- Research and Development, Ottawa Laboratory (Carling), Canadian Food Inspection Agency, Ottawa, ON, Canada
Whole-genome sequencing (WGS) technologies are rapidly being adopted for routine use in food microbiology laboratories worldwide. Examples of how WGS is used to support food safety testing include gene marker discovery (e.g., virulence and anti-microbial resistance gene determination) and high-resolution typing (e.g., cg/wgMLST analysis). This has led to the establishment of large WGS databases representing the genomes of thousands of different types of food pathogenic and commensal bacteria. This information constitutes an invaluable resource that can be leveraged to develop and validate routine test methods used to support regulatory and industry food safety objectives. For example, well-curated raw and assembled genomic datasets of the key food pathogens (Salmonella enterica, Listeria monocytogenes, and Shiga-toxigenic Escherichia coli) have been used in our laboratory in studies to validate bioinformatics pipelines, as well as new molecular methods as a prelude to the laboratory phase of the “wet lab” validation process. The application of genomic information to food microbiology method development will decrease the cost of test development and lead to the generation of more robust methodologies supporting risk assessment and risk management actions.
Introduction
Conventional “wet lab” methodologies used for detection, identification and typing of foodborne pathogenic bacteria are based on relatively fixed phenotypic properties, such as cell surface antigens and biochemical capabilities, as well as defined genetic traits, such as portions of well-characterized gene sequences that are unique to a given species or subtype. The phenotypic attributes of bacteria lend themselves to the elaboration of target-specific enrichment and recovery techniques (Blais et al., 2019), as well as analytical methods intended for the identification and typing of bacteria for risk assessment and risk management purposes. In addition, certain genetic features such as virulence genes and phylogenetic markers can be determined using highly specific genetic techniques such as polymerase chain reaction (PCR), DNA probes and sequencing (Blais et al., 2012; Huszczynski et al., 2013; Carrillo et al., 2016). Such analyses can generally be carried out using “home-made” reagents and procedures, or using commercially available test kits and devices, placing them within easy reach of most food microbiology laboratories. However, before any method can be put into practice in support of food safety objectives—particularly where regulatory risk management outcomes are indicated—certain quality requirements must be met, such as method validation to ensure fitness-for-purpose in the intended application. Traditionally, the so-called “wet lab” methods are subjected to rigorous method validation schemes [e.g., Microbiological Methods Committee (MMC) criteria for Canadian regulatory test methods, Microbiological Methods (Microbiological Methods Committee., 2011, 2016)], and therefore, tend to be locked in a highly prescriptive form with limited scope for flexibility of application and adaptation in addressing novel food safety scenarios.
Generally, microbiology methods encompass the gamut of techniques from enrichment and recovery of the target organism as purified colonies on plating media, to their identification and detailed characterization using biochemical, antigenic, and genetic approaches. More recently, whole-genome sequence (WGS) analysis of colony isolates of foodborne bacterial pathogens has enabled high-resolution characterization to underpin regulatory decisions (Allard et al., 2016; Lindsey et al., 2016; Nadon et al., 2017). Implementation of genomic technologies in food and clinical microbiology laboratories has led to the publication of hundreds of thousands of genomes of foodborne pathogens that can be leveraged for the development and validation of food microbiology methodology, as well as for verifying bioinformatics approaches for pathogen risk characterization (Carrillo et al., 2012; Angers-Loustau et al., 2018; Petrillo et al., 2021). For present purposes, we shall limit our discussion to methods used for the detection, identification and characterization of colony isolates recovered from foods.
Role of Genomics and Whole Genome Sequencing in Food Microbiology
The introduction of PCR technology in the analytical microbiology laboratory over three decades ago has served to redefine the basis for the identification of bacteria from a phenotypic standard to one based on the genotype (Blais et al., 2012, 2013; Huszczynski et al., 2013). The relatively recent implementation of next-generation sequencing technologies has opened new possibilities for conducting detailed analyses of foodborne bacteria; for example, WGS can now produce an entire bacterial genome much faster and at a significantly lower cost than previously possible, making it feasible to sequence isolates in near real-time under certain circumstances (e.g., during foodborne illness outbreak investigations) (Joensen et al., 2014; Lambert et al., 2015; Allard et al., 2016; Ronholm et al., 2016; Carrillo et al., 2020).
