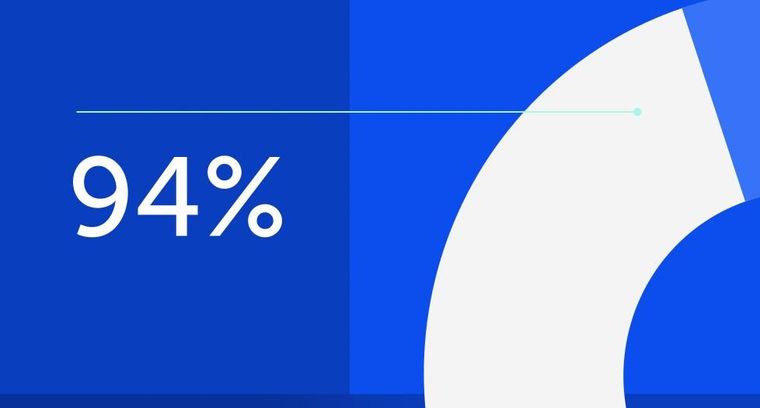
94% of researchers rate our articles as excellent or good
Learn more about the work of our research integrity team to safeguard the quality of each article we publish.
Find out more
ORIGINAL RESEARCH article
Front. Sustain. Food Syst., 16 November 2021
Sec. Land, Livelihoods and Food Security
Volume 5 - 2021 | https://doi.org/10.3389/fsufs.2021.727058
This article is part of the Research TopicAgile Data-Oriented Research Tools to Support Smallholder Farm System TransformationView all 17 articles
Agricultural development projects often struggle to show impact because they lack agile and cost-effective data collection tools and approaches. Due to the lack of real-time feedback data, they are not responsive to emerging opportunities during project implementation and often miss the needs of beneficiaries. This study evaluates the application of the 5Q approach (5Q). It shows findings from analyzing more than 37,000 call log records from studies among five countries. Results show that response rate and completion status for interactive voice response (IVR) surveys vary between countries, survey types, and survey topics. The complexity of question trees, the number of question blocks in a tree, and the total call duration are relevant parameters to improve response and survey completion rate. One of the main advantages of IVR surveys is low cost and time efficiency. The total cost for operating 1,000 calls of 5 min each in five countries was 1,600 USD. To take full advantage of 5Q, questions and question-logic trees must follow the principle of keeping surveys smart and simple and aligned to the project's theory of change and research questions. Lessons learned from operating the IVR surveys in five countries show that the response rate improves through quality control of the phone contact database, using a larger pool of phone numbers to reach the desired target response rate, and using project communication channels to announce the IVR surveys. Among other things, the respondent's first impression is decisive. Thus, the introduction and the consent request largely determine the response and completion rate.
Digitalization as a socio-technical process has become a transformative force to applying digital innovation to agriculture and food systems (Klerkx et al., 2019). However, it raises the question: can smallholders keep up the pace and benefit from the intended transformation? Collaborations between national actors from agricultural institutions and the research body to apply data-driven approaches can make farming more productive for smallholders (Jiménez et al., 2019), increase their net farm income, and transform food systems toward sustainability (Chapman et al., 2021). Digitalization initiatives promise improvements for smallholders in low- and middle-income countries (LMICs). Still, they do not reach a significant number of farmers. However, some of these initiatives have made progress in recent years (Baumüller, 2017), but barriers exist and need to be addressed. The main barriers are lack of technical infrastructure (Mehrabi et al., 2020), lack of access to digital tools and services, lack of ease of use for non-tech-savvy farmers, and lack of design that is targeted for low-literate and marginal groups. Recent studies show that mobile phone-based dissemination of information as a service for smallholders can have a positive impact in promoting farm management practices (Djido et al., 2021), deliver advice as an automated advisory service that collects household data to improve advice over time (Steinke et al., 2019), and use of speech-based services as a viable way for providing information to low-literate farmers (Qasim et al., 2021). The access and availability gaps and challenges with technology (e.g., lack of connectivity in rural areas) will disappear over time.
For this reason, socio-ethical barriers are the main barriers to overcome (Shepherd et al., 2020). Moreover, precisely because of the transformative momentum of digitalization, there is a risk for smallholders to enter the digital divide and power asymmetry gap. The risk is increased when digital technologies are embedded in the science community, private sector, and larger farms only. Smallholders are left behind in a big data divide (Carbonell, 2016).
The use of data-oriented tools in agriculture research has increased over the last few years. An important task of science is to support the design of new tools and services, evidence the use of digital tools by smallholders, and observe unintended consequences (Shepherd et al., 2020), especially for smallholders on the brink of the digital divide (May, 2012). Investment in last-mile infrastructure, universal access to information and data (Mehrabi et al., 2020), and out-of-the-box interoperable systems (Kruize et al., 2016) are important research areas for coming years. Transforming research toward more agile data collection, using IVR, an automated phone system using recorded messages that allows callers to interact with the system without speaking to an agent, as a medium to reach people in LMICs, and overcome language and literacy barriers has become relevant recently. Advantages of IVR compared to other communication channels, e.g., text message services, mobile phone applications, radio programs, among others, are factually precise; voice messages can be recorded in different local languages and accessed on-demand, and farmers can easily follow the voice message even if they do not know how to read. For scientists, the advantages are more cost-effective data collection since operating mobile phone calls is usually cheap and produces ready-to-analyze data in near real-time because being stored just-in-time while operating the call in cloud storage.
