacted by the 845 service. Although there is the possibility that potential respondents may be shortlisted multiple times for the sample cohort, we do not track the same individuals intentionally. In this pilot, we are only looking to gain insights on diet quality metrics at an administrative aggregate level. Future iterations could easily track diet quality for the same individuals across time.
Submissions
The sample cohort of potential respondents were sent a preliminary information message via Short Message Service (SMS). This message informs the cohort to respond if they would like to perform the DQ-Q survey. Participants then have 7 days to respond to the questionnaire, with data collection opening on Monday morning and closing on Sunday evening. Reminder messages are sent daily to those participants who respond positively to the initial message, but do not complete the survey. Respondents can drop-in and out of the survey with their responses saved. Before submitting, respondents are required to provide their sector (sub district administrative unit) and their economic group. The Rwandan government implements a social stratification of households, classifying across five categories (A-E), based upon income and asset ownership (Table 2).
A submission was only finalised when respondents answered all questions. Once the required number of respondents was achieved in each location and for each sex, submission of the questionnaire was no longer available. However, because USSD allows concurrent submissions, there is the possibility of exceeding the required sample—usually between 1 and 10 as the system will only close for new respondents, respondents already in the process of responding can continue till the end.
Data collection started on the 9th of August 2021 (Week 1), with results for the first 8 weeks (27th September 2021—Week 8) presented. Data collection continues until early February 2022.
Micro-Reward Incentives
To encourage engagement, we provided meagre, yet non-promissory, reward incentives in the form of airtime. Each respondent who fully completed the questionnaire received 250RWF (~0.25 USD) of airtime, sent via a digital and traceable payment platform. This incentive was sent en masse to all completed respondents on the Monday following their submission.
Data Processing and Analysis
An automated process of data curation, metric calculation, and visualisation was implemented using R (R Core Team, 2021).
Ethical and Institutional Approval
Approval for the data collection was received from the Rwanda National Ethics Committee (740/RNEC/2021) and the National Institute of Statistics of Rwanda (2058/2021/10/NISR), based on extensive review of the substance and merits of the innovation to support initiatives and policy-action.
Results
Data Flows
The data collection system went live in early August 2021, with 8 weekly data collection moments so far completed. Within this period, 80,000 Rwandans were contacted, with 12,821 unique interactions recorded with the system (Figure 3A). The interaction rate fluctuated between 17 and 18%, excluding the early weeks of the project (Figure 3B). The completion rate, where interactions are submitted to the system, fluctuated between 60 and 90% (Figure 3D) with 9,726 submissions collected (Figure 3C). Completion of the survey took around 13 min (Figure 3E), with the most active day for submissions being the day of the first SMS message sent per data collection moment- Monday (Figure 3F). Subsequent daily reminders to respondents yielded diminishing returns of responses.
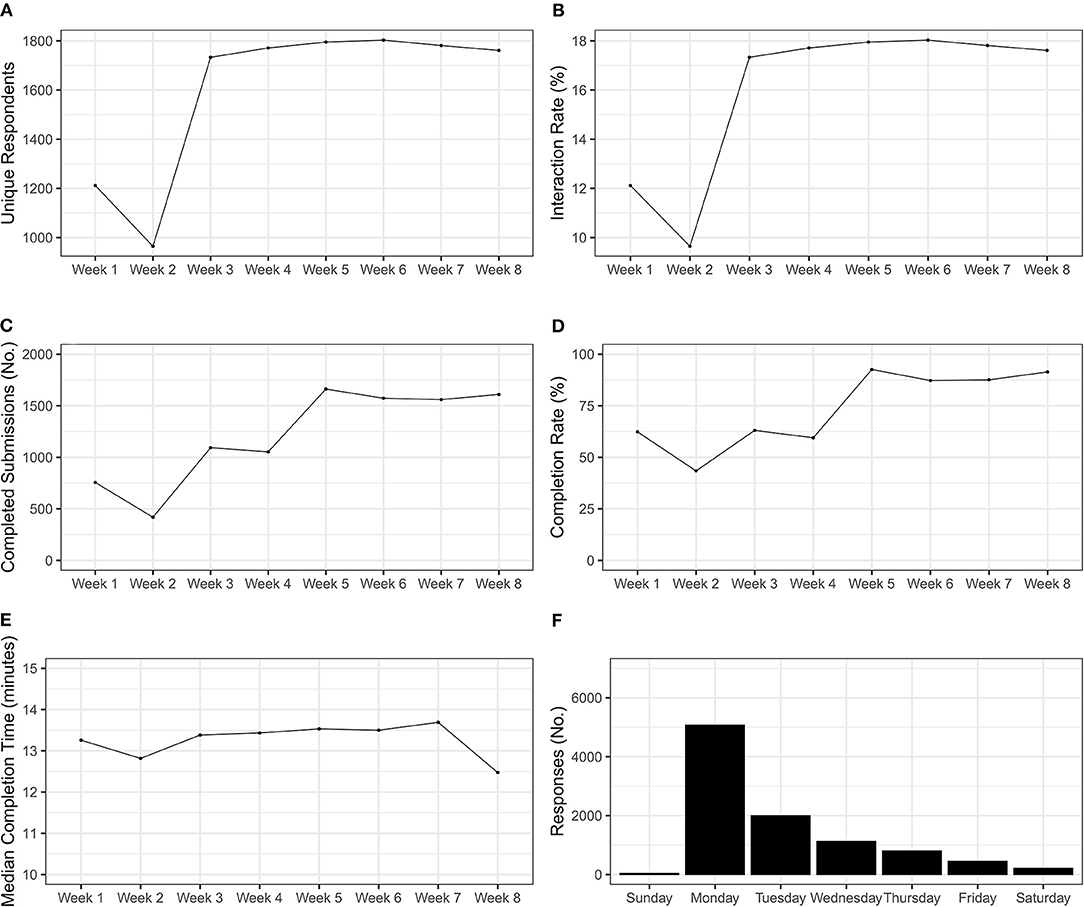
Figure 3. Digital survey insights. (A) Total number of respondents per week of data collection. (B) Percentage of respondents who interacted with the survey following initial push messages. (C) Total number of completed submissions per week of data collection. (D) Percentage of respondents who completed the survey after starting an interaction. (E) Average time (in minutes) taken by respondents to complete the survey. (F) Number of unique responses per day across all data collection weeks.