Sequencing pathogenic bacteria, whether in the context of outbreak investigations or information gathering in the course of research, can yield an unprecedented level of information regarding the presence of virulence and other marker genes relevant to the identification and risk characterization of food isolates (Joensen et al., 2014, 2015; Kleinheinz et al., 2014; Allard et al., 2016; Carrillo et al., 2016, 2020; Lindsey et al., 2016; Ronholm et al., 2016; Yoshida et al., 2016). WGS data can provide an exquisite degree of resolution in ascertaining differences between strains and determining phylogenetic relationships among different bacterial isolates for precise attribution of contamination sources (Allard et al., 2016; Ronholm et al., 2016; Carrillo et al., 2020). Finally, the identification of strain-specific features such as unique DNA sequences, metabolic properties and antimicrobial resistance (AMR) enables testing laboratories to deploy customized tests addressing specific strains of interest in determining the scope of contamination events (Blais et al., 2019), all while incorporating quality control features and approaches (Lambert et al., 2017; Low et al., 2019) upholding the reliability of analyses.
Canadian Food Inspection Agency (CFIA) food microbiology testing programs primarily targeting Salmonella enterica, Listeria monocytogenes, and Shiga toxin-producing Escherichia coli (STEC) now routinely include WGS analysis of bacterial isolates using bioinformatics workflows such as the GeneSeekr pipeline (Carrillo et al., 2020) which incorporates modules for phylogenetic, virulence, serotype and AMR markers for hazard characterization, sub-typing analysis for trace-back investigations, and quality control features to ensure that sequence data meets quality and purity (Low et al., 2019) criteria for the intended purpose. Broadly speaking, the types of analytical objectives for bioinformatics in the context of regulatory food microbiology fall into two categories: (1) gene marker discovery (e.g., virulence and AMR gene determination) and (2) high resolution typing (e.g., SNPs, cg/wgMLST, etc.).
Why Genomic Analyses Are Different
In the implementation of any novel technology for regulatory purposes, important considerations such as harmonization, validation and quality assurance need to be addressed. WGS technologies pose unique challenges in part due to their reliance on bioinformatics for data processing and interpretation. Bioinformatics tools and analytical applications may vary due to (1) differences in local needs, (2) individual-specific approaches to the development of algorithms and bioinformatics workflows, (3) variable and site-specific nature of computers, their environments and dependencies (Tong et al., 2015; Lambert et al., 2017). Thus, these types of analytical methods are not amenable to conventional prescriptive approaches for tabling test protocols and validating their performance characteristics to meet national MMC, (Microbiological Methods Committee., 2011, 2016) and international (International Organization for Standardization, Association Française de Normalization, Association of Official Analytical Chemists, etc.) guidelines, which were developed for traditional “wet lab” methods where all aspects of reagents, materials and conditions can be standardized and controlled to ensure consistency and reproducibility among different users.
Genomic methods differ from traditional “wet-lab” approaches in that they offer virtually unlimited opportunities to conduct detailed analyses of pathogens, inviting “custom” queries to answer novel questions arising within a specific food investigative context. WGS-based methods require flexibility and plasticity to adapt to new problems on a case-by-case basis if their full potential is to be realized. Therefore, a different approach will be required to expedite the performance validation of bioinformatics workflows and new analytical modules which may be added from time to time, or ad hoc queries made in the course of a food safety investigation.
Benchmarking With Genomic Datasets
Benchmarking studies using WGS datasets are increasingly being used to assess performance of computational tools used for sequence analysis (Timme et al., 2017; Angers-Loustau et al., 2018; Weber et al., 2019). For example, an international consortium of researchers organized by the European Commission Joint Research Center is currently addressing the problem of standardization of genomic AMR analyses, giving rise to the proposition that well-designed benchmarking resources are the best means of evaluating, validating and ensuring continued quality control over the bioinformatics component of the process (Angers-Loustau et al., 2018; Petrillo et al., 2021). In the proposed approach, rather than attempting to rigidly control the bioinformatics tools used to conduct genomic analyses, the emphasis is on the distribution of curated, high-quality genomic datasets (e.g., bacterial WGS data) to users so that they may demonstrate their analytical proficiency using a set of standardized test samples, rather than the tests themselves being standardized. Similarly, genomic datasets for assessing performance of phylogenomic pipelines for food safety investigations have been developed and applied to the validation of methods for foodborne pathogen surveillance in the United States (Timme et al., 2017). Such a proficiency verification approach is commonly used in quality assurance schemes for analytical chemistry and microbiology testing laboratories, which may use test methods of their choice, as dictated by local capabilities, but must periodically demonstrate their ability to arrive at the “correct answer” through analyses of “blind” panels of test samples distributed through a central reference agency.
In the case of genomics, the use of benchmark datasets for this purpose is highly advantageous in that these are in silico rather than biological in nature, and hence, much less expensive to produce, distribute, and are not subject to variations which may occur with biological materials as a result of sample preparation heterogeneity, shipping conditions, etc. Curated benchmark WGS datasets could prove a valuable adjunct in helping regulatory testing agencies such as the CFIA meet national and international requirements for the use of validated methods and would support a performance-based approach to the harmonization of bioinformatics test routines.