IVR has been used for a longer time in health applications, and research on response rates in LMICs has been done for health risk prevention. Global data of IVR response rates in health research shows between 30 and 50% (Gibson et al., 2019; Pariyo et al., 2019). Experiences in using IVR for health in Ghana and Uganda showed positive attitudes toward IVR by respondents and constant response rates over a more extended time (L'Engle et al., 2018; Byonanebye et al., 2021). The ease of use, empathy, trust in information source, cultural and language factors, availability and accessibility, reduced costs, and women's empowerment supports the willingness to use IVR systems. On the other hand, the barriers to use are lack of human interaction, the complexity of information, and facilitating conditions, especially lack of technical infrastructure (Brinkel et al., 2017).
The above arguments suggest that science should experiment and pilot new digital tools to provide inclusive two-way communication channels that include smallholders by overcoming the digital divide's burden and bringing to life transdisciplinary research initiatives that include all food system stakeholders. In traditional, non-digital participatory research, the leading farmers' barrier to participation is not having a voice to communicate needs. At the same time, using translational research, scientists work on digital solutions to use science for applicable digital solutions to improve agricultural productivity (Passioura, 2020). However, two-way communication in participatory processes has been used in the past successfully, for example, to understand the vulnerability context of farmers in value chains (Valdivia et al., 2014), or the use of the power of crowdsourcing to significantly improve the data basis for algorithm training (Hampf et al., 2021).
This paper presents the details of evaluating 5Q, a concept of keeping data collection smart and simple by asking five thoughtful questions, combined with IVR for agile data collection, developed to effectively collect feedback from agricultural development projects with a potential for massive data collection (Jarvis et al., 2015). Its principle is to incorporate feedback mechanisms in projects and build an evidence base that improves decision-making, adoption, and impact, keeping it smart, simple, and easy to use. This publication shows the iterative improvement of configurations and measures taken during individual studies in five countries and over six years of operating IVR survey campaign calls. The analysis processed 37'503 call metadata in 44 IVR call campaigns and five countries and provides insights into call status, average call duration, reached IVR blocks, and differences in response rate between different call types and survey topics.
The paper is structured as follows; first, it presents materials and methods on how call metadata were analyzed and evaluates the implementation of 5Q in nine studies in Tanzania, Uganda, and Rwanda in East Africa, Ghana in West Africa, and Colombia in South America. Second, the results from the analysis and evaluation are presented. Finally, findings are discussed, and future research needs are laid out in the discussion and conclusions.
Call metadata were analyzed from 44 IVR call campaigns from nine studies in five countries between 2015 and 2021. The design of all campaigns followed the 5Q approach, which was first proposed by (Jarvis et al., 2015) as an agile data-oriented approach to incorporate feedback mechanisms in agricultural development projects.
5Q is a concept that uses the principle of keeping data collection smart and simple by asking five thoughtful questions in question-logic-trees in repeated cycles or rounds, and by using cost-effective digital communication tools for data collection. 5Q supports the idea that the adoption of new technologies or services is a process that goes through several stages (Glover et al., 2019). The recuring feedback loops can help to understand better how the technology fits into farmers' context, perceptions, barriers, and enablers for adoption. Besides more conventional variables such as farm characteristics and economic variables, the role of knowledge, perceptions, and attitudes as intrinsic factors toward adoption play a key role in farmers' decision-making process for adoption and use (Meijer et al., 2015). 5Q collects intrinsic factors in feedback loops from farmers as potential adopters to inform promoters and implementers, and sends information back to farmers. Thus, it moves from simply collecting data to using data for building evidence on knowledge (perception), attitudes, and skills for practice (KAS). The feedback loops can be embedded in a project monitoring and evaluation plan and used complementary to participatory tools and traditional data collection methods (Figure 1). As part of a two-way information flow strategy, feedback generation can start before defining a project's theory of change or research question by asking the potential benefiting community about their needs or specific barriers to adopt a new technology. During the implementation of project activities, the approach can be used to design a workflow of design feedback loops between implementers and beneficiaries. Nevertheless, most importantly, it provides recurring data for decision-making during project implementation and contributes to monitoring and evaluation outcomes. After a project ends, 5Q can be used to feed into an impact assessment study.
Figure 1. 5Q embedded in a traditional project's data collection timeline (5Q components in shapes with black filled background). Symbols are question-tree QT, survey rounds R, stakeholder groups G, and group typologies T.
5Q starts by identifying questions that respond to a research question or a project's theory of change. Questions can recall a farmers' perception, monitor the effects of implemented activities, or evaluate adoption, among others. Next, a logic-tree structure is used to define the sequence of the survey. Questions are linked in a tree structure by branches and decision nodes, connecting a respondent-based answer to the following questions block. A 5Q survey using a question-tree requests about five answers from a respondent within one survey round, depending on the respondent's pathway through the question-tree branches and nodes (Figure 2, left). At the end of each survey round, respondents can be grouped based on typologies from survey answers. The created groups can be used for tree variations for the next survey round (Figure 2, right).
Figure 2. Questions Q in a tree structure with branches and decision nodes (left) and survey rounds creating typology groups G for follow-up survey round R (right). Unfilled shapes show an example of a respondent's pathway in the tree structure.
Survey rounds carried out in cycles during the implementation of projects provide feedback for decision-making, making the process responsive and effective, and ensuring mutual accountability and integration of stakeholders in the project implementation phase. Using 5Q suggests asking stakeholders more frequently about their needs and perceptions of activities carried out within a project; more specifically, it explores how the project can serve beneficiaries. For example, a survey round collects data from project beneficiaries about the usefulness of project activities. The collected data serve project implementers to make a corrective action on the project implementation process. A plan for survey rounds and sequential question trees can be designed at the beginning of a project and adjusted as new data are produced in each survey round.
