- 1The Alliance of Bioversity International and the International Center for Tropical Agriculture (CIAT), Rome, Italy
- 2Department of Agricultural Sciences, University of Naples Federico II, Naples, Italy
There is evidence that in many situations the use of a diverse set of two or more crop varieties in the field has benefits for production. The benefits of varietal diversification include lower crop disease incidence, higher productivity, and lower yield variability. Targeted interventions could increase varietal diversity where smallholder farmers lack the knowledge and access to seeds needed to diversify their varieties. Innovations based on crowdsourced citizen science make it possible to involve a large number of households in farmer participatory varietal selection. This study analyses varietal diversification in Bihar, India, focusing on the effects of the largest citizen science-based intervention to date, involving 25,000 farmers and 47,000 plots * seasons. The study examines if an increase in the varietal diversity of major staple crops, namely wheat and rice, under real farming conditions contributed to: (1) crop productivity and (2) the ability of households to recover from agricultural production shocks. We used the Rural Household Multi-Indicator Survey (RHoMIS) as a survey tool for rapid characterization of households and the sustainable rural livelihoods framework to understand the potential multiple interactions that are activated within the system by the intervention. We found that an increase in varietal diversification produced livelihood benefits in terms of crop productivity and the ability of households to recover from the occurrence agricultural shocks. Finally, outcomes highlight the effectiveness of development programmes aimed at strengthening rural livelihoods through participatory approaches and use of local crop varietal diversity.
Introduction
Smallholder farmers are exposed to growing uncertainty and risks (IPCC, 2014; Castells-Quintana et al., 2018). Weather disturbances are increasingly affecting agricultural systems and alternative sources of income are often limited (Lobell et al., 2011; Gitz and Meybeck, 2012). The likelihood for an agricultural system to be adversely affected by climatic stressors depends on both social and biophysical factors (Nelson et al., 2009). Vulnerability is a result of exposure and sensitivity of agricultural systems to climatic variation, as well as the capacity of producers to adapt within their livelihood systems (Turner et al., 2003; Adger, 2006). Short-term and long-term climate variation can jointly contribute to vulnerability. For example, smallholders might erode their assets and resources to cope with the short-term consequences of climatic shocks, and thereby undermine their long-term adaptive capacity (Otto et al., 2017; Call et al., 2019; Hansen et al., 2019).
Smallholders can adopt different strategies in response to climate stressors. These strategies include a more efficient use of the production factors (including natural resources) (Paavola, 2008; Speranza, 2013), changes in production technology through the introduction of novel crop management techniques or the adoption of stress-tolerant varieties or crops (Cho et al., 2014; Moniruzzaman, 2015; Mutabazi et al., 2015; Salazar-Espinoza et al., 2015; Call et al., 2019). Different strategies can help households to manage risk through resource allocation (Ellis, 2000) or (financial or non-financial) insurance (Yachi and Loreau, 1999; Barrett et al., 2001). Unfortunately, smallholders often lack the capital or knowledge to effectively implement some of these strategies (Gallopín, 2006; Burnham et al., 2018). Thus, farmers tend to manage risk largely through seed management, as well as through labor and land allocation (Di Falco et al., 2007).
An important option for responding to climate risk is on-farm diversification. It may be achieved through the diversification of the portfolio of farming-generating activities through increasing the types or varieties of crops in the field (Di Falco et al., 2011), crop rotation (Helmers et al., 2001), intercropping (Raseduzzaman and Jensen, 2017), integration of crops and livestock (Yesuf et al., 2008; Di Falco et al., 2011) or integration of trees into crop and/or livestock systems (i.e., agroforestry) (Verchot et al., 2007; Hansen et al., 2019).
In this paper, we focus on the use of a diverse set of two or more crop varieties on farms. This strategy relies on the genetic diversity among the range of varieties used by the farmer. Varietal diversity can help the farming system to buffer against adverse environmental conditions (Wolfe, 1985; Lannou and Mundt, 1996; Akem et al., 2000; Zhu et al., 2000; Østergård and Jensen, 2005; Kiær et al., 2009). There is evidence that varietal diversification can reduce crop disease through three mechanisms: (a) reducing the spread of pathogens, (b) increasing the distance between sensitive host plants, or (c) increasing the presence of resistant plants that form a barrier to prevent dispersion of pathogens (Chin and Wolfe, 1984; Smithson and Lenne, 1996; Finckh and Wolfe, 1998; Mundt et al., 1999; Zhu et al., 2000; Mundt, 2002). Further studies provided empirical evidence that variety richness is associated with an increase of productivity and a reduction of yield variability (Yachi and Loreau, 1999; Østergård and Jensen, 2005; Di Falco et al., 2007). Varietal diversity reduces yield variability because different varieties respond in different ways to different stresses. Different varieties can be combined into a portfolio that has a more stable average yield than any of the individual varieties (Nalley and Barkley, 2010; Sukcharoen and Leatham, 2016). The risk-buffering effect of variety portfolios is one reason why rural households often maintain more than one variety on their farm (Jarvis et al., 2008; Bellon et al., 2015a).
The above-mentioned studies analyzed the benefits generated by a varietal diversification strategy mainly through two types of studies. Observational studies look at empirical relationships in existing farming systems (e.g., Di Falco et al., 2007). Experimental studies look at biological mechanisms and experimentally control for a large number of factors (e.g., Nalley and Barkley, 2010; Sukcharoen and Leatham, 2016). Even though there is evidence for a causal relationship between varietal diversity and positive livelihood outcomes, neither type of study provides evidence that interventions that introduce new varieties succeed in activating this causal mechanism. Several things could stand in the way. Smallholder farmers may lack the knowledge needed to properly manage and deploy the varietal diversity available to them (Mulumba et al., 2012; Nankya et al., 2017). Farmers themselves can generate new knowledge to enable varietal diversification, but this requires them to be able to identify those varieties that are suitable for risk reduction and yield increase under diverse field conditions (Creissen et al., 2016; van Etten et al., 2019). To test if varietal diversification leads to positive livelihood outcomes under real conditions, a third type of study would be needed, focusing on the effectiveness of concrete interventions in shaping the nexus between the smallholder's adoption of the varietal diversification strategy and the livelihood benefits at household level.
Until recently, such interventions were rarely conducted at scale. Under real farming conditions, for a farmer, the variety selection process can be time-consuming and costly (Joshi et al., 1997). Also, farmer demand for a diverse set of varieties needs to lead to a more regular supply of these varieties by modern plant breeding and the commercial seed sector, which often struggles to create and distribute varieties suited for marginal niches (Ceccarelli, 1989; van Etten et al., 2017). Even though participatory varietal selection is now a legitimate exercise in crop research, the demand expressed by farmers is not always translated into breeding and seed production decisions (Sumberg et al., 2013). This requires that expressed demand for varietal diversity has a certain critical mass and is expressed in terms of the key decisions to be taken. Recent innovations make participatory varietal evaluation more scalable, more diversity-oriented (more varieties in the trials) and more informative regarding environmental adaptation. van Etten et al. (2019) have shown that crowdsourced citizen science can support farmer evaluation of varieties to include a much larger number of farmers and varieties in participatory trials than was previously possible. They also showed that varietal evaluation based on citizen science can generate results that show quantitatively the causal effect of seasonal climate on crop variety performance.
The present study examines the effect of smallholder adoption of varietal diversification as a livelihood strategy and the livelihood benefits at household level that ensue, evaluating an intervention using the citizen science approach to varietal evaluation introduced by van Etten et al. (2016, 2019). This intervention took place in Bihar, India from 2010 to 2017 and focused on rice and wheat.
We assume that this development programme represents an exogenous change of the institutional context that provides a quasi-experimental framework that allows to better identify the outcomes of varietal diversification as an intervention strategy. We focus on two specific potential benefits of varietal diversity: (1) crop productivity, and (2) the ability of households to recover from agricultural shocks. We compare the responses between households who obtained seeds and knowledge on how to diversify the variety portfolio and households who were not directly exposed to the development intervention and have managed their farming practices as usual.
The current analysis is structured as follows: section Conceptual Framework presents the theoretical approach adopted; section Study Context introduces the S4N initiative and the context in which it was carried out; section Methodological Approach describes the data collection process, the outcome variables of interest and the methodological approach to the analysis; while sections Results and Discussion report and discuss the main results and their implications. The study ends with some concluding remarks.
Conceptual Framework
The current study aims to investigate if the implementation of a varietal diversification programme is associated with: (1) a change in the farming strategies implemented by the rural households and (2) derived livelihood benefits at household level. In order to assess this objective, the theoretical foundation of this study relies on the Sustainable Rural Livelihoods (SRL) framework (Figure 1) (Scoones, 1998; Bebbington, 1999; Carney, 1999; Ellis, 1999; Niehof, 2004; Martin and Lorenzen, 2016). The advantage of the SRL approach is that it provides a framework for a holistic interpretation of the dynamics of development (Helmore and Singh, 2001; Butler and Mazur, 2007). Indeed, it proposes a comprehensive insight that emphasizes the livelihood system of rural households and analyses the ways in which they adapt their farming strategies to manage external changes to preserve their livelihoods (Scoones, 1998).