Development of Benchmark Datasets for Computational and “Wet Lab” Method Validation
While the importance of benchmark datasets for the validation of bioinformatics methods is clear, they can equally play a role in the evaluation of “wet lab” methodology. This data could be used in the development of new molecular methods and review of current methods falling within the purview of standard setting organizations in Canada and abroad. For example, PCR techniques where primers could be validated electronically against the relevant benchmark datasets as an adjunct to their validation using biological materials. In both scenarios, datasets must be carefully designed to ensure suitability for intended use.
Current Standards for Method Validation
The International Standards Organization standard (ISO/IEC 17025:2017) on method validation states that “the laboratory shall validate non-standard methods, laboratory-developed methods and standard methods used outside their intended scope or otherwise modified. The validation shall be as extensive as is necessary to meet the needs of the given application or field of application.” In Canada, guidelines for the validation of methods used to support regulatory food microbiology objectives are issued by the MMC, which produces the Compendium of Analytical Methods (CAM) tabling validated method protocols for the determination of pathogenic microorganisms in foods. The CAM includes chapters outlining the requirements for method validation and is based on national and international procedures, standards and protocols from ISO, AOAC, Health Canada and the CFIA (Microbiological Methods Committee., 2011, 2016). For colony identification methods, the main objective of validation studies is to determine salient performance characteristics such as inclusivity (i.e., the ability of the method to determine expected features of the target community, generally verified using a panel of at least 100 target strains for most bacteria), exclusivity (i.e., the property of not generating false positive test results or “signals” using a panel of at least 50 non-target bacteria which may be present in food samples and/or cause interference with the detection of target bacteria), and reproducibility (e.g., generating similar results using different media, in different laboratories).
For these types of studies care must be taken to include well-characterized strains (e.g., strains implicated in foodborne outbreaks) that reflect the diversity of the target organism. For genus level methods (e.g., Salmonella spp.), strains representing different species (e.g., S. bongori), subspecies (e.g., S. enterica subsp. houtenae) and further subgroups (e.g., serovars) should be selected, with an emphasis on the use of a variety of strains, serotypes, genotypes or species relevant to the food categories falling within the scope of the method. Non-target bacteria bearing properties that might confound the correct identification of the target organism (e.g., similarities in terms of displayed antigens, metabolic properties or DNA sequences) should be included in the study. For example, depending on which features are targeted by the assay, difficulties may be encountered in distinguishing L. monocytogenes from L. innocua, or commensal E. coli from STEC. The CAM sets out the criteria for microbiological method performance as follows: sensitivity ≥98%; specificity ≥90.4%; false negative rate < 2%; false positive rate ≤ 9.6%; and efficacy ≥94%; and for methods intended as alternatives to established methods the level of detection must be comparable to or exceed the lower limit of detection of the reference method (Microbiological Methods Committee., 2011). More robust criteria are applied to colony identification methods which require a false negative rate ≤ 1% and a false positive rate ≤ 2.0% (Microbiological Methods Committee., 2016).
Considerations for the Assembly of Benchmark Datasets for Method Validation
We have been working on the production of well-curated raw and assembled genomic datasets of the key pathogens (S. enterica, L. monocytogenes, and STEC) which are the subject of CFIA food microbiology inspection programs (Table 1). Datasets are used to validate bioinformatics processes, thus ensuring that they meet a common performance standard. Strains included in these datasets have also been used to support validation of “wet-lab” methods (e.g., PCR). In addition to pathogen-specific genomic datasets, well-curated datasets of commensal bacteria known to be associated with the types of food commodities typically tested for the above-specified pathogens are being developed to serve in the evaluation of exclusivity characteristics of methods (Blais et al., 2012, 2014). For example, as part of an on-going program of food microbiological test method development and validation in our laboratory, we have undertaken an initiative to characterize and sequence the main bacterial species associated with different food matrices commonly subjected to regulatory inspection, including beef and beef products, leafy greens and sprouts (cf. NCBI bioproject PRJNA254477, Manninger et al., 2016).
The design of these datasets is intended to ensure suitability for method validation purposes. Inclusivity panels for should be composed of genetically diverse bacterial isolates, ideally consisting of more strains than the minimum number required by validation bodies (Microbiological Methods Committee., 2016; Carlin et al., 2020). Wherever possible strains included in datasets should span the spectrum of diversity in terms of salient characteristics for the organism under consideration which are known to occur in the target commodities. For example, STEC panels should include a variety of different serotypes and sequence types (e.g., MLST) implicated in human illness as well as those occurring in animal reservoirs (which can be garnered form public health and national surveillance databases), and efforts should be made to include outliers (e.g., phylogenetically distant) individuals to test the scope of applicability for the subject methodologies. Both internal (e.g., CFIA historical food isolate collection) and external (e.g., public repositories) databases should be mined to ensure the selection of representative isolates. An appropriate subset of the strains represented in the assembled database (e.g., from the in-house culture collection) should be well-characterized by both conventional and genomic methodologies to ensure their authenticity and typical behavior in the wet-lab. Wherever possible, at least a subset of strains should be available for distribution to other testing laboratories to promote standardization and foster true equivalency of different methods.