Digital communication tools facilitate more cost-effective data collection than traditional approaches. Therefore, 5Q selects the most appropriate digital channel for the context of stakeholder groups. For example, on the one hand IVR calls are the most time- and cost-effective way of collecting data but are ineffective when a socio-cultural context or low literacy level is prevalent within stakeholders. On the other hand, survey interviews facilitated by hired enumerators or volunteer community members using mobile apps can overcome the literacy barrier but are less cost- and time-effective.
This study compared data from IVR survey calls only. Findings of comparing IVR data collection with mobile phone data collection can be found in Eitzinger et al. (2019).
Several studies used the 5Q to collect data or obtain feedback from farmers during project implementation. In Table 1, project goals were summarized, and the 5Q call campaigns were described.
The goal of the studies in the West Usambara Mountains in Tanzania and Northern Region of Uganda carried out between 2015 and 2017 was wide-scale adoption of climate-smart agriculture (CSA) among farmers through prioritizing practices and technologies (Mwongera et al., 2016) and demonstration of CSA practices in training sessions and farmer-managed demonstration plots. 5Q was applied to obtain farmers' feedback after implementing project activities. Using regular IVR survey calls, the adoption of practices were measured in the three levels of KAS. The studies in Colombia focused on better understanding farmers' perception of climate risks on agricultural livelihoods (Eitzinger et al., 2018) and farmers' perception of the seasonal weather forecast for Colombian maize and rice agriculture (Sotelo et al., 2020). In Ghana and Mount Elgon in Uganda, a project sought to ensure that farmers continue to invest in coffee and cocoa by breaking down recommended CSA practices into smaller, incremental investment steps (Jassogne et al., 2017). Likewise, in Tanzania and Uganda studies, KAS of farmers were queried for incremental steps for investing in CSA practices. In Rwanda, climate services were disseminated as participatory integrated climate services for agriculture, also known as the PICSA approach. Farmers in four provinces across Rwanda were trained to read weather forecasts related to an agricultural advisory. 5Q was used to collect farmers' feedback about climate services (Birachi et al., 2020). During the covid-19 pandemic, two surveys were implemented in Rwanda to collect information about the farmers' perceived impact on agricultural production and households' food security.
The contact database of respondents' phone numbers was provided in all studies by local partners, except in Tanzania, where the phone numbers were collected during a baseline interview. Results and data visualizations for all studies can be accessed on the 5Q results dashboard1.
Call metadata of 37'503 IVR calls were analyzed from 44 call campaigns among five countries. Three different call statuses were analyzed. Call status complete is used when a respondent reached one of the end blocks in a question tree during a call, incomplete when the respondent responded to some of the question blocks but did not reach the end block and failed when the respondent did not respond to the call until the maximum number of repetitions was reached defined by the call campaign configuration. For all call campaigns, default call configurations were used, as a defined call time window between seven in the morning until eight at night, repeat settings of two intentions in quick successions of five min, and repetitions up to two times every hour; on detection of a voice mail, the call intention was stopped until the next programmed repetition. Question tree complexity was defined as the number of blocks (questions) reached during a call. Call duration was measured in seconds, and its relations to call status and reached blocks were analyzed.
Further, call metadata were examined if the percentage of completed calls depends on the call type or topic. Different call types were introduced as voice message calls to obtain the respondent's consent, feedback surveys, recall a respondent's perceptions, and collecting survey data. Responses from farmers and other project stakeholders were examined separately. Finally, differences in the distribution of call status percentages with the call or survey topic were analyzed. Earlier call campaign types were related to climate change research. More recent campaigns focused on the impact of covid-19 on agriculture production and food security.
The paper evaluates how 5Q has been implemented in the different studies and reviews the configurations and measures taken during the individual studies for the operation of campaign calls to improve the response rate. The measures have evolved and have not been tested in an experimental setup. However, lessons learned from call campaign configurations have been implemented incrementally as new campaigns were started. Most relevant learnings were listed, and further details were provided on how the question trees were developed with project teams in the different countries.
Metadata analysis of 44 call campaigns shows that response rate and call completion status for IVR survey calls varies between countries, survey types, and research topics (Figures 3, 5). The overall response rate was highest (farmer picked up the call and stayed until the first block) in Colombia with 95%, followed by Rwanda with 78%. In Colombia, however, only 18% finished all blocks and reached a call status completed. In comparison, in Rwanda, the rate of call completion was higher, with 38%. Response rate and calls status distribution were similar in Uganda and Ghana, 57% response rate in Uganda, and 46% in Ghana. Call metadata from Tanzania show the lowest rate of completed calls (16%). However, Tanzania ranks before Ghana when combined with incomplete calls (see Figure 3, left). At the sub-national level, differences can be found in Uganda. Response rate and survey completion were lower in Nwoya (6%) and Bushenyi (16%) than 27% completed surveys in Mount Elgon. Also, results from Colombia show differences between Cauca and the two other Colombian regions (Figure 3, right).