Through preliminary data curation and analyses, we noted that a significant minority of respondents were trying to “game” the system, where individuals answer yes to all questions. We found that around 100 individuals each week were reporting in this manner. Although individuals may eat food items from 26 food groups, we assumed this unlikely and that these respondents were responding yes to complete the survey rapidly and receive the payment. To address this, we added a warning at the start of the survey announcing abnormal responses would not be accepted.
Respondent Distribution
Basic respondent information is provided in Table 3. The collected data shows a slight female bias, females (50.22%) and males (49.78%). The distribution of respondents' ages was found to be skewed towards younger individuals, with ~70% (6,773) under the age of 24, with only 6% (581) over 44. The economic grouping of respondents shows a normal distribution, with 85% of respondents coming from the middle-income groupings. The poorest economic grouping only represents 3% of responses.
Common Foods Items
In Figure 4, the presence of food groups in respondents' diets are displayed. The results suggest a core set of food groups: other vegetables (e.g., tomato, cabbage, and aubergine), tubers and starchy vegetables (e.g., potato, cassava, and plantain), dark green leafy vegetables (e.g., green amaranth, cassava leaves, and pumpkin leaves), other fruits (e.g., banana, watermelon, and pineapple), and legumes (e.g., beans and peas). These ubiquitous food groups were consumed by at least 70% of respondents. Invariably, these groups co-occurred in respondents' diets too (Supplementary Figure S1).
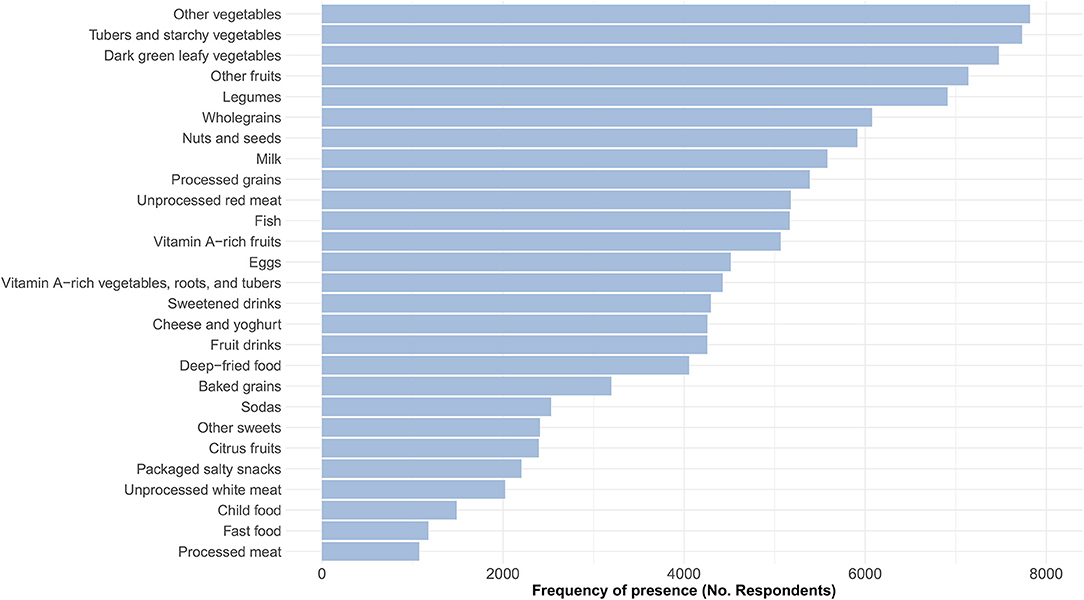
Figure 4. Frequency of presence of food groups in Rwandan respondents' diets. Food groups are derived from the Diet Quality Questionnaire.
Diet Quality Metrics
To reduce the length of the Results section, we present only the results for the summary indicators from Table 1: Global Dietary Recommendation Score (GDR); Global Dietary Recommendation Healthy Score (GDR-Healthy); and Global Dietary Recommendation Limit Score (GDR-Limit). The results for the other 9 indicators (e.g., fruits and vegetables, dietary fibre, free sugars, and saturated) are presented in Supplementary Figures S2–S4. We disaggregate the results for the three focus diet quality metrics across sex, age, economic group (Table 3, Figure 5), and location (Figure 6). The preliminary results demonstrate that Rwandan female respondents have marginally better GDR scores (3.19), compared to their male counterparts (3.05) (Table 3, Figure 5A). Interestingly, Rwandan women were noted to have higher GDR-healthy (Figure 5B) and GDR-Limit (Figure 5C) scores. Deriving temporal trends from such a short time series is difficult, but nascent temporal fluctuations in both the healthy and limit metrics are apparent, with both peaking between Week 5-6 (mid-September 2021).