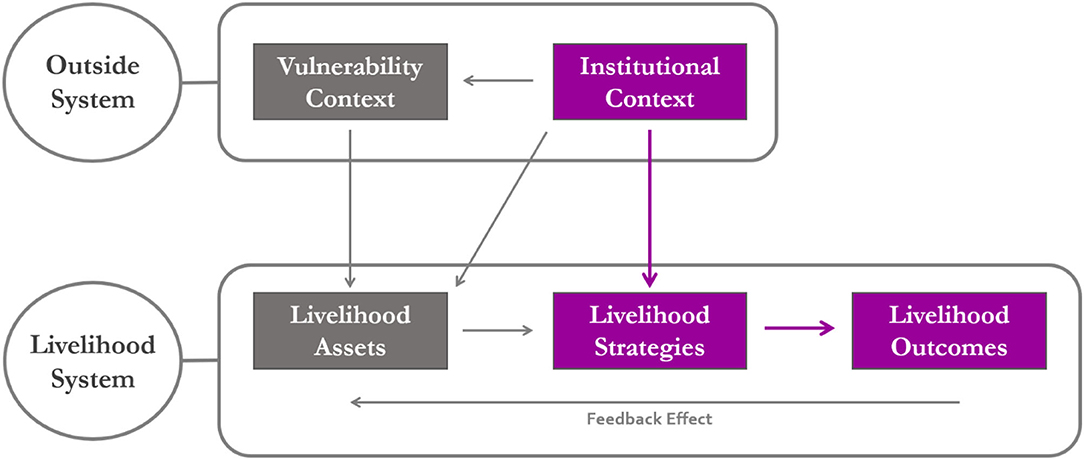
Figure 1. The sustainable rural livelihood framework. Source: adapted from Scoones (1998) and Carney et al. (1999).
In the SRL framework, changes in the institutional context can affect livelihood outcomes in two ways. One is an indirect route through changes in livelihood assets and the capacity of these assets to cope with the vulnerability context, which can enhance or diminish the overall livelihood strategy and thereby affect livelihood outcomes. A second route is that institutional change can affect livelihood strategies directly and thereby affect livelihood outcomes.
Our study focuses on this second route: how can a change in the institutional context, the participation of farming households in a more information-rich environment about the performance of assets (crop varieties), affect their livelihood strategy and holding a broader portfolio of livelihood assets (varietal diversification)? Within the SRL framework, this paper mainly focuses on the role of the institutional context, aiming to identify its effective impact on shaping the relation between the smallholder's adoption of a specific livelihood strategy and the resulting livelihood outcomes. Modest but well-targeted changes in the institutional context can make adaptive strategies more efficient and even sustainable in the long term, thanks to the potential multiple interactions that are activated within the system (Helmore and Singh, 2001; Butler and Mazur, 2007). Such changes can consist in targeted scientific advice, improved technologies, financial facilities, or changes in government policies.
More in detail, following the SRL framework, the livelihood system of the rural households is based on three main elements: livelihood assets, livelihood strategies and sustainable livelihood outcomes. The asset base upon which households build their livelihoods comprehends a portfolio of five different types of assets: natural, financial, physical, human and social capitals (Scoones, 1998). A household will combine the different categories of assets available to it in a strategy designed to accomplish desirable livelihood outcomes (FAO, 2019). However, a household will modify its farming practices to cope with the various challenges coming from the outside system. The outside system is composed of the vulnerability context and the institutional context, which are both the entry points for development initiatives. The vulnerability context refers to the unpredictable events that are beyond the control of the household and can undermine their livelihoods. The institutional context refers to a set of formal and informal institutions and organizations that mediate the ability to implement specific strategies and achieve tangible results. This aspect is of particular interest in the SRL framework. Indeed, policies, institutions and processes influence how households use their assets to pursue different livelihood strategies. Household assets interact with structures (government and private sector) and processes (policies, laws and institutions) responsible for social, economic and political transformation that can shape the vulnerability context, the access to the assets and the choice of livelihood strategies (Adato and Meinzen-Dick, 2002). This may take place on multiple levels, from the household to community, national and even global levels. The institutional focus of the SRL approach gives a practical gain when considering policy applications, by identifying the structures that play an important role in resource allocation, and by identifying social rules and norms that would have an impact on the outcome of an external intervention (Brock, 1999). This makes it possible to observe how policies and programmes are able to influence the households' portfolio of assets and the vulnerability context of reference and how this, in turn, leads to the adoption of specific strategies capable of managing the negative impacts on income and food security caused by extreme climatic events, uncertain agricultural production and unexpected market shocks.
The analysis perspective offered by the SRL framework makes it an adequate theoretical framework for the current analysis, as it highlights the potential multiple interactions that are activated within the system following a change in the institutional context. The next paragraph will provide a more detailed picture of the study context.
Study Context
This study focuses on the Seeds for Needs (S4N) initiative. S4N started in the 2010 and has been implemented in 14 countries in Africa, Asia and Central America with the aim of promoting and using the diversity of plant genetic resources as a means to reduce farmers' vulnerability to climate change (van Etten et al., 2016; Bioversity International, 2018). More specifically, the main component of the S4N initiative addressed the scarce availability of stress-tolerant cultivars, as cropping systems' adaptation requires the continuous delivery of varieties able to address “genotype by environment” interaction (van Etten et al., 2019). After seed varieties that are potentially adapted to the local agroecological and climatic conditions were identified, they were distributed to farmers for participatory selection by means of on-farm experiments in collaboration with scientific and extension staff (Dawson et al., 2008). The range of collaborative research activities engaging farmers together with scientist is defined as “citizen science,” an emerging trend that enables research and development (R&D) to be faster, larger in scale and more focussed on addressing community needs and contextual factors (Resnik et al., 2015), in this case, in terms of agricultural research (Ryan et al., 2018). A second, complementary component addressed the need to raise farmers' awareness by conducting capacity-building activities on sustainable production techniques and the importance of a diversified agricultural production. Trainings were conducted in the form of Farmer Field Schools (FFS), a bottom-up and participatory approach used by scientists and national extension officers to engage with smallholder farmers (Braun et al., 2000). These trainings were based on a “learning by doing” concept and were meant to build farmers' capacity for informed decision-making through hands-on experimentation and frequent interaction for knowledge and experience sharing (Chandra et al., 2017). For the above-mentioned characteristics, Nelson (2020) recognized the S4N initiative as effective implementation of the participatory approach.
In India, the S4N initiative has involved over 25,000 farmers from 600 villages of 49 districts in 7 states, participating as “citizen scientists” in around 46,000 participatory varietal trials (Bioversity International, 2017; van Etten et al., 2019). In this study, we analyse the resulting outcomes of the activities carried out in India, in the Vaishali district of Bihar1 that started in 2010. For the current analysis, the State of Bihar was chosen as a case study for two reasons: firstly, it is the State where S4N implementation first started, offering the possibility to study the potential benefit of a change of the institutional context affecting the livelihood strategies over a longer time span. Secondly, Bihar is one of the most climate-sensitive states in India. Rainfall fluctuates greatly from one season to another; it is also densely populated, with high levels of poverty and 90% of its rural dwellers are directly employed in agriculture (Tesfaye et al., 2017; Pagnani et al., 2021) with land holding sizes of <2.5 acres.2
The implementation of this initiative in Bihar provides a source of exogenous change to the institutional context, which allows the social scientist a better perspective for an empirical identification of the link between the different domains of the SRL framework. Indeed, thanks to the institutional activities, it is possible to compare the livelihood strategies and their outcomes for households under the effect of an institutional change with a counterfactual provided by similar communities and households that were not explicitly covered by the S4N development initiative.
The hypothesis is that the S4N approach can improve the livelihood strategies of smallholder farmers and influence their livelihood assets. Particularly, the intervention provided knowledge, skills and practices to enhance the human capital of those who actively participated in the process. At the same time, the distribution of new, potentially-suitable seed varieties contributed to the improvement of their natural and physical capital. Finally, the participatory approaches of S4N encouraged the connection between farmers within and across communities, expanding the social capital of the rural households.
The changes affecting the human, natural and social capital of smallholder farmers will in turn lead to the adoption of the crop varieties promoted by the initiative, thus increasing the genetic diversity in their fields. Finally, farmers who adopt varietal diversification strategies can obtain further livelihood benefits in terms of: (1) crop productivity, and (2) the ability to recover from the occurrence of agricultural shocks.
Methodological Approach
Data
The data used for this analysis are generated from a household questionnaire administered between February and August 2018 (Gotor et al., 2018). Data are available for 600 stratified, randomly selected rural households of three districts of Bihar: Saran, Samastipur, and Vaishali. The three districts have been identified as particularly vulnerable through regional workshops and therefore suitable for the implementation of climate-smart agriculture under the CGIAR Research Program on Climate Change, Agriculture and Food Security (CCAFS).
The S4N initiative was executed with financial support from the Indian government and strong partnership with national institutions. Expansion of the field activities during the project responded dynamically to local demand and capacity. This precluded the ex-ante definition of project outcomes and thus the execution of a sounding baseline data collection or randomized selection of households or communities. The fact that the S4N initiative did not have a priori control group restrict the options to create a proper counterfactual (Gotor et al., 2017). Furthermore, participation in the initiative was open to all community members and was voluntary. To address these issues, a stratified random sample was drawn based first on the selection of the villages where the initiative was carried out and then on participation in the initiative. Finally, the households within the villages were randomly selected from household lists obtained by local authorities. In total, 12 villages from three districts of Bihar (Saran, Samastipur, and Vaishali3) were identified and 600 rural households were selected, of which 300 participants and 300 non-participants.4 More in detail, the treatment or exposed group consisted of 300 households drawn randomly from project records within the identified villages, while the control or non-exposed group consisted of 150 randomly selected non-participant households within the 12 villages as the exposed group and of 150 households from 9 other villages that were similar and proximate, but where the initiative had never been implemented5 (Table 1). The random assignment of the subjects to the non-exposed group increases the validity of the assessment; however, since the group of participating households has not been randomly assigned to the exposed group, specific statistical adjustments need to be implemented in the empirical analysis, as described in the Empirical Analysis section.