Figure 4 shows the response rate and call completion for each call series operated between 2015 and 2021 across the five countries (see Table 1 to identify the call-series number in the x-axis). A higher percentage as the countries average for completed surveys can be observed for the call series 52, 53, 58–61, 78, and 82–84 (feedback KAS) in Mount Elgon, Uganda. For Rwanda, the call series 93 (IVR training call for farmers), 95 and 99 (voice message introduction), and 96 (the first covid-19 call) show higher rates than the average rate of completed surveys. In Colombia, the call campaigns 20 and 21 (collecting perceptions on climate risks) and 18 and 19 (feedback on climate services) had higher percentages of complete calls than other call campaigns. The series 25 in Colombia had high completion rates because it was an introduction voice message, and call campaign 34 is an outlier (n = 7 calls only) and is therefore not a representative call metadata. The first call campaigns in Tanzania (series 1 to 7) were not included in the country average. During this first call campaigns in Tanzania, some calls had technical issues and did not show any calls with status completed in the data. However, higher rates than the average of completed surveys for Tanzania were achieved in one of the KAS feedback surveys (12) and the feedback call after the capacity-building event for crop management (17). Ghana showed a similar response rate across all call campaigns with the highest response rate of 32% on the call series (89).
Comparing the different survey types and research topics that used 5Q and IVR for data collection shows that, as already observed in Figure 4, introduction voice messages (INTRO), training calls (TRAIN), and calls on perceptions can achieve higher rates of completion (Figure 5, left). In addition, research on covid-19, crop management, and climate services show higher percentages on complete call status than others (Figure 5, right).
Figure 5. Call status per survey type (left) and survey topic (right). Different survey types are one-block (listen-only) voice messages (INTRO), multi-block question-trees on perceptions (QT-P), feedback (QT-F) and surveys (QT-S), initial calls for obtaining the respondent's consent (CONSENT), and test- and training calls (TRAIN). Topics included calls about climate services (CS), impacts from the covid-19 pandemic (COVID), climate-smart agriculture (CSA), climate risks (CR), crop management (CM), and combinations.
Figure 6 shows the given consent (yes) or (no) by the same respondent in Rwanda to different calls and times. The two call campaigns about feedback on climate services in 2019 and 2021 had overall lower agreement rates than the two covid-19 calls in 2020 and 2021, even though the two calls in the year 2021 were carried out within a time window of two weeks (the CS2021 was carried out in March and the COVID2021 in the first week of April with the same sample population).
Figure 6. Respondent's consent (first block of QT) changes between the first and second call round for climate services CS topic (left) and covid-19 COVID topic (right).
The average call duration compared to call status and reached blocks is compared in Figure 7. Since incomplete calls can still provide valuable data, it is essential to understand how much time and reached blocks the respondent's stays in the call. When comparing the two graphs call status complete (left) and incomplete (right), most data in Ghana (blue squares) are yielded from complete calls (the maximum number of reached blocks was 20). The same effect can be observed for the Uganda data (black flipped square). Most calls stop between 20 and 30 blocks, including both complete and incomplete calls. For Rwanda, a drop can be observed after 30 blocks. Since incomplete calls can still provide valuable data, it is essential to understand how much time and reached blocks the respondent's stays in the call.
Figure 7. Blocks reached an average of call duration by call duration and country; calls complete (left) and incomplete (right).
Using 5Q and IVR is a cost-efficient way of collecting feedback for an agricultural development project. It works best when question-logic trees and survey rounds align with the project's theory of change and research questions, being developed in a collaboration between various project members. The studies in Tanzania, Rwanda, and Colombia developed the question-logic trees in workshops lasting several days. In Ghana and Uganda, they were developed in virtual meetings.
When designing the questions and surveys, usually researchers and project implementers want to include many questions. The role of the researcher leading the 5Q component is to remind the group of the 5Q principles, which are, keep it smart and simple. The group needs to mind that 5Q will not replace any of the other data collection components of the project, such as baseline surveys gathering information to characterize the project's beneficiaries. The type of questions that should be considered for a 5Q question tree is closer to polls, where people's choices and understanding of their opinion, perception, understanding what works for them within the project's theory of change.
Once the question trees, logic, and rounds were defined in each project, the next step was preparing the scripts for each planned call. The script is an essential link for the call operations, often carried out by a technical operator unfamiliar with the project itself. Thus, it is crucial to have a consistent self-explaining script to facilitate the process. The script includes the spoken text that needs to be recorded for each question (block in the call campaign) and any additional language. In addition, the script should indicate the linkages between question-blocks and, at best, include a drawing of the tree structure (see Figure 2). The script's best works in a table format, using the following columns: Block number | Question code | Goto code | Transcript | Translation [n] | Audio filename.
The selection of the IVR systems should be made based on the capacity of the project, and there are several options. One option is to set up the IVR system on a server using application programming interfaces (API) from an IVR service provider. Another option is using a programmable voice cloud platform from a communications platform provider. The latter option needs less in-house technical expertise and provides a global range through a single service provider. Therefore, it can be more cost-effective when running call campaigns in several countries and with fewer respondents, like in some of the presented studies in this paper. In the case of operating in one country, partnering with a local telecom provider might be a better option.
Before starting the first call campaign, the contact database of respondents' phone numbers is required. In most cases, it should come from the project consortium. In most studies (see Table 1), the implementation of IVR calls depends on an external contact database of respondents' phone numbers, often obtained through a local project partner. The quality of a contact database can vary widely, and the following steps are recommended. First, ask the project partners to obtain consent from respondents to share the contact data within the project. The consent could be obtained by sending a text message by the local partner to all respondents, requesting them to send back a message if they agree to participate in the planned call campaign. Doing this step via partners would automatically clean out errors and out-of-date contacts and provide a consistent database with a pre-consent of respondents.
Next, pre-testing the question-tree integrity by running the IVR with the project team that developed the trees is recommended, for example, at the end of the tree design workshop. It is easier for them to identify mistaken linkages between tree branches than for the IVR operator. A wrong link can still be fixed before sending it to the target population. The test run is also the last opportunity for the team members to evaluate if the campaign complies with the 5Q principle: Keep it smart and simple.
