- 1Plant Production Systems, Wageningen University, Wageningen, Netherlands
- 2International Livestock Research Institute, Nairobi, Kenya
- 3International Institute of Tropical Agriculture (IITA), Central Africa Hub Office, Nairobi, Kenya
Smallholder farming in sub-Saharan Africa keeps many rural households trapped in a cycle of poor productivity and low incomes. Two options to reach a decent income include intensification of production and expansion of farm areas per household. In this study, we explore what is a “viable farm size,” i.e., the farm area that is required to attain a “living income,” which sustains a nutritious diet, housing, education and health care. We used survey data from three contrasting sites in the East African highlands—Nyando (Kenya), Rakai (Uganda), and Lushoto (Tanzania) to explore viable farm sizes in six scenarios. Starting from the baseline cropping system, we built scenarios by incrementally including intensified and re-configured cropping systems, income from livestock and off-farm sources. In the most conservative scenario (baseline cropping patterns and yields, minus basic input costs), viable farm areas were 3.6, 2.4, and 2.1 ha, for Nyando, Rakai, and Lushoto, respectively—whereas current median farm areas were just 0.8, 1.8, and 0.8 ha. Given the skewed distribution of current farm areas, only few of the households in the study sites (0, 27, and 4% for Nyando, Rakai, and Lushoto, respectively) were able to attain a living income. Raising baseline yields to 50% of the water-limited yields strongly reduced the land area needed to achieve a viable farm size, and thereby enabled 92% of the households in Rakai and 70% of the households in Lushoto to attain a living income on their existing farm areas. By contrast, intensification of crop production alone was insufficient in Nyando, although including income from livestock enabled the majority of households (73%) to attain a living income with current farm areas. These scenarios show that increasing farm area and/or intensifying production is required for smallholder farmers to attain a living income from farming. Obviously such changes would require considerable capital and labor investment, as well as land reform and alternative off-farm employment options for those who exit farming.
Introduction
It has been estimated that of the world's poor, almost two thirds work in agriculture (Olinto et al., 2013). In sub-Saharan Africa (SSA), smallholder farming can be a vicious cycle of low productivity and limited re-investment, keeping farming households trapped in poverty (Tittonell and Giller, 2013). The massive engagement in agriculture is a symptom of lack of access to alternative livelihood sources, with farming often being a last resort (Koning, 2017; Giller et al., 2021). Farming is not the primary interest for youth, who have other aspirations for employment. However, agriculture remains an important option, though often as a fall-back (e.g., Ramisch, 2014; LaRue et al., 2021; Sumberg et al., 2021).
Dorward et al. (2009) differentiate trajectories of farming households that are “stepping up” from those who are “stepping out” or simply “hanging in.” Households with sufficient resources to invest can “step up” toward more lucrative farming, whereas some choose to “step out” of farming when job opportunities arise in other sectors such as industry (Dorward et al., 2009). For some agriculture generates so little that they can only “hang in.” The pressure to step up or out of farming increases, because cultivated areas per farm are decreasing—and more so for those who already have the smallest cultivated areas (Headey and Jayne, 2014; Jayne et al., 2014; Giller et al., 2021).
With ever smaller farms, it becomes increasingly urgent to intensify production or to pursue alternative livelihood strategies. Simultaneously there is a growing demand for food from the burgeoning population in SSA, requiring intensification of farming to achieve self-sufficiency at national level (van Ittersum et al., 2016). Yet even when production is intensified, farms can simply be too small to obtain a decent living (Harris and Orr, 2014; Giller et al., 2021). This creates the imperative to investigate how smallholder incomes can be increased, given their small farm sizes, while at national level, increases in agricultural production are required to achieve food self-sufficiency (Giller, 2020). In pursuit of Sustainable Development Goals (SDGs; United Nations, 2015)—and SDG 1 Zero Poverty and SDG2 No Hunger, in particular—it is important to understand whether and how farming can be(come) a viable livelihood strategy, especially for the smallest farms. Whether through subsidies to increase yields, through land reform to increase farm sizes or other measures (Koning, 2017), the protection and support of the smallest farms needs to be considered to “leave no one behind” in the SDGs.
Many studies have shown that current, small farm sizes limit the incomes of smallholder farmers (e.g., Frelat et al., 2016; Marinus, 2021). Others have calculated what farm area would be required to reach the poverty line in dryland farming systems in SSA and India (Harris and Orr, 2014; Gassner et al., 2019). So far however, no studies have determined the minimum farm area required for households to reach the living income benchmark. Moreover, earlier assessments considered current cropping practices without exploring the effects of growing more profitable crops such as vegetables. In this study we use “living income” as a benchmark for the viability of farming (Anker and Anker, 2017b; van de Ven et al., 2020). The living income concept has recently gained attention (Living Income Community of Practice, 2021). It estimates the income that is required for a decent living (Anker, 2011; van de Ven et al., 2020), on the basis of the principles in the universal declaration of human rights (United Nations General Assembly, 1948). It therefore includes the income needed to provide a nutritious diet, housing, education and health care (Anker, 2011; van de Ven et al., 2020). The commonly used poverty line benchmark considers the minimum cost of living in the poorest countries in the world (Ravallion et al., 1991; Chen and Ravallion, 2010). As such, the living income is an addition to the commonly used poverty line benchmark (van de Ven et al., 2020).
The overall goal of this paper is to explore what farm area would be required to attain a living income from farming which we refer to as the “viable farm size.” We first assessed how current smallholder incomes (reported in survey data) compared with the site-specific living income thresholds, and investigated the contributions from crops, livestock and off-farm income. We then estimated viable farm sizes for several scenarios: first on the basis of current yields and crop area allocation, then on the basis of possible future intensification (increased yields) and then in addition, with more profitable crop configurations. Moreover, we examined contributions from livestock and off-farm income and how they affect the viable farm size. Lastly, we compared current farm sizes with viable farm sizes. Our analysis is focused on three contrasting sites in the East African highlands: Nyando in Kenya, Rakai in Uganda, and Lushoto in Tanzania.
Our research was guided by the following research questions:
1. What percentage of the farming population currently achieves a living income?
2. What farm size can provide a living income with current cropping systems—i.e., what is a viable farm size?
3. What are the implications of (a) intensification of the cropping system and (b) considering other sources of income, on the viable farm size?
Methodology
Three Contrasting Sites
Survey data was used from three contrasting sites in East Africa: Nyando in Kenya (2016), Rakai in Uganda (2017), and Lushoto in Tanzania (2015) (Table 1). All three sites have rainfall patterns that allow two cropping seasons each year. Nyando is located in the mid-lands of western Kenya, on the slopes next to Lake Victoria. Small streams and rivers cross the area from the upland areas toward the lake. As these river valleys often flood, they are commonly used for grazing livestock, while crops are cultivated on the elevated areas. Crops and livestock are both important for household income. Common crops are maize, beans, and sorghum (Mango et al., 2011; Kung'u and Namirembe, 2012). In Nyando, the relative importance of livestock is much larger than in Rakai and Lushoto. Rakai is located in the southern part of central Uganda and is characterized by an undulating landscape. It has a diverse cropping system, distinguishing itself from the other two sites by the importance of perennial crops, i.e., coffee and East African highland banana (referred to as banana hereafter). Other important crops are beans, maize and cassava (Kyazze and Kristjanson, 2011). Lushoto is located in the west Usambara mountains in northern Tanzania and has an undulating, hilly landscape. Valley bottoms are commonly used to grow vegetables such as cabbage and tomato, which are transported for sale in urban markets in Tanga and Dar es Salaam. Other important crops are maize, beans and Irish potato (Lyamchai et al., 2011). Population densities in the three sites (Table 1) are typical for the areas where the largest part of the population of the East African highlands lives (Vanlauwe et al., 2013).
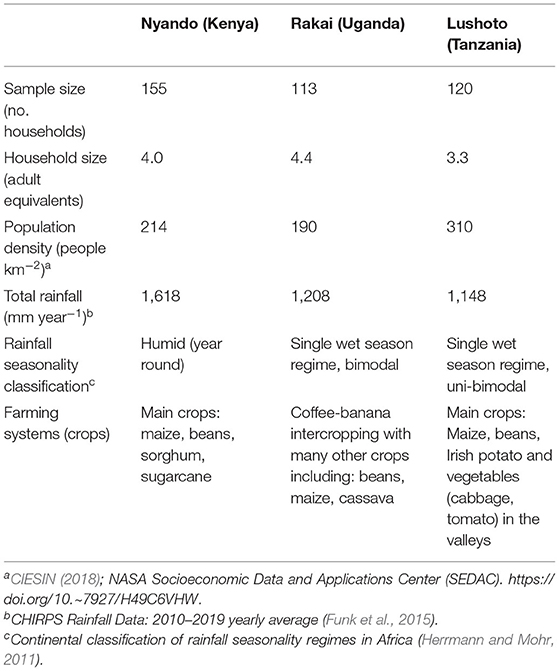
Table 1. Characteristics of the three research sites in the East African highlands from the RHoMIS database (van Wijk et al., 2020).