The data collection team was composed of three enumerators. One of them was appointed team leader and was in charge of cross-checking all data at the end of each day. Enumerators attended a series of four full-day training and field-testing sessions. Questionnaires were translated into Hindi, the local language, for better comprehension of enumerators and farmers. Electronic tablets were used to record the data using the Open Data Kit (ODK) platform (Hartung et al., 2010). At the end of each day, all household data was examined by the team leader and then uploaded to a server. The household questionnaire was composed of 17 sections, of which three were specifically devoted to measuring the possible effect of the S4N intervention and focusing on the: (1) participation of farmers in the S4N activities, (2) households' exposure to shocks and their recovery capacity, and (3) detailed information on the wheat and rice cultivation (S4N target crops). For item (3), specific information was gathered on the number of wheat and rice varieties that were sown in the previous 5 years, the seed source, the characteristics of most-preferred seeds, the quantity produced in the last and second to last growing season, quantities consumed and sold, as well as the average market price. Moreover, the questionnaire explored the frequency of climate-induced harvest losses of rice and wheat cultivation, and a self-reported scale was used to assess the perceived extent of recovery following their occurrence. The remaining sections are adapted from the Rural Household Multi-Indicator Survey (RHoMIS), a household survey tool designed to rapidly characterize a series of standardized indicators across the spectrum of agricultural production and market integration, nutrition, food security, poverty and greenhouse gas emissions, as well as standard socioeconomic information on household demographics, education, landholdings, sources of income, migration and gender-disaggregated decision-making power allocation (Hammond et al., 2017). The survey was designed to reduce the time burden for interviews, to refine the accuracy of responses, and to maximize consistency between different studies. The RHoMIS questions were tailored following enumerators' feedback during the training.6.
Indicators
The Simpson's Diversity Index (SDI) (Simpson, 1949) is used to test the hypothesis that on-farm exposure to new varieties of wheat and rice led to a higher varietal diversity. This index is among the most suitable indexes for measuring crop diversification patterns and is calculated as:
where Pj = Aj/j is the share of the j-th varieties area over the total cultivated area for the specific crop. Value ranges start at zero (0) (only one variety cultivated), and approach 1 when many varieties are cultivated in equal shares.
Following Gotor et al. (2013), the effect on crop productivity was measured in terms of perceived change of yield (PYC) over the last 5 years. This is a self-reported measure, which ranged from −4 (100% decrease of yield) to 4 (increase of 100% or more). The variable assumes a positive (negative) value equal to 3, 2 or 1 when the household perceived an overall yield increase (decrease) of respectively ~75, 50, and 25%.
Moreover, the model controls for both financial and weather-related shocks. A specific set of questions was formulated to capture the ability of households to recover from them. To obtain a measure of ability to recover, households self-assessed their capacity to recover from: (a) a decrease in the sale price, (b) a shock affecting their assets, (c) an increase of pest and disease occurrence, and (d) from direct climatic stressors. Based on the answers to these recovery capacity questions, a cumulative variable on the household recovery capacity (RC) was constructed. We summed the frequency of positive (+1) and negative (−1) answers indicating their ability to recover from shocks. If the household declared that it was not exposed to the specific shock, it was counted as a 0 response. Thus, RC values can range between −4 and +4.
Finally, the specific variables selected to define the different livelihood assets are based on the theoretical and empirical literature. Human capital is associated with the age and level of education of the household head, as well as the number of household members. Social capital is associated with the gender of the household head and a self-assessment of trust in people and levels of trust and cooperation within the community. As concerns the former, female-headed households generally face greater social barriers that may limit their access to information and other resources (Tenge et al., 2004; García de Jalón et al., 2018). Natural and physical capital is associated with the extension of cultivated land and the total amount of agricultural inputs (i.e., fertilizer, manure, compost, pesticides, irrigation facilities, and tillage methods). Lastly, financial capital is represented by four different dummy variables based on: pursuit of off-farm income generating activities, ownership of debts, access to formal sources of credit (from the government, NGOs or other organizations) and access to informal sources of credit (from family, friends or neighbors). The description and descriptive statistics of the variables used in the empirical analysis are shown in Table 2.
Empirical Analysis
Two empirical analyses were carried out: the first analysis consists in the identification of the casual effect of the institutional context change on a set of key livelihood outcomes. The research hypothesis underpinning the overall study is that the household's exposure to the S4N intervention activities may provoke changes to the smallholder farmers' seed portfolio, increasing the genetic diversity in their fields, thus generating livelihood benefits for the households in terms of crop productivity and the ability to recover from agricultural shocks.
However, the institutional change does not occur randomly, since, even if there are households from communities that are not involved in the intervention, the sample obviously includes a group of households that have autonomously decided to participate in the initiative activities. Thus, the group of participating households has not been randomly assigned to the exposure, and therefore large differences in terms of compounding factors may exist between the two groups, yielding to biased estimates of the initiative's effects. For this reason, this empirical analysis relies on a specific estimator used in quasi-experimental study, the doubly robust (DR) (Bang and Robins, 2005), to quantify if any substantial differences between households participating in the initiative, compared to those that have not been involved, can be effectively attributed to the institutional change.
DR estimator combines two different approaches to estimate the causal effect of an exposure on the outcome: a specification for the outcome regression and a specification for the exposure. This ensures the robustness of the results because possible forms of misspecification of the model due to selection bias and confounding effects are both considered (Emsley et al., 2008; Caracciolo and Furno, 2017).
where Yi,1 is the observed outcome when the i-th household was exposed to the initiative and Yi,0 is the outcome if the household was not exposed, xi is a vector of the livelihood assets (capturing human, physical, natural, financial and social capital of the i-th household) and p(Xi) the conditional probability of being exposed or propensity score (Wi = 1) vs. unexposed (Wi = 0):
The second empirical analysis consists in the assessment of the specific consequentiality of the steps as theorized in the SRL framework, linking the livelihood benefits (i.e., positive change in productivity and capacity to recover) to the households' adoption of varietal diversification strategies and the institutional context. To assess the above-mentioned relationships, it is necessary to link how the exposure to the S4N activities may influence the on-farm varietal diversification, and if the latter can be reasonably linked to the yield change and the household recovery capacity to shocks. In order to test all the above-mentioned relationships, a simultaneous system of equation has to be formulated ad hoc and estimated via a Generalized Method of Moments (GMM).
The stochastic version of the system is formulated for the i-th household and for the j-th crop in the following way:
This system of equations explicitly analyses the dynamic linkages among initiative participation (Participation), adoption of the wheat and rice varieties supported by the initiative (Adoption) and initiative's outputs, such as varietal diversification measures (Simpson's Diversity Index - SDI). Moreover, it analyses the link between the initiative's outputs (varietal diversification) and two livelihood outcomes, the perceived change of yield (PYC) and the overall recovery capacity of the households from shocks (RC).
The system of equations includes as confounding variables the livelihood assets xi (variables capturing human, physical, natural, financial and social capital of the i-th household) while θ, α, and ω are the parameter vectors of the equations' system that measure the effects of the livelihood assets on the dependent variables; while vji, uji, and ei are the error components. Finally, the estimation of the parameters τ, β, and δ allows us to test the consequential links between the outputs and outcomes of the initiative. Indeed, through the estimation of the parameter τ, the model measures whether adoption of the varieties disseminated through the initiative affects varietal diversity of wheat and rice (Equations 1, 2). The β parameter tests, for each crop, the existence of a linear relation between the varietal diversity and the perceived change of yield (Equations 3, 4), while δ measures the association between the perceived changes of the two crops' yield and the i-th household's capacity to recover from shocks (RC) (Equation 5). Since two target crops exist, a total of five simultaneous equations will be estimated (two for the SDI, two describing the perceived change of yield and one for the overall recovery capacity).
The above-mentioned approach controls for reverse causality and other possible sources of endogeneity (Heckman and Vytlacil, 2005), conditionally on the variables chosen as instruments. Instruments have been selected according to the plausibility of the assumptions, as well as the outcomes of the diagnostic tests. Household participation to the initiative (yes or no) and the number of adopted wheat and rice varieties supported by the initiative have been used as instruments, assuming that they may influence the perceived change of yield only through the use of varietal diversification. Similarly, the varietal diversification is assumed to influence the households' recovery capacity only through an effect on the perceived change of yield. Finally, following Bellon et al. (2015b), households were weighted by the inverse probability (IPW) of initiative participation, which controls for potential sources of selection bias. The IPW weighting considers the observable differences of the livelihood assets between households that have the opportunity to be exposed to the initiative and the households that were excluded. Diagnostic tests were carried out to confirm the validity of the instruments (Durbin–Wu–Hausman test for endogeneity and the Weak Instrument test) (Cameron and Trivedi, 2005).