Sequence Quality
Datasets composed of draft genome assemblies should conform to established minimum quality standards (e.g., PHRED quality scores, depth of genome coverage, and assembly metrics) (Gurevich et al., 2013). In addition, some considerations for the curation of benchmark WGS datasets might include verification that samples are not contaminated (Low et al., 2019), and that there are minimal gaps (e.g., < 100 bp) in genome coverage (Lambert et al., 2015). Draft genome assemblies may not be appropriate in cases where method targets are likely to be present in multiple copies (e.g., rRNA genes, Shiga toxin genes) or occur within repetitive DNA regions as de novo assembly of draft genomes generated with short-read sequence technologies often cannot resolve repeat regions (Utturkar et al., 2017). In these cases, use of complete polished genomes may be preferable. Raw reads can be simulated from closed genomes (Huang et al., 2012), thus ensuring that datasets are not compromised due to undefined quality issues (Timme et al., 2017; Low et al., 2019).
Maximizing Diversity
Selection of samples to include in a benchmark dataset should be done with the aim of maximizing the phylogenetic, genotypic and phenotypic diversity. To develop phylogenetically diverse datasets, we have implemented StrainChoosr (https://github.com/OLC-Bioinformatics/StrainChoosr) to select a subset of samples from a user defined list of sequences (Pardi and Goldman, 2005; Steel, 2005). This ultimately helps to ensure removal of highly similar samples, while reducing datasets to manageable sizes for intended analyses. An example of the use of this tool to identify a diverse subset of 15 S. enterica serovar Enteritidis from a panel of 79 strains available in the laboratory is provided (red text, Figure 1). Datasets should further be curated to ensure the diversity of important genetic markers such as AMR genes, or virulence factors such as STEC Shiga toxin subtypes are captured (Scheutz et al., 2012). Wherever possible strains with unusual features that are known to confound certain types of determinations (e.g., new STEC Shiga toxin subtypes) should be included. Similarly, phenotypic diversity should be captured by ensuring that the panel includes strains with representative phenotypes as determined by methods such as conventional biochemical assays/PCR and includes strains with unusual phenotypic profiles.
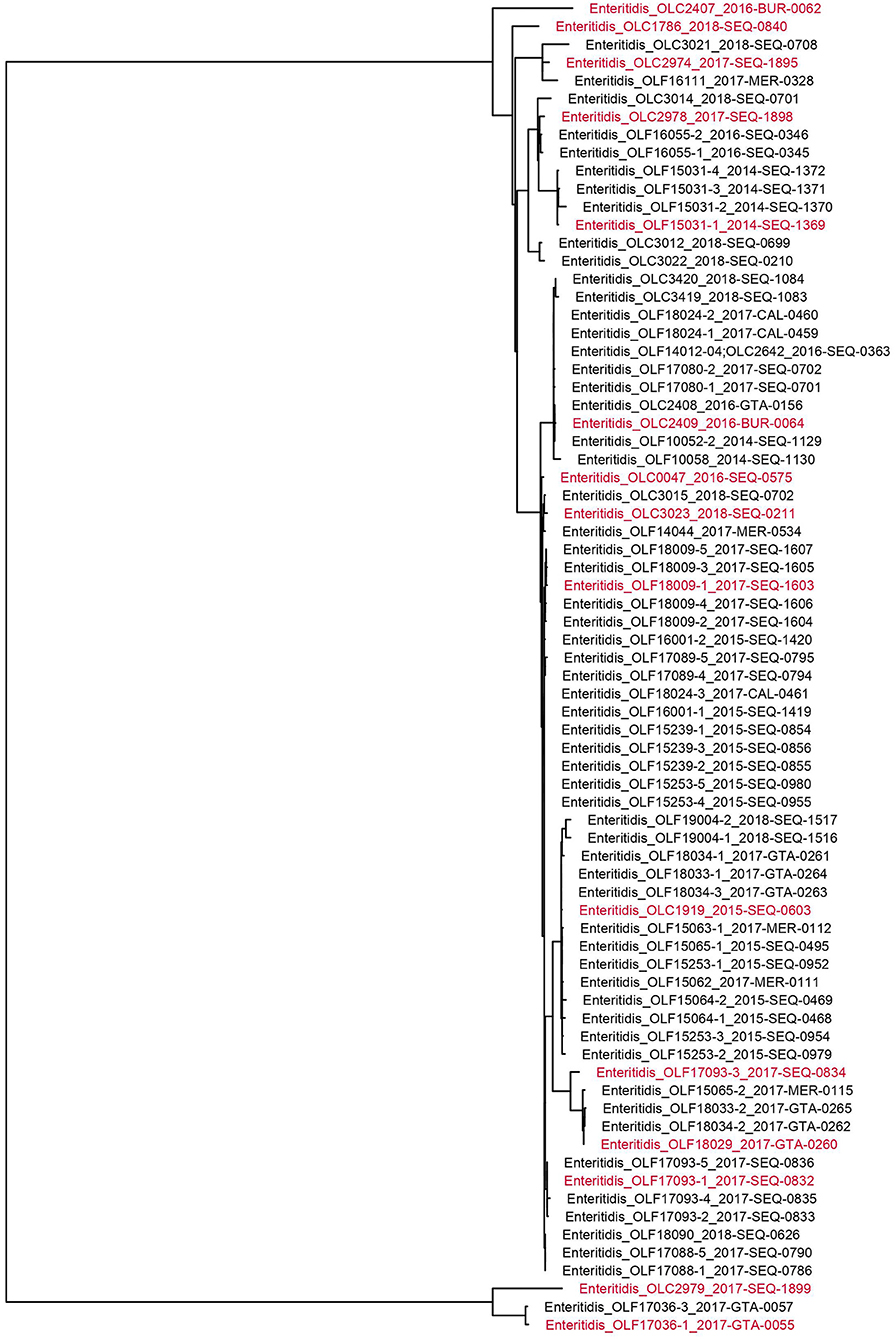
Figure 1. Selection of genetically diverse isolates of S. enteritidis with StrainChoosr. A phylogenetic tree was generated from a set of 79 S. enteritidis strains using Mashtree to cluster genomes with a neighbor-joining algorithm (Katz et al., 2019). The 15 most diverse strains were selected based on genetic distance among isolates (highlighted in red).
Confirmation of Phenotype Information
In the case where a genomic dataset is being established for the validation of methods for prediction of phenotypic properties, it is critical to further verify selected panels. For example, we developed a panel of S. enterica genomes for benchmarking tools for AMR prediction from genomic sequence data and identified discrepancies in genotype predictions relative to previously assessed phenotypes (Cooper et al., 2020). Through repeat testing we were able to confirm sequence-based predictions, and update metadata associated with the dataset, thus improving the reliability of the panel.
In-house vs. Public Databases as Sources of Benchmark Datasets