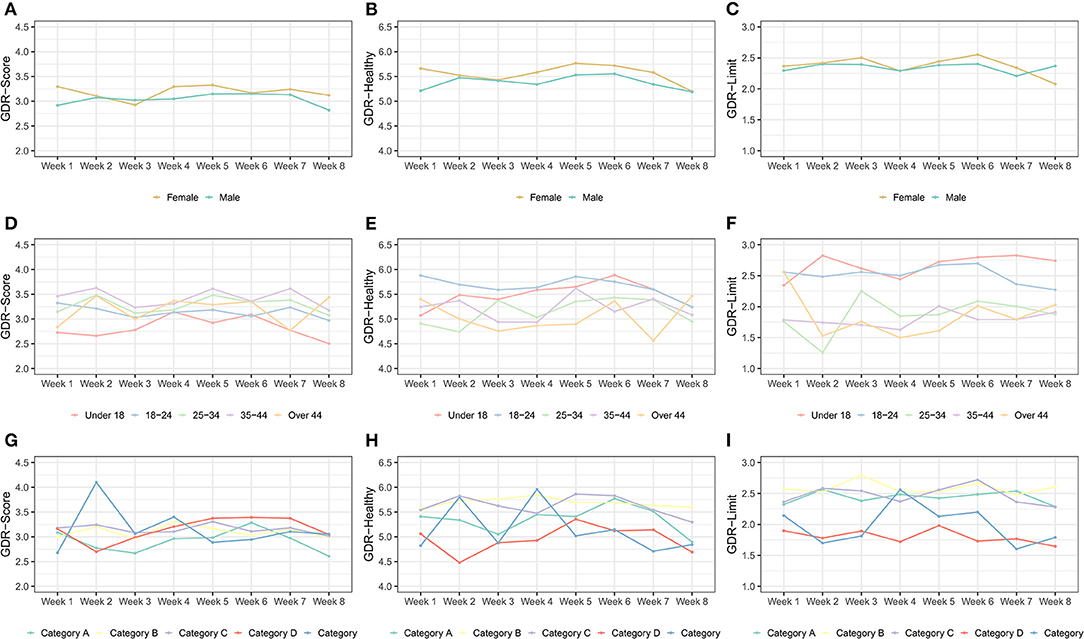
Figure 5. Temporal patterns of diet quality across sex, age, and economic groups. (A) GDR Score across sexes. (B) GDR-Healthy Score across sexes. (C) GDR-Limit Score across sexes. (D) GDR Score across age groups. (E) GDR-Healthy Score across age groups. (F) GDR-Limit Score across age groups. (G) GDR Score across economic categories. (H) GDR-Healthy Score across economic categories. (I) GDR-Limit Score across economic categories.
We observed that younger respondents (<24) had the lowest GDR scores of all respondents (2.84 and 3.12—Table 3). As respondents get older their diet quality (GDR score) improves, with some weekly exceptions (Figure 5D). The diet quality of the younger respondents was limited by their high consumption of food items that should be limited (2.65 and 2.51; Table 3), far higher than other age groups who had GDR-Limit scores of <2 (Figure 5F). In contrast, elder groups were observed to have consistently lower GDR-Healthy scores (Figure 5E), with the eldest age group having average GDR-Healthy scores of 5.06, notably lower than the youngest group (5.50; Table 3).
The relationship between economic category and diet quality highlights that individuals in the extreme categories are those with the lowest diet quality (Table 3). Individuals from the wealthiest category had especially low diet quality with a GDR score of 2.92, with individuals from the poorest category only having marginally better scores of 3.04. This pattern holds largely true throughout the data collection (Figure 5G). These GDR scores can be explained by wealthier individuals eating moderate levels of healthier foods (Figure 5H), but some of the highest amounts of unhealthy foods (Figure 5I). Individuals with the best diet quality were found in category D, with an average GDR Score of 3.22 (Table 3).
In Figure 6, we map the mean GDR, GDR-Healthy, and GDR-Limit scores by district. These maps reflect the scores for all respondents from within each district. We found that the urban districts with the highest population densities (see Figure 1) are associated with the lowest GDR Score. Respondents in these locations also had the highest GDR-Healthy and GDR-Limit scores. Rural, less densely populated areas were found to have notably lower healthy and limit scores, for example in northeastern and southern Rwanda.
Discussion
Understanding diet quality across socio-economic groupings, location, and time is critical to guide policy decisions and nutrition interventions to support health outcomes. Popkin et al. (2020) suggest that this requires innovative systems and tools to generate insights about diet quality across domains. We have developed and tested a data collection system that crowdsources diet quality information from across Rwanda. Leveraging digital tools, we have directly engaged across socio-economic strata, age groups, and location for equitable representation of Rwandan diet quality realities in near real-time. The tool and approach reinforce the utility and benefits of digital data collection and crowd engagement and could be easily adapted to other national contexts.