Results
Sample Description
The mean value and the standard deviation of the variables employed in this study are shown in Table 2. The variables related to the five capitals (i.e., human, social, natural, physical and financial) are shown in top half of Table 2. The principal differences between the two groups (exposed and non-exposed) are most notable in terms of human, social, natural and financial capitals. Households exposed to the initiative have on average a greater number of members and are headed by older people, besides having a higher level of confidence in people and among community members. Moreover, exposed households have a smaller extension of cultivated land (1.29 acres compared to 1.71 acres for the non-exposed), but exhibit a higher level of indebtedness (an average value of 0.60 compared to 0.52 for the non-exposed). The average size of the land holdings in Bihar is <2.5 acres (91% farmers), with that of marginal and small farmers ranging from 0.80 to 1.25 acres, respectively (Government of Bihar, 2020). They are often resource-poor farmers with lower ability to afford mechanization and services, due to which they exhibit a higher level of indebtedness. Compared to other north-western states of India, Bihar is characterized by poverty and high population density. Therefore, the farmers there are more prone to agricultural risks, which in turn leads to indebtedness. Conversely, we saw no significant differences in terms of physical capital between those exposed to the initiative and those who were not exposed.
When considering the variables related to the vulnerability context, the households participating in the initiative on average registered a higher exposure to financial shocks but a lower exposure to pest and disease, and climatic stressors. However, the difference among the two groups is statistically significant only in terms of exposure to financial shocks.
As expected, the number of varieties adopted by the households is higher for those exposed to the initiative, even if the differences in terms of varietal diversification between the two groups are not particularly evident (the differences between exposed and non-exposed are significant only for the level of varietal diversity of wheat). With regard to the perceived change of yield, the mean value of the exposed households is higher than the value of the non-exposed one (as can be seen from Figure 2). Lastly, data reported in Table 2 show that there are no noticeable differences between exposed and non-exposed households in terms of the ability to recover from agricultural shocks.
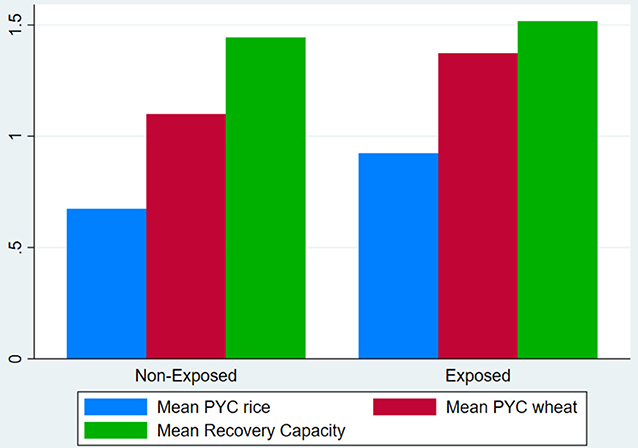
Figure 2. Perceived change of yield (PYC) for wheat and rice, and recovery capacity index (RC) by exposure to the S4N initiative.
S4N Initiative's Impact
As discussed in the previous paragraph, both exposed and non-exposed groups of households showed some differences in terms of livelihood assets. The DR estimator addresses this difference to allow for a proper comparison between the two groups. Results of the exposure equation are detailed in the Appendix (Table A1). Results of the DR estimator are shown in Table 3, identifying the effect of the institutional context change on livelihood outcomes. It is evident that exposure to initiative activities generated positive and significative changes on the variety portfolio of smallholder farmers, specifically on the varietal diversification of target crops. The Simpson's Diversity Index for rice was around 0.6 for the non-exposed and 5% higher (+0.03) for exposed households. The varietal diversity of wheat increased even more. In this case, Simpson's Diversity Index for wheat for non-exposed households was similar of those for rice (0.56), while the effect of participation in the initiative increased this to 11% (+0.06) (Table 3).
The DR results also confirm the research hypothesis underpinning the overall study, namely that exposed households can obtain livelihood benefits in terms of crop productivity and ability to recover from shocks. As can be seen from the equations we applied, the effect on the perceived change of yield is positive and significant. In this case, the impact generated by the S4N initiative was still higher for wheat: exposed households benefitted from an increase of the mean PYC value of 0.185 points for rice and 0.245 points for wheat, which corresponds to a yield increase of +3.94% for rice and +4.80% for wheat. Lastly, at the bottom of Table 3, the effect on households' recovery capacity is reported, showing an increase in their ability to recover from shocks of around 0.40 points in the RC scale ranging from −4 to +4 compared to non-exposed households that have an increase corresponding to around 7% of the actual mean value. The above-mentioned results could be considered a conservative estimate of the S4N initiative since they ignored the existence in the control group of any spillover effect.
Econometric Results
The last part of our analysis is based on the estimation of five simultaneous equations (Table 4). This analysis aims to test the Theory of Change based on the SRL framework. Equations (1) and (2) analyse the relationship between the change in the institutional context (measured in terms of participation in the activities proposed by the initiative and the intensity of adoption of the varieties promoted by the initiative) and the level of varietal diversity maintained on-farm by the households (proxied by the Simpson's Diversity Index).
The results of Equations (1) and (2) show a positive and significant relation between the adoption of the introduced varieties and the level of diversification, both for rice and wheat. This is also evident from Figure 3, which shows that the Simpson's Diversity Index increases as the number of introduced varieties that are adopted increases. However, the relation seems to change course when the number of varieties adopted is greater than six.
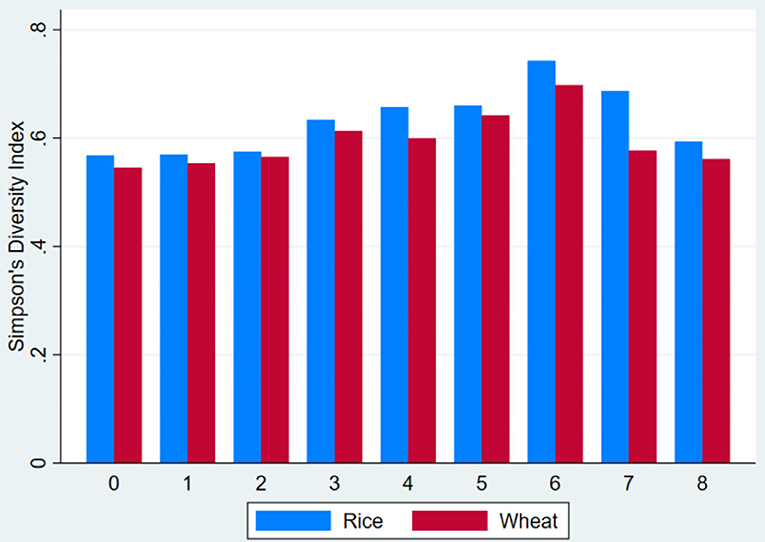
Figure 3. Relations between the number of initiative-introduced rice and wheat varieties adopted by the household and varietal diversification of wheat and rice (Simpson's Diversity Index) (average per household).
The positive and significant relation between diversification and the perceived change in rice and wheat yields is evident from Equations (3) and (4). For rice, the perceived yield increase is negatively associated with female heads of households. The perceived change in rice yield was positively influenced by the level of education of the household head, acres of land cultivated and the access to informal sources of credit. Perceived change in wheat yield is negatively associated with the presence of animals on the farm, measured in Tropical Livestock Units (TLUs).
Equation (5) analyses the influence of the perceived change in yield on the overall recovery capacity of the households. This relation is significant only for wheat, but not for rice. This result is probably due to the fact that the initiative's impact was lower for the latter crop, as previously indicated. The recovery capacity is even influenced by the social capital; explicitly it is positively related to female-headed households and negatively related to high levels of trust in people. Finally, it is possible to observe that the recovery capacity is positively linked to financial and weather-related shocks. These results highlight that a perceived increase in resilience occurs only if households have been exposed to shocks.
The system of equations demonstrates the consequentiality and causality of the relations between the outputs and outcomes of the initiative. Regression results provide evidence that: (a) the adoption of the varieties disseminated through S4N positively affects varietal diversity of rice and wheat (Equations 1, 2); (b) a more diversified production has in turn positively influenced the perceived changes of the yield of the two crops (Equations 3, 4); and lastly, the improved wheat yield trends have enhanced overall recovery capacity of the households from agricultural shocks (Equation 5). Figure 4 helps us to understand in more detail the relation between the observed level of wheat diversification and the estimated perceived wheat yield trend (left panel) and the relation between the latter and the estimated overall recovery capacity of households (right panel). A Simpson's Diversity Index of 0.8 is associated with a perceived increase in wheat yield of over 50% (left panel), that in turn is linked to positive levels of the household's recovery capacity (right panel).
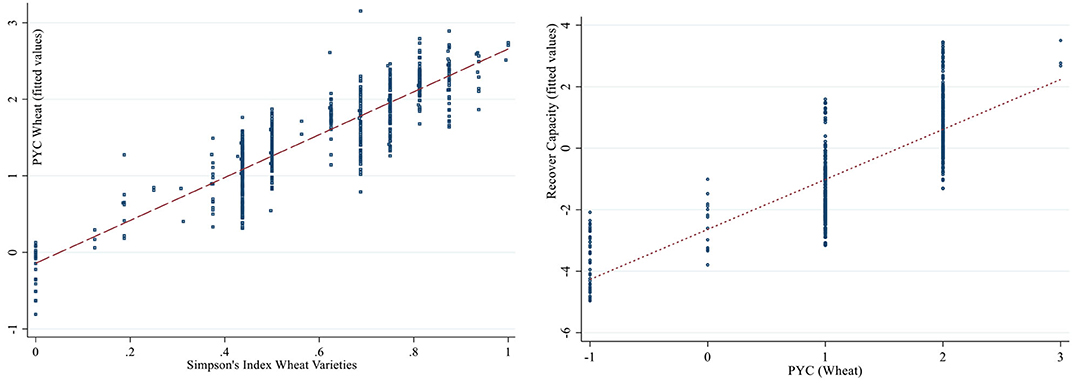
Figure 4. Relation between observed SDI of wheat and the estimated PYC (left) and the relation between the observed PYC (wheat) and the estimated RC (right).