Estimating Current Value of Crops and Household Income
The Rural Household Multi-Indicator Survey (RHoMIS; Hammond et al., 2017) formed the primary data source and data were obtained from van Wijk et al. (2020). RHoMIS offers a relatively rapid and largely standardized questionnaire, aimed at estimating the well-being of farming households. The RHoMIS survey adheres to the principles of the 1964 WMA declaration of Helsinki (van Wijk et al., 2020). The survey was executed in 2016 in Nyando (155 households), in 2017 in Rakai (113 households), and in 2015 in Lushoto (120 households) (Table 1). From this household-level dataset, we extracted variables on household composition, total area cultivated, production metrics and economic value received (step 1a and 1b in Figure 1). Production in the previous year was reported for each type of crop grown and livestock owned, as well as the fractions consumed and sold, and the total income received for the sold amount. From these variables we derived the price per crop and livestock product per household. Following the RHoMIS approach (Hammond et al., 2017), prices were triangulated with prices from literature and where needed replaced by prices from literature. This was in particular the case for crops for which it is difficult to derive prices per kg, e.g., banana which is sold per bunch and when reported prices deviated a lot from literature (Supplementary Material 1). We then calculated the total value of crop and livestock produce per household, at the median price per site of each product. All prices of products were standardized to 2017 (year of the latest survey and converted to USD purchasing power parity (USD PPP) to enable comparison among sites. Income from off-farm sources was reported as the proportion of total income at household level, so we derived its value from the total value of sold farm produce (Hammond et al., 2017). We refer to “value of produce” when considering the value of crops and/or livestock produced on the farm and refer to “income” when all sources of household income are considered: i.e., value of crop produce, value of livestock produce and off-farm income. Income per household was expressed per Adult Equivalent (AE) following (OECD, 2011), using the household composition from the survey.
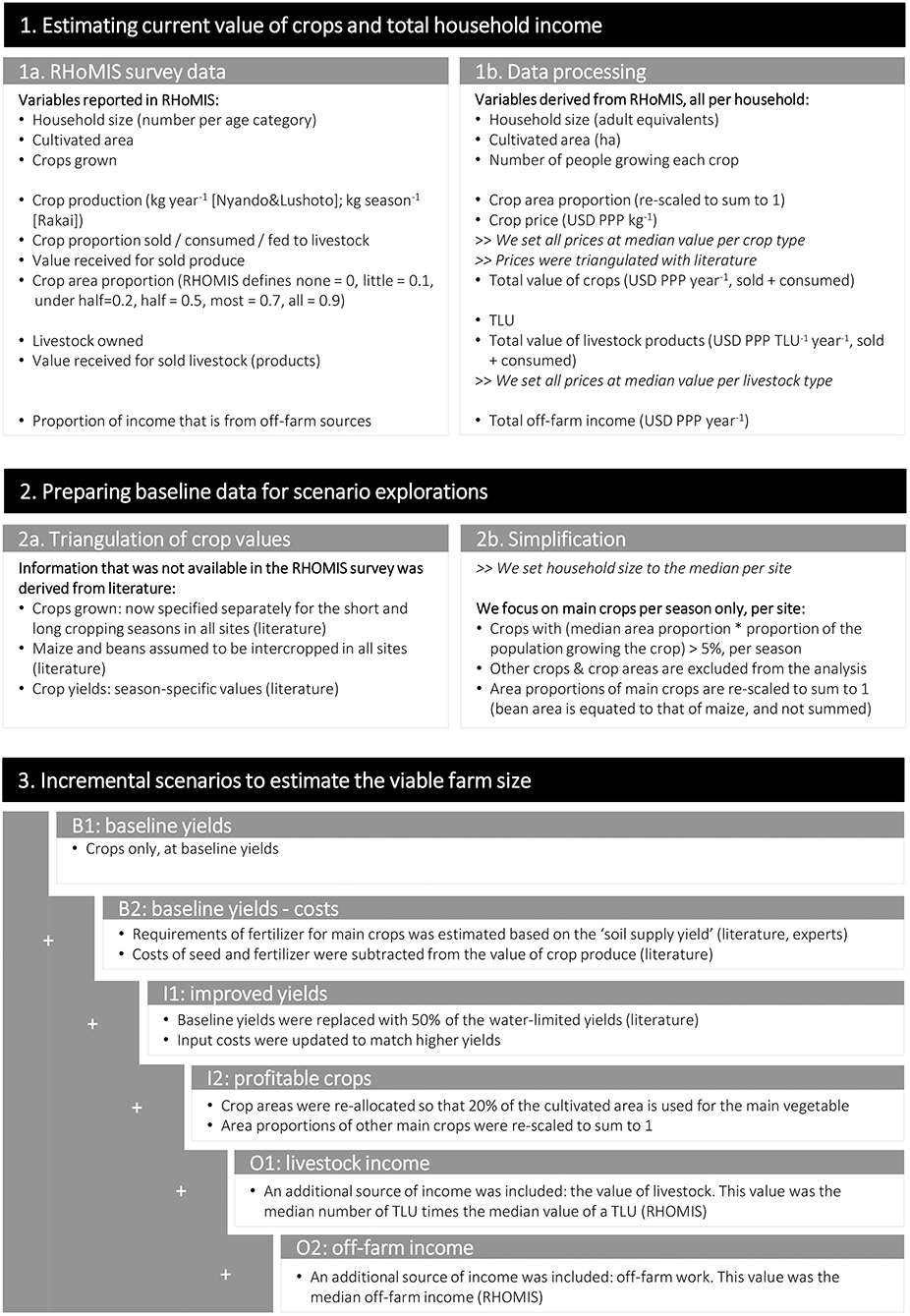
Figure 1. A schematic overview of the scenarios and progression of variables and values with every step in the methodology. TLU, tropical livestock unit; PPP, purchasing power parity (2017).
Living income estimates were used from Anker and Anker (2017a) for Kenya and from van de Ven et al. (2020) for Tanzania and Uganda. Living income estimates were all standardized to 2017 (van de Ven et al., 2020). The living income includes costs for a low-cost nutritious diet, housing, education, health care and unforeseen costs and is based on an average household composition and size (see van de Ven et al., 2020). The extreme poverty line benchmark of USD PPP 1.90 was assumed to be per adult equivalent and was corrected for inflation up till 2017, so that the extreme poverty line benchmark was set at USD PPP 2.08 per adult equivalent per day in all three study sites.
Preparing Baseline Data for Scenario Exploration
For the exploration of viable farm sizes in current and intensified cropping systems, we first established what was a representative, baseline cropping system per site. The RHoMIS data provided information on production per farm and per year and was not designed to capture crop yields or intercropping, and information on seasonal crop area allocation was only available for Rakai. In each of the study sites, intercropping is a common practice, and the bimodal rainfall pattern enabled that some crops are cultivated in one or in both cropping seasons. Because of this, crop yields could not be derived adequately from the survey, and resulted in unrealistically low estimates (Supplementary Material 2). Therefore, baseline yields were derived from literature instead of the survey (Supplementary Material 2). Seasonal crop cultivation patterns (which crop is cultivated in which season) were also based on literature for Nyando and Lushoto (MoALF, 2016; Marinus, 2021), while season-specific data was available for this from the survey in Rakai. For each of the sites, we assumed that maize was intercropped with common bean, whenever maize was cultivated. The survey-reported crop area proportions did not always add up to one (Supplementary Material 3), and were therefore rescaled proportionally to add up to one for each farm, for the main cropping season in each site. It was then assumed that if a crop was grown also in the minor season (based on literature), it was allocated the same area. We determined what were the main crops per site, by weighting the median proportion of farm area allocated to a crop by the proportion of the population growing it. In our simulated baseline cropping systems, we included only the main crops per site: i.e., those with a weighted area proportion equal to or larger than 5%. The weighted area proportions were then proportionally scaled to add up to one.
Scenarios Exploring the Viable Farm Sizes
Viable farm sizes were assessed for six incremental scenarios (Figure 1). The baseline-scenarios (B1: baseline yields and B2: baseline yield - costs) were used to explore the viable farm size within the baseline cropping system. The crop intensification-scenarios (I1: improved yields and I2: profitable crops) were used to explore how possible future options for intensification—increasing yields and cultivating more profitable crops—would change the viable farm size. The other income sources-scenarios (O1: livestock income and O2: off-farm income) assessed the impact of incorporating current income from sources other than crops, namely livestock and off-farm income sources.
Baseline crop yields, crop prices and crop configuration were used to calculate the value of crop produce per ha, which was then used to calculate the viable farm size in the most basic scenario B1: baseline yield. This scenario only included value of produce of crops and no income from livestock or other sources. Scenario B1: baseline yield does not include any input costs (which were not incorporated in RHoMIS) and therefore underestimates the viable farm size. This issue was addressed in scenario B2: baseline yield - costs, where input costs were subtracted from the value of produce. Input cost were calculated for mineral fertilizer and for the seed of annual crops. These inputs are commonly bought in the area, although rates and use strongly differ among households (e.g., Tittonell et al., 2005). Information on input use, rates or costs per crop per household was not available from the survey. Fertilizer requirements per crop were calculated based on the baseline yield and the “soil supply yield”: the yield obtained when no fertilizers are applied, which was derived from literature. For each crop, we assumed this soil supply yield to be the same as the lowest yield commonly obtained by farmers per site, while the baseline yield was the average yield commonly obtained by farmers per site. The difference between the baseline yield and the soil supply yield (baseline yield – soil supply yield) was then used to calculate fertilizer requirements based on nutrients concentrations in harvested product, the dry matter content and nutrient use efficiencies from literature. Only relevant macro-nutrients for fertilization were considered, e.g., N and P for maize and N and K for banana (East African highland banana in Uganda). Prices were based on the commonly used mineral fertilizers per crop and site. Costs for seed were based on commonly used varieties per site and advised sowing rates.