The main advantage of including in-house strains in benchmark datasets includes control over quality of the entire process, from handling of isolates, sequencing and data processing, as well as precise knowledge of source and the attendant factors of geographic, temporal and public health provenance. Furthermore, for datasets derived from in-house culture collections, genotypic features can be verified against phenotypes ensuring validity of observed discrepancies (Cooper et al., 2020). Disadvantages include limited capacity to identify and sequence a large representative number of isolates, especially for some bacterial types which occur infrequently, and the significant costs associated with a large scale sequencing operation, storage maintenance and distribution of the collection.
Public databases such as NCBI are repositories for huge collections of WGS data for various bacteria, including most that are of interest to the food microbiology laboratorian, and are an incredibly rich resource representing a huge diversity of microorganisms that have been collected around the world. For example, at the time of this writing, the NCBI Pathogen Detection database contained a total of 183220 E. coli/Shigella, 367745 S. enterica, and 43230 L. monocytogenes genomes (NCBI Pathogen Detection Database, n.d.). These databases are freely available, and offer many tools that are useful for genome analysis. Their main shortcomings include a lack of metadata which can make source identification and comparisons difficult and potential sequence data quality issues (e.g., incomplete or contaminated sequence).
Discussion
The main benefits of benchmark datasets for the regulatory testing laboratory is that they provide (1) a new resource for validation of WGS-based analytical tools to meet performance requirements for regulatory testing supporting risk assessment and risk management actions; (2) a new tool that can be readily distributed to federal, provincial and international jurisdictions to harmonize standards and underpin confidence in the performance of WGS labs delivering results impacting critical public health and food trade; and (3) a resource to enhance validation protocols for all food microbiology test methods through provision of standardized, curated bacterial strains with desired characteristics. Challenges of developing and maintaining such databases include the need to update these resources as new strains are discovered, lack of representation of strains from countries with insufficient resources to complete these analyses.
WGS has been a disruptive technology for the food microbiology laboratory, providing better and more comprehensive characterization of foodborne pathogens. Publicly available data from thousands of genomes can now be used as part of the food microbiology “tool box” to inform the development of improved methods for food testing. The establishment of well-curated, high-quality benchmark WGS datasets for key food pathogens and commensal food bacteria will provide a highly accessible resource for the performance verification of new laboratory methods, including bioinformatics workflows and modules, promoting international harmonization of method validation standards. This could be particularly critical to maintain the open-ended potential of WGS technology, which must remain highly plastic and adaptable to novel queries and interpretations, thus prescriptive approaches such as used for conventional wet lab methodology should avoided.