Provisional Learning From a Crowd-Based Data Collection System
Developing this novel data collection system reinforced both the benefits of digital data collection and, consistent with other researchers, its shortfalls (e.g., Trucano, 2014; Lamanna et al., 2019). One of the greatest benefits is that digital data collection can be highly cost-efficient (Zeug et al., 2017; Adewopo et al., 2021). Preliminary estimates place our cost per respondent at around $1, with this cost largely associated with the system set-up and maintenance, with the incentive payment making up a small portion of the cost. There remains considerable scope for further cost-efficiencies as the system matures and automates, reducing the cost of each data collection moment. In contrast, an identical, but in-person application of the survey in Rwanda had a per respondent cost of $33 (staff costs, travel, car rental, and fuel). Further, the digital survey was more efficient in terms of time per response, taking around 15 min, compared to almost an hour for in-person application.
As noted in the results, we identified a small (<100/week), but not insignificant, cohort of respondents who were suspected of taking advantage of the simplicity of the system to receive the incentive by providing a convenient response of “yes” to all questions. These respondents were excluded from further consideration in the data analyses. Similar instances of suspected gaming/hacking have been reported in other crowd-based data collection efforts (Solano-Hermosilla et al., 2020). To mitigate this challenge, warning messages were sent to respondents whose submissions were flagged as suspicious. Based on the consideration that it is unrealistic that a person would have consumed 80% (or more) of the food classes within a 24-hr period, submissions that showed response of “yes” to more than 80% of questions were excluded. This brings one of the major concerns about digital data collection into focus, the trustworthiness of the data. This is a subject of ongoing discourse in recent literature (Adewopo et al., 2021). Crowdsourced data can be susceptible to errors and bias due to absence of direct control or verification of each datapoint at the time and location of submission. This may be linked to various factors including the clarity of questions or data required and personality/profile of the respondents (including candour, educational level, perception, age, and mental retention/recall). Yet, the advantages of crowdsourced data systems usually outweigh these limitations, especially considering the volume of data that are often generated, with little or no direct contact with respondents. According to Solano-Hermosilla et al. (2020), the robustness of acquired data provides strong leverage for high-precision data filtering which allows noisy errors to be filtered out prior to detailed data analysis, and to generate meaningful insights.
We also experienced lower response rates (18%), compared to previous similar data collection data where the response rate (or crowd engagement) hovered around 20% (Solano-Hermosilla et al., 2020). In this initial set-up and deployment, it is uncertain if the initial messaging to respondents could have significantly influenced the response rate or participation. One way to address this in future iterations would be to implement A/B testing, where sub-groups are provided different information (e.g., opening messages) or offered different incentives for completion. Previous examples of this testing mechanism have yielded results and improved the targeting and efficiencies of projects (e.g., Katmada et al., 2011; Arriagada et al., 2021).
Another issue is the representativeness of the data collected. Although sex and location-based weighting was applied to the sampling, our results are largely skewed towards younger, middle-income respondents. Although Rwandan society (and the 845# dataset) is dominated by individuals under the age of 40 (National Institute of Statistics Rwanda, 2021), our respondents appear to be an extreme reflection of the younger population in Rwanda. The demographics of Rwandan society are not truly reflected in our results, where more than 84% of respondents are below 34. The age-based skew is somewhat consistent with other similar studies (e.g., Dabalen et al., 2016), where older respondents were noted to be less likely to respond. We also found that respondents were normally distributed across the 5 economic classes, consistent with the findings of Dabalen et al. (2016).
We view these early problems as a learning curve and provide an exercise in improving the user experience and functionality of the system, rather than being a reflection of any fundamental flaws in crowd-based data collection. This learning can be used to develop and improve protocols for citizen-led, digital tool-based approaches for data collection in Rwanda and beyond.
Diet Quality in Rwanda
From the initial data collection a number of diet quality patterns were observed. The dietary composition of a “traditional” Rwandan diet emerged, with the virtual ubiquity (>70% of responses) of tubers and starchy vegetables, leafy vegetables, fruits, legumes, and wholegrains in respondents' diets. The consumption of these food items is independent of gender, location, age, or economic grouping, suggesting an almost exclusively healthy core to Rwandan diets. These findings are consistent with the findings of the Global Nutrition Report Independent Expert Group (2020), which found relatively high consumption of fruits and legumes in Rwandan diets. In contrast, we found far lower prevalence of processed meat, fatty foods, and higher prevalence of vegetables (Global Nutrition Report Independent Expert Group, 2020). We also note that dairy (excluding milk) products, processed meats, and fast foods were the least consumed food items. However, their consumption was observed to be high in younger, wealthier individuals, conforming with findings in other LMICs (e.g., Li et al., 2020).
In general, Rwandan female respondents consumed better quality diets than male respondents. Female respondents were also observed to consume a greater diversity of both healthy and unhealthy food items, consistent with global studies (e.g., Imamura et al., 2015). However, female Rwandan respondents diverge from their counterparts in other countries, who were found to consume less unhealthy food items than men (Imamura et al., 2015; Abassi et al., 2019; Darling et al., 2020).