The crop intensification scenarios I1: improved yields and I2: profitable crops I2: profitable crops considered two options for intensification: increasing yields and cultivating more profitable crops. Scenario I1: improved yields uses the crop configuration of the baseline scenarios, while crop yields were increased to 50% of the water-limited yield. The costs of inputs were updated relative to scenario B2: baseline yield - costs, proportionally to the increase in yield. Fifty percent of the water-limited yield is considered as a possible goal for intensified crop production in SSA by 2050, which is needed to feed the burgeoning population (van Ittersum et al., 2016). Scenario I2: more profitable crops adds a crop area re-configuration, so that 20% of the cultivated area is allocated to the most common vegetable per site. Areas of other crops in the baseline crop configuration were scaled back proportionally.
Scenarios B1, B2, I1, and I2 focused on the contribution of only crops to household income. In the study sites however, livestock and off-farm income are also important contributors to incomes. When other sources of income are available besides crop production, the contribution from crops to attain a living income can be smaller and hence a smaller farm area can be viable. Livestock requires land as well, but no information was available in the survey data about the private and/or common land used for livestock keeping and almost no fodder production was reported. We could therefore only include the value of livestock produce (reported in the RHoMIS survey) in our scenarios, and not its relation to farm area required. In scenario O1: livestock income, the current median number of tropical livestock units (TLUs) owned per household per site was multiplied by the median value per TLU per site as reported in the survey, to estimate the total value of produce of livestock per household and its effect on the viable farm size. In scenario O2: off-farm income current median off-farm income as reported in the survey was included and its effect of the viable farm size assessed.
Understanding Variation in Scenario Outcomes Among Sites
Site-specific values for each of the variables were used in calculating the viable farm size. To reveal which variables most strongly determined variation among sites in the scenario outcomes, we ran the model for calculating the viable farm size five times, once for each additional variable used in the calculations for scenario B1: baseline yield. In the first run, variable values (crops and crop allocation, yields, prices, living income threshold, household size) in all sites were set at the same value: the value for Rakai. In the next step, crops cultivated and their area allocation were made site-specific, so that the site-specific yields (for site-specific crops) could be investigated in next step. In every next step, one more variable was made site-specific, starting with variables that were more related to the cropping system: first yields, then prices, then the living income threshold, then household sizes. Relative differences among steps and among sites were compared to assess which variables most strongly explained differences in outcomes among the three sites. The order of the steps did not influence the analysis as we only compared the relative differences between steps and sites.
Results
Current Income
Current Income From All Sources
When considering all sources of current household income, only 29% of the households in Nyando, 27% in Rakai and 17% in Lushoto obtained a living income (Figure 2A). The poverty line was reached by 61% of the households in Nyando, and just 50% in Rakai and 35% in Lushoto. At the left tail of the income distribution, crop produce for own consumption made the largest contribution to incomes in all three sites (Figure 2B). More than three quarters of the households had some off-farm income in Nyando and Rakai, while almost all households in Lushoto relied on farming only. In Nyando and Rakai, the contribution of off-farm income to the total household income was larger among households with a medium and high income than among households with a low income. Median off-farm income, for those receiving it, was also highest in Nyando (0.64 USD PPP AE−1 day−1), followed by Rakai (0.32 USD PPP AE−1 day−1) and Lushoto (0.23 USD PPP AE−1 day−1), and see also Supplementary Material 4. No information was available from the survey about whether off-farm income sources were used to invest in farm activities. The contribution of livestock to value of farm produce was much larger in Nyando and Lushoto than in Rakai. In Nyando, the contribution of livestock was often larger than that of crops. This may largely be due to the relatively large numbers of livestock—mainly cattle—kept in Nyando (Supplementary Material 5), at a median of 8.8 TLU per household compared to 1.6 and 1.4 TLU per household in Rakai and Lushoto (Table 2). The value of produce obtained per TLU was largest in Lushoto, however, where marketing dairy products is common, resulting in relatively high value of produce per TLU owned. In Rakai many households were holding pigs (Supplementary Material 5). Survey data revealed no relation between cultivated area and the number of TLU owned (Supplementary Material 6).
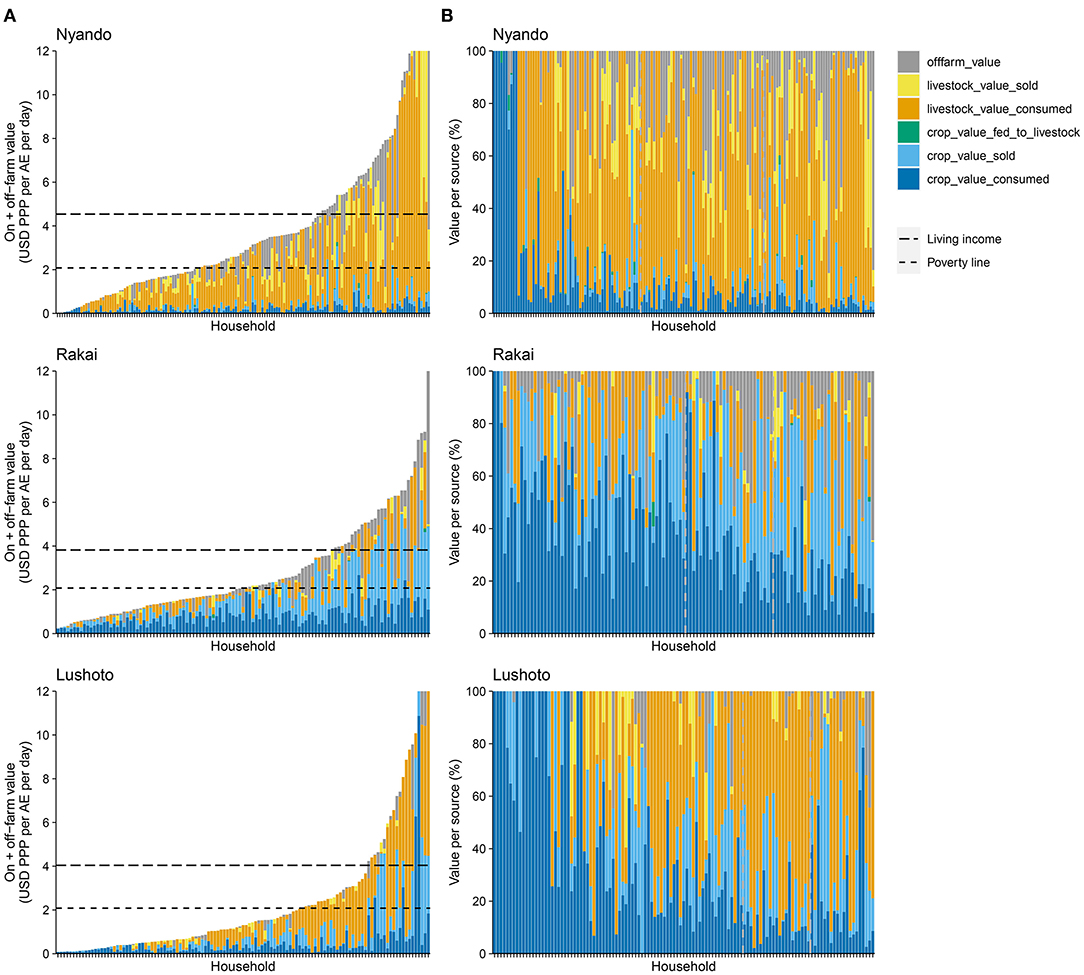
Figure 2. Current household income in relation to the poverty line and living income benchmarks (A) and the relative contribution of different income sources to the current income (B). Households in (B) are ordered the same as in (A).
Value of Crop Produce
None of the households in Nyando obtained a living income from the total value of crops alone (Figure 3A). In Rakai 20% of the households and in Lushoto about 10% of the households obtained a living income from value of crops alone. Income from crops was generally highest in Rakai, where high-value perennial cash crops were more common. The most important crops in terms of value produced differed per site (Figure 3B). Maize was most important in Nyando and Lushoto, constituting 49 and 42% on average of the total value of crops, respectively. In Rakai coffee (29%) and banana (23%) were the most important crops in terms of value of produce. Some other specific crops, that were important per site are sorghum (13%) and sugarcane (7%) in Nyando, Irish potato in Rakai (11%) and Lushoto (10%). Beans were common in all three sites and most important in terms of value of produce in Lushoto (23%). Among households that obtained a low total value of crops, specific crops were relatively more prevalent: sorghum in Nyando and beans in Lushoto.