Finally, we analyse whether the estimated relationships and effects of the change in the institutional context are the same for all the households or whether they may vary according to the initial level of outcomes and output characterizing each household. For instance, it could be desirable for positive effects to be larger for the households that need more assistance than others. Figure 5 reports the estimated differences of percentiles for each of the five outputs and outcomes of the intervention between exposed and unexposed households, as predicted by the system of equations. Again, it is clear that household exposure to the initiative significantly increases the intra-species diversity of wheat and rice on farm. For rice, the exposure to the initiative has an effect on diversification that is proportional to the prior level of rice diversification of households. For wheat, however, the effect is not sensitive to the level of diversification. When observing the impact on the perceived yields, similar patterns can be identified: the effects on wheat productivity are positive and similar across percentiles, while they can vary significantly across percentiles in rice, suggesting an inverse U-shaped relationship between rice productivity and the benefits provided by exposure to the initiative. Finally, the change in the institutional context is beneficial to the most of the households' ability to recover from agricultural shocks, benefitting, in particular, those households that, being at lower percentiles for the recovery index, are more vulnerable.
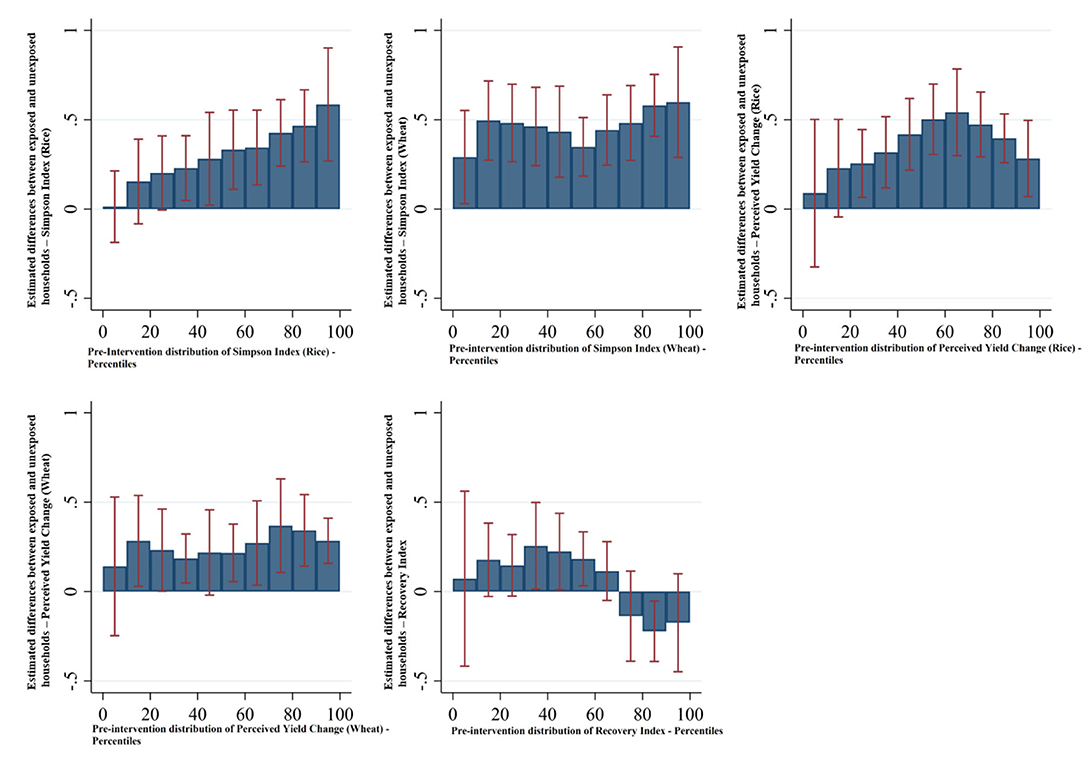
Figure 5. Average differences between exposed and non-exposed groups across percentiles of the distribution of the pre-intervention value of the respective variable. For comparative purposes, outcomes are expressed as standardized values (mean = 0 and standard deviation = 1).
Discussion
It is acknowledged that the use of a diverse set of two or more crop varieties in the field can help the farming system to buffer against adverse environmental conditions. Different studies analyzed the benefits generated by a varietal diversification mainly through experimental trials under controlled conditions or through observational studies of existing systems (Sukcharoen and Leatham, 2016; Nankya et al., 2017). These studies strongly suggested that varietal diversification can be an effective strategy, but do not provide empirical evidence on actual interventions. The current study provides this evidence on livelihood benefits stemming from the implementation of a strategy focused on varietal diversification through the analysis of the effects of the largest intervention so far based on citizen science: the Seeds for Needs initiative.
DR estimator results indicated that exposure to initiative activities generated positive and significative changes on the variety portfolio of smallholder farmers of the target crops, rice and wheat. Moreover, the DR results confirmed the research hypothesis underpinning the overall study, namely that exposed households can obtain substantial livelihood benefits in terms of increased crop productivity and improved ability to recover from shocks. In accordance with the findings of Joshi et al. (1997) and Gotor et al. (2017), outcomes of the current empirical analysis highlight the effectiveness of development programmes aimed at strengthening rural livelihoods through participatory approaches and use of local agrobiodiversity.
The second empirical analysis (system of simultaneous equations) identified strong causal linkages between households' exposure to the S4N activities and increased varietal diversification of farms and livelihood benefits. As shown by van Etten et al. (2019), access to crop varietal diversity through crowdsourced citizen science overcomes the lack of capital and knowledge of Indian farmers and provides a unique opportunity for them to evaluate and identify varieties that better adapt to the local context. This, in turn, stimulates farmers to adopt varietal diversification as a livelihood strategy. They will then use these varieties in their production fields to boost yields and improve households' recovery ability. Results are in line with previous studies that pinpoint varietal richness as an effective strategy capable of guaranteeing a more stable average yield and beneficial effects on crop productivity (Kiær et al., 2009; Nalley and Barkley, 2010; Sukcharoen and Leatham, 2016), as well as making farming systems more resilient and less vulnerable to weather disturbances (Akem et al., 2000; Mulumba et al., 2012).
Interestingly, the results highlight contrasting effects generated by livelihood assets on livelihood strategies and livelihood outcomes. Consistently with previous studies (i.e., Deressa et al., 2009; Bahinipati and Venkatachalam, 2015; Malaiarasan et al., 2021), the current study shows that the presence of animals on the farm (physical capital), the extension of cultivated land (natural capital) and the access to informal sources of credit (financial capital) positively influence the adoption of a strategy focused on varietal diversification of rice and wheat, although the effect of these assets is negative on the yield change (livelihood outcome). Female-headed households are less likely to increase genetic diversity of rice in their fields, in fact they are associated with negative yield changes, even if they show positive levels of recovery capacity. This could be related to the fact that in Bihar (and India in general) women tend to be excluded from agricultural work due to socio-cultural restrictions (Government of Bihar, 2020). However, despite the pronounced gender gap, female-headed households seem able to act on other forces that allow them to increase their household's resilience from unpredictable agricultural shocks.
We also showed how the benefits were distributed according to pre-intervention levels. For wheat, the results were more encouraging than for rice, as wheat diversification and yield increases were insensitive to prior levels, while rice diversification and yield increases benefitted those households with lower prior levels. However, the intervention influenced the ability to recover from shocks that was largest for households that had intermediate prior levels of shock recovery ability. The most vulnerable households, which are at lower percentiles for the recovery index, also benefitted. Unexpectedly, the results of the analysis indicate that exposure to the initiative had a negative effect on the ability to recover from agricultural shocks for households with high prior levels, indicating some degree of increased risk for the less vulnerable households.
Limitations of the Study
This study is not exempt of limitations. The main one is that although the SRL framework assumes that changes in the institutional context can affect livelihood outcomes in two ways, we analyzed only the pathway from institutional change via livelihood strategies to livelihood outcomes. Moreover, only the existence of linear relationships within the SRL framework has been tested, while other livelihood outcomes could be included in the analysis. Furthermore, this study does not provide a detailed understanding of the distribution of the effects generated by the S4N initiative. Indeed, we do not have a plausible explanation for the different distribution of benefits between rice and wheat: surely, it will be important to target interventions in such a way that the most vulnerable households benefit as much as possible. Also, information generated by crowdsourced citizen science is especially rich and could be connected in more direct ways to the econometric analysis. For example, some varieties could have a larger effect on the reduction of vulnerability than others. Further research could improve the methodological approach of the current analysis by adopting a qualitative approach in order to better understand the relationships and interactions between the different domains of the SRL framework or by refining and outspreading the range of livelihood outcomes that could be pursued by the households and drilling down on more detail the effects generated by the intervention. Future interventions could benefit from better understanding the way in which the benefits of the intervention are distributed across households. This could in turn provide information to better target the range of varieties offered to farmers in diversification interventions.