We also note that diet quality improved with age, aligning with both Imamura et al. (2015) and Andrade et al. (2016). Respondents under the age of 24 were observed to have the lowest quality diets. Poor diet quality is widespread in adolescents (Akseer et al., 2017), driving alarming levels of obesity (WHO, 2021), and micronutrient deficiencies (Akseer et al., 2017). The impacts of low diet quality in early life have been well-documented to have considerable health, mental well-being, and life opportunity implications (e.g., Florence et al., 2008; UNICEF, 2019).
The curvilinear relationship of economic status and diet quality demonstrates that both economic extremes suffer from poor diet quality. These results reflect observed global dietary and nutritional transitions, where individuals become wealthier, shifts towards consumption of processed, sweetened, and salted food stuffs are noted (Popkin, 2015). In a recent global study, Popkin et al. (2020) observed that higher-wealth individuals have seen the greatest increases in obesity and overweight individuals in sub-Saharan Africa. These economic trends have been found to co-occur with geographical transitions, where urban populations move away from traditional diets (Steyn and McHiza, 2014). Although we found that, generally, urban respondents consume higher amounts of healthy foods, their overall diet quality tends to be worse than rural respondents.
These early results reflect the dietary realities and point towards an underlying transition that may already be underway in Rwanda, evidenced by increased prevalence of childhood and adolescent obesity (Global Nutrition Report Independent Expert Group, 2020; Kinyonki et al., 2020). With Rwanda having one the fastest growing global economies (pre-COVID) (World Bank, 2021b), the country may be primed for continued reductions in diet quality, continued malnutrition issues, and increased prevalence of associated non-communicable diseases (GBD 2016 Risk Factors Collaborators, 2017; Marivoet et al., 2020). However, Rwanda has repeatedly demonstrated itself as being an enabling environment for pioneering data-driven decision-making to support healthy diets (Wagenaar et al., 2017). Implementation of long-term tracking of diet quality metrics, as outlined here, could help flag populations and locations with low diet quality and potential malnutrition implications to guide the allocation of resources for effective intervention. The information generated from this novel data collection system feed a dashboard (https://www.dietqualitymap.org) providing in [near] real time information on diet quality across Rwanda.
Next Steps
As data collection continues, we endeavour to identify mechanisms for improving efficiencies in the system. Envisioned content improvements include the deployment of a feedback system, where respondents become the recipients of tailored feedback to improve diet quality. This would represent a notable innovation in this field allowing respondents to be both data suppliers and information recipients. A powerful outcome of such improvements would be quantifying the extent to which dietary-based messaging can improve diet quality.
The use of the Diet Quality Questionnaire provided a validated, easy to use, and agile survey. Beyond this, its application also allows for comparison with results from a wider body of research, with the DQ-Q currently deployed across ~50 countries (Herforth et al., 2020b). Our implementation of the DQ-Q represents its first high frequency application and offers an invaluable opportunity to position our highly resolved (spatially and temporally) data for Rwanda, whilst simultaneously providing a learning opportunity for other researchers/ organisations wishing to implement similar high frequency data collection.
We will also look to contextualise our results with those of other high frequency data collections, like that of the World Food Programme's World Hunger Map [World Food Programme (WFP), 2021]. The aim of which being to identify if any linkages between patterns of diet quality and malnutrition can be identified for Rwanda. Our work will further explore how socio-economic, geographic, and market factors contribute to diet quality.
We will also investigate if any relationship between diet quality and weather conditions can be observed from high frequency weather data (Funk et al., 2015; Verdin et al., 2020). We hope to identify potential weather-based shocks and ascertain how, if at all, these affect regional and national diet quality.
Finally, we demonstrate how an agile and adaptable data collection system can be deployed for high frequency diet quality data collection, at minimal cost. We believe the developed system could easily be adapted to the dietary and technological contexts of other countries and are investigating potential opportunities to scale activities into neighbouring countries in Central-East Africa.
Conclusions
Innovation for food system development transcends the optimisation of on-farm production or prevention of post-harvest losses. It must also focus on consumers. Understanding and tracking diet quality is critical to guide decisions and support health outcomes. We have developed and tested an innovative system that leverages digital tools to directly crowdsource diet quality information from across Rwandan society. In collecting dietary information from 9,726 Rwandans in 8 weeks, we demonstrate the benefits of crowd-based systems deployed on digital tools for high frequency data collection, at a reasonable cost. Our preliminary results conform to dietary realities in other LMICs. We observed that female, older, and middle-income Rwandans tend to have better quality diets. The outputs also point towards nascent dietary transitions across groups. These basic insights will be complemented with further data collection, more complex analytics, and contextualisation within global studies. The sustained data flow from the system set-up constitutes an indispensable, scalable, and promising step towards robust data-driven policies and interventions for food security in LMICs. These advances are invaluable to researchers, policy-makers, and development actors who are interested in addressing diet quality and nutrition security at the most critical location(s) and time.
Data Availability Statement
The annonymised raw data supporting the conclusions of this article will be made available by the authors, without undue reservation, and in agreement with data availability policies of the Government of Rwanda. A fully interactive dashboard of updated project results is available at www.dietqualitymap.org.
Ethics Statement
The studies involving human participants were reviewed and approved by Rwanda National Ethics Committee. Written informed consent to participate in this study was provided by the participants' legal guardian/next of kin.
Author Contributions
RM and JA: idea generation, system development, analysis, and writing. MN, RR, and MS: idea generation and writing. AG: analysis and writing. SE: system development and implementation and writing. RK: analysis. AF: system implementation and writing. All authors contributed to the article and approved the submitted version.