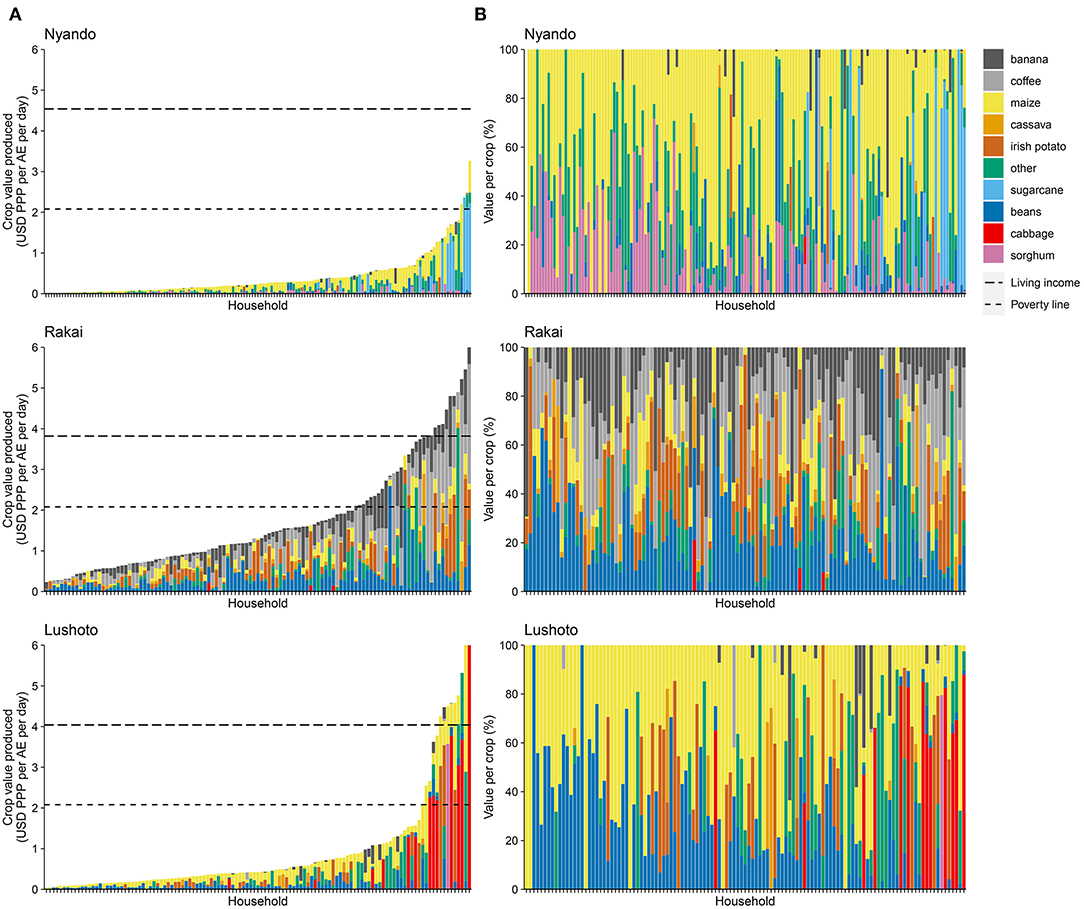
Figure 3. The current value of crop produce in relation to the poverty line and living income benchmarks (A) and the relative contribution of different crops to the total value of crops (B). The value of crop produce is the sum of the value sold, consumed and fed to livestock. Households in (B) are ordered the same as in (A).
Farm areas and the total value of crop produce were unequally distributed (distributions shown in Supplementary Material 4). In Nyando and Rakai, those who obtained a larger value of crops (>85 percentile) tended to have larger farms than those who produced less crop value (Figure 4).
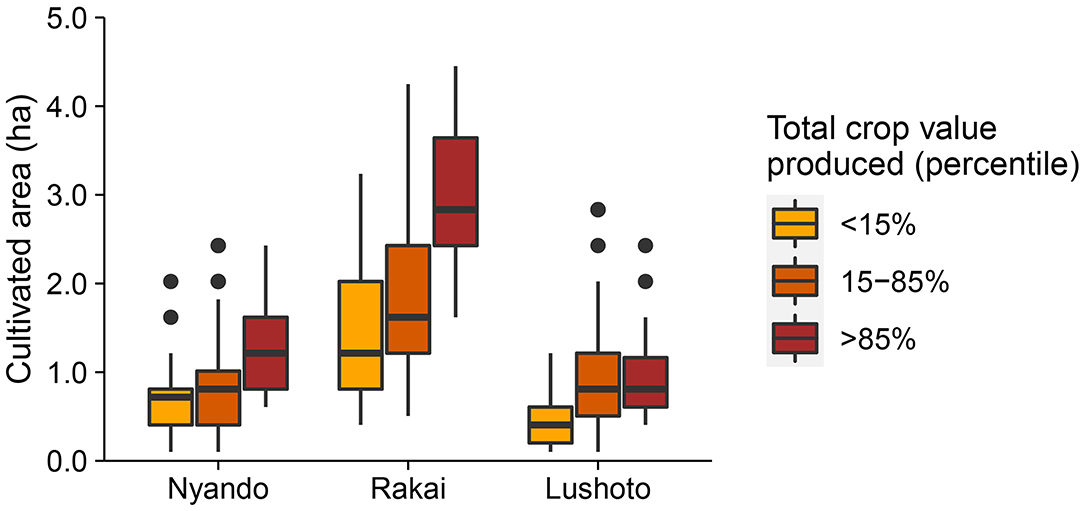
Figure 4. Cultivated areas for households in relation to a low (<15 percentile), medium (15–85 percentile) or high (>85 percentile) current total value of crop produce.
Viable Farm Size
Scenario B1: baseline yield resulted in viable farm sizes of 2.5, 2.0, and 1.6 ha for Nyando, Rakai and Lushoto, respectively (Figure 5; Supplementary Material 7). This was a three-fold difference with the current median cultivated area in Nyando (0.8 ha) and a two-fold difference for Lushoto (0.8 ha), while for Rakai the viable farm size was similar to the current median cultivated area in Rakai (1.8 ha). The relatively small viable farm size estimate for Lushoto can be explained primarily by the combination of relatively high-value crops (see effect of variation in crops and crop allocation, Step 1, Table 3), and the smallest median household size of all sites (Step 6), which both result in a smaller viable farm size. In Nyando, crop prices were relatively low (Step 4), while the living income was relatively high (Step 5), resulting in a relatively large viable farm size. Crop prices were most favorable in Rakai (Step 4), e.g., beans were most expensive in Rakai, although less than double the price in the other two sites. Yield differences had the smallest effect on the variation in outcomes among the three sites (Step 3).
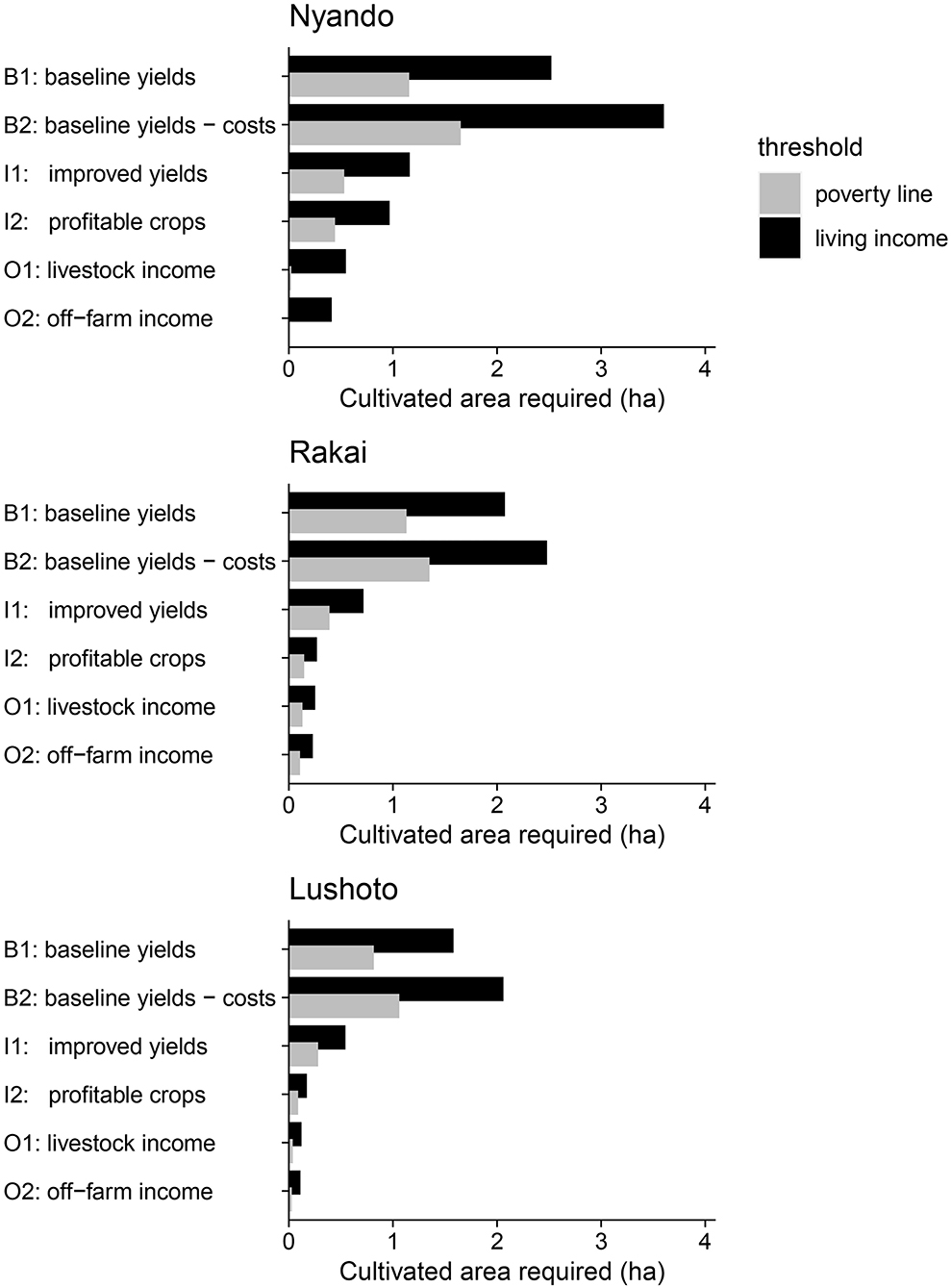
Figure 5. The cultivated area required to reach the poverty line or obtain a living income (viable farm size) for a household of median size for six scenarios. All scenarios are incremental, meaning that each scenario builds on all improvements and assumptions of the previous scenario.