Operating an IVR call should be straightforward when the above-listed recommendations are followed. However, before starting the call campaign, the lessons learned from all studies showed that respondents' awareness should be raised to achieve an acceptable call response rate (see Eitzinger et al. (2019) for evidence from Tanzania). Farmers who are informed by a project activity are more likely to respond to IVR. In most studies, the project implementers informed farmers through their project networks about the planned 5Q call campaigns. In the cases of extensive respondent lists, or when farmers cannot be informed through project activities, voice messages were sent introducing the research and explaining the purpose of the planned survey.
Furthermore, like a text message sent by a local partner for consent purposes, the voice message is another way of cleaning an extensive phone number database. For example, in Rwanda, the local project team started with 11'000 contact numbers. However, almost half of them did not connect to the voice message, and the first survey was started with a sample of 5'330 respondents. A text message was sent as a final reminder 30 min before every call to improve the response rate.
The configuration of the call campaign in the IVR system can also affect the response rate. In all studies, call campaigns were configured in similar settings. For example, a default call configuration setting was used to use a defined call time window between seven in the morning and eight at night. Also, the best time to operate the calls and have the highest possible response rate without interfering with the farmers' daily tasks was defined together with local project teams. Usually, between 2 pm and 7 pm was recommended as the best time to reach farmers in the afternoon. IVR was programmed to repeat calls with a call status failed in two intentions in quick successions of five min and repetitions up to two times, trying every hour (total six intentions); on detection of a voice mail, the call intention was stopped until the next programmed repetition.
Finally, cost estimation of programmed IVR call campaigns should be done and confirmed with the available budget. One of the main advantages of 5Q IVR surveys is low cost and time efficiency. Once an IVR system, either as its system or service subscription, is set up, call operations are often the lowest national costs for mobile phone airtime. In the studies presented in this paper, an IVR subscription service was used. A subscription service typically charges a monthly subscription fee (approximately between 500 and 1,500 USD) and operation costs for airtime. A rate of 0.04 USD per minute was paid in Rwanda, 0.05 USD per minute in Colombia and Ghana, 0.08 USD per minute in Uganda, and 0.1 USD per minute in Tanzania. The total cost of operating 1,000 calls of 5 min in all five countries, without including the costs for the IVR system, makes the sum of 1,600 USD: 200 USD for Rwanda, 250 USD for Colombia and Ghana, 400 USD for Uganda, and 500 USD for Tanzania. The call campaign could be operated in less than one hour.
Lessons learned from applying 5Q combined with IVR for agile data collection were presented. Call metadata from 44 call campaigns collected between 2015 and 2021 in Tanzania, Colombia, Uganda, Ghana, and Rwanda were analyzed.
Farmers can change phone numbers rapidly or share sim cards within the household or even the community. In the first project piloting 5Q in Tanzania, farmer's phone numbers were registered as part of the pilot in resource-intensive door-to-door data collection. Unfortunately, many farmers changed from one service provider to another shortly after collecting the first IVR calls in 2015. A better way of collecting the phone numbers would be through inbound campaigns. At best, farmers would call into an offered service, e.g., a digital extension system or market price information system. After receiving the service, a 5Q feedback survey could be sent after time to the farmer. Farmers call into the system (or send an opt-in text message) and leave their phone number to get called back by the IVR system for operating the surveys.
For the following studies in Uganda, Colombia, Ghana, and Rwanda, farmer's phone contacts were obtained from project partners. When using contact data from external sources, it is more difficult to anticipate how many phone numbers might be outdated or collected a long time ago with a chance that it might have changed. In the findings of this study, there are some uncertainties in the analysis of call metadata about the comparison of failed calls among countries. In Figure 3, while calls in Uganda, Tanzania, and Ghana show a high rate of failed calls, Colombia only had 5% failed calls. In fact, in Colombia, the partner who provided the phone numbers sent a text message to all contacts in his database for the department of Cauca in the Southwest of Colombia (>400,000 text messages) and asked farmers to accept participating in the study by sending a text message with the text 'yes' back. In total, 1,240 farmers gave consent by responding positively to the text message. Also, in Rwanda, 10,998 phone numbers were received from the local project partners. As the first introduction and training call, a voice message was sent to all farmers. For the subsequent call campaigns in Rwanda, 5,330 phone numbers were used from contacts who successfully participated in the introduction and training call, partly explaining the lower rate of failed calls, 22% in Rwanda. Comparing a series of four calls between the years 2019 and 2021 in Rwanda (Figure 6) confirms that most failed call intents remained the same all four calls, which further indicates that these contacts were not updated or the sim cards were not used anymore.
Finally, another option would be using a random digit dial (RDD) sampling strategy and start the survey with an eligibility question. The response rate in random digit dial surveys may be lower but could achieve representative sampling at a low cost. A recent study that carried out RDD surveys in nine LMICs shows that the average response rate for RDD IVR surveys vary between 7 and 60% (39% in Rwanda, 11% in Uganda, and 25% in Colombia) and found that the most significant limitation on response rates is to reach the start of the survey, even if they have responded to the call (Dillon et al., 2021). Other studies calculated a standardized response rates from the number of completed interviews, partial interviews, refusal or break-off, non contact and others to validate RDD surveys against other survey research methodologies (L'Engle et al., 2018).