Funding
This work was supported by CGIAR Big Data Platform and their Inspire Challenge 2020. Further funding support was provided by the Belgian Directorate General for Development Cooperation and Humanitarian Aid (DGDC) through the Consortium for Improving Agricultural Livelihoods in Central Africa (CIALCA—www.cialca.org).
Conflict of Interest
SE and AF were employed by company VIAMO.
The remaining authors declare that the research was conducted in the absence of any commercial or financial relationships that could be construed as a potential conflict of interest.
Publisher's Note
All claims expressed in this article are solely those of the authors and do not necessarily represent those of their affiliated organizations, or those of the publisher, the editors and the reviewers. Any product that may be evaluated in this article, or claim that may be made by its manufacturer, is not guaranteed or endorsed by the publisher.
Supplementary Material
The Supplementary Material for this article can be found online at: https://www.frontiersin.org/articles/10.3389/fsufs.2021.804821/full#supplementary-material
References
Abassi, M. M., Sassi, S., El Ati, J., Gharbia, H. B., Delpeuch, F., and Traissac, P. (2019). Gender inequalities in diet quality and their socioeconomic patterning in a nutrition transition context in the Middle East and North Africa: a cross sectional study in Tunisia. Nutr. J. 18:18. doi: 10.1186/s12937-019-0442-6
Adewopo, J., Solano-Hermosilla, G., Colen, L., and Micale, F. (2021). Using crowd-sourced data for real-time monitoring of food price during the COVID-19 pandemic: insights from a pilot project in northern Nigeria. Global Food Secur. 29:523. doi: 10.1016/j.gfs.2021.100523
Akseer, N., Al-Gashm, S., Mehta, S., Mokdad, A., and Bhutta, Z. A. (2017). Global and regional trends in the nutritional status of young people: a critical and neglected age group. Ann. New York Acad. Sci. 1393, 3–20. doi: 10.1111/nyas.13336
Andrade, S. C., Previdelli, Á. N., Cesar, C. L., Marchioni, D. M., and Fisberg, R. M. (2016). Trends in diet quality among adolescents, adults and older adults: a population-based study. Prevent. Med. Rep. 4, 391–396. doi: 10.1016/j.pmedr.2016.07.010
Arriagada, J., Munizaga, M., Schwartz, D., and Mena, C. (2021). Nudging Contributions in Crowdsourced Public Transport Technologies. Available online at: https://ssrn.com/abstract=3795622 or http://dx.doi.org/10.2139/ssrn.3795622 (accessed December 22, 2021).
Arsenault, J. E., and Olney, D. K. (2021). Review of the micronutrient situation in Rwanda. Food Nutr. Bull. 42, 133–154. doi: 10.1177/0379572120975298
Beal, T., Herforth, A., Sundberg, S., Hess, S. Y., and Neufeld, L. M. (2021). Differences in modelled estimates of global dietary intake. Lancet. 397, 1708–1709. doi: 10.1016/S0140-6736(21)00714-5
Dabalen, A., Etang, A., Hoogeveen, J., Mushi, E., Schipper, Y., and von Engelhardt, J. (2016). Mobile Phone Panel Surveys in Developing Countries: A Practical Guide for Microdata Collection. Directions in Development. Washington, DC: World Bank.
Darling, A. M., Sunguya, B., Ismail, A., Manu, A., Canavan, C., Assefa, N., et al. (2020). Gender differences in nutritional status, diet and physical activity among adolescents in eight countries in sub-Saharan Africa. Trop. Med. Int. Healthy. 25, 33–43. doi: 10.1111/tmi.13330
Doak, C. M., Adair, L. S., Bentley, M., Monteiro, C., and Popkin, B. M. (2005). The dual burden household and the nutrition transition paradox. Int. J. Obes. 29, 129–36.5. doi: 10.1038/sj.ijo.0802824
Doak, C. M., Adair, L. S., Monteiro, C., and Popkin, B. M. (2000). Overweight and underweight coexist within households in Brazil, China and Russia. J. Nutr. 130, 2965–2971. doi: 10.1093/jn/130.12.2965
FAO. (2018). The State of Food Security and Nutrition in the World. Building Climate Resilience for Food Security and Nutrition. Available online at: https://www.fao.org/3/i9553en/i9553en.pdf (accessed August 21, 2021).
Ferrara, G., Kim, J., Lin, S., Hua, J., and Seto, E. (2019). A focused review of smartphone diet-tracking apps: usability, functionality, coherence with behavior change theory, and comparative validity of nutrient intake and energy estimates. JMIR mHealth uHealth 7:e9232. doi: 10.2196/mhealth.9232
Florence, M. D., Asbridge, M., and Veugelers, P. J. (2008). Diet quality and academic performance. J. School Health. 78, 209–15 doi: 10.1111/j.1746-1561.2008.00288.x
Funk, C., Peterson, P., Landsfeld, M., Pedreros, D., Verdin, J., Shukla, S., et al. (2015). The climate hazards infrared precipitation with stations - a new environmental record for monitoring extremes. Sci. Data. 2:150066. doi: 10.1038/sdata.2015.66
GBD 2016 Risk Factors Collaborators. (2017). Global, regional, and national comparative risk assessment of 84 behavioural, environmental and occupational, and metabolic risks or clusters of risks, 1990-2016: a systematic analysis for the Global Burden of Disease Study 2016. Lancet (London, England) 390, 1345–1422. doi: 10.1016/S0140-6736(17)32366-8
Gibson, D. G., Pereira, A., Farrenkopf, B. A., Labrique, A. B., Pariyo, G. W., and Hyder, A. A. (2017). Mobile phone surveys for collecting population-level estimates in low- and middle-income countries: a literature review. J. Med. Int. Res. 19:e139. doi: 10.2196/jmir.7428
Global Nutrition Report Independent Expert Group (2018). Global Nutrition Report: Shining a Light to Spur Action on Nutrition. Available online at: https://globalnutritionreport.org/reports/global-nutrition-report-2018 (accessed May 5, 2020).