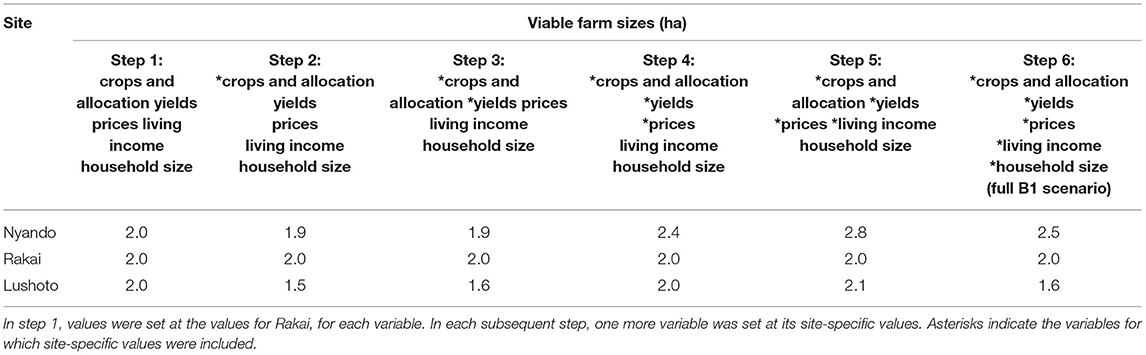
Table 3. Viable farm sizes without or with the site-specific values per variable that were included in estimating the viable farm size, using scenario B1: baseline yields.
Including basic input costs of fertilizer and seed (scenario B2: improved yields - costs) had a strong effect, as it resulted in a 30, 20, and 25% larger cultivated area needed to attain a living income than in the previous scenario without input costs (scenario B1: baseline yield) in Nyando, Rakai, and Lushoto, respectively (Figure 5).
The crop intensification scenarios strongly reduced the viable farm sizes. Increasing yields to 50% of the water-limited yield (scenario I1: improved yields) had the largest effect and resulted in viable farm size estimates that were three times smaller than in scenario B2: baseline yields - costs (Figure 5). Allocating 20% of the cultivated area to the most common vegetable per site (scenario I2: profitable crops) resulted in a larger area reduction in Rakai and Lushoto than in Nyando due to the higher gross margin of tomato and cabbage in Rakai and Lushoto, respectively, as compared to kale in Nyando. Vegetables however, currently only occupied a minor part of the cultivated area and only few households had >20% of their cultivated area under vegetables: 6, 15, and 9% of households in Nyando, Rakai, and Lushoto, respectively, and these were commonly the households that obtained a high value of crop produce (Figure 3).
Including livestock as an additional income source (scenario O1: livestock income) had only a limited reducing effect on the viable farm sizes, in comparison to the crop intensification scenarios (Figure 5). The largest effect was found in Nyando, where the number of cattle owned was relatively large (Table 3). This cattle was likely sustained from grazing on common land around nearby streams and wetlands. Households in Rakai and Lushoto owned much fewer TLUs on average and therefore had less income from livestock (despite the relatively high value per TLU in Lushoto, due to dairy marketing). The estimates of the viable farm size therefore decreased only very little. Including off-farm income as a contributor to a living income (scenario O2: off-farm income), again resulted in a relatively large decrease in the viable farm size in Nyando (Table 3). The sum of income from livestock and off-farm sources was USD PPP 2.12, which is more than the poverty line, indicating the importance of alternative income sources in Nyando. Income from crops would not be required to reach the poverty line, with median incomes from livestock and off-farm sources in Nyando, but a living income was not reached with these non-crop sources only. Including off-farm income had only a small effect in Rakai and Lushoto.
Comparing Viable Farm Areas With Current Cultivated Areas
By comparing viable farm sizes with the current cultivated areas we assessed what proportion of the current population would be able to attain a living income with their current farm area, for each of the scenarios. Because the scenarios were incremental, every next scenario resulted in a smaller estimate of the viable farm size (except scenario B2: baseline yields - costs which incorporated costs) and a larger number of households in the study populations had access to the estimated viable farm size. This number strongly depended on the shape of the distribution of current farm sizes (Figure 6), which was skewed toward smaller farm sizes in Nyando and Lushoto. In each of the sites, a small proportion or none of the households currently cultivated an area larger than the viable farm sizes of the baseline scenarios (B1: baseline yield, B2: baseline yields - costs). In the conservative scenario B2: baseline yields - costs this was 0, 27, and 4% for Nyando, Rakai, and Lushoto, respectively. The yield-improvement scenario (I1: improved yields) decreased the viable farm size so much in Rakai and Lushoto, that it covered the flattest part of the curve with a major shift in the proportion of the population having a viable farm size, 92 and 70% in Rakai and Lushoto, respectively. In Nyando, apart from crop intensification, income from livestock was required (scenario O1: livestock income) for the majority of the study population (73%) to be able to attain a living income from their currently cultivated area.
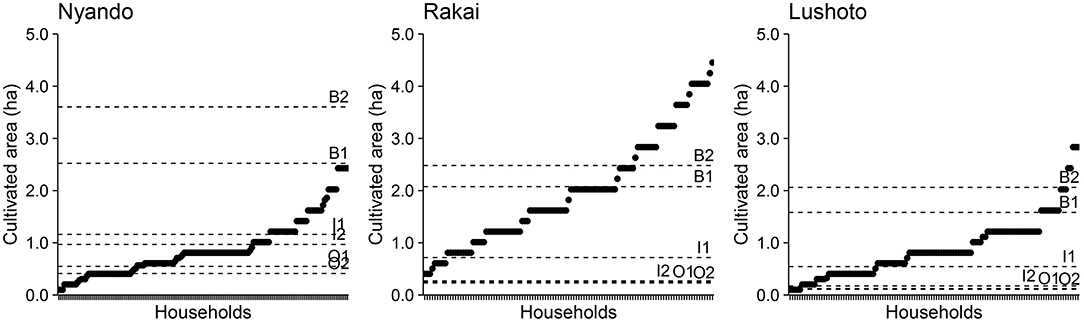
Figure 6. Distributions of current cultivated areas per household as reported in the survey, and the cultivated area required to attain a living income (viable farm size; dashed horizontal lines) for six scenarios. Full scenario names: B1: baseline yields, B2: baseline yields - costs, I1: improved yields, I2: profitable crops, O1: livestock income, O2: off-farm income.
Discussion
We first compared current smallholder farmers' incomes in three sites in the East African highlands with the living income benchmark. We then assessed what area would be required to attain a living income from smallholder farming—the viable farm size—and compared this with current cultivated areas. We explored six incremental scenarios, which included intensification (increased yields and a change in crop configuration) and other sources of income (livestock and off-farm). For each scenario, we estimated the viable farm size. This study is the first that uses the living income as a benchmark for establishing what would be a viable farm size. It builds on earlier work in SSA that used the poverty line as a benchmark (Harris and Orr, 2014), and similar historical assessments of what would be “decent” incomes for farmers in Europe after the second world war (Van Merriënboer, 2019). Such calculations are still made by the European Union to estimate subsidy requirements for farmers' incomes to be comparable with non-farm jobs in the EU (2020). Our results explored viable farm sizes but do not provide a precise answer to the question what a future farm size would need to be, as the analysis is based on simple assumptions and does not consider all complexities of making a living from farming. The scenario with baseline yields and input costs (scenario B2: baseline yields - costs) was the most conservative, providing a first rough estimate of what a viable farm sizes would be under current production levels and market prices for an average sized family: 3.6, 2.4, and 2.1 ha for Nyando, Rakai, and Lushoto, respectively, which is 4.5, 1.3, and 2.5 times the current, median cultivated area in the three sites. Currently, only 0, 27, and 4% of the population had a cultivated area that was larger than the viable farm size in scenarios B2: baseline yields - costs, in Nyando, Rakai, and Lushoto, respectively. Current cultivated areas were large enough for most households to attain a living income only in the intensification scenarios for Rakai and Lushoto (Figure 6). For Nyando a living income could not be attained unless other sources of income, i.e., livestock, were also included. This indicates that the cultivated area per household would have to increase and/or that cropping systems would have to intensify considerably for farming households to attain a living income from farming.