Evidence from RDD surveys shows that strategies to increase response rates should focus on increased pick-up rates and improved first impressions of respondents (Dillon et al., 2021). In the context of agricultural development projects, increased pick-up rates and effective start of the survey can be achieved by several measures. First, a bigger pool of phone numbers increases the chance to reach the desired response rate. Second, using the project's communication channels to announce IVR call campaigns increases response rate, e.g., announcing them during a focal-group workshop, through the voice of community leaders, or sending text messages to respondents ahead of the first call campaign. Next, if the phone numbers were received from partners, ensure the partner runs a quality control to remove outdated contacts before handing over the database. Finally, to avoid a respondent hanging up shortly after starting the call, the introduction is vital for staying in the call. The introduction should reveal who or what institution is calling, explain the purpose of the call, and benefit from the collected data. For research and academia, ethical standards for research that involves human subjects are often institutional policy and provide clear guidelines for the consent of a respondent of phone calls in research activities.
If the study requires several call campaigns, it is also essential to consider that respondents might not pick up in a subsequent call or change their consent between calls from yes to no. Figure 6 shows the change of the same respondents' responses to the first consent block in each call. Finally, the type and topic of the call are relevant for the response rate and completion status. Results show that calls with only one block to introduce the research to a farmer or explain how the IVR call works (training call) had a much higher completion rate than other call types that involve a set of question blocks. The finding supports the basic idea of 5Q to keep call campaigns short.
Furthermore, it suggests setting up call campaigns in small packages that can be run as connected blocks on an IVR system and allowing a resumption of the call campaign on uncompleted blocks. Besides the length of a call, the research topic was also relevant for a higher response rate. Although some topics like covid-19 can attract more attention by respondents, and the imposed travel restrictions for agricultural fieldwork can also increase call campaigns response rate during the time of imposed restrictions, the higher response rate was also found on research topics about services that farmers receive. For example, the response rate of call campaigns evaluating climate services for farmers in Rwanda (CS in Figure 5) showed similarly high levels as the call campaigns on impact from covid-19 (COVID in Figure 5). The same response rate can also be observed at call campaigns collecting feedback after a training workshop in Tanzania (CM in Figure 5). Finally, the response rate also depends on cultural and regional differences.
Unlike other data collection methods and tools, 5Q moves from simply collecting data to using data from multiple sources to give a clearer idea of KAS. Following the 5Q key message of keeping it simple and asking five smart questions suggests using the KAS approach as a framework for developing question trees. Since knowledge is the first step for many farmers to adopt a new agricultural innovation (outcome propositions), attitudes toward new practices depend on a combination of the individual's belief that it will lead to the desired outcome (outcome beliefs) and the values they attribute to those outcomes. KAS identifies people's perceptions of a new practice, technology, or service. Understanding cognitive barriers and drivers for adoption is essential for knowledge transfer strategies in agricultural development projects. Skill for subsumption knowledge transfer into farmer practice is the last and main desirable change (outcome skills). It generally occurs because of previous knowledge, skills, and attitude toward a practice or service. Thus, following KAS for developing question trees is the simplest way of applying 5Q to a project, but is not the only one, and the best strategy for developing a project questions tree should be developed in participatory sessions between project implementers and an experienced researcher of developing a 5Q strategy for an agricultural development project.
In this study, call metadata was analyzed to demonstrate how the 5Q concept combined with IVR systems can achieve cost-efficient and agile data collection. Though results show some evidence of response and saturation rates in different call campaign types, the study did not validate sample interactions to understand better why some respondents carried on to the end of the blocks while others dropped the call earlier. Also, differences of respondents in terms of social inclusion, literacy, and digital literacy have not been considered for the analysis, which might represent a gap in the presented findings drawn from call metadata and experiences from implementers of 5Q call campaigns only. Therefore, future research should identify respondents' saturation rates and reasons for early dropout during IVR call campaigns. Further, issues of unintended social exclusion should be studied to understand better what external factors, like lack of access, resources, or knowledge, lead to exclusion and identify the best-bet digital communication channels to reach them using the 5Q approach for feedback collection. Finally, other barriers like the social norm, lack of self-efficacy, and lack of perceived usefulness, can lead to low response rate or quick saturation of call respondents in 5Q call campaigns and need to be further studied.
The study demonstrates how 5Q can be combined with cost-effective IVR call campaigns and help agricultural development projects incorporate feedback mechanisms, such as building evidence of what works and what does not in terms of adoption. 5Q is a concept that uses the principle of keeping data collection smart and simple by asking five thoughtful questions in question-logic-trees in repeated cycles or rounds and using cost-effective digital communication tools for data collection. 5Q moves from simply collecting data to building evidence of farmers knowledge (perception), attitudes, and skills for adopting a practice.
5Q follows a process that starts by identifying questions that are linked to a project's theory of change. Next, a logic question-tree structure and question blocks are used to create a automatable sequence that can be used to program a IVR system. This study analyzed call metadata from 44 IVR call campaigns in five countries. Three different call statuses were analyzed as percentage of complete, incomplete, and failed calls, to understand differences between countries, call type, and campaign topic.
Overall, results show that response rate and call completion for IVR calls vary between countries, call types, and survey topics. Response rates, including complete and incomplete surveys, were highest in Colombia with 95%, followed by Rwanda with 78%. However, in Rwanda, the rate of call completion was higher than in other countries, with 38%. The study also found that higher response rates can be achieved by increasing the pick-up rate and improve the first impressions of respondents about the call campaign topic.
Future research should focus on better understanding what leads to a respondents' saturation and early call dropout during IVR call campaigns. Further, issues of social exclusion that can happen unintentionally, should be studied to understand better what external factors, like lack of access, resources, or knowledge, lead to exclusion. Finally, by identifying wich digital channel works best in a region and for a social group, possible social exclusion could be avoided.