Global Nutrition Report Independent Expert Group (2020). Global Nutrition Report: Action on Equity to End Malnutrition. Available online at: https://globalnutritionreport.org/reports/2020-global-nutrition-report/ (accessed October 17, 2021).
Gómez, M. I., Barrett, C. B., Raney, T., Pinstrup-Andersen, P., Meerman, J., et al. (2013). Post-green revolution food systems and the triple burden of malnutrition. Food Policy. 42, 129–138. doi: 10.1016/j.foodpol.2013.06.009
Herforth, A., Beal, T., and Rzepa, A. (2020b). Global Diet Quality Project Aims to Bridge Data Gap. Gallup Blog. Available online at: https://news.gallup.com/opinion/gallup/321968/global-diet-quality-project-aims-bridge-data-gap.aspx (accessed October 15, 2021).
Herforth, A. W., Wiesmann, D., Martínez-Steele, E., Andrade, G., and Monteiro, C. A. (2020a). Introducing a suite of low-burden diet quality indicators that reflect healthy diet patterns at population level. Curr. Dev. Nutr. 4:nzaa168. doi: 10.1093/cdn/nzaa168
Imamura, F., Micha, R., Khatibzadeh, S., Fahimi, S., Shi, P., Powles, J., et al. (2015). Dietary qualityamong men and women in 187 countries in 1990 and 2010: a systematic assessment. Lancet GlobalHealth. 3, E-132–142. doi: 10.1016/S2214-109X(14)70381-X
Katmada, A., Satsiou, A., and Loannis, K. (2011). Incentive Mechanism for Crowdsourcing Platforms. Springer-Verlag Berlin Heidelberg.
Kinyonki, D. K., Ross, J. M., Lazzar-Atwood, A., et al. (2020). Mapping local patterns of childhood overweight and wasting in low- and middle-income countries between 2000-2017. Nat. Med.
Lamanna, C., Hachhethu, K., Chesterman, S., Singhal, G., Mwongela, B., Ng'endo, M., et al. (2019). Strengths and limitations of computer assisted telephone interviews (CATI) for nutrition data collection in rural Kenya. PLoS ONE 14:e0210050. doi: 10.1371/journal.pone.0210050
Li, L., Sun, N., Zhang, L., Xu, G., Liu, J, Hu, J., et al. (2020). Fast food consumption among young adolescents aged 12–15 years in 54 low- and middle-income countries. Global Health Action 13:1. doi: 10.1080/16549716.2020.1795438
Marivoet, W., Ulimwengu, J. M., and Maty Sall, L. (2020). Rwanda: Policy Atlas on Food and Nutrition Security. Available online at: https://snv.org/assets/explore/download/policy_atlas_rw_2020-06-23_press.pdf (accessed November 29, 2021).
National Institute of Statistics of Rwanda and ICF (2020). Rwanda Demographic and Health Survey 2019-20: Key Indicators Report. Kigali, Rwanda, and Rockville, Maryland: NISR and ICF.
National Institute of Statistics Rwanda. (2021). Sustainable Development Goals. Available online at: https://sustainabledevelopment-rwanda.github.io/2-1-2/ (accessed October 19, 2021).
Pew Research Center. (2019). Smartphone Ownership is Growing Rapidly Around the World, But Not Always Equally. Available online at: https://www.pewresearch.org/global/2019/02/05/smartphone-ownership-is-growing-rapidly-around-the-world-but-not-always-equally/ (accessed October 25, 2021).
Popkin, B. M (2015). Nutrition transition and the global diabetes epidemic. Curr. Diab. Rep. 15:64. doi: 10.1007/s11892-015-0631-4
Popkin, B. M., Corvalon, C., and Grummer-Strawn, L. M. (2020). Dynamics of the double burden of malnutritioin and the changing nutrition reality. Lancet. 395, 65–74. doi: 10.1016/S0140-6736(19)32497-3
R Core Team. (2021). R: A Language and Environment for Statistical Computing. Vienna: R Foundation for Statistical Computing. Available online at: https://www.R-project.org/ (accessed November 23, 2021).
Reinhardt, K., and Fanzo, J. (2014). Addressing chronic malnutrition through multi-sectoral, sustainable approaches: a review of the causes and consequences. Front. Nutr. 1:13. doi: 10.3389/fnut.2014.00013
Rwanda Utilities Regulatory Authority. (2019). Statistics and Tariff Information in Telecom, Media and Postal Service as of the Fourth Quarter 2018. Available online at: https://rura.rw/fileadmin/Documents/ICT/statistics/Telecom_Statistics_report_as_of_December_2018.pdf (accessed October 5, 2021).