Conclusions
The purpose of this study was two-fold: (1) to analyse the effects of the largest citizen science-based intervention to date, the S4N initiative that took place in Bihar, India from 2010 to 2017 and focused on rice and wheat cultivations; and (2) to provide evidence on the consequentiality and causality of the relationships between the outputs and outcomes of the initiative, following the sustainable rural livelihoods (SRL) framework. For this purpose, we implemented the RHoMIs as a survey instrument on 600 rural households in three districts of Bihar and we used the sustainable rural livelihoods (SRL) framework to understand the potential multiple interactions that are activated within the system by the intervention.
The quantitative analysis of this study provides evidence that exposure to the initiative's activities generated positive and significative changes on the variety portfolio of smallholder farmers. In turn, an increase in varietal diversification produced substantial livelihood benefits in terms of crop productivity, as well as strengthening the ability of households to recover from the unpredictable shocks associated with agricultural production. Furthermore, the analysis highlights the effectiveness of development programmes aimed at strengthening rural livelihoods through participatory approaches and use of local crop varietal diversity.
These findings are not surprising, as the initiative under analysis was explicitly designed to promote the conservation and use of a wider variety of rice and wheat by exposed farmers. However, it is important to understand the magnitude of its effects and its statistical validation. Moreover, these findings can be considered in order to offer useful insights about the effectiveness of different initiatives to policymakers.
We hope that the findings of this analysis can stimulate further research on knowledge transfer and will be used in programmes geared at reinforcing rural livelihoods through participatory approaches and use of local variety richness, while sustaining the conservation of important genetic resources. This is because rural households are the main custodians of intraspecific crop genetic variation, and they need to be recognized as such and supported in their efforts to conserve it for current and future use.
Data Availability Statement
The datasets presented in this study can be found in online repositories. The names of the repository/repositories and accession number(s) can be found below: Gotor et al. (2018).
Ethics Statement
Ethical review and approval was not required for the study on human participants in accordance with the local legislation and institutional requirements. The patients/participants provided their written informed consent to participate in this study. Informed consent was obtained from all subjects involved in the study. A written statement was read to the surveyed for their understanding and consent. As a non-clinical study, no ethical approval was required from national authorities or Bioversity International at the time of the execution of the field survey.
Author Contributions
All authors contributed to the writing and review of the manuscript, read, and approved the submitted version.
Funding
This work was implemented as part of the CGIAR Research Program on Climate Change, Agriculture and Food Security (CCAFS), which is carried out with support from CGIAR Fund Donors and through bilateral funding agreements. For details, please visit https://ccafs.cgiar.org/donors.
Author Disclaimer
The views expressed in this document cannot be taken to reflect the official opinions of these organisations.
Conflict of Interest
The authors declare that the research was conducted in the absence of any commercial or financial relationships that could be construed as a potential conflict of interest.
Publisher's Note
All claims expressed in this article are solely those of the authors and do not necessarily represent those of their affiliated organizations, or those of the publisher, the editors and the reviewers. Any product that may be evaluated in this article, or claim that may be made by its manufacturer, is not guaranteed or endorsed by the publisher.
Acknowledgments
Authors wish to thank the participating households for their availability. Mark Van Wijk and Jim Hammond for their support and useful advices in implementing the use of RHoMIS during the data collection phase. We thank Olga Spellman (The Alliance of Bioversity International and CIAT) for English editing of this manuscript.
Footnotes
1. ^Since 2011, the S4N initiative has been further extended to nine more Indian states: Uttar Pradesh, Odisha, Madhya Pradesh, Chhattisgarh, Orissa, Punjab, Haryana, Jammu and Kashmir.
2. ^Bihar ranks lowest amongst the other Indian states in terms of literacy and lags in socio-economic conditions compared with the national average. Due to high poverty, inequality and a poor education system, resulting from low investment and poor governance, Bihar has poor education and health conditions. The population density in Bihar is double (800 persons/km2) the national average (329 persons/km2) (Rasul and Sharma, 2014).
3. ^Floods in Bihar during the southwest monsoons compromised the selection of some villages in the districts of Saran and Samastipur, making the sample skewed in favour of Vaishali district.
4. ^From project records, a total of 6,500 rural households participated in the S4N initiative, of which 1,500 in Saran, 1,500 in Samastipur and 3,500 in Vaishali.
5. ^This study does not analyse the differences between the two different types of non-exposed groups, while they are considered as a unique group in the empirical approach. Households without cultivated land were excluded from the non-exposed groups.
6. ^The original data and the survey are available on request at the following doi: 10.7910/DVN/DW2W9J.
References
Adato, M., and Meinzen-Dick, R. S. (2002). Assessing the Impact of Agricultural Research on Poverty Using the Sustainable Livelihoods Framework (No. 128). Washington, DC: International Food Policy Research Institute (IFPRI).
Adger, W. N. (2006). Vulnerability. Global Environ. Change 16, 268–281. doi: 10.1016/j.gloenvcha.2006.02.006
Akem, C., Ceccarelli, S., Erskine, W., and Lenne, J. (2000). Using genetic diversity for disease resistance in agricultural production. Outlook Agric. 29, 25–30. doi: 10.5367/000000000101293013
Bahinipati, C. S., and Venkatachalam, L. (2015). What drives farmers to adopt farm-level adaptation practices to climate extremes: empirical evidence from Odisha, India. Int. J. Disaster Risk Reduct. 14, 347–356. doi: 10.1016/j.ijdrr.2015.08.010
Bang, H., and Robins, J. M. (2005). Doubly robust estimation in missing data and causal inference models. Biometrics 61, 962–973. doi: 10.1111/j.1541-0420.2005.00377.x
Barrett, C. B., Reardon, T., and Webb, P. (2001). Nonfarm income diversification and household livelihood strategies in rural Africa: concepts, dynamics, and policy implications. Food Policy 26, 315–331. doi: 10.1016/S0306-9192(01)00014-8
Bebbington, A. (1999). Capitals and capabilities: a framework for analyzing peasant viability, rural livelihoods and poverty. World Dev. 27, 2021–2044. doi: 10.1016/S0305-750X(99)00104-7
Bellon, M., Gotor, E., and Caracciolo, F. (2015a). Conserving landraces and improving livelihoods: how to assess the success of on-farm conservation projects? Int. J. Agric. Sustain. 13, 167–182. doi: 10.1080/14735903.2014.986363
Bellon, M., Gotor, E., and Caracciolo, F. (2015b). Assessing the effectiveness of projects supporting on-farm conservation of native crops: Evidence from the High Andes of South America. World Dev. 70, 162–176. doi: 10.1016/j.worlddev.2015.01.014
Bioversity International (2017). Seeds for Needs – India: A Pathway to Diversification. Available online at: https://www.bioversityinternational.org/news/detail/seeds-for-needs-india-a-pathway-to-diversification/ (accessed November 3, 2019).
Bioversity International (2018). Seeds for Needs. Available online at: https://www.bioversityinternational.org/seeds-for-needs/ (accessed September 4, 2019)
Braun, A. R., Thiele, G., and Fernández, M. (2000). Farmer Field Schools and Local Agricultural Research Committees: Complementary Platforms for Integrated Decision-Making in Sustainable Agriculture. London, UK: Overseas Development Institute.
Brock, K. (1999). Implementing a Sustainable Livelihoods Framework for Policy-Directed Research: Reflections From Practice in Mali, IDS Working Paper 90. Brighton: IDS.
Burnham, M., Rasmussen, L. V., and Ma, Z. (2018). Climate change adaptation pathways: synergies, contradictions and tradeoffs across scales. World Dev. 108, 231–234. doi: 10.1016/j.worlddev.2018.04.014
Butler, L. M., and Mazur, R. E. (2007). Principles and processes for enhancing sustainable rural livelihoods: collaborative learning in Uganda. Int. J. Sustain. Dev. World Ecol. 14, 604–617. doi: 10.1080/13504500709469758
Call, M., Gray, C., and Jagger, P. (2019). Smallholder responses to climate anomalies in rural Uganda. World Dev. 115, 132–144. doi: 10.1016/j.worlddev.2018.11.009
Cameron, A. C., and Trivedi, P. K. (2005). Microeconometrics: Methods and Applications. New York, NY: Cambridge University Press.
Caracciolo, F., and Furno, M. (2017). Quantile treatment effect and double robust estimators: an appraisal on the Italian labor market. J. Econ. Stud. 44, 585–604. doi: 10.1108/JES-02-2016-0026
Carney, D. (1999). Livelihoods Approaches Compared: A Brief Comparison of the Livelihoods Approaches of the UK Department for International Development (DFID), CARE, Oxfam and the United Nations Development Programme (UNDP). London: Department for International Development.
Carney, D., Drinkwater, M., Rusinow, T., Neefjes, K., Wanmali, S., and Singh, N. (1999). Livelihoods Approaches Compared: A Brief Comparison of the Livelihoods Approaches of the UK Department for International Development (DFID), CARE, Oxfam and the United Nations Development Programme (UNDP). London: Department for International Development.