Current Smallholder Incomes
The analysis of current income clearly showed the limited value that is currently accrued from cultivating crops, with currently only 11% of the households obtaining a living income from crops in Rakai, while this held for 8% in Lushoto and none of the households in Nyando. Smallholder farmers relied on diverse livelihood activities besides crop cultivation, although poorer households tended to rely primarily on cropping, i.e., the 5–15% of the households with the lowest household income. With all income sources combined, only 29% of the households in Nyando, 27% in Rakai and 17% in Lushoto obtained a living income, based on the survey data. Crops contributed only to part of the total household income and this contribution strongly varied per site. Considering crops alone, at best, <20% of the households currently obtained a living income (Rakai), while none made a living income in Nyando. Households with low total household income often depended solely on farming and used the largest part of their farm produce for home consumption. This may imply that investing in crops and obtaining a good income from crops alone is difficult in current farming systems. In order to increase yields and intensify, farmers need viable options in which to invest (Vanlauwe and Dobermann, 2020). Livestock and off-farm income were most important for household income in Nyando, with all households having livestock and 63% of households having off-farm income. In all three sites, these sources of income were primarily important for households with a relatively higher income. The importance of livestock and off-farm income as an income source for better-off households in the study sites is in line with earlier studies (Frelat et al., 2016; Wichern et al., 2017; Waha et al., 2018). Among the households that obtained a low total value of crops, staple crops were common (beans in Lushoto, sorghum in Nyando), rather than high-value cash crops (sugarcane in Nyando). It is unclear from the data whether the production of low-input, low-value crops was the result of preference or necessity. Limited opportunity to invest or access markets could be major constraints for possible improvements like sustainable intensification, for these households. The sparse contributions of off-farm sources to incomes in Rakai and Lushoto point to the limited current off-farm opportunities in rural areas in SSA (Headey and Jayne, 2014). Toward the left tail of the income distribution graphs, reported incomes were very low and often well below the poverty line and the living income. This suggests that the survey data may have under-reported current household incomes. Under-reporting of incomes is a common problem in this type of surveys (Fraval et al., 2019) that may be partly explained by food sharing among households during the lean season when food stocks start to run out (Djurfeldt and Wambugu, 2011), something that was not captured in the survey. Livestock holdings seemed not to be related to farm area, and fodder production was only reported a few times in the survey. Additional, more specific, data on land use by livestock is needed to assess the potential role of livestock in providing a living income, in relation to the area that is cropped.
Viable Farm Sizes to Attain a Living Income
Our analysis showed that current farm areas are in most cases too small to attain a living income from farming, if no changes in cropping systems are made. For instance, only 0, 27, and 4% of the households had a current farm area that was the same or larger than the viable farm size in the scenario B2: baseline yields - costs in Nyando, Rakai, and Lushoto, respectively (Figure 6). This means that for farms to be viable, the area under cultivation needs to be increased and/or production intensified. There was a large gap between yields of major crops in the baseline scenarios and the improved yields in the intensification scenarios (50% of the water-limited yields), which were more than three times larger. Hence, the estimate of the viable farm size was also reduced by a factor three approximately in scenario I1: improved yields, compared to scenario B2: baseline yields - costs (Figure 5). This meant that 27, 92, and 70% of the households currently had a farm area that was the same or larger than the viable farm size in Nyando, Rakai, and Lushoto, respectively (Figure 6). Intensification to yield levels that were 50% of the water-limited yield (as in the I1: improved yields scenario) is possible at farm level in western Kenya, e.g., by providing a USD 100 input voucher per season (Marinus, 2021). Our results are therefore slightly more optimistic than those of Harris and Orr (2014), who looked at the impact of options for agronomic improvement at household level. They found that these improvements would not raise most households above the poverty line because cultivated areas were too small. Their analysis, however, did not consider income from livestock, nor areas with high-value crops such as banana, coffee and vegetables, although they considered variable costs in detail (e.g., labor). Among the study sites, crops were least profitable in Nyando, and it would be a challenge to attain a decent living from crops alone with current farm areas. Including livestock value of produce in Nyando, a living income could be attained with current farm sizes, i.e., 73% of the households had a current farm area that was the same or larger than the viable farm size in scenario O1: livestock income. By including only basic input costs (seed and mineral fertilizers) and no other costs in our study, we may have overestimated incomes from farming, and hence underestimated the farm size required to provide a living income. This and our other assumptions (e.g., using median yields and seasonal cropping patterns from literature) were made on the grounds of data availability and quality. Further research would be required to provide more detailed estimates, preferably from on-farm studies, to assess the profitability of crops across farms, the yields that can be attained, and the input costs required. Our calculated viable farm sizes should therefore be seen as minimum viable farm sizes, which likely need to be larger if other costs and other limiting factors such as production risks (e.g., due to price or climate variability) would be included.
Scenarios were based on the baseline crop configurations, up to scenario I2: profitable crops. This choice was data-driven. We realize that once people gain investment capacity, their livelihood strategies may change, and they might move toward more capital-intensive farming strategies. Some of the crops in the baseline crop configurations are currently cultivated because they can provide at least some yield with low inputs, for instance cassava (Fermont et al., 2008). Once higher incomes are achieved, such crops may be replaced by more profitable crops. Opportunities for cultivating high-value crops however, are limited as crops such as vegetables often have a limited demand, high input cost and highly varying prices. Moreover, suitable land for cultivating vegetables is limited, which also explains why currently only few households cultivated vegetables on more than 20% of their cultivated area. Vegetables, for instance, are cultivated only in inland valleys in Lushoto because of water availability, which limits the options to increase the cultivated area with vegetables (Sakané et al., 2013). Once production levels and/or the types of crops produced change, market prices will change as well, as was for instance found when maize production increased in Ethiopia (Spielman et al., 2010; Abate et al., 2015). Such fluctuations would again influence the profitability of the scenarios explored. For the case of vegetables in particular, demand may be fairly inelastic.
Expanding Farm Sizes and/or Intensifying Production? Implications of Moving Toward Viable Farms
A large proportion of the study population did not have a viable farm size. With intensification however, a decent income appears to be within reach on the farm areas that were cultivated at the time of the survey. To attain a decent living, farming households could therefore expand and/or intensify production, or combine both options (Giller et al., 2021). All choices would require substantial changes at farm level, and it is unlikely that these options are either feasible or attractive for all. Intensification and/or expansion may only be achieved when supportive measures are in place, such as input subsidies. Moreover, such strategies would only be relevant if they are in line with households' objectives and aspirations: do they pursue farming as a livelihood strategy, or seek alternative employment, or a combination of the two (e.g., LaRue et al., 2021; Sumberg et al., 2021)? Supportive measures should also consider that intensification and/or expansion of production could influence inequalities within households, where women often have less access to land or control over production resources and outputs (Beuchelt, 2016; Tavenner et al., 2019).
We assessed the farm area required at household level, while only considering capital through a simple assessment of input costs. More elements of farm structure—labor and capital—however, would have to be dedicated to intensification and/or expansion. Although the use of inputs such as mineral fertilizer and improved seed can be profitable in current smallholder farming systems, their use is often limited (Nin-Pratt and McBride, 2014). Increasing yields to 50% of the water-limited yield, would require considerable increases in input use: the N fertilizer requirements in scenario I1: improved yields for instance, were three to five times larger than at baseline yields. Such an increase in input use may require input subsidies (Jayne et al., 2018), along with other supportive policies such as price protection and improving access to markets (Wiggins, 2016; Koning, 2017), which together have shown to be able to increase yields to 50% of the water-limited yield at farm level (Sanchez et al., 2007; Marinus, 2021). Also Fraval et al. (2018) found that considerable improvements in farm performance can happen in a relatively short time span of three years for part of the population. Increasing the cultivated area of a farm would require more efficient labor use. Labor constraints explain part of the current yield gap (Silva et al., 2019), and current crop choices of farmers might become more labor-constrained on larger areas. Small-scale mechanization may therefore be required to improve labor productivity (Van Loon et al., 2020), in particular if farm areas would increase to attain a living income. Lastly, apart from land, labor and capital, additional knowledge will also be needed when moving toward for instance scenario I1: improved yields. Marinus et al. (2021) for instance describe how farmers required knowledge on specific intercropping arrangements for maize and legumes when maize growth became prolific—reaching 50% of the water-limited yield level—and thereby smothering intercropped legumes.
At the national or regional level, if farms would grow in area to attain a living income from farming, there is insufficient land available for all households without massive expansion of the area under agriculture. For instance, moving from current farm areas to the viable farm areas as calculated in scenario B2: baseline yields - costs, would require farm areas that are 440, 130, and 260% that of the current, median cultivated area in Nyando, Rakai, and Lushoto, respectively. Hence, for all farming households to be able to attain a living income, off-farm employment would be needed for those who leave farming (Koning, 2017; Giller, 2020). In the study sites, off-farm income sources contributed less to incomes than crop and livestock production. Land is currently unequally distributed, and the poorest and smallest farms are in an unfavorable competitive position (Chamberlin and Jayne, 2020). Competition for land may further marginalize the smallest farms in future, while the largest grow (Headey and Jayne, 2014; Jayne et al., 2014, 2021).
Concluding Remarks
Our study is the first to use the living income to establish what would be a “viable farm size,” as a benchmark for smallholder farming. We applied the approach in three sites with contrasting farming systems and explored scenarios, which considered crop intensification strategies, income from livestock and off-farm income to explore which households could achieve a living income. With current yields, cultivated areas would have to increase considerably to attain a living income from crops: for instance to more than four times the median cultivated area in Nyando. Intensification scenarios indicated that feasible yield increases would lift 70% of the households to a living income on their current cultivated area. Only in Nyando would also other sources of income, such as livestock, be needed for the majority of the population to attain a living income from farming. Households who are unable to earn a living income from farming would need social protection for the poorest, and alternative employment for those who choose to step out of farming.
In this study we highlight the current constraints faced by farming households, rather than to propose the explored scenarios as pathways for rural development. Clearly fundamental changes in the institutional and policy environment are needed to address both rural poverty and the need to increase agricultural productivity to meet the national food demand of countries in SSA in the face of rapid population growth. The viable farm size methodology may be a useful tool in understanding what is required for smallholder farming to provide a decent living.
Data Availability Statement
Publicly available datasets were analyzed in this study. This data can be found here: https://doi.org/10.1038/s41597-020-0388-8.