Data Availability Statement
The original contributions presented in the study are included in the article/supplementary material, further inquiries can be directed to the corresponding author.
Author Contributions
BB and CC conceived the study and contributed equally to the preparation of the manuscript. All authors contributed to the article and approved the submitted version.
Funding
This study has received funding from the Government of Canada Genomic Research and Development Initiative (GRDI).
Conflict of Interest
The authors declare that the research was conducted in the absence of any commercial or financial relationships that could be construed as a potential conflict of interest.
Publisher's Note
All claims expressed in this article are solely those of the authors and do not necessarily represent those of their affiliated organizations, or those of the publisher, the editors and the reviewers. Any product that may be evaluated in this article, or claim that may be made by its manufacturer, is not guaranteed or endorsed by the publisher.
Acknowledgments
We gratefully acknowledge assistance from Dr. Adam Koziol, Andrew Low and Julie Shay for bioinformatics analyses, Ray Allain, Martine Dixon, and Mylène Deschênes, for technical assistance, as well as Drs. Ashley Cooper and Lisa Hodges for critical review of the manuscript.
References
NCBI Pathogen Detection Database. (n.d.). Available online at: https://www.ncbi.nlm.nih.gov/pathogens/ (accessed November 12, 2021).
Allard, M. W., Strain, E., Melka, D., Bunning, K., Musser, S. M., Brown, E. W., et al. (2016). Practical value of food pathogen traceability through building a whole-genome sequencing network and database. J. Clin. Microbiol. 54, 1975–1983. doi: 10.1128/JCM.00081-16
Angers-Loustau, A., Petrillo, M., Bengtsson-Palme, J., Berendonk, T., Blais, B., Chan, K-G, et al. (2018). The challenges of designing a benchmark strategy for bioinformatics pipelines in the identification of antimicrobial resistance determinants using next generation sequencing technologies. F1000Research. 7:459. doi: 10.12688/f1000research.14509.1
Blais, B., Deschênes, M., Huszczynski, G., and Gauthier, M. (2014). Enterohemorrhagic Escherichia coli colony check assay for the Identification of Serogroups O26, O45. O103, O111, O121, O145, and O157 colonies isolated on plating media. J. Food Prot. 77, 1212–8. doi: 10.4315/0362-028X.JFP-13-555
Blais, B. W., Gauthier, M., Deschênes, M., and Huszczynski, G. (2012). Polyester cloth-based hybridization array system for identification of enterohemorrhagic Escherichia coli serogroups O26, O45, O103, O111, O121, O145, and O157. J. Food Prot. 75, 1691–1697. doi: 10.4315/0362-028X.JFP-12-116
Blais, B. W., Gauthier, M., Deschenes, M., and Huszczynski, G. (2013). “Characterization of verotoxigenic Escherichia coli O157, H7. Colonies by polymerase chain reaction (PCR) and cloth-based hybridization array system (CHAS) (MFLP-22),” in: Compendium of Analytical Methods (Ottawa, ON: Health Canada). Available online at: https://www.canada.ca/en/health-canada/services/food-nutrition/research-programs-analytical-methods/analytical-methods/compendium-methods.html
Blais, B. W., Tapp, K., Dixon, M., and Carrillo, C. D. (2019). Genomically informed strain-specific recovery of Shiga toxin-producing Escherichia coli during foodborne illness outbreak investigations. J. Food Prot. 82, 39–44. doi: 10.4315/0362-028X.JFP-18-340
Carlin, C. R., Lau, S. S., Cheng, R. A., Buehler, A. J., Kassaify, Z., and Wiedmann, M. (2020). Validation using diverse, difficult-to-detect Salmonella strains and a dark chocolate matrix highlights the critical role of strain selection for evaluation of simplified, rapid PCR-based methods offering next-day time to results. J. Food Prot. 83, 1374–1386. doi: 10.4315/JFP-20-066
Carrillo, C. D., Koziol, A., Vary, N., and Blais, B. W. (2020). “Applications of genomics in regulatory food safety testing in Canada.” in: New Insight into Brucella Infection and Foodborne Diseases, eds M. Ranjbar, M, Nojomi, M. T. Mascellino (London: IntechOpen).