Castells-Quintana, D., del Pilar Lopez-Uribe, M., and McDermott, T. K. (2018). Adaptation to climate change: a review through a development economics lens. World Dev. 104, 183–196. doi: 10.1016/j.worlddev.2017.11.016
Chandra, A., Dargusch, P., McNamara, K. E., Caspe, A. M., and Dalabajan, D. (2017). A study of climate-smart farming practices and climate-resiliency field schools in Mindanao, the Philippines. World Dev. 98, 214–230. doi: 10.1016/j.worlddev.2017.04.028
Chin, K. M., and Wolfe, M. S. (1984). The spread of Erysiphe graminis f. sp. hordei in mixtures of barley varieties. Plant Pathol. 33, 89–100. doi: 10.1111/j.1365-3059.1984.tb00592.x
Cho, S. J., McCarl, B. A., and Wu, X. (2014). “Climate change adaptation and shifts in land use for major crops in the US,” in 2014 Annual Meeting, July 27-29, 2014, Minneapolis, Minnesota (No. 170015). Agricultural and Applied Economics Association.
Creissen, H. E., Jorgensen, T. H., and Brown, J. K. (2016). Increased yield stability of field-grown winter barley (Hordeum vulgare L.) varietal mixtures through ecological processes. Crop Protect. 85, 1–8. doi: 10.1016/j.cropro.2016.03.001
Dawson, J. C., Murphy, K. M., and Jones, S. S. (2008). Decentralized selection and participatory approaches in plant breeding for low-input systems. Euphytica 160, 143–154. doi: 10.1007/s10681-007-9533-0
Deressa, T. T., Hassan, R. M., Ringler, C., Alemu, T., and Yesuf, M. (2009). Determinants of farmers' choice of adaptation methods to climate change in the Nile Basin of Ethiopia. Glob. Environ. Change 19, 248–255. doi: 10.1016/j.gloenvcha.2009.01.002
Di Falco, S., Chavas, J. P., and Smale, M. (2007). Farmer management of production risk on degraded lands: the role of wheat variety diversity in the Tigray region, Ethiopia. Agric. Econ. 36, 147–156. doi: 10.1111/j.1574-0862.2007.00194.x
Di Falco, S., Veronesi, M., and Yesuf, M. (2011). Does adaptation to climate change provide food security? A micro-perspective from Ethiopia. Am. J. Agric. Econ. 93, 829–846. doi: 10.1093/ajae/aar006
Ellis, F. (1999). Rural Livelihood Diversity in Developing Countries: Evidence and Policy Implications. Vol. 40. London: Overseas Development Institute, 1–10.
Ellis, F. (2000). Rural Livelihoods and Diversity in Developing Countries. Oxford: Oxford University Press.
Emsley, R., Lunt, M., Pickles, A., and Dunn, G. (2008). Implementing double-robust estimators of causal effects. Stata J. 8, 334–353. doi: 10.1177/1536867X0800800302
FAO (2019). “The state of the world's biodiversity for food and agriculture,” in FAO Commission on Genetic Resources for Food and Agriculture Assessments, eds J. Bélanger and D. Pilling (Rome: FAO), 572.
Finckh, M. R., and Wolfe, M. S. (1998). “Diversification strategies,” in The Epidemiology of Plant Diseases, ed G. D. Jones (Dordrecht: Springer), 231–259.
Gallopín, G. C. (2006). Linkages between vulnerability, resilience, and adaptive capacity. Glob. Environ. Change 16, 293–303. doi: 10.1016/j.gloenvcha.2006.02.004
García de Jalón, S., Iglesias, A., and Neumann, M. B. (2018). Responses of sub-Saharan smallholders to climate change: strategies and drivers of adaptation. Environ. Sci. Policy 90, 38–45. doi: 10.1016/j.envsci.2018.09.013
Gitz, V., and Meybeck, A. (2012). “Risks, vulnerabilities and resilience in a context of climate change,” in Building Resilience for Adaptation to Climate Change in the Agriculture Sector. Vol. 23, eds A. Meybeck, J. Lankoski, S. Redfern, N. Azzu, V. Gitz [Rome: Food and Agriculture Organization of the United Nations (FAO)], 19.
Gotor, E., Bellon, A., Polar, V., and Caracciolo, F. (2017). Assessing the benefits of andean crop diversity on farmers' livelihood: insights from a development programme in Bolivia and Peru. J. Int. Dev. 29, 877–898. doi: 10.1002/jid.3270
Gotor, E., Caracciolo, F., Cantoa, G. M. B., and Al Nassiri, M. (2013). Improving rural livelihoods through the conservation and use of underutilized species: evidence from a community research project in Yemen. Int. J. Agric. Sustain. 11, 347–362. doi: 10.1080/14735903.2013.796173
Gotor, E., Scafetti, F., Paliwal, A., van Etten, J., van Wijk, M., Hammond, J., et al. (2018). Seeds for Needs India Impact Assessment, Rome: Harvard Dataverse, V2. doi: 10.7910/DVN/DW2W9J
Government of Bihar (2020). Finance Department, Bihar Economic Survey 2019–20. Bihar: Government of Bihar.
Hammond, J., Fraval, S., van Etten, J., Suchini, J. G., Mercado, L., Pagella, T., et al. (2017). The rural household multi-indicator survey (RHoMIS) for rapid characterisation of households to inform climate smart agriculture interventions: description and applications in East Africa and Central America. Agric. Syst. 151, 225–233. doi: 10.1016/j.agsy.2016.05.003
Hansen, J., Hellin, J., Rosenstock, T., Fisher, E., Cairns, J., Stirling, C., et al. (2019). Climate risk management and rural poverty reduction. Agric. Syst. 172, 28–46. doi: 10.1016/j.agsy.2018.01.019
Hartung, C., Lerer, A., Anokwa, Y., Tseng, C., Brunette, W., and Borriello, G. (2010). “Open data kit: tools to build information services for developing regions,” in Proceedings of the 4th ACM/IEEE International Conference on Information and Communication Technologies and Development, 1–12.
Heckman, J. J., and Vytlacil, E. (2005). Structural equations, treatment effects, and econometric policy evaluation 1. Econometrica 73, 669–738. doi: 10.1111/j.1468-0262.2005.00594.x
Helmers, G. A., Yamoah, C. F., and Varvel, G. E. (2001). Separating the impacts of crop diversification and rotations on risk. Agron. J. 93, 1337–1340. doi: 10.2134/agronj2001.1337
Helmore, K., and Singh, N. (2001). Sustainable Livelihoods: Building on the Wealth of the Poor (No. 362.52091734 H481). Sterling, VA: Kumarian Press.
IPCC (2014). “Climate change 2014. Synthesis report. Versión inglés,” in Contribution of Working Groups I, II and III to the Fifth Assessment Report of the Intergovernmental Panel on Climate Change. Geneva. doi: 10.1017/CBO9781107415324
Jarvis, D. I., Brown, A. H., Cuong, P. H., Collado-Panduro, L., Latournerie-Moreno, L., Gyawali, S., et al. (2008). A global perspective of the richness and evenness of traditional crop-variety diversity maintained by farming communities. Proc. Nat. Acad. Sci. 105, 5326–5331. doi: 10.1073/pnas.0800607105
Joshi, K. D., Subedi, M., Rana, R. B., Kadayat, K. B., and Sthapit, B. R. (1997). Enhancing on-farm varietal diversity through participatory varietal selection: a case study for Chaite rice in Nepal. Exp. Agric. 33, 335–344. doi: 10.1017/S0014479797003049
Kiær, L. P., Skovgaard, I. M., and Østergård, H. (2009). Grain yield increase in cereal variety mixtures: a meta-analysis of field trials. Field Crops Res. 114, 361–373. doi: 10.1016/j.fcr.2009.09.006
Lannou, C., and Mundt, C. C. (1996). Evolution of a pathogen population in host mixtures: simple race–complex race competition. Plant Pathol. 45, 440–453. doi: 10.1046/j.1365-3059.1996.d01-138.x
Lobell, D. B., Schlenker, W., and Costa-Roberts, J. (2011). Climate trends and global crop production since 1980. Science 333, 616–620. doi: 10.1126/science.1204531
Malaiarasan, U., Paramasivam, R., and Felix, K. T. (2021). Crop diversification: determinants and effects under paddy-dominated cropping system. Paddy Water Environ. 19, 417–432. doi: 10.1007/s10333-021-00843-w
Martin, S. M., and Lorenzen, K. A. I. (2016). Livelihood diversification in rural Laos. World Dev. 83, 231–243. doi: 10.1016/j.worlddev.2016.01.018
Moniruzzaman, S. (2015). Crop choice as climate change adaptation: evidence from Bangladesh. Ecol. Econ. 118, 90–98. doi: 10.1016/j.ecolecon.2015.07.012
Mulumba, J. W., Nankya, R., Adokorach, J., Kiwuka, C., Fadda, C., De Santis, P., et al. (2012). A risk-minimizing argument for traditional crop varietal diversity use to reduce pest and disease damage in agricultural ecosystems of Uganda. Agric. Ecosyst. Environ. 157, 70–86. doi: 10.1016/j.agee.2012.02.012
Mundt, C. C. (2002). Use of multiline cultivars and cultivar mixtures for disease management. Annu. Rev. Phytopathol. 40, 381–410. doi: 10.1146/annurev.phyto.40.011402.113723
Mundt, C. C., Cowger, C., and Hoffer, M. E. (1999). “Disease management using varietal mixtures,” in Septoria and Stagonospora Diseases of Cereals: A Compilation of Global Research: Proceedings of the Fifth International Septoria Workshop, eds M. van Ginkel, A. McNab, and J. Krupinsky (Mexico: CIMMYT), 111–116.