Ethics Statement
The data originated from van van Wijk et al. (2020), who state the following: the data collection efforts conformed with the principles of the 1964 WMA declaration of Helsinki. Ethical approvals for the survey applications was obtained by the Internal Ethical Review Committees of the different institutes [e.g., the Internal Review Ethics Committee (IREC) of the International Livestock Research Institute] or for those partners without an Internal Ethical Committee, by ethical evaluation by the senior management at each organization after careful evaluation of the content, methodology, and with oral informed consent statement built-in to the survey. The patients/participants provided their written informed consent to participate in this study.
Author Contributions
WM, ET, MW, KD, GV, BV, and KG designed research and wrote the paper. WM and ET performed research and analyzed data. MW supplied data. All authors contributed to the article and approved the submitted version.
Funding
We gratefully acknowledge the CGIAR Research Program MAIZE (https://maize.org/), the CGIAR Research Program on Livestock (https://livestock.cgiar.org/) and the Plant Production Systems group of Wageningen University for funding. KG acknowledges support from the NWO-WOTRO Strategic Partnership NL-CGIAR.
Conflict of Interest
The authors declare that the research was conducted in the absence of any commercial or financial relationships that could be construed as a potential conflict of interest.
Publisher's Note
All claims expressed in this article are solely those of the authors and do not necessarily represent those of their affiliated organizations, or those of the publisher, the editors and the reviewers. Any product that may be evaluated in this article, or claim that may be made by its manufacturer, is not guaranteed or endorsed by the publisher.
Supplementary Material
The Supplementary Material for this article can be found online at: https://www.frontiersin.org/articles/10.3389/fsufs.2021.759105/full#supplementary-material
References
Abate, T., Shiferaw, B., Menkir, A., Wegary, D., Kebede, Y., Tesfaye, K., et al. (2015). Factors that transformed maize productivity in Ethiopia. Food Secur. 7, 965–981. doi: 10.1007/s12571-015-0488-z
Anker, R. (2011). Estimating a Living Wage: A Methodological Review. Geneva: International Labour Office.
Anker, R., and Anker, M. (2017a). Living Wage Report Kenya With a Focus on Rural Mount Kenya Area - Context Provided in Horticulture Industry. Global Living Wage Coalition. Available online at: https://www.globallivingwage.org/living-wage-benchmarks/rural-kenya/ (accessed June 7, 2021).
Anker, R., and Anker, M. (2017b). Living Wages Around the World - Manual for Measurement. Northampton: Edward Elgar Publishing. Available online at: https://www.elgaronline.com/view/9781786431455/9781786431455.xml (accessed May 31, 2021).
Beuchelt, T. (2016). “Gender, social equity and innovations in smallholder farming systems: pitfalls and pathways,” in Technological And Institutional Innovations For Marginalized Smallholders In Agricultural Development (Springer, Cham), 181–198.
Chamberlin, J., and Jayne, T. S. (2020). Does farm structure affect rural household incomes? Evidence from Tanzania. Food Policy 90:101805. doi: 10.1016/j.foodpol.2019.101805
Chen, S., and Ravallion, M. (2010). The developing world is poorer than we thought, but no less successful in the fight against poverty. Q. J. Econ. 125, 1577–1625. doi: 10.1162/qjec.2010.125.4.1577
CIESIN (2018). Gridded Population of the World, Version 4 (GPWv4): Population Density, Revision 11. Center for International Earth Science Information Network, New York: Colombia University.
Djurfeldt, A. A., and Wambugu, S. K. (2011). In-kind transfers of maize, commercialization and household consumption in Kenya. J. East. African Stud. 5, 447–464. doi: 10.1080/17531055.2011.611671
Dorward, A., Anderson, S., Bernal, Y. N., Vera, E. S., Rushton, J., Pattison, J., et al. (2009). Hanging in, stepping up and stepping out: livelihood aspirations and strategies of the poor. Dev. Pract. 19, 240–247. doi: 10.1080/09614520802689535
EU (2020). Communication From the Commission to the European Parliament, the Council, the European Economic and Social Committee and the Committee of the Regions - Recommendations to the Member States as Regards Their Strategic Plan for the Common Agricultural Polic. COM(2020) 846 Final. Brussels: European Commission.
Fermont, A. M., van Asten, P. J. A., and Giller, K. E. (2008). Increasing land pressure in East Africa: the changing role of cassava and consequences for sustainability of farming systems. Agric. Ecosyst. Environ. 128, 239–250. doi: 10.1016/j.agee.2008.06.009
Fraval, S., Hammond, J., Lannerstad, M., Oosting, S. J., Sayula, G., Teufel, N., et al. (2018). Livelihoods and food security in an urban linked, high potential region of Tanzania: changes over a three year period. Agric. Syst. 160, 87–95. doi: 10.1016/j.agsy.2017.10.013
Fraval, S., Hammond, J., Wichern, J., Oosting, S. J., de Boer, I. J. M., Teufel, N., et al. (2019). Making the most of imperfect data: a critical evaluation of standard infrmation collect in farm household surveys. Exp. Agric. 55, 230–250. doi: 10.1017/S0014479718000388
Frelat, R., Lopez-Ridaura, S., Giller, K. E., Herrero, M., Douxchamps, S., Djurfeldt, A. A., et al. (2016). Drivers of household food availability in sub-Saharan Africa based on big data from small farms. Proc. Natl. Acad. Sci. 113, 458–463. doi: 10.1073/pnas.1518384112
Funk, C., Peterson, P., Landsfeld, M., Pedreros, D., Verdin, J., Shukla, S., et al. (2015). The climate hazards infrared precipitation with stations - A new environmental record for monitoring extremes. Sci. Data 2, 1–21. doi: 10.1038/sdata.2015.66
Gassner, A., Harris, D., Mausch, K., Terheggen, A., Lopes, C., Finlayson, R. F., et al. (2019). Poverty eradication and food security through agriculture in Africa: rethinking objectives and entry points. Outlook Agric. 48, 309–315. doi: 10.1177/0030727019888513
Giller, K. E. (2020). The food security conundrum of sub-Saharan Africa. Glob. Food Sec. 26:100431. doi: 10.1016/j.gfs.2020.100431
Giller, K. E., Delaune, T., Vasco, J., Wijk, M., Van Hammond, J., Descheemaeker, K., et al. (2021). Small farms and development in sub - Saharan Africa: farming for food, for income or for lack of better options? Glob. Food Secur. doi: 10.1007/s12571-021-01209-0
Hammond, J., Fraval, S., van Etten, J., Suchini, J. G., Mercado, L., Pagella, T., et al. (2017). The Rural Household Multi-Indicator Survey (RHoMIS) for rapid characterisation of households to inform climate smart agriculture interventions: description and applications in East Africa and Central America. Agric. Syst. 151, 225–233. doi: 10.1016/j.agsy.2016.05.003
Harris, D., and Orr, A. (2014). Is rainfed agriculture really a pathway from poverty? Agric. Syst. 123, 84–96. doi: 10.1016/j.agsy.2013.09.005
Headey, D. D., and Jayne, T. S. (2014). Adaptation to land constraints: is Africa different? Food Policy 48, 18–33. doi: 10.1016/j.foodpol.2014.05.005
Herrmann, S. M., and Mohr, K. I. (2011). A continental-scale classification of rainfall seasonality regimes in Africa based on gridded precipitation and land surface temperature products. J. Appl. Meteorol. Climatol. 50, 2504–2513. doi: 10.1175/JAMC-D-11-024.1
Jayne, T. S., Chamberlin, J., and Headey, D. D. (2014). Land pressures, the evolution of farming systems, and development strategies in Africa: a synthesis. Food Policy 48, 1–17. doi: 10.1016/j.foodpol.2014.05.014
Jayne, T. S., Chamberlin, J., Holden, S., Ghebru, H., Ricker-Gilbert, J., and Place, F. (2021). Rising land commodification in sub-Saharan Africa: reconciling the diverse narratives. Glob. Food Sec. 30:100565. doi: 10.1016/j.gfs.2021.100565
Jayne, T. S., Mason, N. M., Burke, W. J., and Ariga, J. (2018). Review: taking stock of Africa's second-generation agricultural input subsidy programs. Food Policy 75, 1–14. doi: 10.1016/j.foodpol.2018.01.003
Koning, N. (2017). Food Security, Agricultural Policies and Economic Growth: Long-Term Dynamics in the Past, Present and Future. Abingdon: Routledge.
Kung'u, J. B., and Namirembe, S. (2012). The Nyando Atlas: Mapping out the Ecosystem Condition by Agro Ecological Landscape of Nyando River Basin. Nairobi: World Agroforestry Centre. Available online at: http://www.academia.edu/download/36811806/The-Nyando-Atlas1.pdf (accessed May 31, 2021).
Kyazze, F. B., and Kristjanson, P. (2011). Summary of Baseline Household Survey Results: Rakai District, South Central Uganda. Copenhagen, Denmark: CGIAR Research Program on Climate Change, Agriculture and Food Security (CCAFS).
LaRue, K., Daum, T., Mausch, K., and Harris, D. (2021). Who wants to farm? Answers depend on how you ask: a case study on youth aspirations in Kenya. Eur. J. Dev. Res 33, 885–909. doi: 10.1057/s41287-020-00352-2
Living Income Community of Practice (2021). Towards a Decent Standard of Living for Smallholder Farmers. Available online at: https://www.living-income.com (accessed August 3, 2021).