The call metadata used for the analysis are available on: https://doi.org/10.7910/DVN/CMIVQK.
The studies involving human participants were reviewed and approved by Institutional Review Board of the Alliance of Bioversity International and CIAT. Written informed consent for participation was not required for this study in accordance with the national legislation and the institutional requirements.
AE supported the implementation of research in all studies countries, analyzed all metadata, and wrote the manuscript.
This work was supported by the Bill & Melinda Gates Foundation [OPP1107891]; the OPEC Fund for International Development (OFID) [TR335]; and the Deutsche Gesellschaft für Internationale Zusammenarbeit (GIZ) [81206685].
The author declares that the research was conducted in the absence of any commercial or financial relationships that could be construed as a potential conflict of interest.
All claims expressed in this article are solely those of the authors and do not necessarily represent those of their affiliated organizations, or those of the publisher, the editors and the reviewers. Any product that may be evaluated in this article, or claim that may be made by its manufacturer, is not guaranteed or endorsed by the publisher.
Baumüller, H. (2017). The little we know: an exploratory literature review on the utility of mobile phone-enabled services for smallholder farmers. J. Int. Dev. 154, 134–154. doi: 10.1002/jid.3314
Birachi, E., Hansen, J., Radeny, M., Mutua, M., Mbugua, M. W., Munyangeri, Y., et al. (2020). Rwanda Climate Services for Agriculture: Evaluation of farmers' Awareness, Use and Impacts. Wageningen, the Netherlands Available online at: https://hdl.handle.net/10568/108052.
Brinkel, J., May, J., Krumkamp, R., Lamshöft, M., Kreuels, B., Owusu-Dabo, E., et al. (2017). Mobile phone-based interactive voice response as a tool for improving access to healthcare in remote areas in Ghana - an evaluation of user experiences. Trop. Med. Int. Heal. 22, 622–630. doi: 10.1111/tmi.12864
Byonanebye, D. M., Nabaggala, M. S., Naggirinya, A. B., Lamorde, M., Oseku, E., King, R., et al. (2021). An interactive voice response software to improve the quality of life of people living with hiv in uganda: randomized controlled trial. JMIR mHealth uHealth 9, e22229. doi: 10.2196/22229
Carbonell, I. M. (2016). The ethics of big data in big agriculture. Internet Policy Rev. 5, 1–13. doi: 10.14763/2016.1.405
Chapman, J., Power, A., Netzel, M. E., Sultanbawa, Y., Smyth, H. E., Truong, V. K., et al. (2021). Challenges and opportunities of the fourth revolution: a brief insight into the future of food. Crit. Rev. Food Sci. Nutr. doi: 10.1080/10408398.2020.1863328. [Epub ahead of print].
Dillon, A., Glazerman, S., and Rosenbaum, M. (2021). Understanding Response Rates in Random Digit Dial Durveys. Global Poverty Research Lab Working Paper No. 21–105. doi: 10.2139/ssrn.3836024
Djido, A., Zougmor,é, R. B., Houessionon, P., Ouédraogo, M., Ouédraogo, I., and Seynabou Diouf, N. (2021). To what extent do weather and climate information services drive the adoption of climate-smart agriculture practices in Ghana? Clim. Risk Manag. 32, 100309. doi: 10.1016/j.crm.2021.100309
Eitzinger, A., Binder, C. R., and Meyer, M. A. (2018). Risk perception and decision-making : do farmers consider risks from climate change? Clim. Change. Risk. 151, 507–524 doi: 10.1007/s10584-018-2320-1
Eitzinger, A., Cock, J., Atzmanstorfer, K., Binder, C. R., Läderach, P., Bonilla-findji, O., et al. (2019). GeoFarmer : a monitoring and feedback system for agricultural development projects. Comput. Electron. Agric. 158, 109–121. doi: 10.1016/j.compag.2019.01.049
Gibson, D. G., Wosu, A. C., Pariyo, G. W., Ahmed, S., Ali, J., Labrique, A. B., et al. (2019). Effect of airtime incentives on response and cooperation rates in non-communicable disease interactive voice response surveys: randomised controlled trials in Bangladesh and Uganda. BMJ Glob. Heal. 4, 1–11. doi: 10.1136/bmjgh-2019-001604
Glover, D., Sumberg, J., Ton, G., Andersson, J., and Badstue, L. (2019). Rethinking technological change in smallholder agriculture. Outlook Agric. 48, 169–180. doi: 10.1177/0030727019864978
Hampf, A. C., Nendel, C., Strey, S., and Strey, R. (2021). Biotic yield losses in the southern amazon, brazil: making use of smartphone-assisted plant disease diagnosis data. Front. Plant Sci. 12, 548. doi: 10.3389/fpls.2021.621168
Jarvis, A., Eitzinger, A., Koningstein, M., Benjamin, T., Howland, F., Andrieu, N., et al. (2015). Less is More : The 5Q Approach. Cali, Colombia.
Jassogne, L., Mukasa, D., Bukomeko, H., Kemigisha, E., Kirungi, D., Giller, O., et al. (2017). Redesigning delivery: boosting adoption of coffee management practices in Uganda. The climate smart investment pathway approach and the farmer segmentation tool. CCAFS Info Note, 5. Available online at: https://cgspace.cgiar.org/rest/bitstreams/110288/retrieve.