Mutabazi, K. D., Amjath-Babu, T. S., and Sieber, S. (2015). Influence of livelihood resources on adaptive strategies to enhance climatic resilience of farm households in Morogoro, Tanzania: an indicator-based analysis. Region. Environ. Change 15, 1259–1268. doi: 10.1007/s10113-015-0800-7
Nalley, L. L., and Barkley, A. P. (2010). Using portfolio theory to enhance wheat yield stability in low-income nations: an application in the Yaqui valley of northwestern Mexico. J. Agric. Resour. Econ. 35, 334–347. doi: 10.22004/ag.econ.93223
Nankya, R., Mulumba, J., Caracciolo, F., Raimondo, M., Schiavello, F., Gotor, E., et al. (2017). Yield perceptions, determinants and adoption impact of on farm varietal mixtures for common bean and banana in Uganda. Sustainability 9:1321. doi: 10.3390/su9081321
Nelson, G. C., Rosegrant, M. W., Koo, J., Robertson, R., Sulser, T., Zhu, T., et al. (2009). Climate Change: Impact on Agriculture and Costs of Adaptation. Vol. 21. Washington, DC: International Food Policy Research Institute.
Nelson, R. (2020). International agriculture's needed shift from energy intensification to agroecological intensification. Food Policy 91:101815. doi: 10.1016/j.foodpol.2019.101815
Niehof, A. (2004). The significance of diversification for rural livelihood systems. Food Policy 29, 321–338. doi: 10.1016/j.foodpol.2004.07.009
Østergård, H., and Jensen, J. W. (2005). Increased Yield and Yield Stability in Variety Mixtures of Spring Barley. Denmark: DARCOFenews.
Otto, I. M., Reckien, D., Reyer, C. P., Marcus, R., Le Masson, V., Jones, L., et al. (2017). Social vulnerability to climate change: a review of concepts and evidence. Region. Environ. Change 17, 1651–1662. doi: 10.1007/s10113-017-1105-9
Paavola, J. (2008). Livelihoods, vulnerability and adaptation to climate change in Morogoro, Tanzania. Environ. Sci. Policy 11, 642–654. doi: 10.1016/j.envsci.2008.06.002
Pagnani, T., Gotor, E., and Caracciolo, F. (2021). Adaptive strategies enhance smallholders' livelihood resilience in Bihar, India. Food Secur. 13, 419–437. doi: 10.1007/s12571-020-01110-2
Raseduzzaman, M. D., and Jensen, E. S. (2017). Does intercropping enhance yield stability in arable crop production? A meta-analysis. Eur. J. Agron. 91, 25–33. doi: 10.1016/j.eja.2017.09.009
Rasul, G., and Sharma, E. (2014). Understanding the poor economic performance of Bihar and Uttar Pradesh, India: a macro-perspective. Region. Stud. Region. Sci. 1, 221–239. doi: 10.1080/21681376.2014.943804
Resnik, D. B., Elliott, K. C., and Miller, A. K. (2015). A framework for addressing ethical issues in citizen science. Environ. Sci. Policy 54, 475–481. doi: 10.1016/j.envsci.2015.05.008
Ryan, S. F., Adamson, N. L., Aktipis, A., Andersen, L. K., Austin, R., Barnes, L., et al. (2018). The role of citizen science in addressing grand challenges in food and agriculture research. Proc. R. Soc. B 285:20181977. doi: 10.1098/rspb.2018.1977
Salazar-Espinoza, C., Jones, S., and Tarp, F. (2015). Weather shocks and cropland decisions in rural Mozambique. Food Policy 53, 9–21. doi: 10.1016/j.foodpol.2015.03.003
Scoones, I. (1998). “Sustainable rural livelihoods: a framework for analysis,” in Working Paper-Institute of Development Studies, University of Sussex (United Kingdom).
Smithson, J. B., and Lenne, J. M. (1996). Varietal mixtures: a viable strategy for sustainable productivity in subsistence agriculture. Ann. Appl. Biol. 128, 127–158. doi: 10.1111/j.1744-7348.1996.tb07096.x
Speranza, C. I. (2013). Buffer capacity: capturing a dimension of resilience to climate change in African smallholder agriculture. Region. Environ. Change 13, 521–535. doi: 10.1007/s10113-012-0391-5
Sukcharoen, K., and Leatham, D. (2016). Mean-variance versus mean–expected shortfall models: an application to wheat variety selection. J. Agric. Appl. Econ. 48, 148–172. doi: 10.1017/aae.2016.8
Sumberg, J., Thompson, J., and Woodhouse, P. (2013). Why agronomy in the developing world has become contentious. Agric. Hum. Values 30, 71–83. doi: 10.1007/s10460-012-9376-8
Tenge, A. J., De Graaff, J., and Hella, J. P. (2004). Social and economic factors affecting the adoption of soil and water conservation in West Usambara highlands, Tanzania. Land Degrad. Dev. 15, 99–114. doi: 10.1002/ldr.606
Tesfaye, K., Aggarwal, P., Mequanint, F., Shirsath, P., Stirling, C., Khatri-Chhetri, A., et al. (2017). Climate variability and change in Bihar, India: challenges and opportunities for sustainable crop production. Sustainability 9:1998. doi: 10.3390/su9111998
Turner, B. L., Kasperson, R. E., Matson, P. A., McCarthy, J. J., Corell, R. W., Christensen, L., et al. (2003). A framework for vulnerability analysis in sustainability science. Proc. Nat. Acad. Sci. 100, 8074–8079. doi: 10.1073/pnas.1231335100
van Etten, J., Beza, E., Calderer, L., Van Duijvendijk, K., Fadda, C., Fantahun, B., et al. (2016). First experiences with a novel farmer citizen science approach: Crowdsourcing participatory variety selection through on-farm triadic comparisons of technologies (tricot). Exp. Agric. 55, 1–22. doi: 10.1017/S0014479716000739
van Etten, J., de Sousa, K., Aguilar, A., Barrios, M., Coto, A., Dell'Acqua, M., et al. (2019). Crop variety management for climate adaptation supported by citizen science. Proc. Nat. Acad. Sci. 116, 4194–4199. doi: 10.1073/pnas.1813720116
van Etten, J., López Noriega, I., Fadda, C., and Thomas, E. (2017). “The contribution of seed systems to crop and tree diversity in sustainable food systems,” in Mainstreaming Agrobiodiversity in Sustainable Food Systems: Scientific Foundations for an Agrobiodiversity Index, eds W. de Boef, M. Haga, L. Sibanda, M.S. Swaminathan, and P. Winters (Rome: Bioversity International), 81–101.
Verchot, L. V., Van Noordwijk, M., Kandji, S., Tomich, T., Ong, C., Albrecht, A., et al. (2007). Climate change: linking adaptation and mitigation through agroforestry. Mitig. Adapt. Strateg. Glob. Change 12, 901–918. doi: 10.1007/s11027-007-9105-6
Wolfe, M. (1985). The current status and prospects of multiline cultivars and variety mixtures for disease resistance. Annu. Rev. Phytopathol. 23, 251–273. doi: 10.1146/annurev.py.23.090185.001343
Yachi, S., and Loreau, M. (1999). Biodiversity and ecosystem productivity in a fluctuating environment: the insurance hypothesis. Proc. Nat. Acad. Sci. 96, 1463–1468. doi: 10.1073/pnas.96.4.1463
Yesuf, M., Di Falco, S., Deressa, T., Ringler, C., and Kohlin, G. (2008). The Impact of Climate Change and Adaptation on Food Production in Low-Income Countries: Evidence From the Nile Basin, Ethiopia. Washington, DC: International Food Policy Research Institute.
Zhu, Y., Chen, H., Fan, J., Wang, Y., Li, Y., Chen, J., et al. (2000). Genetic diversity and disease control in rice. Nature 406:718. doi: 10.1038/35021046
Appendix
Keywords: varietal diversification, citizen science, livelihood benefits, sustainable rural livelihoods, India, RHoMIS
Citation: Gotor E, Pagnani T, Paliwal A, Scafetti F, van Etten J and Caracciolo F (2021) Smallholder Farmer Engagement in Citizen Science for Varietal Diversification Enhances Adaptive Capacity and Productivity in Bihar, India. Front. Sustain. Food Syst. 5:726725. doi: 10.3389/fsufs.2021.726725
Received: 17 June 2021; Accepted: 30 September 2021;
Published: 28 October 2021.
Edited by:
Tapan Kumar Nath, University of Nottingham Malaysia Campus, MalaysiaReviewed by:
Keshav Lall Maharjan, Hiroshima University, JapanElena Lioubimtseva, Grand Valley State University, United States
Copyright © 2021 Gotor, Pagnani, Paliwal, Scafetti, van Etten and Caracciolo. This is an open-access article distributed under the terms of the Creative Commons Attribution License (CC BY). The use, distribution or reproduction in other forums is permitted, provided the original author(s) and the copyright owner(s) are credited and that the original publication in this journal is cited, in accordance with accepted academic practice. No use, distribution or reproduction is permitted which does not comply with these terms.
*Correspondence: Elisabetta Gotor, ZS5nb3RvckBjZ2lhci5vcmc=