Carrillo, C. D., Koziol, A. G., Mathews, A., Goji, N., Lambert, D., Huszczynski, G., et al. (2016). Comparative evaluation of genomic and laboratory approaches for determination of Shiga toxin subtypes in Escherichia coli. J. Food Prot. 79, 2078–2085. doi: 10.4315/0362-028X.JFP-16-228
Carrillo, C. D., Kruczkiewicz, P., Mutschall, S., Tudor, A., Clark, C., and Taboada, E. N. A. (2012). Framework for assessing the concordance of molecular typing methods and the true strain phylogeny of Campylobacter jejuni and C. coli using draft genome sequence data. Front. Cell Infect. Microbiol. 2:57. doi: 10.3389/fcimb.2012.00057
Cooper, A. L., Low, A. J., Koziol, A. G., Thomas, M. C., Leclair, D., Tamber, S., et al. (2020). Systematic evaluation of whole genome sequence-based predictions of Salmonella serotype and antimicrobial resistance. Front Microbiol. 11:549. doi: 10.3389/fmicb.2020.00549
Gurevich, A., Saveliev, V., Vyahhi, N., and Tesler, G. (2013). QUAST: quality assessment tool for genome assemblies. Bioinformatics. 29, 1072–1075. doi: 10.1093/bioinformatics/btt086
Huang, W., Li, L., Myers, J. R, and Marth, G. T. (2012). ART: a next-generation sequencing read simulator. Bioinformatics. 28, 593–594. doi: 10.1093/bioinformatics/btr708
Huszczynski, G., Gauthier, M., Mohajer, S., Gill, A., and Blais, B. (2013). Method for the detection of priority Shiga toxin–producing Escherichia coli in beef trim. J. Food Prot. 76, 1689–1696. doi: 10.4315/0362-028X.JFP-13-059
Joensen, K. G., Scheutz, F., Lund, O., Hasman, H., Kaas, R. S., Nielsen, E. M., et al. (2014). Real-time whole-genome sequencing for routine, typing, surveillance, and outbreak detection of verotoxigenic Escherichia coli. J. Clin. Microbiol. 52, 1501–1510. doi: 10.1128/JCM.03617-13
Joensen, K. G., Tetzschner, A. M. M., Iguchi, A., Aarestrup, F. M., and Scheutz, F. (2015). Rapid and easy in silico serotyping of Escherichia coli isolates by use of whole-genome sequencing data. J. Clin. Microbiol. 53, 2410–2426. doi: 10.1128/JCM.00008-15
Katz, L. S., Griswold, T., Morrison, S. S., Caravas, J. A., Zhang, S., Bakker, H. C., et al. (2019). Mashtree: a rapid comparison of whole genome sequence files. J. Open Source Softw. 4:1762. doi: 10.21105/joss.01762
Kleinheinz, K. A., Joensen, K. G., and Larsen, M. V. (2014). Applying the ResFinder and VirulenceFinder web-services for easy identification of acquired antibiotic resistance and E. coli virulence genes in bacteriophage and prophage nucleotide sequences. Bacteriophage. 4:e27943. doi: 10.4161/bact.27943
Lambert, D., Carrillo, C. D., Koziol, A. G., Manninger, P., and Blais, B. W. (2015). GeneSippr: a rapid whole-genome approach for the identification and characterization of foodborne pathogens such as priority Shiga toxigenic Escherichia coli. PLoS ONE. 10:e0122928. doi: 10.1371/journal.pone.0122928
Lambert, D., Pightling, A., Griffiths, E., Van Domselaar, G., Evans, P., Berthelet, S., et al. (2017). Baseline practices for the application of genomic data supporting regulatory food safety. J. AOAC Int. 100, 721–731. doi: 10.5740/jaoacint.16-0269
Lindsey, R. L., Pouseele, H., Chen, J. C., Strockbine, N. A., and Carleton, H. A. (2016). Implementation of whole genome sequencing (WGS) for identification and characterization of Shiga Toxin-producing Escherichia coli (STEC) in the United States. Front Microbiol. 7:e00766. doi: 10.3389/fmicb.2016.00766
Low, A. J., Koziol, A. G., Manninger, P. A., Blais, B., and Carrillo, C. D. (2019). ConFindr: rapid detection of intraspecies and cross-species contamination in bacterial whole-genome sequence data. PeerJ. 7:e6995. doi: 10.7717/peerj.6995
Manninger, P., Koziol, A., and Carrillo, C. D. (2016). Draft whole-genome sequences of Escherichia fergusonii strains isolated from beef trim (GTA-EF02), ground beef (GTA-EF03), and chopped kale (GTA-EF04). Genome Announc. 4, e00185–e00116. doi: 10.1128/genomeA.00185-16
Microbiological Methods Committee. (2011). “Part 4: guidelines for the relative validation of indirect qualitative food microbiological, methods,” in: Compendium of Analytical, Methods, and Volume 1. Ottawa: Health, Canada. Available online at: https://www.canada.ca/en/health-canada/services/food-nutrition/research-programs-analytical-methods/analytical-methods/compendium-methods/official-methods-microbiological-analysis-foods-compendium-analytical-methods.html