Lyamchai, C., Yanda, P., Sayula, G., and Kristjanson, P. (2011). Summary of Baseline Household Survey Results: Lushoto, Tanzania. Copenhagen, Denmark: CGIAR Research Program on Climate Change, Agriculture and Food Security (CCAFS).
Mango, J., Mideva, A., Osanya, W., and Odhiambo, A. (2011). Summary of Baseline Household Survey Results: Lower Nyando, Kenya. Copenhagen, Denmark: CGIAR Research Program on Climate Change, Agriculture and Food Security (CCAFS). Available online at: https://hdl.handle.net/10568/16427 (accessed May 31, 2021).
Marinus, W. (2021). It is time to harvest - towards sustainable farming systems in the East African highlands. PhD thesis, Wageningen University, The Netherlands.
Marinus, W., Descheemaeker, K. K. E., van de Ven, G. W. J., Waswa, W., Mukalama, J., Vanlauwe, B., et al. (2021). “That is my farm” – An integrated co-learning approach for whole-farm sustainable intensification in smallholder farming. Agric. Syst. 188:103041. doi: 10.1016/j.agsy.2020.103041
MoALF (2016). 2014/15 Annual Agricultural Sample Survey Report. Tanzania: Ministry of Agriculture, Livestock and Fisheries (MoALF). Available online at: https://nbs.go.tz/nbs/takwimu/Agriculture/2016_17_AASS_report.pdf (accessed June 8, 2021).
Nin-Pratt, A., and McBride, L. (2014). Agricultural intensification in Ghana: evaluating the optimist's case for a Green Revolution. Food Policy 48, 153–167. doi: 10.1016/j.foodpol.2014.05.004
OECD (2011). What Are Equivalence Scales? 2 p. Available online at: http://www.oecd.org/els/soc/OECD-Note-EquivalenceScales.pdf (accessed August 9, 2020).
Olinto, P., Beegle, K., Sobrado, C., and Uematsu, H. (2013). The state of the poor: Where are the poor, where is extreme poverty harder to end, and what is the current profile of the world's poor. Economic premise 125, 1–8.
Ramisch, J. J. (2014). “We will not farm like our fathers did' Multilocational livelihoods, cellphones, and the continuing challenge of rural development in in western Kenya,” in Rural Livelihoods, Regional Economies, and Processes of Change, ed. D. Sick (London: Wiley), 103–189. doi: 10.13140/2.1.1557.6003
Ravallion, M., Datt, G., and van de Walle, D. (1991). Quantifying absolute poverty in the developing world. Rev. Income Wealth 37, 345–361. doi: 10.1111/j.1475-4991.1991.tb00378.x
Sakané, N., Becker, M., Langensiepen, M., and Van Wijk, M. T. (2013). Typology of smallholder production systems in small east-African wetlands. Wetlands 33, 101–116. doi: 10.1007/s13157-012-0355-z
Sanchez, P., Palm, C., Sachs, J., Denning, G., Flor, R., Harawa, R., et al. (2007). The African Millennium Villages. Proc. Natl. Acad. Sci. 104, 16775–16780. doi: 10.1073/pnas.0700423104
Silva, J. V., Baudron, F., Reidsma, P., and Giller, K. E. (2019). Is labour a major determinant of yield gaps in sub-Saharan Africa? A study for cereal-based production systems in Southern Ethiopia. Agric. Syst. 174, 39–51. doi: 10.1016/j.agsy.2019.04.009
Spielman, D. J., Byerlee, D., Alemu, D., and Kelemework, D. (2010). Policies to promote cereal intensification in Ethiopia: the search for appropriate public and private roles. Food Policy 35, 185–194. doi: 10.1016/j.foodpol.2009.12.002
Sumberg, J., Szyp, C., Yeboah, T., Oosterom, M., Crossouard, B., and Chamberlin, J. (2021). “Young people and the rural economy: syntheses and implications,” in Youth and the Rural Economy in Africa: Hard Work and Hazard, ed J. Sumberg (Wallingford: CABI), 173–180.
Tavenner, K., van Wijk, M., Fraval, S., Hammond, J., Baltenweck, I., Teufel, N., et al. (2019). Intensifying inequality? Gendered trends in commercializing and diversifying smallholder farming systems in East Africa. Front. Sustain. Food Syst. 3, 1–14. doi: 10.3389/fsufs.2019.00010
Tittonell, P., Vanlauwe, B., Leffelaar, P. A., Shepherd, K. D., and Giller, K. E. (2005). Exploring diversity in soil fertility management of smallholder farms in western Kenya - II. Within-farm variability in resource allocation, nutrient flows and soil fertility status. Agric. Ecosyst. Environ. 110, 166–184. doi: 10.1016/j.agee.2005.04.003
Tittonell, P. A., and Giller, K. E. (2013). When yield gaps are poverty traps: the paradigm of ecological intensification in African smallholder agriculture. F. Crop. Res. 143, 76–90. doi: 10.1016/j.fcr.2012.10.007
United Nations (2015). Resolution Adopted by the General Assembly on 25 September 2015. 70/1 Transforming Our World: The 2030 Agenda for Sustainable Development. Washington DC: United Nations General Assembly.
United Nations General Assembly (1948). Universal Declaration of Human Rights. Resolution 217 A (III) of December 1948. New York, NY: United Nations.
van de Ven, G. W. J., de Valença, A., Marinus, W., de Jager, I., Descheemaeker, K. K. E., Hekman, W., et al. (2020). Living income benchmarking of rural households in low-income countries. Food Secur. 13, 1–21. doi: 10.1007/s12571-020-01099-8
van Ittersum, M. K., van Bussel, L. G. J., Wolf, J., Grassini, P., van Wart, J., Guilpart, N., et al. (2016). Can sub-Saharan Africa feed itself? Proc. Natl. Acad. Sci. 113, 14964–14969. doi: 10.1073/pnas.1610359113
Van Loon, J., Woltering, L., Krupnik, T. J., Baudron, F., Boa, M., and Govaerts, B. (2020). Scaling agricultural mechanization services in smallholder farming systems: case studies from sub-Saharan Africa, South Asia, and Latin America. Agric. Syst. 180:102792. doi: 10.1016/j.agsy.2020.102792
Van Merriënboer, J. (2019). Mansholt een biografie (Mansholt a biography). 2nd Edn. Gorredijk: Noordboek.
van Wijk, M., Hammond, J., Gorman, L., Adams, S., Ayantunde, A., Baines, D., et al. (2020). The rural household multiple indicator survey, data from 13,310 farm households in 21 countries. Sci. Data 7, 1–9. doi: 10.1038/s41597-020-0388-8
Vanlauwe, B., and Dobermann, A. (2020). Sustainable intensification of agriculture in sub-Saharan Africa: first things first. Front. Agric. Sci. Eng. 7:376. doi: 10.15302/J-FASE-2020351
Vanlauwe, B., van Asten, P., and Blomme, G. (2013). “Agro-ecological intensification of farming systems in the East and Central African highlands,” in Agro-Ecological Intensification of Agricultural Systems in the African Highlands, eds. B. Vanlauwe, P. van Asten, and G. Blomme (Abingdon: Routledge), 1–19. doi: 10.4324/9780203114742
Waha, K., van Wijk, M. T., Fritz, S., See, L., Thornton, P. K., Wichern, J., et al. (2018). Agricultural diversification as an important strategy for achieving food security in Africa. Glob. Chang. Biol. 24, 3390–3400. doi: 10.1111/gcb.14158
Wichern, J., van Wijk, M. T., Descheemaeker, K., Frelat, R., van Asten, P. J. A., and Giller, K. E. (2017). Food availability and livelihood strategies among rural households across Uganda. Food Secur. 9, 1385–1403. doi: 10.1007/s12571-017-0732-9
Wiggins, S. (2016). Agricultural and Rural Development Reconsidered - A Guide to Issues and Debates. IFAD. Available online at: https://www.ifad.org/documents/38714170/39135332/01_ODI_web.pdf/cac62a47-ff82-433c-8a99-b864056ecdbf (accessed December 10, 2021).
Keywords: household income, income distribution, livelihood strategies, scenario exploration, future farming systems, intensification, poverty
Citation: Marinus W, Thuijsman ES, van Wijk MT, Descheemaeker K, van de Ven GWJ, Vanlauwe B and Giller KE (2022) What Farm Size Sustains a Living? Exploring Future Options to Attain a Living Income From Smallholder Farming in the East African Highlands. Front. Sustain. Food Syst. 5:759105. doi: 10.3389/fsufs.2021.759105
Received: 15 August 2021; Accepted: 25 November 2021;
Published: 06 January 2022.
Edited by:
Ademola Braimoh, World Bank Group, United StatesReviewed by:
Meine van Noordwijk, World Agroforestry Centre (ICRAF), IndonesiaAlisher Mirzabaev, Center for Development Research (ZEF), Germany
Copyright © 2022 Marinus, Thuijsman, van Wijk, Descheemaeker, van de Ven, Vanlauwe and Giller. This is an open-access article distributed under the terms of the Creative Commons Attribution License (CC BY). The use, distribution or reproduction in other forums is permitted, provided the original author(s) and the copyright owner(s) are credited and that the original publication in this journal is cited, in accordance with accepted academic practice. No use, distribution or reproduction is permitted which does not comply with these terms.
*Correspondence: Wytze Marinus, wytze.marinus@wur.nl; wytzemarinus@gmail.com