Jiménez, D., Delerce, S., Dorado, H., Cock, J., Muñoz, L. A., Agamez, A., et al. (2019). A scalable scheme to implement data-driven agriculture for small-scale farmers. Glob. Food Sec. 23, 256–266. doi: 10.1016/j.gfs.2019.08.004
Klerkx, L., Jakku, E., and Labarthe, P. (2019). A review of social science on digital agriculture, smart farming and agriculture 4.0: New contributions and a future research agenda. NJAS - Wageningen J. Life Sci. 90, 100315. doi: 10.1016/j.njas.2019.100315
Kruize, J. W., Wolfert, J., Scholten, H., Verdouw, C. N., Kassahun, A., and Beulens, A. J. M. (2016). A reference architecture for Farm Software Ecosystems. Comput. Electron. Agric. 125, 12–28. doi: 10.1016/j.compag.2016.04.011
L'Engle, K., Sefa, E., Adimazoya, E. A., Yartey, E., Lenzi, R., Tarpo, C., et al. (2018). Survey research with a random digit dial national mobile phone sample in Ghana: methods and sample quality. PLoS ONE 13, e0190902. doi: 10.1371/journal.pone.0190902
May, J. D. (2012). Digital and other poverties: exploring the connection in four east african countries. Inf. Technol. Int. Dev. 8, 33–50. Available at: http://itidjournal.org/index.php/itid/article/view/896
Mehrabi, Z., McDowell, M. J., Ricciardi, V., Levers, C., Martinez, J. D., Mehrabi, N., et al. (2020). The global divide in data-driven farming. Nat. Sustain. 14, 154–160. doi: 10.1038/s41893-020-00631-0
Meijer, S. S., Catacutan, D., Ajayi, O. C., Sileshi, G. W., and Nieuwenhuis, M. (2015). The role of knowledge, attitudes and perceptions in the uptake of agricultural and agroforestry innovations among smallholder farmers in sub-Saharan Africa. Int. J. Agric. Sustain. 13, 40–54. doi: 10.1080/14735903.2014.912493
Mwongera, C., Shikuku, K. M., Twyman, J., Läderach, P., Ampaire, E., Van Asten, P., et al. (2016). Climate smart agriculture rapid appraisal (CSA-RA): a tool for prioritizing context-specific climate smart agriculture technologies. Agric. Syst. 151, 192–203. doi: 10.1016/j.agsy.2016.05.009
Pariyo, G. W., Greenleaf, A. R., Gibson, D. G., Ali, J., Selig, H., Labrique, A. B., et al. (2019). Does mobile phone survey method matter? Reliability of computer-assisted telephone interviews and interactive voice response non-communicable diseases risk factor surveys in low and middle income countries. PLoS ONE 14, 1–25. doi: 10.1371/journal.pone.0214450
Passioura, J. B. (2020). Translational research in agriculture. Can we do it better? Crop Pasture Sci. 71, 517–528. doi: 10.1071/CP20066
Qasim, M., Zia, H., Bin Athar, A., Habib, T., and Raza, A. A. (2021). Personalized weather information for low-literate farmers using multimodal dialog systems. Int. J. Speech Technol. 24, 455–471. doi: 10.1007/s10772-021-09806-2
Shepherd, M., Turner, J. A., Small, B., and Wheeler, D. (2020). Priorities for science to overcome hurdles thwarting the full promise of the ‘digital agriculture’ revolution. J. Sci. Food Agric. 100, 5083–5092. doi: 10.1002/jsfa.9346
Sotelo, S., Guevara, E., Llanos-Herrera, L., Agudelo, D., Esquivel, A., Rodriguez, J., et al. (2020). Pronosticos AClimateColombia: a system for the provision of information for climate risk reduction in Colombia. Comput. Electron. Agric. 174, 105486. doi: 10.1016/j.compag.2020.105486
Steinke, J., Achieng, J. O., Hammond, J., Kebede, S. S., Mengistu, D. K., Mgimiloko, M. G., et al. (2019). Household-specific targeting of agricultural advice via mobile phones: Feasibility of a minimum data approach for smallholder context. Comput. Electron. Agric. 162, 991–1000. doi: 10.1016/j.compag.2019.05.026
Keywords: digital agriculture, ICT, IVR, interactive voice response, farmers feedback, two-way communication, 5Q approach
Citation: Eitzinger A (2021) Data Collection Smart and Simple: Evaluation and Metanalysis of Call Data From Studies Applying the 5Q Approach. Front. Sustain. Food Syst. 5:727058. doi: 10.3389/fsufs.2021.727058
Received: 17 June 2021; Accepted: 05 October 2021;
Published: 16 November 2021.
Edited by:
James Hammond, International Livestock Research Institute (ILRI), KenyaReviewed by:
Rhys Manners, International Institute of Tropical Agriculture (IITA), NigeriaCopyright © 2021 Eitzinger. This is an open-access article distributed under the terms of the Creative Commons Attribution License (CC BY). The use, distribution or reproduction in other forums is permitted, provided the original author(s) and the copyright owner(s) are credited and that the original publication in this journal is cited, in accordance with accepted academic practice. No use, distribution or reproduction is permitted which does not comply with these terms.
*Correspondence: Anton Eitzinger, YS5laXR6aW5nZXJAQ0dJQVIub3Jn
Disclaimer: All claims expressed in this article are solely those of the authors and do not necessarily represent those of their affiliated organizations, or those of the publisher, the editors and the reviewers. Any product that may be evaluated in this article or claim that may be made by its manufacturer is not guaranteed or endorsed by the publisher.
Research integrity at Frontiers
Learn more about the work of our research integrity team to safeguard the quality of each article we publish.