Microbiological Methods Committee. (2016). “Part 9: guidelines for the validation of colony identification, methods,” in: Compendium of Analytical, Methods, and Volume 1 (Ottawa, ON: Health, Canada). Available online at: https://www.canada.ca/en/health-canada/services/food-nutrition/research-programs-analytical-methods/analytical-methods/compendium-methods/official-methods-microbiological-analysis-foods-compendium-analytical-methods.html
Nadon, C., Van Walle, I., Gerner-Smidt, P., Campos, J., Chinen, I., Concepcion-Acevedo, J., et al. (2017). PulseNet international: vision for the implementation of whole genome sequencing (WGS) for global food-borne disease surveillance. Eurosurveillance. 22:pii=30544. doi: 10.2807/1560-7917.ES.22,23.30544
Pardi, F., and Goldman, N. (2005). Species choice for comparative genomics: being greedy works. PLoS Genet. 1:e71. doi: 10.1371/journal.pgen.0010071
Petrillo, M., Fabbri, M., Kagkli, D. M., Querci, M., Van den Eede, G., Alm, E., et al. (2021). A roadmap for the generation of benchmarking resources for antimicrobial resistance detection using next generation sequencing. F1000Research. 10:80. doi: 10.12688/f1000research.39214.1
Ronholm, J., Nasheri, N., Petronella, N., and Pagotto, F. (2016). Navigating microbiological food safety in the era of whole-genome sequencing. Clin. Microbiol. Rev. 29, 837–857. doi: 10.1128/CMR.00056-16
Scheutz, F., Teel, L. D., Beutin, L., Piérard, D., Buvens, G., Karch, H., et al. (2012). Multicenter evaluation of a sequence-based protocol for subtyping Shiga toxins and standardizing stx nomenclature. J. Clin. Microbiol. 50, 2951–2963. doi: 10.1128/JCM.00860-12
Steel, M. (2005). Phylogenetic diversity and the greedy algorithm. Syst. Biol. 54, 527–529. doi: 10.1080/10635150590947023
Timme, R. E., Rand, H., Shumway, M., Trees, E. K., Simmons, M., Agarwala, R., et al. (2017). Benchmark datasets for phylogenomic pipeline validation, applications for foodborne pathogen surveillance. PeerJ. 5:e3893. doi: 10.7717/peerj.3893
Tong, W., Ostroff, S., Blais, B., Silva, P., Dubuc, M., Healy, M., et al. (2015). Genomics in the land of regulatory science. Regul Toxicol Pharmacol RTP. 72, 102–6. doi: 10.1016/j.yrtph.2015.03.008
Utturkar, S. M., Klingeman, D. M., Hurt, R. A. J., and Brown, S. D. A. C. (2017). Microbial genome assembly gap sequences and finishing strategies. Front. Microbiol. 8:1272. doi: 10.3389/fmicb.2017.01272
Weber, L. M., Saelens, W., Cannoodt, R., Soneson, C., Hapfelmeier, A., Gardner, P. P., et al. (2019). Essential guidelines for computational method benchmarking. Genome Biol. 20:125. doi: 10.1186/s13059-019-1738-8
Yoshida, C. E., Kruczkiewicz, P., Laing, C. R., Lingohr, E. J., Gannon, V. P. J., Nash, J. H. E., et al. (2016). The Salmonella in silico typing resource (SISTR): an open web-accessible tool for rapidly typing and subtyping draft Salmonella genome assemblies. PLoS ONE. 11:e0147101. doi: 10.1371/journal.pone.0147101
Keywords: whole-genome sequencing (WGS), Salmonella, validation, benchmark datasets, Listeria (L.) monocytogenes, food pathogen testing, Shiga toxin-producing Escherichia coli (STEC)
Citation: Carrillo CD and Blais BW (2021) Whole-Genome Sequence Datasets: A Powerful Resource for the Food Microbiology Laboratory Toolbox. Front. Sustain. Food Syst. 5:754988. doi: 10.3389/fsufs.2021.754988
Received: 07 August 2021; Accepted: 03 November 2021;
Published: 26 November 2021.
Edited by:
Matthew D. Moore, University of Massachusetts Amherst, United StatesReviewed by:
Kien-Pong Yap, University of Malaya, MalaysiaVasco Augusto Pilão Cadavez, Agricultural College of Bragança, Portugal
Copyright © 2021 Carrillo and Blais. This is an open-access article distributed under the terms of the Creative Commons Attribution License (CC BY). The use, distribution or reproduction in other forums is permitted, provided the original author(s) and the copyright owner(s) are credited and that the original publication in this journal is cited, in accordance with accepted academic practice. No use, distribution or reproduction is permitted which does not comply with these terms.
*Correspondence: Burton W. Blais, burton.blais@inspection.gc.ca