Rwanda Utilities Regulatory Authority. (2020). Active Mobile Cellular Telephone Subscriptions. Available online at: https://rura.rw/fileadmin/Documents/ICT/statistics/Mobile_cellular__Statistics_report_as_of_January_2020.pdf (accessed October 5, 2021).
Solano-Hermosilla, G., Adewopo, J., Peter, H., Arbia, G., Nardelli, V., Barreiro-Hurle, J., et al (2020). A Quality Approach to Food Price Crowdsourcing: The Case of Food Price Crowdsourcing Africa (FPCA) in Nigeria. Report of the European Commission Joint Research Center (EC-JRC), Luxembourg (2020). Available online at: https://publications.jrc.ec.europa.eu/repository/bitstream/JRC119273/fpca_a_quality_approach_to_real_time_prices_final_online.pdf (accessed October 25, 2021).
Steyn, N. P., and McHiza, Z. J. (2014). Obesity and the nutrition transition in Sub-Saharan Africa. Ann. New York Acad. Sci. 1311, 88–101. doi: 10.1111/nyas.12433
Trucano, M (2014). World Bank Blogs: Using Mobile Phones in Data Collection: Opportunities, Issues and Challenges. Available online at: https://blogs.worldbank.org/edutech/using-mobile-phones-data-collection-opportunities-issues-and-challenges (accessed September 10, 2021).
Tuffrey, V., and Hall, A. (2016). Methods of nutrition surveillance in low-income countries. Emerg. Themes Epidemiol. 13:4. doi: 10.1186/s12982-016-0045-z
UNICEF. (2019). The State of the World's Children. Available onlin at: https://www.unicef.org/documents/state-of-worlds-children-2019-regional-briefs (accessed October 1, 2021).
Verdin, A., Funk, C., Peterson, P., Landsfeld, M., Tuholske, C., and Grace, K. (2020). Development and validation of the CHIRTS-daily quasi-global high-resolution daily temperature data set. Sci. Data. 7:303. doi: 10.1038/s41597-020-00643-7
Vorster, H. H., Kruger, A., and Margetts, B. M. (2011). The nutrition transition in Africa: can it be steered into a more positive direction? Nutrients. 3, 429–441. doi: 10.3390/nu3040429
Wagenaar, B. H., Hirschhorn, L. R., Henley, C., Gremu, A., Sindano, N., and Chilengi, R. (2017). The AHI PHIT Partnership Collaborative. Data-driven quality improvement in low-and middle income country health systems: lessons from seven years of implementation experience across Mozambique, Rwanda, and Zambia. BMC Health Serv. Res. 17:830. doi: 10.1186/s12913-017-2661-x
Wells, J. C., Sawaya, A. L., Wibeak, R., Mwangome, M., Poullas, M. S., Yajnik, C. S., et al. (2019). The double burden of malnutrition: aetiological pathways and consequences for health. Lancet. 395, 75–88. doi: 10.1016/S0140-6736(19)32472-9
WHO. (2021). World Health Statistics. Available online at: https://apps.who.int/iris/bitstream/handle/10665/342703/9789240027053-eng.pdf (accessed September 18, 2021).
World Bank (2019). The World Bank in Rwanda. Available online at: https://www.worldbank.org/en/country/rwanda/overview (accessed May 7, 2020).
World Bank (2021a). PovcalNet: An Online Analysis Tool for Global Poverty Monitoring. Available online at: http://iresearch.worldbank.org/PovcalNet/home.aspx (accessed March 17, 2021).
World Bank (2021b). The World Bank in Rwanda. Available online at: https://www.worldbank.org/en/country/rwanda/overview#1 (accessed October 25, 2021).
World Food Programme (WFP) (2021). Hunger Map. Available online at: https://hungermap.wfp.org/ (accessed October 21, 2021).
Keywords: citizen science, dietary patterns, digital data collection, USSD, Rwanda
Citation: Manners R, Adewopo J, Niyibituronsa M, Remans R, Ghosh A, Schut M, Egoeh SG, Kilwenge R and Fraenzel A (2022) Leveraging Digital Tools and Crowdsourcing Approaches to Generate High-Frequency Data for Diet Quality Monitoring at Population Scale in Rwanda. Front. Sustain. Food Syst. 5:804821. doi: 10.3389/fsufs.2021.804821
Received: 29 October 2021; Accepted: 13 December 2021;
Published: 07 January 2022.
Edited by:
Fatih Ozogul, Çukurova University, TurkeyReviewed by:
Sneh Punia, Clemson University, United StatesCengiz Gokbulut, Balikesir University, Turkey
Copyright © 2022 Manners, Adewopo, Niyibituronsa, Remans, Ghosh, Schut, Egoeh, Kilwenge and Fraenzel. This is an open-access article distributed under the terms of the Creative Commons Attribution License (CC BY). The use, distribution or reproduction in other forums is permitted, provided the original author(s) and the copyright owner(s) are credited and that the original publication in this journal is cited, in accordance with accepted academic practice. No use, distribution or reproduction is permitted which does not comply with these terms.
*Correspondence: Rhys Manners, ci5tYW5uZXJzJiN4MDAwNDA7Y2dpYXIub3Jn